- 1Research Institute of Non-Timber Forestry, Chinese Academy of Forestry, Zhengzhou, China
- 2Key Laboratory of Non-timber Forest Germplasm Enhancement & Utilization of National Forestry and Grassland Administration, Zhengzhou, China
The leaves of Eucommia ulmoides contain various active compunds and nutritional components, and have successively been included as raw materials in the Chinese Pharmacopoeia, the Health Food Raw Material Catalogue, and the Feed Raw Material Catalogue. Core collections of E. ulmoides had been constructed from the conserved germplasm resources basing on molecular markers and morphological traits, however, the metabolite diversity and variation in this core population were little understood. Metabolite profiles of E. ulmoides leaves of 193 core collections were comprehensively characterized by GC-MS and LC-MS/MS based non-targeted metabolomics in present study. Totally 1,100 metabolites were identified and that belonged to 18 categories, and contained 120 active ingredients for traditional Chinese medicine (TCM) and 85 disease-resistant metabolites. Four leaf chemotypes of the core collections were established by integrated uses of unsupervised self-organizing map (SOM), supervised orthogonal partial least squares discriminant analysis (OPLS-DA) and random forest (RF) statistical methods, 30, 23, 43, and 23 chemomarkers were screened corresponding to the four chemotypes, respectively. The morphological markers for the chemotypes were obtained by weighted gene co-expression network analysis (WGCNA) between the chenomarkers and the morphological traits, with leaf length (LL), chlorophyll reference value (CRV), leaf dentate height (LDH), and leaf thickness (LT) corresponding to chemotypes I, II, III, and IV, respectively. Contents of quercetin-3-O-pentosidine, isoquercitrin were closely correlated to LL, leaf area (LA), and leaf perimeter (LP), suggesting the quercetin derivatives might influence the growth and development of E. ulmoides leaf shape.
1 Introduction
Although have not yet been fully explored, plant metabolites are important sources for food, medicines, health care products, feed additives and some industrial materials. Plants were estimated to produce above 200,000 metabolites in nature (Dixon, 2003), while a single species was thought to contain 4,000~20,000 metabolites that exerted a wide range of effects on growth, development, and interactions with biotic or abiotic environments (Fernie et al., 2004; Wang et al., 2019a). E. ulmoides had been widespread in continents of the northern hemisphere according to the fossil records, however, after the quaternary glaciation it only survived in northern and central China (Call and Dilcher, 1997). By the introduction and cultivation, at present E. ulmoides is widely planted in China as one of the important tree species of national strategic resources, which horizontally distributed in 24.5 ~ 41.5° N, 76 ~ 126° E, and vertically below 2500 m (Li et al., 2019). Many valuable metabolites including iridoids, flavonoids, phenylpropanoids, and lignans had been extracted from E. ulmoides leaves, and those exerted good functions in antibacterial, anti-inflammatory, anti-oxidant, immune regulation, and hypoglycemic, and anti-hypertension (Zhu et al., 2018; Wang et al., 2019b). E. ulmoides leaves were listed as traditional medicinal herbs in the 2005 and 2015 editions of the Chinese Pharmacopoeia, and included in the pilot project list for drug & food homologation by the National Health Commission in 2019 (Gong et al., 2021). Besides, the extracts of E. ulmoides leaves were stipulated for uses of growing-finishing pigs, fish, and shrimp in the Catalogue of Feed Additives (2013) that issued by the Ministry of Agriculture of China (Yang et al., 2021).
Phytochemotype was regarded as a plant phenotype differentiated by content, composition or structure of the endogenous chemicals within a species, which indicated the intraspecific variation and diversity (Rovesti, 1957; Eilers et al., 2021). Analyses of phytochemotype classification and its formation mechanism had both theoretical and practical values in guiding the development & utilization, quality evaluation and oriented cultivation of plant germplasm resources (Desjardins, 2008). Although standards of chemotype classification and nomenclature for different plant species have not been unified (Hua et al., 2009), intraspecific chemotype variations were found and studied in several tens plants including Agastache rugosa, Cannabis sativa, and Valeriana jatamansi since the concept proposed (He et al., 2018; Jin et al., 2021; Dang et al., 2022). Because of the development of statistical analysis for high dimensional data, and the maturity of chromatograph-mass spectrometer technologies, the high-throughput detection of plant metabolomics provided whole and new perspectives for accurate chemotype classification and evaluation, and related metabolic regulatory mechanisms (Deng et al., 2020; Yang et al., 2020). Metabolites in different plant chemotypes could be the causes or markers of the morphological traits (Saito, 2013). Co-expression analysis between metabolites and morphological traits facilitated early selection and prediction of complex traits in breeding, and that had been successfully applied to several staple crops (Shepherd et al., 2006; Wei et al., 2017; Shi et al., 2020). E. ulmoides was a monotypic species of Eucommiaceae. Germplasm genetic diversity of E. ulmoides had been evaluated by morphological traits, major active metabolites, and a number of molecular markers from RAPD to SNP (Wu et al., 2011; Yao et al., 2012; Yu et al., 2015; Du et al., 2017; Liu et al., 2022). Most results tended to believe that the genetic diversity of E. ulmoides at the intra-population level was higher than at the inter-population level, and its genetic distance was not evidently correlated to geographical distribution. However, at systematic chemotypic level, E. ulmoides germplasm resources were not been fully evaluated and classified except of the male flower core collections (Liu et al., 2020). E. ulmoides core collections from nearly 2,000 germplasm resources had been constructed and conserved in the National Germplasm Resources Bank of Major Famous Tree Species in the North (34°55′22″ N, 113°46′16″ E), Yuanyang, Henan Province (Li et al., 2018a).
In this study, we conducted a large scale metabolomics study by GC-MS and LC-MS/MS in leaves of 3 clonal replicates of 193 core collections (579 individuals) form 43 geographic origins that grew under similar growth environment and cultivation practices, to determine the possible leaf chemotypes and chemical markers across the collections. We processed the non-targeted metabolomics data by two clustering methods, K-means and SOM, and evaluated the results by comprehensive uses of RF model, OPLS-DA, and targeted determination of 13 metabolites. Additionally, we analyzed the correlations between the leaf metabolites and morphological traits, and the morphological markers of the chemotypes. These results would provide valuable references and be conductive to the germplasm collection strategy, directed breeding, quality control, and leaf resources utilization of E. ulmoides.
2 Materials and methods
2.1 Plant materials and sampling
Six individuals of 193 core collections have been planted after grafting propagation at tree spacing of 3 m × 3 m and implemented by basically consistent cultivation measures and managements in the E. ulmoides germplasm pool since 2013. Mature leaves grew at the middle annual branch were sampled from 2~4 individuals of each core collection in late August 2020. The samples were separated to 6 biological replications for GC-MS non-targeted determination, and to 3 biological replications for LC-MS/MS non-targeted determination, respectively. After sampling the materials were firstly frozen in liquid nitrogen, then stored at -80°C in laboratory. Samples for HPLC targeted determination were sampled in late August 2021 and followed the similar methods. Geographical origins of 193 collections and their leaf morphology characters were summarized and analyzed in Supplementary Table 1.
2.2 GC-MS analysis
Powdery sample was weighed (ca. 80.0 mg) after ground by liquid nitrogen and was added with 60 µL 0.2 mg·mL-1 ribitol and 1.5 mL extraction solvent (methanol, chloroform and water in volume ratio of 2.5:1:1) for 30 min sonication in cold water bath. After centrifuging the mixture at 12,000 rpm for 10 min at 4°C, the supernatant of 1ml was transferred, followed by the addition of 400 µL water. The supernatant of 100 µL was transferred and dried by nitrogen after the mixture vortexed for 30 s and centrifuged at 12,000 rpm for 5 min at 4°C. The methoxyamine hydrochloride of 60 µL (20 mg·mL-1) was added in pyridine to the resident solution and shaken for 90 min at 37°C for derivatization. Then 100 µL MSTFA and 1% TMCS were added to the mixture and shaken 30 min at 37°C. At last, the solution was derived at room temperature for 30 min and transferred to the liner tube for test after filtered by 0.50 µm filter membrane (Weckwerth et al., 2004).
