- 1National Professor Project, ICAR-Indian Institute of Rice Research, Hyderabad, India
- 2Department of Biotechnology, Acharya Nagarjuna University, Guntur, India
Wild introgressions play a crucial role in crop improvement by transferring important novel alleles and broadening allelic diversity of cultivated germplasm. In this study, two stable backcross alien introgression lines 166s and 14s derived from Swarn/Oryza nivara IRGC81848 were used as parents to generate populations to map quantitative trait loci (QTLs) for yield-related traits. Field evaluation of yield-related traits in F2, F3, and F4 population was carried out in normal irrigated conditions during the wet season of 2015 and dry seasons of 2016 and 2018, respectively. Plant height, tiller number, productive tiller number, total dry matter, and harvest index showed a highly significant association to single plant yield in F2, F3, and F4. In all, 21, 30, and 17 QTLs were identified in F2, F2:3, and F2:4, respectively, for yield-related traits. QTLs qPH6.1 with 12.54% phenotypic variance (PV) in F2, qPH1.1 with 13.01% PV, qTN6.1 with 10.08% PV in F2:3, and qTGW6.1 with 15.19% PV in F2:4 were identified as major effect QTLs. QTLs qSPY4.1 and qSPY6.1 were detected for grain yield in F2 and F2:3 with PV 8.5 and 6.7%, respectively. The trait enhancing alleles of QTLs qSPY4.1, qSPY6.1, qPH1.1, qTGW6.1, qTGW8.1, qGN4.1, and qTDM5.1 were from O. nivara. QTLs of the yield contributing traits were found clustered in the same chromosomal region. qTGW8.1 was identified in a 2.6 Mb region between RM3480 and RM3452 in all three generations with PV 6.1 to 9.8%. This stable and consistent qTGW8.1 allele from O. nivara can be fine mapped for identification of causal genes. From this population, lines C212, C2124, C2128, and C2143 were identified with significantly higher SPY and C2103, C2116, and C2117 had consistently higher thousand-grain weight values than both the parents and Swarna across the generations and are useful in gene discovery for target traits and further crop improvement.
Introduction
Rice is one of three major food crops across the world especially in the most populated regions, and it provides up to 23% of calories for human consumption (Fisher et al., 2000). A total of 503.5 million tonnes (milled basis) of rice was consumed around the globe during 2017–18 (FAO et al., 2017). Rice breeders have major challenges in increasing the yield potential of the cultivars as there is stagnation due to narrow genetic diversity available in cultivated germplasm. Wild genetic material is a source of important alleles or genes for agronomic traits including yield. Most of the earlier studies using conventional plant breeding methods helped increase of the yield levels of rice by improving the related traits (Brondani et al., 2002). Wild rice species are more diverse in physiological, morphological, and agronomical characteristics than the existing cultivars. Because of the narrow genetic base of the cultivars, there is a need to transfer genes of desirable traits from wild to cultivated rice, and it is an essential strategy in pre-breeding. Wild species have beneficial alleles for yield improvement, but the expression of these alleles is frequently masked due to the presence of other detrimental loci. Yet, several yields enhancing quantitative trait loci (QTLs) have been mapped in the last 25 years from wild species of rice for genetical improvement (Swamy and Sarla, 2008; Gaikwad et al., 2021). The wild rice species, Oryza nivara, is the closest wild progenitor of cultivated rice O. sativa (Haritha et al., 2018). O. nivara accessions showed high genetic diversity in its gene pool with adaptability in different environments (Sarla et al., 2003; Juneja et al., 2006) and is a proven choice to improve the yield levels of cultivars (Vaughan et al., 2003, 2008; Swamy et al., 2014; Ma et al., 2016).
An advanced back cross method is a technique to introduce the favorable alleles from wild into cultivar background (Ma et al., 2016). There are many reports on mapping yield QTLs using wild species (Swamy and Sarla, 2008; Swamy et al., 2011, 2014; Wickneswari et al., 2012; Ma et al., 2016; Bhatia et al., 2018). Back cross inbred lines are useful for mapping QTLs and gene pyramiding (Bhatia et al., 2018; Wang et al., 2018). In addition, QTL pyramiding using introgression lines (ILs) is an effective method in molecular breeding for complex traits (Feng et al., 2018). Back cross introgression lines (BILs) derived from cultivar/wild crosses or alien introgression lines were known for improving yield traits along with different desirable traits, such as quality-related traits and biotic and abiotic stress resistance (Mahmoud et al., 2008; Brar and Singh, 2011; Swamy et al., 2012, 2014). BILs generated by repeated backcrosses are useful in restoring the pollen fertility and eliminating the undesirable trait effects on the cultivar background (Swamy and Sarla, 2008). Several studies used BC2F2 populations derived from interspecific crosses to map QTLs. However, when introgression lines that are genetically similar to each other but phenotypically different are used, e.g., near-isogenic lines, the power to map the phenotype increases considerably. Compared to BC2F2, these advanced ILs in BC2F8 do not simultaneously have the confounding effects of segregation of several QTLs all over the genome or the complex epistatic interactions in the genome. Therefore, two high-yielding stable, fine grain BC2F8 Ils 166s and 14s derived from Swarna/O. nivara cross, were chosen to map QTLs for yield-related traits. These BC2F8 are derived from 166s (IET21938) and 14s (IET2274) which were identified as two fine-grain introgression lines earlier in BC2F2 (Swamy et al., 2012) and were shown in BC2F6–7 to be high-yielding in multilocation trials (Haritha et al., 2018) and are also salt tolerant (Ganeshan et al., 2016). The selected parental lines, viz., 166s and 14s with 75.8 and 77.8% recurrent parent genome, respectively, were part of a library of Swarna chromosome segment substitution lines (CSSLs) having chromosome segment substitutions from O. nivara (Balakrishnan et al., 2016; Surapaneni et al., 2017) and showed stability in yield levels over the generations. Among several traits to differentiate the two lines, 166s has a larger number of grains, and 14s has higher thousand-grain weight. Both of which are major yield contributing traits (Supplementary Table 1).
Previously, Pang et al. (2017) and Feng et al. (2018) reported QTLs in the population, generated by crossing Ils developed from local rice varieties. Similarly, QTLs were reported in the F2 population derived from a cross between a back cross inbred line (japonica × indica) and a japonica cultivar Z550 (Wang et al., 2018). Various studies reported QTLs for yield traits in rice using primary mapping populations like F2:3 (Sabouri et al., 2009; Zhang et al., 2010; Kim et al., 2014; Tian et al., 2015; Biswas et al., 2017; Kumar et al., 2019) and F2:4 (Rabiei et al., 2015; Verma et al., 2017; Jeon et al., 2018; Kumar et al., 2019). Crossing of two wild-derived back cross inbred lines generate hybrid lines which can closely resemble the parental lines (Wang et al., 2018) with combination of improved traits. We selected these two high yielding BILs with contrasting yield contributing traits to develop F2:3:4 populations with the objective of mapping consistent and precise QTLs for yield-related traits across three generations.
Materials and Methods
Plant Material and Field Evaluation
Initially, a set of back cross introgression lines at BC2F2 were developed from a cross of Swarna × O. nivara (Swamy et al., 2014), and this material was advanced up to BC2F8 through single panicle selection of selected individual lines. These lines were screened for three consecutive seasons to study G × E interaction of yield and related traits, and two BC2F8 lines, viz., 166s and 14s, were identified as most stable BILs for different yield-related traits (Balakrishnan et al., 2016; Kavitha et al., 2019). BIL 166s had high biomass, total dry matter, number of grains, seedling vigor, and high photosynthetic rate compared to parent Swarna and was also tolerant to aerobic and saline conditions based on our previous studies. BIL 14s had high single plant yield, bulk yield, per-day productivity, harvest index, 1,000 grain weight, and low unfilled grains. These BC2F8 BILs, 166s [IET27223] as female parent and 14s [IET 26772] as male parent, were taken as starting material for the experiment and F1 was generated by crossing them (Figure 1). In Rabi 2015, F1 plant was raised and selfed to generate F2 mapping population. F2 population was raised in Kharif 2015 and forwarded to F3 and F4 populations in Rabi 2016 and Rabi 2018, respectively, at the Indian Institute of Rice Research (IIRR) field. The farm is located in Hyderabad, India at 17° 19′ N latitude and 78° 29′ E longitude.
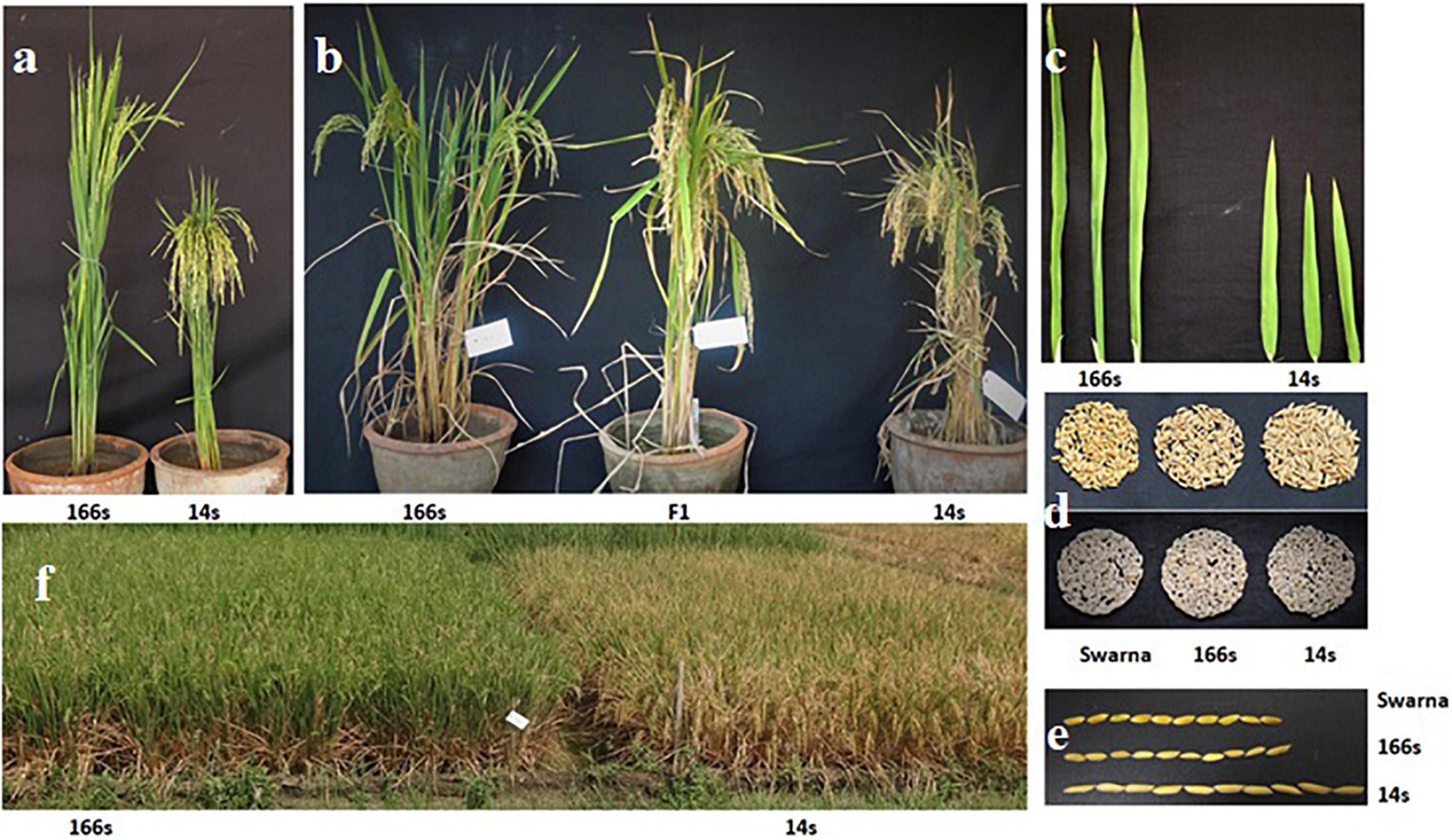
Figure 1. Phenotypic characteristics of parental lines, plant, and grain traits of 166s, 14s, and Swarna. (a) Parental lines 166s and 14s, (b) 166s, 14s parents with F1 (166s × 14s hybrid-middle one) plant, (c) Flag leaf length of 166s and 14s, (d,e) Swarna, 166s and 14s grains with husk and without husk and length of grains, (f) Field view of 166s and 14s.
