- 1State Key Laboratory of Cotton Biology, Institute of Cotton Research, Chinese Academy of Agricultural Sciences, Anyang, China
- 2Cotton Research Institute of Jiangxi Province, Jiujiang, China
Estimating the precise nutritional status of crop nitrogen (N) after flowering period is not only important to predict deficiency but the excess that could be revised by fertilization in future crops. Critical N dilution curves describing the critical N concentration ([N]c) in plant tissues during crop growth have been used to estimate the N status of whole plants in cotton. Little is known, however, about the critical N dilution curve for specific plant organs such as cotton fruits. The objective of this study was to verify the feasibility of fruits-based critical N dilution curve as a useful diagnostic tool for diagnosing the N status of cotton crops. A 3-year field experiment was conducted with seven N application rates (0–360 kg N ha–1) using the high-yielding cultivars Jimian 228 and Lumian 28, which differ in maturity. The relationship between fruits dry mass (DM) and N concentration ([N]) was analyzed, and a model of [N]c for cotton fruits was constructed and validated. The results showed that fruits [N]c decreased with increasing fruits DM. The critical N dilution curve based on cotton fruits was described by the equation [N]c = 2.49 × DM–0.12 (R2 = 0.649, P < 0.0001) across cultivar-years. The N nutrition index (NNI) of the fruits (NNIf) with the N dilution curve was significantly related to the NNI of shoot DM, relative yield (RY), and boll density at most sampling dates. For an NNIf of approximately 1, the RY was nearly 95%, while it decreased with a decreasing NNIf below 1. The petiole nitrate-N (NO3-N) concentration was also linearly related to the NNIf, suggesting that the NO3-N concentration in the petiole was a good predictor of the NNIf. Therefore, fruits-based critical N dilution curve and the derived NNIf values will serve as a useful diagnostic tool for diagnosing N status in cotton crops.
Introduction
Cotton is the most important natural textile fiber crop worldwide. Cotton production provides income for approximately 100 million families and its economic impact is estimated to be approximately $500 billion yr–1 (Chen et al., 2007; Cotton Research Institute Chinese Academy of Agricultural Sciences [CRI], 2013). Currently, China is the largest producer and consumer of raw cotton in the world. However, the yield is quite low (802 kg ha–1) in the Yellow River Valley of China compared with the national average (1720 kg ha–1) (United States Department of Agriculture [USDA], 2019; National Bureau of Statistics of China, 2020). One of the main reasons for such a low yield level may be inappropriate crop nutrient management, irrespective of cultivars and climatic variability (Cotton Research Institute Chinese Academy of Agricultural Sciences [CRI], 2013).
Nitrogen (N) is the most common limiting nutrient required by many field crops (Lebauer and Treseder, 2008), including cotton. Currently, China is the largest user of fertilizer N in the world. The production of mineral N fertilizers depends on large amounts of energy from natural gas (oil), a non-renewable resource, and N fertilizer in agricultural production also causes environmental concerns by an unreasonable use (Reis et al., 2016). For example, over-application of N fertilizers in excess of crop requirements is lost to the environment via denitrification, NH3 volatilization, surface runoff and leaching (Vitousek et al., 2009; Yan et al., 2014; Reis et al., 2016). There is thus a critical need to optimize the use of N fertilizer to reduce N inputs and to maintain cotton production in sustainable agricultural systems (Zhang et al., 2017; Luo et al., 2018). One way to minimize N fertilization without negatively affecting yield is to match the N supply with crop demand at the correct rate during the growing season (Diacono et al., 2013). Therefore, it is important to develop crop N status diagnostic tools to improve N fertilizer management and achieve sustainable agricultural development goals in China and worldwide.
The N nutrition status of crops can be diagnosed by plant analysis. One of the most frequently used plant N diagnostic methods is based on the determination of critical plant N concentration ([N]c) (Plénet and Lemaire, 1999; Debaeke et al., 2012; Ata-Ul-Karim et al., 2014; Lemaire and Gastal, 2018; Giletto et al., 2020). The minimum plant N concentration ([N]) required to achieve its maximum growth rate at a given amount of biomass accumulation, time, and field situation is referred to as [N]c (Greenwood et al., 1990). This concept is based on the premise that an allometric relationship exists between nutrient concentration and biomass accumulation through a dilution process (Plénet and Lemaire, 1999). The critical N concentration can thereafter be used to calculate the N nutrition index (NNI) by dividing the actual [N] in the plant biomass by the [N]c (Lemaire et al., 2008a; Ata-Ul-Karim et al., 2013; Zhao et al., 2016b; Lv et al., 2020).
N critical dilution curves based on the whole plant basis have been established for a range of crops (e.g., wheat, rice, maize, oilseed rape, sunflower, and potato; Debaeke et al., 2012; Ata-Ul-Karim et al., 2013; Yao et al., 2014; Weymann et al., 2017; Giletto et al., 2020; Rodríguez et al., 2020; Zhao et al., 2020), defining scenarios of luxury (N excess), sufficiency and deficiency for plant N status, but only three peer-reviewed studies have been conducted for cotton N critical dilution curve (see Chen et al., 2021 for a bibliometric analysis). The determinations of the critical N dilution curves of cotton have been based on whole plants related to specific cultivars and environmental conditions (Xue et al., 2007; Wang et al., 2012; Ma et al., 2019). These critical N dilution curves differ between species with contrasting genotypes, environments and management combinations (Flénet et al., 2006; Lemaire et al., 2008b; Ata-Ul-Karim et al., 2013; Du et al., 2020; Ciampitti et al., 2021), indicating inter- and intra-species dissimilarities. Moreover, N deficiencies can alter biomass allocation among plant organs and thus the shape of the dilution curves may vary depending on which plant organs are included (Weymann et al., 2017; Giletto et al., 2020; Lv et al., 2020). While the concept of critical N dilution curves to specific plant organs is similar to the whole plant basis, critical N dilution curves for specific plant organs have not yet been determined for cotton, which could be useful for assessing cotton N nutrition status.
The use of specific organs for the diagnosis of N must be sensitive to changes in N in the soil and that absorbed by the plant during cultivation, which allows for correction of N content and productivity. Although the leaf is the most frequently used in plant N diagnosis (Yao et al., 2014; Zhao et al., 2017), the theory of critical N dilution has recently been used to develop critical N dilution curves based on plant reproductive organs, such as wheat spikes, maize ears (Zhao et al., 2020) and potato tubers (Giletto et al., 2020; Lv et al., 2020). N dynamic of the reproductive organ may be more relevant with the formation of yield (Zhao et al., 2020). These findings offer an opportunity to diagnose plant N status for crop-specific N fertilization management during crop post-anthesis period (Zhao et al., 2020). Cotton plants are unique because they are perennial with an indeterminate growth habit, and consequently, the vegetable and reproductive phases can last up to 90 days in parallel (Cotton Research Institute Chinese Academy of Agricultural Sciences [CRI], 2013). The flowering and boll-setting stages are the recommended moment for N analysis and diagnosis because of high nutrient demand by cotton plants. During these stages, for example, the uptake of N is approximately 60–62% of the entire growth period (Zhang and Dong, 2020). Moreover, fruits N uptake and production have a close correlation with final yield (Li et al., 2020). Hence, the accuracy of fruits N status after the flowering period is of prime significance for cotton yield improvement.
Nutrient diagnostic tools should allow easy measurement and interpretation. The direct measurement of plant [N] can offer sufficient knowledge of the limiting and non-limiting factors governing N demand, yet it is expensive, time-consuming and labor-intensive, which considerably limits its adaptation in a large range of situations (Lemaire et al., 2008a; Ata-Ul-Karim et al., 2014). Numerous studies have demonstrated the importance of leaf chlorophyll meter readings as an indirect indicator of plant N status in various crops (Wu et al., 1998; Arregui et al., 2006; Ziadi et al., 2008a; Zhao et al., 2016b). However, chlorophyll meter readings may be highly affected by many external factors other than N, such as environment, position, disease and cultivars (Lemaire et al., 2008a). Due to the relatively more robust and accurate response of chlorophyll meters to N deficiency, the nitrate-N (NO3-N) concentration in sap could be an alternative to evaluate N nutrition status (Wu et al., 2007).
Here, a 3-year field experiment with seven N application rates and two cultivars was conducted to evaluate crop N nutrition status using a critical N dilution curve based on cotton fruits for diagnosing the N status of cotton crops. The objectives of this study were: (1) to determine the critical N dilution curve based on cotton fruits for two cultivars; (2) to quantify the critical fruits NNI and its relationship with yield and yield components; and (3) to explore the use of petiole NO3-N concentration determined in the fully expanded functional leaves to predict the fruits NNI in cotton.
Materials and Methods
Study Site
This study was conducted in 2018–2020 at an experimental station (36°13′N, 114°35′W) in Anyang County, Henan Province, China. The site has a typical semi-humid climate with an annual average air temperature of 14.2°C and precipitation of 553.8 mm (1973–2017) (Li et al., 2020). In 2018, 2019, and 2020, annual growing season precipitation was 569.3, 454.1, and 317.5 mm, respectively, and the respective mean growing season temperatures were 22.6, 22.6, and 21.6°C (Figure 1). The soil type is classified as Inceptisols (USDA Soil Taxonomy). The topsoil (0–20 cm) of the experimental field soil is a sandy loam, with a pH (H2O) of 8.03, organic matter of 13.8 g kg–1, total nitrogen (N) of 0.9 g kg–1, Olsen phosphorus (P) of 17.9 mg kg–1 and available potassium (K) of 168.4 mg kg–1 at the beginning of the experiment.
