- 1National Key Laboratory of Crop Genetic Improvement, Huazhong Agricultural University, Wuhan, China
- 2Hubei Hongshan Laboratory, Wuhan, China
Flowering time is strongly related to the environment, while the genotype-by-environment interaction study for flowering time is lacking in Brassica napus. Here, a total of 11,700,689 single nucleotide polymorphisms in 490 B. napus accessions were used to associate with the flowering time and related climatic index in eight environments using a compressed variance-component mixed model, 3VmrMLM. As a result, 19 stable main-effect quantitative trait nucleotides (QTNs) and 32 QTN-by-environment interactions (QEIs) for flowering time were detected. Four windows of daily average temperature and precipitation were found to be climatic factors highly correlated with flowering time. Ten main-effect QTNs were found to be associated with these flowering-time-related climatic indexes. Using differentially expressed gene (DEG) analysis in semi-winter and spring oilseed rapes, 5,850 and 5,511 DEGs were found to be significantly expressed before and after vernalization. Twelve and 14 DEGs, including 7 and 9 known homologs in Arabidopsis, were found to be candidate genes for stable QTNs and QEIs for flowering time, respectively. Five DEGs were found to be candidate genes for main-effect QTNs for flowering-time-related climatic index. These candidate genes, such as BnaFLCs, BnaFTs, BnaA02.VIN3, and BnaC09.PRR7, were further validated by the haplotype, selective sweep, and co-expression networks analysis. The candidate genes identified in this study will be helpful to breed B. napus varieties adapted to particular environments with optimized flowering time.
Introduction
As the world’s most important oilseed crop, planting of Brassica napus spans a wide range of growth periods and climate zones (Yang et al., 2014). To meet the needs of adaptation, B. napus adjusts the correct time to flower. Flowering time determines the transition from the vegetative to the reproductive phase, and therefore, the nutrients are available for remobilization at seed filling (Han et al., 2021). Early flowering facilitates mechanical harvesting and rotation with other crops, whereas late flowering enhances stem development, thus improving lodging resistance (Cui et al., 2021). Although previous studies have revealed the genetic basis of flowering time in B. napus, no studies have been reported on the genetic dissection of flowering time plasticity, namely, genotype-by-environment interaction (G by E).
The genetic basis of flowering time has been well-studied in the model plant Arabidopsis thaliana (Mouradov et al., 2002; Putterill et al., 2004; Bouché et al., 2016). The genetic networks underlying flowering consist of six major pathways interconnected, namely, photoperiod, vernalization, gibberellin, autonomous, thermal clock, and aging pathways (Putterill et al., 2004). Epigenetic regulation, miRNAs, phytohormones, sugar status, and signaling also play important roles in flowering time control (Bouché et al., 2016). In B. napus, the polyploid nature of B. napus has resulted in flowering-time-related genes undergoing extensive subfunctionalization (Schiessl, 2020). It has been demonstrated that there is a sophisticated network of interactions among FLOWERING LOCUS C homologs with different expression patterns in organs and development stages (Zou et al., 2012). LOWERING LOCUS T and TERMINAL FLOWER 1 were found to have pleiotropic effects on flowering time, despite their redundancy in B. napus genome (Guo et al., 2014). Therefore, it demands more genetic basis research on flowering time in B. napus.
Flowering time is strongly influenced by the environment. A decrease in day length delays flowering in B. napus. A period of cooler temperature will determine vernalization and ensure reproductive development (Matar et al., 2021). Precipitation has been reported to have different effects on flowering phenology in different species (Zhang et al., 2018). Many genes have been reported to influence flowering time in response to the environment. FLOWERING LOCUS T (FT) was found to induce flowering through long-distance signaling by activating seasonal changes in day length (Corbesier et al., 2007). The epigenetic silencing of FLC accelerates flowering by prolonged cold vernalization (Bastow et al., 2004). H2A.Z incorporates BraA.FT.a chromatin at high ambient temperature and delays flowering time in B. rapa (Del Olmo et al., 2019). In B. napus, Cycling Dof Factor1 delays the flowering time and was induced in response to low temperature (Xu and Dai, 2016). BnNAC485 altered flowering time in response to abiotic stress (Ying et al., 2014). However, B. napus has developed two eco-types in China, namely, semi-winter oilseed rapes (SWORs) and spring oilseed rapes (SORs), to adapt different geographical environments and climates, leading to more complex molecular mechanisms of flowering time (Song et al., 2020).
In response to climate change, G by E is of fundamental importance in plant breeding and adaptation (Arnold et al., 2019; Zhao et al., 2022). In B. napus, the G by E of seed yield and oil content were found to exert specific adaptation to climates (Zhang et al., 2013a). Genotype and temperature interactions of seed oil content were found to be differential at the level of gene expression profiles (Zhu et al., 2012). Moreover, quantitative and population genetics have shown great power to bridge the gap between genomic diversity and phenotypic plasticity (Wu, 1998; Kusmec et al., 2017; Liu et al., 2021). For G by E studies on flowering time, four environmentally sensitive quantitative trait loci for flowering time identified in 473 Arabidopsis accessions were found to be related to adaptation (Li et al., 2010). It has been found that interacting flowering-time-related genes differentially respond to the temperature at the early growth stage in rice (Guo et al., 2020). Quantitative trait nucleotide (QTN)-by-environment interaction (QEI) mapping for flowering time has been performed in a doubled haploid B. napus population (Shen et al., 2018). Although many genome-wide association studies (GWAS) for flowering time have been reported in B. napus (Xu et al., 2016; Song et al., 2020; Helal et al., 2021; Hu et al., 2022), knowledge about QEI for flowering time detected by GWAS is scarce.
Recently, the newly published method 3VmrMLM provides a solution for QEI detection in GWAS (Li et al., 2022a). Here, we investigated the landscape of flowering time plasticity of 490 B. napus accessions in eight environments. A total of 11,700,689 single nucleotide polymorphisms (SNPs) were used to detect main-effect QTNs for flowering time and related climatic index and QEIs for flowering time. The transcriptome of SWORs and SORs before and after vernalization was used to identify the candidate genes around QTNs and QEIs. Co-expression, haplotype, and selection sweep analysis were used to further validate the candidate flowering time genes in specific eco-oilseed rapes. Our finding will facilitate the breeding for adaptation to particular environments with optimized flowering time in B. napus.
Materials and methods
Germplasm, phenotypic, and genomic data
A diversity panel of 490 B. napus accessions collected from Xu et al. (2016) was used in this study. This panel was cultivated in eight natural environments, i.e., Wuhan 2013 and 2014 (WH2013 and WH2014), Changsha 2013 and 2014 (CS2013 and CS2014), Nanjing 2013 and 2014 (NJ2013 and NJ2014), Ezhou 2013 (EZ2013), and Chongqing 2013 (CQ2013). Additionally, the Gangan and ZS11 cultivars for RNA-seq were planted in Wuhan 2018 at the experimental stations of Huazhong Agricultural University. The design of field trial of the above materials and the acquisition of phenotypic data were the same as those used in the previous study (Xu et al., 2016). The re-sequencing genome data were obtained from Tang et al. (2021). The B. napus genome (B. napus ZS11 v0) from BnPIR (Song et al., 2020; Song et al., 2021) (http://cbi.hzau.edu.cn/bnapus/index.php) was used as the reference genome.
Statistical analysis for phenotypic data
By using the “lme4” R package (Bates et al., 2015), the best linear unbiased prediction (BLUP) model was fitted to each B. napus accession:
Taking into account the variations between eight environments as phenotypic variance derived from environmental factors, broad-sense heritability () was estimated using the following equation by treating populations as a random effect and the environments as an environment effect, where and is the variance derived from genetic and environmental effects, respectively (Knapp et al., 1985).
Identification of flowering-time-related climatic index
Climatic data for daily average temperature (TAVG, °F) and precipitation (PRCP, in) were retrieved from the National Oceanic and Atmospheric Administration (https://www.noaa.gov/weather). Due to the lack of climatic data for Ezhou, there were climatic datasets of seven environments in total, i.e., WH2013 and WH2014 (114.05°E, 30.60°N; Station ID: GHCND: CHM00057494), CS2013 and CS2014 (112.87°E, 28.23°N; GHCND: CHM00057687), NJ2013 and NJ2014 (118.90°E, 31.93°N; GHCND: CHM00058238), and CQ2013 (106.48°E, 29.58°N; GHCND: CHM00057516). Climatic data were obtained from the day after being planted to the 200 days after planting (DAP). For each window from a starting day (3 DAP) to an end day (41 DAP) during B. napus growth, the average value of the climatic index and their correlation with the environmental mean vector for flowering time was calculated by CERIS analytical package (Li et al., 2021; https://github.com/jmyu/CERIS_JGRA). The most relevant climatic index for flowering time was chosen according to the highest correlation between environmental means and climatic index with corresponding window. Reaction norms were calculated as described in Guo et al. (2020) and Liu et al. (2020), using environmental mean and environmental climatic index as x-axis and phenotype as y-axis. Each line represented an individual and was shown by fitted linear regression. The intercept and slope were used to perform GWAS further.