Field evaluation of yield-related traits in the F2 population was carried out in normal irrigated field conditions during Kharif 2015 using the standard evaluation system of IRRI (SES, IRRI) for 19 phenotypic traits. These were days to initial flowering (DIF), plant height (PH), tiller number (TN), productive tiller number (PTN), flag leaf length (FLL), leaf length (LL), leaf width (LW), culm length (CL), single plant yield (SPY), biomass (BM), total dry matter (TDM), harvest index (HI), panicle length (PL), filled grains (FG), unfilled grains (UFG), grain number (GN), spikelet fertility (SPF), panicle weight (PW), and thousand-grain weight (TGW). In addition, 18 of these traits were also measured in F3 population, viz., DIF, PH, TN, PTN, CL, SPY, BM, TDM, HI, PL, FG, UFG, GN, SPF, PW, TGW, PDP, and Bulk yield (BY), and 8 traits in F4 population, viz., PH, TN, PTN, SPY, BM, TDM, HI, and TGW, in randomized with three replications.
DNA Extraction
Fresh leaf samples from 1-month old seedlings were collected from 174 F2 plants and parents in Kharif 2015. DNA was extracted using cetyltrimethyl ammonium bromide (CTAB) mini prep method (Doyle and Doyle, 1990). In this method, 400 μl of CTAB was added to small pieces of leaf in a mini prep plate for grinding. Four hundred microliters of CTAB was added to it and was mixed as well in a 2 ml microcentrifuge tube. To the leaf sample, equal amounts of chloroform:isoamyl alcohol (24:1) was added in each tube while shaking vigorously until it was dissolved. Then tubes were kept for centrifugation at 15,000 rpm for 15 min, and the supernatant was removed gently into a fresh 1.5 ml microcentrifuge tube. An equal amount of isopropanol was added to this supernatant before it was mixed slowly and kept in a freezer at −20°C for about 10 min to 2 days for DNA pellet formation. Afterward, these tubes were centrifuged at 10,000 rpm for 10 min, and the supernatant was removed in this step very slowly to retain the pellet in the tube. Then, 100 μl of 70% of ethanol was added to the pellet and kept for centrifugation at 8,000 rpm for 5 min for pellet cleaning. This step was repeated again to get a clear pellet of DNA before this DNA pellet was dissolved in 100 μl of 1× TE buffer or distilled water for further use.
Genotyping
Parental polymorphism between 166s and 14s using 830 simple sequence repeats (SSRs) was conducted. Out of this, only 79 showed polymorphism. F2 population of 174 plants was screened using these 79 primers of which only 64 were clearly segregated in the population. The remaining 15 primers only showed 14s kind of bands. Polymerase chain reaction (PCR) was carried out using 10 μl reaction mixture containing 3 μl of DNA sample (50 ng), 3.8 μl of millipore water, 0.1 μl of dNTPs, 1.2 μl of 25 mM Mgcl2, 1 μl of 10× PCR buffer with Mg, 0.8 μl of SSR primer, and 0.1 μl of taq polymerase in each PCR plate. PCR was conducted with the initial denaturation step maintained for 5 min at 95°C. It was also conducted for each cycle with a denaturation temperature of 95°C for 30 s, annealing temperature of 55°C for 30 s, and an extension temperature of 72°C for 30 s which was maintained for up to 35 cycles before a final extension of up to 7 min at 72°C. The final PCR product after 35 cycles was maintained at a temperature of 10°C before collecting from PCR. For agarose gel electrophoresis, 3% gels were prepared with wells to load the DNA samples, and electrophoresis was carried out at 180 volts in 0.5× Tris/Borate/EDTA (TBE) buffer. The band pattern was documented in UV-light in the gel documentation unit. Gel scoring of samples along with parents was conducted by noting ‘A’ (P1) for homozygous 166s allele, ‘B’ (P2) for homozygous 14s allele, and ‘H’(P1P2) for heterozygous, i.e., presence of both the alleles.
Statistical Analyses and Quantitative Trait Loci Mapping
Analysis of variance (ANOVA) was performed using the statistical tool for agricultural research (STAR v2.0.1) software, and association between the traits was estimated with plant breeding tools (PB tools) (Ver. 1.41) using Pearson’s product-moment correlation method at the significant levels of *p = 0.05–0.001 and **p ≥ 0.001. QTL mapping involves the construction of a linkage map and QTL analysis. Inclusive component interval mapping (ICIM) QTL mapping (IciMapping v4.1) integrated software (Wang, 20092) was used for both linkage mapping and QTL analysis. Single marker analysis, interval mapping, and composite interval mapping were performed using this software using F2 genotypic data and F2, F3 (F2:3), and F4 (F2:4) phenotypic data for QTL mapping. Further genotypic dissection within a major QTL region for TGW was carried out in the extreme phenotypes using 5 low TGW (LTGW) and 5 high TGW (HTGW) F2 lines with SSR markers within the detected QTL region. The co-segregating markers were then used to genotype 10 LTGW and 10 HGTW F4 lines.
Results
Phenotyping F2, F3, and F4 Populations
Yield-related traits of populations were evaluated in Kharif (wet season) 2015, Rabi (dry season) 2016, and Rabi (dry season) 2018 for F2, F3, and F4, respectively, using standard evaluation system (SES) of Standard Evaluation System for Rice [SES] (2014). The yield-related traits in F2 (19 traits), F2:3 (18 traits), and in F2:4 (8 traits) were measured and significant differences among the individuals or lines were observed in the three populations for each trait. The SPY of lines C212, C2124, C2128, C2143, and C2 162 showed a positively significant difference with Swarna and P2 in F3 (Supplementary Tables 2, 3) and showed higher yield in F3 and F4. Supplementary Table 2 shows the cumulative number of lines that are significantly different than parents for each trait. The details of significantly different traits in individual lines as compared to parents are given in Supplementary Table 3. The descriptive statistical data for all these yield-related traits of F2, F3, and F4 populations is given in Table 1, and frequency distribution is shown in Supplementary Figures 1–3, respectively. Box plots for 8 traits in F2, F3, and F4 and 16 traits in F2 and F3 are shown in Supplementary Figures 4, 5, respectively.
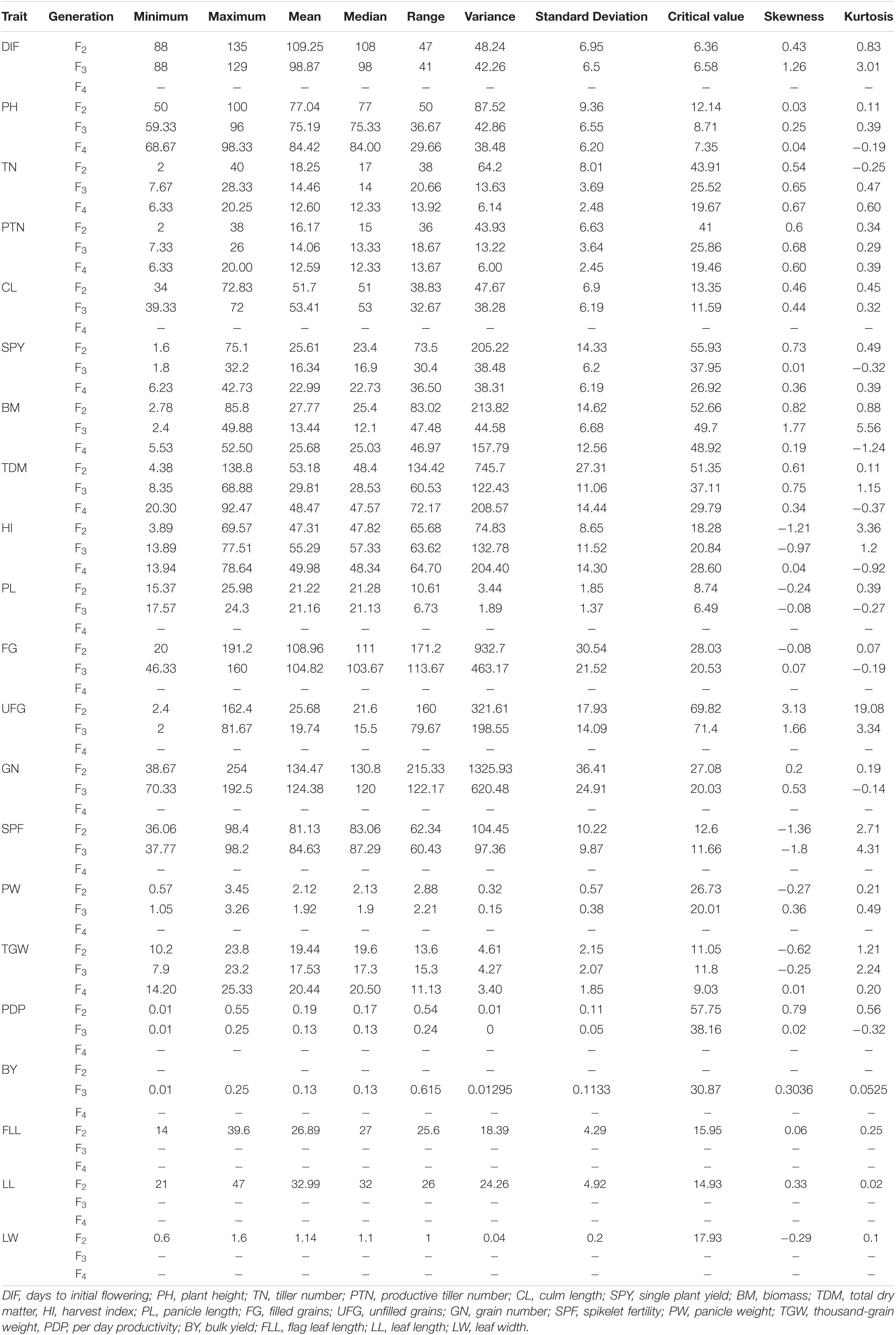
Table 1. Descriptive statistics for the yield-related traits in F2, F3, and F4 population of 166s × 14s.
Trait Correlation
Correlation analysis among all yield-related traits in F2 and F3 was evaluated using Pearson’s product-moment correlation and probability values of *p = 0.05–0.001 as significant and **p ≥ 0.001 as a highly significant correlation between traits. Results are shown in Table 2. Some traits consistently showed significant association in F2, F2:3, and F2:4. The traits which showed highly significant positive correlations in F2 were as follows: FLL with PH, CL, SPY, BM, HI, PL, FG, UFG, GN, PW, and PDP; LL with PH, TN, PTN, CL, SPY, BM, TDM, HI, PL, FG, UFG, GN, PW, TGW, PDP, and FLL; and LW with PH, TN, PTN, CL, SPY, BM, TDM, PL, FG, UFG, GN, PW, PDP, FLL, LL, and LW. A highly significant negative association was observed for FLL, LL, and LW with DIF.
