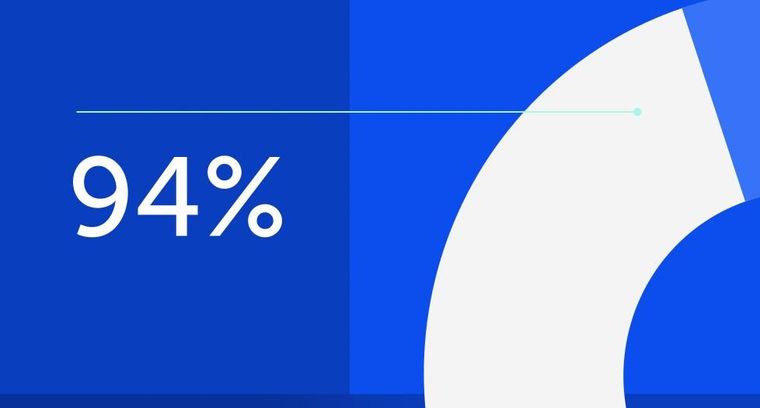
94% of researchers rate our articles as excellent or good
Learn more about the work of our research integrity team to safeguard the quality of each article we publish.
Find out more
ORIGINAL RESEARCH article
Front. Plant Sci., 21 December 2022
Sec. Plant Symbiotic Interactions
Volume 13 - 2022 | https://doi.org/10.3389/fpls.2022.1050967
This article is part of the Research TopicMicrobe Assisted Plant Resistance to Abiotic StressesView all 11 articles
Both biotic and abiotic factors continually affect the phyllospheric ecology of plants. A better understanding of the drivers of phyllospheric community structure and multitrophic interactions is vital for developing plant protection strategies. In this study, 16S rRNA high-throughput sequencing was applied to study how summer climatic factors and bacterial wildfire disease have affected the composition and assembly of the bacterial community of tobacco (Nicotiana tabacum L.) phyllosphere. Our results indicated that three time series groups (T1, T2 and T3) formed significantly distinct clusters. The neutral community model (NCM) and beta nearest taxon index (betaNTI) demonstrated that the overall bacterial community assembly was predominantly driven by stochastic processes. Variance partitioning analysis (VPA) further showed that the complete set of the morbidity and climatic variables together could explain 35.7% of the variation of bacterial communities. The node numbers of the molecular ecological networks (MENs) showed an overall uptrend from T1 to T3. Besides, Pseudomonas is the keystone taxa in the MENs from T1 to T3. PICRUSt2 predictions revealed significantly more abundant genes of osmoprotectant biosynthesis/transport in T2, and more genes for pathogenicity and metabolizing organic substrate in T3. Together, this study provides insights into spatiotemporal patterns, processes and response mechanisms underlying the phyllospheric bacterial community.
The leaf surface on which microorganisms inhabit is called phyllosphere, which is an independent microhabitat of plant foliar surface. Bacteria, fungi, algae and other microorganisms living in these regions are called phyllospheric microorganisms, the structure of which is affected by a range of abiotic and biotic factors, including space (Rastogi et al., 2012) , growth season (Copeland et al., 2015) , climate variation (Medina-Martínez et al., 2015) , solar radiation (Truchado et al., 2017) and disease severities (Zheng et al., 2022) . The phyllospheric area has long been considered an unfavorable habitat for microbial colonization due to long time exposure to solar/ultraviolet radiation, severe diurnal temperature, desiccation, fluctuation of humidity, scouring rainfall, and the scarcity of available nutrients. Recent high-throughput sequencing technologies have enabled the characterization exhaustively the spatio-temporal structure of phyllosphere microbiome. It has also been found that there are a large number of microorganisms inhabiting the phyllosphere, with a density as high as 106-107 cells per square centimeter with multiple biological functions, such as improving plant disease resistance, biocontrol of phytopathogens, enhancing nitrogen fixation, decomposing toxic and harmful substances, and producing plant hormones, volatile organic compounds (VOCs) to promote plant growth (Vorholt, 2012; Xu et al., 2022) . Besides, the phyllosphere also stands for a suitable model system for testing basic principles in ecology since it is amenable for experiments and visual inspection. Such research has implications for fields like plant health and environmental chemistry (Redford and Fierer, 2009; Remus-Emsermann and Schlechter, 2018) .
A thorough understanding of the ecological drivers of phyllospheric community assembly and multitrophic interactions is vital to develop strategies for plant protection. However, many research aspects of phyllospheric microbiome still lag behind in comparison with rhizospheric studies.
Tobacco (Nicotiana tabacum L.), is a model plant and important economic crop, and an ideal research object to study plant-microbe interactions under multiple stresses (Xiang et al., 2022). Tobacco is usually grown in summer and harvested at the end of August. Tobacco’s leaves are continually subjected to excessive strong sunlight on summer days, which makes it an ideal material to study the mechanism of bacterial community assembly and succession in the face of strong abiotic stresses (i.e., UV radiation, desiccation and heat), accompanied with pathogen invasion (Bringel and Couée, 2015; Xing et al., 2022). Dai et al. (2022) investigated the spatiotemporal variation of the community tobacco leaves affected by brown spot disease and found that the relative abundance of Pseudomonas, Sphingomonas, and Methylobacterium increased as tobacco leaves aging gradually. Besides, Liu et al. (2022) found that the inoculation of Bacillus velezensis SYL-3 could increase the abundance of beneficial bacteria, (Pseudomonas and Sphingomonas), while suppress the pathogens Alternaria alternata and tobacco mosaic virus (TMV).