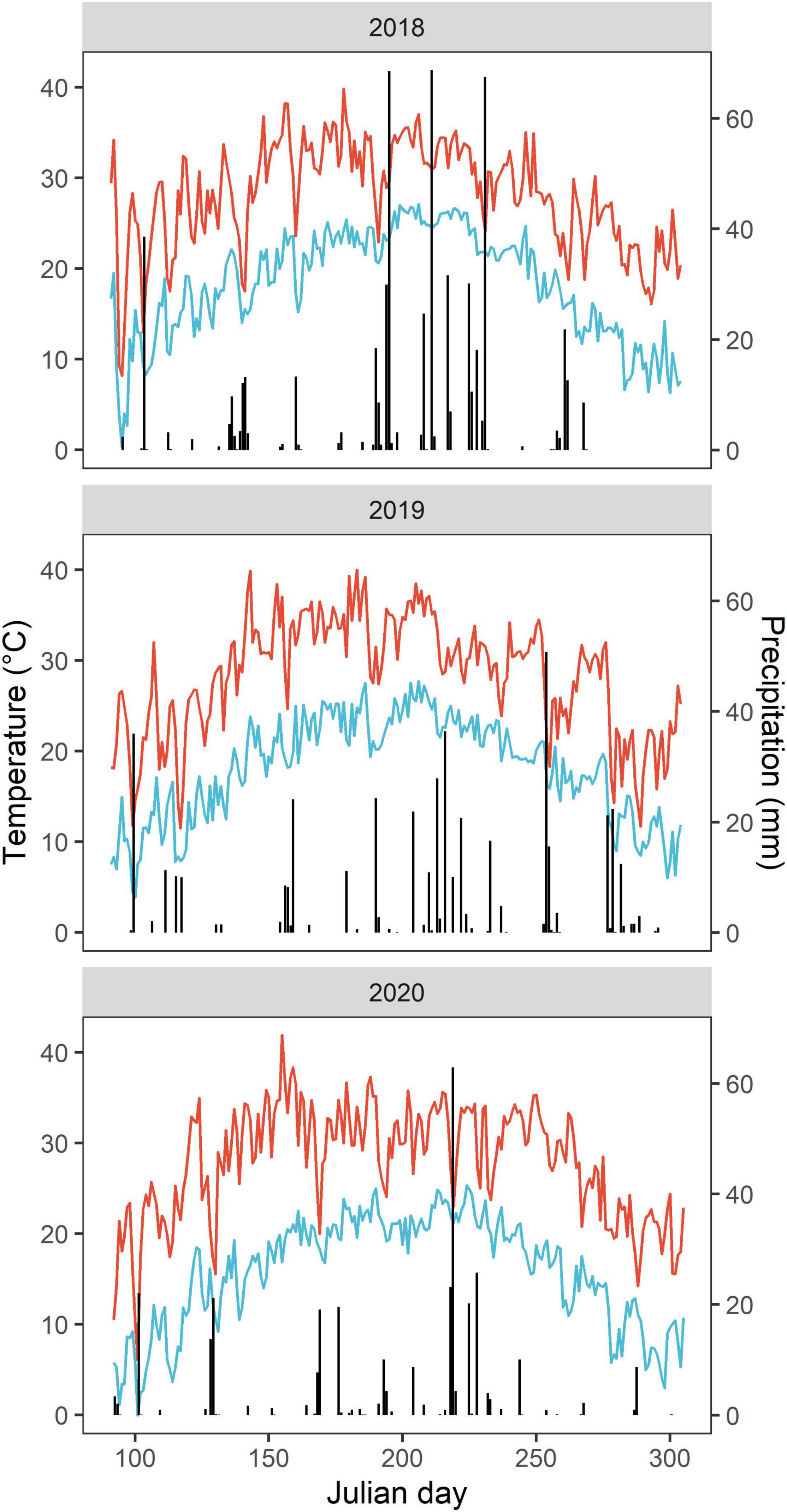
Figure 1. Daily air temperature and precipitation during the cotton growing season from April to October in 2018–2020. The evolution of daily maximum and minimum temperature values are presented as continuous red and blue lines, respectively. The vertical bars depict daily precipitation.
Experimental Design and Crop Management
The experiment was a split-plot design with three replicates. The same layout was maintained in the three consecutive years. The main plot factors were seven N application rates (0, 60, 120, 180, 240, 300, and 360 kg N ha–1). The subplot factors were two cotton cultivars (Jimian 228 and Lumian 28). Jimian 228 and Lumian 28 were used for their middle and mid-early maturity characteristics, with sprout-to-harvest times of approximately 130 and 125 days, respectively, and they are the main high-yielding cultivars grown in areas of the Yellow River Valley. Each plot was 28.8 m2 in area, 3.2 m wide and 9 m long, and included four rows (north-south orientation). Adjacent plots were separated by a 15-cm-high ridge to avoid contamination caused by N dynamics between the treatments. The planting density was 6.0 plants m–2 in rows spaced 80 cm apart.
Planting of cotton seeds took place on 28, 30, and 21 April in 2018, 2019, and 2020, respectively. All plots were overseeded and then manually thinned to the desired density at the squaring stage. Cotton plants absorbed about 58% of fertilizer N at 18–20 days after first bloom (Yang et al., 2013), thus allocating more fertilizer N to the later growth stages is of importance to enhance nutrient use efficiency and cotton yield (Yang et al., 2011; Geng et al., 2015). As such, two fifths of the N fertilizer (urea, 46% N) was evenly broadcasted and incorporated into soil at sowing, while the remaining N was applied with irrigation at the early flowering stage to avoid N losses (e.g., N volatilization). Flood irrigation was adopted according to the local farmers’ practice. In addition, P and K were applied as basal fertilizers at 90 kg P2O5 ha–1 and 120 kg K2O ha–1 in the form of triple superphosphate (44% P2O5) and potassium sulfate (50% K2O), respectively. Other field management practices, such as pest and weed control, were conducted following local practices and remained uniform and normal for all treatments. After harvest, cotton straws for each plot were wholly removed from the field, leaving the soil bare till sowing the next year.
Plant Sampling and Measurements
Due to variation in maturity of the two cultivars, phenological events (plant emergence, squaring, flowering, and boll opening) were not synchronized but had little difference (Supplementary Table 1). In this study, therefore, sampling started only when 50% of observed plants in each plot reached flowering using the middle maturity cultivar Jimian 228 as a reference. Plant samples were collected at 0, 15, 30, 45, and 60 days after flowering (DAF) in 2018; 0, 17, 44, 60, and 81 DAF in 2019; and 35 and 60 DAF in 2020. Note that two samplings in 2020 were made to validate critical fruits dilution curve. For each sampling, three plants were randomly selected from the middle rows in each plot to avoid border effects. The samples were partitioned into leaves (including petioles), stems, squares, flowers and bolls. All samples were placed in an oven at 120°C for 30 min and then further dried at 80°C until a constant weight was reached. The dry mass (DM) of each plant organ was determined. Dried plant samples were finally ground to a fine powder, after which [N] was determined using the micro-Kjeldahl distillation method (Bao, 2005). Shoot N uptake was obtained from the sum of N in each organ, which was estimated from the product of DM and [N]. Six petiole samples from the fully expanded functional leaves (i.e., the 4th leaf from the apex) on the main stem were collected at each plant sampling date per plot in 2019. The petioles were placed into an ice box immediately after removal and transported to the laboratory for the measurement of nitrate-N (NO3-N) concentration using Reflectoquant® (RQ flex@ 10 plus, Merck KGaA, Darmstadt, Germany).
Cotton Yield and Yield Components
We presented cotton yield and yield components in 2018 and 2019. The seedcotton was handpicked from the entire area of each plot on 12 September and 30 October in 2018 and 27 October in 2019. In 2019, only once cotton harvest was made, as the number of opening bolls was lower as a result of bad weather in September (heavy precipitation and low temperature, Figure 1). In both years, we also considered large bolls that opened only after sun drying when determining the final yield. The seedcotton was weighed after sun-drying. Prior to harvest, ten plants were randomly tagged in each plot to record observations on the number of open bolls per plant. For each harvest, all open bolls of the ten plants were collected in each plot, and dried to determine boll weight (seedcotton weight per boll). Lint percentage was calculated as the ratio of lint weight after ginning to seedcotton weight before ginning. The seedcotton yield was multiplied by the lint percentage to estimate the lint yield. In this study, lint yield was used as a proxy for cotton yield, as cotton production is mainly conducted to obtain cotton fibers. Shoot DM was determined as the seedcotton plus stalk yield.
Data Analysis
Critical Nitrogen Dilution Curve for Cotton Fruits
The critical fruits N dilution curve was determined by a negative power function according to the methodology proposed by Justes et al. (1994). Briefly, the data from each sampling occasion were categorized into two groups, namely, (i) N limiting, where an increasing N application rate resulted in a significant response in fruits DM, and (ii) N non-limiting, where an additional N application rate did not lead to a further increase in fruits DM. The [N]c was determined by using the intersection points of the oblique (simple linear regression of N limiting points) and vertical lines (mean values of N non-limiting points) at each sampling time for Jimian 228 and Lumian 28, respectively.
Where [N]c and DM are the critical N concentration (%) and dry mass (t ha–1) of fruits, respectively. Parameter a is [N]c for fruits when fruits DM is exactly 1 t ha–1. Parameter b is a dilution coefficient, which describes the decrease in [N] associated with fruits DM increase. The datasets in 2018 and 2019 were used to determine [N]c and establish fruits-based critical N dilution curve. The critical fruits N dilution curve was validated using two sampling datasets in 2020. The preceding formula was also used to calculate the critical N dilution curve for cotton stems and leaves.
Nitrogen Nutrition Index Based on Cotton Fruits
To characterize N status, fruits N nutrition index (NNIf) was calculated as the ratio of the actual N concentration ([N]) to the critical N concentration ([N]c) of fruits (Lemaire and Gastal, 1997):
Where NNIf values lower than 1 indicate an N deficiency in fruits, values close to 1 indicate that N status is non-limiting for fruits development, and values greater than 1 indicate luxury N accumulation in fruits. The preceding formula was also used to calculate the N nutrition index of the shoot (NNIsh).
Relative Yield
The RY was calculated as follows (Ata-Ul-Karim et al., 2017):
Where Yi is the lint yield of each plot, and Ymax is the maximum lint yield of each cultivar in each year.
Statistical Analysis
Shoot DM, fruits DM, [N] and petiole NO3-N concentrations for each sampling date, along with lint yield, RY and yield components at harvest were subjected to linear mixed effects models with N rate, cultivar and year set as fixed effects and N rate nested within replicate treated as a random effect. In the analysis of petiole NO3-N concentration, year was excluded because data were from a single year. Where significant, differences between the means were compared using Tukey’s honestly significant difference (HSD) test at P < 0.05.
Non-linear regression analyses were used to examine the relationship between stems, leaves and fruits DM and stems, leaves and fruits [N]. The differences in two N dilution curves between cultivars or years were tested using analysis of covariance after data were log-transformed. We also used simple linear regression to investigate the relationships of NNIsh, N rate, RY, boll density, boll weight, and petiole NO3-N concentration with the NNIf. All statistical analyses were performed in R version 3.6.2 (R Core Team, 2019), with the “nlme” package for the linear mixed effects model (Pinheiro et al., 2020) and the “basicTrendline” package for non-linear and linear regression (Mei and Yu, 2018).
Results
Cotton Fruits Dry Mass, Shoot Dry Mass, and the Corresponding Nitrogen Concentration
Overall, N fertilization affected fruits and shoot DM in 80 and 95% of the sampling dates across cultivar-years, respectively. Fruits and shoot DM increased over the growing season, with values ranging from 0.10 to 8.52 t ha–1 for fruits and from 1.36 to 15.70 t ha–1 for shoot across sampling dates and cultivar-years (Supplementary Tables 2, 3).
In general, fruits and shoot [N] were significantly affected by N fertilization in 45 and 55% of sampling dates across cultivar-years, respectively. As expected, N concentrations decreased during the growing season. Across sampling dates and cultivar-years, fruits [N] ranged from 1.55 to 3.71%, while shoot [N] ranged from 1.38 to 2.77% (Supplementary Tables 2, 3).
Lint Yield, Relative Yield, and Yield Components
Lint yield and yield components are shown in Table 1. Lint yield and yield components were considerably affected by the N rate and cultivar, but not by their interactions, indicating consistency of the N effect over cultivars and vice versa. Lint yield, boll density and boll weight changed little between N fertilizer rates except when no N was included, and no significant increase above 60 kg N ha–1 was observed. Jimian 228 showed a higher lint yield and lint percentage than Lumian 28, with a significant difference between the 2 years. The averaged greatest lint yield and lint percentage was 1725 kg ha–1 and 40.2% for Jimian 228, and 1655 kg ha–1 and 38.8% for Lumian 28 across 2 years, respectively. Similar results were also observed for RY (Supplementary Figure 1).