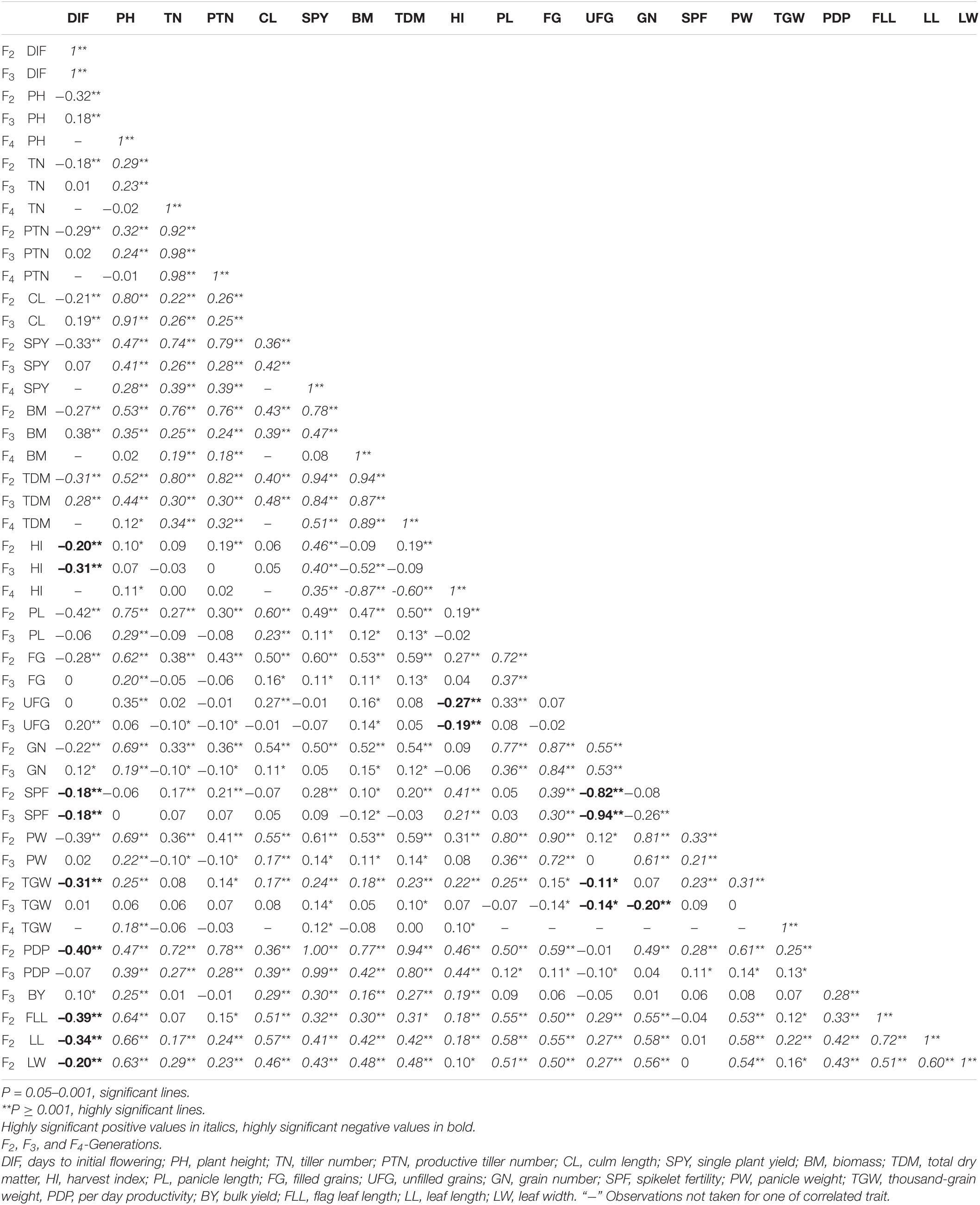
Table 2. Correlation coefficients among yield-related traits of F2, F3, and F4 population of 166s × 14s.
In F3, highly significant positive correlations were observed for BY with PH, CL, SPY, BM, TDM, HI, and PDP. PH, TN, PTN, CL, BM, TDM, HI, and PDP showed significant and positive association to single plant yield, and significant negative association was observed for TGW, SPF, and HI with UFG in both F2 and F2:3. In F2:4, a highly significant positive association was observed between PTN and TN; SPY with PH, TN, and PTN; BM and TDM with both TN and PTN; TDM with SPY and BM; HI with SPY; and TGW with PH. There was also a highly significant negative association of HI with BM and TDM. A significant positive association was identified for PH with TDM and HI and TGW with SPY.
Traits PH, TN, PTN, TDM, and HI showed a highly significant positive association with single plant yield in all three generations of F2, F3, and F4. TGW showed a highly significant association with SPY in F2, and a significant association was observed between TGW and SPY in F3 and F4. The traits PH, TN, PTN, and TDM consistently showed a highly significant positive association to single plant yield in all three generations. Highly significant positive correlations were observed for the traits SPY with PH, TN, PTN, CL, BM, TDM, HI, and PDP in F2 and F2:3. The traits which showed a highly significant negative correlation in F2 and F2:3 were HI with DIF and UFG; SPF with DIF and UFG. A significant negative association between TGW with UFG was also observed, in addition to the highly significant negative association in both F2:3 and F2:4 between traits HI with BM. A significant positive association was observed between traits TGW with SPY.
Genotyping
The F2 population consisting of 174 lines was genotyped using 79 polymorphic markers. Among these polymorphic SSR markers, segregation distortion was observed for nearly 20 markers. In this study, heterozygous bands ranged from 14.3 (RM1189) to 69.5% (RM430) in the whole population. The 166s (female P1) parental type bands ranged between 1.1% (RM202) and 47.1% (RM1189), while the 14s (male P2) parent type bands ranged from 9.7 (RM430) to 89% (RM3708). The maximum percentage of missing/null alleles was observed for the marker RM4996 (35.6%), followed by RM16649 with 28.1%, and RM1189 with 25.8%. The percentage alleles similar to 166s ranged from 1.15 to 47.13% with an average of 20.89%, while allelic similarity to 14s in the population was 9.77 to 58.62% with an average of 25.74%. Heterozygous bands in the F2 population ranged from 14.37 to 69.54% with an average of 43.28%, and non-amplified/missing/null alleles were 0.57–35.63% (Supplementary Table 4). The parental lines of the mapping population were part of the set of CSSLs. 166s had 12.1% and 14s had 17.2% chromosomal segments from O. nivara based on genotyping using 111 SSR markers and 75.8 and 77.8% of Swarna alleles, respectively, with remaining heterozygous or unamplified bands. These BC2F8 parental lines are fixed sib lines, which explain the similarity between them.
Quantitative Trait Loci Mapping
F2 linkage map was constructed using ICIM v4.1 software. There were 33 QTLs mapped using single marker analysis in F2, F2:3, and F2:4 (Table 3). Eleven QTLs were mapped in F2 with phenotypic variance (PV) between 5.17 and 9.71% with a maximum logarithm of odds (LOD) value of 5.04. Thousand-grain weight QTL showed the maximum LOD. In F2:3, 17 QTLs were identified with PV between 4.37 and 12.49% with a maximum LOD value of 3.85. Four QTLs were mapped in F2:4 with PV from 6.52 to 14.38%. The maximum phenotypic variance explained (PVE) was shown by QTL for thousand-grain weight or plant height in each generation. In F2, using inclusive composite interval, mapping 21 QTLs were identified on chromosomes 1, 2, 3, 4, 5, 6, 7, 8, 9, and 11 for 19 yield-related traits, with PV ranging from 4.03 to 12.54%. In F2:3, 30 QTLs were identified on chromosomes 1, 3, 4, 5, 6, 7, 8, 10, and 12 for 18 yield-related traits, with PV ranging from 1.32 to 13.01%. In F2:4, 17 QTLs were identified on chromosomes 2, 3, 5, 6, 7, 8, and 12 for 8 yield-related traits, with PV ranging from 2.28 to 15.19%. In F2, F2:3, and F2:4 (Figure 2) PV of QTLs ranged from 1.32 to 15.19%.
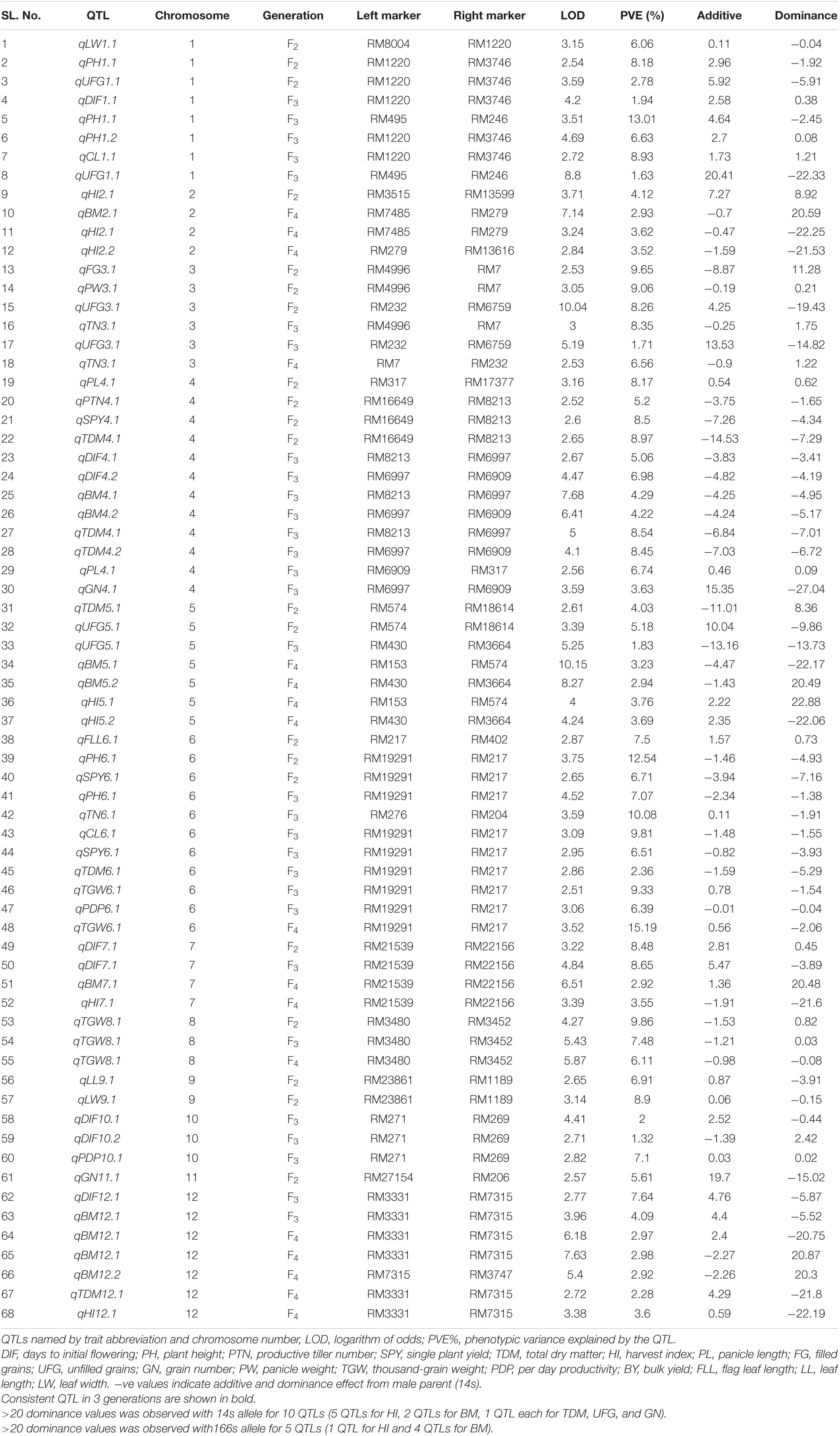
Table 3. Additive and dominance effects of quantitative trait loci (QTLs) identified for yield-related traits in F2, F2:3, and F2:4 populations of 166s × 14s using ICIM.
