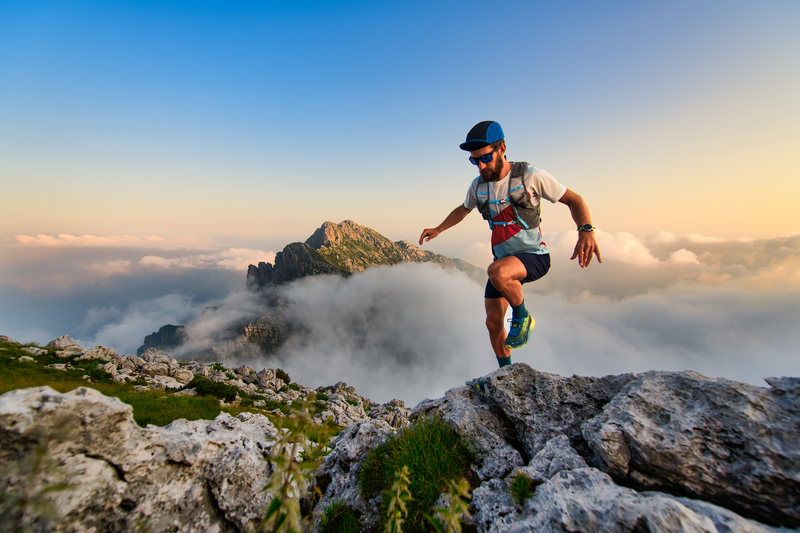
94% of researchers rate our articles as excellent or good
Learn more about the work of our research integrity team to safeguard the quality of each article we publish.
Find out more
ORIGINAL RESEARCH article
Front. Plant Sci. , 25 March 2022
Sec. Functional Plant Ecology
Volume 13 - 2022 | https://doi.org/10.3389/fpls.2022.757077
This article is part of the Research Topic Patterns, Functions, and Processes of Alpine Grassland Ecosystems under Global Change. View all 76 articles
Leaf traits are important indicators of plants’ adaptive strategy to environmental changes. It is an established fact that leaf traits are jointly regulated by climatic and edaphic factors besides genetic factors. However, the relative importance of these abiotic forces in determining the general patterns of herbaceous plant leaf traits across different climatic regions in China is far from clear. We collected 1,653 observations of 542 species of herbaceous plant leaf traits including leaf mass per area, leaf nitrogen, and leaf phosphorus from 316 sampling sites across four climatic regions. We found that the leaf mass per area in the arid region was apparently larger than the others, whereas the smallest mass-based leaf nitrogen and mass-based leaf phosphorus were found in the humid region. Increased growing season temperature and evapotranspiration consistently promoted a conservative growth strategy indicated by higher relative benefit of leaf mass per area, especially in the arid region. Solar radiation in growing season promoted an acquisitive growth strategy indicated by higher relative benefits of mass-based leaf nitrogen and phosphorus in the humid region, but opposite patterns were found in the arid region and semi-humid region. Of all the soil nutrients including soil organic matter, total nitrogen, total phosphorus, and available nitrogen, soil available nitrogen was the strongest predictor of relative benefits of leaf traits associated with a nutrient acquisitive strategy, except in the nutrient-rich semi-humid region. There was a relatively larger number of abiotic factors contributing to relative benefits of leaf traits in the arid and humid regions. We concluded that plant functionality could respond divergently to the same factor facing different habitat conditions. Moreover, the relative benefits of leaf traits tended to be more vulnerable to abiotic filtering in more stressful conditions. Our findings have important implications for understanding the context-dependency of plant functionality to environmental filtering and further improving the predictability of plant dynamics under global change.
Leaf traits are important predictors of evolved plant adaptations to particular inhabiting environments (Violle et al., 2014). They are related to numerous ecological functions: the leaf mass per area (LMA) indicates the investment of photosynthesis and nutrients acquired per unit leaf area; the leaf nitrogen (N) is an essential component of proteins for photosynthesis; and the leaf phosphorus (P) is integral to the nucleic acids and bioenergetics molecules fundamental for metabolism (Wright et al., 2004). Generally, a higher LMA with denser mesophyll tissues and thicker-walled cells allows for a longer leaf lifespan (Wright et al., 2006; Wright and Suttongrier, 2012), whereas higher leaf N and P-values, which are associated with higher photosynthetic rates, are observed in leaves with shorter longevity (Gusewell and Koerselman, 2002; Han et al., 2005). The trait-based ecology theory states that environmental changes shape leaf traits (Wang, 2007), which in turn affect ecosystem processes (Van Bodegom et al., 2007; Pearson et al., 2013; Bjorkman et al., 2018), and then soil quality (Buzzard et al., 2019). Variability of leaf traits allows individual plants to adapt to new environmental conditions. The plasticity of traits provides a mechanism for selection by altered environmental conditions that will drive future ecosystem processes.
The adaption and evolution of the leaf traits regulate plant survival and growth under a given set of conditions (Reichstein et al., 2014; Asner et al., 2016). There are two strategies reflecting the trade-off between plant persistence and productivity, and with contrary investment-return patterns, i.e., a slow-growing versus a fast-growing strategy. The former corresponds to greater resource conservation indicated by high relative benefit of LMA, low relative benefit of leaf N and leaf P under a stressful environment (Reich et al., 2003; Carvajal et al., 2019), whereas the latter refers to a quick investment-return pattern that is often associated with rapid resource acquisition indicated by low relative benefit of LMA, high relative benefits of leaf N and leaf P in optimal conditions (Shipley et al., 2006; Wright and Suttongrier, 2012). These strategies provide the most promising methods for determining how an ecosystem functions, such as indicating how the primary productivity and carbon/N cycling vary when facing different environmental conditions. Therefore, obtaining knowledge of leaf traits is a high priority for understanding terrestrial ecosystem adaptations to changing environments, and predicting how ecological processes shift with rapid climate change.
In the past decades, a compelling number of studies have attempted to reveal the relationships between leaf trait and its drivers (Wright et al., 2005; Ordonez and Olff, 2013; Carvajal et al., 2019). Both natural selection (Asner et al., 2016) and genetic constraints (Lusk et al., 2008) control the strength and direction of leaf trait evolution, and the given genetic constraints might play a relatively weaker role in shaping the leaf traits (Reich et al., 2003). In fact, the leaf traits may vary with the plant growth form (Simova et al., 2018; Gong and Gao, 2019), climate (Moles et al., 2014), soil fertility (Asner et al., 2016), taxonomy and phylogeny (He et al., 2009), and sampling scale (Borgy et al., 2017). Thus, no consistent patterns were found from the different studies. For instance, the trait-based ecology theory considers temperature as the central driver of leaf trait variation (Michaletz et al., 2016; Buzzard et al., 2019), but leaf N has been observed to significantly increase (Ordonez et al., 2009; Swenson et al., 2012) or decrease with increasing temperature (Moles et al., 2014; Simova et al., 2017); the soil nutrient supply may play a minor (Bowman et al., 2003) or influential (Ordonez et al., 2009; Reich et al., 2009) role in shaping the leaf traits; and precipitation also shows either strong (Swenson et al., 2012; Asner et al., 2016) or weak (Moles et al., 2014) relationships with leaf traits. To date, there has been no general pattern for the drivers of leaf traits, challenging its universality and predictability (Shipley et al., 2016).