Detecting QTNs and QEIs by GWAS
The intersection of the accessions in phenotypic and genotypic datasets, i.e., 490 accessions with 11,700,689 SNPs, were used for GWAS using 3VmrMLM (Li et al., 2022a) via software IIIVmrMLM (Li et al., 2022b). Flowering time QTNs were obtained from separate analyses of phenotypic data from eight environments and joint environmental analyses of these datasets. The reaction norms between flowering time and climatic index were also used to conduct GWAS by 3VmrMLM. QEIs for flowering time were obtained by joint environment analyses of the above phenotypic datasets in eight environments. Population structure and kinship matrix were considered in 3VmrMLM analysis, and the “svpal” parameter was set as 0.01. According to Tang et al. (2021), the population structure calculated as K=3 was used in the analysis. The threshold was set at 0.05/m for significant QTNs and QEIs and LOD score ≥ 3.0 for suggested QTNs and QEIs, where m is the number of markers (Li et al., 2022a; Li et al., 2022b). According to the LD interval estimated by Tang et al. (2021), stable QTNs were defined as QTNs identified in at least three environments within the 100-kb upstream and downstream regions.
Identification of candidate genes
To identify candidate genes for flowering-time-related QTNs and QEIs, genes within the 100 kb upstream and downstream regions of each QTN or QEI were extracted according to the LD interval estimated by Tang et al. (2021). Then, two strategies were employed. First, the B. napus homologs of Arabidopsis flowering time genes downloaded from FLOR-ID (http://www.flor-id.org) were selected and considered as known genes. Second, new candidate genes were identified using differentially expressed genes (DEGs) in two SWORs (Gangan and ZS11) before and after vernalization and in two SORs (Westar and No. 2127). The t-test was adopted in the hypothesis testing for haplotype analysis; p< 0.05, p< 0.01, and p< 0.001 indicated the significances at 0.05, 0.01, and 0.001 probability levels, respectively.
Differential expression analysis based on RNA-seq
The leaves of Westar, No. 2127, Gangan, ZS11 at 24 and 147 DAP were collected for RNA-seq with two biological replicates. Total RNA was extracted using the TIANGEN RNAprep Pure Plant Kit. Sequencing libraries were generated using the NEBNext® UltraTM RNA Library Prep Kit for Illumina® (NEB, USA) and were sequenced on an Illumina Hiseq 4000 platform. The detailed processes were described in Tan et al. (2022). We used MultiQC (Ewels et al., 2016) to perform quality control and Salmon (Patro et al., 2017) to quantify the RNA-seq reads of annotated genes in the reference ZS11. DESeq2 was used for differential expression analysis (Love et al., 2014). The threshold for DEG is set as the absolute value of log2FoldChange >1 and adjusted p< 0.05 (two-tailed Student’s t-test; Tan et al., 2022).
Identification of selective sweep signals
To detect the regions under selective sweeps between SWOR and SOR, XP-CLR (v1.1.1), a genome scan using the composite likelihood approach was performed in sub-populations (Chen et al., 2010). Each chromosome was analyzed using the XP-CLR command with the parameters “–ld 0.99 –phased –maxsnps 200 –size 100000 –step 10000.” Non-overlapping 20-kp windows within the top 20% XP-CLR scores were merged into one single region, and then, these regions in the top 1% of XP-CLR scores were considered as candidate selective regions (An et al., 2019).
Construction of co-expression network
According to the above RNA-seq datasets, Pearson correlation analysis was calculated between candidate genes and DEGs in SWORs and SORs, respectively. Significant genes were considered to be co-expressed when Pearson correlation coefficient was >0.80 and p-value was<0.05. Network visualization was implemented with the Cytoscape package (Shannon et al., 2003).
Results
Flowering time plasticity and related climatic index for B. napus
Complex flowering time variation was observed in diversity group of 490 B. napus oilseed rapes, including 49 SORs, 20 winter oilseed rapes, 326 SWORs, and 95 mixed type oilseed rapes, grown in eight natural environments (Figure 1A; Supplementary Table S1). The means plus standard deviations of the eight environments WH2013, WH2014, CS2013, CS2014, NJ2013, NJ2014, CQ2013, EZ2013, and BLUP values were 155.49 ± 3.80, 153.56 ± 9.61, 160.27 ± 4.29, 166.55 ± 5.44, 160.50 ± 5.49, 167.55 ± 6.38, 151.31 ± 7.82, 162.57 ± 5.30, and 159.68 ± 4.83 (DAP), respectively (Figure 1B). The correlation of each pair of environments ranged from 0.37 to 0.72 (0.50 ± 0.09). The coefficients of variation, skewness, and kurtosis of the trait in eight environments illustrated that flowering time is a typical quantitative trait (Supplementary Table S2). The broad-sense heritability for flowering time is 0.86. More importantly, joint regression analysis modeled with environmental mean showed the presence of a significant phenotypic plasticity (Figure 1C).
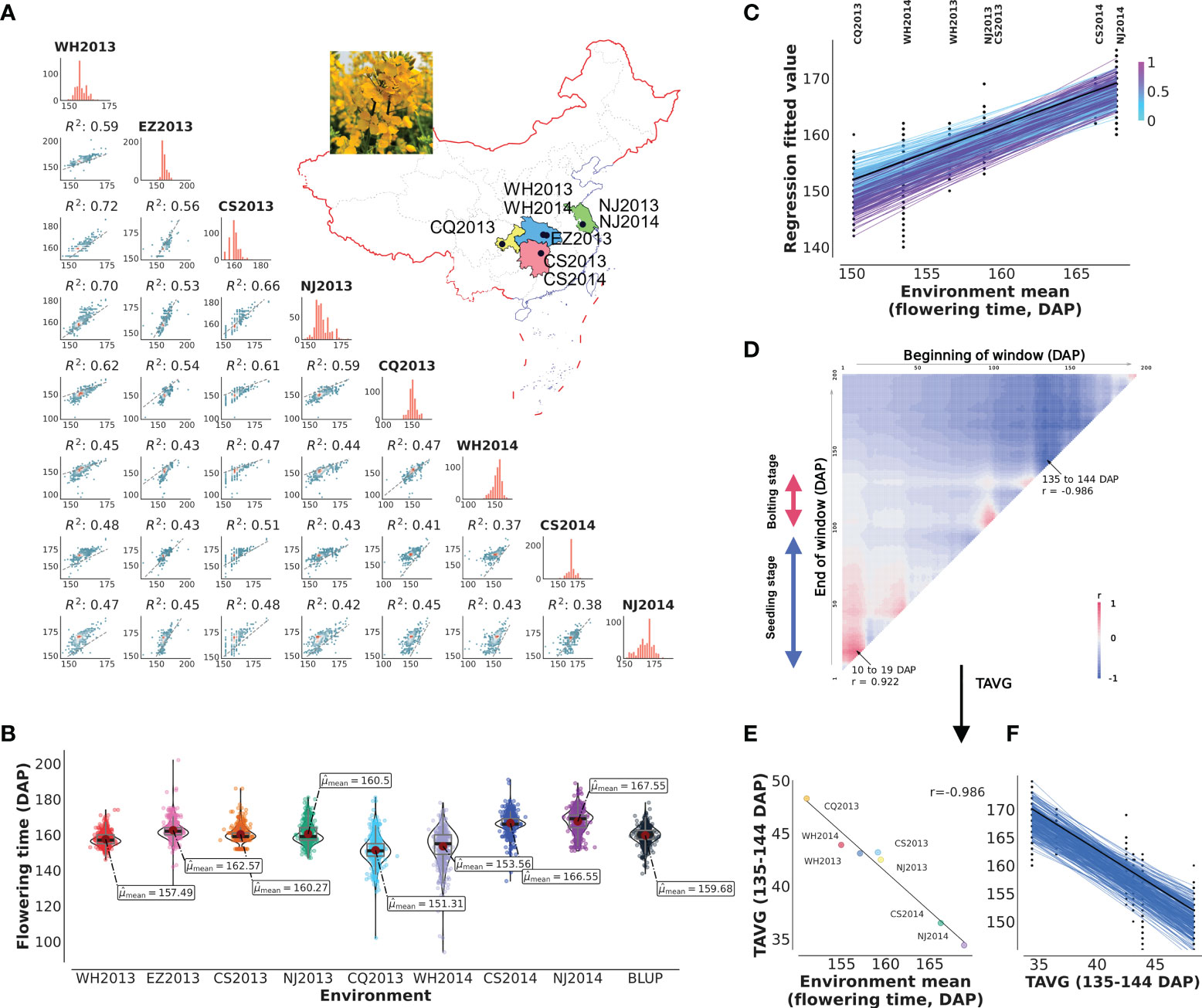
Figure 1 Plasticity of flowering and reaction norm of its associated window to daily average temperature (TAVG). (A, B) Characteristics and pairwise correlations of flowering time of 490 B napus in eight environments. WH2013, Wuhan in 2013; WH2014, Wuhan in 2014; CS2013, Changsha in 2013; CS2014, Changsha in 2014; NJ2013, Nanjing in 2013; NJ2014, Nanjing in 2014; CQ2013, Chongqing in 2013; EZ2013, Ezhou in 2013; BLUP, the best linear unbiased prediction value. (C) Reaction norm for flowering time based on a numerical order of environmental mean. Dots are the observed flowering time phenotypic values. The line with black color represents the ZS11 cultivar. The color of the line represents the value of the slope. (D) Search for the window to TAVG, which is highly correlated with environmental mean of flowering time (from planting to 200 days after planting, DAP). TAVG within the window of 10–19 and 135–144 DAP was chosen and denoted as TAVG10–19 and TAVG135–144. (E, F) Significant correlation and reaction norm between TAVG135–144 and environmental mean of flowering time.