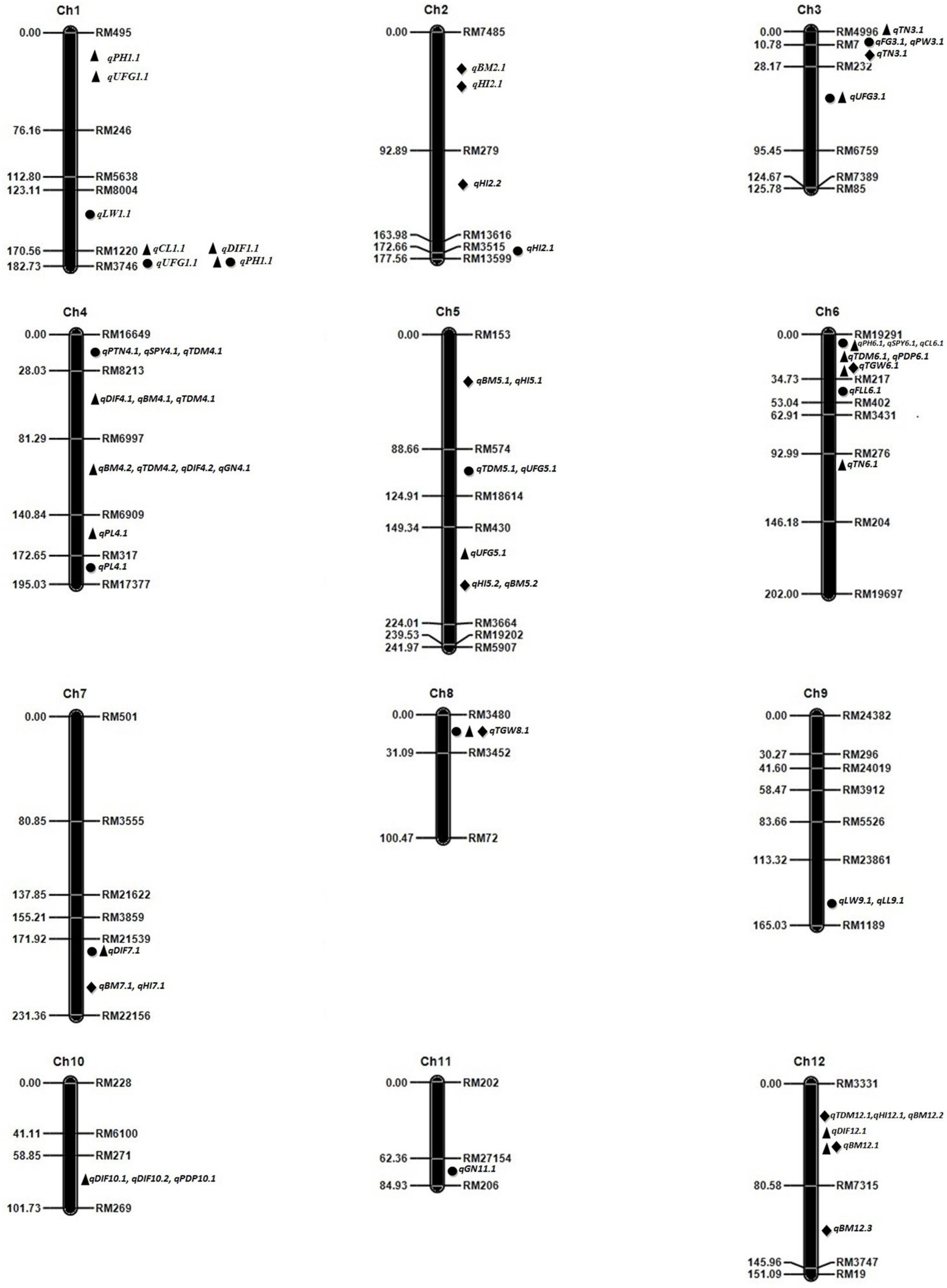
Figure 2. Molecular linkage map of 12 chromosomes with position of Quantitative Train Loci (QTLs) for yield-related traits in F2:3:4 generations detected using ICIM. QTLs named by trait abbreviation and chromosome number, ● – F2 generation, ▲ – F3 generation, ◆ – F4 generation. DIF, days to initial flowering; PH, plant height; PTN, productive tiller number; SPY, single plant yield; TDM, total dry matter; HI, harvest index; PL, panicle length; FG, filled grains; UFG, unfilled grains; GN, grain number; PW, panicle weight; TGW, thousand-grain weight; PDP, per day productivity; BY, bulk yield; FLL, flag leaf length; LL, leaf length; LW, leaf width.
Pleiotropic Region for Quantitative Trait Loci
Many QTLs were clustered in the same region. There were six such regions found on chromosomes 1, 3, 4, 5, 6, and 9 in F2 mapping population, on chromosome 1, 4, 6, 10, and 12 in F2:3 mapping, and on chromosome 2, 5, 7, and 12 in F2:4 mapping population. In F2, three loci with the same marker interval responsible for multiple traits of yield QTLs were qPTN4.1, qSPY4.1, and qTDM4.1 for productive tiller number, single plant yield, total dry matter mapped at RM16649- RM8213 on chromosome 4, and two QTLs qPH1.1, qUFG1.1 identified in the region between RM1220 and RM3746. Two QTLs for filled grain qFG3.1 and panicle weight qPW3.1 mapped between RM4996 and RM7, and two QTLs qUFG5.1 and qTDM5.1 mapped at interval RM574- RM18614. qPH6.1 and qSPY6.1 were also identified between the same locus between RM19291 and RM217 on chromosome 6. Two QTLs, qLL9.1 and qLW9.1, for leaf length and leaf width were located between RM23861 and RM1189 on chromosome 9.
In F2:3, the QTLs for qPH6.1, qCL6.1, qSPY6.1, qTDM6.1, qTGW6.1, and qPDP6.1 are located at RM19291-RM217 marker interval were detected. Four QTLs, qDIF4.2, qBM4.2, qTDM4.2, and qGN4.1, were located between RM6997 and RM6909. Three QTLs, qDIF1.1, qPH1.2, and qCL1.1, were located in the same marker interval RM1220 - RM3746. QTLs qDIF4.1, qBM4.1, and qTDM4.1 were located in the same locus between RM8213 and RM6997, while the locus between RM271 and RM269 harbored qDIF10.1, qDIF10.2, and qPDP10.1 QTLs. Two QTLs for qPH1.1 and qUFG1.1 between RM495-RM246 and qBM12.1 and qDIF12.1 were located in the same marker interval of RM3331-RM7315.
In F2:4, the QTLs for biomass and harvest index were colocated at marker interval regions of RM7485-RM279, RM153-RM574, and RM21539-RM22156, RM3331-RM7315 on chromosomes 2, 5, 5, 7, and 12, respectively. Harvest index (HI) QTL was associated with the last cluster on chromosome 12. The regions strongly associated with more than one trait in both F2, F2:3 include the region between RM19291 and RM217 which showed a cluster of QTLs for PH and SPY in F2 and PH, CL, SPY, TDM, TGW, and PDP in F3. The other region, RM1220-RM3746, showed a cluster of QTLs for PH and UFG in F2 and DIF, PH, and CL in F2:3.
Consistent Quantitative Trait Loci Across Generations
In all three populations, the QTL qTGW8.1 for thousand-grain weight was consistently mapped at the same marker interval RM3480- RM3452 on chromosome 8 with LOD ranging from 4.2 to 5.8 and PV ranging from 6.1 to 9.8% (Figure 3). QTLs detected in only F2 and F2:3 were for PH and SPY between RM19291 and RM217 on chromosome 6. Three QTLs, qDIF7.1, qBM12.1, and qTGW6.1, were detected in only F2:3 and F2:4. qTN3.1 was mapped in F2:3 and F2:4, but with different marker intervals. QTL qTGW8.1 was selected for further genomic dissection as it was consistently identified in F2, F3, and F4 generations at the same 2.6 Mb region. SSR markers located between these flanking markers were selected to enrich this 2.6 Mb region and used for further genotyping. Five lines each for high and low TGW were selected in F2 based on mean TGW and if the same high or low TGW trait was present in at least two of the three generations. First, F2 leaf DNA samples of five lines, each with high and low TGW, were separately used for genotyping with eight SSR markers within QTL. These samples showed clear polymorphism between high and low TGW lines. Marker trait association was detected between TGW and the locus RM 502- RM3480 with a PVE of 47% at LOD 1.49. Later, DNA samples of 10 high and 10 low TGW F4 lines were taken and genotyped using two co-segregating markers for validation (Figure 3). The high (20.1 to 25.3 g) and low (15.2 to 18.1 g) TGW lines were significantly different from both parents 166s (17.2 g) and 14s (21.6 g) as well as from Swarna (14.2 g). Genotyping of contrasting lines with 8 SSRs showed that two markers RM23407 and RM23447 showed clear polymorphism between high and low TGW lines in F2. However, only RM23447 showed polymorphism between extreme TGW phenotypes of F4. In F2, among five low TGW lines, the percentage of 166s type alleles was higher than 14s alleles, while the five high TGW lines showed more of 14s type alleles than 166s type. Considering all alleles of the 5 high TGW lines, 50% alleles were of 14s type in C2 117, and 37.5% alleles were of 14s type in the other four lines (C2 103, C2 126, C2 128, and C2 152). Three lines (C2 103, C2 126, and C2 128) had 25% heterozygous alleles and two lines C2 117, and C2 152 had only 12.5% heterozygous alleles. The statistically significant lines identified in our study, compared to both the parents and their common cultivar parent Swarna, were further used for small-scale fine mapping of qTGW8.1 to detect causative genes for grain weight improvement (Figure 3). Five lines, each with high and low TGW, were selected in F2 based on their extreme placement in the frequency distribution curve and if the same high or low TGW trait were present in the same extremes. Likewise, at least two of the three generations were genotyped using SSR markers within the QTL region. Trait marker association using interval mapping detected RM502-RM3480 with PVE of 47% at 1.49 LOD, but no trait association was detected with RM23447 even though 2 groups showed clear co-segregation. Further genotyping in F4 identified two co-segregating markers, RM23407 and RM23447, covering a 602.4 kbp region {25148785bp to 25751225bp [QTARO database (affrc.go.jp)]} within qTGW8.1.
