- 1School of Chemical and Environmental Engineering, Wuhan Polytechnic University, Wuhan, China
- 2Guangdong Provincial Key Laboratory of Utilization and Conservation of Food and Medicinal Resources in Northern Region, Shaoguan University, Shaoguan, Guangdong, China
RNA-seq has become a state-of-the-art technique for transcriptomic studies. Advances in both RNA-seq techniques and the corresponding analysis tools and pipelines have unprecedently shaped our understanding in almost every aspects of plant sciences. Notably, the integration of huge amount of RNA-seq with other omic data sets in the model plants and major crop species have facilitated plant regulomics, while the RNA-seq analysis has still been primarily used for differential expression analysis in many less-studied plant species. To unleash the analytical power of RNA-seq in plant species, especially less-studied species and biomass crops, we summarize recent achievements of RNA-seq analysis in the major plant species and representative tools in the four types of application: (1) transcriptome assembly, (2) construction of expression atlas, (3) network analysis, and (4) structural alteration. We emphasize the importance of expression atlas, coexpression networks and predictions of gene regulatory relationships in moving plant transcriptomes toward regulomics, an omic view of genome-wide transcription regulation. We highlight what can be achieved in plant research with RNA-seq by introducing a list of representative RNA-seq analysis tools and resources that are developed for certain minor species or suitable for the analysis without species limitation. In summary, we provide an updated digest on RNA-seq tools, resources and the diverse applications for plant research, and our perspective on the power and challenges of short-read RNA-seq analysis from a regulomic point view. A full utilization of these fruitful RNA-seq resources will promote plant omic research to a higher level, especially in those less studied species.
Introduction
RNA-seq and its-derived techniques have been commercially available and routinely used by biological scientists, largely owing to the rapidly increased outputs of major sequencing platforms, improved sequencing accuracy and ever reduced costs (Stark et al., 2019). RNA-seq has shaped nearly every aspects of our understanding in plant research, from plant development and phytohorome signaling to plant metabolism and stress tolerance.
RNA-seq can be divided into the short-read (Nagalakshmi et al., 2008) and long-read RNA-seq technologies (Sharon et al., 2013). In short-read RNA-seq, Illumina sequencing platform has been dominant, while other platforms, such as Thermo Scientific platforms (e.g., Ion PGM and Ion S5) or the BGI Genomics platforms (e.g., DNBSEQ), have been frequently used in certain circumstances or been gaining attentions recently (Patterson et al., 2019; Foox et al., 2021). A short-read RNA-seq library is typically sequenced to a read depth of 10~30 million reads per sample with a read length varied from 50 to 200 bp. By contrast, a number of approaches (e.g., Pacific Bioscience, PacBio and Oxford Nanopore, ONT) provide long, uninterrupted sequencing of a single RNA or DNA molecules, constituting the third generation of real-time fluorescence sequencing paradigm (Sharon et al., 2013; Cartolano et al., 2016; Oikonomopoulos et al., 2016). A typical long-read RNA-seq produces 500,000 to 10 million reads per run with a read length ranging from 1,000 to 50,000 bp depending on the technologies and platforms (Stark et al., 2019). The long-read sequencing platforms are particularly suited for de novo transcriptome assembly and identification of novel transcripts and isoforms, as these approaches overcome some intrinsic issues related to short-read sequencing.
While the rise of the long-read RNA-seq, the short-read RNA-seq still is dominating the current utilizations in plant sciences and has provided the majority of the data sets deposited in public sequencing databases. With the recent advancement of tools developed for analyzing short-read sequencing data, the RNA-seq technology can be used for various applications, including but not limited to: (1) de novo assembly of transcriptome with or without a reference genome; (2) detection of new transcripts or correction of existing gene structures based on RNA-seq evidence; (3) to obtaining the expression profiles at gene or transcript levels and to construct the expression atlas covering a range of conditions and tissue types; (4) to identify alternative splicing and alternative 5’ or 3’ untranslated regions (5’UTR or 3’UTR, respectively); (5) to construct gene co-expression networks (GCNs) and predict gene regulatory relationships in a large scale (also known as gene regulatory networks, GRN). Here, GCN stand for a network that can be constructed from a large set of RNA-seq data and includes multiple clusters or modules. The module represents a group of genes determined statistically with high correlation in their expression profiles and usually associations in their functions (reviewed in Gupta and Pereira, 2019). Notably, many genes within the same module do not represent the direct targets of their upstream regulators. Thus, to further disentangle the direct regulator-targets pairs from the indirectly regulated or co-expressed genes, prediction of GRNs is another important task in RNA-seq data analysis. Identification of GRNs can be achieved by harnessing the following resources: (1) identifying transcription factors (TFs) from co-expressed modules; (2) identifying a group of co-expressed genes with the statistically enriched cis-regulatory elements from a certain family of TF; (3) leveraging the information of direct TF targets by using existing results from chromatin immunoprecipitation sequencing (ChIP-seq) or DNA affinity purification sequencing (DAP-seq) experiments (O’Malley et al., 2016; Galli et al., 2020); (4) applying the well-established algorithms for GRN inference. While the many utilizations of RNA-seq, the differential gene expression (DGE) is still the most often used analysis in many plant researches, especially those carried on in crop species.
Here, we highlight typical examples of the tools and applications that have been used in the model plants (Arabidopsis and rice) and other major crops (e.g., tomato, wheat, maize and soybean) (Table 1). These applications demonstrate the power and comprehensiveness of short-read, bulk RNA-seq analyses. Meanwhile, it is worth noting that DGE has long been the primary analysis in the RNA-seq studies of other less-studied plant species. In fact, many species, especially those minor crops, biomass crops or orphan crops, are key to provide sustainable agriculture and to reach global food and energy security. Particularly, major biomass crops, such as sorghum, sugarcane, Miscanthus, and switchgrass, have large yield of biomass and stress tolerance (Mullet et al., 2014; Boyles et al., 2019), justifying the significance for researching on gene expression and regulation associated with biomass composition and production.
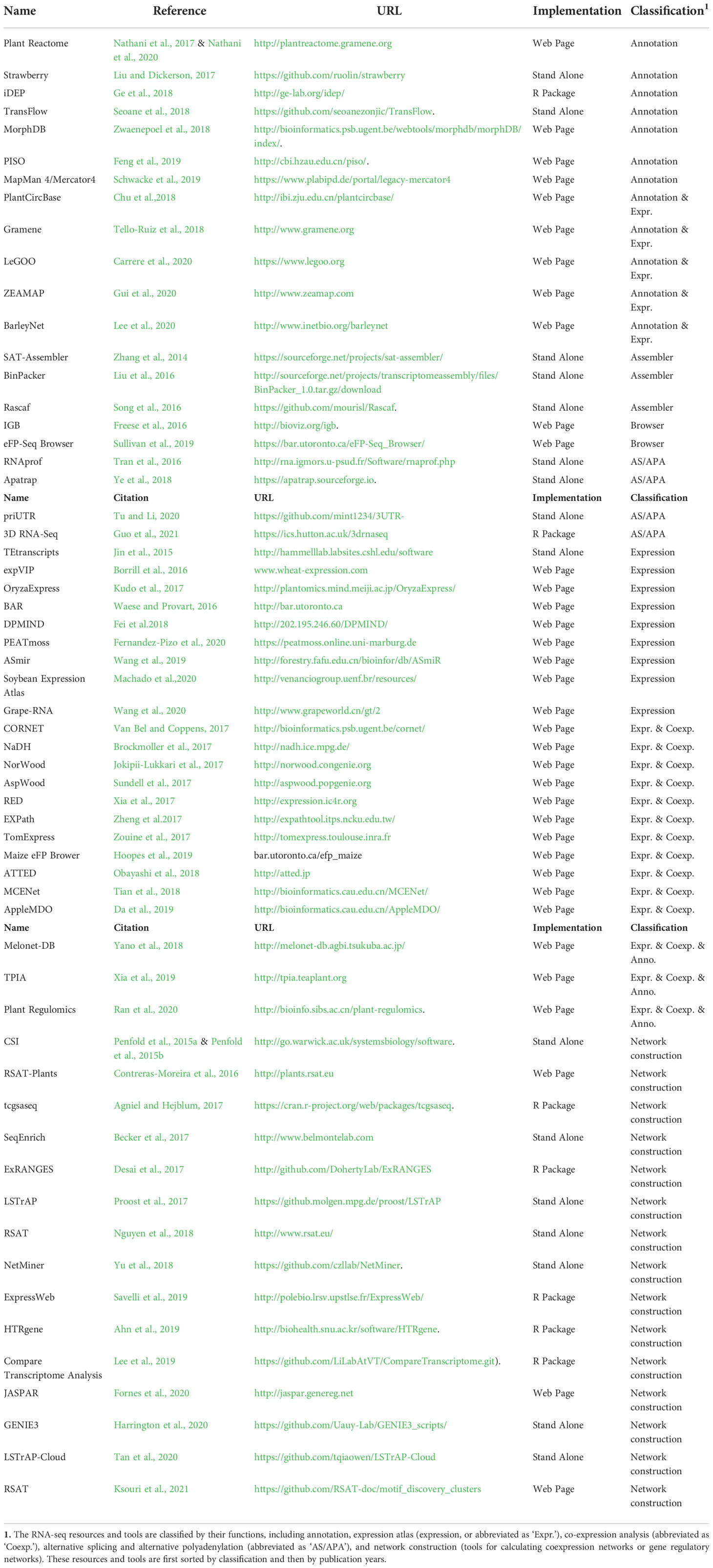
Table 1 Summary of the representative resources and tools for analyzing the short-read RNA-seq data in plants.
The limited utilization of RNA-seq in the minor plant species has been partly due to: (1) the limited genomic resources; (2) lacking bioinformatic tools that are user friendly, with a graphical user interface, or well adapted to the omics data of various species. In this context, we summarize a variety of bioinformatic tools covering the diverse applications of bulk RNA-seq analysis to facilitate the full use of short-read RNA-seq data, and to help unleash the power of bulk RNA-seq in studies of plants, especially in the minor and under-utilized crops (Table 1; Figure 1). Notably, there have been several excellent reviews regarding the development of RNA-seq technologies, comprehensive summary of RNA-seq tools and calculation of GCNs and GRNs in plant sciences (Van Verk et al., 2013; Conesa et al., 2016; Proost and Mutwil, 2016; Gaudinier and Brady, 2016; Sahraeian et al., 2017; Saelens et al., 2018; Haque et al., 2018; Stark et al., 2019; Gupta and Pereira, 2019). We aim at neither comprehensively cataloguing the RNA-seq analysis tools for plant research, nor summarizing the achievements that RNA-seq have been reached in plant research. We emphasize that recent advancements in RNA-seq analysis tools allow to fully unleash the power of short-read, bulk RNA-seq in many plant species like biomass crops, to provide deep insights into gene regulation at multiple levels and to go toward regulomics, an analogous term to other omics that portraits transcription control in a genome-wide manner (Werner, 2003; Werner, 2004). Particularly, regulomics refers to the omic-scale study of gene expression regulation happened at transcriptional or post-transcriptional levels (Werner, 2004), such as the regulation between transcription factors/coregulators and their targets and the interaction between non-coding RNAs (e.g., miRNAs anf lncRNAs) and mRNAs.