Water and heat availability greatly affect nutrient cycling (Schuur and Matson, 2001), and play crucial roles in mediating plant growth (Sun and Du, 2017; Sun et al., 2020). The favourability hypothesis in biogeography suggests that species in a temperate zone with temperature seasonality should closely follow environmental filtering rules (Fischer, 1960). Previous studies have revealed that species with a narrow geographical range often adapt an extreme drought habitat, with stress-tolerant leaf traits (Thuiller et al., 2004; Geng et al., 2012). Long-term specific water and heat patterns would induce shifts in plant functional compositions (Griffinnolan et al., 2019). That is, the water and heat regime can shape the plant adaptations to the environment, and thereby affect the spatial distribution of the leaf traits (Schwinning and Ehleringer, 2001; Zhang et al., 2017). Though winter climate can affect plant growth especially in more northern latitudes and for cold climate systems, the climatic conditions in growing season play important roles in the growth of plants and thereby their leaf traits.
China has an east-to-west precipitation and temperature gradient as the dominant basis of the climate-region divisions (Zhang and Shen, 2008; Zhang et al., 2016). Meanwhile, herbaceous plants in China cover the broadest geographic range, from a hot arid desert steppe at the lowlands to a cold humid alpine meadow with a ≥4,000 m altitude (Chen et al., 2013), offering an ideal macrocosm for evaluating the spatial pattern of the leaf traits and interpreting plant adaptations to environmental gradients (Westoby and Wright, 2006). However, early studies focusing on the leaf trait response to environmental gradients were mostly performed at small scales (Stubbs and Wilson, 2004; He et al., 2006; Wang, 2007; Werden et al., 2018; Zhang X. L. et al., 2019), and a large-scale leaf trait pattern across China has not been previously reported. We hypothesised that the leaf traits might be divergent and mediated in different ways among different climatic regions across China. In this study, we aimed to address two questions. First, how does China’s herbaceous plant leaf trait change along climatic regions? Second, how do edaphic and climatic factors regulate the leaf traits, specifically in different climatic regions? The answers will advance our knowledge of the ecological filtering and evolution shaping the leaf traits. Moreover, they will help in developing more quantitative and predictive ecological models for incorporating leaf functional traits applicable for China’s terrestrial ecosystems under climate change and anthropogenic activities.
The raw data were either obtained from tables or figures (extracted by using the GetData Graph Digitizer, version 2.24)1, and a list of all contributing papers were included in Supplementary 2 (Supplementary Material). For each publication, we recorded the following data: site location (longitude and latitude) as well as herbaceous plant LMA, leaf N concentration per mass (Nmass), and leaf P concentration per mass (Pmass) as three key leaf functional traits associated with the leaf traits and growing strategies (Diaz et al., 2016). Finally, our database consists of 1,653 observations of 542 species of herbaceous plant from 316 sampling sites across China [shown in Supplementary 3 (Supplementary Material)], with longitude ranging from 74.93°E to 123.92°E, and latitude ranging from 19.10°N to 50.19°N (Figure 1). According to the dry and wet climate-region division in China2 (Thornthwaite, 1948), our sampling sites are distributed in four climatic regions: arid, semi-arid, semi-humid, and humid regions in China (Figure 1).
Figure 1. The distributions of sampling sites collected in this study across four China’s climatic regions which are divided based on the method of Thornthwaite (1948) and the data from http://www.resdc.cn/data.aspx?DATAID=253. The inlay included in the current map presents nine-dotted line of China.
The climate (precipitation, temperature, evapotranspiration, and solar radiation) data were derived from the database of WorldClime 2.0 Beta version 1 (June 2016), as downloaded from http://worldclim.org. They represent average monthly climate data for 1970–2000. The soil attributes [soil organic matter (SOM); total N (STN) and available N (SAN); and total P (STP)] were extracted from a Chinese dataset of soil properties, which was constructed based on a 1:1,000,000 soil map of China and 8,595 soil profiles (Shangguan et al., 2013). Due to the constraint based on data availability and accuracy, there are many measurements of leaf traits at the same soil property level especially for STP. To explore the effects of climatic factors on plant functional traits, we used the mean values from May to September, the growing season, for temperate zone where our sites are mostly located, including the mean growing season precipitation (GSP), mean growing season temperature (GST), mean growing season evapotranspiration (GSE), and mean growing season solar radiation (GSR). Abbreviations of these variables are shown in Table 1. The edaphic and climatic information of the sampling sites were extracted according to geographical location (latitude and longitude) through ArcGIS 10.1 (ESRI, Inc., Redlands, CA, United States). The frequency distributions of the leaf traits (LMA, Nmass, and Pmass) were used to ensure the normality of the distribution (Zhou et al., 2019).
The benefit for a single objective leaf trait is defined as the relative deviation from the mean of a given observation. The relative benefit for the objective leaf trait (LMA, Nmass, or Pmass) indicates the relative magnitude of the values of these leaf traits, and is calculated as follows (Bradford and Damato, 2012; Sun et al., 2018):
where i is a certain leaf trait (LMA, Nmass, or Pmass), Bi is the magnitude of the relative benefit for objective leaf trait i, xi, xmin, and xmax are the observed value of i, minimum, and maximum values for all measured i, respectively. A three-dimensional (3D) relative benefit method was used to describe the leaf traits (relative benefits among LMA, Nmass, or Pmass) (Li et al., 2019). As shown in Supplementary Figure 1, the vertical distance (l) from the 3D point to the 1:1:1 line represents the magnitude of benefit for the three objective leaf traits, forming 3D coordinates in the LMA-Nmass-Pmass economic spectrum. The relative benefit was decomposed into the LMA (a), Nmass (c), and Pmass (b) directions, whose values could reflect a plant’s growing strategy (Supplementary Figure 1): a low relative benefit of LMA combined with high relative benefits of Nmass and Pmass implied a quick investment-return growth strategy; in contrast, a slow investment-return growth strategy was indicated by a high relative benefit of the LMA combined with low relative benefits of Nmass and Pmass.
A principal component analysis (PCA) was performed with all the potential explanatory variables pooled together, to determine the explanatory powers of variance in the leaf functional traits, soil properties, and climatic factors among different climatic regions. The packages of “FactoMineR,” “factoextra,” and “corrplot” in R software (R Core Team, 2016) were used for the PCA. A one-way analysis of variance was performed with SPSS 19.0 software (SPSS Inc., Chicago, IL, United States) to detect differences in the plant, soil, and climate variables among the four climatic regions, using the least significant difference (LSD) as post hoc analysis. Regression and correlation analyses were conducted with R Version 3.3.2 (R Core Team, 2016) and SigmaPlot 14.0 software (Systat Software, Inc., Chicago, IL, United States) in each climatic region, to explore the correlations of leaf traits with edaphic and climatic variables. Besides, the correlogram of intercorrelations among relative benefits of leaf traits, soil properties, and climate factors was graphed by “mgcv” package in software R (R Core Team, 2016).
The LMA in the arid region, with a mean value of 124.34 g m–2, was significantly higher than the mean values of 97.64, 81.90, and 79.73 g m–2 for the semi-arid, semi-humid, and humid regions, respectively (P < 0.05, Figure 2A). Nmass and Pmass tended to initially increase and then decrease from arid to humid regions. The average values of Nmass were 23.51, 24.82, 25.92, and 16.64 mg g–1 for the arid, semi-arid, semi-humid, and humid regions, respectively (Figure 2B). Pmass was significantly different among the four climatic regions (P < 0.05), with mean values of 1.52, 1.70, 1.86, and 1.27 mg g–1 for the arid, semi-arid, semi-humid, and humid regions, respectively (Figure 2C). The LMA was negatively associated with Nmass and Pmass, with the deepest slope in the arid region (Figures 2D,E), whereas Nmass was positively correlated with Pmass (Figure 2F).