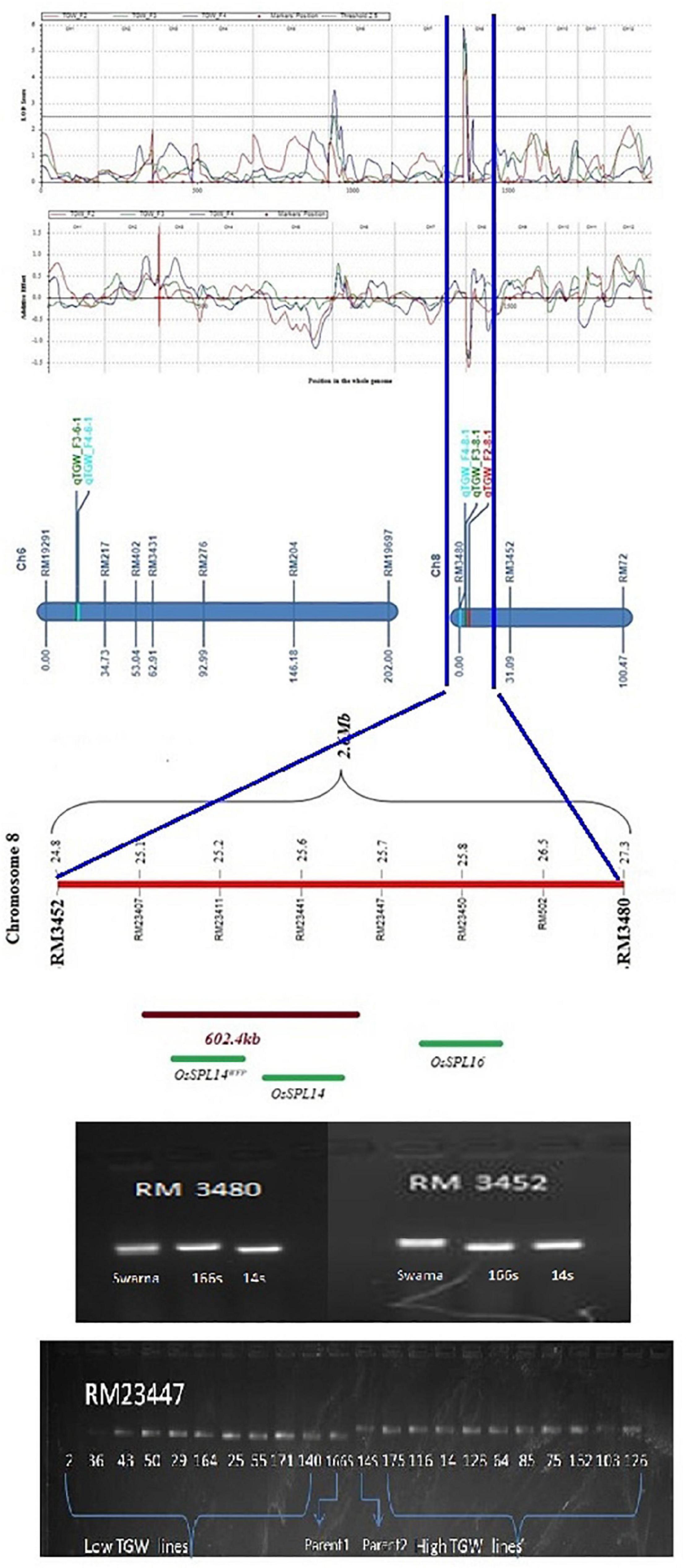
Figure 3. Genetic dissection of qTGW8.1 QTL from 2.6 Mb region between RM3480-RM3452 and 602.4 Kb region between RM23407 and RM23447 based on genotypic segregation in high and low TGW phenotypes in F4.
Quantitative Trait Loci Clusters Detected
In this study, among all QTLs detected in F2, F2:3, and F2:4, two specific marker regions were strongly associated with more than one trait. RM19291-RM217 had a cluster of QTLs for PH, SPY in F2, and PH, CL, SPY, TDM, TGW, and PDP in F3. The other region of RM1220- RM3746 had a cluster of PH, UFG in F2, and DIF, PH, and CL in F2:3. In addition, the QTLs present in each region were correlated except PH with UFG in F2:3 mapping. In F2:4, one specific marker interval, RM3331- RM7315, had a cluster of QTLs for BM (two QTLs), TDM, and HI on chromosome 12. In addition, the region at marker intervals of RM153- RM574 and RM430- RM3664 had a cluster of QTLs for BM and HI on chromosome 5. Lastly, the same QTL cluster for BM and HI were identified on chromosomes 2 and 7 at the regions RM7485-RM379 and RM21539-RM22156, respectively.
Discussion
Phenotyping the mapping population for 3 generations showed a significant positive association of yield contributing traits PH, TN, PTN, and TDM to single plant yield. Consistent character association revealed the importance of tiller number per plant, productive tillers per plant, and thousand-grain weight as selection criteria for effective yield improvement. Our study showed that F2 population is more powerful for detecting QTLs of additive effect and can also be used to estimate the degree of dominance for detected QTLs. All the QTLs mapped in F4 were observed with low additive effects from both the parent alleles. A high dominant effect from male parent alleles for the 9 QTLs qBM5.1, qBM12.1, qTDM12.1, qHI2.1, qHI2.2, qHI5.2, qHI7.1, qHI2.1, and qTGW6.1 was also observed. Dominance effects were high for the QTLs mainly because of the superiority of heterotic loci identified with low phenotypic variances. QTLs with the low dominant effects showed high phenotypic variances except for the QTLs FG and UFG in F2. About 38.1% in F2 and 30% in F2:3 QTLs explained positive dominance effect, and 61.9% QTLs in F2 and 70% in F2:3 explained negative dominance values. The QTL qTGW6.1 showed additive effects from female parents in F3 (0.78) and F4 (0.56), and also showed dominance effects from male parents in both F3 (−1.54) and F4 (−2.06). qTGW8.1 showed a higher additive effect (O. nivara) in F2 than in F3 and F4, along with a small dominance effect (0.82) in F2. The high dominance effects were identified for the QTLs in F4 (82%), followed by F2 (14.2%), and F3 (13.2%).
In this study, significant QTLs were identified in F2, F2:3, and F2:4, with 9.0–15.19% PV for several yield-related traits. Trait enhancing allele in major QTLs qPH1.1, qTGW6.1, and qTGW8.1 and minor QTLs qGN4.1 and qTDM5.1 was from O. nivara. Swamy et al. (2011, 2012) identified 40% of O. nivara alleles were trait enhancing in QTLs in Swarna/O. nivara BC2F2 populations. Sarla (2014) reported that two QTLs yld9.1 (yield) and nfg9.1 (number of filled grains) were from O. nivara in BIL 248S (DRRDhan40), derived from the same cross. Surapaneni et al. (2017) identified 15 QTLs in a 94 BILs mapping population using 111 SSRs and, of these, 26% QTLs had trait enhancing alleles from O. nivara. For the yield QTLs qSPY4.1 and qSPY6.1, trait enhancing allele was from Swarna. The common QTLs qPH1.1 and qTGW8.1 at RM1220-RM3746 and RM3480-RM3452, respectively, in F2 and F2:3 shared the same locus. In these QTLs, PV value was reduced (qPH1.1-6.63% and qTGW8.1-7.48%) and LOD values increased (qPH1.1-4.69 and qTGW8.1-5.43) in F2:3 compared to F2. This reduction of the phenotypic variance of the traits in advanced generations may be due to a reduction in heterozygosity and stabilization of introgression lines. Another common QTL for single plant yield qSPY6.1 at the locus between RM19291 and RM217 in F2 and F2:3 showed no significant change in LOD (2.65 in F2, 2.95 in F3) and PV (6.71% in F2, 6.51% in F3) values across the generations.
Major Yield Quantitative Trait Loci Identified in This Study
Among the eight major QTLs, six qPH1.1, qPH6.1, qTGW6.1, qFG3.1, qTN6.1, and qCL6.1 were previously reported in the population derived from cultivated rice varieties for the same trait. The other 2 QTLs, qTGW8.1 and qPW3.1, were reported in wild introgression lines in different genomic regions. qPH1.1 was identified with the same flanking marker, RM246, by Zhang et al. (2010). qPH1.1 was also reported previously from O. nivara (Swamy et al., 2014; Surapaneni et al., 2017; Haritha et al., 2018; Balakrishnan et al., 2020). qTGW6.1 was reported previously by Zhu et al. (2019) in the O. sativa recombinant inbred line (RIL) population [Teqing/IRBB lines (TI), Zhenshan97/Milyang46 (ZM) and Xieqingzao/Milyang46 (XM)] within the marker region of our study (Table 4).
The QTL, qSPY4.1, with PV% 8.5 and LOD of 2.6, which was detected in this study along with Xing et al. (2014), Ma et al. (2016), and Haritha et al. (2018), reported grain yield QTLs on chromosome 4. Fan et al. (2019) also reported qGYPP4 in the BIL population derived from Oryza longistaminata. In our study, 8 QTLs for biomass were identified on chromosomes 2, 4, 5, 7, and 12 with LOD values ranging from 3.96 to 10.15 with a maximum PV of 4.29%. Zhang et al. (2017) mapped the BM QTL on chromosome 12 in F2:3 and RILs population. Six QTLs, qHI2.1, qHI2.2, qHI5.1, qHI5.2, qHI7.1, and qHI12.1, were mapped with a maximum LOD of 3.7 and PV of 4.12% for qHI2.1 QTL. In a previous study of Sabouri et al. (2009), they identified qHI2 with 21.35% of phenotypic variance. Grain number of panicle is one of the most important traits to contribute to yield, with qGN4.1 QTL identified as the highest additive effect QTL and maximum LOD value of 3.5. This QTL was previously reported by Xing et al. (2014), Kim et al. (2017), and Deng et al. (2017). Singh et al. (2018) also reported the same qGN4.1 QTL in bi-parental RIL population.
The QTL qFG3.1 was found with 9.6% of phenotypic variance. Feng et al. (2015) and Zhang et al. (2018) reported QTLs for filled grain numbers as qFGP3a and qFGN3, respectively. QTLs for unfilled grains per panicle were identified on chromosomes 1, 3, and 5 with LOD value of 10.0. Five QTLs were observed for UFG on chromosomes 1, 3, and 5 with different flanking markers in both the populations except for qUFG3.1 (Rabiei et al., 2015), with 8.2% of phenotypic variance in F2 population. Two QTLs, qTN3.1 and qTN6.1, for tiller number and qPTN4.1 for productive tiller number, were identified. Lim et al. (2014) also reported the same QTL on chromosome 6 with LOD of 3.79. In the present study, qPL4.1 was identified with different flanking markers in F2 and F2:3 with 8.1% of the phenotypic variance on chromosome 4 with above 2.5 LOD value. It was mapped by Lim et al. (2014) and Kim et al. (2017) in indica-japonica recombinant inbred line population. Xing et al. (2014) and Zhang et al. (2018) reported QTL for productive tiller number on chromosome 4. Zhang et al. (2018) reported qPL4 QTL in the background of IL population derived from cross between ZGX1 (high-quality indica elite variety) × IR75862 (high iron and zinc japonica variety).
Among the QTLs detected, the plant height QTL, qPH1.1, was identified in F2:3 with 13% of phenotypic variance value and with LOD value of 3.5. The same flanking marker RM246 for plant height QTL was previously reported by Zhang et al. (2010) in F2 population derived from cross between PA64s (indica) × Nipponbare (japonica) and Rabiei et al. (2015) in F2:4 population derived by crossing two indica rice varieties (Sepidrood × Gharib). Sabouri et al. (2009) also identified the same plant height QTL in F2 and F2:3 populations derived by crossing two genetically divergent indica type high-yielding rice varieties. Qiao et al. (2016) reported qPH1.1 and qPH1.2 with 7.01 and 9.05% PV, respectively, in the 198 CSSLs population derived from cross between indica var. 9311 and wild species O. rufipogon as donor parent. QTL qPH1.1, in BILs/ILs population previously derived from Swarna/O. nivara but with different flanking markers in chromosome 1, was reported by Swamy et al. (2014), Surapaneni et al. (2017), Haritha et al. (2018), and Balakrishnan et al. (2020). Another plant height QTL was mapped in F2 population with 3.7 LOD with a phenotypic variance of 12.5% on chromosome 6. qPH6.1 QTL was previously reported in the F2 mapping population (Zhang et al., 2010) and in F2:7 recombinant inbred line population derived from a cross between Xiaobaijingzi (upland rice) and Kongyu 131 (Xing et al., 2014). Lim et al. (2014) also identified plant height QTL on chromosome 6 in F2:7 RIL population by crossing indica × japonica (Milyang23/SNUSG1) using the QGene 4.3.10 software.
qFLL6.1 was identified with phenotypic variance of 7.4% and LOD value of 2.8 in the present study with the flanking region between RM217 and RM402. RM402 was detected in the same region in the study reported by Shen et al. (2011) who identified qFLL6.1 and qFLL6.2 in the 4.2 Mb region between flanking markers RM4923 and RM402 on short arm of chromosome 6 in NILs. Shao et al. (2009) reported flag leaf length QTL on chromosome 6. Matsubara et al. (2016) and Yang et al. (2018) reported qFLL QTL on chromosome 6 in RIL population and DH population, respectively. Leaf length QTL qLL9.1 was identified with phenotypic variance of 6.9% with LOD of 2.6, while two QTLs for leaf width, qLW9.1, qLW1.1, were identified with 11 and 5.7% phenotypic variance on chromosome 9 and 1, respectively.