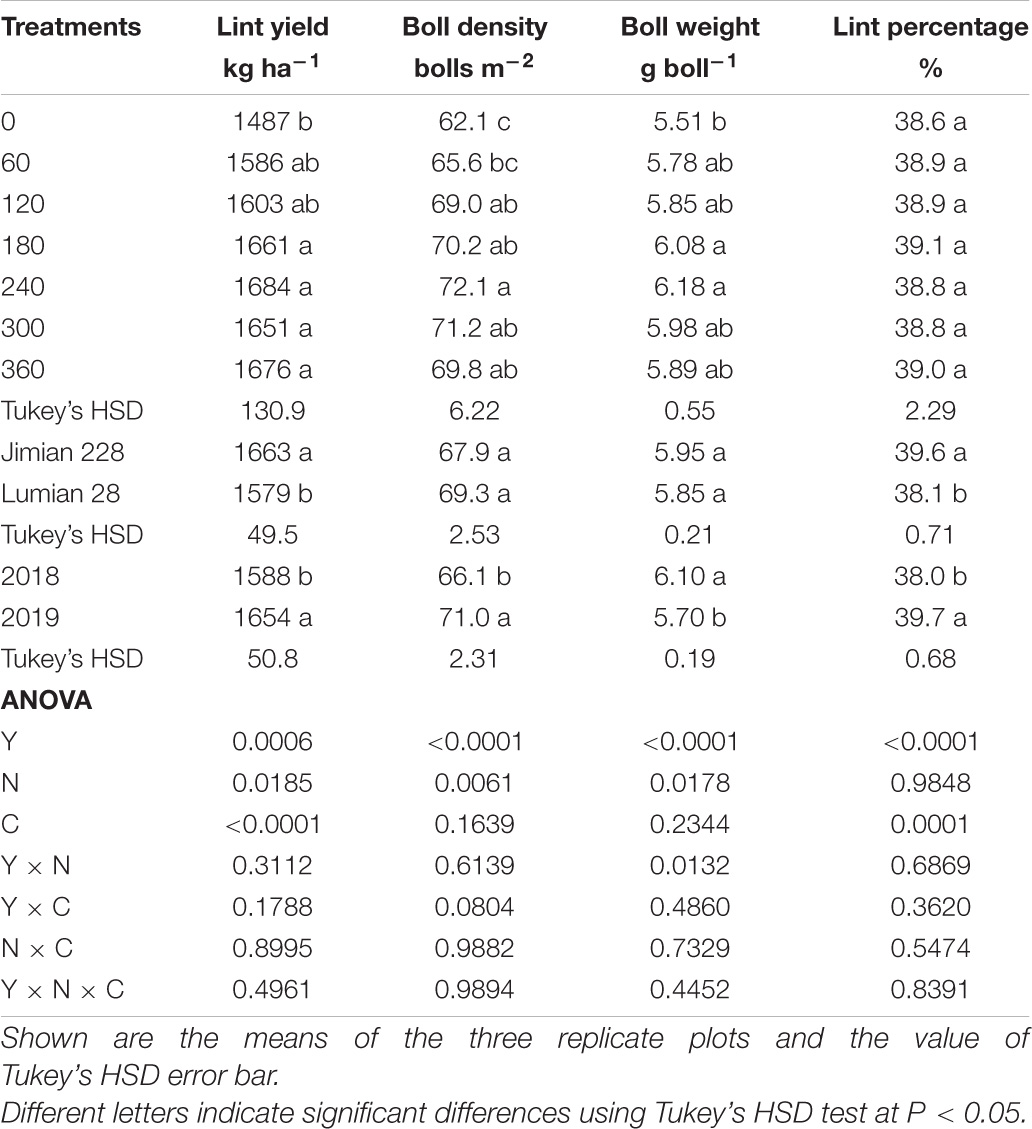
Table 1. Lint yield, boll density, boll weight, and lint percentage in response to year (Y), N application rate (N), and cultivar (C).
The Critical Nitrogen Concentration and Nitrogen Dilution Curves in Fruits, Stems, and Leaves
Fruits [N]c showed a gradually declining trend and the decrease was more rapid for the lower N fertilizer rates corresponding to the lower DM values (Figure 2). Fruits [N]c decreased from a maximum of 3.21% to a minimum of 1.86% while the corresponding fruits DM increased from a minimum of 0.33 t ha–1 to a maximum of 7.80 t ha–1 across cultivar-years. Similar results were also observed for stems and leaves [N]c (Supplementary Figure 2).
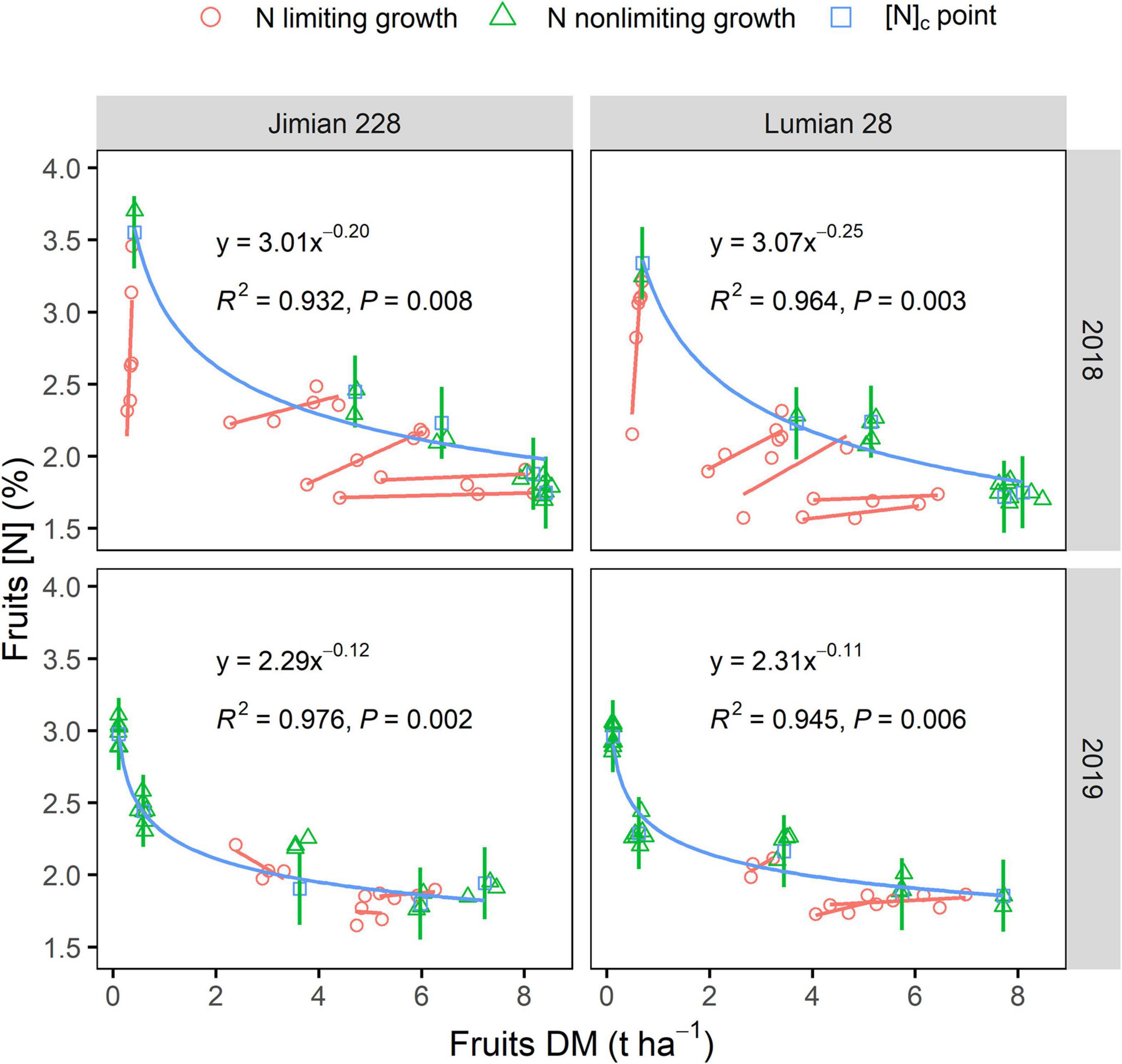
Figure 2. Critical fruits N dilution curves for Jimian 228 and Lumian 28 in 2018 and 2019. DM, dry mass; [N], N concentration and [N]c, critical N concentration. Data show means of the three replicate plots. The red lines represent simple linear regression of N limiting growth points at each sampling date. The green lines represent the mean values of fruits dry mass by N non-limiting growth points at each sampling date. The blue lines represent the fitted critical N curves for cotton fruits using [N]c points. Two curves were not significantly different between cultivars in each year (P = 0.3910 in 2018; P = 0.6605 in 2019) and were significantly different between years for Lumian 28 (P = 0.0052) based on ANCOVA. The calculation for the critical N dilution point is shown in the section “Materials and Methods.”
The decline in fruits [N]c was closely related to fruits DM regardless of the climatic conditions of the year or cultivar, which was described by a negative power function (Figure 2). The N dilution curves between the two cultivars were not significantly different (P > 0.05). Therefore, a unique dilution curve across cultivar-years was fitted as follows: [N]c = 2.49 × DM–0.12 (R2 = 0.649, P < 0.0001). However, the critical N dilution curves for stems and leaves were not well determined by a negative power function in some cultivar-years cases (Supplementary Figure 2). The explanatory powers of the stems and leaves N dilution curve model (R2) were also weaker (Figure 2 and Supplementary Figure 2). Moreover, fruits specific N dilution followed a similar trend as that in the case of the entire plant level, but it was a notably lower than the general curve for C3 crops (Figure 3).