The injection of 1 µL of solution into a Thermo scientific Trace 1310 GC-MS system (Thermo Fisher Scientific, USA) was performed in the non-split mode. Separation was carried out on a non-polar DB-5 capillary column (30 m × 0.25 mm I.D., J&W Scientific, USA), with high purity helium as the carrier gas at a constant flow rate of 1.0 mL/min. The column temperature program was of 80°C for 5 min, following ramped at 10°C. min to 195°C and held for 4 min, and then ramped at 3°C. min to 260°C and held for 6 min, and finally ramped at 4°C. min to 305°C and held for 5 min. Temperature of the ion source, the transmission line and the inlet temperature was set to 310°C, 260°C, and 280°C, respectively. Full scan mode (SCAN) was employed and the mass scan range was of 50~600 m/z. The quality control (QC) sample was prepared by mixing aliquots of the samples to be a pooled sample. To monitor and assess GC-MS system stability, 1 injection of QC sample was tested behind every 10 injections of the normal samples (Subbaraj et al., 2019).
2.3 LC-MS/MS analysis
The powder sample of 100 mg was ground and weighed after vacuum freeze-drying The sample was added with 1 mL of 50% methanol and shaken for 1 h. Then the mixture was centrifuged at 14,000 rpm for 10 min after placed in a refrigerator at 4°C overnight. The supernatant was transferred to an inner tube for test after filtered through 0.22 µm membrane.
The injection of 5 µL of solution into liquid chromatography Q-Exactive Orbitrap mass spectrometry (Thermo Fisher Scientific, USA) was performed by the autosampler at 10°C. Chromatographic separation was carried out on the Hypersil GOLD™ AQC18 column (2.1 mm × 100 mm, particle size 1.9 µm) at 30°C applying the following binary gradient at a flow rate of 300 µL. min-1: 0-2.5 min, isocratic 90%-95% A (water/formic acid, 99.9/0.1 [v/v]), 5%-10% B (acetonitrile/formic acid, 99.9/0.1 [v/v]); 2.5-6 min, 10%-12% B; 6-10 min, 12%-15% B; 10-13 min, 15%-17% B; 13-14 min, 17%-25% B; 14-20 min, 25%-80% B; 20-22 min, 80%-100% B 22-25 min, 100% B; 25-25.1 min, linear from 100% to 5% B; 25.1-28 min, 5% B. Eluted compounds were detected from m/z 70 to 1,050 using a Q-Exactive Orbitrap mass spectrometer equipped with an HESI electrospray ion source in positive and negative ion modes using the following instrument settings: spray voltage, ± 3.2 kV; Full MS resolution, 70,000; dd-MS2 resolution,17,500; collision gas, high purity N2; step collision energy, 20/40/60 V. QC samples were scanned at interval of 10 samples (Li et al., 2018b).
2.4 HPLC analysis
Powder samples of 500 mg were ground and weighed after vacuum freeze-drying. The sample was added with 10 mL of 60% methanol and extracted by ultrasonication for 30 min. Then the mixture was centrifuged at 12,000 rpm for 10 min after placed in a refrigerator at 4°C overnight. The supernatant was transferred to injection bottle for test after filtered through 0.22 µm membrane.
The injection of 5 µL solution into high performance liquid chromatography (Waters ACQUITY Arc system, USA) coupled with a 2998 photodiode array (PDA) detector was performed. Chromatographic separation was carried out on Thermo Syncronis C18 column (250 mm × 4.6 mm, particle size 5 µm) at 30°C applying the following binary gradient at a flow rate of 800 µL·min-1: 0-10 min, isocratic 83%-95% A (water/formic acid, 99.8/0.2 [v/v]), 5%-17% B (acetonitrile/formic acid, 99.9/0.1 [v/v]); 10-25 min, 17%-20% B; 25-30 min, 20%-30% B; 30-50 min, 30%-50% B; 50-55 min, 50%-70% B; 55-57 min, linear from 70% to 5% B. The detection wavelengths were set to 204 nm for aucubin, 239 nm for geniposide acid and asperuloside, 247 nm for protocatechuic acid, 276 nm for catechin and pinoresinol diglucoside, 264 nm for methyl gallate, genipin and rutin, 325 nm for chlorogenic acid, and 364nm for isoquercitrin, quercetin and kaempferol. Above 13 compounds were identified respectively by comparison of the retention time to corresponding standard, and their contents were determined according to the external standard method (Supplementary Table 2 and Supplementary Figure 1).
Standards of aucubin, chlorogenic acid, rutin, methyl gallate, and kaempferol with purity≧98% were purchased from Shanghai Anpu Experimental Technology Co., Ltd. standards of genipin, catechin, and asperuloside with purity = 98% were from Shanghai Yuanye Bio-technology Co., Ltd. and the standards with purity = 98% including geniposide acid, protocatechuic acid, quercetin, and pinoresinol diglucoside were from Chengdu Manst Bio-technology Co., Ltd. Isoquercitrin standard with purity = 97% was purchased from National Institute for the Control of Pharmaceutical and Biological Products (Beijing, China).
2.5 Identification and quantification
The MS spectra data determined by GC-MS and LC-MS/MS were firstly converted to analysis base file (Abf) format by ABF Converter. Initial characteristic features were obtained after peak detection, peak identification, deconvolution, peak alignment, filtering, characterization and retention time correction of MS spectra data by MS-DIAL (V4.38) (Tsugawa et al., 2015). The main MS-DIAL parameter settings are provided in Supplementary Table 3. The feature values were removed when the features were undetected in 80% of the biological samples, then the extrapolated values were filled using the k-nearest neighbor algorithm (KNN) (Dunn et al., 2011).
High quality features in QC samples were obtained after their peak values calibrated comparatively by three algorithms including Random forest (RF), locally weighted scatter plot smoothing (LOESS), and support vector regression (SVR). For GC-MS features, the criterion of optimal algorithm was relative standard deviation (RSD) of the peak values of the reserved features below 50% and their quantity proportion in total features above 70%, and for LC-MS/MS features, the criterion was RSD below 30% and the proportion above 70%. RF and LOESS analyses were completed by statTarget R package (V1.16.1), and SVR analysis was completed by MetNormalizer R package (V1.0) (Luan et al., 2018; Shen et al., 2019).
The metabolites determined by GC-MS were annotated basing on the Fiehn database (https://fiehnlab.ucdavis.edu/) and retention index (retention index, RI) with C8~C40 n-alkanes (Lai et al., 2018), and those determined by LC-MS/MS were annotated basing on Massbank (http://www.massbank.jp), GNPS (http://gnps.ucsd.edu) and ReSpect (http://spectra.psc.riken.jp/) (Tsugawa et al., 2015). Traditional Chinese Medicine Systems Pharmacology Database and Analysis Platform (TCMSP) (https://tcmsp-e.com/tcmsp.php) was also used to screen the active ingredients for traditional Chinese medicine (TCM) and disease-resistant metabolites in the annotated metabolites (Li et al., 2022). For large-scale samples, unstable injection often occurred in the determination of GC-MS, which could result in variable metabolome data in the biological duplicates, PCA analysis were carried out to remove 3 outliers of each collection. The final metabolome data were combined from the GC-MS and LC-MS/MS after removing repeat annotated metabolites.
2.6 Statistical analysis
The data set was firstly log standardized, and then averaged to enhance normality. SOM unsupervised clustering and K-means were used to perform the chemotype classification by analyzing the metabolite composition and content, and RF discriminant model was used to evaluate difference in the chemotypes. The optimal clustering method and the number of chemotypes classification were identified by evaluating the classification effect of the two clustering methods. SOM analysis was carried out by Python 3.6 software, K-means and RF analyses were carried out by R 3.6 software, in which the tree number of RF modle was set to 1,000 (Chavent et al., 2021). Principal component analysis (PCA) was also used by SIMCA-P (V14.0) software to evaluate the difference in the germplasms (Saccenti et al., 2014). The differences between certain chemotype and the other chemotypes were evaluated by OPLS-DA, in which the values of R2Y(cum) and Q2Y(cum) were used to judge the validity of the model (Triba et al., 2015). Latent biomarkers of each chemotype were screened basing on the OPLS-DA results and using 4 criteria: (1) a variable importance of projection (VIP) value ≥2, (2) a Student’s t-test p value < 0.05, (3) a fold change (FC) value > 0.5, and (4) a mean decrease accuracy (MDA) value ≥ 2 in the RF model, and finally the results were shown in volcano plots using R software (V3.6).
Metabolite co-expression network was constructed to explore potential correlations between metabolites and leaf morphological traits using WGCNA by R package (V1.70), and a visual correlation network between key metabolite and leaf morphological traits was construct using Cytoscape software (V3.7.0) (DiLeo et al., 2011). RF regression model using metabolites in the key module as independent variables and leaf morphological traits as dependent variables was developed, in which the predictability was evaluated by determination coefficient R2. In addition, leaf morphological trait-related markers were screened according to the variable importance measured in the RF model (Dan et al., 2021). Finally, leaf morphological traits were predicted by least absolute shrinkage and selection operator (LASSO) regression using the non-targeted metabolome data (Xu et al., 2017).