Seven QTLs for days to initial flowering were identified on chromosomes 1, 4, 7, 10, and 12. The QTL with the highest PV of 8.65% was observed with LOD value of 4.84 on chromosome 7. Mohammadi et al. (2013) reported QTLs for number of days to flowering on chromosomes 4 and 10 in the F2 population of Sadri/FL478 cross under saline field conditions. The major effect heading date QTLs was previously identified in chromosome 7 by Li et al. (2003) in IR64/Azucena doubled-haploid population. Yano et al. (2000) and Xue et al. (2008) identified Hd1 on chromosome 6 and Ghd7 on chromosome 7. Salgotra et al. (2015) reported Ghd7 gene with associated traits of grains per panicle and plant height along with heading date. These previous reports also confirmed detection of the major QTLs in the same chromosomal regions as identified in our study.
Consistent Quantitative Trait Loci Detected in This Study
qTGW8.1 is a novel QTL consistently identified within the flanking marker region between RM3480 and RM3452 in three populations. GW8.1 was previously mapped from another wild species O. rufipogon (Tian et al., 2006; Kang et al., 2018), and QTL for panicle weight was also reported from O. nivara on chromosome 3 but with different flanking markers. The number of traits studied was different across the generations and only 8 traits were phenotyped in all three generations. Therefore, we could check the consistency of QTLs of only these 8 traits. Further, we checked the consistency for the selected QTLs above threshold level after 1,000 permutations so QTLs below the threshold level, even in one generation, were not considered consistent even though the QTLs were mapped. As the QTL mapping was carried out in different generations, reduction in segregation, heterozygosity, change in context (background genome), and further stabilization of lines and environmental factors may also have contributed to identification of only few consistent QTLs. Stable QTLs which are consistently detected in different generations are useful in marker assisted selection (MAS) for crop improvement (Guo et al., 2005; Su et al., 2010).
Quantitative Trait Loci for Grain Weight and Further Genetic Dissection
Of all the major QTLs detected, qTGW8.1 was mapped in all three generations of F2, F2:3, and F2:4 at the same marker interval of RM3480-RM3452, covering a 2.6 Mb region with significant PVE ranging from 6.1 to 9.8%. Previous studies showed qTGW QTL in the background of O. nivara parent on other chromosomes 1, 2, 4, and 5 by Kaladhar et al. (2008), on chromosomes 1, 2, 3, and 4 by Swamy et al. (2014), and on chromosomes 1, 4, and 5 by Haritha et al. (2018). qTGW8.1, identified in the present study, is a novel QTL with O. nivara contributing to the additive effect. This region is already reported to harbor genes, viz., Wealthy Farmer’s Panicle (WFP) encoding OsSPL14WFP (Miura et al., 2010) and Ideal Plant Architecture (IPA1) encoding OsSPL14 (Jiao et al., 2010) and located 875 kbp upstream of already reported QTL qGW8, with gene Os08g0531600 (GW8/OsSPL16) (Wang et al., 2012). The identified QTL in this study could be a novel allele of these reported genes from O. nivara or a novel gene/QTL colocated in this region since there is an overlap with three known genes. However, this requires further genetic dissection and confirmation. The QTL trait enhancing allele is from the male parent 14s. Gel pictures of these markers showed a lower band size at 14s compared to Swarna which is indicative of the presence of a novel allele with deletion at the locus RM3480 triggered by recombination or any unknown factors contributed by O. nivara or Swarna genome.
Three meta-QTLs for yield on chromosome 8 (MQTL 8.1, 8.2, 8.3) were detected for various yield and grain-related traits. MQTL8.2 (326 kb) was identified as a recombination hot spot suitable for fine mapping yield-related traits including grain weight (Swamy and Sarla, 2011). It is interesting to note that the only meta-QTL for TGW on chromosome 8 (MQTL-GW4 S3680-RM3689 at 18.25–19.33 Mb), reported recently (Wang et al., 2021), is proximal to our qTGW8.1. The identified QTL in this study could be a novel allele of these reported genes from O. nivara or a novel gene colocated in this region. As the trait enhancing QTL allele is from male parent 14s and the gel pictures of these markers showed a lower band size at 14s compared to Swarna, it indicates the presence of a novel allele with possible deletions in 14s at loci RM3480, RM3452, and RM23447 compared to Swarna and can be confirmed with sequencing.
Thousand-grain weight QTL, detected in an advanced back cross between Nipponbare as recipient and Xihui 18 as the donor parent, was linked with RM6845. In addition, it is 169.5 kb away from RM3480, the flanking marker of qTGW8.1 in the present study. qGL-8, a novel QTL for grain length, was identified at 22.70–22.05 Mb region at chromosome 8 using a set of recombinant inbred sister lines and high-yielding hybrid rice variety Nei2You No. 6 (Kang et al., 2021). Three QTLs for grain size-related traits, viz., qGL8 (RM408–RM3702), qLWR8, and qGT8 (RM3845–RM6948), were identified in chromosome 8 in F2 and F2:3 populations derived from an introgression line ‘IL188’ of Nipponbare/Oryza minuta cross (Feng et al., 2021). Thus, this consistent effect region on chromosome 8 seems important for several grain traits and is worthy of further fine mapping, cloning, and functional analysis, similar to that shown in a recent study using CSSLs (Wang et al., 2021). Most of the previous reports detected grain weight QTL in different genomic region compared to qTGW8.1 of our study which is located between 24867542 to 27481361bp region [QTARO database (affrc.go.jp)] at marker interval of RM3480-RM3452, covering a 2.6 Mb region in the long arm of chromosome 8.
Another TGW QTL, qTGW6.1, was mapped in both F2:3 and F2;4 with LOD value of 2.51–3.52 (F2:4) and PV of 9.33–15.19% (F2:4) in the present study. Ishimaru (2003) reported that the qTGW6.1 in backcross inbred lines was derived from cross between japonica (Nipponbare)/indica (Kasalath) after over 3 years of testing. qTGW6.1, at marker interval of RM402- RM5963, was detected in the IL population of ZGX1 × IR75862 (Zhang et al., 2018). One of the flanking markers of this QTL RM3452 was reported to be linked with grain width in the F2 population of Kasalath × BG23 (Segami et al., 2016) and was also associated with leaf traits (Yang et al., 2018). Zhu et al. (2019) also identified thousand-grain weight QTLs on chromosomes 6 and 8 in three recombinant inbred line populations of Teqing/IRBB lines (TI), Zhenshan97/Milyang46 (ZM), and Xieqingzao/Milyang46 (XM).
Quantitative Trait Loci Clusters Detected
In this study, several QTL clusters were detected, and some of the clusters contained more than four QTL, signifying a heavily populated region of QTL on the chromosome. Xie et al. (2008) detected a cluster of yield-related QTLs on chromosome 9, in BC3F4 population from an interspecific cross of Hwaseong/O. rufipogon (IRGC 105491). Jeon et al. (2021) employed map-based cloning, gene sequencing, expression analysis, and transgenic approaches to detect putative genes and the genetic architecture of the cluster. The study using BC4F4 NILs of the same population demonstrated that the cluster is regulated by a single pleiotropic gene (ascorbate peroxidase gene, APX9). Multiple traits mapping in the same region of a chromosome are beneficial to breeders, as QTL clusters allow breeders to focus their efforts on regions of the genome containing the most QTLs of interest. The identification of gene clusters is useful in marker-assisted selection since the markers delineating these regions can be chosen for selecting the traits of interest. Our study demonstrated that favorable alleles from stable wild introgression lines with minimum linkage drag can be introduced to develop high-yielding lines.
Conclusion
Inter-specific crosses are bridging tools to introgress beneficial alleles from wild donor into cultivar background to get improved lines for breeding and cultivation. Alien introgression lines/BILs were used as a crossing material in this study, which is a novel approach to detect QTLs and is further useful for QTL/gene pyramiding. In this study, 21, 30, and 17 QTLs were mapped in F2, F2:3, and F2:4, derived from a two BILs for a total of 20, 18, and 8 yield-related traits, respectively. This breeding strategy helped to converge beneficial alleles from both the parents to produce superior lines. Five lines, C212, C2124, C2128, C2143, and C2162 were significantly higher yielding than Swarna and 14s and carried alleles for qTGW8.1 from O. nivara in either a homozygous or heterozygous condition. The F2, F2:3, and F2:4 QTL mapping helped to identify 8 major QTLs, namely, qPH1.1, qPH6.1, qTGW6.1, qTGW8.1, qTN6.1, qPW3.1, qCL6.1, and qFG3.1 for yield-related traits. qTGW8.1 is found as a potential candidate region of 602.4 kb for genetic dissection. The significantly different lines with major yield QTLs will be utilized in further fine mapping and identification of genes for yield improvement. Populations generated from these BIL/CSSL are useful to detect the candidate gene location for important agronomical traits by further fine mapping. This strategy of intercrossing advanced back cross introgression lines can be employed in other backgrounds with advanced genomics tools for crop improvement programs. The statistically significant lines identified by pair-wise mean comparison with both the parents and their common cultivar parent Swarna is useful in gene discovery for target traits and to mine possibly better alleles from the wild progenitors for further crop improvement.
Data Availability Statement
The original contributions presented in the study are included in the article/Supplementary Material, further inquiries can be directed to the corresponding author/s.
Author Contributions
SN and DB conceptualized, designed, and supervised the experiments, and contributed to the final revision of the manuscript. KB performed the experiments. KA, MS, and VR supported conducting the field experiments. KB and DB analyzed the data and wrote the manuscript with contributions from SN. All authors contributed to the article and approved the submitted version.
Funding
This research was part of Ph.D. work of KB and was conducted in the project (ABR/CI/BT/11) on Mapping quantitative trait loci (QTLs) for yield and related traits using backcross inbred lines from elite × wild crosses of rice (Oryza sativa L.) as part of ICAR-National Professor Project (F.No.: Edn/27/4/NP/2012-HRD) (2013–2020) funded by Indian Council of Agricultural Research, New Delhi, India. The support of P. Sudhakar and A. Krishna Satya, Department of Biotechnology, Acharya Nagarjuna University, Guntur, Andhra Pradesh, India, and Director, ICAR-IIRR, Hyderabad is acknowledged.
Conflict of Interest
The authors declare that the research was conducted in the absence of any commercial or financial relationships that could be construed as a potential conflict of interest.
Publisher’s Note
All claims expressed in this article are solely those of the authors and do not necessarily represent those of their affiliated organizations, or those of the publisher, the editors and the reviewers. Any product that may be evaluated in this article, or claim that may be made by its manufacturer, is not guaranteed or endorsed by the publisher.
Acknowledgments
We thank the reviewers for valuable comments that helped improve the manuscript.