Figure 2. The boxplot (A–C) and linear regression relationship (D–F) of herbaceous plant LMA, Nmass, Pmass among four China’s climatic regions. The different letters in (A–C) represent significance at the 5% confidence level. The black line in (D–F) indicates the linear regression of the total data. LMA, Nmass, and Pmass represent leaf mass per area, mass-based leaf nitrogen, and mass-based leaf phosphorus, respectively.
The PCA results indicated that two components explained 57.4% of the total variance (Supplementary Figure 2). Specifically, the first principal component (PC1) was highly relevant to the GSP, GSE, GSR, Nmass, and Pmass; the second principal component (PC2) was highly relevant to the SOM, STN, SAN, SAP, and LMA (Supplementary Figure 2). For the soil properties, SOM, STN, STP, and SAN presented a unimodal pattern along climatic regions, and peaked in the semi-humid region, with average values of 4.77, 0.23, 0.08, and 173.65 mg kg–1, respectively (Supplementary Table 1). For the climatic variables, from arid to humid region, the GST initially decreased, and then increased with a minimum value of 9.55°C in the semi-humid region, while there was a significant decrease in the GSR (Supplementary Table 1).
In regard to the climatic factors, GST, GSE, and GSR positively affected the relative benefit of the LMA, but negatively influenced the relative benefits of Nmass and Pmass in the arid and semi-humid regions, similar to the effect of GST in the humid region (P < 0.05, Figures 3A1–C1, A3–C3, A4–C4 and Table 2). However, the relationship patterns of the GSR with the leaf traits in the humid region were converse to those in the arid and semi-arid regions (Figures 3A4–C4). The GSP showed weak negative correlations with the relative benefits of the LMA but was positively associated with the relative benefits of Nmass and Pmass in the semi-arid and semi-humid regions (Figures 3A2–C2).
Figure 3. Relationships between the relative benefits of leaf traits with growing season temperature (A1–C1), precipitation (A2–C2), evapotranspiration (A3–C3) and solar radiation (A4–C4). The red, blue, green, and purple colours represent the arid, semi-arid, semi-humid, and humid climatic regions, respectively. LMA, leaf mass per area; Nmass, mass-based leaf nitrogen; Pmass, mass-based leaf phosphorus; GST, growing season temperature; GSP, growing season precipitation; GSE, growing season evapotranspiration; GSR, growing season solar radiation. The statistical information of linear regression are shown in Table 2.
Table 2. Statistical information of linear regression relationships between the relative benefits of leaf traits and climatic factors.
For the edaphic factors, SOM, STN, and STP were negatively related to the relative benefit of the LMA, but positively affected the relative benefits of Nmass and Pmass in the semi-arid regions (Figures 4A1–C2 and Supplementary Figure 7). Similar correlations were observed between the SAN with the relative benefits of the LMA, Nmass, and Pmass, except in the semi-humid region (Figures 4A3–C3). The number of key edaphic and climatic factors driving the leaf traits in the arid and humid regions was greater than those in the semi-arid and semi-humid regions (Figure 5).
Figure 4. Relationships between the relative benefits of leaf traits with soil organic matter (A1–C1), total nitrogen (A2–C2) and available nitrogen (A3–C3). The red, blue, green, and purple colours represent the arid, semi-arid, semi-humid, and humid climatic region, respectively. LMA, leaf mass per area; Nmass, mass-based leaf nitrogen; Pmass, mass-based leaf phosphorus; SOM, soil organic matter; STN, soil total nitrogen; SAN, soil available nitrogen. The statistical information of linear regression are shown in Table 3.
Table 3. Statistical information of linear regression relationships between the relative benefits of leaf traits and edaphic factors.
Figure 5. Conceptual frameworks of relationship between leaf traits and environment reflect changes in the responses of plant growing strategies to environmental conditions. Subgraph (A) the relative benefit of LMA decreases while relative benefits of Nmass and Pmass increase when the environmental stress weakens, indicating an investment-return strategy changing from slow to quick (① and ②), ④ and ⑤ represent opposite trends from optimal to extreme environmental conditions; the leaf traits are more sensitive to environmental drivers with steeper slopes (① and ⑤) in an extreme environment than in an optimal environment (②, ③, and ④). The bar chart shows the numbers of the key climatic and edaphic factors controlling the relative benefits of leaf traits, with R square values no less than 0.20 according to the correlogram of intercorrelations (Supplementary Figure 5); the red and black colours represent climatic and edaphic factors, respectively. LMA, leaf mass per area; Nmass, mass-based leaf nitrogen; Pmass, mass-based leaf phosphorus. The shades of the colours represent the investment-return rate, the extent of environmental stress, and the magnitude of relative benefits of LMA, Nmass, and Pmass, respectively. Subgraph (B) a conceptual framework of environmental stress and cost for resource-regulating plant growth rate and resource acquisition indicated by leaf traits. Arrow ① and ②: the relationship curve turns but keeps a downward sloping trend when the environmental stress and costs for resources shift. The slope of curve b under high environmental stress is generally steeper than that of curve a in a less stressful environment. Arrow ③: plant growth rate and resource acquisition slide along curve a or b driven by environmental filtering. For example, as the environmental stress characterised by low resource availability strengthens, the cost for resources increases, so that the plants select a conservative strategy with a slow growth rate and long-life span.
Our study showed that the LMA in the arid region was drastically higher than those in other regions (Figure 2A), indicating that plants in hyper-arid conditions invest more photosynthate per unit leaf area. This result is in agreement with many previous studies indicating that plants inhabiting arid areas have a high LMA as manifested by thicker leaves with denser mesophyll (Fonseca et al., 2000; Niinemets, 2001; Wright et al., 2001), often combined with smaller and thicker-walled cells for reducing water loss (Wright et al., 2006). As the maximum photosynthetic rate of plants in a dry environment is relatively lower at the same level of leaf N content, the high LMA increases the photosynthetic tissue per unit area supporting photosynthesis, and allows for continued leaf function in arid conditions (Evans and Poorter, 2001). In addition, the SOM, STN, STP, and SAN in the arid region are significantly lower than those in other regions (Supplementary Table 1), and the low available water decreases the soil nutrient availability (Sardans and Penuelas, 2004) and further restricts plant nutrient absorption (Farooq et al., 2009). Consequently, survival is the primary goal for plants, which tend to select a relatively conservative investment strategy. The higher LMA in the arid region should be interpreted as an adaptive strategy of plants against extreme environmental stress, which was alleviated in other climatic regions, and the LMA was relatively smaller.
The leaf N and P values play pivotal roles in photosynthesis and protein synthesis, respectively (Niklas et al., 2005; Westoby and Wright, 2006). The Nmass and Pmass values in the humid region were dramatically lower than those in the other regions (Figures 2B,C), indicating a small carbon gain rate. This is probably because frequent strong precipitations accelerated the soil erosion and nutrient losses in the humid region, indicated by that the soil nutrients were significantly lower than those in the semi-humid region (Supplementary Table 1). Moreover, the soils were often waterlogged and anoxic, depressing the oxidative metabolic processes of the roots and microbes in the mesic lands, where the plant growth rates were greatly inhibited by excessive rainfall (Zhang S. S. et al., 2019; Zheng Q. et al., 2019).
We observed significantly negative correlations of the LMA with Nmass and Pmass (Figures 2D,E), which agrees with previous studies demonstrating that a higher LMA is often associated with lower leaf N and P concentrations, indicating slow growth rates (Santiago and Wright, 2007; Donovan et al., 2011). Nevertheless, the leaves with a high LMA and low Nmass and Pmass are more tolerant to unfavourable growing conditions. In contrast, plants would be vulnerable to damages from environmental stress if a high LMA was combined with high Nmass and Pmass, as it would accelerate energy losses via respiration (Wright et al., 2004). This might explain why negative-relationship slopes were the deepest in the arid region (Figures 2D,E).