3 Results
3.1 Data correction and metabolite annotation
TIC plots of typical QC samples of GC-MS and LC-MS/MS untargeted metabolomics assays were shown in Supplementary Figure 2. For both GC-MS and LC-MS/MS mass spectra data of E. ulmoides leaves, RF model was more optimal and suitable for QC calibration than the LOESS and SVR algorithms according to the employed criterions, and 85.38%, 82.25% and 71.94% of the total features were eserved in QC samples determined by GC-MS, ESI+ and ESI- mode of LC-MS/MS, respectively (Supplementary Table 4 and Supplementary Figure 3). Totally 7,438 initial characteristic features, including 5,910 high quality features, were obtained from GC-MS mass spectra data, and 30,881 and 15,553 initial characteristic features, including 27,526 and 14,633 high quality features, were obtained from LC-MS/MS mass spectra data in the ESI+ and ESI- mode, respectively (Supplementary Table 4). In addition, RSD of the internal standard ribitol by GC-MS determination was 30.84%, and that also showed the calibrated results were stable and reliable after QC calibration by RF model (Supplementary Figure 3).
For metabolite annotation, 209 and 891 metabolites were annotated from the GC-MS and LC-MS/MS determination, respectively, and finally 1,100 metabolites were annotated in leaves of 193 E. ulmoides core collections after metabolome assay (Supplementary Tables 5, 6). The metabolites broadly classified into 18 categories according to KEGG database, the top 6 of which were flavonoids, organic acids, amino acids, phenylpropanoids, lipids, and terpenoids, accounting for 14%, 11%, 11%, 10%, 8%, and 8% of the total metabolites, respectively (Figure 1). 120 active ingredients were identified among the annotated metabolites through the TCMSP database. In addition, 85 disease-resistant metabolites were identified and functioned to at least one disease (Supplementary Table 7), with 10, 30, 19, 43, 23, and 38 metabolites of effects on anti-cancer/tumor, anti-diabetic, anti-cardiovascular, anti-hypertensive, anti-atherosclerotic, and anti-thrombotic, respectively. The above information indicated that E. ulmoides leaves were rich in medicinal health substance and could be excellent raw material for pharmatical use.
3.2 Chemotype classification
A matrix consisted of 193 samples ×1,100 metabolites was used to classify the potential chemotypes of E. ulmoides leaves. According to K-Means gap statistic, the whole collections were suitable to divide into 4, 5, and 6 groups by K-Means cluster, and incomplete coincidently, similar results were obtained by SOM cluster (Figure 2A). To determine which one was the optimal classification method, discriminant RF model was employed to evaluate the results of K-Means and SOM basing on the content of the annotated metabolites. 75% of the 193 core collections were selected as the training set for constructing the prediction model, and the other 25% as the independent set for cross validation. Four chemotypes classified by SOM clustering was considered an optimal result, for the average prediction accuracy of the training set and independent set were highest in the RF model, respectively reaching to 92.52% and 91.30% (Table 1).
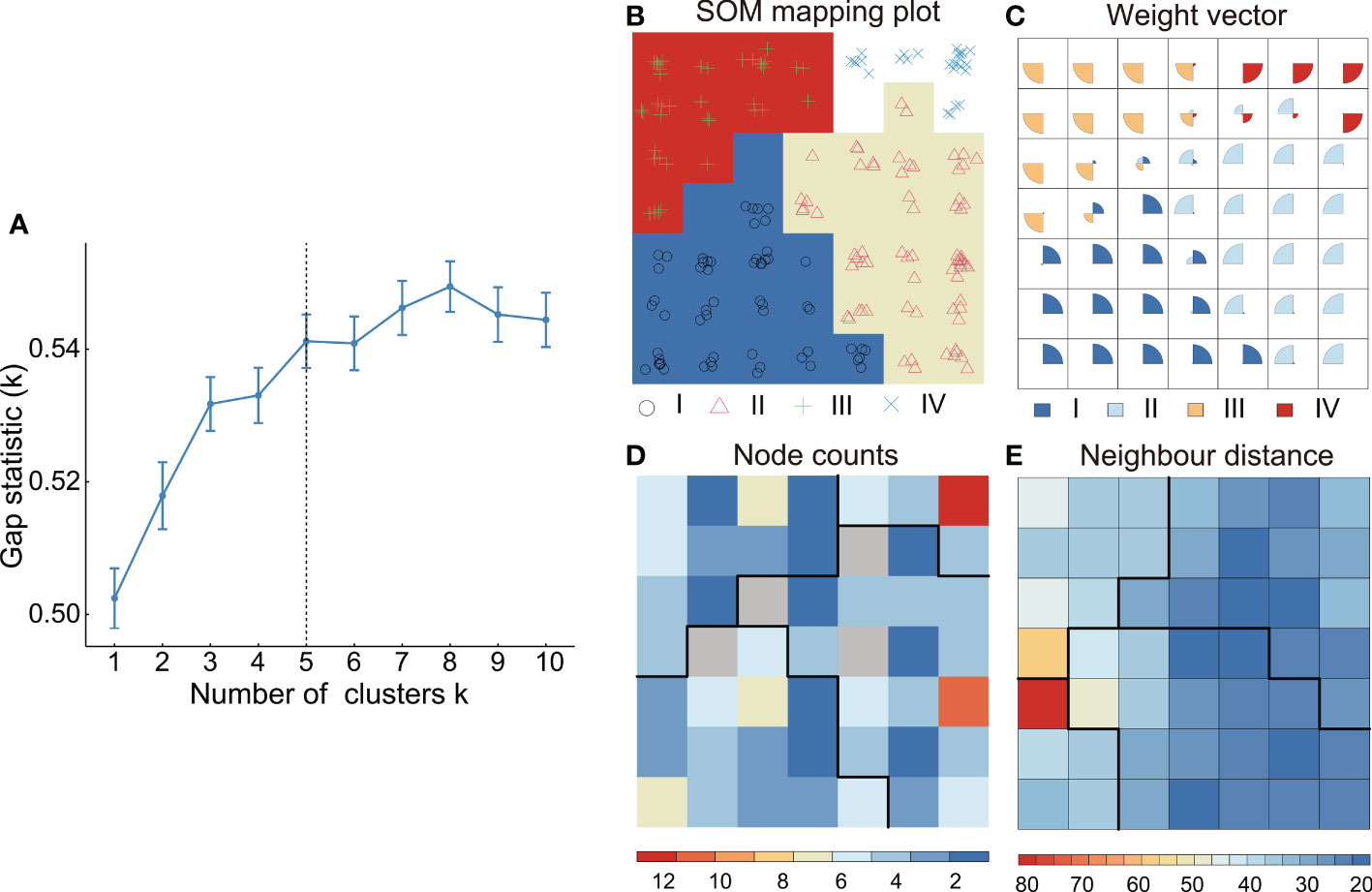
Figure 2 Chemotype classification by K-Means cluster and SOM cluster. (A) Number of clusters K via the K-means gap statistic. (B) Topological mapping plot of the SOM cluster. (C) Distribution patterns of the samples and variables of the SOM cluster. (D) Variable allocations in each grid node of the SOM cluster. (E) Distance of neighboring grid nodes of the SOM cluster.
Topological mapping maps of SOM showed the projection of the metabolome dataset from high-dimension to low-dimension. For topological mapping maps of four chemotypes classified by SOM, 7 × 7 rectangular topology was selected as the final output layer after 100 iterations of training on the data matrix, in which the minimum quantization error and topology error were taken as training termination criteria. In the obtained topological mapping plots, Figure 2B showed that four modules were classified in the samples and similar patterns were expressed in the same group, Figure 2C showed the distribution patterns of the samples and variables, with weight vector of the node representing the variables mapped to the node and the fan charts in the grids representing the sample size in the weight vector, Figure 2D showed the variable allocations in each grid node, with even allocations in the grid nodes representing accurate classification of the corresponded groups, Figure 2E showed the distances between the grid nodes and its adjacent nodes, with the distances lengthening followed to the difference in the nodes increasing. Finally, the 193 collections were classified into four leaf chemotypes, and for each chemotype, consisted of 58, 69, 39, and 27 collections, respectively (Supplementary Table 8).