Climate change is altering the environment in which all plants grow. To understand the effect of climatic index on flowering time plasticity, the correlation between environmental means and climatic index (TAVG and PRCP) for different growth windows was predicted by CERIS (Supplementary Table S3). The results of the correlation pattern between TAVG and flowering time showed a positive correlation at early seedling stage and a negative trend after bolting stage, while the pattern of PRCP was exactly opposite (Figure 1D; Supplementary Figure S1A; Supplementary Table S4). The windows with the highest negative (TAVG135–144 and PRCP3–41) and positive correlations (TAVG10–19 and PRCP133–169) were chosen as the most related climatic index for further analysis (Figures 1E, F; Supplementary Figures S2A–F; Supplementary Table S4). TAVG135–144 (r = −0.986) showed higher correlation with flowering time than PRCP3–41 (r = −0.809). TAVG10–19 (r = 0.922) showed higher correlation with flowering time than PRCP133–169 (r = 0.901). It is noted that these windows are surrounded by other windows with slightly decreasing correlation values (Figure 1D; Supplementary Figure S1).
Detection of QTNs for flowering time
To detect QTNs for flowering time, the phenotypes in each of the eight environments were used to associate with 11,700,689 SNPs using 3VmrMLM under population structure and polygenic background control. As a result, 55, 57, 42, 49, 54, 50, 44, and 43 significant QTNs at the critical p-value of 4.27e−09 (=0.05/m, where m is the number of markers) and 10, 5, 14, 10, 8, 13, 13, and 13 suggested QTNs (with the LOD score ≥ 3.0 but the p > 0.05/m) were identified for WH2013, WH2014, CS2013, CS2014, NJ2013, NJ2014, CQ2013, and EZ2013, respectively (Supplementary Table S5; Supplementary Figure S3). In addition, flowering phenotypes from eight environments were used to perform joint analysis by 3VmrMLM. Sixty-eight significant and 11 suggested QTNs were identified. Based on the above QTNs in single and multiple environments analyses, 19 stable QTNs were identified in at least three environments (Figure 2A; Table 1).
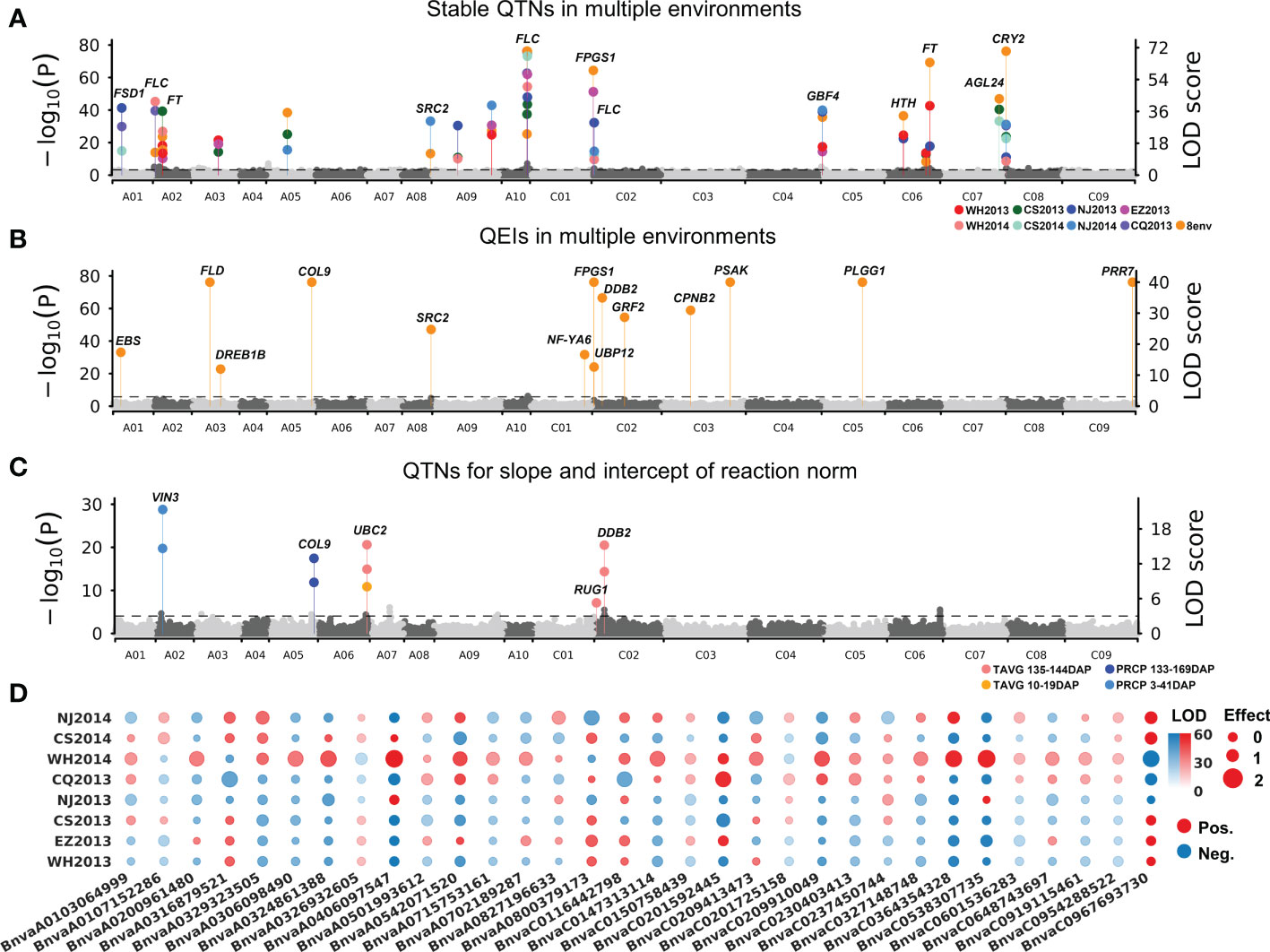
Figure 2 Manhattan plots for flowering time of 490 B napus accessions. (A) Nineteen stable main-effect QTNs and their candidate genes for flowering time in eight single environment analyses and multiple environments joint analysis. (B) QTN-by-environment interactions (QEIs) and their candidate genes for flowering time in multiple environments joint analysis. (C) Ten main-effect QTNs for slope and intercept of reaction norm for flowering-time-related climatic indexes. (D) Additive-by-environment interaction effects of 32 QEIs in eight environments. The size of dot: absolute value of additive-by-environment interaction effect. Red/blue dot: positive/blue value. WH2013, Wuhan in 2013; WH2014, Wuhan in 2014; CS2013, Changsha in 2013; CS2014, Changsha in 2014; NJ2013, Nanjing in 2013; NJ2014, Nanjing in 2014; CQ2013, Chongqing in 2013; EZ2013, Ezhou in 2013.
Detection of QTN-by-environment interactions for flowering time in multiple environments
All the datasets in eight environments were used to conduct joint analysis for identifying QEIs using 3VmrMLM. As a result, 32 significant QEIs and 4 suggested QEIs were identified, including 10 significant QEIs overlapped with the above stable QTNs (Supplementary Table S6). Among these significant QEIs, 20 were found to have the highest absolute value of additive-by-environment interaction effects in WH2014 than those in other environments (Figures 2B, D), e.g., BnvaC0967693730 has an additive-by-environment interaction effect of −1.85 in WH2014 than those in other environments (Supplementary Table S6; LOD = 67.17; R2 = 1.07%). The two loci BnvaC0967693730 and BnvaA0406097547 have the highest R2 (LOD = 67.17; R2 = 1.07% and LOD = 66.42; R2 = 1.06%, respectively).
Detection of QTNs for flowering-time-related climatic index
To obtain reaction norms of flowering-time-related climatic index, joint regression analyses were performed on phenotypes and the above flowering-time-related climatic indexes (TAVG135–144, PRCP3–41, TAVG10–19, and PRCP133–169; Supplementary Table S4). The intercept and slope of reaction-norm parameters were used to detect QTNs for flowering-time-related climatic indexes using 3VmrMLM. As a result, 10 QTNs for reaction norm parameters of B. napus flowering time were commonly identified with the above stable QTNs or QEIs, including 5, 2, 1, and 2 for TAVG135–144, PRCP3–41, TAVG10–19, and PRCP133–169, respectively (Figure 2C; Supplementary Table S7).
Prediction of candidate genes for flowering time
To mine candidate genes among the above QTNs and QEIs, DEGs analysis was conducted before and after vernalization. A total of 5,511 DEGs were identified in two SORs before and after vernalization (Figure 3A; Supplementary Table S8), and 5,850 DEGs were identified in two SWORs before and after vernalization (Figure 3A; Supplementary Table S9). Then, according to Arabidopsis gene annotation, 12 candidate genes were found to be associated with flowering time in approximately above 19 stable QTNs, including 7 known flowering-time-related homologs in Arabidopsis and 5 newly discovered genes (Table 1). Using the same methods, 14 candidate genes were identified to be located in the above 32 QEIs, including 9 homologs of known genes, in which their homologs are related to flowering time and environments in Arabidopsis and 5 newly identified genes (Table 2). In addition, five candidate genes were found to be associated with flowering-time-related climatic index, including two genes (BnaC02.DDB2 and BnaA05.COL9) commonly identified in QEIs and three genes (BnaA02.VIN3, BnaC02.RUG1, and BnaA06.UBC2) commonly found to be associated with the flowering time QTNs (Supplementary Table S7).