The favourability hypothesis suggests that plants tend to choose certain optimum trait assemblages when facing particular inhabiting conditions, either through trait plasticity within a species or via species turnover in community composition (Swenson et al., 2012; Simova et al., 2015). Temperature which influences plant hydraulics, nutrient utilization, and leaf energy balance, is a central controller of leaf trait variation (Fonseca et al., 2000; Michaletz et al., 2016). In the present study, it was obvious that the orientations of GST and LMA exhibited the smallest angle (Supplementary Figure 2), indicating that the GST tended to promote an increase in the LMA, which conflicts with prior studies (Swenson et al., 2012; Simova et al., 2015; Simova et al., 2018). We propose two probable explanations for the contradictory results. First, the sampling sites in our study covered a relatively narrower GST range (from –1 to 28.63°C) than the former studies. The plant growth form was mainly herbaceous plant species in the temperate zone, unlike, e.g., boreal coniferous species, with higher LMAs in cold conditions for modulating the temperatures of leaves (Michaletz et al., 2016). Second, the soil nutrients such as SOM, STN, STP, and SAN apparently decreased with increases in GST (Supplementary Figure 4). Plants exhibited low potential for resource capture and a slow photosynthetic rate under the more unfavourable inhabiting conditions (Wright et al., 2010; Swenson et al., 2012). They can also explain why the GST negatively influences Pmass and thereby affects the photosynthesis-N relationship (Reich et al., 2009), in accordance with previous studies (Kerkhoff et al., 2005; Sun et al., 2019). In addition, the negative correlation of the GST with Nmass (Supplementary Figure 2) provides evidence for the temperature–plant physiological hypothesis, i.e., leaf N would increase with decreasing temperature, as a high N concentration could compensate for physiological inefficiency in a cold environment (Reich and Oleksyn, 2004).
In this study, we used the benefit metrics for leaf traits to determine changes in plant growth strategy by analysing their relationship with different environmental drivers. Specifically, low relative benefits of LMA, high relative benefits of Nmass and Pmass implied an acquisitive growth strategy; conversely, a conservative growth strategy. Consistent with previous studies indicating that the responses of plant physiological processes to the same factor and underlying rules may be largely divergent in face of different environments (Quan et al., 2019), we found that the relative benefit of LMA was distinctly elevated, while the relative benefits of Nmass and Pmass decreased with increasing GSR in the arid and semi-humid regions, whereas in the humid region, the leaf traits showed the opposite pattern (Figures 3A4–C4). In that regard, the GSR is strongly positively associated with the GST and GSE in the arid and semi-humid regions and displays a significantly negative relation to the GSP in the semi-humid region (Supplementary Figures 3D–F). The radiation-induced warming leads to the reduction of soil water through intensifying evaporation (Wang et al., 2009), thereby aggravating the negative influences of drought in the arid area (Quan et al., 2019). Moreover, an increase in the GSE with increasing GSR accelerates the water consumption from transpiration (Decker et al., 2013). As the GSR increases, plants are expected to adopt conservative investment-return strategies with slow growth rates and higher LMAs with smaller areas exposed to solar radiation per gram leaf, to reduce radiative heating and water loss (Wright et al., 2004; Chen et al., 2013; Moles et al., 2014).
However, for the humid region, the overall relationships of the GIR with the GST, GSP, and GSR are considerably weaker than those in the other regions (Supplementary Figure 3). Indeed, the GIR is the lowest in this region (Supplementary Table 1), implying a relatively limited light resource availability. As a vital factor directly influencing plant growth, solar radiation plays crucial roles in many plant physiological processes, such as leaf N and P accumulations (Takashima et al., 2004; Sun et al., 2019), photosynthesis (Waite and Sack, 2010), and protein synthesis (Xu, 2003). Consistent with Wright et al. (2006), who revealed that plants in higher-irradiance regions had a lower LMA and higher photosynthetic capacity than those in lower-irradiance areas, we observed that the GSR presented a negative impact on the relative benefit of LMA, but remarkably positively affected the relative benefits of Nmass and Pmass in the radiation-lacking region. This indicated that the plant growing strategy shifted from slow to fast as the GSR increased.
Unexpectedly, the GSP showed no significant effect on the relative benefit of leaf traits in the arid region (Figures 3A2–C2). The following several mechanisms might contribute to this. First, although precipitation data are relatively easier to obtain, they are not always sufficient proxies for soil water availability (Hickler et al., 2009), especially in hyper-arid grassland mostly belonging to desert steppe, where the soil water holding capacity are small. Thus, the soil available water is still limited, even if there is relatively high rainfall (Li et al., 2008; Xie, 2011). Second, it may be attributed to the inherent characteristics of plant species evolutionarily adapted to the hyper-arid conditions, with corresponding trait syndromes such as a high LMA and small stature (Wellstein et al., 2017), thereby inducing the plant community to be relatively less sensitive to rainfall (White et al., 2000). Finally, the low soil nutrient contents in this region (Supplementary Table 1) greatly restrict plant growth, which might weaken the relative importance of the GSP in mediating the leaf traits.
Soil nutrients play positive roles in multiple plant physiological activities. For example, the SOM provides energy for heterotrophic N-fixing microbes, and further facilitates plant N fixation (Reed et al., 2011; Zheng M. H. et al., 2019); the soil N can strongly affect the leaf N and plant photosynthesis (Lu et al., 2011; Yue et al., 2019); and a low soil P may lead to a lower leaf P and thus limit plant function (Han et al., 2005; Quesada et al., 2009; Sun et al., 2019). Plants invest more energy for resource acquisition in nutrient-insufficient soil (Nasto et al., 2014). Soil nutrient changes can induce species turnover, by influencing plant facilitation and competitive exclusion (Marriott et al., 2002; Guo, 2003). The regression relation between relative benefits of each leaf trait and soil nutrients indicated that the SOM, STN, and STP consistently presented significant promotion of an acquisitive growth strategy in the semi-arid region (Figure 4, Table 3, and Supplementary Figure 7). This may be ascribed to the poorer soil quality in this region (Supplementary Table 1). In contrast, the soils in the semi-humid region were relatively more fertile, providing plentiful nutrients for plant growth. In that regard, the leaf traits will not respond to the nutrient supply after the soil fertility fully meets the plant demand (Figure 5③; Bowman et al., 2003).
We found no evidence that the SOM, STN, and STP caused variations of relative benefits of leaf traits in the arid region with insufficient soil nutrients (Figure 4 and Supplementary Figure 7). This may be owing to the narrow ranges of the SOM, STN, and STP content. Moreover, drought constrains the effectiveness of soil fertility on plant growth, and thus the sensitivity of the leaf traits to nutrients in the hyper-arid area (Sardans and Penuelas, 2004; Farooq et al., 2009). Additionally, the SAN presented negative effects on the relative benefit of LMA, but positively affected the relative benefits of Nmass and Pmass, promoting an acquisitive investment strategy except in the SAN-rich semi-humid region (Figures 4A3–C3). This agrees with early studies, i.e., that the leaf traits are more related to the N supply at a global scale (Ordonez et al., 2009).