3.3 Chemotype comparison
The separation and similarity across the chemical groups were examined using PCA model, in which 12 principal components were obtained that accounting for 42.6% of the total variance. PC1, PC2, and PC3 accounted for 10.70%, 8.16%, and 5.91% of the total variance, respectively. In the score plot of PC1 and PC2, chemotypes III and chemotype IV were well separated but chemotype I and chemotype II showed partial overlap, while in the score plot of PC1 and PC3 (Figure 3), each chemotype was evidently separated. However, no evident correlation was observed between the leaf chemotypes and their geographical origins in the PCA plots. Four supervised OPLS-DA models were constructed to measure the differences between a specific leaf chemotype and the other three chemotypes. From the results of ranking test in each group, values of R2Y and Q2 of the model after Y replacement were lower than those of the original model as conducting the replacement validation (Figure 3 and Supplementary Table 9), and that indicated the employed OPLS-DA models had good robustness and each classified leaf chemotype was of special characteristic.
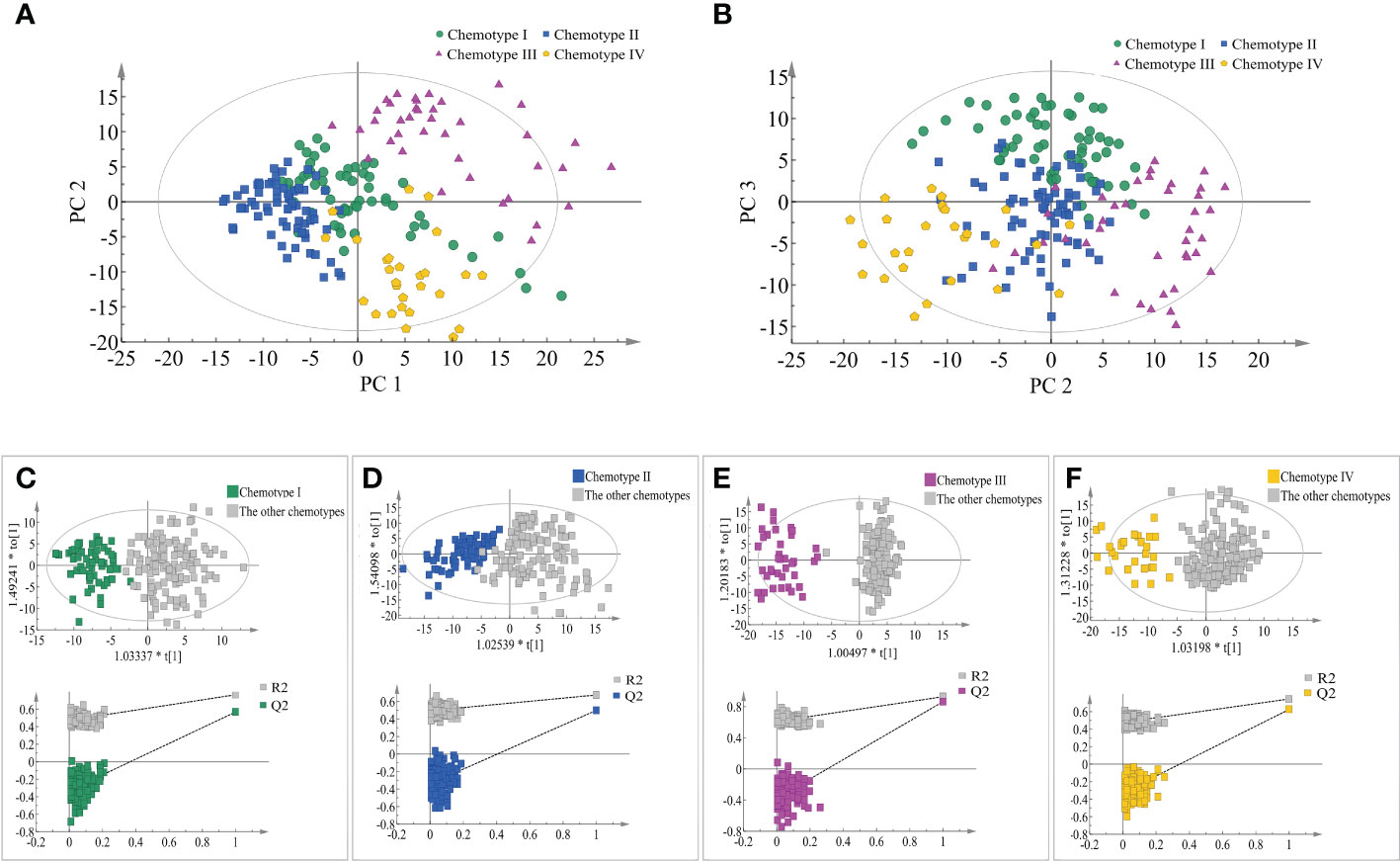
Figure 3 Comparisons of the four classified chemotypes. (A) The four chemotypes compared by PC1 and PC2 of PCA model. (B) The four chemotypes compared by PC2 and PC3 of PCA model. (C) Comparisons between chemotype I and the other chemotypes by OPLS-DA model. (D) Comparisons between chemotype II and the other chemotypes by OPLS-DA model. (E) Comparisons between chemotype III and the other chemotypes by OPLS-DA model. (F) Comparisons between chemotype IV and the other chemotypes by OPLS-DA model.
3.4 Chemomarkers screening
VIP values, P-values, FC values, and MDA values were used to build the criteria of chemomarkers screening. A total of 103 markers were screened in the four chemotypes, in which 30, 23, 43, and 23 markers corresponded to chemotype I, chemotype II, chemotype III, and chemotype IV, respectively. In addition, 20, 17, 33, and 17 markers were found exclusive to the chemotype I, chemotype II, chemotype III, and chemotype IV, respectively, and that suggested these chemomarkers were divergent and could be effective in indicating the chemotypes (Figure 4 and Supplementary Table 10). For chemotype I, the chemomarkers mainly belonged to flavonoids and phenylpropanoids, and three terpenes of 20 content up-regulated chemomarkers were TCM active ingredients. Chemotype II contained the only lactone marker, linderalactone, also identified as active ingredients for TCM and disease-resistant metabolites, and contained the only indole marker, indoline. For chemotype III, the chemomarkers were almost terpenes, lipids, and organic acids, and three of 31 content up-regulated chemomarkers were TCM active ingredients and one up-regulated chemomarkers belonged to disease-resistant metabolites. For chemotype IV, the chemomarkers were mainly phenolics and phenylpropanoids, and contained one up-regulated chemomarkers belonged to the active ingredients for TCM.