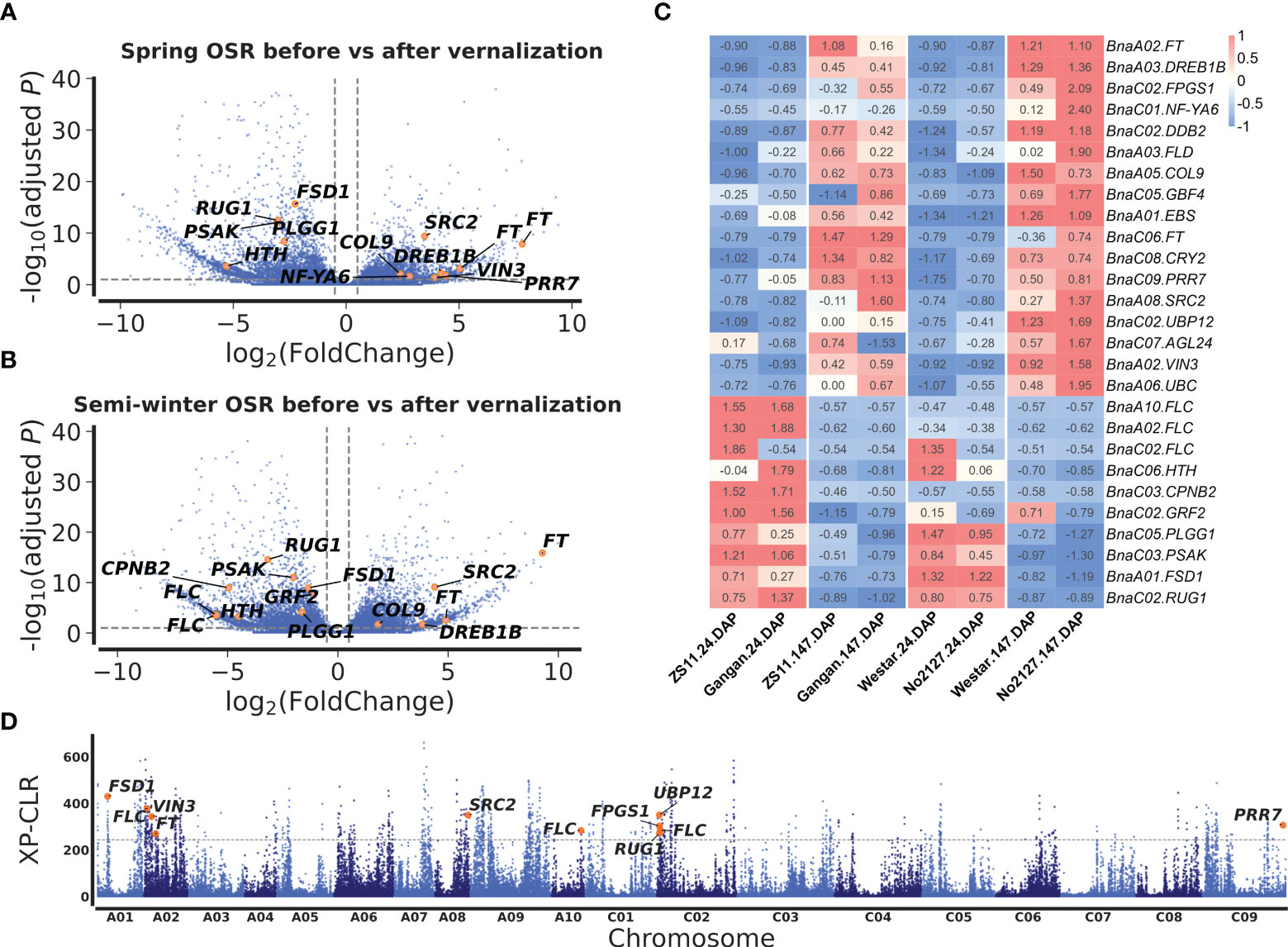
Figure 3 Differentially expressed gene (DEG) analysis before and after vernalization and selection sweeps between semi-winter and spring oilseed rapes (SWORs and SORs). Volcano plots of DEGs in SORs (A) and SWORs (B). The y-axis is the adjusted p-value and the x-axis is log2 fold-change (FC) before and after vernalization. Gray lines are at the absolute value of log2FC = 1 or adjusted p-value = 0.05. (C) The expression profiling of 27 candidate genes around main-effect QTNs and QEIs for flowering time in two SWORs and two SORs in the 2018–2019 growing season in Wuhan. DAP, days after planting. (D) Selective sweeps between SWORs and SORs by XP-CLR. The horizontal dashed lines indicate the cutoff in the top 1% of XP-CLR scores. Candidate genes for flowering time are marked above the selective sweep peaks.
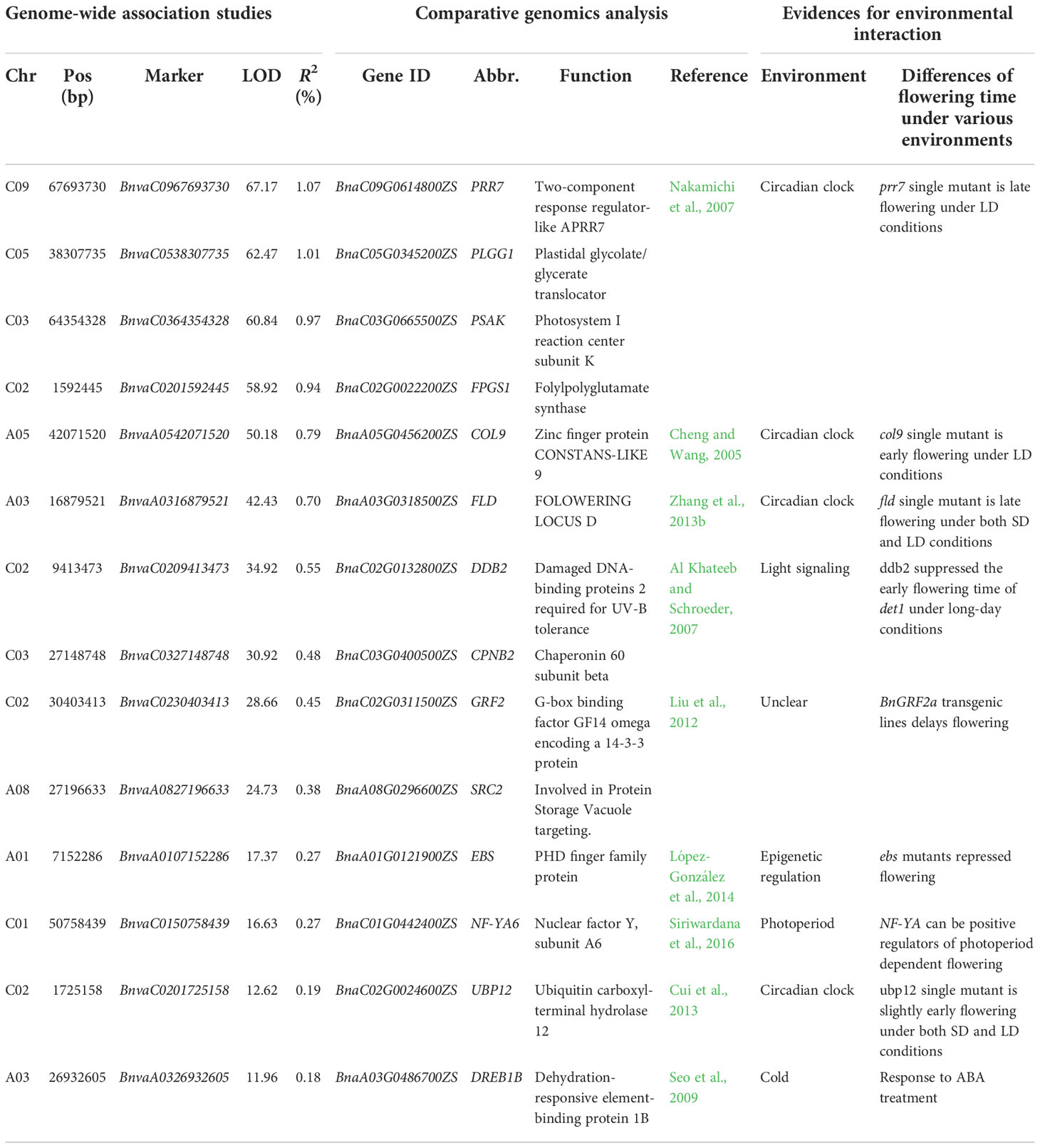
Table 2 Fourteen candidate genes for B. napus flowering time around significant QTN-by-environment interactions.
Among these candidate genes, BnaFTs, BnaA05.COL9, BnaA08.SRC2, and BnaA03.DREB1B were significantly upregulated before vernalization in both SWORs and spring SORs, while BnaFLCs, BnaA01.FSD1, BnaC02.RUG1, BnaC05.PLGG, and BnaC03.PSAK were significantly upregulated after vernalization (Figures 3A, B). Interestingly, BnaA02.VIN3 and BnaC02.FPGS were only significantly upregulated before vernalization in SOR, which may indicate different functions between eco-types.