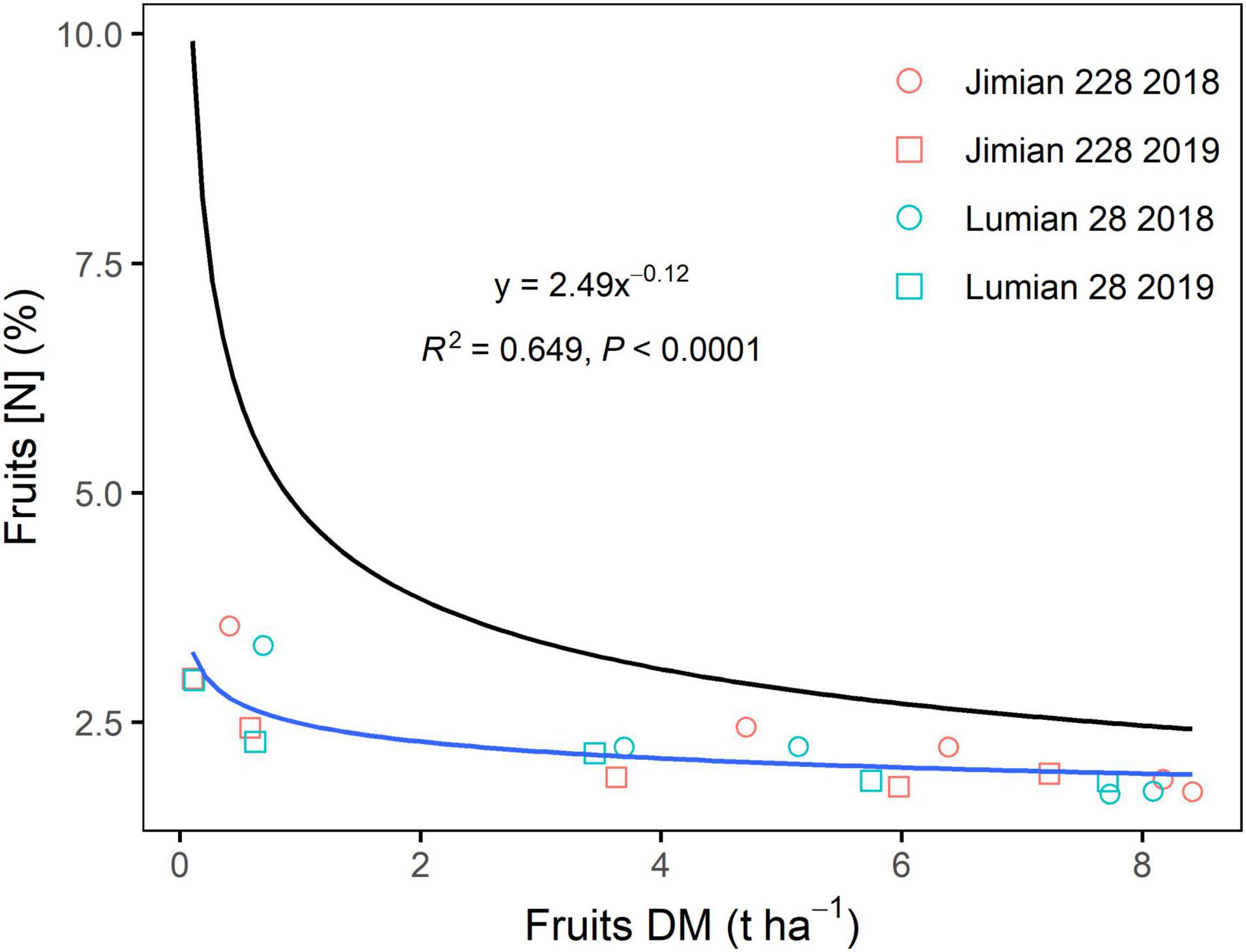
Figure 3. The overall critical fruits N dilution curve across cultivar-years. DM, dry mass and [N], N concentration. Points shown for the mean values of the three replicate plots. The equation and fit statistics (R2 and P-value) are indicated for the relationship. Additionally, the general critical N curve ([N]c = 4.80 × DM–0.34) is presented for C3 crops based on plant biomass (the black line).
The datasets in 2020 were used to test the accuracy of the critical fruits N dilution curve determined with the 2018 and 2019 datasets (Figure 4 and Supplementary Table 4). The fruits N dilution curve could effectively discriminate between N limiting and N non-limiting situations within the range for which it was established. Most data points from N limiting points were located under the curve, while those from N non-limiting points were close to and above the curve.
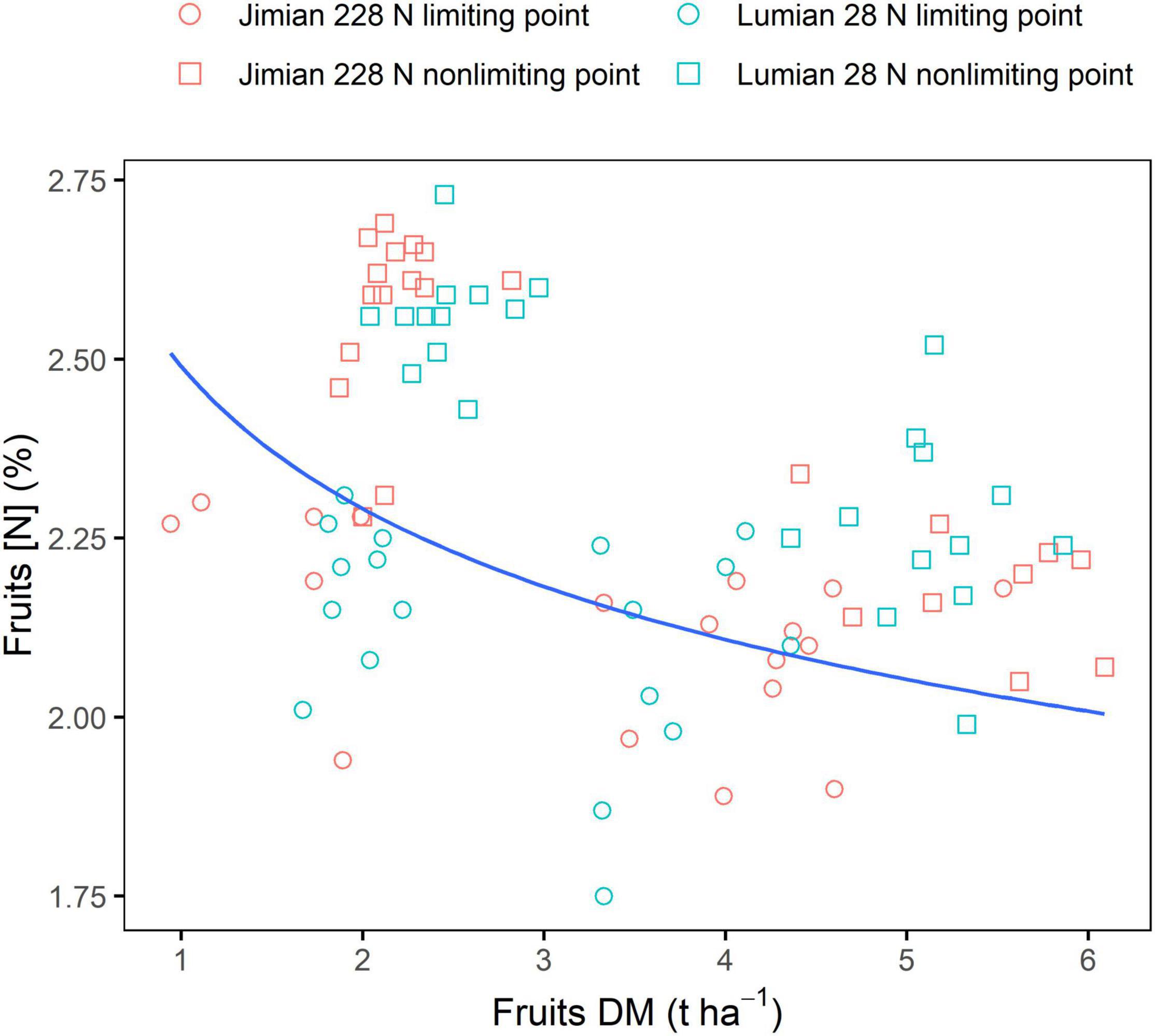
Figure 4. Validation of critical fruits dilution curve using independent datasets from two cotton cultivars in the 2020 cropping season. DM, dry mass; and [N], N concentration. Points shown for the values of the three replicate plots at each sampling date. The data for fruits DM and [N] in 2020 are shown in Supplementary Table 4.
Nitrogen Nutrition Status
From the dilution curve developed for fruits DM, the NNIf was calculated for all sampling dates. The NNIf changed little between sampling dates. Across sampling dates, N rates and years, NNIf values ranged from 0.822 to 1.033 (average 0.994) for Jimian 228, and 0.838–0.998 (average 0.943) for Lumian 28, respectively (Figures 5A,B). In both cultivars, moreover, N rates positively affected the NNIf at most sampling dates (Figures 5A,B), and the highest NNIf value was recorded with the highest N application. The NNIf was not affected (P > 0.05) by cultivar on average (Supplementary Table 5).
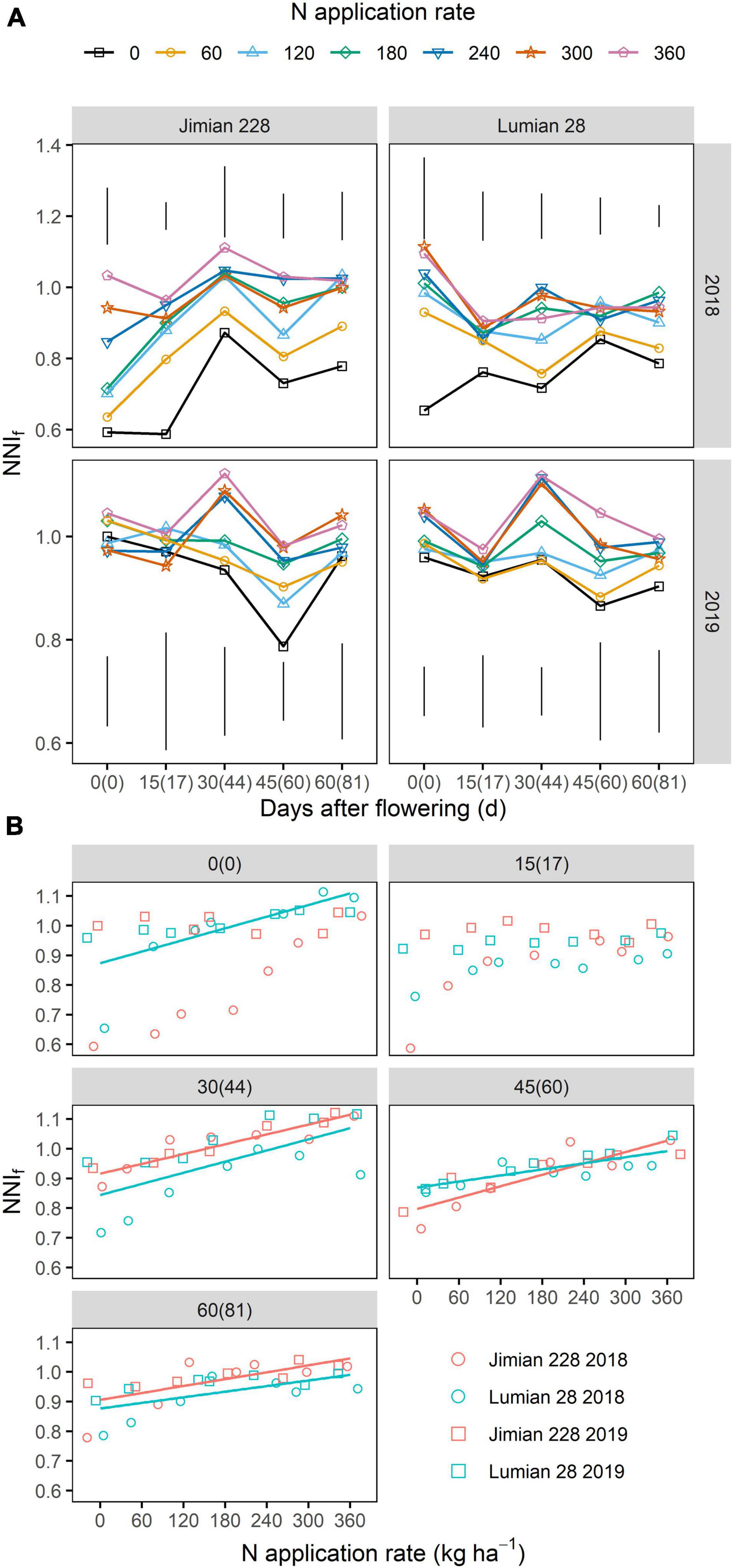
Figure 5. The nitrogen nutrition index of cotton fruits (NNIf) after flowering (A) and its relationship with N application rate (B). The numbers without and with brackets are the days after flowering corresponding to sampling dates in 2018 and 2019, respectively. (A) The vertical bars indicate Tukey’s HSD error bars at each sampling date. For clarity purposes, standard error at each sampling date is not shown in the graphs. ANOVA table is shown in Supplementary Table 5. (B) Points shown for the mean values of the three replicate plots. The red and blue solid lines represent significant linear relationships (P < 0.05) for Jimian 228 and Lumian 28 across 2 years, respectively; non-significant relationships are not shown (P > 0.05).