In the current study, we have tried to (i) elucidate successions of the taxonomic and functional profile in the phyllospheric bacterial community under abiotic and biotic stresses of three time periods T1, T2 and T3 in summer (corresponding to the June, July and August of the year 2021), (ii) investigate which members in different bacterial community conferred positive effects on the plant upon abiotic and biotic stresses, and (iii) compare the networks of different period to provide insights into the key taxa of communities.
A total of 2,302,148 high-quality paired 16S rRNA sequences and 3,276 operational taxonomic units (OTU) were obtained from 36 tobacco phyllospheric bacterial DNA samples, (average: 63,949; range: 47,651–79,418 reads per sample). The ternary phase diagrams (Figure 1A) show the relative abundance and relationships of the different taxonomic categories in the three time series groups (T1, T2 and T3), with the circle’s size and vicinity to the vertex proportional to relative abundance in the respective group. We found that the phylum Proteobacteria, class Gammaproteobacteria and orders Pseudomonadales and Enterobacteriales made up the majority of phyllospheric bacterial taxa (Figures 1A, B). In comparison, the phyllosphere of other species from the solanaceous family such as tomato (Lycopersicon esculentum) is also additionally dominant by Rhizobium, Methylobacterium, and Xanthomonas (Ottesen et al., 2013; Toju et al., 2019).
Figure 1 Bacterial community composition and diversity in tobacco phyllosphere. (A) The ternary phase diagrams showing the relative abundance and relationships of the different taxonomic categories (top, phylum; middle, class; bottom, order) in the three time series groups (three vertices, T1, T2 and T3). The size of each circle is proportional to relative abundance; the closer the circle is to the vertex, the higher the relative abundance of the group in that group. (B) Stack bar chart showing the twenty most abundant OUT taxa in each sample. (C) Principal coordinate analysis (PCoA) of Bray–Curtis dissimilarity matrices showing effects of time series (T1, T2 and T3 groups) on the tobacco phyllospheric bacterial community structure. (D) Venn diagram depicting number of shared or unique OTUs in each time series group (T1, T2 and T3).
Principal coordinate analysis (PCoA) of Bray–Curtis distance (Figure 1C) revealed that the microbiome from three time series groups (T1, T2 and T3) formed three significantly distinct clusters, indicating that phyllospheric microbiome from different time periods exhibited distinct community compositions. The first three axes together explained 75.4% of the cumulative variation (ANOISM analysis, p <0.001, R=0.597). A total of 556 OTUs are shared across three groups (Figure 1D), and T3 group comparatively has more unique OTUs (795), followed by T2 group (628) and T1 group (591). Alpha diversity indexes, including Richness, Shannon, Simpson, Pielou, and invsimpson showed an obvious uptrend from the T1 group to T3 group (Figure 2).
Figure 2 Alpha diversity indexes including Richness, Shannon, Simpson, Pielou, invsimpson, Chao1, ACE, and goods coverage of tobacco phyllospheric bacterial communities in each time series group (T1, T2 and T3).
To determine the changes in bacterial community composition across time series, LEfSe analysis was applied to find the differential taxa in each group. The linear discriminant analysis (LDA) score is positively correlated with the significance of bacterial biomarkers in each group (Figure 3). Comparatively, T1 group shows enrichment of bacterial families like Enterobacteriaceae (LDA =5.89) and Haliangiaceae (LDA =3.12), genra Azorhizobium (LDA =3.37) and Bradyrhizobium (LDA =3.34), and species Azorhizobium doebereinerae (LDA =3.34). Members of Enterobacteriaceae in plant phyllosphere are sensitive to abiotic or biotic stresses (Erlacher et al., 2015), whose population sizes decline upon desiccation stress on leaves (Brandl and Mandrell, 2002; Brandl, 2006; Whipps et al., 2008), and they contribute to the overall phyllospheric resistome (Cernava et al., 2019).
Figure 3 The linear discriminant analysis effect size (LEfSe) analysis at species level of bacterial communities (with LDA score >3.1 and p < 0.05) among T1, T2 and T3 groups presented by (A) cladogram (B) and distribution histogram.
At the same time, T2 group shows enrichment of bacterial phylum Cyanobacteria (LDA =5.13), Firmicutes (LDA =3.37) and Actinobacteria (LDA =3.09), class like Alphaproteobacteria (LDA =3.59), orders like Burkholderiales (LDA =3.57) and Oceanospirillales (LDA =3.23). In previous studies, Firmicutes (represented by Bacillus sp.) and Cyanobacteria were considered beneficial to plant growth and pathogen control (Priya et al., 2015; Han et al., 2016; Liu et al., 2022), indicating that plant host might have induced the enrichment of these pathogen antagonists in the phyllosphere during T2 to rescue itself from disease invasion.