Validation of candidate genes
To validate the above flowering time candidate genes, we conducted selective sweep, haplotype, and co-expression analysis. First, by performing XP-CLR between SWORs and SORs, 954 selective sweeps were detected (Supplementary Table S10). Eleven candidate genes for flowering time were found in the selective sweep, e.g., BnaFLCs, BnaFTs, BnaC02.FPGS1, BnaA08.SRC2, BnaA01.FSD1, BnaA02.VIN3, and BnaC09.PRR7. Second, haplotype analyses were further conducted in these genes. For BnaA02.FT, BnaA10.FLC, BnaA02.VIN3, and BnaC09.PRR7, significant difference exists between each haplotype in different environments (Figures 4A–D; Supplementary Figures S4A–D). Interestingly, the haplotype for early flowering tends to exist in SORs, while the haplotype for late flowering prefers to exist in SWORs. Moreover, the co-expression networks of BnaA02.VIN3 and BnaC09.PRR7 have been constructed using DEGs in SWORs and SORs, respectively (Figures 5A, B). The co-expressed genes of BnaA02.VIN3 mainly participated in the circadian clock, photoperiodism, light perception, and signaling. Eight genes are specific co-expressed in SORs, including BnaA07.ZEP and BnaC09.ABCG22 in response to water deprivation. Five genes are specific co-expressed in SWORs. On the other hand, the co-expressed genes of BnaC09.PRR7 mainly participated in the circadian clock and autonomous pathway. Five and one genes are specific co-expressed in SORs and SWORs, respectively. BnaCKA2s and BnaPKDM7s participated in epigenetic regulation.
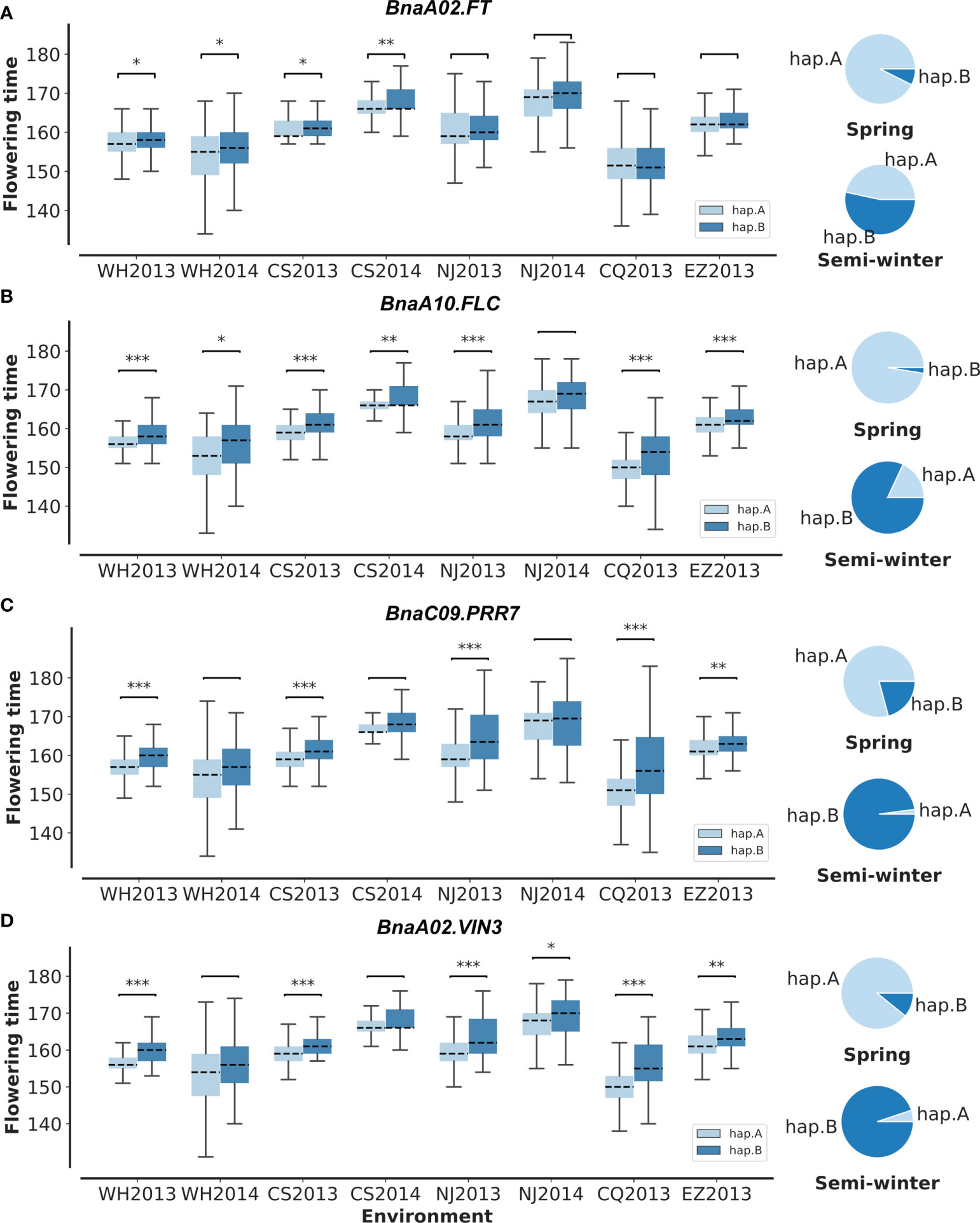
Figure 4 Haplotype analysis of BnaC02.FT, BnaA10.FLC, BnaC09.PRR7, and BnaA02.VIN3 (A–D). In the boxplot, significant differences for flowering time between each haplotype are calculated in eight environments with t-test. In pie plots, the haplotype frequencies of each gene in semi-winter and spring oilseed rapes are marked. WH2013, Wuhan in 2013; WH2014, Wuhan in 2014; CS2013, Changsha in 2013; CS2014, Changsha in 2014; NJ2013, Nanjing in 2013; NJ2014, Nanjing in 2014; CQ2013, Chongqing in 2013; EZ2013, Ezhou in 2013. *p = 0.05, **p = 0.01, and ***p = 0.001.
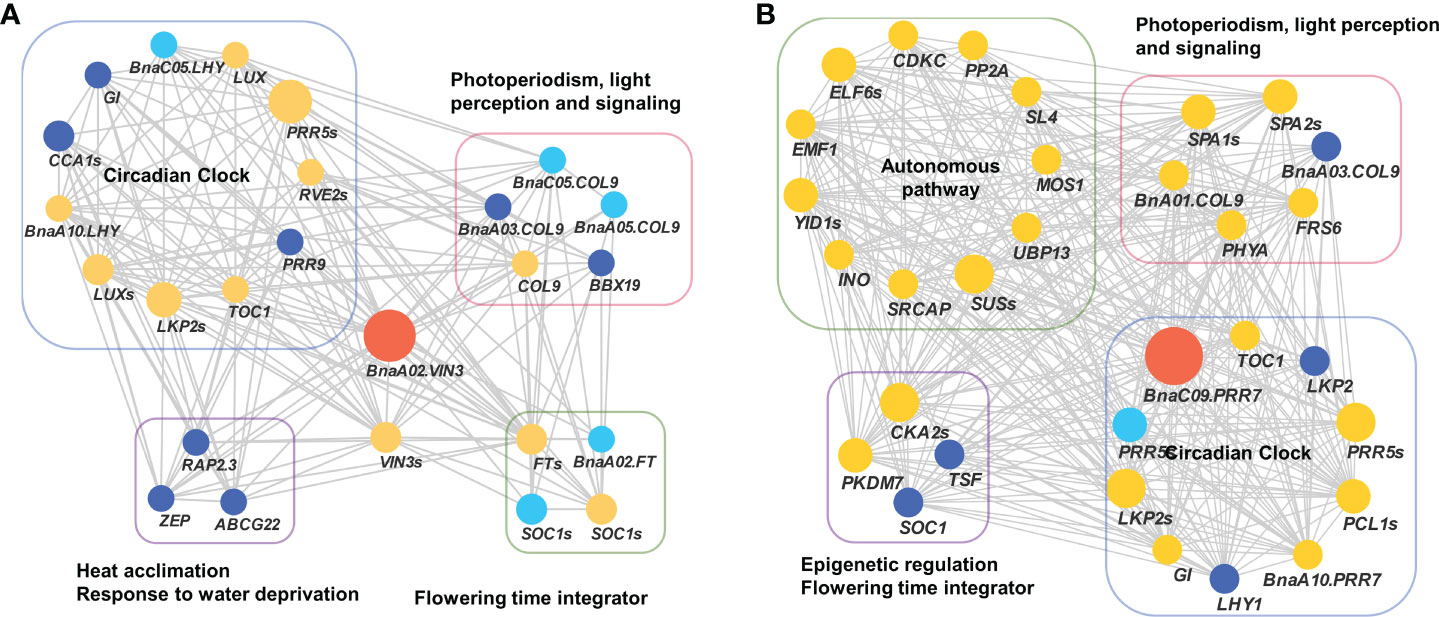
Figure 5 Co-expression network of BnaVIN3 and BnaPRR7 with co-expressed genes related to flowering time (A, B). Light blue, dark blue, and yellow node indicate co-expressed genes that were detected in semi-winter, spring, and both types of oilseed rapes, respectively. The size of each node represents the number of genes in each gene family.