The NNIf values were highly related to the NNIsh for both cultivars in most cases, with the exception of Jimian 228 at the fourth sampling after the flowering period (Figure 6), indicating the strong synchrony between fruits and shoot N status. When the NNIsh was equal to one, the average NNIf was 0.968 and 0.963 for Jimian 228 and Lumian 28 across sampling dates and years, respectively.
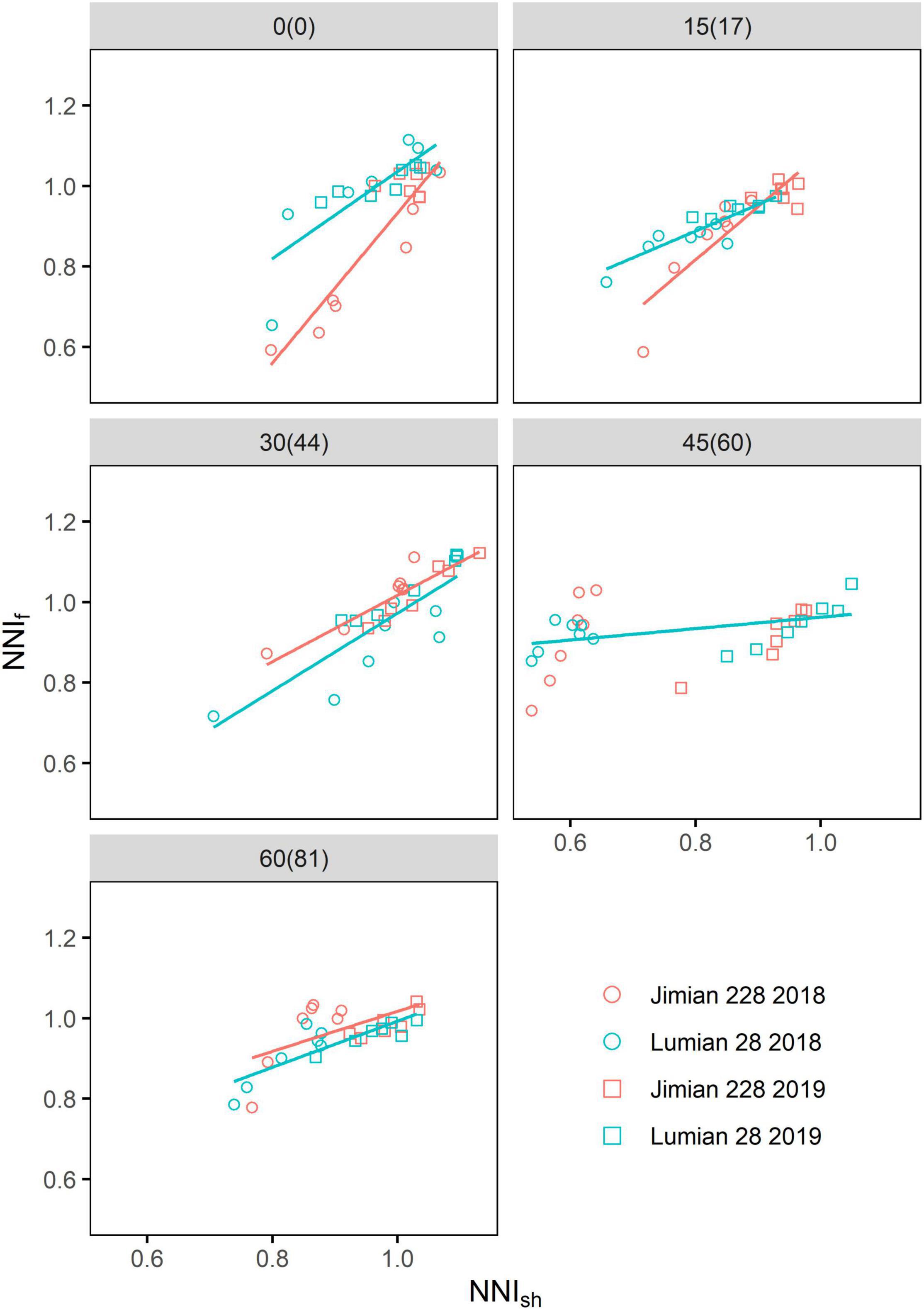
Figure 6. The relationships between the shoot nitrogen nutrition index (NNIsh) and fruits nitrogen nutrition index (NNIf) for the two cotton cultivars at each sampling date. The NNIf (NNIsh) is calculated as the ratio between the actual fruits (shoot) N concentration and the critical fruits (shoot) N concentration. Points shown for the mean values of the three replicate plots. The values without and with brackets are the days after flowering corresponding to different sampling dates in 2018 and 2019, respectively. The red and blue solid lines represent significant linear relationships (P < 0.05) for Jimian 228 and Lumian 28 across the 2 years, respectively; non-significant relationships are not shown (P > 0.05).
Relationships of Nitrogen Nutrition Index of Fruits With Relative Yield and Yield Components
Across the 2-year period, the relationship between RY vs. NNIf was described by a simple linear model under two cultivars at most sampling dates, except for 0 and 17 DAF for Jimian 228 (Figure 7A). However, RY could not reach 1 at an NNIf of approximately 1. When the RY was 0.95, the NNIf reached 1.143 and 1.063 for Jimian 228 and Lumian 28, respectively. Moreover, the responses of yield components to the NNIf showed that boll density was positively correlated with the NNIf in both cultivars (Figure 7B), although this pattern did not repeat in terms of boll weight vs. NNIf (Figure 7C). On average, when the NNIf = 1.0, the boll density reached 69.3 and 73.2 bolls m–2 for Jimian 228 and Lumian 28, respectively.
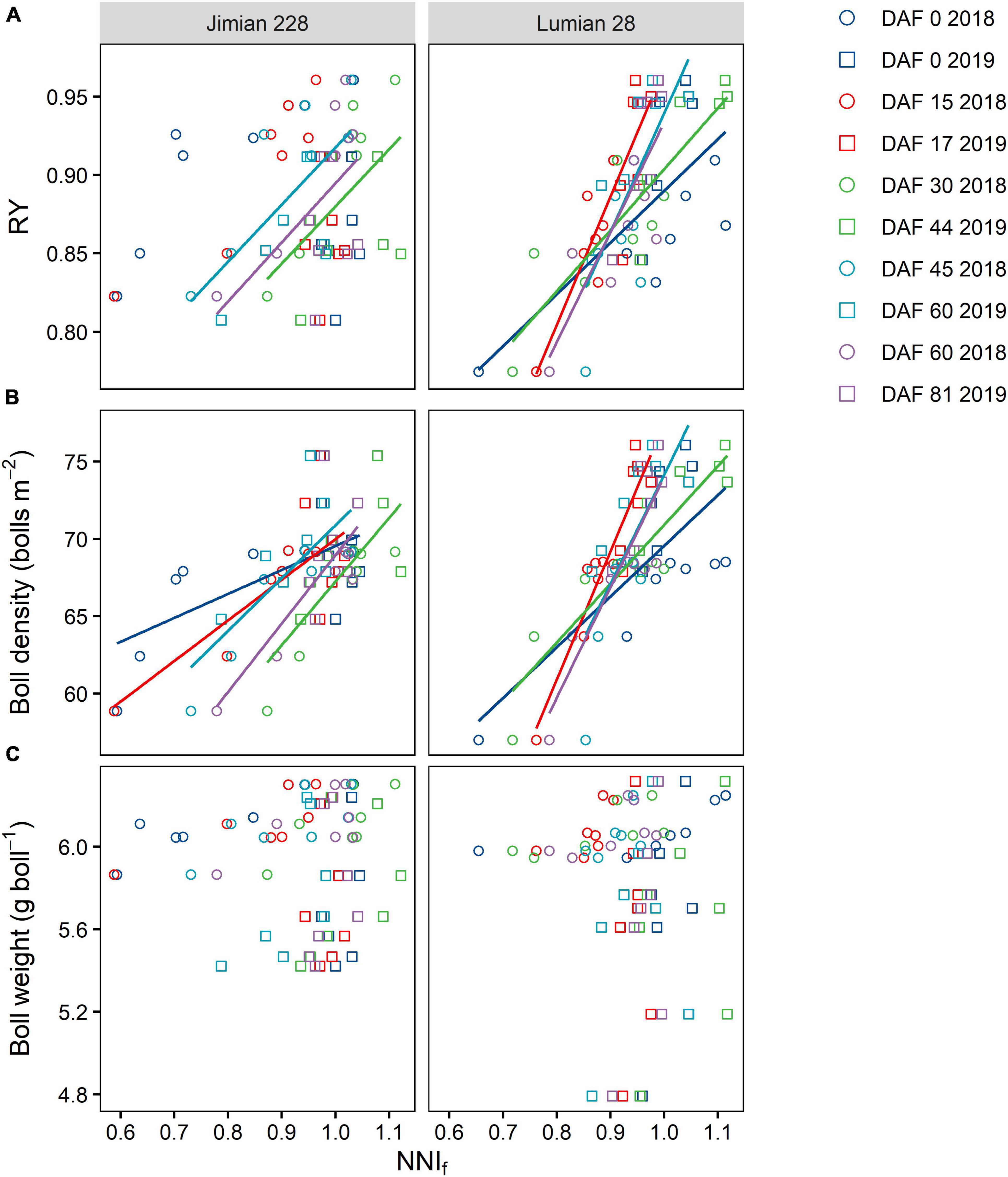
Figure 7. The relationships of RY (A), boll density (B), and boll weight (C) with the fruits nitrogen nutrition index (NNIf) for each cultivar at each sampling date. RY, relative yield; DAF, days after flowering; and the NNIf is calculated as the ratio between the actual fruits N concentration and the critical fruits N concentration. Calculation for relative yield is presented in section “Materials and Methods.” Different symbols indicate mean values of the three replicate plots. The color solid lines represent significant linear relationships (P < 0.05) for Jimian 228 and Lumian 28 at each sampling time across 2 years; non-significant relationships are not shown (P > 0.05).