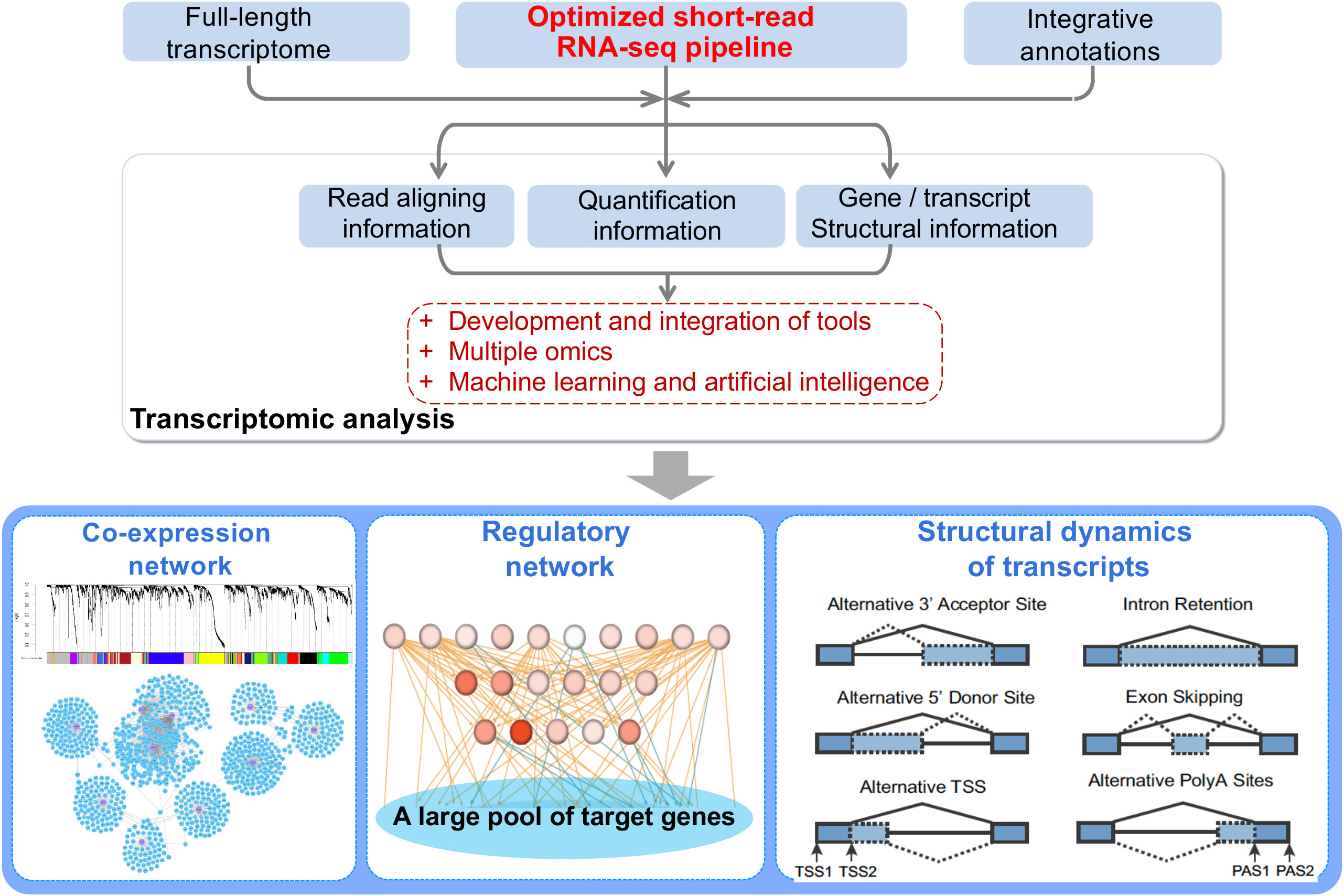
Figure 1 The power of short-read, bulk RNA-seq can be unleashed by integrating the following resources and tools related to RNA-seq analysis: (1) Full-length transcriptome can be achieved by full-length cDNA sequencing, PacBio Iso-seq or the Oxford Nanopore sequencing technologies, and these full-length transcriptomes can help to better annotate gene structures and serve as the basis for expression profiling at the transcript-level. (2) For many less-studied plant species, multiple functional annotation resources can be applied to provide a comprehensive annotation, facilitating biological interpretation of sets of DEG or gene networks. (3) Through application of the tools introduced here and in previous reviews, high-quality GCNs and GRNs can be made to prioritize hub genes or key regulators involved in the certain biological process or phenotypes.
The applications of the short-read, bulk RNA-seq in plant sciences
The short-read RNA-seq technique includes several core steps, from RNA extraction, cDNA synthesis, adapter ligation, PCR amplification, to the sequencing of library and data analysis. Four key stages are required for the RNA-seq data analysis: (1) The first stage takes the raw sequencing reads to quality control and maps the quality-controlled reads to the transcriptome, which can be obtained from a reference genome or be assembled from transcriptomic data; (2) The second stage quantifies the number of reads mapped to each gene or transcript, producing an expression matrix; (3) The third stage modifies the expression matrix by normalization between samples, accounting for technical differences, and removing lowly expressed genes/transcripts; (4) The last stage calculates differentially expressed genes or transcripts by statistical models. Particularly, the number of computational tools for analyzing RNA-seq data has been increased dramatically in the recent decade (Stark et al., 2019). As such, substantial influences can be generated on the biological conclusions drawn from the RNA-seq data due to several aspects: differences in the computational approaches used, software parameters or statistical models selected and distinct combinations of the tools in a pipeline (Conesa et al., 2016). The optimal set of computational approaches for RNA-seq depends on the experimental setup, the biological questions being addressed and other factors, and is beyond the scope of our mini-review (Conesa et al., 2016; Sahraeian et al., 2017). However, several sets of RNA-seq tools are well recognized, representing the classic pipelines (Trapnell et al., 2012; Grabherr et al., 2012; Pertea et al., 2017). These includes five main components: (1) the splice-aware aligners (e.g., TopHat, STAR, HISAT and HISAT 2; Kim et al., 2019) to map RNA-seq reads to the reference genome; (2) the tools for reads extraction [e.g., HTSeq (Anders et al., 2014) and featureCount (Liao et al., 2014)]; (3) the tools for transcript construction (e.g., CuffLinks, StringTie) (Trapnell et al., 2012; Pertea et al., 2017); (4) the tools for estimates gene/transcript abundance [e.g., CuffDiff2, Ballgown and RSEM (Li and Dewey, 2011)]; and (5) the tools to identify differentially expressed genes or transcripts based on statistical analyses (such as edgeR (Robinson et al., 2010), DESeq2 (Love et al., 2014), Ballgown and CuffDiff2). The majority of the applications and computational tools summarized in the follow are compatible with these classic RNA-seq pipelines.
RNA-seq data enhance transcriptome assembly
The number of plant species with at least one reference genome have multiplied dramatically over the past few years, with 798 land plant species having genome assemblies (as of Jan. 2021) (Marks et al., 2021). While these genomic resources greatly ease the RNA-seq analysis, still the complexity in plant genomes and transcriptomes presents major challenges in RNA-seq analysis. Many plant species feature large genomes (for example, the median sizes of currently sequenced monocots and eudicots respectively are more than 500 Mb) or complex auto- or allo- polyploid genomes with some hybridization and introgressions (Zhang et al., 2018; Zhao et al., 2021; Sun et al., 2022). Many genomes are expanded by repetitive sequences (such as transposons), making it difficult to achieve complete and accurate annotation of multi-exonic genes. Besides, alternative splicing (AS) and alternative polyadenylation (APA) further enhance transcriptome complexity. In addition, gene families commonly seen in the plant genomes are shaped by whole genome duplication, segmental duplication and tandem duplication. The members within a gene family or the homo-/homoeo-logous alleles (in polyploid) usually share high sequence similarity between each other, thus posing ad-ditional challenges in accurate quantification of the expression levels by using RNA-seq data.
To overcome these challenges, two strategies have been evolved when a reference genome is available: (1) to assembly transcripts first and then to quantify expression; (2) to simultaneously construct transcripts and to quantify expression. For the genome-guided transcriptome analysis, multiple pipelines have been established that differ in the algorithms used and the speed and computational resources required, including the classic TopHat-Cufflink-Cuffdiff pipeline (Trapnell et al., 2012) and HISAT-StringTie-Ballgown pipeline (Pertea et al., 2017), as well as the new “Strawberry” tool (Liu and Dickerson, 2017). By contrast, when a reference genome and gene annotations do not exist, a transcriptome needs to be firstly de novo assembled to facilitate expression quantification. However, de novo assembly based on short-read RNA-seq data usually leads to fractured and incomplete view of transcriptome, complicating downstream analysis (Malik et al., 2018). Several tools for de novo assembling full-length transcripts have become popular with different algorithms and features, such as Trinity (Haas et al., 2013), Oasis (Schulz et al., 2012), Trans-AbySS (Robertson et al., 2010), SOAPdenovo-Trans (Xie et al., 2014), Corset (Garber et al., 2011) and BinPacker (Liu et al., 2016). More recently, Grouper provides a complete pipeline for processing de novo transcriptomic analysis by using a new method for clustering assembled contigs (Malik et al., 2018). TransFlow provides a versatile workflow to enhance de novo transcriptome analyses and to annotate transcript structures more accurately by combining short-read and long-read sequencing data (Seoane et al., 2018).