Overall, our results confirm that abiotic filtering shapes the leaf trait pattern, i.e., higher costs for resources and environmental stress lead to a slow-growing strategy with conservative leaf traits, whereas optimal conditions result in a fast tissue turnover with acquisitive leaf traits across climatic regions in China (Figure 5). This supports the favourability hypothesis, i.e., that the plant leaf traits should be governed mainly via environmental filtering in a climatically harsh temperate zone (Fischer, 1960). Furthermore, more key environmental drivers and steeper leaf-trait-environment relationship slopes were found in the arid and humid regions (Figure 5), suggesting that the degree of abiotic filtering is stronger in more stressful environments characterised by e.g., nutrient limitations or drought or over-wet conditions, in line with previous studies (Swenson et al., 2012; Simova et al., 2015). In that regard, the more stressful ecosystems are commonly inhabited by stress-tolerant species with a narrow geographical range size owing to natural selection (Thuiller et al., 2004), which respond more sensitively to environmental changes than wide-ranging species (Geng et al., 2012). Together with the finding that the environmental signal for the leaf traits would be relatively weaker in the optimal environmental conditions represented by the semi-humid region (Supplementary Figure 6), we conclude that an extreme environment elicits extreme ecological responses (Zhang F. Y. et al., 2019) and predict that frequent extreme climatic events caused by future climate change may enhance the fluctuations of the leaf traits in the terrestrial ecosystems (Jentsch and Beierkuhnlein, 2008; Stott et al., 2016).
Our findings provide valuable information for the emerging field of functional biogeography, particularly across the different climatic regions in China. First, the large LMA and small Nmass and Pmass were observed in the arid region, implying that plants under environmental stress as characterised by drought and low nutrient availability are slow-growing, with a conservative investment-return strategy. In contrast, acquisitive leaf traits were found in the semi-humid region, which presented optimal inhabiting conditions. Second, the GST and GSE consistently promoted conservative leaf traits, especially in the arid region. However, the GSR induced an opposite leaf trait pattern between the humid region and the others, confirming that plant functionality could respond divergently to the same factor in the face of different habitat conditions. Of all the studied soil nutrients, the SAN was the best indicator of a large-scale leaf trait pattern. Finally, we revealed that the leaf-trait-environment relationships were stronger under more stressful environmental conditions. These findings will help in refining ecological models and improving their applicability for China’s ecosystems, further enabling us to evaluate the influence of global change. Owing to limited data availability, we only focused on the spatial patterns of the leaf traits and environmental controls with all herbaceous species pooled together; the influences of biotic interactions were not examined in the current study. Further in-depth systematic studies to evaluate the co-effects of abiotic and biotic factors on the leaf traits while considering the plant growth form are therefore highly desirable.
The raw data supporting the conclusions of this article will be made available by the authors, without undue reservation.
ZZ and JS conceived the study and wrote the manuscript. ZZ, JS, ML, HS, JNW, and JSW collected and analysed the data. ZZ, JS, ML, JNW, HZ, and YW drew the graphs. All authors reviewed and revised the manuscript.
This work was financially supported by the National Science Foundation of China (Grant No. 41871040), the Second Tibetan Plateau Scientific Expedition and Research (Grant No. 2019QZKK0405), the Open Project of the Qinghai Provincial Key Laboratory of Restoration Ecology in Cold Area (2020-KF-05), the China Postdoctoral Science Foundation (2021M702682), the Agricultural Research System of China (CARS-34), and the First Class Grassland Science Discipline Programme in Shandong Province of China.
The authors declare that the research was conducted in the absence of any commercial or financial relationships that could be construed as a potential conflict of interest.
All claims expressed in this article are solely those of the authors and do not necessarily represent those of their affiliated organizations, or those of the publisher, the editors and the reviewers. Any product that may be evaluated in this article, or claim that may be made by its manufacturer, is not guaranteed or endorsed by the publisher.
The authors thank Tiancai Zhou and the anonymous referees for their constructive suggestions on this manuscript.
The Supplementary Material for this article can be found online at: https://www.frontiersin.org/articles/10.3389/fpls.2022.757077/full#supplementary-material
Asner, G. P., Knapp, D. E., Anderson, C. B., Martin, R. E., and Vaughn, N. R. (2016). Large-scale climatic and geophysical controls on the leaf economics spectrum. Proc. Natl. Acad. Sci. U.S.A. 113:201604863. doi: 10.1073/pnas.1604863113
Bjorkman, A. D., Myerssmith, I. H., Elmendorf, S. C., Normand, S., Ruger, N., Beck, P. S. A., et al. (2018). Plant functional trait change across a warming tundra biome. Nature 562, 57–62. doi: 10.1038/s41586-018-0563-7
Borgy, B., Violle, C., Choler, P., Garnier, E., Kattge, J., Loranger, J., et al. (2017). Sensitivity of community-level trait–environment relationships to data representativeness: a test for functional biogeography. Glob. Ecol. Biogeogr. 26, 729–739. doi: 10.1111/geb.12573
Bowman, W. D., Bahn, L., and Damm, M. (2003). Alpine landscape variation in foliar nitrogen and phosphorus concentrations and the relation to soil nitrogen and phosphorus availability. Arct. Antarct. Alp. Res. 35, 144–149. doi: 10.1657/1523-04302003035
Bradford, J. B., and Damato, A. W. (2012). Recognizing trade-offs in multi-objective land management. Front. Ecol. Environ. 10:210–216. doi: 10.1890/110031
Buzzard, V., Michaletz, S. T., Deng, Y., He, Z., Ning, D., Shen, L., et al. (2019). Continental scale structuring of forest and soil diversity via functional traits. Nat. Ecol. Evol. 3, 1298–1308. doi: 10.1038/s41559-019-0954-7
Carvajal, D. E., Loayza, A. P., Rios, R. S., Delpiano, C. A., and Squeo, F. A. (2019). A hyper-arid environment shapes an inverse pattern of the fast–slow plant economics spectrum for above-, but not below-ground resource acquisition strategies. J. Ecol. 107, 1079–1092. doi: 10.1111/1365-2745.13092
Chen, L. T., Niu, K. C., Wu, Y., Geng, Y., Mi, Z. R., Flynn, D. F. B., et al. (2013). UV radiation is the primary factor driving the variation in leaf phenolics across Chinese grasslands. Ecol. Evol. 3, 4696–4710. doi: 10.1002/ece3.862
Decker, M., Pitman, A. J., and Evans, J. P. (2013). Groundwater constraints on simulated transpiration variability over southeastern Australian forests. J. Hydrometeorol. 14, 543–559. doi: 10.1175/JHM-D-12-058.1