Petiole NO3-N Concentration and Its Relationship With the Nitrogen Nutrition Index of Fruits
The nitrogen fertilization effect (P = 0.0004) was more pronounced than the cultivar effect on the petiole NO3-N concentration (P = 0.0141) (Supplementary Table 6). N fertilization increased the NO3-N concentration but no significant increase beyond 120 kg ha–1 was recorded. On average, the petiole NO3-N concentration was 4,143 and 4,449 mg L–1 for Jimian 228 and Lumian 28, respectively. Moreover, the petiole NO3-N concentration of Lumian 28 was consistently greater than that of Jimian 228 during any growth stage and N application rate (Figure 8A and Supplementary Table 6).
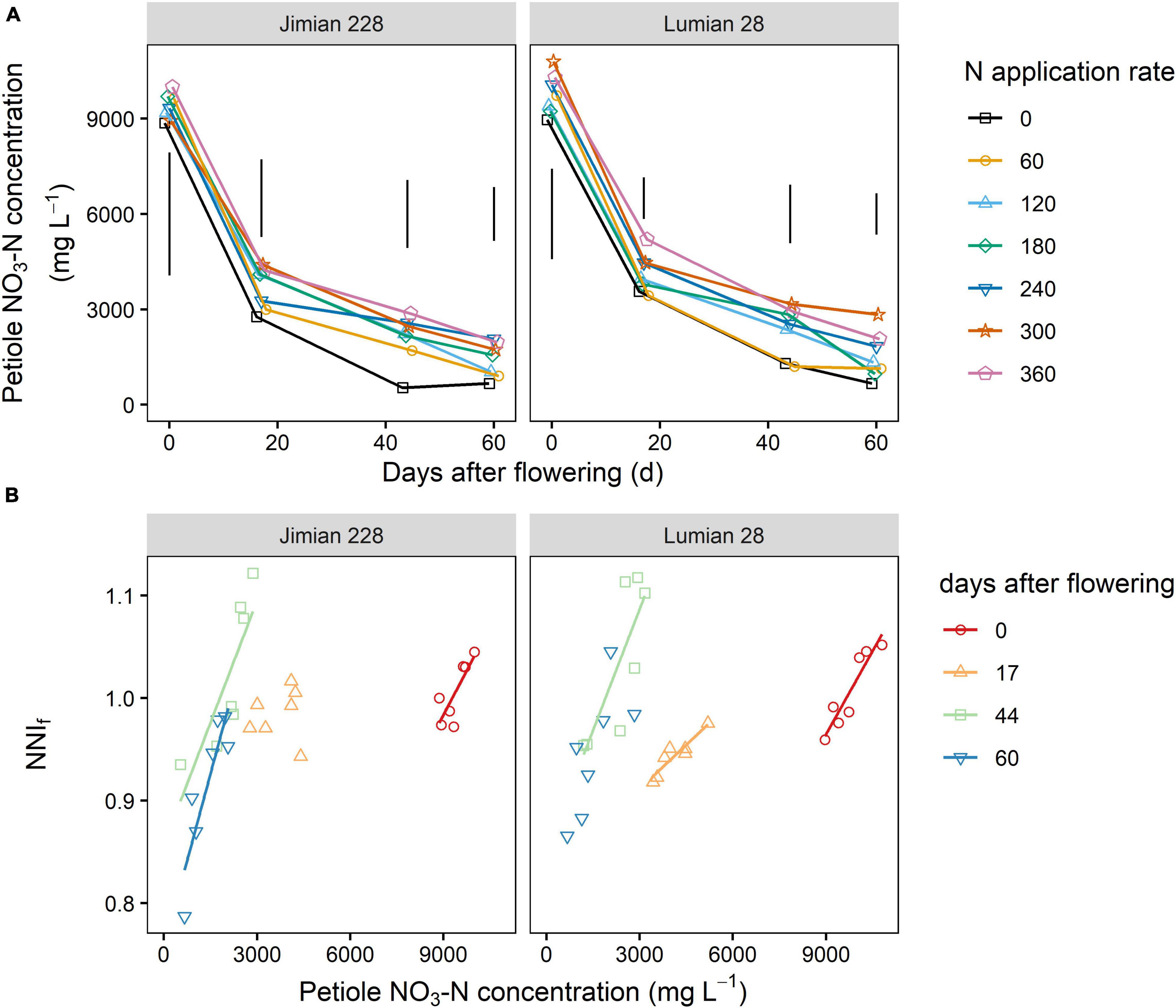
Figure 8. Petiole NO3-N concentration (A) and its relationship to the fruits nitrogen nutrition index (NNIf) (B) for Jimian 228 and Lumian 28 in 2019. Data show means of the three replicate plots. The NNIf is calculated as the ratio between the actual fruits N concentration and the critical fruits N concentration. (A) The vertical bars indicate Tukey’s HSD error bars at each sampling date. Full model ANOVA results are provided in Supplementary Table 6. (B) The color solid lines represent significant linear relationships (P < 0.05); non-significant relationships are not shown (P > 0.05).
Overall, there was a positive relationship between petiole NO3-N concentration and the NNIf under the two cotton cultivars, although it was not significant at 17 DAF for Jimian 228 and 60 DAF for Lumian 28 (Figure 8B). This relationship indicated that the NO3-N concentration in the petiole was a good predictor of the NNIf and consequently could be an alternative approach to evaluate fruits N status.
Discussion
Cotton Growth, Yield, and Nitrogen Responses
In the present study, significant effects on cotton shoot DM, fruits DM and [N] were observed in response to the N application rate. The shoot and fruits DM observed here were 8.88–15.7 and 4.02–8.48 t ha–1 for Jimian 228, 6.9–12.43 t ha–1 and 4.34–7.72 t ha–1 for Lumian 28, respectively, which are comparable to those reported by previous studies, depending on the treatments applied and environmental conditions (Bange and Milroy, 2004; Zhang et al., 2008; Dai et al., 2015). For example, in a similar agro-ecological region (near our study site), aboveground DM was 12.7 t ha–1 and fruits DM was 8.0 t ha–1 at a high plant density of 10.5 plants m–2 (Dai et al., 2015). Bange and Milroy (2004) also reported final shoot dry matter up to 18.7 t ha–1 varying significantly between diverse cotton genotypes in New South Wales of Australia. In addition, significant effects of the N fertilizer rate on DMs in shoot and fruits were more pronounced than on the respective [N] in the same sampling date, suggesting that fruits and shoot DMs were more responsive to N fertilizer than the respective [N].
However, a limited cotton yield response to the N fertilizer rate was observed, with a significant cultivar effect, indicating that the effects of the cultivar were far greater than those of N fertilizer. Little or no yield response to fertilizer N application was also reported by Dong et al. (2012) in the Yellow River Delta of China (near our study site) and Stamatiadis et al. (2016) in central Greece. The former observed a yield response to the N fertilizer rate only at low plant density (3 plants m–2). Contrary to our results, Li et al. (2017) found a linear increase in N concentration with increasing rates of N fertilizer despite the leveling-off or decline of yield. This conflict is likely derived from the difference between the critical soil N level or tested cultivar sensitivity to N fertilizer (Stamatiadis et al., 2016).
Critical Nitrogen Dilution Curve for Cotton Fruits
Fruits [N] gradually decreased with increasing fruits DM, due to the N dilution effect resulting from an increase in biomass accumulation that was greater than the N uptake (Lemaire et al., 2007). The N dilution effect has also been widely reported in several crop species, including winter wheat (Justes et al., 1994), maize (Ziadi et al., 2008b) and rice (Ata-Ul-Karim et al., 2013). In this study, the mean value of the [N]c in fruits with DM of 1 t ha–1 (2.49), estimated by the coefficient (a) of the N dilution curves, was much lower than that in the shoot biomass (greater than 5.0) (Xue et al., 2007; Zhang et al., 2008). This discrepancy may be related to the growth stage (Zhao et al., 2016a). The development stage when cotton fruits was equal to 1 t ha–1 is later than that when shoot biomass was 1 t ha–1. The dilution coefficient (b), however, was similar to that observed previously (Xue et al., 2007; Zhang et al., 2008), suggesting that there was a similar dilution process between fruits and the shoot as a result of the plants’ indeterminate growth habit. However, fruits N is more dependent on physiological N mobilization from stems and leaves, while only N added at fruit formation is directly mobilized to fruits (see below).
The critical N dilution curve for cotton fruits, in our study, had a notably lower dilution of N with increasing dry mass than a universal curve for C3 crops reported by Lemaire and Gastal (1997). The limited N dilution of fruits is partly explained by the indeterminate nature. Cotton plants continuously produce new organs, including fruits, simultaneously, which differs in many respects from C3 crops. Continued production of new fruits in cotton crops maintains a relatively high N content throughout the crop growth, which was attributed to the high translocation of N from stems and leaves to fruits. In other crops, relatively limited dilution was also observed in sunflower (Debaeke et al., 2012) and sweet pepper (Rodríguez et al., 2020).
In addition, fruits-based critical N curves showed small variations between the tested cotton cultivars differing in maturity. A recent study reported that the changes in plant canopy architecture may be a potential interpretation of variations in the N dilution curve (Ciampitti et al., 2021). Therefore, plant architecture, rather than precocity, needs to be considered with a focus on the genotypic effects on the N dilution curve in future studies.
Although the critical N curve developed for cotton fruits could effectively differentiate between N limiting and N non-limiting situations within the range for which it was established, note that this study was conducted in a single field site. Therefore, further study with additional sites will be necessary since the determination of [N] with shoot biomass might be affected by sites as reported for rice (Ata-Ul-Karim et al., 2013) and forage grasses (Agnusdei et al., 2010).
Nitrogen Nutrition Index
The critical N dilution curve allows for the determination of the NNI, which quantifies the intensity of both N deficiency and N luxury consumption for a given crop (Lemaire and Gastal, 1997). Values of the NNI close to 1 indicate that the crop is not limited by the N supply, while values lower or higher than 1 indicate N deficiency or N luxury consumption, respectively. The NNIf changed little with cotton growth after the flowering period, indicating a stable N requirement in fruits after the flowering period.
The positive relationship between the NNIf and NNIsh under the two cultivars at most sampling dates suggested that the NNI in shoot biomass could be easily estimated using the NNI in fruits. These results were aligned with similar recent findings in which a close relationship between the NNI in vines or tubers and the NNI in total biomass was observed for potato (Giletto et al., 2020). Therefore, N nutrition sufficiency can be estimated with derived NNIf values from fruits based on N dilution curve by only sampling for cotton fruits biomass, which would represent a fast and cost-effective option.
The linear relationship of RY with the NNIf indicated that the RY was nearly 95% when the NNIf was approximately one, and it decreased with a decreasing NNIf below 1. Therefore, our results demonstrated that the NNIf adequately identified the N status in cotton cultivars and had potential as a plant diagnostic tool to estimate the N status of cotton crops.
In this study, the NNIf was used to predict the yield response to N fertilization and to develop strategies to manage crop N nutrition to match the N supply with crop demand after the flowering period. As a result, attention should be paid to the time lag if we use it to guide N fertilizer application in cotton production, especially at the early stage of cotton growth.
