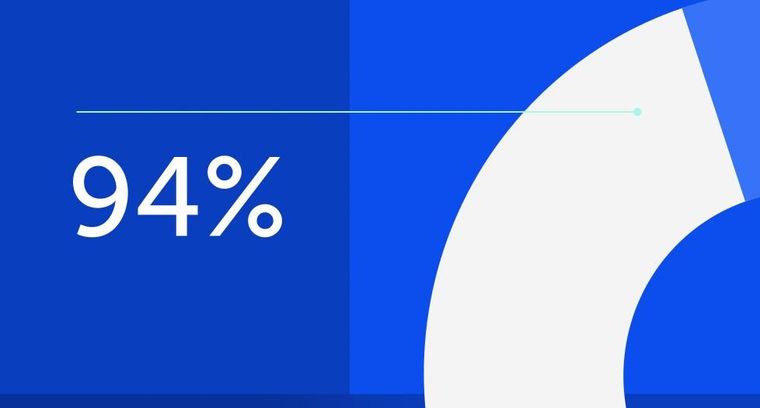
94% of researchers rate our articles as excellent or good
Learn more about the work of our research integrity team to safeguard the quality of each article we publish.
Find out more
ORIGINAL RESEARCH article
Front. Plant Sci., 09 November 2022
Sec. Functional Plant Ecology
Volume 13 - 2022 | https://doi.org/10.3389/fpls.2022.1026731
This article is part of the Research TopicSustainable Grasslands: Management Options for Ecosystem Functioning and ServiceView all 23 articles
Ecological stability contains multiple components, such as temporal invariability, resistance and resilience. Understanding the response of stability components to perturbations is beneficial for optimizing the management of biodiversity and ecosystem functioning. Although previous studies have investigated the effects of multiple perturbations on each stability component, few studies simultaneously measure the multiple stability components and their relationships. Alpine grasslands on the Tibetan Plateau are exposed to co-occurring perturbations, including climate change and human activities. Here, we quantified three stability components (temporal invariability, resistance, and resilience) of alpine grasslands on the Tibetan Plateau during periods of high (2000-2008) and low (2009-2017) human activity intensity, respectively. We focused on the effects of climate variables (temperature, precipitation, radiation) and human activities (grazing intensity) on covariation among stability components. The results show that (1) for periods of high and low human activity, temporal invariability was positively correlated with resistance and resilience, while resistance was independent of resilience; (2) the dimensionality of alpine grasslands decreased by almost 10%, from 0.61 in the first period to 0.55 in the second period, suggesting the increasing connections among temporal invariability, resistance and resilience of alpine grasslands; and (3) temperature but not grazing intensity dominated the changes in the dimensionality of stability. These findings improve our understanding of multi-dimensional stability and highlight the importance of climate variability on alpine grassland stability on the Tibetan Plateau.
Climate change and human activity are increasingly affecting the ecological processes of terrestrial ecosystems (IPCC, 2014; Yang et al., 2017b). Ecosystem stability becomes the core of understanding the ecosystems’ ability to maintain or recover their structure and functions under global change (Bai et al., 2004; Pennekamp et al., 2018; Radchuk et al., 2019). In arid and semiarid regions, biodiversity loss and productivity reduction due to the unsustainable land use and drastic climate change have weakened grassland stability strongly (Hooper et al., 2012; Haddad et al., 2015). There is a consensus that intense stressors may transit grassland to a catastrophic state until grassland stability is completely lost (Qin et al., 2019; Song et al., 2020). At the plot level, mounting empirical studies have investigated the underlying mechanisms by which grassland ecosystems maintain relatively stable levels to avoid catastrophic transition at the plot scale (Grman et al., 2010; Ma et al., 2017; Saruul et al., 2019). However, although the impacts of climate change and human activities on ecosystem functions have been studied globally, little research has assessed the ecosystem stability in response to these perturbations in a large scale.