Diaz, S., Kattge, J., Cornelissen, J. H. C., Wright, I. J., Lavorel, S., Dray, S., et al. (2016). The global spectrum of plant form and function. Nature 529, 167–171. doi: 10.1038/nature16489
Donovan, L. A., Maherali, H., Caruso, C. M., Huber, H., and De Kroon, H. (2011). The evolution of the worldwide leaf economics spectrum. Trends Ecol. Evol. 26, 88–95. doi: 10.1016/j.tree.2010.11.011
Evans, J. R., and Poorter, H. (2001). Photosynthetic acclimation of plants to growth irradiance: the relative importance of specific leaf area and nitrogen partitioning in maximizing carbon gain. Plant Cell Environ. 24, 755–767. doi: 10.1046/j.1365-3040.2001.00724.x
Farooq, M., Wahid, A., Kobayashi, N., Fujita, D., and Basra, S. M. A. (2009). Plant drought stress: effects, mechanisms and management. Agron. Sustain. Dev. 29, 185–212. doi: 10.1051/agro:2008021
Fischer, A. G. (1960). Latitudinal variation in organic diversity. Evolution 14, 64–81. doi: 10.1111/j.1558-5646.1960.tb03057.x
Fonseca, C. R., Overton, J. M., Collins, B., and Westoby, M. (2000). Shifts in trait-combinations along rainfall and phosphorus gradients. J. Ecol. 88, 964–977. doi: 10.1046/j.1365-2745.2000.00506.x
Geng, Y., Wang, Z. H., Liang, C. Z., Fang, J. Y., Baumann, F., Kuhn, P., et al. (2012). Effect of geographical range size on plant functional traits and the relationships between plant, soil and climate in Chinese grasslands. Glob. Ecol. Biogeogr. 21, 416–427. doi: 10.1111/j.1466-8238.2011.00692.x
Gong, H. D., and Gao, J. (2019). Soil and climatic drivers of plant SLA (specific leaf area). Glob. Ecol. Conserv. 20:e00696. doi: 10.1016/j.gecco.2019.e00696
Griffinnolan, R. J., Blumenthal, D. M., Collins, S. L., Farkas, T. E., Hoffman, A. M., Mueller, K. E., et al. (2019). Shifts in plant functional composition following long-term drought in grasslands. J. Ecol. 107, 2133–2148. doi: 10.1111/1365-2745.13252
Guo, Q. F. (2003). Temporal species richness-biomass relationships along successional gradients. J. Veg. Sci. 14, 121–128. doi: 10.1658/1100-92332003014
Gusewell, S., and Koerselman, W. (2002). Variation in nitrogen and phosphorus concentrations of wetland plants. Perspect. Plant Ecol. Evol. Syst. 5, 37–61. doi: 10.1078/1433-8319-0000022
Han, W. X., Fang, J. Y., Guo, D., and Zhang, Y. (2005). Leaf nitrogen and phosphorus stoichiometry across 753 terrestrial plant species in China. New Phytol. 168, 377–385. doi: 10.1111/j.1469-8137.2005.01530.x
He, J. S., Wang, X. P., Flynn, D. F. B., Wang, L., Schmid, B., and Fang, J. Y. (2009). Taxonomic, phylogenetic, and environmental trade-offs between leaf productivity and persistence. Ecology 90, 2779–2791. doi: 10.1890/08-1126.1
He, J. S., Wang, Z. H., Wang, X. P., Schmid, B., Zuo, W., Zhou, M., et al. (2006). A test of the generality of leaf trait relationships on the Tibetan Plateau. New Phytol. 170, 835–848. doi: 10.1111/j.1469-8137.2006.01704.x
Hickler, T., Fronzek, S., Araujo, M. B., Schweiger, O., Thuiller, W., and Sykes, M. T. (2009). An ecosystem model-based estimate of changes in water availability differs from water proxies that are commonly used in species distribution models. Glob. Ecol. Biogeogr. 18, 304–313. doi: 10.1111/j.1466-8238.2009.00455.x
Jentsch, A., and Beierkuhnlein, C. (2008). Research frontiers in climate change : effects of extreme meteorological events on ecosystems. C. R. Geosci. 340, 621–628. doi: 10.1016/j.crte.2008.07.002
Kerkhoff, A. J., Enquist, B. J., Elser, J. J., and Fagan, W. F. (2005). Plant allometry, stoichiometry and the temperature-dependence of primary productivity. Glob. Ecol. Biogeogr. 14, 585–598. doi: 10.1111/j.1466-822X.2005.00187.x
Li, C. L., Hao, X. Y., Zhao, M. L., Han, G. D., and Willms, W. D. (2008). Influence of historic sheep grazing on vegetation and soil properties of a Desert Steppe in Inner Mongolia. Agric. Ecosyst. Environ. 128, 109–116. doi: 10.1016/j.agee.2008.05.008
Li, P., Deng, W., Zhang, H., Sun, J., and Xiong, J. N. (2019). Focus on economy or ecology? A three-dimensional trade-off based on ecological carrying capacity in southwest China. Nat. Resour. Model. 32:e12201. doi: 10.1111/nrm.12201
Lu, M., Yang, Y. H., Luo, Y. Q., Fang, C. M., Zhou, X. H., Chen, J. K., et al. (2011). Responses of ecosystem nitrogen cycle to nitrogen addition: a meta-analysis. New Phytol. 189, 1040–1050. doi: 10.1111/j.1469-8137.2010.03563.x
Lusk, C. H., Reich, P. B., Montgomery, R. A., Ackerly, D. D., and Cavenderbares, J. M. (2008). Why are evergreen leaves so contrary about shade. Trends Ecol. Evol. 23, 299–303. doi: 10.1016/j.tree.2008.02.006
Marriott, C. A., Bolton, G. R., Barthram, G. T., Fisher, J. M., and Hood, K. (2002). Early changes in species composition of upland sown grassland under extensive grazing management. Appl. Veg. Sci. 5, 87–98. doi: 10.1111/j.1654-109X.2002.tb00538.x
Michaletz, S. T., Weiser, M. D., Mcdowell, N. G., Zhou, J., Kaspari, M., Helliker, B. R., et al. (2016). The energetic and carbon economic origins of leaf thermoregulation. Nat. Plants 2:16129. doi: 10.1038/nplants.2016.129
Moles, A. T., Perkins, S., Laffan, S. W., Floresmoreno, H., Awasthy, M., Tindall, M. L., et al. (2014). Which is a better predictor of plant traits: temperature or precipitation? J. Veg. Sci. 25, 1167–1180. doi: 10.1111/jvs.12190
Nasto, M. K., Alvarezclare, S., Lekberg, Y., Sullivan, B. W., Townsend, A. R., and Cleveland, C. C. (2014). Interactions among nitrogen fixation and soil phosphorus acquisition strategies in lowland tropical rain forests. Ecol. Lett. 17, 1282–1289. doi: 10.1111/ele.12335
Niinemets, U. (2001). Global-scale climatic controls of leaf dry mass per area, density, and thickness in trees and shrubs. Ecology 82, 453–469.
Niklas, K. J., Owens, T. G., Reich, P. B., and Cobb, E. D. (2005). Nitrogen/phosphorus leaf stoichiometry and the scaling of plant growth. Ecol. Lett. 8, 636–642. doi: 10.1111/j.1461-0248.2005.00759.x
Ordonez, A., and Olff, H. (2013). Do alien plant species profit more from high resource supply than natives? A trait-based analysis. Glob. Ecol. Biogeogr. 22, 648–658. doi: 10.1111/geb.12019
Ordonez, J. C., Van Bodegom, P. M., Witte, J. P. M., Wright, I. J., Reich, P. B., and Aerts, R. (2009). A global study of relationships between leaf traits, climate and soil measures of nutrient fertility. Glob. Ecol. Biogeogr. 18, 137–149. doi: 10.1111/j.1466-8238.2008.00441.x
Pearson, R. G., Phillips, S. J., Loranty, M. M., Beck, P. S. A., Damoulas, T., Knight, S. J., et al. (2013). Shifts in Arctic vegetation and associated feedbacks under climate change. Nat. Clim. Chang. 3, 673–677. doi: 10.1038/nclimate1858
Quan, Q., Tian, D. S., Luo, Y. Q., Zhang, F. Y., Crowther, T. W., Zhu, K., et al. (2019). Water scaling of ecosystem carbon cycle feedback to climate warming. Sci. Adv. 5:eaav1131. doi: 10.1126/sciadv.aav1131
Quesada, C. A., Lloyd, J., Schwarz, M., Baker, T. R., Phillips, O. L., Patino, S., et al. (2009). Regional and large-scale patterns in Amazon forest structure and function are mediated by variations in soil physical and chemical properties. Biog. Disc. 6, 3993–4057. doi: 10.5194/bgd-6-3993-2009
R Core Team (2016). R: A language and Environment for Statistical Computing. Vienna: R Foundation for Statistical Computing.