Whereas the T3 group shows strong enrichment of genra including Pseudomonas (LDA =5.29), Sphingomonas (LDA =4.19), Agrobacterium (LDA =3.82), and family like Xanthomonadaceae (LDA =3.94). Most Pseudomonas species are beneficial for plants, producing phytohormones and siderophores to the inhibit pathogens (McSpadden et al., 2001; Hsu and Micallef, 2017) and induce host systemic resistance to improve morphological and biochemical traits of plants at the same time (Kumar et al., 2021). Besides, the abundance of Pseudomonas was negatively correlated with the disease index caused by pathogens Alternaria alternate (leaf spot or blight disease) tobacco mosaic virus (TMV) both in tobacco (Liu et al., 2022) and tomato (Gupta et al., 2021). Likewise, Sphingomonas sp., is a kind of gram-negative, aerobic foliar and phytohormone-producing bacterium capable of protecting plants from foliar diseases caused by Pseudomonas syringae (wildfire disease pathogen) via substrate competition (Vogel et al., 2012) and various pathogenic fungi (e.g., Alternaria and Arthrinium) (Enya et al., 2007; Vogel et al., 2012; Luo et al., 2019) and improving plant growth during stress conditions (Asaf et al., 2020). The enrichment of Pseudomonas and Sphingomonas in T3 might be a”cry for help” strategy of tobacco for the recruitment of microbes upon biotic (pathogen invasion) and abiotic stresses (drought and heat) (Wang and Song, 2022).
The neutral community model (NCM) is a validated method for deducing stochastic processes related to community assembly, which has been helpful in explaining various ecological phenomena (Roguet et al., 2015) . This model could quantify the significance of processes that are not easy to observe directly but might have a great impact on microbial communities (i.e., dispersal and ecological drift). In our study, the neutral community model (NCM) has successfully predicted a large fraction of the relation between the occurrence frequency of OTUs and the relative abundance (Figure 4A), with 72.1%, 70.8%, 71.6% and 71.5% of explained community variance for T1, T2, T3 groups and overall, respectively, indicating similar responses of species in different groups to stochastic processes. Besides, the Nm value is relatively higher for T2 group (Nm = 38,332) than T1 group (Nm = 24,237) and T3 group (Nm = 25,770), indicating that the species dispersal was higher in T2 group. Consistently, the majority of betaNTI values of these tobacco phyllospheric bacterial communities fall within -2 and +2 (stochastic process) in all groups (Figure 4B). The distributions of betaNTI shifted with a downtrend from higher betaNTI in T1 showing more deterministic community assembly processes (betaNTI > 2) to lower betaNTIs in T2 and T3 predominant with stochastic community assembly processes (-2 < betaNTI < +2). Besides, the estimated niche width of T3 group is significantly greater than that of T2 and T1 (Figure 4C). These results suggested that stochasticity was more important than determinism in influencing the tobacco phyllospheric bacterial community
Figure 4 Analyses on factors that impacted the relative abundance and occurrence frequency of microbes in tobacco phyllosphere. (A) Fit of the neutral community model (NCM) of community assembly. The OTUs more frequently present than predicted are in cyan, whereas those less frequently are in red. The blue dashed lines represent 95% confidence intervals around the model prediction and the OTUs fallen into the confidence intervals are regarded as neutrally distributed. Nm indicates the meta-community size times immigration, Rsqr indicates the fit to the neutral model. Neutral processes are the part within 95% confidence interval (red) while non-neutral are the parts including above and below prediction (dark green); (B) beta nearest taxon index (betaNTI) comparison; (C) niche breadth comparison; (D) redundancy analysis (RDA) of the relationships between bacterial community in tobacco leaves and environmental variables, including morbidity variables (disease incidence rate: IR, and disease index: DI) and climatic factors (temperature: TEMP, humidity: HM, and rainfall capacity: RC), (E) variance partitioning analysis (VPA) showing contributions of morbidity and climatic variables to tobacco phyllospheric bacterial community variation. Asterisks indicate significance; *, p < 0.05; ***, P< 0.001.
It has been demonstrated that environmental factors (e.g., water, temperature, nutrient and metal concentrations) affect the microbial community composition, which further impact the relative abundance and occurrence frequency of microbes in the neutral or non-neutral distribution . (Liu et al., 2013; Logares et al., 2013; Zhang et al., 2018) Rainfall always concentrates in the summer season in the subtropical area of China. Zhangjiajie region (the sample collection site) generally has the annual precipitation peak in July (corresponding to T2), according to data from national meteorological center of China (http://www.nmc.cn/). Such rainfall abounding conditions, along with accompanying high aerial humidity, could increase the air retention time of microbe (pathogen) and facilitate their passive dispersal across space with higher immigration rate and spores germination on the leaf surface. This might explain why the T2 group has the highest Nm-value in the neutral community model, similar to what have observed in previous studies (Chen et al., 2019) .
Redundancy analysis (RDA) was further applied to reveal the relationship between phyllospheric bacterial populations and factors (Figure 4D). RDA results showed that morbidity variables, including wildfire disease incidence rate (IR) and disease index (DI), are positively correlated with temperature (TEMP) and OTU406145 (Pseudomonas syringae, the pathogen of bacterial wildfire disease), whereas they negatively correlated with OTU467605 (Cyanobacteria), indicating that Cyanobacteria is the potential disease biocontrol agent. Consistently, a previous study also indicated that Cyanobacteria was a major phylum on the leaf of tobacco (Xing et al., 2021) and Cyanobacteria may play a major role in nutrient cycling and water storage in the phyllosphere (Fürnkranz et al., 2008). Besides, humidity (HM) and rainfall capacity (RC) are consistently and positively correlated with Enterobacteriaceae (OTU559204, OTU581021, OTU813217, OTU656889, OTU922761). This is consistent with previous reports that members of Enterobacteriaceae in the phyllosphere are capable of rapid reproduction and formation of aggregates under high moisture conditions and are sensitive to fluctuations in water availability on plant surfaces (Brandl and Mandrell, 2002; Brandl, 2006; Whipps et al., 2008).