Supplementary Material
The Supplementary Material for this article can be found online at: https://www.frontiersin.org/articles/10.3389/fpls.2022.790221/full#supplementary-material
Footnotes
References
Balakrishnan, D., Subrahmanyam, D., Badri, J., Raju, A. K., Rao, V. Y., Kavitha, B., et al. (2016). Genotype × environment interactions of yield traits in backcross introgression lines derived from Oryza sativa cv. Swarna /Oryza nivara. Front. Plant Sci. 7:1530. doi: 10.3389/fpls.2016.01530
Balakrishnan, D., Surapaneni, M., Yadavalli, V. R., Addanki, K. R., Mesapogu, S., Beerelli, K., et al. (2020). Detecting CSSLs and yield QTLs with additive, epistatic and QTL× environment interaction effects from Oryza sativa × O. nivara IRGC81832 cross. Sci. Rep. 10, 1–17.
Bhatia, D., Wing, R. A., Yu, Y., Chougule, K., Kudrna, D., Lee, S., et al. (2018). Genotyping by sequencing of rice interspecific backcross inbred lines identifies QTLs for grain weight and grain length. Euphytica 214:41.
Biswas, P. S., Khatun, H., Das, N., Sarker, M. M., and Anisuzzaman, M. (2017). Mapping and validation of QTLs for cold tolerance at seedling stage in rice from an indica cultivar Habiganj boro VI (Hbj.BVI). 3 Biotech 7:359. doi: 10.1007/s13205-017-0993-1
Brar, D. S., and Singh, K. (2011). “Oryza,” in Wild Crop Relatives: Genomic and Breeding Resources: Cereals, ed. C. Kole (Berlin: Springer), 321–365.
Brondani, C., Rangel, P. H. N., Brondani, R. P. V., and Ferreira, M. E. (2002). QTL mapping and introgression of yield-related traits from Oryza glumaepatula to cultivated rice (Oryza sativa) using microsatellite markers. Theor. Appl. Genet. 104, 1192–1203. doi: 10.1007/s00122-002-0869-5
Deng, Z., Cui, Y., Han, Q., Fang, W., Li, J., and Tian, J. (2017). Discovery of consistent QTLs of wheat spike-related traits under nitrogen treatment at different development stages. Front. Plant Sci. 8:2120. doi: 10.3389/fpls.2017.02120
Fan, F., Long, W., Liu, M., Yuan, H., Pan, G., Li, N., et al. (2019). Quantitative trait locus mapping of the combining ability for yield-related traits in wild rice Oryza longistaminata. J. Agric. Food Chem. 67, 8766–8772. doi: 10.1021/acs.jafc.9b02224
FAO, IFAD, UNICEF, WFP, and WHO (2017). The State of Food Security and Nutrition in the World 2017. Building Resilience for Peace and Food Security. Rome: Food and Agriculture Organization.
Feng, B., Chen, K., Cui, Y., Wu, Z., Zheng, T., Zhu, Y., et al. (2018). Genetic dissection and simultaneous improvement of drought and low nitrogen tolerances by designed QTL pyramiding in rice. Front Plant Sci. 9:306. doi: 10.3389/fpls.2018.00306
Feng, Y., Yuan, X., Wang, Y., Yang, Y., Zhang, M., Yu, H., et al. (2021). Validation of a QTL for grain size and weight using an introgression line from a cross between Oryza sativa and Oryza minuta. Rice 14, 1–12.
Feng, Y., Zhai, R.-R., Lin, Z. C., Cao, L. Y., Wei, X. H., and Cheng, S. H. (2015). Quantitative trait locus analysis for rice yield traits under two nitrogen levels. Rice Sci. 22, 108-115.
Fisher, K. S., Barton, J., Khush, G. S., Leung, H., and Cantrell, R. (2000). Genomics and agriculture. Collaborations in rice. Science 290, 279–280. doi: 10.1126/science.290.5490.279
Gaikwad, K. B., Singh, N., Kaur, P., Rani, S., Babu, P. H., and Singh, K. (2021). Deployment of wild relatives for genetic improvement in rice (Oryza sativa). Plant Breed. 140, 23–52. doi: 10.1111/pbr.12875
Ganeshan, P., Ajay, J., Parmar, B., Rao, A. R., Sreenu, K., Mishra, P., et al. (2016). Identification of salt tolerant rice lines among interspecific BILs developed by crossing Oryza sativa /O. rufipogon and O. sativa /O. nivara. Austral. J. Crop Sci. 10, 220–228.
Guo, W. Z., Zhang, T. Z., Ding, Y. Z., Zhu, Y. C., Shen, X. L., and Zhu, X. F. (2005). Molecular marker assisted selection and pyramiding of two QTLs for fiber strength in upland cotton. Acta Genet. Sin. 32, 1275–1285.
Haritha, G., Swamy, B. P. M., Naik, M. L., Jyothi, B., Divya, B., and Sarla, N. (2018). Yield traits and associated marker segregation in elite introgression lines derived from O. sativa ×O. nivara. Rice Sci. 25, 19–31. doi: 10.1016/j.rsci.2017.11.001
Ishimaru, K. (2003). Identification of a locus increasing rice yield and physiological analysis of its function. Plant Physiol. 133, 1083–1090. doi: 10.1104/pp.103.027607
Jeon, Y. A., Kang, Y. J., Shim, K. C., Lee, H. S., Xin, L., Kang, J. W., et al. (2018). Genetic analysis and mapping of genes for culm length and internode diameter in progeny from an interspecific cross in rice. Plant Breed. Biotechnol. 6, 140–146. doi: 10.9787/pbb.2018.6.2.140
Jeon, Y. A., Lee, H. S., Kim, S. H., Shim, K. C., Kang, J. W., Kim, H. J., et al. (2021). Natural variation in rice ascorbate peroxidase gene APX9 is associated with a yield-enhancing QTL cluster. J. Exp. Bot. 72, 4254–4268. doi: 10.1093/jxb/erab155
Jiao, Y., Wang, Y., Xue, D., Wang, J., Yan, M., Liu, G., et al. (2010). Regulation of OsSPL14 by OsmiR156 defines ideal plant architecture in rice. Nat. Genet. 42, 541–544. doi: 10.1038/ng.591
Juneja, S., Das, A., Joshi, S., Sharma, S., Vikal, Y., Patra, B., et al. (2006). Oryza nivara (Sharma et Shastry) the progenitor of O. sativa (L.) subspecies indica harbours rich genetic diversity as measured by SSR markers. Curr. Sci. 91, 1079–1085.
Kaladhar, K., Swamy, B. P. M., Babu, A. P., Reddy, C. S., and Sarla, N. (2008). Mapping quantitative trait loci for yield traits in BC2F2 population derived from Swarna x O. nivara cross. Rice Genet. Newslett. 24, 34–36.
Kang, Y., Zhang, M., Zhang, Y., Wu, W., Xue, P., Zhan, X., et al. (2021). Genetic mapping of grain shape associated qtl utilizing recombinant inbred sister lines in high yielding rice (Oryza sativa L.). Agronomy 11:705. doi: 10.3390/agronomy11040705
Kang, Y. J., Shim, K. C., Lee, H. S., Jeon, Y. A., Kim, S. H., Kang, J. W., et al. (2018). Fine mapping and candidate gene analysis of the quantitative trait locus gw8. 1 associated with grain length in rice. Genes Genomics 40, 389–397. doi: 10.1007/s13258-017-0640-6
Kavitha, B., Divya, B., Malathi, S., Krishnamraju, A., Venkateshwara Rao, Y., and Sarla, N. (2019). Evaluation of yield and seedling vigour related traits of Swarna/Oryza nivara backcross introgression lines under three environment conditions. Ecol. Genet. Genomics 11:100036.
Kim, C.-K., Chu, S.-H., Park, H. Y., Seo, J., Kim, B., Lee, G., et al. (2017). Identification of heterosis QTLs for yield and yield-related traits in indica-japonica recombinant inbred lines of rice (Oryza sativa L.). Plant Breed. Biotechnol. 5, 371–389. doi: 10.9787/pbb.2017.5.4.371
Kim, D. M., Lee, H. S., Kwon, S. J., Fabreag, M. E., Kang, J. W., Yun, Y. T., et al. (2014). High-density mapping of quantitative trait loci for grain-weight and spikelet number in rice. Rice 7, 1–11. doi: 10.1186/s12284-014-0014-5
Kumar, N., Jain, R. K., and Chowdhury, V. K. (2019). Linkage mapping of QTLs for grain minerals (iron and zinc) and physio-morphological traits for development of mineral rich rice (Oryza sativa L.). Indian J. Biotechnol. 18, 69–80.
Li, Z. K., Yu, S. B., Lafitte, H. R., Huang, N., Courtois, B., Hittalmani, S., et al. (2003). QTL x environment interactions in rice. I. Heading date and plant height. Theor. Appl. Genet. 108, 141–153. doi: 10.1007/s00122-003-1401-2
Lim, J. H., Yang, H. J., Jung, K. H., Yoo, S. C., and Paek, N. C. (2014). Quantitative trait locus mapping and candidate gene analysis for plant architecture traits using whole genome re-sequencing in rice. Mol. Cells 37, 149–160. doi: 10.14348/molcells.2014.2336
Lin, T., Zhou, C., Chen, G., Yu, J., Wu, W., Ge, Y., et al. (2020). Heterosis-associated genes confer high yield in super hybrid rice. Theor. Appl. Genet. 133, 3287–3297. doi: 10.1007/s00122-020-03669-y
Ma, X., Fu, Y., Zhao, X., Jiang, L., Zhu, Z., Gu, P., et al. (2016). Genomic structure analysis of a set of Oryza nivara introgression lines and identification of yield-associated QTLs using whole-genome resequencing. Sci. Rep. 6:27425. doi: 10.1038/srep27425
Mahmoud, A. A., Sukumar, S., and Krishnan, H. B. (2008). Interspecific rice hybrid of Oryza sativa x Oryza nivara reveals a significant increase in seed protein content. J. Agric. Food Chem. 56, 476–482. doi: 10.1021/jf071776n
Matsubara, K., Yamamoto, E., Kobayashi, N., Ishii, T., Tanaka, J., Tsunematsu, H., et al. (2016). Improvement of rice biomass yield through QTL-based selection. PLoS one 11:e0151830. doi: 10.1371/journal.pone.0151830
Miura, K., Ikeda, M., Matsubara, A., Song, X. J., Ito, M., Asano, K., et al. (2010). OsSPL14 promotes panicle branching and higher grain productivity in rice. Nat. Genet. 42, 545–549. doi: 10.1038/ng.592
Mohammadi, R., Mendioro, M. S., Diaz, G. Q., Gregorio, G. B., and Singh, R. K. (2013). Mapping quantitative trait loci associated with yield and yield components under reproductive stage salinity stress in rice (Oryza sativa L.). J. Genet. 92, 433–443. doi: 10.1007/s12041-013-0285-4
Pang, Y., Chen, K., Wang, X., Wang, W., Xu, J., Ali, J., et al. (2017). Simultaneous improvement and genetic dissection of salt tolerance of rice (Oryza sativa L.) by designed QTL pyramiding. Front. Plant Sci. 8:1275. doi: 10.3389/fpls.2017.01275
Qiao, W., Qi, L., Cheng, Z., Su, L., Li, J., Sun, Y., et al. (2016). Development and characterization of chromosome segment substitution lines derived from Oryza rufipogon in the genetic background of O. sativa spp. indica cultivar 93-11. BMC Genomics 17:580. doi: 10.1186/s12864-016-2987-5
Rabiei, B., Kordrostami, M., Sabouri, A., and Sabouri, H. (2015). Identification of QTLs for yield related traits in indica type rice using SSR and AFLP Markers. Agric. Conspectus Sci. 80, 91–99.