Discussion
Although flowering time is strongly related to the environment, G by E studies for flowering time are lacking in B. napus. The current study analyzed the G by E for flowering time in the following three aspects. First, four windows of flowering-time-related climatic index were identified (TAVG135–144, PRCP3–41, TAVG10–19, and PRCP133–169) by CERIS. Second, 19 stable QTNs and 32 QEIs were found to be significantly associated with flowering time of 490 B. napus accessions in eight environments, and 10 QTNs were found to be associated with flowering-time-related climatic index. Finally, based on DEGs and homology with Arabidopsis, 12, 14, and 5 candidate genes were found to be associated with stable QTNs, QEIs, and QTNs for flowering-time-related climatic index, respectively. These candidate genes were further validated by the haplotype, selective sweep, and co-expression network analysis.
Flowering-time-related climatic index in B. napus whole growth stages
It is well-known that the flowering time regulation of B. napus is in response to day length or vernalization (Reeves and Coupland, 2000). This study calculated the correlations between two climatic factors, TAVG and PRCP, and flowering time in seven environments. TAVG correlated positively with flowering time in vernalization and negatively with flowering time after the seedling stage (Figure 1D). In a previous study, a reduction in autumn or winter chilling delays floral transition in B. napus (O’Neill et al., 2019). An elevated growth temperature is equally efficient in inducing the flowering of Arabidopsis (Balasubramanian et al., 2006). However, the transition or critical point of these two stages is unclear. For PRCP, this study reported the relationships between PRCP and flowering time in B. napus for the first time. Although the correlation coefficients are lower than TAVG, PRCP was found to be correlated negatively with flowering time in early development and positively later (Supplementary Figure S1). This result is consistent with a previous study in Arabidopsis that flowering time correlated negatively with fall and winter precipitations and positively with summer precipitation (Vidigal et al., 2016).
Genetic basis for flowering time in B. napus
In this study, multi-environment joint GWAS improved the power on identifying more QTNs than single environment GWAS. We dissected the genetic basis for flowering time in the following three aspects. First, 12 flowering time candidate genes were mined in approximately 19 stable QTNs for flowering time. Seven genes are previously reported, e.g., BnaFLCs (Tadege et al., 2001), BnaFTs (Wang et al., 2009), BnaAGL24 (Yu et al., 2002), and BnaCRY2 (Sharma et al., 2022), whereas five genes are newly identified, which are differentially expressed before and after the vernalization of different ecotypes (Figure 3; Supplementary Tables S8, S9). Second, it is worth noting that this study focused on the mining of flowering time genes related to the environments. Fourteen candidate genes were identified around 32 QEIs, including 9 known flowering time genes related to environments. For example, BnaCOL9 and BnaUBP12 are regulated by the circadian clock in the photoperiod pathway (Cheng and Wang, 2005; Cui et al., 2013). BnaFLD is subjected to the direct regulation by brassinosteroids (Zhang et al., 2013b). It has been reported that the overexpression of BnaDREB1B not only delayed flowering but also responded to cold (Seo et al., 2009). BnaEBS functions in the chromatin-mediated repression of floral initiation by H3K4me3 (López-González et al., 2014). Finally, five genes were found to be associated with flowering-time-related climatic index. BnaC02.DDB2 and BnaA05.COL9 were commonly identified in QEIs, and BnaA02.VIN3, BnaC02.RUG1, and BnaA06.UBC2 were commonly found to be associated with the main effect flowering time QTNs.
In this study, the missing heritability exists, in which the total phenotypic variance explained of QEIs and QTNs is much less than the estimated broad-sense heritability. This can be explained in several ways. First, the population is not enough to detect rare variants. Second, allelic heterogeneity may be the reason for this phenomenon. Lastly, epigenetic variation is likely to be a source of missing heritability (Brachi et al., 2011). Moreover, some candidate genes for stable QTNs, e.g., BnaA02.FT and BnaA10.FLC, were found to be related to environments but were not identified in QEIs (Figure 4). This result is explained by multiple facets, e.g., the difference in phenotypic data among environments, the diversity of population accessions, and the power of QEI detection. In the previous study, COL9 and FLD have been reported to regulate FT and FLC, respectively (Cheng and Wang, 2005; Jiang et al., 2009). BnA05.COL9 and BnaA03.FLD were found to be candidate genes for QEIs in this study. We hypothesized that QEI may be associated with direct environmental response upstream regulators due to the complexity of transcription and epigenetic regulations of flowering (Bouché et al., 2016).
BnaA02.VIN3 and BnaC09.PRR7 are potential G by E genes for flowering time
In Arabidopsis, VIN3 acts together with PRC2 to repress histone marks at FLC in response to vernalization (Kim and Sung, 2013). PRR7 was reported to coordinate with PRR9 and PRR5 and regulate flowering time through the canonical CO-dependent photoperiodic pathway (Nakamichi et al., 2007). In this study, BnaA02.VIN3 and BnaC09.PRR7 have been shown to be crucial G by E genes for flowering time. There are three pieces of evidence. First, BnaA02.VIN3 is significantly associated with ChrA02-6152101 (LOD = 13.14) for flowering-time-related climatic factors and with ChrA02-6374324 (LOD = 12.17) for flowering time in WH2013. BnaC09.PRR7 is significantly associated with the QEI, ChrC09-67693730 (LOD = 67.17), by multi-environment GWAS. Second, BnaA02.VIN3 and BnaC09.PRR7 are DEGs before and after vernalization and in the selective sweep between SORs and SWORs (Figure 2). Then, in these genes with significant haplotype differences, their haplotypes for early flowering tend to exist more in SORs (Figures 4C, D). Lastly, co-expression networks were constructed for BnaA02.VIN3 and BnaC09.PRR7. Some relationships have been proven, e.g., PRR7 with LHY (Liu et al., 2013), PRR7 with PRR5 (Nakamichi et al., 2007), and VIN3 with CCA1 and LHY (Kyung et al., 2022).
In summary, we dissected the G by E for flowering time for B. napus from different eco-types in eight environments. Four windows of flowering-time-related climatic index were identified. Stable QTNs and QEIs for flowering time and their candidate genes were identified. These findings provide valuable information that can be used to breed B. napus varieties with optimized flowering time by pyramiding favorable alleles. The candidate genes will also greatly promote the dissection of flowering time mechanisms in different eco-types.
Data availability statement
The original contributions presented in the study are publicly available. This data can be found here: NGDC, PRJCA012445 and CRA008501.
Author contributions
LG and XH conceived this study. QT, LX, ZG, JT, and BY performed the field experiments. XH performed the bioinformatics analysis and wrote the manuscript. JT, BY, KL, XY, SL, and LG revised the manuscript. All authors approved the submitted version.
Funding
This work was supported by grants from the National Natural Science Foundation of China (U2102217), Key Research and Development Program of Hubei (2021ABA011) and Higher Education Discipline Innovation Project (B20051).
Acknowledgments
We would like to thank Prof. Yuan-Ming Zhang (College of Plant Science and Technology, Huazhong Agricultural University, Wuhan) for improving the language within the manuscript.
Conflict of interest
The authors declare that the research was conducted in the absence of any commercial or financial relationships that could be construed as a potential conflict of interest.
Publisher’s note
All claims expressed in this article are solely those of the authors and do not necessarily represent those of their affiliated organizations, or those of the publisher, the editors and the reviewers. Any product that may be evaluated in this article, or claim that may be made by its manufacturer, is not guaranteed or endorsed by the publisher.