Overall, the morbidity (IR, DI) and climatic factors (TEMP, HM, RC) have significantly affected the phyllospheric bacterial community. Variance partitioning analysis (VPA) further showed that the complete set of the morbidity and climatic variables together could explain 35.7% of the variation of tobacco phyllospheric bacterial communities, with climatic variables contributing most (Figure 4E). Still, the high proportion of unexplained variation in VPA also suggested the potential importance of neutral or stochastic processes during community assembly.
Molecular ecological networks (MENs) were constructed to unravel how the combinations of bacterial wildfire disease and climatic factors have affected microbial interactions across the three time periods (Figure 5). The topological properties of the three sub networks were shown in Table 1. The node numbers of the phyllosphere networks showed an overall uptrend from T1 to T3. Whereas link numbers increase sharply from T1 (639) to T2 (14,401), followed by a rapid decrease to T3 (3,533). Similar trend is also found in MEN properties like the average number of neighbors, network density (comparison between the edges available in a graph and a graph with all possible edges), network centralization (measure of how much the degree of every node is far from the degree of the highest degree node), and connected components (a maximal set of nodes such that each pair of nodes is connected by a path). This indicated that T2 was more complex, with abundant interactions in a highly connected microbial community, which might also be explained by the rainfall peak at T2 that has provided great growth opportunity for microbes to multiply and make connections as mentioned above. A similar phenomenon of relatively higher connections was also observed in the wilt diseased rhizoplane of tobacco (Tao et al., 2022) . The percentage of positive correlation follow largely a downtrend from T1 (57.4%) to T3 (53.6%), indicating the decrease of cooperative relation. Besides, it is predicted by the program cytoHubba (Chin et al., 2014) that Pseudomonas is the keystone taxa and is present in the top 10 important nodes in the MENs from T1 to T3 (Figure 5). Other frequently present keystone taxa includes Sphingomonas and Labrys. These taxa are reported to be dominant in plant phyllosphere and might play important roles in inhibiting plant disease (Liu et al., 2021; Liu et al., 2022).
Figure 5 Molecular ecological networks of phyllospheric bacterial community. Each node represents an OTU. The size of each node is proportional to the number of connections (that is, degree) and the colors of nodes represent different order. The links between the nodes indicate strong and significant (P < 0.01) correlations. A red line indicates a negative interaction between two individual nodes, while a blue line indicates a positive interaction. (A) Overall molecular ecological network; (B) spearman correlation between environmental factor, alpha diversity index, and OTUs. (C) molecular ecological network of T1 group (left) and predicted keystone nodes (right); (D) molecular ecological network of T2 group (left) and predicted keystone nodes (right); (E) molecular ecological network of T3 group (left) and predicted keystone nodes (right).
To assess the putative effect of stress factors on the bacterial community functions of different time periods, metagenome of tobacco phyllospheric bacterial communities were predicted with PICRUSt2 (Douglas et al., 2020) followed by annotation in referring to the KEGG database. A total of 7,557 KOs (KEGG Orthologs) were predicted across three groups, and some of them are significantly different among groups (Figures 6A, B). For example, biosynthesis/transport genes of osmoprotectant against osmotic stress (opuA, opuBD, treA, otsA) in T2 were predicted to be more abundant than T3 (Figure 6B). At the same time, the relative abundance of genes encoding resistance–nodulation–division (RND) type cobalt−zinc−cadmium resistance protein, periplasmic protein TonB, sirohydrochlorin ferrochelatase, twitching motility protein PilU and several metabolic genes for organic substrate (atoB, paaH, prnA, prpB, ctpA, pepO, aguA, abfA) is significantly increased in T3. We proposed that these genes enriched in the T3 group were related with the deterioration of wildfire disease, since previous studies have found RND efflux pumps of plant-pathogenic P. syringae pathovars essential for in planta reproduction and evasion of the host native immune response (Stoitsova et al., 2008) . Besides, TonB-dependent receptor is regarded as a feature shared by phytopathogenic bacteria for the uptake of various carbohydrates under environmental conditions (Blanvillain et al., 2007) . Motility can help wildfire disease pathogen search for favorable sites, facilitate spreading and locate the most preferred site (Haefele and Lindow, 1987) . Toxin such as syringomycin produced by Pseudomonas syringae is responsible for pore formation and nutrient leakage through the host cell membrane (Pritchard et al., 2009) , Besides, plants generally produce phenolic substances to counteract increased stress levels (Lucas et al., 2022). This might explain the significant enrichment of organic metabolic genes in the T3 group community.
Figure 6 PICRUSt predicted metagenome functions with significant difference in abundance between groups at KO level. (A) T1 vs. T3; (B) T2 vs. T3.
Collectively, this study has contributed to improving the understanding of the spatiotemporal patterns of tobacco phyllospheric microbiome and shed light on the putative underlying mechanism.
The standards used to examine tobacco bacterial wildfire disease were based on the tobacco pest classification and survey methods (GB/T 23222–2008), P.R. China. Disease incidence was calculated by the percentage of diseased tobacco in each field. Disease index was calculated using the formula:
r is the disease severity; N is the number of infected tobaccos with a rating of r; n is the total number of tobaccos tested, and R is the value of the highest disease severity in each field. Meteorological data were retrieved from the National Meteorological Center of China (http://www.nmc.cn/).