RNA-seq data empower the construction of expression atlas
Rapid accumulation of immense sets of RNA-seq data allows the establishment of expression atlantes. An expression atlas collects a large number of RNA-seq data from a certain species and re-analyzes these data using standardized, open-source pipelines to remove potential batch effects and any influences caused by other factors, such as different research groups, sequencing platforms and experiments (Papatheodorou et al., 2018). Establishing expression atlas has been proved very valuable in model organisms to promote not only omics studies but more importantly our understanding in gene functions, as clues to gene function can often be inferred by examining when and where a gene is expressed in the organism (Alberts et al., 2002). In model plants and major crops, such expression atlantes have served as key resources to the research community. For example, the information hub of Arabidopsis (TAIR; Berardini et al., 2015) and maize (MaizeGDB; Lawrence et al., 2008) have implemented with the expression atlas for each species. Maize expression atlas websites have been updated or built separately by multiple groups to integrate more RNA-seq data, other omics data sets or visualizations (Sekhon et al., 2013; Stelpflug et al., 2015; Tian et al., 2018; Hoopes et al., 2019; Gui et al., 2020). Similarly, the rice expression atlas has been updated from microarray to RNA-seq data sets and established by several groups respectively (Sato et al., 2013; Kudo et al., 2017; Xia et al., 2017). Recently, the expression atlantes have also been built for other important crops, such as tomato (TomExpress, Zouine et al., 2017), soybean (Machado et al., 2020), wheat (Borrill et al., 2016), barley (BarleyNet, Lee et al., 2020) and sorghum (Makita et al., 2015). The trend of building RNA-seq-based expression atlas has been spread to many less-studied plant species, for example, Picea abies (the Norwood database, Jokipii-Lukkari et al., 2017), Populus tremula (the Aspwood database, Sundell et al., 2017), chickpea (Kudapa et al., 2018), Physcomitrella Paten (Perroud et al., 2018; Fernandez-Pizo et al., 2020), tabacco (NaDH- Brockmoller et al., 2017), water melon (Melonet-DB - Yano et al., 2018), apple (AppleMDO- Da et al., 2019), tea (TPIA - Xia et al., 2019), grape (Wang et al., 2020), and Medicago truncatula (LeGOO- Carrere et al., 2020).
Notably, two types of the integrative websites are particularly valuable in facilitating comparative functional genomics and molecular breeding. (1) The expression atlas website includes a number of useful functions, from the visualization, comparison and functional enrichment of the omics data to comprehensive annotations of genes or gene families and useful functions such as primer design, BLAST and ortholog identification. (2) The RNA-seq data are further utilized to construct co-expression modules and integrated with other types of omics data, for example epigenomic data sets. In addition, major plant genomics websites (for instance, the Phytozome (Goodstein et al., 2011) Ensembl Plants (Bolster et al., 2017), and Gramene (Tello-Ruiz et al., 2018)) serve as the central data hub to link numerous plant genomes to those of the model species, which are well characterized and annotated. These iconic plant genomic hubs lay a solid foundation for transferring and comparing the omic information from model plants to less-studied species.
RNA-seq data capture large-scale co-expression networks
One major cornerstone of the data-driven biological interpretation of large-scale RNA-seq data is to transform expression data into networks and modules. Among the network representation methods, co-expression network is the one that has been widely applied and successful in many species (Farber and Lusis, 2008). In a co-expression network, genes are connected by edges that quantify the similarity between gene expression patterns, and the genes expressed similarly are grouped together forming a co-expression module. Co-expression network can be calculated by different approaches, from correlation-based methods like Pearson Correlation Coefficiency (PCC) (D’haeseleer et al., 2000) and weighted gene co-expression network analysis (WGCNA) (Langfelder and Horvath, 2008; Langfelder and Horvath, 2012), to linear modelling (Vasilevski et al., 2012) and mutual information methods (Daub et al., 2004). Through the “guilt-by-association” principle, genes in a co-expression module possibly indicate similar functions and modes of transcriptional regulation (Wolfe et al., 2005), or similar cellular compartments of the protein products (Ryngajllo et al., 2011).
Over the past decade, high-quality co-expression networks and their hosting data hubs have served as a valuable resource to facilitate the gene functional studies in model plant species and many major crops, including Arabidopsis (Van Bel et al., 2017; Obayashi et al., 2018), rice (Xia et al., 2017), maize (Miao et al., 2017; Tian et al., 2018; Hoopes et al., 2019), and tomato (Zouine et al., 2017). More recently, co-expression networks have been built in other plant species (Kudapa et al., 2018), including some forest species with biomass purposes (Jokipii-Lukkari et al., 2017; Sundell et al., 2017), demonstrating the power of network representation in providing molecular functional insights into biomass production. Nonetheless, the biologists who work on less-studied plant species might neither have the bioinformatic skills nor afford the computational resources that are required to integrate large-scale RNA-seq data sets and to construct high-quality networks. Thus, user-friendly online or offline tools have been developed to lower the bar for co-expression-based analysis, such as the Kallisto-based LSTrAP pipeline (Proost et al., 2017), the LSTrAP-Cloud (Tan et al., 2020) and the ExpressWeb (Savelli et al., 2019). Besides, computational methods have been reported to improve the quality of co-expression network identification (NetMiner, Yu et al., 2018; PCC-HRR Liesecke et al., 2018). These tools aim toward paving the way to perform co-expression analysis in plant species without limitations.
Leveraging these resources related to network analysis can enhance our understanding in biomass production in different plant species. On one hand, several expression atlas or co-expression resources contains a number of samples from the grass species (i.e., rice, wheat and maize) across stem elongation, thus making possible to identify co-expressed modules associated with stem growth or straw biomass accumulation (Borrill et al., 2016; Kudo et al., 2017; Hoopes et al., 2019; Obayashi et al., 2018). On the other hand, valuable web resources (the AspWood and NorWood database for Populus tremula and Picea abies, respectively) demonstrate the power for generating insights into wood formation and cell wall biosynthesis (Jokipii-Lukkari et al., 2017; Sundell et al., 2017). Moreover, AspWood exemplifies comparative analysis between the coexpression networks from two species, highlighting that conserved coexpression patterns are detected for many processes during wood formation (e.g., cambial growth, secondary cell wall deposition and xylem maturation). In addition, many of the cell wall metabolic regulators identified by coexpression analysis still maintain relatively conserved functions in biomass accumulation in other grasses, such as sorghum (Hennet et al., 2020). To facilitate such comparative analysis between model and non-model species, ATTED and Plant Regulomics have laid foundation for cross-study and cross-species comparisons and retrieving upstream regulators of certain genes of interest (Obayashi et al., 2018; Ran et al., 2020).
While the efforts made in co-expression analyses, three types of challenges remain in: (1) analysis of time-course expression data, (2) inference of gene regulatory networks (GRNs) from the co-expression data, and (3) comparison of co-expression modules between plant species.
First, clustering or co-expression analysis particularly for time-course data emphasizes on capturing the nonstationary time dependence in the data, for which multivariate clustering algorithms or nonlinear regression modelling methods usually perform better than the traditional clustering approaches (Heard et al., 2005). Thus, computational tools such as Smoothing spline clustering (SSClust) (Ma et al., 2006) or tcgsaSeq (Agniel and Hejblum, 2017) have been developed to identify gene clusters from time-course expression data.
Second, new computational approaches have also been available to predict gene regulatory cascade from large-scale RNA-seq data, e.g. the nonparametric Bayesian and Markov clustering methods (Penfold et al., 2015a; Penfold et al., 2015b; Desai et al., 2017; Yu et al., 2019). Successful examples have been shown in crops, i.e. Harrington et al. (2020) report the GRNs in wheat built with the GENIE3 software. Another group develops the tool HTRgene to specifically extract stress-responsive regulatory network, highlighting the value of GRNs in underpinning particular biological questions (Ahn et al., 2019). Another key to infer GRNs is to identify overrepresented known cis-regulatory motifs in the gene promoters that are possibly functional in the regulation of gene expression. Computational search of cis-motifs in the promoter region can be readily conducted by using online websites, such as PlantCARE (Lescot et al., 2002), PlantPAN (Chow et al., 2019), or Jaspar (Fornes et al., 2020). Recently, identification of the overrepresented cis-motifs has been achieved by the Regulatory Sequence Analysis Tools (RSAT; Nguyen et al., 2018; Ksouri et al., 2021) and its plant-adopted version RSAT-plant (Contreras-Moreira et al., 2016; Ksouri et al., 2021). Lately, resources for visualization and efficient deployment of gene regulatory omics data (ChIP-seq, for instance) have been also available at ChIP-Hub (Fu et al., 2022) and Connec-TF (Brooks et al., 2021), making possible for transferring the TF-target regulatory relationship from the model plants to non-model species.
Last, for the comparison of coexpression networks between species, successful examples have been reported in Brassicaceae (Becker et al., 2017). ATTED-II (Obayashi et al., 2018) is a database hosting 16 co-expression platforms from nine species, allowing the comparison of co-expression modules between the species. In particular, as the resources and tools to move RNA-seq analysis toward regulomics have become mature, the Plant Regulomics database has been built, hosting a huge volume of transcriptomic and epigenomic data sets for six representative species (i.e., Arabidopsis, rice, maize, soybean, tomato and wheat) and enabling the query of upstream regulators of genes (Ran et al., 2020). The Plant Regulomics database sets a nice example for future RNA-seq-centered web interface and analysis direction for other plant species.
RNA-seq data identify alternative splicing and alternative polyadenylation
While the expression atlas and co-expression analysis are based mainly on gene expression levels, RNA-seq data can also capture structural changes in the transcripts, presenting another layer of regulatory information with biological significance. Two major structural alterations are frequently detected in the transcriptome: (1) Alternative splicing (AS), a phenomenon in which particular exons of a gene may be included or excluded from the processed messenger RNA (mRNA), leading to multiple proteins encoded from a single gene; (2) Alternative polyadenylation (APA), a phenomenon in which a transcript is processed to produce multiple isoforms differing in their untranslated regions (UTRs), in most of the cases, 3’UTRs. Both AS and APA greatly increase the complexity of transcriptome or the repertoire of proteins, and are involved in the molecular, physiological and developmental pathways (Seo et al., 2013; Srivastava et al., 2018). In human, Arabidopsis and maize, respectively, ~95%, 61% and 57% of multi-exonic genes are alternatively spliced, respectively (Pan et al., 2008; Reddy et al., 2013; Wang et al., 2016). In parallel, over 80% and 75% of the genes in human and Arabidopsis respectively can produce multiple mRNA isoforms through APA (Mayr, 2016; Guo et al., 2016). The 3’UTR regions harbor cis-acting elements, which regulate various mRNA properties, including RNA stability, transportation, subcellular movement and translation efficiency (Srivastava et al., 2018).