Reed, S. C., Cleveland, C. C., and Townsend, A. R. (2011). Functional ecology of free-living nitrogen fixation: a contemporary perspective. Annu. Rev. Ecol. Evol. Syst. 42, 489–512. doi: 10.1146/annurev-ecolsys-102710-145034
Reich, P. B., and Oleksyn, J. (2004). Global patterns of plant leaf N and P in relation to temperature and latitude. Proc. Natl. Acad. Sci. U.S.A. 101, 11001–11006. doi: 10.1073/pnas.0403588101
Reich, P. B., Oleksyn, J., and Wright, I. J. (2009). Leaf phosphorus influences the photosynthesis–nitrogen relation: a cross-biome analysis of 314 species. Oecologia 160, 207–212. doi: 10.1007/s00442-009-1291-3
Reich, P. B., Wright, I. J., Cavenderbares, J. M., Craine, J. M., Oleksyn, J., Westoby, M., et al. (2003). The evolution of plant functional variation: traits, spectra, and strategies. Int. J. Plant Sci. 164, 143–164. doi: 10.1086/374368
Reichstein, M., Bahn, M., Mahecha, M. D., Kattge, J., and Baldocchi, D. D. (2014). Linking plant and ecosystem functional biogeography. Proc. Natl. Acad. Sci. U.S.A. 111, 13697–13702. doi: 10.1073/pnas.1216065111
Santiago, L. S., and Wright, S. J. (2007). Leaf functional traits of tropical forest plants in relation to growth form. Funct. Ecol. 21, 19–27. doi: 10.1111/j.1365-2435.2006.01218.x
Sardans, J., and Penuelas, J. (2004). Increasing drought decreases phosphorus availability in an evergreen Mediterranean forest. Plant Soil 267, 367–377. doi: 10.1007/s11104-005-0172-8
Schuur, E. A. G., and Matson, P. A. (2001). Net primary productivity and nutrient cycling across a mesic to wet precipitation gradient in Hawaiian montane forest. Oecologia 128, 431–442. doi: 10.2307/4223025
Schwinning, S., and Ehleringer, J. R. (2001). Water use trade-offs and optimal adaptations to pulse-driven arid ecosystems. J. Ecol. 89, 464–480. doi: 10.1046/j.1365-2745.2001.00576.x
Shangguan, W., Dai, Y. J., Liu, B. Y., Zhu, A. X., Duan, Q. Y., Wu, L. Z., et al. (2013). A China data set of soil properties for land surface modeling. J. Adv. Model. Earth Syst. 5, 212–224. doi: 10.1002/jame.20026
Shipley, B., De Bello, F., Cornelissen, J. H. C., Laliberte, E., Laughlin, D. C., and Reich, P. B. (2016). Reinforcing loose foundation stones in trait-based plant ecology. Oecologia 180, 923–931. doi: 10.1007/s00442-016-3549-x
Shipley, B., Lechowicz, M. J., Wright, I. J., and Reich, P. B. (2006). Fundamental trade-offs generating the worldwide leaf economics spectrum. Ecology 87, 535–541. doi: 10.2307/20068973
Simova, I., Rueda, M., and Hawkins, B. A. (2017). Stress from cold and drought as drivers of functional trait spectra in North American angiosperm tree assemblages. Ecol. Evol. 7, 7548–7559. doi: 10.1002/ece3.3297
Simova, I., Violle, C., Kraft, N. J. B., Storch, D., Svenning, J., Boyle, B., et al. (2015). Shifts in trait means and variances in North American tree assemblages: species richness patterns are loosely related to the functional space. Ecography 38, 649–658. doi: 10.1111/ecog.00867
Simova, I., Violle, C., Svenning, J., Kattge, J., Engemann, K., Sandel, B., et al. (2018). Spatial patterns and climate relationships of major plant traits in the New World differ between woody and herbaceous species. J. Biogeogr. 45, 895–916. doi: 10.1111/jbi.13171
Stott, P. A., Christidis, N., Otto, F. E. L., Sun, Y., Vanderlinden, J., Van Oldenborgh, G. J., et al. (2016). Attribution of extreme weather and climate-related events. Wiley Interdiscip. Rev. Clim. Chang. 7, 23–41. doi: 10.1002/wcc.380
Stubbs, W. J., and Wilson, J. B. (2004). Evidence for limiting similarity in a sand dune community. J. Ecol. 92, 557–567. doi: 10.1111/j.0022-0477.2004.00898.x
Sun, J., and Du, W. P. (2017). Effects of precipitation and temperature on net primary productivity and precipitation use efficiency across China’s grasslands. GISci. Remote Sens. 54, 881–897. doi: 10.1080/15481603.2017.1351147
Sun, J., Liu, B. Y., You, Y., Li, W. P., Liu, M., Shang, H., et al. (2019). Solar radiation regulates the leaf nitrogen and phosphorus stoichiometry across alpine meadows of the Tibetan Plateau. Agr. For. Meteorol. 271, 92–101. doi: 10.1016/j.agrformet.2019.02.041
Sun, J., Ma, B. B., and Lu, X. Y. (2018). Grazing enhances soil nutrient effects: trade-offs between aboveground and belowground biomass in alpine grasslands of the Tibetan Plateau. Land Degrad. Dev. 29, 337–348. doi: 10.1002/ldr.2822
Sun, J., Zhou, T. C., Liu, M., Chen, Y., Liu, G., Xu, M., et al. (2020). Water and heat availability are drivers of the aboveground plant carbon accumulation rate in alpine grasslands on the Tibetan Plateau. Glob. Ecol. Biogeogr. 29, 50–64. doi: 10.1111/geb.13006
Swenson, N. G., Enquist, B. J., Pither, J., Kerkhoff, A. J., Boyle, B., Weiser, M. D., et al. (2012). The biogeography and filtering of woody plant functional diversity in North and South America. Glob. Ecol. Biogeogr. 21, 798–808. doi: 10.1111/j.1466-8238.2011.00727.x
Takashima, T., Hikosaka, K., and Hirose, T. (2004). Photosynthesis or persistence: nitrogen allocation in leaves of evergreen and deciduous Quercus species. Plant Cell Environ. 27, 1047–1054. doi: 10.1111/j.1365-3040.2004.01209.x
Thornthwaite, C. W. (1948). An approach toward a rational classification of climate. Geogr. Rev. 38, 55–94. doi: 10.1111/10.2307/210739
Thuiller, W., Lavorel, S., Midgley, G. F., Lavergne, S., and Rebelo, T. (2004). Relating plant traits and species distributions along bioclimatic gradients for 88 Leucadendron taxa. Ecology 85, 1688–1699. doi: 10.1890/03-0148
Van Bodegom, P. M., Callaghan, T. V., Chapin, F. S., Gerdol, R., Gwynn, D., Hartley, A. E., et al. (2007). Global negative vegetation feedback to climate warming responses of leaf litter decomposition rates in cold biomes. Ecol. Lett. 10, 619–627. doi: 10.1111/j.1461-0248.2007.01051.x
Violle, C., Reich, P. B., Pacala, S. W., Enquist, B. J., and Kattge, J. (2014). The emergence and promise of functional biogeography. Proc. Natl. Acad. Sci. U.S.A. 111, 13690–13696. doi: 10.1073/pnas.1415442111
Waite, M., and Sack, L. (2010). How does moss photosynthesis relate to leaf and canopy structure? Trait relationships for 10 Hawaiian species of contrasting light habitats. New Phytol. 185, 156–172. doi: 10.1111/j.1469-8137.2009.03061.x
Wang, C. T., Long, R. J., Wang, Q. L., Jing, Z. C., and Shi, J. J. (2009). Changes in plant diversity, biomass and soil C, in alpine meadows at different degradation stages in the headwater region of three rivers, China. Land Degrad. Dev. 20, 187–198. doi: 10.1002/ldr.879
Wang, G. H. (2007). Leaf trait co-variation, response and effect in a chronosequence. J. Veg. Sci. 18, 563–570. doi: 10.1111/j.1654-1103.2007.tb02570.x