Sabouri, H., Sabouri, A., and Dadras, A. R. (2009). Genetic dissection of biomass production, harvest index and panicle characteristics in indica-indica crosses of Iranian rice (Oryza sativa L.) cultivars. Austral. J. Crop Sci. 3, 155–166.
Salgotra, R. K., Gupta, B. B., Bhat, J. A., and Sharma, S. (2015). Genetic diversity and population structure of Basmati rice (Oryza sativa L.) germplasm collected from North Western Himalayas using trait linked SSR markers. PLoS One 10:e0131858. doi: 10.1371/journal.pone.0131858
Sarla, N., Bobba, S., and Siddiq, E. A. (2003). ISSR and SSR markers based on AG and GA repeats delineate geographically diverse Oryza nivara accessions and reveal rare alleles. Curr. Sci. 84, 683–690.
Sato, H., Ideta, I. O., Ando, I., Kunihiro, Y., Hirabayashi, H., Iwano, M., et al. (2004). Mapping QTLs forsheath blight resistance in the rice line WSS2. Breed. Sci. 54, 265–271.
Segami, S., Yamamoto, T., Oki, K., Noda, T., Kanamori, H., Sasaki, H., et al. (2016). Detection of novel QTLs regulating grain size in extra-large grain rice (Oryza sativa L.) lines. Rice 9:34. doi: 10.1186/s12284-016-0109-2
Shao, G. N., Tang, S. Q., Luo, J., Jiao, G. A., Tang, A., and Hu, P. S. (2009). QTL analysis for flag leaf and grain shape and populations construction derived from related residual heterozygous lines in rice. Plant Mol. Breed. 7, 16–22.
Shen, B., Yu, W. D., Du, J. H., Fan, Y. Y., Wu, J. R., and Zhuang, J. Y. (2011). Validation and dissection of quantitative trait loci for leaf traits in interval RM4923-RM402 on the short arm of rice chromosome 6. J. Genet. 90, 39–44. doi: 10.1007/s12041-011-0019-4
Singh, V. K., Ellur, R. K., Singh, A. K., Nagarajan, M., Singh, B. D., and Singh, N. K. (2018). Effect of qGN4.1 QTL for grain number per panicle in genetic backgrounds of twelve different mega varieties of rice. Rice 11:8. doi: 10.1186/s12284-017-0195-9
Su, C., Lu, W., Zhao, T., and Gai, J. (2010). Verification and fine-mapping of QTLs conferring days to flowering in soybean using residual heterozygous lines. Chinese Sci. Bull. 55, 499–508. doi: 10.1007/s11434-010-0032-7
Sun, P., Zheng, Y., Li, P., Ye, H., Zhou, H., Gao, G., et al. (2019). Dissection and validation of minor quantitative trait loci (QTLs) conferring grain size and weight in rice. bioRxiv [Preprint]. doi: 10.1101/511139
Surapaneni, M., Divya, B., Sukumar, M., Raju, A. K., Rao, Y. V., Tripura Venkata, V. G. N., et al. (2017). Identification of major effect QTLs for agronomic traits and CSSLs in rice from Swarna/O nivara derived backcross inbred lines. Front. Plant Sci. 8:1027. doi: 10.3389/fpls.2017.01027
Swamy, B. P., Kaladhar, K., Reddy, G. A., Viraktamath, B. C., and Sarla, N. (2014). Mapping and introgression of QTL for yield and related traits in two backcross populations derived from Oryza sativa cv. Swarna and two accessions of O.nivara. J. Genet. 93, 643–654. doi: 10.1007/s12041-014-0420-x
Swamy, B. P. M., Kaladhar, K., Ramesha, M. S., Viraktamath, B. C., and Sarla, N. (2011). Molecular mapping of QTLs for yield and related traits in Oryza sativa cv Swarna x O. nivara (IRGC81848) backcross population. Rice Sci. 18, 178–186. doi: 10.1016/s1672-6308(11)60025-5
Swamy, B. P. M., Kaladhar, K., Shobha Rani, N., Prasad, G. S. V., Viraktamath, B. C., Reddy, A. G., et al. (2012). QTL analysis for grain quality traits in 2 BC2F2 populations derived from crosses between Oryza sativa cv Swarna and 2 accessions of O.nivara. J. Hered. 103, 442–452. doi: 10.1093/jhered/esr145
Swamy, B. P. M., and Sarla, N. (2008). Yield enhancing quantitative trait loci (QTLs) from wild 19 species. Biotechnol. Adv. 26, 106–120.
Swamy, B. P. M., and Sarla, N. (2011). Meta-analysis of yield QTLs derived from inter-specific crosses of rice reveals consensus regions and candidate genes. Plant Mol. Biol. Rep. 29, 663–680.
Tian, F., Li, D. J., Fu, Q., Zhu, Z. F., Fu, Y. C., Wang, X. K., et al. (2006). Construction of introgression lines carrying wild rice (Oryza rufipogon Griff.) segments in cultivated rice (Oryza sativa L.) background and characterization of introgressed segments associated with yield-related traits. Theor. Appl. Genet. 112, 570–580. doi: 10.1007/s00122-005-0165-2
Tian, Y., Zhang, H., Xu, P., Chen, X., Liao, Y., Han, B., et al. (2015). Genetic mapping of a QTL controlling leaf width and grain number in rice. Euphytica 20, 1–11. doi: 10.1007/s10681-014-1263-5
Vaughan, D. A., Lu, B. R., and Tomooka, N. (2008). The evolving story of rice evolution. Plant Sci. 174, 394–408. doi: 10.1016/j.plantsci.2008.01.016
Vaughan, D. A., Morishima, H., and Kadowaki, K. (2003). Diversity in the Oryza genus. Curr. Opin. Plant Biol. 6, 139–146.
Verma, R. K., Chetia, S. K., Dey, P. C., Baruah, A. R., and Modi, M. K. (2017). Mapping of QTLs for grain yield and its component traits under drought stress in elite rice variety of assam. Int. J. Curr. Microbiol. Appl. Sci. 6, 1443–1455.
Wang, D., Sun, W., Yuan, Z., Sun, Q., Fan, K., Zhang, C., et al. (2021). Identifcation of a novel QTL and candidate gene associated with grain size using chromosome segment substitution lines in rice. Sci. Rep. 11:189. doi: 10.1038/s41598-020-80667-6
Wang, H., Zhang, X., Yang, H., Liu, X., Li, H., Yuan, L., et al. (2016). Identifcation of heterotic loci associated with grain yield and its components using two CSSL test populations in maize. Sci. Rep. 6:38205. doi: 10.1038/srep38205
Wang, J. (2009). Inclusive composite interval mapping of quantitative trait genes. Acta Agron. Sin. 35, 239–245.
Wang, S., Cui, G., Wang, H., Ma, F., Xia, S., Li, Y., et al. (2018). Identification and QTL mapping of Z550, a rice backcrossed inbred line with increased grains per panicle. J. Integr. Agric. 17, 60345–60347.
Wang, S. K., Wu, K., Yuan, Q. B., Liu, X. Y., Liu, Z. B., Lin, X. Y., et al. (2012). Control of grain size, shape and quality by OsSPL16 in rice. Nat. Genet. 44, 950–954. doi: 10.1038/ng.2327
Wickneswari, R., Bhuiyan, M. A. R., Sabu, K. K., Lim, L. S., Thomson, M. J., Narimah, M. K., et al. (2012). Identification and validation of quantitative trait loci for agronomic traits in advanced backcross breeding lines derived from Oryza rufipogon x Oryza sativa cultivar MR219. Plant Mol. Biol. Rep. 30, 929–939.
Xie, X., Jin, F., Song, M. H., Suh, J. P., Hwang, H. G., Kim, Y. G., et al. (2008). Fine mapping of a yield-enhancing QTL cluster associated with transgressive variation in an Oryza sativa× O. rufipogon cross. Theor. Appl. Genet. 116, 613–622. doi: 10.1007/s00122-007-0695-x
Xie, X., Song, M. H., Jin, F., Ahn, S. N., Suh, J. P., Hwang, H. G., et al. (2006). Fine mapping of a grain weight quantitative trait locus on rice chromosome 8 using near-isogenic lines derived from a cross between Oryza sativa and Oryza rufipogon. Theor. Appl. Genet. 113, 885–894. doi: 10.1007/s00122-006-0348-5
Xing, W., Zhao, H., and Zou, D. (2014). Detection of main-effect and epistatic QTL for yield-related traits in rice under drought stress and normal conditions. Can. J. Plant Sci. 94, 633–641.
Xue, W., Xing, Y., Weng, X., Zhao, Y., Tang, W., Wang, L., et al. (2008). Natural variation in Ghd7 is an important regulator of heading date and yield potential in rice. Nat. Genet. 40, 761–767. doi: 10.1038/ng.143
Yang, J., Luo, D., Yang, B., Frommer, W. B., and Eom, J. S. (2018). SWEET11 and 15 as key players in seed filling in rice. New Phytol. 218, 604–615. doi: 10.1111/nph.15004
Yano, M., Katayose, Y., Ashikari, M., Yamanouchi, U., Monna, L., Fuse, T., et al. (2000). Hd1, a major photoperiod sensitivity quantitative trait locus in rice, is closely related to the Arabidopsis flowering time gene constans. Plant Cell 12, 2473–2484. doi: 10.1105/tpc.12.12.2473
Zhang, J., Ou, X., Hu, H., Du, B., Lv, W., Yang, L., et al. (2018). Identification of QTLs for yield-related traits using two sets of introgression lines with a common donor parent in rice. Int. J. Agric. Biol. 20, 15–24.
Zhang, L., Wang, S., Li, H., Deng, Q., Zheng, A., Li, S., et al. (2010). Effects of missing marker and segregation distortion on QTL mapping in F2 populations. Theor. Appl. Genet. 121, 1071–1082. doi: 10.1007/s00122-010-1372-z
Zhang, S., He, X., Zhao, J., Cheng, Y., Xie, Z., Chen, Y., et al. (2017). Identification and validation of a novel major QTL for harvest index in rice (Oryza sativa L.). Rice 10:44. doi: 10.1186/s12284-017-0183-0
Keywords: Oryza, alien introgression lines, yield QTL, thousand grain weight, wild species
Citation: Beerelli K, Balakrishnan D, Addanki KR, Surapaneni M, Rao Yadavalli V and Neelamraju S (2022) Mapping of QTLs for Yield Traits Using F2:3:4 Populations Derived From Two Alien Introgression Lines Reveals qTGW8.1 as a Consistent QTL for Grain Weight From Oryza nivara. Front. Plant Sci. 13:790221. doi: 10.3389/fpls.2022.790221
Received: 06 October 2021; Accepted: 09 February 2022;
Published: 09 March 2022.
Edited by:
Dayun Tao, Yunnan Academy of Agricultural Sciences, ChinaReviewed by:
Kishor Kumar, Ramakrishna Mission Vivekananda Educational and Research Institute, IndiaWricha Tyagi, Central Agricultural University, India
Copyright © 2022 Beerelli, Balakrishnan, Addanki, Surapaneni, Rao Yadavalli and Neelamraju. This is an open-access article distributed under the terms of the Creative Commons Attribution License (CC BY). The use, distribution or reproduction in other forums is permitted, provided the original author(s) and the copyright owner(s) are credited and that the original publication in this journal is cited, in accordance with accepted academic practice. No use, distribution or reproduction is permitted which does not comply with these terms.
*Correspondence: Divya Balakrishnan, ZGJpaXJyMjNAZ21haWwuY29t; Sarla Neelamraju, c2FybGFfbmVlbGFtcmFqdUB5YWhvby5jb20=