Currently, computational methods for identifying differential AS have been achieved with different quantification schemas, such as those using count-based models (i.e., DEXSeq (Anders et al., 2012), DSGseq (Wang et al., 2013), SpliceCompass (Aschoff et al., 2013), rMATS (Shen et al., 2012), rDiff (Drewe et al., 2013) and RNAprof (Tran et al., 2016)), and those modelling isoform ratios (i.e., Cufflinks and DiffSplice) (Hu et al., 2013). Notably, some new genome assemblies of plants might not have the standard gene annotations as those of human or mouse, and not be readily compatible with some AS quantification tools or need considerable bioinformatic customizations. This issue presents somewhat a technical bar to identify and quantify AS in any plant species, even though identification of differential AS events can be done in major plant species with rMATS and CuffDiff (Liu et al., 2014). Also, new tools for identify intron retention, a particular type of AS frequently seen in plants, has been reported (Mao et al., 2017), enriching the toolbox for AS analysis.
For alternative polyadenylation, user-friendly tools compatible with the genomes of non-model plant species are relatively limited, whereas major efforts have been made to capture 3’UTRs by specific experimental protocols, such as PAT-seq (Harrison et al., 2015), 3’READs (Hoque et al., 2013), and mTAIL-seq (Lim et al., 2016). Only a handful of tools have been reported to identify 3’UTR variations and to calculate differential 3’UTRs using short-read RNA-seq data from plants. The priUTR pipeline detects differential 3’UTR events from Cufflink-derived, genome-guided transcriptome assemblies, discovering the link between 3’UTR and m6A epitranscriptomic modification (Tu and Li, 2020). APAtrap is one of the tools providing flexible and highly efficient APA detection for plant RNA-seq data (Ye et al., 2018). In addition, RNAprof detect both AS and APA events in plant RNA-seq data sets (Tran et al., 2016), while 3D RNA-seq provides three-way differential analysis: differential expression (DE), differential alternative splicing (DAS) and differential transcript usage (DTU) of RNA-seq data (Guo et al., 2021). These recent methods promise the identification of differential AS and APA events as a regular analysis of plant RNA-seq data.
Discussion and concluding remarks
Many of the short-read, bulk RNA-seq data accumulated today from less-studied plants may be under utilized. Thus, making full use of these data by integrating RNA-seq tools presents an exciting yet challenging prospect. Still, improvements can be made in the following aspects: (1) to integrate with the long-read RNA-seq data; (2) to develop tools or optimize the current pipelines to adapt to complex plant genomes.
PacBio isoform sequencing (Iso-seq) has been the main choice for identifying full-length transcripts. Besides, high-quality full-length isoform sequencing has greatly expanded our understanding in genome annotation, isoform phasing, detection of fusion transcript and alternative splicing and alternative polyadenylation (APA). For example, automated annotation pipelines have been developed to combine the advantages of different annotation methods, including ab initio and protein evidence-based prediction and long-read sequencing data (Cook et al., 2018; Tardaguila et al., 2018). However, limited by the medium throughput, Iso-seq-based transcript quantification is far from affordable, especially for the project with a tight budget or a large number of samples. Thus, combining the Iso-seq-derived transcriptome and short-read RNA-seq represents an affordable strategy to both accurately capture a large number of transcripts and to quantify them (Figure 1). On another hand, ONT technology has demonstrated its potential in detection of poly(A) tail length and RNA modifications. Therefore, combination of ONT RNA-seq technologies and short-read RNA-seq results will enable novel insights into epitranscriptomic regulation. It is worth to note that while full-length transcriptomes based on the long-read sequencing technologies are apparently advantageous over the short-read RNA-seq in identification of alternative splicing and polyadenylation, tools analyzing short-read sequencing data for these purposes (such as rMATS, rDiff, RNAProf, APAtrap and priUTR) still have their particular niches because short-read RNA-seq are still dominant in the less-studied plant species and are cost affordably for most of the labs, even in high sequencing depth.
In addition, expression quantification may be complicated by other difficulties associated with plant genomes. Polyploid, including both allopolyploid and autopolyploid, are widespread in land plants. Polyploid species are frequent in biomass crops, such as the allopolyploid Miscanthus species (Mitros et al., 2020) and autopolyploid sugarcane species (Zhang et al., 2018). High levels of sequence similarity between the homo-/homoeologous alleles or gene members pose many challenges to the alignment of short reads and subsequent expression quantification. Thus, tools for the RNA-seq analysis of polyploid species or the pipelines tuned for such expression quantification are necessary (Kuo et al., 2018; Paya-Milans et al., 2018), as polyploid species have begun to be assembled recently.
Notably, short-read RNA-seq also has major merits in other plant-related research areas, especially single-cell/single nuclear RNA-seq and meta-transcriptome analysis, owing to the compatibility and cost affordability. Short-read RNA-seq facilitates meta-transcriptome characterization, profiling gene expression in a microbial community and providing a snapshot for functional exploration (Turner et al., 2013; Salazar et al., 2019). In particular, deep RNA-seq can be used to profile the gene expression from both the host and pathogens to obtain insights into plant-microbial interactions (Rudd et al., 2015).
More recently, short-read RNA-seq has been pushed to single-cell resolution due to a series of technological advancements, including robotics, microfluidics and hydrogel droplets (Zhang et al., 2019). In a few years, efforts in single-cell RNA-seq (scRNA-seq) or single-nuclei RNA-seq (snRNA-seq) have expanded from model plants (Arabidopsis, tomato and rice) to non-model species (e.g., maize and poplar), from organ development and cell differentiation to wood formation (Gutzat et al., 2020; Xu et al., 2020; Li et al., 2021; Kajala et al., 2021; Chen et al., 2021a; Wang et al., 2021; Bezrutczyk et al., 2021; Liu et al., 2022). Undoubtedly, single-cell transcriptomics are leading the fore frontier of plant single-cell biology and playing an ever-increasing role in plant research and breeding. Excellent reviews and public database on plant scRNA-seq datasets are available (Shaw et al., 2021; Chen et al., 2021b; Shahan et al., 2021). Due to the differences in several aspects of the wet- and dry-lab parts between the single-cell and bulk RNA-seq experiments, the merits of short-read RNA-seq in single-cell plant biology is beyond the scope of this review and can be found elsewhere (Shaw et al., 2021).
In summary, our work discusses a representative collection of RNA-seq analysis tools covering gene annotation, construction of expression atlas, gene regulation and alternative splicing. We emphasize that the integration of these tools will unleash the power within RNA-seq analysis, uncover the gene regulatory complexity for many less-studied plant species, and, ultimately, promote the functional genomics of these species.
Author contributions
MT and JiZ developed the conceptual outline and drafted the manuscript. All authors contributed to the article and approved the submitted version.
Funding
This work was supported by the National Natural Science Foundation of China (31901537), the start-up funding for young talents at Wuhan Polytechnic University (No. 53210052172 to M.T.) and the Opening fund of Hubei Key Laboratory of Bioinorganic Chemistry & Materia Medica (No. BCMM202205 to M.T.).
Acknowledgments
We thank the invaluable time and efforts of reviewers in manuscript evaluation.
Conflict of interest
The authors declare that the research was conducted in the absence of any commercial or financial relationships that could be construed as a potential conflict of interest.
Publisher’s note
All claims expressed in this article are solely those of the authors and do not necessarily represent those of their affiliated organizations, or those of the publisher, the editors and the reviewers. Any product that may be evaluated in this article, or claim that may be made by its manufacturer, is not guaranteed or endorsed by the publisher.
Supplementary material
The Supplementary Material for this article can be found online at: https://www.frontiersin.org/articles/10.3389/fpls.2022.1038109/full#supplementary-material
References
Agniel, D., Hejblum, B. P. (2017). Variance component score test for time-course gene set analysis of longitudinal RNA-seq data. Biostatistics 18, 589–604. doi: 10.1093/biostatistics/kxx005
Ahn, H., Jung, I., Chae, H., Kang, D., Jung, W., Kim, S. (2019). HTRgene: a computational method to perform the integrated analysis of multiple heterogeneous time-series data: case analysis of cold and heat stress response signaling genes in arabidopsis. BMC Bioinf. 20 (Suppl16), 588. doi: 10.1186/s12859-019-3072-2
Alberts, B., Johnson, A., Lewis, J., Raff, M., Roberts, K., Walter, P.. (2002). Molecular biology of the cell. 4th edition (New York: Garland Science). Available at: https://www.ncbi.nlm.nih.gov/books/NBK26818.
Anders, S., Pyl, P. T., Huber, W. (2014). HTSeq — a Python framework to work with high-throughput sequencing data. Bioinformatics 31, 166–169. doi: 10.1093/bioinformatics/btu638
Anders, S., Reyes, A., Huber, W. (2012). Detecting differential usage of exons fromRNA-seq data. Genome Res. 22, 2008–2017. doi: 10.1101/gr.133744.111
Aschoff, M., Hotz-Wagenblatt, A., Glatting, K. H., Fischer, M., Eils, R., Kdonig, R. (2013). SplicingCompass: differential splicing detection using RNA-seq data. Bioinformatics 29, 1141–1148. doi: 10.1093/bioinformatics/btt101
Becker, M. G., Walker, P. L., Pulgar-Vidal, N. C., Belmonte, M. F. (2017). SeqEnrich: A tool to predict transcription factor networks from co-expressed arabidopsis and Brassica napus gene sets. PloS One 12, e0178256. doi: 10.1371/journal.pone.0178256
Berardini, T. Z., Reiser, L., Li, D., Mezheritsky, Y., Muller, R., Strait, E., et al. (2015). The arabidopsis information resource: Making and mining the "gold standard" annotated reference plant genome. Genesis 53, 474–485. doi: 10.1002/dvg.22877
Bezrutczyk, M., Zollnet, N. R., Kruse, C. P. S., Hartwig, T., Lautwein, T., Kohrer, K., et al. (2021). Evidence for phloem loading via the abaxial bundle sheath cells in maize leaves. Plant Cell. 33, 531–547.