Wellstein, C., Poschlod, P., Gohlke, A., Chelli, S., Campetella, G., Rosbakh, S., et al. (2017). Effects of extreme drought on specific leaf area of grassland species: a meta-analysis of experimental studies in temperate and sub-Mediterranean systems. Glob. Chang. Biol. 23, 2473–2481. doi: 10.1111/gcb.13662
Werden, L. K., Waring, B. G., Smithmartin, C. M., and Powers, J. S. (2018). Tropical dry forest trees and lianas differ in leaf economic spectrum traits but have overlapping water-use strategies. Tree Physiol. 38, 517–530. doi: 10.1093/treephys/tpx135
Westoby, M., and Wright, I. J. (2006). Land-plant ecology on the basis of functional traits. Trends Ecol. Evol. 21, 261–268. doi: 10.1016/j.tree.2006.02.004
White, T. A., Campbell, B. D., Kemp, P. D., and Hunt, C. L. (2000). Sensitivity of three grassland communities to simulated extreme temperature and rainfall events. Glob. Chang. Biol. 6, 671–684. doi: 10.1046/j.1365-2486.2000.00344.x
Wright, I. J., Reich, P. B., Atkin, O. K., Lusk, C. H., Tjoelker, M. G., and Westoby, M. (2006). Irradiance, temperature and rainfall influence leaf dark respiration in woody plants: evidence from comparisons across 20 sites. New Phytol. 169, 309–319. doi: 10.1111/j.1469-8137.2005.01590.x
Wright, I. J., Reich, P. B., Cornelissen, J. H. C., Falster, D. S., Groom, P. K., Hikosaka, K., et al. (2005). Modulation of leaf economic traits and trait relationships by climate. Glob. Ecol. Biogeogr. 14, 411–421. doi: 10.1111/j.1466-822x.2005.00172.x
Wright, I. J., Reich, P. B., and Westoby, M. (2001). Strategy shifts in leaf physiology, structure and nutrient content between species of high- and low-rainfall and high- and low-nutrient habitats. Funct. Ecol. 15, 423–434. doi: 10.2307/826662
Wright, I. J., Reich, P. B., Westoby, M., Ackerly, D. D., Baruch, Z., Bongers, F., et al. (2004). The worldwide leaf economics spectrum. Nature 428, 821–827. doi: 10.1038/nature02403
Wright, J. P., and Suttongrier, A. E. (2012). Does the leaf economic spectrum hold within local species pools across varying environmental conditions. Funct. Ecol. 26, 1390–1398. doi: 10.1111/1365-2435.12001
Wright, S. J., Kitajima, K., Kraft, N. J. B., Reich, P. B., Wright, I. J., Bunker, D. E., et al. (2010). Functional traits and the growth-mortality trade-off in tropical trees. Ecology 91, 3664–3674. doi: 10.1890/09-2335.1
Xie, Y. Z. (2011). Water Holding Capacity and Capita Storage of Plant Community Litter in Representative Desert Steppe. J. Soil Water Conserv. 25, 144–147.
Xu, J. W. (2003). Effect of low-light stress on nitrogen accumulation, distribution and grains protein content of Indica hybrid. Plant Nutr. Fert. Sci. 9, 288–293.
Yue, K., Peng, Y., Fornara, D. A., Van Meerbeek, K., Vesterdal, L., Yang, W. Q., et al. (2019). Responses of nitrogen concentrations and pools to multiple environmental change drivers: a meta-analysis across terrestrial ecosystems. Glob. Ecol. Biogeogr. 28, 690–724. doi: 10.1111/geb.12884
Zhang, C. C., Li, X. Y., Wu, H. W., Wang, P., Wang, Y., Wu, X. C., et al. (2017). Differences in water-use strategies along an aridity gradient between two coexisting desert shrubs (Reaumuria soongorica and Nitraria sphaerocarpa): isotopic approaches with physiological evidence. Plant Soil 419, 169–187. doi: 10.1007/s11104-017-3332-8
Zhang, C. J., Liao, Y. M., Duan, J. Q., Song, Y. L., Huang, D. P., Sheng, W., et al. (2016). The Progresses of Dry-Wet Climate Divisional Research in China. Clim. Chang. Res. 12, 261–267.
Zhang, F. M., and Shen, S. H. (2008). A Study on Dry/Wet Conditions and Changes of Dry/Wet Climate Boundary in China. J. Nanj. Inst. Met. 31, 574–579. doi: 10.3724/SP.J.1047.2008.00014
Zhang, F. Y., Quan, Q., Ma, F. F., Tian, D. S., Hoover, D. L., Zhou, Q. P., et al. (2019). When does extreme drought elicit extreme ecological responses. J. Ecol. 107, 2553–2563. doi: 10.1111/1365-2745.13226
Zhang, S. S., Zheng, Q., Noll, L., Hu, Y. T., and Wanek, W. (2019). Environmental effects on soil microbial nitrogen use efficiency are controlled by allocation of organic nitrogen to microbial growth and regulate gross N mineralization. Soil Biol. Biochem. 135, 304–315. doi: 10.1016/j.soilbio.2019.05.019
Zhang, X. L., Zhou, J. H., Guan, T. Y., Cai, W. T., Jiang, L. H., Lai, L. M., et al. (2019). Spatial variation in leaf nutrient traits of dominant desert riparian plant species in an arid inland river basin of China. Ecol. Evol. 9, 1523–1531. doi: 10.1002/ece3.4877
Zheng, M. H., Chen, H., Li, D. J., Luo, Y. Q., and Mo, J. M. (2019). Substrate stoichiometry determines nitrogen fixation throughout succession in southern Chinese forests. Ecol. Lett. 23, 336–347. doi: 10.1111/ele.13437
Zheng, Q., Hu, Y. T., Zhang, S. S., Noll, L., Bockle, T., Richter, A., et al. (2019). Growth explains microbial carbon use efficiency across soils differing in land use and geology. Soil Biol. Biochem. 128, 45–55. doi: 10.1016/j.soilbio.2018.10.006
Keywords: herbaceous plant, leaf trait, growth strategy, abiotic filtering, environmental stress, climatic region
Citation: Zhang Z, Sun J, Liu M, Shang H, Wang J, Wang J, Zhou H, Li Y, Wang Y and Chen W (2022) Context-Dependency in Relationships Between Herbaceous Plant Leaf Traits and Abiotic Factors. Front. Plant Sci. 13:757077. doi: 10.3389/fpls.2022.757077
Received: 11 August 2021; Accepted: 21 February 2022;
Published: 25 March 2022.
Edited by:
Boris Rewald, University of Natural Resources and Life Sciences Vienna, AustriaReviewed by:
Amber Churchill, University of Minnesota, United StatesCopyright © 2022 Zhang, Sun, Liu, Shang, Wang, Wang, Zhou, Li, Wang and Chen. This is an open-access article distributed under the terms of the Creative Commons Attribution License (CC BY). The use, distribution or reproduction in other forums is permitted, provided the original author(s) and the copyright owner(s) are credited and that the original publication in this journal is cited, in accordance with accepted academic practice. No use, distribution or reproduction is permitted which does not comply with these terms.
*Correspondence: Jian Sun, c3VuamlhbkBpcHRjYXMuYWMuY24=
Disclaimer: All claims expressed in this article are solely those of the authors and do not necessarily represent those of their affiliated organizations, or those of the publisher, the editors and the reviewers. Any product that may be evaluated in this article or claim that may be made by its manufacturer is not guaranteed or endorsed by the publisher.
Research integrity at Frontiers
Learn more about the work of our research integrity team to safeguard the quality of each article we publish.