The tobacco leaf samples were collected in Gaofeng Town, Cili County, Zhangjiajie City, Hunan Province (29°28′5″N, 110°57′53″E), China, at June (T1), July (T2) and August (T3) of 2021. In the test areas, tobacco fields having typical and serious bacterial wildfire disease levels were selected. In the same plot, with similar conditions, plants with typical symptoms of bacterial wildfire disease were sampled, with three plants per group. The experiment adopted a random block design with three duplicates; the plot area was 90 m2. Other field management measures were carried out in accordance with local planting practices. A total of 18 plants were randomly selected from each plot, and the middle leaves of every sixth plant were taken as a sample, which was kept at 4°C and brought back to the laboratory for subsequent foliar microbial DNA extractions.
Fifteen grams of leaf samples obtained from various parts of the leaf surface (avoiding the main and branch veins) using a sterile puncher were added to 50 mL of 0.1% Tween-80 bacterial phosphate buffer (pH 7.0). The samples were then shaken for 30 min at 170 revolutions/min (rpm) and 28°C. The bacterial suspension was then collected, and the leaf samples were washed twice more. The collected suspensions were centrifuged for 15 min (4°C, 10,000 rpm) to pellet the microorganisms. The pellet was suspended in sterile water and washed three times. Finally, the microorganisms were resuspended with 1 mL of sterile water for subsequent DNA extraction. Genomic DNA extraction of foliar microorganisms was performed using the Plant Genomic DNA Kit (Plant Genomic DNA Kit), following the manufacturer’s protocol. We used the primer pair 341F (5’-CCT ACG GGN GGC WGC AG-3’) and 805R (5’-GAC TAC HVG GGTATC TAA TCC-3’) of V3/V4 regions to amplify the 16S rRNA. Amplicons were sequenced by Illumina NovaSeq PE250 platform (LC-Bio Technology Co., Ltd, Hang Zhou, Zhejiang Province, China). The raw sequencing data were deposited in the European Nucleotide Archive database under accession number PRJEB56205.
Raw sequences were split into sample libraries with perfect matches to barcodes. Low-quality sequences with QC < 20 over a 5-bp window size were trimmed using Btrim (Kong, 2011) , and sequences with a length of < 100 bp were removed. Then, the forward and reverse sequences were spliced together. Any sequences containing ambiguous bases or the incorrect length were removed, and the remaining sequences were compared against the UNITE v8.2 database (Kõljalg et al., 2005) to remove possible chimeras. The length of the sequencing fragment was 200–400 bp. Then, UPARSE (Edgar, 2013) was used to cluster and produce operational taxonomic units (OTUs) at 97% similarity level. To ensure the authenticity of the data, we removed OTUs that were represented by only one sequence in overall data (global singletons). All statistical analyses and calculations were carried out using the R (v 3.6.3) statistical platform (www.r-project.org).
To construct a microbial association network, correlations between pairwise OTUs that were present in more than half of the samples were calculated using the SparCC method (Friedman and Alm, 2012). Only edges with a significant correlation higher than 0.5 (p < 0.01) were retained for network construction. Cytoscape v.3.9.1 (https://cytoscape.org) was used for network visualization. Cytoscape plugin cytoHubba (Chin et al., 2014) with Maximal Clique Centrality (MCC) method was used to predict essential/keystone nodes in the network.
To determine the potential importance of stochastic processes on community assembly, we adopted a neutral community model (NCM) to predict the relationship between OTU detection frequencies and their relative abundance across the wider metacommunity, performed using R (version 3.6.3). The assembly processes of bacterial and fungal communities were evaluated by calculating the nearest taxon index and beta nearest taxon index (betaNTI) using the “picante” package. A betaNTI value < 2 indicates that the contribution is a stochastic process, and when betaNTI > 2 the shifts in community composition were considered to be shaped by deterministic processes.
The Phylogenetic Investigation of Communities by Reconstruction of Unobserved States (PICRUSt2) (Douglas et al., 2020) was applied to predict potential functional profiles of the bacterial community using 16S rRNA gene data.
The datasets presented in this study can be found in online repositories. The names of the repository/repositories and accession number(s) can be found below: https://www.ebi.ac.uk/ena, PRJEB56205.
LL and HY conceived and designed the research. ZW, CF, JT, WW, DP, XD, HT and XZ conducted the experiment and analyzed the data. LL wrote the manuscript. All authors read and approved the manuscript.
This research was supported by the key project of Science and Technology of Hunan Branch of China National Tobacco Corporation (202104, HN2020KJ02).
We are grateful for resources from the High-Performance Computing Center of Central South University.
Author ZW, CF, JT, WW, DP, XD and HT are employed by Zhangjiajie Tobacco Company of Hunan Province. Author XZ is employed by Yongzhou Tobacco Company of Hunan Province.
The remaining authors declare that the research was conducted in the absence of any commercial or financial relationships that could be constructed as a potential conflict of interest.
The authors declare that this study received funding from Science and Technology of Hunan Branch of China National Tobacco Corporation. The funder was not involved in the study design, collection, analysis, interpretation of data, the writing of this article, or the decision to submit it for publication.
All claims expressed in this article are solely those of the authors and do not necessarily represent those of their affiliated organizations, or those of the publisher, the editors and the reviewers. Any product that may be evaluated in this article, or claim that may be made by its manufacturer, is not guaranteed or endorsed by the publisher.