Bolster, D. M., Staines, D. M., Perry, E., Kersey, P. J. (2017). Ensembl plants: Integrating tools for visualizing, mining, and analyzing plant genomic data. Methods Mol. Biol. 1533, 1–31. doi: 10.1007/978-1-4939-6658-5_1
Borrill, P., Ramirez-Gonzalez, R., Uauy, C. (2016). expVIP: a customizable RNA-seq data analysis and visualiza-tion platform. Plant Physiol. 170, 2172–2186. doi: 10.1104/pp.15.01667
Boyles, R. E., Brenton, Z. W., Kresovich, S. (2019). Genetic and genomic resources of sorghum to connect genotype with phenotype in contrasting environments. Plant J. 97, 19–39. doi: 10.1111/tpj.14113
Brockmoller, T., Ling, Z., Li, D., Gaquerel, E., Baldwin, I. T., Xu, S. (2017). Nicotiana attenuata data hub (NaDH): an integrative platform for exploring genomic, transcriptomic and metabolomic data in wild tobacco. BMC Genomics 18, 79. doi: 10.1186/s12864-016-3465-9
Brooks, M. D., Juang, C. L., Katari, M. S., Alvarez, J. M., Pasquino, A., Shih, H. J., et al. (2021). ConnecTF: A platform to integrate transcription factor–gene interactions and validate regulatory networks. Plant Physiol. 185, 49–66. doi: 10.1093/plphys/kiaa012
Carrere, S., Verdenaud, M., Gough, C., Gouzy, J., Gamas, P. (2020). LeGOO: An expertized knowledge database for the model legume Medicago truncatula. Plant Cell Physiol. 61 (1), 203–211. doi: 10.1093/pcp/pcz177
Cartolano, M., Huettel, B., Hartwig, B., Reinhardt, R., Schneeberger, K. (2016). cDNA library enrichment of full length transcripts for SMRT long read sequencing. PloS One 11, e0157779. doi: 10.1371/journal.pone.0157779
Chen, Y., Tong, S., Jiang, Y., Ai, F., Feng, Y., Zhang, J., et al. (2021a). Transcriptional landscape of highly lignified poplar stems at single-cell resolution. Genome Biol. 22, 319.
Chen, H., Yin, X., Guo, L., Yao, J., Ding, Y., Xu, X., et al. (2021b). PlantscRNAdb: A database for plant single-cell RNA analysis. Mol. Plant 14, 855–857.
Chow, C. N., Lee, T. Y., Hung, C. H., Li, G. Z., Tseng, K. C., Liu, Y. H., et al. (2019). PlantPAN3.0: a new and updated resource for reconstructing transcriptional regulatory networks from ChIP-seq experiments in plants. Nucleic Acids Res. 47, D1155–D1163. doi: 10.1093/nar/gky1081
Chu, Q., Bai, P., Zhu, X., Zhang, X., Mao, L., Zhu, Q., et al. (2018). Characteristics of plant circular RNAs. Brief Bioinform. 21, 135–143. doi: 10.1093/bib/bby111
Conesa, A., Madrigal, P., Tarazona, S., Gomez-Cabrero, D., Cervera, A., McPherson, A., et al. (2016). A survey of best practices for RNA-seq data analysis. Genome Biol. 17, 13. doi: 10.1186/s13059-016-0881-8
Contreras-Moreira, B., Castro-Mondragon, J. A., Rioualen, C., Cantalapiedra, C. P., van Helden, J. (2016). RSAT::Plants: Motif discovery within clusters of upstream sequences in plant genomes. Methods Mol. Biol. 1482, 279–295. doi: 10.1007/978-1-4939-6396-6_18
Cook, D., Valle-Inclan, J. E., Pajoro, A., Rovenich, H., Thomma, B., Faino, L. (2018). Long read annotation (LoReAn): automated eukaryotic genome annotation based on long-read cDNA sequencing. Plant Physiol. 179, 38–54. doi: 10.1104/pp.18.00848
Da, L., Liu, Y., Yang, J., Tian, T., She, J., Ma, X., et al. (2019). AppleMDO: A multi-dimensional omics database for apple co-expression networks and chromatin states. Front. Plant Sci. 10. doi: 10.3389/fpls.2019.01333
Daub, C. O., Steuer, R., Selbig, J., Kloska, S. (2004). Estimating mutual information using b-spline functions–an improved similarity measure for analysing gene expression data. BMC Bioinf. 5, 118. doi: 10.1186/1471-2105-5-118
Desai, J. S., Sarto, R. C., Lawas, L. M., Jagadish, S. V. K., Doherty, C. J. (2017). Improving gene regulatory network inference by incorporating rates of transcriptional changes. Sci. Rep. 7, 17244. doi: 10.1038/s41598-017-17143-1
D’haeseleer, P., Liang, S., Somogyi, R. (2000). Genetic network inference: from co-expression clustering to reverse engineering. Bioinformatics 16, 707–726. doi: 10.1093/bioinformatics/16.8.707
Drewe, P., Stegle, O., Hartmann, L., Kahles, A., Bohnert, R., Wachter, A., et al. (2013). Accurate detection of differential RNA processing. Nucleic Acids Res. 41, 5189–5198. doi: 10.1093/nar/gkt211
Farber, C. R., Lusis, A. J. (2008). Integrating global gene expression analysis and genetics. Adv. Genet. 60, 571–601. doi: 10.1016/S0065-2660(07)00420-8
Fei, Y., Wang, R., Li, H., Liu, S., Zhang, H., Huang, J. (2018) DPMIND: Degradome-based plant MiRNA-target interaction and network database. Bioinformatics 34, 1618–1620. doi: 10.1093/bioinformatics/btx824
Feng, J., Huang, S., Guo, Y., Liu, D., Song, J., Gao, J., et al. (2019). Plant ISOform sequencing database (PISO): a comprehensive repertory of full-length transcripts in plants. Plant Biotechnol. J. 17, 1001–1003. doi: 10.1111/pbi.13076
Fernandez-Pizo, N., Hass, F. B., Meyberg, R., Ullrich, K. K., Hiss, M., Perroud, P., et al. (2020). PEATmoss (Physcomitrella expression atlas tool): a unified gene expression atlas for the model plant physcomitrella patens. Plant J. 102, 165–177. doi: 10.1111/tpj.14607
Foox, J., Tighe, S. W., Nicolet, C. M., Zook, M., Byrska-Bishop, M., Clarke, W. E., et al. (2021). Performance assessment of DNA sequencing platforms in the ABRF next-generation sequencing study. Nat. Biotechnol. 39, 1129–1140.
Fornes, O., Castro-Mondragon, J. A., Khan, A., van der Lee, R., Zhang, X., Richmond, P. A., et al. (2020). JASPAR 2020: update of the open-access database of transcription factor binding profiles. Nucleic Acids Res. 48, D87–D92. doi: 10.1093/nar/gkz1001
Freese, N. H., Norris, D. C., Loraine, A. E. (2016). Integrated genome browser: visual analytics platform for genomics. Bioinformatics 32, 2089–2095. doi: 10.1093/bioinformatics/btw069
Fu, L., Zhu, T., Zhou, X., Yu, R., He, Z., Zhang, P., et al. (2022). ChIP-hub provides an integrative platform for exploring plant regulome. Nat. Commun. 13, 3413. doi: 10.1038/s41467-022-30770-1
Galli, M., Feng, F., Gallavotti, A. (2020). Mapping regulatory determinants in plants. Front. Genet. 11, 591194.
Garber, M., Grabherr, M. G., Guttman, M., Trapnell, C. (2011). Computational methods for transcriptome annotation and quantification using RNA-seq. Nat. Methods 8, 469–477. doi: 10.1038/nmeth.1613
Gaudinier, A., Brady, S. M. (2016). Mapping transcriptional networks in plants: Data-driven discovery of novel biological mechanisms. Annu. Rev. Plant Biol. 67, 575–594.
Ge, S. X., Son, E. W., Yao, R. (2018). iDEP: an integrated web application for differential expression and pathway analysis of RNA-seq data. BMC Bioinform. 19, 534. doi: 10.1186/s12859-018-2486-6
Goodstein, D. M., Shu, S., Howson, R., Neupane, R., Hayes, R. D., Fazo, J., et al. (2011). Phytozome: a comparative platform for green plant genomics. Nucleic Acids Res. 40, D1178–D1186. doi: 10.1093/nar/gkr944
Grabherr, M. G., Haas, B. J., Yassour, M., Levin, J. Z., Thompson, D. A., Amit, I., et al. (2012). Full-length transcriptome assembly from RNA-seq data without a reference genome. Nat. Biotechnol. 29, 644–652. doi: 10.1038/nbt.1883
Gui, S., Yang, L., Li, J., Luo, J., Xu, X., Yuan, J., et al. (2020). ZEAMAP, a comprehensive database adapted to the maize multi-omics era. iScience 23, 101241. doi: 10.1016/j.isci.2020.101241
Guo, C., Spinelli, M., Liu, M., Li, Q., Liang, C. (2016). A genome-wide study of “non-3UTR” polyadenylation sites in Arabidopsis thaliana. Sci. Rep. 6, 28060. doi: 10.1038/srep28060
Guo, W., Tzioutziou, N. A., Stephen, G., Milne, I., Cailxtom, C. P., Waugh, R., et al. (2021). 3D RNA-seq: a powerful and flexible tool for rapid and accurate differential expression and alternative splicing analysis of RNA-seq data for biologists. RNA Biol. 18, 1574–1587. doi: 10.1080/15476286.2020.1858253
Gupta, C., Pereira, A. (2019). Recent advances in gene function prediction using context-specific coexpression networks in plants. F1000Research 2019, 8.
Gutzat, R., Rembart, K., Nussbaumer, T., Hofmann, F., Pisuparti, R., Bradamante, G., et al. (2020). Arabidopsis shoot stem cells display dynamic transcription and DNA methylation patterns. EMBO J. 39, e103667.
Haas, B. J., Papanicolaou, A., Yassour, M., Grabherr, M., Blood, P. D., Bowden, J., et al. (2013). De novo transcript sequence reconstruction from rna-seq: reference generation and analysis with trinity. Nat. Protoc. 8, 1494–1512. doi: 10.1038/nprot.2013.084
Haque, S., Ahmad, J. S., Clark, N. M., Williams, C. M., Sozzani, R. (2018). Computational prediction of gene regulatory networks in plant growth and development. Curr. Opin. Plant Biol. 47, 96–105.
Harrington, S. A., Backhaus, A. E., Singh, A., Hassani-Pak, K., Uauy, C. (2020). The wheat GENIE3 network provides biologically-relevant information in polyploid wheat. G3 10, 3675. doi: 10.1534/g3.120.401436
Harrison, P. F., Powell, D. R., Clancy, J. L., Presis, T., Boag, P. R., Traven, A., et al. (2015). PAT-seq: a method to study the integration of 3’-UTR dynamics with gene expression in the eukaryotic transcriptome. RNA 21, 1502–1510. doi: 10.1261/rna.048355.114
Heard, N. A., Holmes, C. C., Stephens, D. A. (2005). A quantitative study of gene regulation involved in the immune response of Anopheline mosquitoes. J. Am. Stat. Assoc. 101, 18–29. doi: 10.1198/016214505000000187
Hennet, L., Berer, A., Trabanco, N., Ricciuti, E., Dufayard, J. F., Bocs, S., et al. (2020). Transcriptional regulation of sorghum stem composition: Key players identified through Co-expression gene network and comparative genomics analyses. Front. Plant Sci. 11, 224.