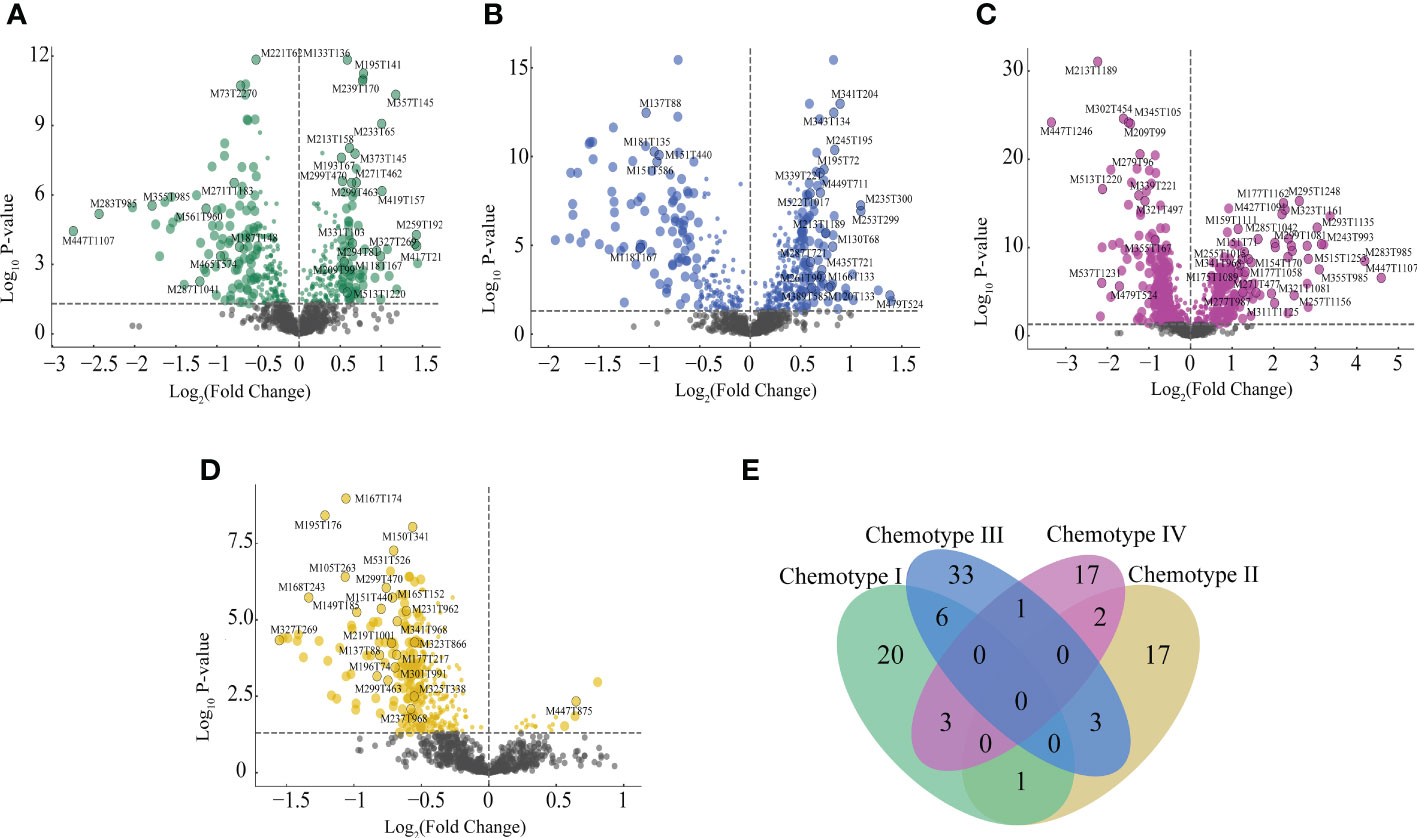
Figure 4 Screened chemomarkers of the four chemotypes. (A) Chemomarkers of chemotype (I) (B) Chemomarkers of chemotype II. (C) Chemomarkers of chemotype III. (D) Chemomarkers of chemotype IV. (E) Venn plot of the chemomarkers in four chemotypes.
RF model was employed to evaluate the accuracy of the chemomarkers in distinguishing the chemotype. Average prediction accuracy of the training set and independent test set for the four chemotypes were 92.52% and 84.78% with AUC value of the independent set 0.972, and specially, the prediction accuracy for chemotype III reached to 100% (Supplementary Table 11). Therefore, the results from RF model also showed the chemomarkers were steady to classify the leaf chemotypes of E. ulmoides.
3.5 Target validation of the chemotypes by 13 metabolites
Contents of 13 important metabolites of E. ulmoides leaves frequently followed in previous studies were determined in 193 core collections, as spot checks on the non-targeted results of the chemotype classification and chemomarker screening. Except for three metabolites, methyl gallate, pinoresinol diglucoside, and genipin, 10 metabolites showed similar varied tendency in each chemotype basing on the comparisons between results of the non-targeted and targeted determination (Supplementary Figure 4). Specifically, isoquercitrin and kaempferol, chemomarkers of the chemotype I, aucubin and chlorogenic acid, chemomarkers of the chemotype III , their contents in the non-targeted results were down regulated in they indicated chemotypes when comparing to the other types and that was observed exactly consistent with the targeted results by HPLC determination.
3.6 Morphological markers of the chemotypes
LASSO model was used to predict the leaf morphological traits basing on contents of 1,100 metabolites, to assess the correlations between leaf metabolites and morphological traits, and examine the feasibility of screening morphological markers. Average predictability of the 13 morphological traits was 0.3566. The top four predictive values were 0.5694, 0.5502, 0.4954, and 0.3914, respectively, corresponding to leaf dentate number (LDN), chlorophyll reference value (CRV), leaf perimeter (LP), and specific leaf dry weight (SLDW) (Figure 5), which indicated a certain degree of associations between the metabolites and morphological traits in E. ulmoides leaves. Significant differences were observed by ANOVA in four leaf morphological traits referring to SLFW, CRV, leaf dentate height (LBH), and leaf thickness (LT) among the four leaf chemotypes. The average values of SLFW and CRV were highest in chemotype II, and value of LBH was highest in chemotype III, while value of LT was highest in chemotype IV after multiple comparisons (Supplementary Table 12).
Nine metabolite modules contained 601 metabolites were obtained by WGCNA between 1100 metabolites and 13 morphological traits, then a correlation heat map was made between the modules and the morphological traits (Figure 6A). For chemotype I, 17 of 20 up-regulated chemomarkers were included to the yellow metabolite module that closely positive correlated with leaf length (LL) in the heat map, and that implicated LL was one of the potential morphological markers of chemotype I. Analogously, for chemotype II, III, and IV, CRV, LBH and LT were their corresponded potential morphological markers, respectively, after examining the correlations between morphological traits and chemomarkers through the MEbrown module, the MEblue module, the MEgreen module, the MEpink module, and the MEyellow module (Supplementary Table 13).
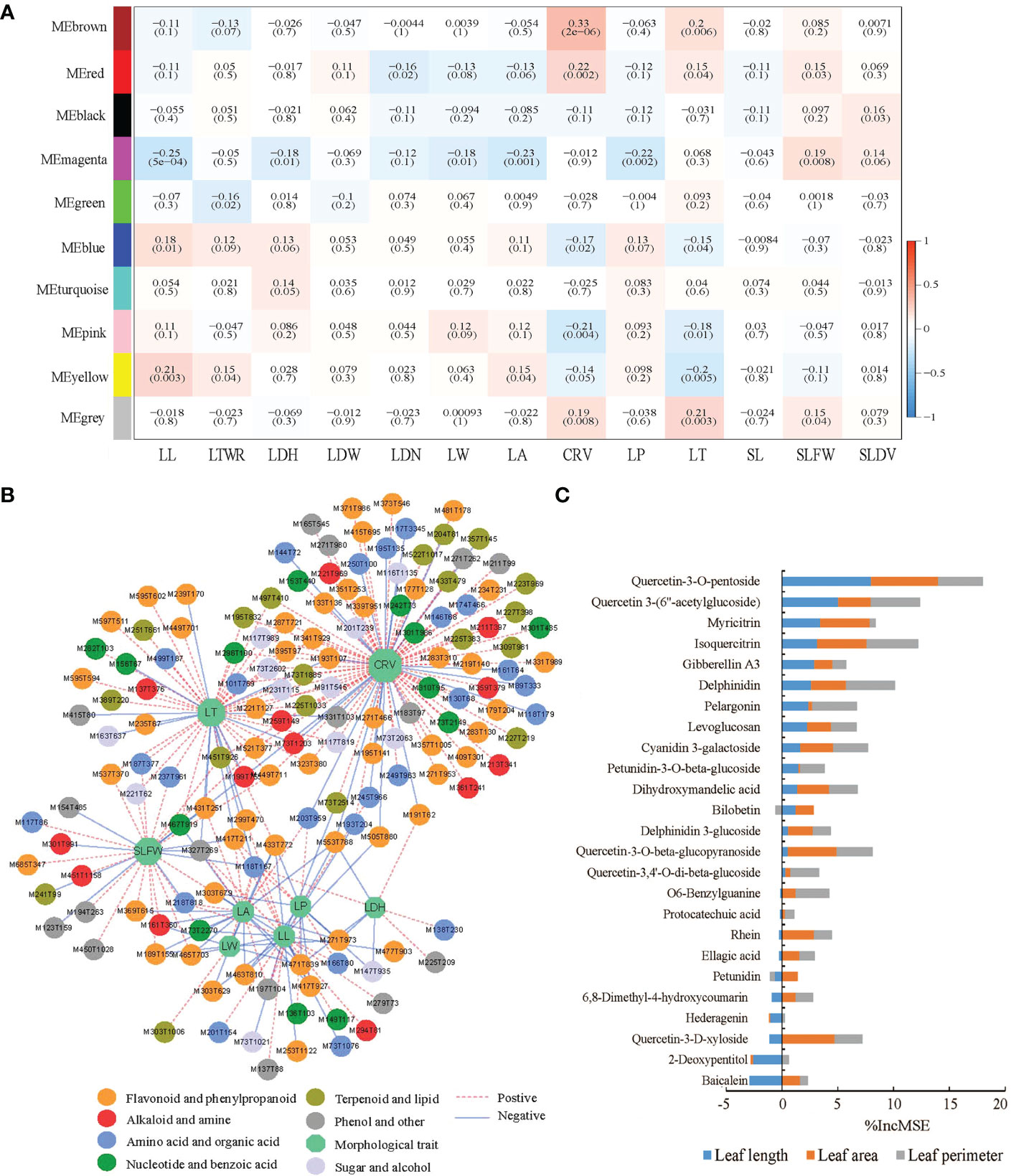
Figure 6 Correlation between the metabolites and the morphological traits of E ulmoides leaves. (A) Heat map of the correlations between the modules obtained by WGCNA and the morphological traits. (B) Correlation analysis between 377 metabolites in six key metabolite modules and eight morphological traits. (C) IncMSE% in the RF regression for 25 metabolites in the Memagenta module. (LL, Leaf length; LTWR, Length to width ratio of leaf; LDH, Leaf dentate height; LDW, Leaf dentate width; LDN, Leaf dentate number; LW, Leaf width; LA, Leaf area; CRV, Chlorophyll reference value; LP, Leaf perimeter; LT, Leaf thickness; SL, Stipe length; SLFW, Specific leaf fresh weight; SLDW, Specific leaf dry weight).