Alternative Diagnostic Methods
Although the NNI can be used to guide N management in crop production, the measurement is a laborious process that greatly limits the practical application of these diagnostic methods. Therefore, a rapid measurement method is needed. A good correlation between petiole NO3-N concentration and the NNIf was observed in our study, suggesting that the NNIf could be estimated with petiole NO3-N concentration. Moreover, the use of petiole NO3-N has the advantages of being a more rapid and simple measurement than determinations of shoot DM and [N] (Wu et al., 2007). Therefore, this indirect method could potentially provide an alternative for estimating the NNI, avoiding time-consuming and labor-intensive shoot biomass sampling, and could characterize crops and environments in situations where the NNI cannot be measured directly.
Future research efforts should attempt to investigate whether other N diagnostic tools (e.g., chlorophyll meters, active crop sensors, and remote sensing) can be used to estimate the N status across different cultivars and environmental conditions (Liu et al., 2020; Shankar et al., 2020), which could complement our results.
Conclusion
The fruits [N]c decreased with increasing fruits DM in cotton due to the N dilution process. A unique critical fruits N dilution curve of [N]c = 2.49 × DM–0.12 (DM, fruits dry mass; R2 = 0.649, P < 0.0001), with [N]c as the critical N concentration in fruits, was developed for cotton fruits across cultivar-years. The fruits N dilution curve could effectively discriminate between N limiting and N non-limiting situations within the range for which it was established. From the dilution curve developed for fruits DM, the NNIf was determined, which can be used as an N status diagnostic tool. The NNIf values were highly related to the NNIsh, suggesting that the NNI in shoot biomass could be easily estimated using the NNI in fruits. On average, the RY was nearly 95% for an NNIf at approximately 1, while it decreased with a decreasing NNIf below 1. The NNIf was also linearly related to petiole NO3-N concentration, suggesting that NO3-N concentration in petioles is a good predictor of the NNIf. The critical fruits N dilution curve developed in this study provides insight into plant N nutrition and can serve as a guide to improve N diagnosis and management in cotton production. Future research efforts should attempt to investigate whether the N nutrition index in specific plant organs can be used to determine threshold values for N sufficiency with other N diagnostic tools (e.g., chlorophyll meters, active crop sensors and remote sensing). Moreover, more attention should be paid to the time lag if we use this method to guide N fertilizer application in cotton production, especially at the early stage of cotton growth.
Data Availability Statement
The raw data supporting the conclusions of this article will be made available by the authors, without undue reservation.
Author Contributions
HD, PL, and CZ designed the experiment. WF performed the field and lab work with help from YQ, MS, and JS. WF and XL analyzed the data and led the writing of the manuscript. All authors contributed to editing the manuscript and gave final approval for publication.
Funding
This work was financially supported by the National Key Research and Development Program of China (2017YFD0200107) and Agricultural Science and Technology Innovation Program of Chinese Academy of Agricultural Sciences.
Conflict of Interest
The authors declare that the research was conducted in the absence of any commercial or financial relationships that could be construed as a potential conflict of interest.
Publisher’s Note
All claims expressed in this article are solely those of the authors and do not necessarily represent those of their affiliated organizations, or those of the publisher, the editors and the reviewers. Any product that may be evaluated in this article, or claim that may be made by its manufacturer, is not guaranteed or endorsed by the publisher.
Acknowledgments
We gratefully acknowledge Hezhong Dong at Institute of Industrial Crops, Shandong Academy of Agricultural Sciences for critical comments on earlier versions of this manuscript. Xiaoxi Li at CSIRO Agriculture and Food and Ruipeng Yu at China Agricultural University assisted in data analysis and visualization. We would like to thank Beili Hou, Baoli Lu, Baoxiang Tian and Zhenshan Zhang at Institute of Cotton Research, Chinese Academy of Agricultural Sciences for their help in the field and/or laboratory.
Supplementary Material
The Supplementary Material for this article can be found online at: https://www.frontiersin.org/articles/10.3389/fpls.2022.801968/full#supplementary-material
Abbreviations
[N]c, critical nitrogen concentration; DAF, days after flowering; DM, dry mass; N, nitrogen; [N], nitrogen concentration; NNI, nitrogen nutrition index; NNIf, nitrogen nutrition index of fruits; NNIsh, nitrogen nutrition index of the shoot; RY, relative yield.
References
Agnusdei, M. G., Assuero, S. G., Lattanzi, F. A., and Marino, M. A. (2010). Critical N concentration can vary with growth conditions in forage grasses: implications for plant N status assessment and N deficiency diagnosis. Nutr. Cycl. Agroecosyst. 88, 215–230. doi: 10.1007/s10705-010-9348-6
Arregui, L. M., Lasa, B., Lafarga, A., Irañeta, I., Baroja, E., and Quemada, M. (2006). Evaluation of chlorophyll meters as tools for N fertilization in winter wheat under humid Mediterranean conditions. Eur. J. Agron. 24, 140–148. doi: 10.1016/j.eja.2005.05.005
Ata-Ul-Karim, S. T., Liu, X., Lu, Z., Zheng, H., Cao, W., and Zhu, Y. (2017). Estimation of nitrogen fertilizer requirement for rice crop using critical nitrogen dilution curve. Field Crops Res. 201, 32–40. doi: 10.1016/j.fcr.2016.10.009
Ata-Ul-Karim, S. T., Yao, X., Liu, X., Cao, W., and Zhu, Y. (2013). Development of critical nitrogen dilution curve of Japonica rice in Yangtze River Reaches. Field Crops Res. 149, 149–158. doi: 10.1016/j.fcr.2013.03.012
Ata-Ul-Karim, S. T., Zhu, Y., Yao, X., and Cao, W. (2014). Determination of critical nitrogen dilution curve based on leaf area index in rice. Field Crops Res. 167, 76–85. doi: 10.1016/j.fcr.2014.07.010
Bange, M. P., and Milroy, S. P. (2004). Growth and dry matter partitioning of diverse cotton genotypes. Field Crops Res. 87, 73–87. doi: 10.1016/j.fcr.2003.09.007
Chen, R. R., Zhu, Y., Cao, W. X., and Tang, L. (2021). A bibliometric analysis of research on plant critical dilution curve conducted between 1985 and 2019. Eur. J. Agron. 123:126199. doi: 10.1016/j.eja.2020.126199
Chen, Z. J., Scheffler, B. E., Dennis, E., Triplett, B. A., Zhang, T., Guo, W., et al. (2007). Toward sequencing cotton (Gossypium) genomes. Plant Physiol. 145, 1303–1310. doi: 10.1104/pp.107.107672
Ciampitti, I. A., Fernandez, J., Tamagno, S., Zhao, B., Lemaire, G., and Makowski, D. (2021). Does the critical N dilution curve for maize crop vary across genotype x environment x management scenarios? - a Bayesian analysis. Eur. J. Agron. 123:126202. doi: 10.1016/j.eja.2020.126202
Cotton Research Institute Chinese Academy of Agricultural Sciences [CRI] (2013). Cultivation of Cotton in China. Shanghai: Shanghai Science and Technology Press.
Dai, J. L., Li, W. J., Tang, W., Zhang, D. M., Li, Z. H., Lu, H. Q., et al. (2015). Manipulation of dry matter accumulation and partitioning with plant density in relation to yield stability of cotton under intensive management. Field Crops Res. 180, 207–215. doi: 10.1016/j.fcr.2015.06.008
Debaeke, P., Van Oosterom, E. J., Justes, E., Champolivier, L., Merrien, A., Aguirrezabal, L. A. N., et al. (2012). A species-specific critical nitrogen dilution curve for sunflower (Helianthus annuus L.). Field Crops Res 136, 76–84. doi: 10.1016/j.fcr.2012.07.024
Diacono, M., Rubino, P., and Montemurro, F. (2013). Precision nitrogen management of wheat. a review. Agron. Sustain. Dev. 33, 219–241. doi: 10.1007/s13593-012-0111-z
Dong, H. Z., Li, W. J., Eneji, A. E., and Zhang, D. M. (2012). Nitrogen rate and plant density effects on yield and late-season leaf senescence of cotton raised on a saline field. Field Crops Res. 126, 137–144. doi: 10.1016/j.fcr.2011.10.005
Du, L., Li, Q., Li, L., Wu, Y., Zhou, F., Liu, B., et al. (2020). Construction of a critical nitrogen dilution curve for maize in Southwest China. Sci. Rep. 10:13084. doi: 10.1038/s41598-020-70065-3
Flénet, F., Guérif, M., Boiffin, J., Dorvillez, D., and Champolivier, L. (2006). The critical N dilution curve for linseed (Linum usitatissimum L.) is different from other C3 species. Eur. J. Agron. 24, 367–373. doi: 10.1016/j.eja.2006.01.002
Geng, J., Ma, Q., Zhang, M., Li, C., Liu, Z., Lyu, X., et al. (2015). Synchronized relationships between nitrogen release of controlled release nitrogen fertilizers and nitrogen requirements of cotton. Field Crops Res. 184, 9–16. doi: 10.1016/j.fcr.2015.09.001
Giletto, C. M., Reussi Calvo, N. I., Sandaña, P., Echeverría, H. E., and Bélanger, G. (2020). Shoot- and tuber-based critical nitrogen dilution curves for the prediction of the N status in potato. Eur. J. Agron. 119:126114. doi: 10.1016/j.eja.2020.126114
Greenwood, D. J., Lemaire, G., Gosse, G., Cruz, P., Draycott, A., and Neeteson, J. J. (1990). Decline in percentage N of C3 and C4 crops with increasing plant mass. Ann. Bot. 66, 425–436. doi: 10.1093/oxfordjournals.aob.a088044
Justes, E., Mary, B., Meynard, J. M., Machet, J. M., and Thelier-Huche, L. (1994). Determination of a critical nitrogen dilution curve for winter wheat crops. Ann. Bot. 74, 397–407. doi: 10.1006/anbo.1994.1133
Lebauer, D. S., and Treseder, K. K. (2008). Nitrogen limitation of net primary productivity in terrestrial ecosystems is globally distributed. Ecology 89, 371–379. doi: 10.1890/06-2057.1
Lemaire, G., and Gastal, F. (1997). “N uptake and distribution in plant canopies,” in Diagnosis of the Nitrogen Status in Crops, ed. G. Lemaire (Berlin: Springer Berlin Heidelberg), 3–43. doi: 10.1007/978-3-642-60684-7_1
Lemaire, G., and Gastal, F. (2018). “Crop responses to nitrogen,” in Encyclopedia of Sustainability Science and Technology, ed. R. A. Meyers (New York, NY: Springer), 1–27. doi: 10.1007/978-1-4939-2493-6_385-3
Lemaire, G., Jeuffroy, M.-H., and Gastal, F. (2008a). Diagnosis tool for plant and crop N status in vegetative stage: theory and practices for crop N management. Eur. J. Agron. 28, 614–624. doi: 10.1016/j.eja.2008.01.005
Lemaire, G., Van Oosterom, E., Jeuffroy, M. H., Gastal, F., and Massignam, A. (2008b). Crop species present different qualitative types of response to N deficiency during their vegetative growth. Field Crops Res. 105, 253–265. doi: 10.1016/j.fcr.2007.10.009
Lemaire, G., Oosterom, E. V., Sheehy, J., Jeuffroy, M. H., Massignam, A., and Rossato, L. (2007). Is crop N demand more closely related to dry matter accumulation or leaf area expansion during vegetative growth? Field Crops Res. 100, 91–106. doi: 10.1016/j.fcr.2006.05.009
Li, P. C., Dong, H. L., Zheng, C. S., Sun, M., Liu, A. Z., Wang, G. P., et al. (2017). Optimizing nitrogen application rate and plant density for improving cotton yield and nitrogen use efficiency in the North China Plain. PLoS One 12:e0185550. doi: 10.1371/journal.pone.0185550
Li, X. F., Han, Y. C., Wang, G. P., Feng, L., Wang, Z. B., Yang, B. F., et al. (2020). Response of cotton fruit growth, intraspecific competition and yield to plant density. Eur. J. Agron. 114:125991. doi: 10.1016/j.eja.2019.125991
Liu, S. S., Li, L. T., Fan, H. Y., Guo, X. Y., Wang, S. Q., and Lu, J. W. (2020). Real-time and multi-stage recommendations for nitrogen fertilizer topdressing rates in winter oilseed rape based on canopy hyperspectral data. Ind. Crop. Prod. 154:112699. doi: 10.1016/j.indcrop.2020.112699
Luo, Z., Liu, H., Li, W., Zhao, Q., Dai, J., Tian, L., et al. (2018). Effects of reduced nitrogen rate on cotton yield and nitrogen use efficiency as mediated by application mode or plant density. Field Crops Res. 218, 150–157. doi: 10.1016/j.fcr.2018.01.003
Lv, Z., He, W., Lin, L., Deng, L., Yu, M., Fei, C., et al. (2020). Development of critical shoot and root N curves for diagnosing sweetpotato shoot and root N status. Field Crops Res. 257:107927. doi: 10.1016/j.fcr.2020.107927
Ma, L., Zhang, Q., Lin, J., Su, W., Yin, C., Lv, X., et al. (2019). The estimation of nitrogen uptake and utilization efficiency in cotton by the fertilizer-response model. Not. Bot. Horti. Agrobo. 47, 1400–1408. doi: 10.15835/nbha47411672
Mei, W., and Yu, G. (2018). BasicTrendline: Add Trendline And Confidence Interval Of Basic Regression Models To Plot. R Package Version 2.0.3. Available online at: https://cran.r-project.org/package=basicTrendline (accessed May 15, 2021).