Hoopes, G. M., Hamilton, J. P., Wood, J. C., Esteban, E., Pasha, A., Vaillancourt, B., et al. (2019). An updated gene atlas for maize reveals organ-specific and stress-induced genes. Plant J. 97, 1154–1167. doi: 10.1111/tpj.14184
Hoque, M., Ji, Z., Zheng, D., Luo, W., Li, W., You, B., et al. (2013). Analysis of alternative cleavage and polyadenylation by 3’ region extraction and deep sequencing. Nat. Methods 10, 133–139. doi: 10.1038/nmeth.2288
Hu, Y., Huang, Y., Du, Y., Orellana, C. F., Singh, D., Johnson, A. R., et al. (2013). DiffSplice: the genome-wide detection of differential splicing events with RNA-seq. Nucleic Acids Res. 41, 39. doi: 10.1093/nar/gks1026
Jin, Y., Tam, O. H., Paniagua, E., Hammell, M. (2015). TEtranscripts: a package for including transposable elements in differential expression analysis of RNA-seq datasets. Bioinformatics 31, 3593–3599. doi: 10.1093/bioinformatics/btv422
Jokipii-Lukkari, S., Sundell, D., Nilsson, O., Hvidsten, T. R., Street, N. R., Tuominen, H. (2017). NorWood: a gene expression resource for evo-devo studies of conifer wood development. New Phyto. 216, 482–494. doi: 10.1111/nph.14458
Kajala, K., Gouran, M., Shaar-Moshe, L., Mason, G. A., Rodriguez-Medina, J., Kawa, D., et al. (2021). Innovation, conservation, and repurposing of gene function in root cell type development. Cell. 184, 3333–3348.
Kim, D., Paggi, J. M., Park, C., Park, C., Salzberg, S. L. (2019). Graph-based genome alignment and genotyping with HISAT2 and HISAT-genotype. Nat. Biotechnol. 37, 907–915. doi: 10.1038/s41587-019-0201-4
Ksouri, N., Castro-Mondragon, J. A., Montardit-Tarda, F., van Helden, J., Contreras-Moreira, B., Gogorcena, Y. (2021). Tuning promoter boundaries improves regulatory motif discovery in nonmodel plants: the peach example. Plant Physiol. 185, 1242–1258. doi: 10.1093/plphys/kiaa091
Kudapa, H., Garg, V., Chitikineni, A., Varshney, R. K. (2018). The RNA-seq-based high resolution gene expression atlas of chickpea (Cicer arietinum l.) reveals dynamic spatio-temporal changes associated with growth and development. Plant Cell Environ. 41, 2209–2225. doi: 10.1111/pce.13210
Kudo, T., Terashima, S., Takaki, Y., Nakamura, Y., Kobayashi, M., Yano, K. (2017). Practical utilization of OryzaExpress and plant omics data center databases to explore gene expression networks in oryza sativa and other plant species. Methods Mol. Biol. 1533, 229–240. doi: 10.1007/978-1-4939-6658-5_13
Kuo, T. C. Y., Hatakeyama, M., Tameshige, T., Shimizu, K. K., Sese, J. (2018). Homeolog expression quantification methods for allopolyploids. Brief. Bioinfo. 21, 395–407. doi: 10.1093/bib/bby121
Langfelder, P., Horvath, S. (2008). WGCNA: an r package for weighted correlation network analysis. BMC Bioinf. 9, 559. doi: 10.1186/1471-2105-9-559
Langfelder, P., Horvath, S. (2012). Fast r functions for robust correlations and hierarchical clustering. J. Stat. Software 46, 11.
Lawrence, C. J., Harper, L. C., Schaeffer, M. L., Sen, T. Z., Seigfried, T.E., Campbell, D.A.. (2008). MaizeGDB: the maize model organism database for basic, translational, and applied research. Intl. J. Plant Genomics 2008, 496957. doi: 10.1155/2008/496957
Lee, J., Heath, L. S., Grene, R., Li, S. (2019). Comparing time series transcriptome data between plants using a network module finding algorithm. Plant Methods 15, 61. doi: 10.1186/s13007-019-0440-x
Lee, S., Lee, T., Yang, S., Lee, I. (2020). BarleyNet: A network-based functional omics analysis server for cultivated barley, Hordeum vulgare l. Front. Plant Sci. 11. doi: 10.3389/fpls.2020.00098
Lescot, M., D’hais, P., Thijs, G., Marchal, K., Moreau, Y., Van de Peer, Y., et al. (2002). PlantCARE, a database of plant cis-acting regulatory elements and a portal to tools for in silico analysis of promoter sequences. Nucleic Acids Res. 30, 325–327. doi: 10.1093/nar/30.1.325
Liao, Y., Smyth, G. K., Shi, W. (2014). featureCounts: an efficient general purpose program for assigning sequence reads to genomic features. Bioinformatics 30, 923–930. doi: 10.1093/bioinformatics/btt656
Li, H., Dai, X., Huang, X., Xu, M., Wang, Q., Yan, X., et al. (2021). Single-cell RNA sequencing reveals a high-resolution cell atlas of xylem in populus. J. Inte. Plant Biol. 63, 1906–1921.
Li, B., Dewey, C. N. (2011). RSEM: Accurate transcript quantification from RNA-seq data with or without a reference genome. BMC Bioinf. 12, 323. doi: 10.1186/1471-2105-12-323
Liesecke, F., Daudu, D., Duge de Bernonville, R., Besseau, S., Clastre, M., Courdavault, V., et al. (2018). Ranking genome-wide correlation measurements improves microarray and RNA-seq based global and targeted co-expression networks. Sci. Rep. 8, 10885. doi: 10.1038/s41598-018-29077-3
Lim, J., Lee, M., Son, A., Chang, H., Kim, V. N. (2016). mTAIL-seq reveals dynamic poly(A) tail regulation in oocyte-to-embryo development. Genes Dev. 30, 1671–1682. doi: 10.1101/gad.284802.116
Liu, R., Dickerson, J. (2017). Strawberry: Fast and accurate genomeguided transcript reconstruction and quantification from RNA-seq. PloS Comput. Biol. 13, e1005851. doi: 10.1371/journal.pcbi.1005851
Liu, J., Li, G., Chang, Z., Yu, T., Liu, B., McMullen, R., et al. (2016). BinPacker: Packing-based de novo transcriptome assembly from RNA-seq data. PloS Comput. Biol. 12, e1004772. doi: 10.1371/journal.pcbi.1004772
Liu, G., Li, J., Li, J., Chen, Z., Yuan, P., Chen, R., et al. (2022). Single-cell transcriptome reveals the redifferentiation trajectories of the early stage of de novo shoot regeneration in arabidopsis thaliana. bioRxiv. doi: 10.1101/2022.01.01.474510
Liu, R., Loraine, A. E., Dickerson, J. A. (2014). Comparisons of computational methods for differential alternative splicing detection using RNA-seq in plant systems. BMC Bioinform. 15, 364.
Love, M. I., Huber, W., Anders, S. (2014). Moderated estimation of fold change and dispersion for RNA- seq data with DESeq2. Genome Biol. 15, 550. doi: 10.1186/s13059-014-0550-8
Ma, P., Castillo-Davis, C. I., Zhong, W., Liu, J. S. (2006). A data-driven clustering method for time course gene expression data. Nucleic Acids Res. 34, 1261–1269. doi: 10.1093/nar/gkl013
Machado, F. B., Moharana, K. C., Almeida-Silva, F., Gazara, R. K., Pedrosa-Silva, F., Coelho, F. S., et al. (2020). Systematic analysis of 1,298 RNA-seq samples and construction of a comprehensive soybean (Glycine max) expression atlas. Plant J. 103, 1894–1909. doi: 10.1101/2019.12.23.886853
Makita, Y., Shimada, S., Kawashima, M., Kondou-Kuriyama, T., Toyoda, T., Matsui, M. (2015). MOROKOSHI: transcriptome database in Sorghum bicolor. Plant Cell Physiol. 56, e6. doi: 10.1093/pcp/pcu187
Malik, L., Almodaresi, F., Patro, R. (2018). Grouper: graph-based clustering and annotation for improved de novo transcriptome analysis. Bioinformatics 34, 3265–3272. doi: 10.1093/bioinformatics/bty378
Mao, R., Liang, C., Zhang, Y., Hao, X., Li, J. (2017). 50/50 expressional odds of retention signifies the distinction between retained introns and constitutively spliced introns in arabidopsis thaliana. Front. Plant Sci. 8, 1728. doi: 10.3389/fpls.2017.01728
Marks, R. A., Hotaling, S., Frandsen, P. B., VanBuren, R. (2021). Representation and participation across 20 years of plant genome sequencing. Nat. Plants 7, 1571–1578. doi: 10.1038/s41477-021-01031-8
Mayr, C. (2016). Evolution and biological roles of alternative 3’UTRs. Trends Cell Biol. 26, 227–237. doi: 10.1016/j.tcb.2015.10.012
Miao, Z., Han, Z., Zhang, T., Chen, S., Ma, C. (2017). A systems approach to a spatiotemporal understanding of the drought stress response in maize. Sci. Rep. 7, 6590. doi: 10.1038/s41598-017-06929-y
Mitros, T., Session, A. M., James, B. T., Wu, G., Belaffif, M. B., Clark, L. V., et al. (2020). Genome biology of the paleotetraploid perennial biomass crop miscanthus. Nat. Commun. 11, 5442. doi: 10.1038/s41467-020-18923-6
Mullet, J., Morishige, D., McCormick, R., Truong, S., Hilley, J., McKinley, B., et al. (2014). Energy sorghum- a genetic model for the design of C4 grass bioenergy crops. J. Exp. Bot. 65, 3479–3489. doi: 10.1093/jxb/eru229
Nagalakshmi, U., Wang, Z., Waern, K., Shou, C., Raha, D., GerstEIN, M., et al. (2008). The transcriptional landscape of the yeast genome defined by RNA sequencing. Science 320, 1344–1350. doi: 10.1126/science.1158441
Naithani, S., Gupta, P., Preece, J., D’Eustachio, P., Elser, J. L., Garg, P., et al. (2020). Plant reactome: a knowledgebase and resource for comparative pathway analysis. Nucleic Acids Res. 48, D1093–D1103. doi: 10.1093/nar/gkz996
Naithani, S., Preece, J., D’Eustachio, P., Gupta, P., Amarasinghe, V., Dharmawardhana, P. D., et al. (2017). Plant reactome: a resource for plant pathways and comparative analysis. Nucl. Acid Res. 45, D1029–D1039. doi: 10.1093/nar/gkw932
Nguyen, N. T. T., Contreras-Moreira, B., Castro-Mondragon, J. A., Santana-Garcia, W., Ossio, R., Robles-Espinoza, C. D., et al. (2018). RSAT 2018: regulatory sequence analysis tools 20th anniversary. Nucleic Acids Res. 46, W209–W216. doi: 10.1093/nar/gky317
Obayashi, T., Aoki, Y., Tadaka, S., Kagaya, Y., Kinoshita, K. (2018). ATTED-II in 2018: A plant coexpression database based on investigation of the statistical property of the mutual rank index. Plant Cell Physiol. 59, e3(1–e37). doi: 10.1093/pcp/pcx191
Oikonomopoulos, S., Wang, Y. C., Djambazian, H., Badescu, D., Ragoussis, J. (2016). Benchmarking of the Oxford nanopore MinION sequencing for quantitative and qualitative assessment of cDNA populations. Sci. Rep. 6, 31602. doi: 10.1038/srep31602
O’Malley, R. C., Huang, S. C., Song, L., Lewsey, M. G., Bartlett, A., Nery, J. R., et al. (2016). Cistrome and epicistrome features shape the regulatory DNA landscape. Cell. 165, 1280–1292.