Asaf, S., Numan, M., Khan, A. L., Al-Harrasi, A. (2020). Sphingomonas: From diversity and genomics to functional role in environmental remediation and plant growth. Crit. Rev. Biotechnol. 40, 138–152. doi: 10.1080/07388551.2019.1709793
Blanvillain, S., Meyer, D., Boulanger, A., Lautier, M., Guynet, C., Denancé, N., et al. (2007). Plant carbohydrate scavenging through tonB-dependent receptors: A feature shared by phytopathogenic and aquatic bacteria. PloS One 2, e224. doi: 10.1371/journal.pone.0000224
Brandl, M. T. (2006). Fitness of human enteric pathogens on plants and implications for food safety. Annu. Rev. Phytopathol. 44, 367–392. doi: 10.1146/annurev.phyto.44.070505.143359
Brandl, M. T., Mandrell, R. E. (2002). Fitness of salmonella enterica serovar Thompson in the cilantro phyllosphere. Appl. Environ. Microbiol. 68, 3614–3621. doi: 10.1128/AEM.68.7.3614-3621.2002
Bringel, F., Couée, I. (2015). Pivotal roles of phyllosphere microorganisms at the interface between plant functioning and atmospheric trace gas dynamics. Front. Microbiol. 6. doi: 10.3389/fmicb.2015.00486
Cernava, T., Erlacher, A., Soh, J., Sensen, C. W., Grube, M., Berg, G. (2019). Enterobacteriaceae dominate the core microbiome and contribute to the resistome of arugula (Eruca sativa mill.). Microbiome 7, 13. doi: 10.1186/s40168-019-0624-7
Chen, W., Ren, K., Isabwe, A., Chen, H., Liu, M., Yang, J. (2019). Stochastic processes shape microeukaryotic community assembly in a subtropical river across wet and dry seasons. Microbiome 7, 138. doi: 10.1186/s40168-019-0749-8
Chin, C. H., Chen, S. H., Wu, H. H., Ho, C. W., Ko, M. T., Lin, C. Y. (2014). CytoHubba: Identifying hub objects and sub-networks from complex interactome. BMC Syst. Biol. 8 Suppl 4, S11. doi: 10.1186/1752-0509-8-S4-S11
Copeland, J. K., Yuan, L., Layeghifard, M., Wang, P. W., Guttman, D. S. (2015). Seasonal community succession of the phyllosphere microbiome. Mol. Plant Microbe Interact. 28, 274–285. doi: 10.1094/MPMI-10-14-0331-FI
Dai, Y. F., Wu, X. M., Wang, H. C., Li, W. H., Cai, L. T., Li, J. X., et al. (2022). Spatio-temporal variation in the phyllospheric microbial biodiversity of alternaria alternata-infected tobacco foliage. Front. Microbiol. 13. doi: 10.3389/fmicb.2022.920109
Douglas, G. M., Maffei, V. J., Zaneveld, J. R., Yurgel, S. N., Brown, J. R., Taylor, C. M., et al. (2020). PICRUSt2 for prediction of metagenome functions. Nat. Biotechnol. 38, 685–688. doi: 10.1038/s41587-020-0548-6
Edgar, R. C. (2013). UPARSE: Highly accurate OTU sequences from microbial amplicon reads. Nat. Methods 10, 996–998. doi: 10.1038/nmeth.2604
Enya, J., Shinohara, H., Yoshida, S., Tsukiboshi, T., Negishi, H., Suyama, K., et al. (2007). Culturable leaf-associated bacteria on tomato plants and their potential as biological control agents. Microb. Ecol. 53, 524–536. doi: 10.1007/s00248-006-9085-1
Erlacher, A., Cardinale, M., Grube, M., Berg, G. (2015). Biotic stress shifted structure and abundance of enterobacteriaceae in the lettuce microbiome. PloS One 10, e118068. doi: 10.1371/journal.pone.0118068
Friedman, J., Alm, E. J. (2012). Inferring correlation networks from genomic survey data. PloS Comput. Biol. 8, e1002687. doi: 10.1371/journal.pcbi.1002687
Fürnkranz, M., Wanek, W., Richter, A., Abell, G., Rasche, F., Sessitsch, A. (2008). Nitrogen fixation by phyllosphere bacteria associated with higher plants and their colonizing epiphytes of a tropical lowland rainforest of Costa Rica. ISME. J. 2, 561–570. doi: 10.1038/ismej.2008.14
Gupta, A. K., Verma, J., Srivastava, A., Srivastava, S., Prasad, V. (2021). A comparison of induced antiviral resistance by the phytoprotein CAP-34 and isolate P1f of the rhizobacterium pseudomonas putida. 3. Biotech. 11, 509. doi: 10.1007/s13205-021-03057-3
Haefele, D. M., Lindow, S. E. (1987). Flagellar motility confers epiphytic fitness advantages upon pseudomonas syringae. Appl. Environ. Microbiol. 53, 2528–2533. doi: 10.1128/aem.53.10.2528-2533.1987
Han, T., You, C., Zhang, L., Feng, C., Zhang, C., Wang, J., et al. (2016). Biocontrol potential of antagonist bacillus subtilis Tpb55 against tobacco black shank. BioControl 61, 195–205. doi: 10.1007/s10526-015-9705-0
Hsu, C. K., Micallef, S. A. (2017). Plant-mediated restriction of salmonella enterica on tomato and spinach leaves colonized with pseudomonas plant growth-promoting rhizobacteria. Int. J. Food Microbiol. 259, 1–6. doi: 10.1016/j.ijfoodmicro.2017.07.012