National Bureau of Statistics of China (2020). China Statistical Yearbook-2020. Beijing: China Statistics Publishing. Available online at: http://www.stats.gov.cn/tjsj/ndsj/2020/indexch.htm (accessed May 15, 2021).
Pinheiro, J., Bates, D., Debroy, S., Sarkar, D., and R Core Team (2020). Nlme: Linear and Nonlinear Mixed Effects Models. R Package Version 3.1-144. Available online at: https://cran.r-project.org/package=nlme (accessed May 20, 2021).
Plénet, D., and Lemaire, G. (1999). Relationships between dynamics of nitrogen uptake and dry matter accumulation in maize crops. Determination of critical N concentration. Plant Soil 216, 65–82. doi: 10.1023/A:1004783431055
R Core Team (2019). R: A Language And Environment For Statistical Computing. Vienna: R Foundation for Statistical Computing.
Reis, S., Bekunda, M., Howard, C. M., Karanja, N., Winiwarter, W., Yan, X., et al. (2016). Synthesis and review: tackling the nitrogen management challenge: from global to local scales. Environ. Res. Lett. 11:120205. doi: 10.1088/1748-9326/11/12/120205
Rodríguez, A., Peña-Fleitas, M. T., Gallardo, M., De Souza, R., Padilla, F. M., and Thompson, R. B. (2020). Sweet pepper and nitrogen supply in greenhouse production: critical nitrogen curve, agronomic responses and risk of nitrogen loss. Eur. J. Agron. 117:126046. doi: 10.1016/j.eja.2020.126046
Shankar, A., Gupta, R. K., and Singh, B. (2020). Site-specific fertilizer nitrogen management in Bt cotton using chlorophyll meter. Exp. Agric. 56, 397–406. doi: 10.1017/S0014479720000046
Stamatiadis, S., Tsadilas, C., Samaras, V., Schepers, J. S., and Eskridge, K. (2016). Nitrogen uptake and N-use efficiency of Mediterranean cotton under varied deficit irrigation and N fertilization. Eur. J. Agron. 73, 144–151. doi: 10.1016/j.eja.2015.11.013
United States Department of Agriculture [USDA] (2019). Foreign Agricultural Service System: Production, Supply and Distribution. Available online at: https://apps.fas.usda.gov/psdonline/app/index.html#/app/home (accessed May 13, 2021).
Vitousek, P. M., Naylor, R., Crews, T., David, M. B., Drinkwater, L. E., Holland, E., et al. (2009). Nutrient imbalances in agricultural development. Science 324, 1519–1520. doi: 10.1126/science.1170261
Wang, Y. H., Zhao, X. H., Chen, B. L., Gao, X. B., and Zhou, Z. G. (2012). Relationship between the N concentration of the leaf subtending boll and the cotton fiber quality. J. Integr. Agric. 11, 2013–2019. doi: 10.1016/S2095-3119(12)60458-4
Weymann, W., Sieling, K., and Kage, H. (2017). Organ-specific approaches describing crop growth of winter oilseed rape under optimal and N-limited conditions. Eur. J. Agron. 82, 71–79. doi: 10.1016/j.eja.2016.10.005
Wu, F., Wu, L., and Xu, F. (1998). Chlorophyll meter to predict nitrogen sidedress requirements for short-season cotton (Gossypium hirsutum L.). Field Crops Res. 56, 309–314. doi: 10.1016/S0378-4290(97)00108-1
Wu, J., Wang, D., Rosen, C. J., and Bauer, M. E. (2007). Comparison of petiole nitrate concentrations. SPAD chlorophyll readings, and QuickBird satellite imagery in detecting nitrogen status of potato canopies. Field Crops Res. 101, 96–103. doi: 10.1016/j.fcr.2006.09.014
Xue, X., Wang, J., Wang, Z., Guo, W., and Zhou, Z. (2007). Determination of a critical dilution curve for nitrogen concentration in cotton. J. Plant Nutr. Soil Sci. 170, 811–817. doi: 10.1002/jpln.200620627
Yan, X. Y., Ti, C. P., Vitousek, P., Chen, D. L., Leip, A., Cai, Z. C., et al. (2014). Fertilizer nitrogen recovery efficiencies in crop production systems of China with and without consideration of the residual effect of nitrogen. Environ. Res. Lett. 9:095002. doi: 10.1088/1748-9326/9/9/095002
Yang, G. Z., Chu, K. Y., Tang, H. Y., Nie, Y. C., and Zhang, X. L. (2013). Fertilizer 15N accumulation, recovery and distribution in cotton plant as affected by N rate and split. J. Integr. Agric. 12, 999–1007. doi: 10.1016/S2095-3119(13)60477-3
Yang, G. Z., Tang, H. Y., Nie, Y. C., and Zhang, X. L. (2011). Responses of cotton growth, yield, and biomass to nitrogen split application ratio. Eur. J. Agron. 35, 164–170. doi: 10.1016/j.eja.2011.06.001
Yao, X., Zhao, B., Tian, Y. C., Liu, X. J., Ni, J., Cao, W. X., et al. (2014). Using leaf dry matter to quantify the critical nitrogen dilution curve for winter wheat cultivated in eastern China. Field Crops Res. 159, 33–42. doi: 10.1016/j.fcr.2013.12.007
Zhang, H. Z., Khan, A., Tan, D. K. Y., and Luo, H. H. (2017). Rational water and nitrogen management improves root growth, increases yield and maintains water use efficiency of cotton under mulch drip irrigation. Front. Plant Sci. 8:912. doi: 10.3389/fpls.2017.00912
Zhang, L., Spiertz, J. H. J., Zhang, S., Li, B., and Van Der Werf, W. (2008). Nitrogen economy in relay intercropping systems of wheat and cotton. Plant Soil 303, 55–68. doi: 10.1007/s11104-007-9442-y
Zhang, Y., and Dong, H. (2020). “Yield and fiber quality of cotton,” in Encyclopedia of Renewable and Sustainable Materials, eds S. Hashmi and I. A. Choudhury (Oxford: Elsevier), 356–364. doi: 10.1016/b978-0-12-803581-8.11166-x
Zhao, B., Liu, Z., Ata-Ul-Karim, S. T., Xiao, J., Liu, Z., Qi, A., et al. (2016b). Rapid and nondestructive estimation of the nitrogen nutrition index in winter barley using chlorophyll measurements. Field Crops Res. 185, 59–68. doi: 10.1016/j.fcr.2015.10.021
Zhao, B., Ata-Ui-Karim, S. T., Yao, X., Tian, Y., Cao, W., Zhu, Y., et al. (2016a). A new curve of critical nitrogen concentration based on spike dry matter for winter wheat in eastern China. PLoS One 11:e0164545. doi: 10.1371/journal.pone.0164545
Zhao, B., Ata-Ul-Karim, S. T., Liu, Z., Ning, D., Xiao, J., Liu, Z., et al. (2017). Development of a critical nitrogen dilution curve based on leaf dry matter for summer maize. Field Crops Res. 208, 60–68. doi: 10.1016/j.fcr.2017.03.010
Zhao, B., Niu, X., Ata-Ul-Karim, S. T., Wang, L., Duan, A., Liu, Z., et al. (2020). Determination of the post-anthesis nitrogen status using ear critical nitrogen dilution curve and its implications for nitrogen management in maize and wheat. Eur. J. Agron. 113:125967. doi: 10.1016/j.eja.2019.125967
Ziadi, N., Brassard, M., Bélanger, G., Claessens, A., Tremblay, N., Cambouris, A. N., et al. (2008a). Chlorophyll measurements and nitrogen nutrition index for the evaluation of corn nitrogen status. Agron. J. 100, 1264–1273. doi: 10.2134/agronj2008.0016
Keywords: cotton yield, fertilization management, nitrogen nutrition index, petiole nitrate concentration, yield components
Citation: Feng W, Li X, Dong H, Qin Y, Sun M, Shao J, Zheng C and Li P (2022) Fruits-Based Critical Nitrogen Dilution Curve for Diagnosing Nitrogen Status in Cotton. Front. Plant Sci. 13:801968. doi: 10.3389/fpls.2022.801968
Received: 26 October 2021; Accepted: 05 January 2022;
Published: 28 January 2022.
Edited by:
Marta Wilton Vasconcelos, Catholic University of Portugal, PortugalReviewed by:
Renato De Mello Prado, São Paulo State University, BrazilCorina Carranca, Instituto Nacional de Investigação Agraria e Veterinaria, I. P. (INIAV), Portugal
Copyright © 2022 Feng, Li, Dong, Qin, Sun, Shao, Zheng and Li. This is an open-access article distributed under the terms of the Creative Commons Attribution License (CC BY). The use, distribution or reproduction in other forums is permitted, provided the original author(s) and the copyright owner(s) are credited and that the original publication in this journal is cited, in accordance with accepted academic practice. No use, distribution or reproduction is permitted which does not comply with these terms.
*Correspondence: Cangsong Zheng, emhlbmdjYW5nc29uZ0BjYWFzLmNu; Pengcheng Li, bGlwZW5nY2hlbmdjcmlAMTYzLmNvbQ==
†These authors have contributed equally to this work and share first authorship