Pan, Q., Shai, O., Lee, L. J., Frey, B. J., Blencowe, B. J. (2008). Deep surveying of alternative splicing complexity in the human transcriptome by high-throughput sequencing. Nat. Genet. 40, 1413–1415. doi: 10.1038/ng.259
Papatheodorou, I., Fonseca, N. A., Keays, M., Tang, Y. A., Barrera, E., Bazant, W., et al. (2018). Expression atlas: gene and protein expression across multiple studies and organisms. Nucleic Acids Res. 46, D246–D251. doi: 10.1093/nar/gkx1158
Patterson, J., Carpenter, E. J., Zhu, Z., An, D., Liang, X., Geng, C., et al. (2019). Impact of sequencing depth and technology on de novo RNA-seq assembly. BMC Genomics 20, 604. doi: 10.1186/s12864-019-5965-x
Paya-Milans, M., Olmstead, J. W., Nunez, G., Rinehart, T. A., Staton, M. (2018). Comprehensive evaluation of RNA-seq analysis pipelines in diploid and polyploid species. GigaScience 7, giy132. doi: 10.1093/gigascience/giy132
Penfold, C. A., Millar, J. B., Wild, D. L. (2015b). Inferring orthologous gene regulatory networks using interspecies data fusion. Bioinformatics 31, i97–i105. doi: 10.1093/bioinformatics/btv267
Penfold, C. A., Shifaz, A., Brown, P. E., Nicholson, A., Wild, D. L. (2015a). CSI: a nonparametric Bayesian approach to network inference from multiple perturbed time series gene expression data. Stat. Appl. Genet. Mol. Biol. 14, 307–310. doi: 10.1515/sagmb-2014-0082
Perroud, P., Haas, F. B., Hiss, M., Ullrich, K. K., Alboresi, A., Amirebrahimi, M., et al. (2018). The physcomitrella patens gene atlas project: large-scale RNA-seq based expression data. Plant J. 95, 168–182. doi: 10.1111/tpj.13940
Pertea, M., Kim, D., Pertea, G. M., Leek, J. T., Salzberg, S. I. (2017). Transcript-level expression analysis of RNA-seq experiments with HISAT, StringTie and ballgown. Nat. Protoc. 11, 1650–1667. doi: 10.1038/nprot.2016.095
Proost, S., Krawczyk, A., Mutwil, M. (2017). LSTrAP: efficiently combining RNA sequencing data into co-expression networks. BMC Bioinfo. 18, 444. doi: 10.1186/s12859-017-1861-z
Proost, S., Mutwil, M. (2016). Tools of the trade: studying molecular networks in plants. Cur Opn Plant Sci. 30, 143–150.
Ran, X., Zhao, F., Wang, Y., Liu, J., Zhang, Y., Ye, L., et al. (2020). Plant regulomics: a data-driven interface for retrieving upstream regulators from plant multi-omics data. Plant J. 101, 237–248. doi: 10.1111/tpj.14526
Reddy, A. S., Marquez, Y., Kalyna, M., Barta, A. (2013). Complexity of the alternative splicing landscape in plants. Plant Cell 25, 3657–3683. doi: 10.1105/tpc.113.117523
Robertson, G., Schein, J., Chiu, R., Corbett, R., Field, M., Jackman, S. D., et al. (2010). De novo assembly and analysis of RNA-seq data. Nat. Methods 7, 909–912. doi: 10.1038/nmeth.1517
Robinson, M. D., McCarthy, D. J., Smyth, G. (2010). edgeR: a bioconductor package for differential expression analysis of digital gene expression data. Bioinformatics 26, 139–140. doi: 10.1093/bioinformatics/btp616
Rudd, J. J., Kanyuka, K., Hassani-Pak, K., Derbyshire, M., Andongabo, A., Devonshire, J. (2015). Transcriptome and metabolite profiling of the infection cycle of zymoseptoria tritici on wheat reveals a biphasic interaction with plant immunity involving differential pathogen chromosomal contributions and a variation on the hemibiotrophic lifestyle definition. Plant Physiol. 167, 1158–1185.
Ryngajllo, M., Childs, L., Lohse, M., Giorgi, F. M., Lude, A., Selbig, J., et al. (2011). Slocx: predicting subcellular localization of arabidopsis proteins leveraging gene expression data. Front. Plant Sci. 2. doi: 10.3389/fpls.2011.00043
Saelens, W., Cannoodt, R., Saeys, Y. (2018). A comprehensive evaluation of module detection methods for gene expression data. Nat. Commun. 9, 1090. doi: 10.1038/s41467-018-03424-4
Sahraeian, S. M., Mohiyuddin, M., Sbra, R., Tilgner, H., Afshar, P. T., Au, K. F., et al. (2017). Gaining comprehensive biological insight into the transcriptome by performing a broad-spectrum RNA-seq analysis. Nat. Commun. 8, 59. doi: 10.1038/s41467-017-00050-4
Salazar, G., Paoli, L., Alberti, A., Huerta-Cepas, J., Ruscheweyh, H. J., Cuenca, M., et al. (2019). Gene expression changes and community turnover differentially shape the global ocean metatranscriptome. Cell. 179, 1068–1083.
Sato, Y., Takehisa, H., Kamatsuki, K., Minami, H., Namiki, N., Ikawa, H., et al. (2013). RiceXPro version 3.0: Expanding the informatics resource for rice transcriptome. Nucleic Acids Res. 41, D1206–D1213. doi: 10.1093/nar/gks1125
Savelli, B., Picard, S., Roux, C., Dunand, C. (2019). ExpressWeb: A web application for clustering and visualization of expression data. bioRxiv. doi: 10.1101/625939
Schulz, M. H., Zerbino, D. R., Vingron, M., Birney, E. (2012). Oases: robust de novo RNA-seq assembly across the dynamic range of expression levels. Bioinformatics 28, 1086–1092. doi: 10.1093/bioinformatics/bts094
Schwacke, R., Ponce-Soto, G. Y., Krause, K., Bolger, A. M., Arsova, B., Hallab, A., et al. (2019). MapMan4: A refined protein classification and annotation framework applicable to multi-omics data analysis. Mol. Plant 12, 879–892. doi: 10.1016/j.molp.2019.01.003
Sekhon, R. S., Briskine, R., Hirsch, C. N., Myers, C. L., Springer, N. M., Buell, C. R., et al. (2013). Maize gene atlas developed by RNA sequencing and comparative evaluation of transcriptomes based on RNA sequencing and microarrays. PloS One 8, e61005. doi: 10.1371/journal.pone.0061005
Seoane, P., Espigares, M., Carmona, R., Polonio, A., Quintana, J., Cretazzo, E., et al. (2018). TransFlow: a modular framework for assembling and assessing accurate de novo transcriptomes in non-model organisms. BMC Genomics 19 (Suppl 14), 416. doi: 10.1186/s12859-018-2384-y
Seo, P. J., Park, M. J., Park, C. M. (2013). Alternative splicing of transcription factors in plant responses to low temperature stress: mechanisms and functions. Planta 237, 1415–1424. doi: 10.1007/s00425-013-1882-4
Shahan, R., Nolan, T. M., Benfey, P. N. (2021). Single-cell analysis of cell identity in the arabidopsis root apical meristem: insights and opportunities. J. Exp. Botany. 72, 6679–6686.
Sharon, D., Tilgner, H., Grubert, F., Snyder, M. (2013). A single- molecule long- read survey of the human transcriptome. Nat. Biotechnol. 31, 1009–1014. doi: 10.1038/nbt.2705
Shaw, R., Tian, X., Xu, J. (2021). Single-cell transcriptome analysis in plants: Advances and challenges. Mol. Plant 14, 115–126.