Five flavonols (quercetin 3-(6″-acetylglucoside), quercetin-3-O-beta-glucopyranoside, quercetin-3-O-pentosidine, isoquercitrin, and kaempferol-3-O-glucoside) and three phloretic glycosides delphinidin, petunidin-3-O-glucoside, and cyanidin-3-O-alpha-arabinoside, were significantly negative correlated to LL after further correlation analysis between six key metabolite modules and eight morphological traits (Figure 6B and Supplementary Table 14). In addition, quercetin-3-O-pentosidine and isoquercitrin were of high importance degrees when their contents analyzed as dependent variables in RF regression analysis with LL, leaf area (LA), and leaf perimeter (LP), and that also suggested the three traits could be the morphological markers denoting the contents of the two metabolites in E. ulmoides leaves (Figure 6C).
4 Discussions
E. ulmoides has been considered of limited intraspecific variation as a monocotyledonous tree species of single family and single genus (Li et al., 2018a), and limited genetic diversity in the breeding are concerned by many E. ulmoides researchers. Accurate evaluation of the chemical composition and chemotype classification of germplasm resources could effectively expand the genetic base, promote the breeding potential and accelerate the directed breeding of E. ulmoides. Related studies had been conducted in diverse tea populations (Yu et al., 2020). Chemical components in different tissues of E.ulmoides including leaves, seeds and barks had been determined by ultra-high-performance liquid chromatography-tandem time-of-flight mass spectrometer (UHPLC-QTOF/MS) untargeted metabolomics, and finally 2,373 metabolites were identified in total (Chen et al., 2022). Besides, the dynamic metabolic models for leaf growth and development of E.ulmoides were constructed by integrated uses of widely targeted metabolomics and transcriptomics (Li et al., 2019). However, few studies employed metabolomics to wholly determine and evaluate the germplasm collections of E.ulmoides, expect of a recent study on the core collections of male flower (Liu et al., 2020). In present study, 1100 metabolites were determined by GC-MS and LC-MS/MS untargeted metabolomics in 579 samples, which sketched a comprehensive and explicit metabolite map of E. ulmoides leaves. The chemical variations in different collections were systematically displayed, and that provided holistic approaches and laid important foundations for germplasm resource evaluation, leaves quality control, metabolic regulation and directed breeding of E. ulmoides.
Recently, advanced machine learning algorithms including SOM and deep belief networks (DBN) were increasingly employed to deal with the high dimensional data in plant omics studies. As an unsupervised artificial neural network, SOM soft clustering was of high generalization ability and outperformed the K-means hard clustering in clustering similar characteristic data in regions of same network topology by self-organized learning space distribution of the eigenvectors (Crespo et al., 2020). RF was a compositive supervised learning method and could be regarded as an extension of the decision tree, which proceeded classification and regression of high dimensional data without dimension reduction and measured the relative importance of variables to the classification results, and that made it of clear advantages in processing large datasets (Chavent et al., 2021). OPLS-DA model was effective in examining the similarities or differences in specified groups, and performed internal and external validation to evaluate the effectiveness of the constructed model (Triba et al., 2015). The comprehensive use of the SOM, RF and OPLS-DA in chemotype classification of E. ulmoides leaves through analyzing matrices of complex sample size and metabolite quantity offered highly reliable and accurate results that were superior to K-means classification generally used in previous studies. However, for the classification principles, standards, and naming rules for specific chemotype have not been unified, the present classification of the leaf chemotype based on the content of metabolites just confirmed that chemical differentiations existed in the classified groups. The formulations of classification standards, naming rules and sub-divisions of the present classification should be given priority and issued in future research. Similar to the results from male flowers of E. ulmoides (Liu et al., 2020), collections belonged to the leaf chemotypes did not corresponded to their geographical distributed regions, indicating the variation in the leaf chemotypes might originate from intra-population variation and were broadly consistent with the genetic variations of E. ulmoides collections (Wuyun et al., 2018).
One or several metabolites can be used as biomarkers to identify collections belonged to specific chemotype, morphological type or genotype in the plant germplasm resource, and even to some biological processes, for instance, hybrid advantage of yield can be predicted by metabolites levels in the tyrosine metabolic pathway (Dan et al., 2021). 103 chemomarkers mostly belonged to terpenoids, flavonoids, phenolic glycosides, and lipids were screened on basis of values of VIP, p, and FC, and additionally, variable importance in RF model. Differences in the plant chemotypes might not be displayed in metabolites composition but also in morphological, growing and developmental traits. To chemotypes formation of Tanacetum vulgare, it was concluded that the number and diameter of flower heads, flowering period, and pollen nutritional quality were the significant indicators (Eilers et al., 2021). The morphological markers of the E. ulmoides leaf chemotypes were screened by hunting the correlations between the chemomarkers and morphological traits. The integrated application of chemomarkers and morphological markers will contribute to identify the chemotype of germplasm resources, and accelerate the efficiency in E. ulmoides breeding.
Correlation analysis between certain metabolites and extrinsic traits was important for understanding the molecular mechanisms of phenotypic variation (Chen et al., 2016). The genetic relationship between metabolism and phenotype in wheat had been revealed by combined analysis of metabolite-growth trait correlations and quantitative trait locus (Shi et al., 2020). Besides, the grain shape and stress resistance of rice was regulated by rice glycosyltransferase GSA1 modulating the phenanthrene metabolism (Dong et al., 2020). The predictability of metabolites values to LDN, CRV, LP, and SLDW of E. ulmoides reached to 0.5694, 0.5502, 0.4954, and 0.3914, respectively, by LASSO. This is comparable to the results from recombinant inbred line (RIL) in 145 wheat, with the predictability to grain per spike and plant height reaching to 0.51 and 0.46 by LASSO (Shi et al., 2020). In addition to significant negative correlations were observed between the content of isoquercitrin from target determination and the external traits such as LL, the contents of quercetin-3-O-pentosidine and isoquercitrin were closely correlated to LL, LA, and LP by RF regression analysis, and these results indicated quercetin derivatives might take an important role in the development of leaf shape of E. ulmoides.
5 Conclusions
A set of GC-MS and LC-MS/MS non-targeted metabolomics methods including sampling processing, metabolite extraction and determination, metabolite annotation and quantification, and data calibration was established for leaves of E. ulmoides, providing a basis for disclosing the metabolic diversity and variation, chemotype classification, and biomarkers screening for the germplasm resource. 1,100 metabolites belonged to 18 categories and contained 120 active ingredients for TCM and 85 disease-resistant metabolites were identified in leaves of 193 core collections of E. ulmoides. The integrated uses of unsupervised SOM, supervised OPLS-DA, and RF statistical methods were suitable to process classification and markers screening basing on the non-targeted metabolomics data. 103 chemomarkers corresponding to four established leaf chemotypes of E. ulmoides were screened, and the morphological markers linked to the leaf chemotypes were examined. Quercetin derivatives may influence the growth and development of the leaf shape of E. ulmoides, and that require further studies to confirm.
Data availability statement
The data presented in the study were deposited in the Metabolights repository, accession number MTBLS5958.
Author contributions
PL designed and supervised the experiments; YM and QD conducted the experiment, analyzed the data, and wrote the paper; HD revised the manuscript, QW, LW, and LD performed sample collection. All authors read and approved the final version of the manuscript.