Supplementary material
The Supplementary Material for this article can be found online at: https://www.frontiersin.org/articles/10.3389/fpls.2022.1065766/full#supplementary-material
References
Al Khateeb, W. M., Schroeder, D. F. (2007). DDB2, DDB1A and DET1 exhibit complex interactions during arabidopsis development. Genetics 176, 231–242. doi: 10.1534/genetics.107.070359
An, H., Qi, X., Gaynor, M. L., Hao, Y., Gebken, S. C., Mabry, M. E., et al. (2019). Transcriptome and organellar sequencing highlights the complex origin and diversification of allotetraploid Brassica napus. Nat. Commun. 10, 2878. doi: 10.1038/s41467-019-10757-1
Arnold, P. A., Kruuk, L. E. B., Nicotra, A. B. (2019). How to analyse plant phenotypic plasticity in response to a changing climate. New Phytol. 222, 1235–1241. doi: 10.1111/nph.15656
Balasubramanian, S., Sureshkumar, S., Lempe, J., Weigel, D. (2006). Potent induction of Arabidopsis thaliana flowering by elevated growth temperature. PloS Genet. 2, e106. doi: 10.1371/journal.pgen.0020106
Bastow, R., Mylne, J. S., Lister, C., Lippman, Z., Martienssen, R. A., Dean, C. (2004). Vernalization requires epigenetic silencing of FLC by histone methylation. Nature 427, 164–167. doi: 10.1038/nature02269
Bates, D., Mächler, M., Bolker, B. M., Walker, S. C. (2015). Fitting linear mixed-effects models using lme4. J. Stat. Software 67, 1–48. doi: 10.18637/jss.v067.i01
Bouché, F., Lobet, G., Tocquin, P., Périlleux, C. (2016). FLOR-ID: an interactive database of flowering-time gene networks in Arabidopsis thaliana. Nucleic Acids Res. 44, D1167–D1171. doi: 10.1093/nar/gkv1054
Brachi, B., Morris, G. P., Borevitz, J. O. (2011). Genome-wide association studies in plants: The missing heritability is in the field. Genome Biol. 12, 232. doi: 10.1186/gb-2011-12-10-232
Cheng, X. F., Wang, Z. Y. (2005). Overexpression of COL9, a CONSTANS-LIKE gene, delays flowering by reducing expression of CO and FT in Arabidopsis thaliana. Plant J. 43, 758–768. doi: 10.1111/j.1365-313X.2005.02491.x
Chen, H., Patterson, N., Reich, D. (2010). Population differentiation as a test for selective sweeps. Genome Res. 20, 393–402. doi: 10.1101/gr.100545.109
Corbesier, L., Vincent, C., Jang, S., Fornara, F., Fan, Q., Searle, I., et al. (2007). FT protein movement contributes to long-distance signaling in floral induction of arabidopsis. Science 316, 1030–1033. doi: 10.1126/science.1141752
Cui, X., Lu, F., Li, Y., Xue, Y., Kang, Y., Zhang, S., et al. (2013). Ubiquitin-specific proteases UBP12 and UBP13 act in circadian clock and photoperiodic flowering regulation in arabidopsis. Plant Physiol. 162, 897–906. doi: 10.1104/pp.112.213009
Cui, Y., Xu, Z., Xu, Q. (2021). Elucidation of the relationship between yield and heading date using CRISPR/Cas9 system-induced mutation in the flowering pathway across a large latitudinal gradient. Mol. Breed. 41, 23. doi: 10.1007/s11032-021-01213-4
Del Olmo, I., Poza-Viejo, L., Piñeiro, M., Jarillo, J. A., Crevillén, P. (2019). High ambient temperature leads to reduced FT expression and delayed flowering in Brassica rapa via a mechanism associated with H2A.Z dynamics. Plant J. 100, 343–356. doi: 10.1111/tpj.14446
Ewels, P., Magnusson, M., Lundin, S., Käller, M. (2016). MultiQC: summarize analysis results for multiple tools and samples in a single report. Bioinformatics 32, 3047–3048. doi: 10.1093/bioinformatics/btw354
Guo, Y., Hans, H., Christian, J., Molina, C. (2014). Mutations in single FT- and TFL1-paralogs of rapeseed (Brassica napus l.) and their impact on flowering time and yield components. Front. Plant Sci. 5. doi: 10.3389/fpls.2014.00282
Guo, T., Mu, Q., Wang, J., Vanous, A. E., Onogi, A., Iwata, H., et al. (2020). Dynamic effects of interacting genes underlying rice flowering-time phenotypic plasticity and global adaptation. Genome Res. 30, 673–683. doi: 10.1101/gr.255703.119
Han, X., Xu, Z. R., Zhou, L., Han, C. Y., Zhang, Y. M. (2021). Identification of QTNs and their candidate genes for flowering time and plant height in soybean using multi-locus genome-wide association studies. Mol. Breed. 41, 39. doi: 10.1007/s11032-021-01230-3
Helal, M. M. U., Gill, R. A., Tang, M., Yang, L., Hu, M., Yang, L., et al. (2021). SNP- and haplotype-based GWAS of flowering-related traits in Brassica napus. Plants 10, 2475. doi: 10.3390/plants10112475
Hu, J., Chen, B., Zhao, J., Zhang, F., Xie, T., Xu, K., et al. (2022). Genomic selection and genetic architecture of agronomic traits during modern rapeseed breeding. Nat. Genet. 54, 694–704. doi: 10.1038/s41588-022-01055-6
Jiang, D., Gu, X., He, Y. (2009). Establishment of the winter-annual growth habit via FRIGIDA-mediated histone methylation at FLOWERING LOCUS c in arabidopsis. Plant Cell 21, 1733–1746. doi: 10.1105/tpc.109.067967
Kim, D. H., Sung, S. (2013). Coordination of the vernalization response through a VIN3 and FLC gene family regulatory network in arabidopsis. Plant Cell 25, 454–469. doi: 10.1105/tpc.112.104760
Knapp, S. J., Stroup, W. W., Ross, W. M. (1985). Exact confidence intervals for heritability on a progeny mean basis. Crop Sci. 25, 192–194. doi: 10.2135/cropsci1985.0011183X002500010046x
Kusmec, A., Srinivasan, S., Nettleton, D., Schnable, P. S. (2017). Distinct genetic architectures for phenotype means and plasticities in zea mays. Nat. Plants 3, 715–723. doi: 10.1038/s41477-017-0007-7
Kyung, J., Jeon, M., Jeong, G., Shin, Y., Seo, E., Yu, J., et al. (2022). The two clock proteins CCA1 and LHY activate VIN3 transcription during vernalization through the vernalization-responsive cis-element. Plant Cell 34, 1020–1037. doi: 10.1093/plcell/koab304
Li, X., Guo, T., Wang, J., Bekele, W. A., Sukumaran, S., Vanous, A. E., et al. (2021). An integrated framework reinstating the environmental dimension for GWAS and genomic selection in crops. Mol. Plant 14, 874–887. doi: 10.1016/j.molp.2021.03.010
Li, Y., Huang, Y., Bergelson, J., Nordborg, M., Borevitz, J. O. (2010). Association mapping of local climate-sensitive quantitative trait loci in Arabidopsis thaliana. Proc. Natl. Acad. Sci. 107, 21199–21204. doi: 10.1073/pnas.1007431107
Li, M., Zhang, Y. W., Xiang, Y., Liu, M. H., Zhang, Y. M. (2022a). IIIVmrMLM: The r and c++ tools associated with 3VmrMLM, a comprehensive GWAS method for dissecting quantitative traits. Mol. Plant 15, 1251–1253. doi: 10.1016/j.molp.2022.06.002
Li, M., Zhang, Y. W., Zhang, Z. C., Xiang, Y., Liu, M. H., Zhou, Y. H., et al. (2022b). A compressed variance component mixed model for detecting QTNs, and QTN-by-environment and QTN-by-QTN interactions in genome-wide association studies. Mol. Plant 0. doi: 10.1016/j.molp.2022.02.012
Liu, T., Carlsson, J., Takeuchi, T., Newton, L., Farré, E. M. (2013). Direct regulation of abiotic responses by the arabidopsis circadian clock component PRR7. Plant J. 76, 101–114. doi: 10.1111/tpj.12276
Liu, J., Hua, W., Yang, H. L., Zhan, G. M., Li, R. J., Deng, L. B., et al. (2012). The BnGRF2 gene (GRF2-like gene from Brassica napus) enhances seed oil production through regulating cell number and plant photosynthesis. J. Exp. Bot. 63, 3727–3740. doi: 10.1093/jxb/ers066
Liu, J. Y., Zhang, Y. W., Han, X., Zuo, J. F., Zhang, Z., Shang, H., et al. (2020). An evolutionary population structure model reveals pleiotropic effects of GmPDAT for traits related to seed size and oil content in soybean. J. Exp. Bot. 71, 6988–7002. doi: 10.1093/jxb/eraa426
Liu, N., Du, Y., Warbuton, M. L., Xiao, Y., Yan, J. (2021). Phenotypic plasticity contributes to maize adaptation and heterosis. Mol. Biol. Evol. 38, 1262–1275. doi: 10.1093/molbev/msaa283
López-González, L., Mouriz, A., Narro-Diego, L., Bustos, R., Martínez-Zapater, J. M., Jarillo, J. A., et al. (2014). Chromatin-dependent repression of the arabidopsis floral integrator genes involves plant specific PHD-containing proteins. Plant Cell 26, 3922–3938. doi: 10.1105/tpc.114.130781
Love, M. I., Huber, W., Anders, S. (2014). Moderated estimation of fold change and dispersion for RNA-seq data with DESeq2. Genome Biol. 15, 550. doi: 10.1186/s13059-014-0550-8
Matar, S., Kumar, A., Holtgräwe, D., Weisshaar, B., Melzer, S. (2021). The transition to flowering in winter rapeseed during vernalization. Plant Cell Environ. 44, 506–518. doi: 10.1111/pce.13946
Mouradov, A., Cremer, F., Coupland, G. (2002). Control of flowering time: Interacting pathways as a basis for diversity. Plant Cell 14, S111–S130. doi: 10.1105/tpc.001362