Kõljalg, U., Larsson, K. H., Abarenkov, K., Nilsson, R. H., Alexander, I. J., Eberhardt, U., et al. (2005). UNITE: A database providing web-based methods for the molecular identification of ectomycorrhizal fungi. New Phytol. 166, 1063–1068. doi: 10.1111/j.1469-8137.2005.01376.x
Kong, Y. (2011). Btrim: A fast, lightweight adapter and quality trimming program for next-generation sequencing technologies. Genomics 98, 152–153. doi: 10.1016/j.ygeno.2011.05.009
Kumar, Y. V., Krishna, J. R., Kaushik, P., Altalayan, F. H., Al, B. T., Alam, P. (2021). Traversing arbuscular mycorrhizal fungi and pseudomonas fluorescens for carrot production under salinity. Saudi. J. Biol. Sci. 28, 4217–4223. doi: 10.1016/j.sjbs.2021.06.025
Liu, T., Gu, Y., Zhou, Z., Liu, Z., Yin, H., Qin, C., et al. (2021). Ecological strategies of biological and chemical control agents on wildfire disease of tobacco (Nicotiana tabacum l.). BMC Microbiol. 21, 184. doi: 10.1186/s12866-021-02237-8
Liu, H., Jiang, J., An, M., Li, B., Xie, Y., Xu, C., et al. (2022). Bacillus velezensis SYL-3 suppresses alternaria alternata and tobacco mosaic virus infecting nicotiana tabacum by regulating the phyllosphere microbial community. Front. Microbiol. 13. doi: 10.3389/fmicb.2022.840318
Liu, L., Yang, J., Yu, X., Chen, G., Yu, Z. (2013). Patterns in the composition of microbial communities from a subtropical river: Effects of environmental, spatial and temporal factors. PloS One 8, e81232. doi: 10.1371/journal.pone.0081232
Logares, R., Lindström, E. S., Langenheder, S., Logue, J. B., Paterson, H., Laybourn-Parry, J., et al. (2013). Biogeography of bacterial communities exposed to progressive long-term environmental change. Isme. J. 7, 937–948. doi: 10.1038/ismej.2012.168
Lucas, J. A., García-Villaraco, A., Ramos-Solano, B., Akdi, K., Gutierrez-Mañero, F. J. (2022). Lipo-chitooligosaccharides (LCOs) as elicitors of the enzymatic activities related to ROS scavenging to alleviate oxidative stress generated in tomato plants under stress by UV-b radiation. Plants (Basel). 11. doi: 10.3390/plants11091246
Luo, L., Zhang, Z., Wang, P., Han, Y., Jin, D., Su, P., et al. (2019). Variations in phyllosphere microbial community along with the development of angular leaf-spot of cucumber. AMB. Express. 9, 76. doi: 10.1186/s13568-019-0800-y
McSpadden, G. B., Mavrodi, D. V., Thomashow, L. S., Weller, D. M. (2001). A rapid polymerase chain reaction-based assay characterizing rhizosphere populations of 2,4-diacetylphloroglucinol-producing bacteria. Phytopathology 91, 44–54. doi: 10.1094/PHYTO.2001.91.1.44
Medina-Martínez, M. S., Allende, A., Barberá, G. G., Gil, M. I. (2015). Climatic variations influence the dynamic of epiphyte bacteria of baby lettuce. Food Res. Int. 68, 54–61. doi: 10.1016/j.foodres.2014.06.009
Ottesen, A. R., González Peña, A., White, J. R., Pettengill, J. B., Li, C., Allard, S., et al. (2013). Baseline survey of the anatomical microbial ecology of an important food plant: Solanum lycopersicum (tomato). BMC Microbiol. 13, 114. doi: 10.1186/1471-2180-13-114
Pritchard, L., Liu, H., Booth, C., Douglas, E., François, P., Schrenzel, J., et al. (2009). Microarray comparative genomic hybridisation analysis incorporating genomic organisation, and application to enterobacterial plant pathogens. PloS Comput. Biol. 5, e1000473. doi: 10.1371/journal.pcbi.1000473
Priya, H., Prasanna, R., Ramakrishnan, B., Bidyarani, N., Babu, S., Thapa, S., et al. (2015). Influence of cyanobacterial inoculation on the culturable microbiome and growth of rice. Microbiol. Res. 171, 78–89. doi: 10.1016/j.micres.2014.12.011
Rastogi, G., Sbodio, A., Tech, J. J., Suslow, T. V., Coaker, G. L., Leveau, J. H. (2012). Leaf microbiota in an agroecosystem: Spatiotemporal variation in bacterial community composition on field-grown lettuce. Isme. J. 6, 1812–1822. doi: 10.1038/ismej.2012.32
Redford, A. J., Fierer, N. (2009). Bacterial succession on the leaf surface: A novel system for studying successional dynamics. Microbial. Ecol. 58, 189–198. doi: 10.1007/s00248-009-9495-y
Remus-Emsermann, M. N. P., Schlechter, R. O. (2018). Phyllosphere microbiology: At the interface between microbial individuals and the plant host. New Phytol. 218, 1327–1333. doi: 10.1111/nph.15054
Roguet, A., Laigle, G. S., Therial, C., Bressy, A., Soulignac, F., Catherine, A., et al. (2015). Neutral community model explains the bacterial community assembly in freshwater lakes. FEMS Microbiol. Ecol. 91. doi: 10.1093/femsec/fiv125