Funding
This work was supported by the Zhengzhou special funds for basic and applied basic research (Research on the efficient utilization of Eucommia germplasm resources based on spectroscopic and chemometric techniques) and National Key R&D Program of China (2017YFD0600702).
Conflict of interest
The authors declare that the research was conducted in the absence of any commercial or financial relationships that could be construed as a potential conflict of interest.
Publisher’s note
All claims expressed in this article are solely those of the authors and do not necessarily represent those of their affiliated organizations, or those of the publisher, the editors and the reviewers. Any product that may be evaluated in this article, or claim that may be made by its manufacturer, is not guaranteed or endorsed by the publisher.
Supplementary material
The Supplementary Material for this article can be found online at: https://www.frontiersin.org/articles/10.3389/fpls.2022.1029907/full#supplementary-material
Abbreviations
SOM, self-organizing map; PCA, principal component analysis; OPLS-DA, orthogonal partial least squares discriminant analysis; WGCNA, weighted gene co-expression network analysis; KNN, k-nearest neighbor algorithm; RF, random forest; LOESS, locally weighted scatter plot smoothing; SVR, support vector regression; LASSO, least absolute shrinkage and selection operator; RSD, relative standard deviation; TCM, traditional Chinese medicine; QC, quality control; LL, leaf length; LTWR, length to width ratio of leaf; LDH, leaf dentate height; LDW, leaf dentate width; LDN, leaf dentate number; LW, leaf width; LA, leaf area; CRV, chlorophyll reference value; LP, leaf perimeter; LT, leaf thickness; SL, stipe length; SLFW, specific leaf fresh weight; SLDW, specific leaf dry weight.
References
Call, V. B., Dilcher, D. L. (1997). The fossil record of Eucommia (Eucommiaceae) in north America. Am. J. Bot. 84, 798–814. doi: 10.2307/2445816
Chavent, M., Genuer, R., Saracco, J. (2021). Combining clustering of variables and feature selection using random forests. Commun. Stat - Simulation Comput. 50, 426–445. doi: 10.1080/03610918.2018.1563145
Chen, J. Y., Wang, W. T., Kong, J. Q., Yue, Y. D., Dong, Y. Y., Zhang, J. C., et al. (2022). Application of UHPLC-Q-TOF MS based untargeted metabolomics reveals variation and correlation amongst different tissues of Eucommia ulmoides Oliver. Microchem. J. 172, 106919. doi: 10.1016/j.microc.2021.106919
Chen, W., Wang, W. S., Peng, M., Gong, L., Gao, Y. Q., Wan, J., et al. (2016). Comparative and parallel genome-wide association studies for metabolic and agronomic traits in cereals. Nat. Commun. 7, 12767. doi: 10.1038/ncomms12767
Crespo, R., Alvarez, C., Hernandez, I., García, C. (2020). A spatially explicit analysis of chronic diseases in small areas: A case study of diabetes in Santiago, Chile. Int. J. Health Geograph. 19, 24. doi: 10.1093/plphys/kiab273
Dan, Z. W., Chen, Y. P., Li, H., Zeng, Y. F., Xu, W. W., Zhao, W. B., et al. (2021). The metabolomic landscape of rice heterosis highlights pathway biomarkers for predicting complex phenotypes. Plant Physiology 187, 1011–1025. doi: 10.1093/plphys/kiab273
Dang, J. J., Lin, G. Y., Liu, L. C., Zhou, P. N., Shao, Y. F., Dai, S. L., et al. (2022). Comparison of pulegone and estragole chemotypes provides new insight into volatile oil biosynthesis of Agastache rugosa. Front. Plant Sci. 13. doi: 10.3389/fpls.2022.850130
Deng, M., Zhang, X. H., Luo, J. Y., Liu, H. J., Wen, W., Luo, H. B., et al. (2020). Metabolomics analysis reveals differences in evolution between maize and rice. Plant J. 103, 1710–1722. doi: 10.1111/tpj.14856
Desjardins, A. E. (2008). Natural product chemistry meets genetics: When is a genotype a chemotype? J. Agric. Food Chem. 56, 7587–7592. doi: 10.1021/jf801239j
DiLeo, M. V., Strahan, G. D., Bakker, M., Hoekenga, O. A. (2011). Weighted correlation network analysis (WGCNA) applied to the tomato fruit metabolome. PloS One 6, e26683. doi: 10.1371/journal.pone.0026683
Dixon, R. A. (2003). Phytochemistry meets genome analysis, and beyond. Phytochemistry 62, 815–816. doi: 10.1016/S0031-9422(02)00712-4
Dong, N. Q., Sun, Y. W., Guo, T., Shi, C. L., Zhang, Y. M., Kan, Y., et al. (2020). UDP-Glucosyltransferase regulates grain size and abiotic stress tolerance associated with metabolic flux redirection in rice. Nat. Commun. 11, 2629. doi: 10.1038/s41467-020-16403-5
Dunn, W. B., Broadhurst, D., Begley, P., Zelena, E., Francis-McIntyre, S., Anderson, N., et al. (2011). Procedures for large-scale metabolic profiling of serum and plasma using gas chromatography and liquid chromatography coupled to mass spectrometry. Nat. Protoc. 6, 1060–1083. doi: 10.1038/nprot.2011.335
Du, Q. X., Wei, Y. X., Liu, P. F., Du, H. Y. (2017). Diversity of the content of main active components in Eucommia ulmoides male flowers. Scientia Silvae Sinicae 53, 35–43. doi: 10.11707/j.1001-7488.20170205
Eilers, E. J., Kleine, S., Eckert, S., Waldherr, S., Müller, C. (2021). Flower production, headspace volatiles, pollen nutrients, and florivory in Tanacetum vulgare chemotypes. Front. Plant Sci. 11. doi: 10.3389/fpls.2020.611877
Fernie, A. R., Trethewey, R. N., Krotzky, A. J., Willmitzer, L. (2004). Metabolite profiling: from diagnostics to systems biology. Nat. Rev. Mol. Cell Biol. 5, 763–769. doi: 10.1038/nrm1451
Gong, M., Su, C. F., Fan, M. Z., Wang, P., Cui, B. D., Guo, Z. Y., et al. (2021). Mechanism by which Eucommia ulmoides leaves RegulateNonalcoholic fatty liver disease based on system pharmacology. J. Ethnopharmacol. 282, 114603. doi: 10.1016/j.jep.2021.114603
He, X. C., Wang, S. Y., Shi, J. Y., Sun, Z. L., Lei, Z. T., Yin, Z. L., et al. (2018). Genotypic and environmental effects on the volatile chemotype of Valeriana jatamansi Jones. Front. Plant Sci. 9. doi: 10.3389/fpls.2018.01003
Hua, Y. L., Huang, L. Q., Chen, M. L., Xiao, P. G. (2009). Discussing on significance, position and classification standard of chemotype of medicinal plants. China J. Chin. mater. Med. 34, 924–928.
Jin, D., Henry, P., Shan, J., Chen, J. (2021). Identification of chemotypic markers in three chemotype categories of cannabis using secondary metabolites profiled in inflorescences, leaves, stem bark, and roots. Front. Plant Sci. 12. doi: 10.3389/fpls.2021.699530
Lai, Z., Tsugawa, H., Wohlgemuth, G., Mehta, S., Mueller, M., Zheng, Y., et al. (2018). Identifying metabolites by integrating metabolome databases with mass spectrometry cheminformatics. Nat. Methods 15, 53–5+. doi: 10.1038/nmeth.4512
Li, Y. K., Fang, J. B., Qi, X. J., Lin, M. M., Zhong, Y. P., Sun, L. M., et al. (2018b). Combined analysis of the fruit metabolome and transcriptome reveals candidate genes involved in flavonoid biosynthesis in Actinidia arguta. Int. J. Mol. Sci. 19, 1471. doi: 10.3390/ijms19051471
Li, L., Liu, M. H., Shi, K., Yu, Z. J., Zhou, Y., Fan, R. S., et al. (2019). Dynamic changes in metabolite accumulation and the transcriptome during leaf growth and development in Eucommia ulmoides. Int. J. Mol. Sci. 20, 4030. doi: 10.3390/ijms20164030
Li, H. Y., Lv, Q. Y., Liu, A., Wang, J. R., Sun, X. Q., Deng, J., et al. (2022). Comparative metabolomics study of tartary (Fagopyrum tataricum (L.) gaertn) and common (Fagopyrum esculentum moench) buckwheat seeds. Food Chem. 371, 131125. doi: 10.1016/j.foodchem.2021.131125
Liu, P. F., Wang, L., Du, Q. X., Du, H. Y. (2020). Chemotype classification and biomarker screening of male Eucommia ulmoides oliv. flower core collections using UPLC-QTOF/MS-based non-targeted metabolomics. PeerJ 8, e9786. doi: 10.7717/peerj.9786
Liu, C. L., Wang, L., Lu, W. J., Zhong, J., Du, H. Y., Liu, P. F., et al. (2022). Construction of SNP-based high-density genetic map using genotyping by sequencing (GBS) and QTL analysis of growth traits in Eucommia ulmoides oliv. Forests 13, 1479. doi: 10.3390/f13091479
Li, H. G., Xu, J. H., Wuyun, T. N., Liu, P. F., Du, Q. X., Du, H. Y. (2018a). Preliminary construction of core collection of Eucommia ulmoides based on allele number maximization strategy. Scientia Silvae Sinicae 54, 42–51. doi: 10.11707/j.1001-7488.20180205
Luan, H. M., Ji, F. F., Chen, Y., Cai, Z. W. (2018). statTarget: A streamlined tool for signal drift correction and interpretations of quantitative mass spectrometry-based omics data. Anal. Chimica Acta 1036, 66–72. doi: 10.1016/j.aca.2018.08.002
Rovesti, P. (1957). Essential oils of some chemotypes of aromatic eritrean labiates. Pharmaceutisch. weekblad. 92, 843–845.