Shen, S., Park, J. W., Huang, J., Dittmar, K. A., Lu, Z. X., Zhou, Q., et al. (2012). MATS: a Bayesian framework for flexible detection of differential alternative splicing fromRNA-seq data. Nucleic Acids Res. 40, 61. doi: 10.1093/nar/gkr1291
Srivastava, A. K., Lu, Y., Zinta, G., Lang, Z., Zhu, J. K. (2018). UTR-dependent control of gene expression in plants. Trends Plant Sci. 23, 248–259. doi: 10.1016/j.tplants.2017.11.003
Song, L., Shankar, D. S., Florea, L. (2016). Rascaf: Improving genome assembly with RNA sequencing data. Plant Genome 9, 1–12. doi: 10.3835/plantgenome2016.03.0027
Stark, R., Grzelak, M., Hadfield, J. (2019). RNA Sequencing: the teenage years. Nat. Rev. Genet. 20, 631–656. doi: 10.1038/s41576-019-0150-2
Stelpflug, S. C., Sekhon, R. S., Vaillancourt, B., Hirsch, C. N., Buell, C. R., de Leon, N., et al. (2015). An expanded maize gene expression atlas based on RNA sequencing and its use to explore root development. Plant Genome 9, 1–16. doi: 10.3835/plantgenome2015.04.0025
Sullivan, A., Purohit, P. K., Freese, N. H., Pasha, A., Ewaese, J., et al. (2019). An ‘eFP-seq browser’ for visualizing and exploring RNA sequencing data. Plant J. 100, 641–654. doi: 10.1111/tpj.14468
Sun, Y., Shang, L., Zhu, Q., Fan, L. (2022). Twenty years of plant genome sequencing: achievements and challenges. Trends Plant Sci. 27, 391–401. doi: 10.1016/j.tplants.2021.10.006
Sundell, D., Street, N. R., Kumar, M., Mellerowicz, E. J., Kucukoglu, M., Johnsson, C., et al. (2017). AspWood: high-spatial-resolution transcriptome profiles reveal uncharacterized modularity of wood formation in Populus tremula. Plant Cell. 29, 1585–1604. doi: 10.1105/tpc.17.00153
Tan, Q., Goh, W., Mutwil, M. (2020). LSTrAP-cloud: A user-friendly cloud computing pipeline to infer coexpression networks. Genes 11, 428. doi: 10.3390/genes11040428
Tardaguila, M., de la Fuente, L., Marti, C., Pereira, C., Pardo-Palacios, F. J., Del Risco, H., et al. (2018). SQANTI: extensive characterization of long-read transcript sequences for quality control in full-length transcriptome identification and quantification. Genome Res. 28, 396–411. doi: 10.1101/gr.222976.117
Tello-Ruiz, M. K., Naithani, S., Stein, J. C., Gupta, P., Campbell, M., Olson, A., et al. (2018). Gramene 2018: unifying comparative genomics and pathway resources for plant research. Nucleic Acids Res. 46, D1181–D1189. doi: 10.1093/nar/gkx1111
Tian, T., You, Q., Yan, H., Xu, W., Su, Z. (2018). MCENet: A database for maize conditional co-expression network and network characterization collaborated with multi-dimensional omics levels. J. Genet. Genomics 45, 351–360. doi: 10.1016/j.jgg.2018.05.007
Tran, V. D., Souiai, O., Romero-Barrios, N., Crespi, M., Gautheret, D. (2016). Detection of generic differential RNA processing events from RNA-seq data. RNA Biol. 13, 59–67. doi: 10.1080/15476286.2015.1118604
Trapnell, C., Roberts, A., Goff, L., Pertea, G., Kim, D., Kelley, D. R., et al. (2012). Differential gene and transcript expression analysis of RNA-seq experiments with TopHat and cufflinks. Nat. Protoc. 7, 562–578. doi: 10.1038/nprot.2012.016
Tu, M., Li, Y. (2020). Profiling alternative 3’untranslated regions in sorghum using RNA-seq data. Front. Genet. 11. doi: 10.3389/fgene.2020.556749
Turner, T. R., Ramakrishnan, K., Walshaw, J., Heavens, D., Alston, M., Swarbreck, D., et al. (2013). Comparative metatranscriptomics reveals kingdom level changes in the rhizosphere microbiome of plants. ISME J. 7, 2248–2258.
Van Bel, M., Coppens, F. (2017). Exploring plant co-expression and gene-gene interactions with CORNET 3.0. Methods Mol. Biol. 1533, 201–212. doi: 10.1007/978-1-4939-6658-5_11
Van Verk, M. C., Hickman, R., Pieterse, C. M., Van Wees, S. C. (2013). RNA-Seq: revelation of the messengers. Trend Plant Sci. 18, 175–179. doi: 10.1016/j.tplants.2013.02.001
Vasilevski, A., Giorgi, F. M., Bertinetti, L., Usadel, B. (2012). LASSO modeling of the arabidopsis thaliana seed/seedling transcriptome: a model case for detection of novel mucilage and pectin metabolism genes. Mol. Biosyst. 8, 2566–2574. doi: 10.1039/C2MB25096A
Waese, J., Provart, N. J. (2016). The bio-analytic resource: Data visualization and analytic tools formultiple levels of plant biology. Curr. Plant Biol. 7-8, 2–5. doi: 10.1016/j.cpb.2016.12.001
Wang, Y., Huan, Q., Li, K., Qian, W. (2021). Single-cell transcriptome atlas of the leaf and root of rice seedlings. J. Genet. Genomics 48, 881–898.
Wang, B., Kumar, V., Olson, A., Ware, D. (2019). Reviving the transcriptome studies: An insight into the emergence of single-molecule transcriptome sequencing. Front. Genet. 10. doi: 10.3389/fgene.2019.00384
Wang, W., Qin, Z., Feng, Z., Wang, X., Zhang, X. (2013). Identifying differentially spliced genes from two groups of RNA-seq samples. Gene 518, 164–170. doi: 10.1016/j.gene.2012.11.045
Wang, B., Tseng, E., Regulski, M., Clark, T. A., Hon, T., Jiao, Y., et al. (2016). Unveiling the complexity of the maize transcriptome by single-molecule long-read sequencing. Nat. Commun. 7, 11708. doi: 10.1038/ncomms11708
Wang, Y., Zhang, R., Liang, Z., Li, S. (2020). Grape-RNA: A database for the collection, evaluation, treatment, and data sharing of grape RNA-seq datasets. Genes 11, 315. doi: 10.3390/genes11030315
Werner, T. (2003). Promoters can contribute to the elucidation of protein function. Trends Biotechnol. 21, 9–13. doi: 10.1016/s0167-7799(02)00003-3
Werner, T. (2004). Proteomics and regulomics: the yin and yang of functional genomics. Mass Spectrom. Rev. 23, 25–33. doi: 10.1002/mas.10067
Wolfe, C. J., Kohane, I. S., Butte, A. J. (2005). Systematic survey reveals general applicability of ‘guilt-by-association’ within gene coexpression networks. BMC Bioinf. 6, 227. doi: 10.1186/1471-2105-6-227
Xia, E., Li, F., Tong, W., Li, P., Wu, Q., Zhao, H., et al. (2019). Tea plant information archive: a comprehensive genomics and bioinformatics platform for tea plant. Plant Biotechnol. J. 17, 1938–1953. doi: 10.1111/pbi.13111
Xia, L., Zou, D., Sang, J., Xu, X., Yin, H., Li, M., et al. (2017). Rice expression database (RED): An integrated RNA-seq-derived gene expression database for rice. J. Genet. Genomics 44, 235–241. doi: 10.1016/j.jgg.2017.05.003
Xie, Y., Wu, G., Tang, J., Luo, R., Patterson, J., Liu, S., et al. (2014). SOAPdenovo- trans: De novo transcriptome assembly with short RNA-seq reads. Bioinformatics 30, 1660–1666. doi: 10.1093/bioinformatics/btu077
Xu, X., Crow, M., Rice, B. R., Li, F., Harris, B., Liu, L., et al. (2020). Single-cell RNA sequencing of developing maize ears facilitates functional analysis and trait candidate gene discovery. Dev. Cell. 56, 1–12.
Yano, R., Nonaka, S., Ezura, H. (2018). Melonet-DB, a grand RNA-seq gene expression atlas in melon (Cucumis melo l.). Plant Cell Physiol. 59, e4(1–e15). doi: 10.1093/pcp/pcx193
Ye, C., Long, Y., Ji, G., Li, Q. S., Wu, X. (2018). APAtrap: identification and quantification of alternative polyadenylation sites from RNA-seq data. Bioinformatics 34, 1841–1849.
Yu, H., Jiao, B., Lu, L., Wang, P., Chen, S., Liang, C., et al. (2018). NetMiner-an ensemble pipeline for building genome-wide and high-quality gene coexpression network using massive-scale RNAseq samples. PloS One 13, e0192613. doi: 10.1371/journal.pone.0192613
Yu, H., Lu, L., Jiao, B., Liang, C. (2019). Systematic discovery of novel and valuable plant gene modules by large-scale RNA-seq samples. Bioinformatics 35, 361–364. doi: 10.1093/bioinformatics/bty642
Zhang, Y., Sun, Y., Cole, J. R. (2014). A scalable and accurate targeted gene assembly tool (SAT-assembler) for next-generation sequencing data. PloS Comput. Biol. 10, e1003737. doi: 10.1371/journal.pcbi.1003737
Zheng, H., Wu, N., Chow, C. N., Tseng, K. C., Chien, C. H., Hung, Y. C., et al. (2017). EXPath tool–a systemfor comprehensively analyzing regulatory pathways and coexpression networks from high-throughput transcriptome data. DNA Res. 24, 371–375. doi: 10.1093/dnares/dsx009
Zhang, T. Q., Xu, Z. G., Shang, G. D., Wang, J. W. (2019). A single-cell RNA sequencing profiles the developmental landscape of arabidopsis root. Mol. Plant 12, 648–660.
Zhang, J., Zhang, X., Tang, H., Zhang, Q., Hua, X., Ma, X., et al. (2018). Allele-defined genome of the autopolyploid sugarcane Saccharum spontaneum l. Nat. Genet. 50, 1565–1573. doi: 10.1038/s41588-018-0237-2
Zhao, X., Fu, X., Yin, C., Lu, F. (2021). Wheat speciation and adaptation: perspectives from reticulate evolution. aBIOTECH 2, 386–402. doi: 10.1007/s42994-021-00047-0
Zouine, M., Maza, E., Djari, A., Lauvernier, M., Frasse, P., Smouni, A., et al. (2017). TomExpress, a unified tomato RNA-seq platform for visualization of expression data, clustering and correlation networks. Plant J. 92, 727–735. doi: 10.1111/tpj.13711
Keywords: plant transcriptomics, RNA-seq data analysis, alternative splicing, alternative polyadenylation, coexpression network, gene regulatory network, regulomics
Citation: Tu M, Zeng J, Zhang J, Fan G and Song G (2022) Unleashing the power within short-read RNA-seq for plant research: Beyond differential expression analysis and toward regulomics. Front. Plant Sci. 13:1038109. doi: 10.3389/fpls.2022.1038109
Received: 06 September 2022; Accepted: 21 November 2022;
Published: 08 December 2022.
Edited by:
Weizhen Liu, Wuhan University of Technology, ChinaReviewed by:
Yubin Li, Qingdao Agricultural University, ChinaAtsushi Fukushima, Kyoto Prefectural University, Japan
Chongjing Xia, Southwest University of Science and Technology, China
Copyright © 2022 Tu, Zeng, Zhang, Fan and Song. This is an open-access article distributed under the terms of the Creative Commons Attribution License (CC BY). The use, distribution or reproduction in other forums is permitted, provided the original author(s) and the copyright owner(s) are credited and that the original publication in this journal is cited, in accordance with accepted academic practice. No use, distribution or reproduction is permitted which does not comply with these terms.
*Correspondence: Min Tu, NzE5Mzc4NzA1QHFxLmNvbQ==; MTI3MzlAd2hwdS5lZHUuY24=; Guangsen Song, MTY5NzQ0NjExOUBxcS5jb20=
†These authors have contributed equally to this work