Stoitsova, S. O., Braun, Y., Ullrich, M. S., Weingart, H. (2008). Characterization of the RND-type multidrug efflux pump MexAB-OprM of the plant pathogen pseudomonas syringae. Appl. Environ. Microbiol. 74, 3387–3393. doi: 10.1128/AEM.02866-07
Tao, J., Yu, S., Jin, J., Lu, P., Yang, Z., Xu, Y., et al. (2022). The wilt pathogen induces different variations of root-associated microbiomes of plant. Front. Plant Sci. 13. doi: 10.3389/fpls.2022.1023837
Toju, H., Okayasu, K., Notaguchi, M. (2019). Leaf-associated microbiomes of grafted tomato plants. Sci. Rep. 91787. doi: 10.1038/s41598-018-38344-2
Truchado, P., Gil, M. I., Reboleiro, P., Rodelas, B., Allende, A. (2017). Impact of solar radiation exposure on phyllosphere bacterial community of red-pigmented baby leaf lettuce. Food Microbiol. 66, 77–85. doi: 10.1016/j.fm.2017.03.018
Vogel, C., Innerebner, G., Zingg, J., Guder, J., Vorholt, J. A. (2012). Forward genetic in planta screen for identification of plant-protective traits of sphingomonas sp. strain Fr1 against pseudomonas syringae DC3000. Appl. Environ. Microbiol. 78, 5529–5535. doi: 10.1128/AEM.00639-12
Vorholt, J. A. (2012). Microbial life in the phyllosphere. Nat. Rev. Microbiol. 10, 828–840. doi: 10.1038/nrmicro2910
Wang, Z., Song, Y. (2022). Toward understanding the genetic bases underlying plant-mediated “cry for help” to the microbiota. IMeta 1, e8. doi: 10.1002/imt2.8
Whipps, J. M., Hand, P., Pink, D. A., Bending, G. D. (2008). Human pathogens and the phyllosphere. Adv. Appl. Microbiol. 64, 183–221. doi: 10.1016/S0065-2164(08)00407-3
Xiang, L. G., Wang, H. C., Wang, F., Cai, L. T., Li, W. H., Hsiang, T., et al. (2022). Analysis of phyllosphere microorganisms and potential pathogens of tobacco leaves. Front. Microbiol. 13. doi: 10.3389/fmicb.2022.843389
Xing, L., Yang, J., Jia, Y., Hu, X., Liu, Y., Xu, H., et al. (2021). Effects of ecological environment and host genotype on the phyllosphere bacterial communities of cigar tobacco (Nicotiana tabacum l.). Ecol. Evol. 11, 10892–10903. doi: 10.1002/ece3.7861
Xing, L., Zhi, Q., Hu, X., Liu, L., Xu, H., Zhou, T., et al. (2022). Influence of association network properties and ecological assembly of the foliar fugal community on crop quality. Front. Microbiol. 13. doi: 10.3389/fmicb.2022.783923
Xu, N., Zhao, Q., Zhang, Z., Zhang, Q., Wang, Y., Qin, G., et al. (2022). Phyllosphere microorganisms: Sources, drivers, and their interactions with plant hosts. J. Agric. Food Chem. 70, 4860–4870. doi: 10.1021/acs.jafc.2c01113
Zhang, W., Pan, Y., Yang, J., Chen, H., Holohan, B., Vaudrey, J., et al. (2018). The diversity and biogeography of abundant and rare intertidal marine microeukaryotes explained by environment and dispersal limitation. Environ. Microbiol. 20, 462–476. doi: 10.1111/1462-2920.13916
Keywords: tobacco, phyllosphere, bacterial community, high-throughput sequencing, molecular ecological networks, neutral community model
Citation: Wang Z, Fu C, Tian J, Wang W, Peng D, Dai X, Tian H, Zhou X, Li L and Yin H (2022) Responses of the bacterial community of tobacco phyllosphere to summer climate and wildfire disease. Front. Plant Sci. 13:1050967. doi: 10.3389/fpls.2022.1050967
Received: 22 September 2022; Accepted: 08 November 2022;
Published: 21 December 2022.
Edited by:
Juan Li, Hunan Agricultural University, ChinaReviewed by:
Yi Cheng, Chinese Academy of Agricultural Sciences (CAAS), ChinaCopyright © 2022 Wang, Fu, Tian, Wang, Peng, Dai, Tian, Zhou, Li and Yin. This is an open-access article distributed under the terms of the Creative Commons Attribution License (CC BY). The use, distribution or reproduction in other forums is permitted, provided the original author(s) and the copyright owner(s) are credited and that the original publication in this journal is cited, in accordance with accepted academic practice. No use, distribution or reproduction is permitted which does not comply with these terms.
*Correspondence: Huaqun Yin, eWluaHVhcXVuX2NzQHNpbmEuY29t; Liangzhi Li, MjA1NjAxMDA2QGNzdS5lZHUuY24=
Disclaimer: All claims expressed in this article are solely those of the authors and do not necessarily represent those of their affiliated organizations, or those of the publisher, the editors and the reviewers. Any product that may be evaluated in this article or claim that may be made by its manufacturer is not guaranteed or endorsed by the publisher.
Research integrity at Frontiers
Learn more about the work of our research integrity team to safeguard the quality of each article we publish.