Saccenti, E., Hoefsloot, H. C. J., Smilde, A. K., Westerhuis, J. A., Hendriks, M. M. W. B. (2014). Reflections on univariate and multivariate analysis of metabolomics data. Metabolomics 10, 361–374. doi: 10.1007/s11306-013-0598-6
Saito, K. (2013). Phytochemical genomics–a new trend. Curr. Opin. Plant Biol. 16, 373–380. doi: 10.1016/j.pbi.2013.04.001
Shen, X. T., Wang, R. H., Xiong, X., Yin, Y. D., Cai, Y. P., Ma, Z. J., et al. (2019). Metabolic reaction network-based recursive metabolite annotation for untargeted metabolomics. Nat. Commun. 10, 1516. doi: 10.1038/s41467-019-09550-x
Shepherd, L. V. T., Mcnicol, J. W., Razzo, R., Taylor, M. A., Davies, H. V. (2006). Assessing the potential for unintended effects in genetically modified potatoes perturbed in metabolic and developmental processes. targeted analysis of key nutrients and anti-nutrients. Transgenic Res. 15, 409–425. doi: 10.1007/s11248-006-0012-5
Shi, T. T., Zhu, A. T., Jia, J. Q., Hu, X., Chen, J., Liu, W., et al. (2020). Metabolomics analysis and metabolite-agronomic trait associations using kernels of wheat (Triticum aestivum) recombinant inbred lines. Plant J. 103, 279–292. doi: 10.1111/tpj.14727
Subbaraj, A. K., Huege, J., Fraser, K., Cao, M. S., Rasmussen, S., Faville, M., et al. (2019). A large-scale metabolomics study to harness chemical diversity and explore biochemical mechanisms in ryegrass. Commun. Biol. 2, 87. doi: 10.1038/s42003-019-0289-6
Triba, M. N., Le Moyec, L., Amathieu, R., Goossens, C., Bouchemal, N., Nahon, P., et al. (2015). PLS/OPLS models in metabolomics: The impact of permutation of dataset rows on the K-fold cross-validation quality parameters. Mol. Biosyst. 11, 13–19. doi: 10.1039/C4MB00414K
Tsugawa, H., Cajka, T., Kind, T., Yan, M., Higgins, B., Ikeda, K., et al. (2015). MS-DIAL: data-independent MS/MS deconvolution for comprehensive metabolome analysis. Nat. Methods 12, 523–526. doi: 10.1038/nmeth.3393
Wang, S. C., Alseekh, S., Fernie, A. R., Luo, J. (2019a). The structure and function of major plant metabolite modifications. Mol. Plant 12, 899–919. doi: 10.1016/j.molp.2019.06.001
Wang, C. Y., Tang, L., He, J. W., Li, J., Wang, Y. Z. (2019b). Ethnobotany, phytochemistry and pharmacological properties of Eucommia ulmoides: A review. Am. J. Chin. Med. 47, 259–300. doi: 10.1142/S0192415X19500137
Weckwerth, W., Wenzel, K., Fiehn, O. (2004). Process for the integrated extraction, identification and quantification of metabolites, proteins and RNA to reveal their co-regulation in biochemical networks. Proteomics 4, 78–83. doi: 10.1002/pmic.200200500
Wei, J. L., Wang, A. G., Li, R. D., Qu, H., Jia, Z. Y. (2017). Metabolome-wide association studies for agronomic traits of rice. Heredity 120, 342–355. doi: 10.1038/s41437-017-0032-3
Wu, M. Q., Chen, S. L., Wang, M. X., Yan, S. Z. (2011). An analysis of the genetic diversity and genetic structure of Eucommia ulmoides using inter-simple sequence repeat (ISSR) markers. Afr. J. Biotechnol. 10, 19505–19513. doi: 10.5897/AJB11.1715
Wuyun, T. N., Wang, L., Liu, H. M., Wang, X. W., Zhang, L. S., Bennetzen, J. L., et al. (2018). The hardy rubber tree genome provides insights into the evolution of polyisoprene biosynthesis. Mol. Plant 11, 429–442. doi: 10.1016/j.molp.2017.11.014
Xu, Y., Xu, C., Xu, S. (2017). Prediction and association mapping of agronomic traits in maize using multiple omic data. Heredity 119, 174–184. doi: 10.1038/hdy.2017.27
Yang, W., Su, Y., Dong, G. Q., Qian, G. T., Shi, Y. H., Mi, Y. L., et al. (2020). Liquid chromatography–mass spectrometry-based metabolomics analysis of flavonoids and anthraquinones in Fagopyrum tataricum l. gaertn. (Tartary buckwheat) seeds to trace morphological variations. Food Chem. 331, 127354. doi: 10.1016/j.foodchem.2020.127354
Yang, H., Xu, Z., Li, X. Q., Tan, S. M., Cheng, Z., Leng, X. J. (2021). Influences of dietary Eucommia ulmoides extract on growth, flesh quality, antioxidant capacity and collagen-related genes expression in grass carp (Ctenopharyngodon idellus). Anim. Feed Sci. Technol. 277, 114965. doi: 10.1016/j.anifeedsci.2021.114965
Yao, X. H., Deng, J. Y., Huang, H. W. (2012). Genetic diversity in Eucommia ulmoides (Eucommiaceae), an endangered traditional Chinese medicinal plant. Conserv. Genet. 13, 1499–1507. doi: 10.1007/s10592-012-0393-3
Yu, J., Wang, Y., Peng, L., Ru, M., Liang, Z. S. (2015). Genetic diversity and population structure of Eucommia ulmoides Oliver, an endangered medicinal plant in China. Genet. Mol. Res. 14, 2471–2483. doi: 10.4238/2015.March.30.5
Yu, X. M., Xiao, J. J., Chen, S., Yu, Y., Ma, J. Q., Lin, Y. Z., et al. (2020). Metabolite signatures of diverse camellia sinensis tea populations. Nat. Commun. 11, 5586. doi: 10.1038/s41467-020-19441-1
Keywords: self-organizing map, random forest, non-targeted metabolomics, chemotype classification, marker screening
Citation: Meng Y, Du Q, Du H, Wang Q, Wang L, Du L and Liu P (2023) Analysis of chemotypes and their markers in leaves of core collections of Eucommia ulmoides using metabolomics. Front. Plant Sci. 13:1029907. doi: 10.3389/fpls.2022.1029907
Received: 28 August 2022; Accepted: 02 December 2022;
Published: 09 January 2023.
Edited by:
Zhentian Lei, University of Missouri, United StatesReviewed by:
Khanh-Van Ho, University of Missouri, United StatesAnil Bhatia, University of California, Riverside, United States
Copyright © 2023 Meng, Du, Du, Wang, Wang, Du and Liu. This is an open-access article distributed under the terms of the Creative Commons Attribution License (CC BY). The use, distribution or reproduction in other forums is permitted, provided the original author(s) and the copyright owner(s) are credited and that the original publication in this journal is cited, in accordance with accepted academic practice. No use, distribution or reproduction is permitted which does not comply with these terms.
*Correspondence: Panfeng Liu, cGZlbmdsaXVAY2FmLmFjLmNu
†These authors have contributed equally to this work and share first authorship