Nakamichi, N., Kita, M., Niinuma, K., Ito, S., Yamashino, T., Mizoguchi, T., et al. (2007). Arabidopsis clock-associated pseudo-response regulators PRR9, PRR7 and PRR5 coordinately and positively regulate flowering time through the canonical CONSTANS-dependent photoperiodic pathway. Plant Cell Physiol. 48, 822–832. doi: 10.1093/pcp/pcm056
O’Neill, C. M., Lu, X., Calderwood, A., Tudor, E. H., Robinson, P., Wells, R., et al. (2019). Vernalization and floral transition in autumn drive winter annual life history in oilseed rape. Curr. Biol. 29, 4300–4306.e2. doi: 10.1016/j.cub.2019.10.051
Patro, R., Duggal, G., Love, M. I., Irizarry, R. A., Kingsford, C. (2017). Salmon provides fast and bias-aware quantification of transcript expression. Nat. Methods 14, 417–419. doi: 10.1038/nmeth.4197
Putterill, J., Laurie, R., Macknight, R. (2004). It’s time to flower: the genetic control of flowering time. BioEssays 26, 363–373. doi: 10.1002/bies.20021
Reeves, P. H., Coupland, G. (2000). Response of plant development to environment: control of flowering by daylength and temperature. Curr. Opin. Plant Biol. 3, 37–42. doi: 10.1016/S1369-5266(99)00041-2
Schiessl, S. (2020). Regulation and subfunctionalization of flowering time genes in the allotetraploid oil crop Brassica napus. Front. Plant Sci. 11. doi: 10.3389/fpls.2020.605155
Seo, E., Lee, H., Jeon, J., Park, H., Kim, J., Noh, Y. S., et al. (2009). Crosstalk between cold response and flowering in arabidopsis is mediated through the flowering-time gene SOC1 and its upstream negative regulator FLC. Plant Cell 21, 3185–3197. doi: 10.1105/tpc.108.063883
Shannon, P., Markiel, A., Ozier, O., Baliga, N. S., Wang, J. T., Ramage, D., et al. (2003). Cytoscape: A software environment for integrated models of biomolecular interaction networks. Genome Res. 13, 2498–2504. doi: 10.1101/gr.1239303
Sharma, P., Mishra, S., Burman, N., Chatterjee, M., Singh, S., Pradhan, A. K., et al. (2022). Characterization of Cry2 genes (CRY2a and CRY2b) of B. napus and comparative analysis of BnCRY1 and BnCRY2a in regulating seedling photomorphogenesis. Plant Mol. Biol. 110, 161–186. doi: 10.1007/s11103-022-01293-6
Shen, Y., Xiang, Y., Xu, E., Ge, X., Li, Z. (2018). Major co-localized QTL for plant height, branch initiation height, stem diameter, and flowering time in an alien introgression derived Brassica napus DH population. Front. Plant Sci. 9. doi: 10.3389/fpls.2018.00390
Siriwardana, C. L., Gnesutta, N., Kumimoto, R. W., Jones, D. S., Myers, Z. A., Mantovani, R., et al. (2016). NUCLEAR FACTOR y, subunit a (NF-YA) proteins positively regulate flowering and act through FLOWERING LOCUS t. PloS Genet. 12, e1006496. doi: 10.1371/journal.pgen.1006496
Song, J., Guan, Z., Hu, J., Guo, C., Yang, Z., Wang, S., et al. (2020). Eight high-quality genomes reveal pan-genome architecture and ecotype differentiation of Brassica napus. Nat. Plants 6, 34–45. doi: 10.1038/s41477-019-0577-7
Song, J., Liu, D., Xie, W., Yang, Z., Guo, L., Liu, K., et al. (2021). BnPIR: Brassica napus pan-genome information resource for 1689 accessions. Plant Biotechnol. J. 19, 412–414. doi: 10.1111/pbi.13491
Tadege, M., Sheldon, C. C., Helliwell, C. A., Stoutjesdijk, P., Dennis, E. S., Peacock, W. J. (2001). Control of flowering time by FLC orthologues in Brassica napus. Plant J. 28, 545–553. doi: 10.1046/j.1365-313X.2001.01182.x
Tang, S., Zhao, H., Lu, S., Yu, L., Zhang, G., Zhang, Y., et al. (2021). Genome- and transcriptome-wide association studies provide insights into the genetic basis of natural variation of seed oil content in Brassica napus. Mol. Plant 14, 470–487. doi: 10.1016/j.molp.2020.12.003
Tan, Z., Xie, Z., Dai, L., Zhang, Y., Zhao, H., Tang, S., et al. (2022). Genome- and transcriptome-wide association studies reveal the genetic basis and the breeding history of seed glucosinolate content in Brassica napus. Plant Biotechnol. J. 20, 211–225. doi: 10.1111/pbi.13707
Vidigal, D. S., Marques, A. C. S. S., Willems, L. A. J., Buijs, G., Méndez-Vigo, B., Hilhorst, H. W. M., et al. (2016). Altitudinal and climatic associations of seed dormancy and flowering traits evidence adaptation of annual life cycle timing in Arabidopsis thaliana. Plant Cell Environ. 39, 1737–1748. doi: 10.1111/pce.12734
Wang, J., Long, Y., Wu, B., Liu, J., Jiang, C., Shi, L., et al. (2009). The evolution of Brassica napus FLOWERING LOCUS t paralogues in the context of inverted chromosomal duplication blocks. BMC Evol. Biol. 9, 271. doi: 10.1186/1471-2148-9-271
Wu, R. (1998). The detection of plasticity genes in heterogeneous environments. Evolution 52, 967–977. doi: 10.1111/j.1558-5646.1998.tb01826.x
Xu, J., Dai, H. (2016). Brassica napus cycling dof Factor1 (BnCDF1) is involved in flowering time and freezing tolerance. Plant Growth Regul. 80, 315–322. doi: 10.1007/s10725-016-0168-9
Xu, L., Hu, K., Zhang, Z., Guan, C., Chen, S., Hua, W., et al. (2016). Genome-wide association study reveals the genetic architecture of flowering time in rapeseed (Brassica napus l.). DNA Res. 23, 43–52. doi: 10.1093/dnares/dsv035
Yang, C., Gan, Y., Harker, K. N., Kutcher, H. R., Gulden, R., Irvine, B., et al. (2014). Up to 32 % yield increase with optimized spatial patterns of canola plant establishment in western Canada. Agron. Sustain. Dev. 34, 793–801. doi: 10.1007/s13593-014-0218-5
Ying, L., Chen, H., Cai, W. (2014). BnNAC485 is involved in abiotic stress responses and flowering time in Brassica napus. Plant Physiol. Biochem. PPB 79, 77–87. doi: 10.1016/j.plaphy.2014.03.004
Yu, H., Xu, Y., Tan, E. L., Kumar, P. P. (2002). AGAMOUS-LIKE 24, a dosage-dependent mediator of the flowering signals. Proc. Natl. Acad. Sci. U. S. A. 99, 16336–16341. doi: 10.1073/pnas.212624599
Zhang, H., Berger, J. D., Milroy, S. P. (2013a). Genotype×environment interaction studies highlight the role of phenology in specific adaptation of canola (Brassica napus) to contrasting Mediterranean climates. Field Crops Res. 144, 77–88. doi: 10.1016/j.fcr.2013.01.006
Zhang, Y., Li, B., Xu, Y., Li, H., Li, S., Zhang, D., et al. (2013b). The cyclophilin CYP20-2 modulates the conformation of BRASSINAZOLE-RESISTANT1, which binds the promoter of FLOWERING LOCUS d to regulate flowering in arabidopsis. Plant Cell 25, 2504–2521. doi: 10.1105/tpc.113.110296
Zhang, J., Yi, Q., Xing, F., Tang, C., Wang, L., Ye, W., et al. (2018). Rapid shifts of peak flowering phenology in 12 species under the effects of extreme climate events in Macao. Sci. Rep. 8, 13950. doi: 10.1038/s41598-018-32209-4
Zhao, H., Savin, K. W., Li, Y., Breen, E. J., Maharjan, P., Tibbits, J. F., et al. (2022). Genome-wide association studies dissect the G × E interaction for agronomic traits in a worldwide collection of safflowers (Carthamus tinctorius l.). Mol. Breed. 42, 24. doi: 10.1007/s11032-022-01295-8
Zhu, Y., Cao, Z., Xu, F., Huang, Y., Chen, M., Guo, W., et al. (2012). Analysis of gene expression profiles of two near-isogenic lines differing at a QTL region affecting oil content at high temperatures during seed maturation in oilseed rape (Brassica napus l.). Theor. Appl. Genet. 124, 515–531. doi: 10.1007/s00122-011-1725-2
Keywords: Brassica napus, flowering time, QTN-by-environment interactions, multiple genome-wide association studies, differentially expressed gene, climatic index
Citation: Han X, Tang Q, Xu L, Guan Z, Tu J, Yi B, Liu K, Yao X, Lu S and Guo L (2022) Genome-wide detection of genotype environment interactions for flowering time in Brassica napus. Front. Plant Sci. 13:1065766. doi: 10.3389/fpls.2022.1065766
Received: 10 October 2022; Accepted: 31 October 2022;
Published: 21 November 2022.
Edited by:
Zhenyu Jia, University of California, Riverside, United StatesReviewed by:
Ling Qiao, Shanxi Agricultural University, ChinaYang-Jun Wen, Nanjing Agricultural University, China
Copyright © 2022 Han, Tang, Xu, Guan, Tu, Yi, Liu, Yao, Lu and Guo. This is an open-access article distributed under the terms of the Creative Commons Attribution License (CC BY). The use, distribution or reproduction in other forums is permitted, provided the original author(s) and the copyright owner(s) are credited and that the original publication in this journal is cited, in accordance with accepted academic practice. No use, distribution or reproduction is permitted which does not comply with these terms.
*Correspondence: Liang Guo, Z3VvbGlhbmdAbWFpbC5oemF1LmVkdS5jbg==