The satellite-derived vegetation indices, such as normalized difference vegetation index (NDVI) and Enhanced Vegetation Index (EVI), are directly associated with vegetation activity and have been widely used to investigate the responses of ecosystem productivity to perturbation (Del Grosso et al., 2018; Lu et al., 2019). From NDVI or EVI anomalies in time series, previous studies have successfully extracted different large-scale ecosystem stability components, such as temporal invariability, resistance and resilience (De Keersmaecker et al., 2015; Li et al., 2020; White et al., 2020). Temporal invariability measures the fluctuations of community biomass production over time, resistance quantifies ecosystems’ ability to absorb perturbations, and resilience reflects the capacity of a disturbed ecosystem recovering to its original state (Pimm, 1984; White et al., 2020). These stability components are usually correlated each other (Pennekamp et al., 2018; Kefi et al., 2019). For example, resistance is expected to correlate positively with temporal invariability but negatively with resilience (Li et al., 2020; Chen et al., 2021). Donohue et al. (2013) argued that correlations among stability components construct the dimensionality of ecosystem stability which contains abundant information for predicting the response of ecosystems to environmental change. Strong correlations among stability components can shape a low effective dimensionality of stability, implying similar processes and maintenance mechanisms underlie ecosystem stability components. Therefore, the monitoring schemes can be optimized by quantifying only a few stability components when the dimensionality of stability is low (Radchuk et al., 2019). In contrast, if these stability components are uncorrelated, ecosystem stability will shape a high dimensionality, suggesting that ecosystems may respond to perturbations in many compensatory ways. In this case, policymakers should measure all stability components to manage natural systems (Donohue et al., 2013; Radchuk et al., 2019).
Theoretically, ecosystems generally keep a relatively stable level in fluctuating environmental conditions. However, large or high-frequency perturbations tend to prompt ecosystems in a highly variable state (Pimm, 1984). On the one hand, rapid climate change, e.g., global warming accompanied by precipitation patterns change, can shift community composition and influence species interactions, thus changing ecosystem stability (Crossman et al., 2012; Ma et al., 2017; Antão et al., 2022). On the other hand, anthropogenic perturbations that affect species diversity and their interactions may therefore decrease the ability of an ecosystem to adapt to or resist environmental changes (Tilman and Lehman, 2001; Venter et al., 2016). For example, overgrazing can typically shift dominant species and change functional traits of persistent species, further reducing biomass stability (Grice et al., 2008; Wu et al., 2013). The intermediate disturbance hypothesis supported that moderate grazing can reorganize the spatial pattern of plants and nutrients to optimize utilization of the limiting resources, such as water and light, ultimately enhancing plants’ ability to resist perturbations (Song et al., 2020). Therefore, investigating the coupling effects of climate change and human activity on ecosystem stability help understand how ecosystems respond to perturbations.
Alpine grassland on the Tibetan Plateau is one of the fragile ecosystems on Earth and is crucial for maintaining the social economy’s sustainable development and the plateau’s ecological balance (Yao et al., 2012; Yang et al., 2017a). Over the last several decades, this plateau has experienced rapid and general warming and a tremendous increase in livestock numbers (Chen et al., 2013; Cao et al., 2019). These perturbations have caused extensive grassland degradation across the plateau (Harris, 2010). Since 2000, various ecological protection policies have been continuously renewed and implemented to protect and restore degraded grasslands on the plateau. After 2009, human activity intensity on alpine grasslands declined significantly, gradually shifting the ecological environment in a positive direction (Li et al., 2021b). Although many studies have assessed the response of grassland productivity to these policies and climate change, how grassland stability responds to these perturbations is still unknown. In this study, based on the inter-annual dynamic of grassland NDVI and its corresponding climate variables, i.e., temperature, precipitation and radiation, we first calculated three stability components of temporal invariability, resistance, and resilience at the regional scale. Then, we quantified the dimensionality of stability based on correlations among the three stability components. Finally, we compared the difference in stability components between the two periods to clarify the relative contribution of climate variables (refers to temperature, precipitation and radiation) and human activities (refers to grazing intensity) to grassland stability changes. This study aims (a) to investigate the changes in grassland stability components and their relationships, and (b) to explore the primary factor in regulating grassland stability on the Tibetan Plateau.
The Tibetan Plateau in China starts from the foot of the Himalayas in the south, and reaches the foot of the Kunlun Mountains and the Qilian Mountains in the north. This plateau occupies around one-quarter of surface areas in China, with an average altitude of 4,000 m above sea level. Known as the Asian Water Tower, this region is the headwaters of the most important Asian rivers, such as the Yellow River, Yangtze River, Mekong River and Brahmaputra River, and thus plays an essential role in maintaining biodiversity and biogeochemical cycles of its surrounding countries (Yao et al., 2012). Alpine grassland, the most distributed vegetation type, is the critical animal husbandry base and ecological barrier of the Tibetan Plateau (Long et al., 1999). Xizang Autonomous Region and Qinghai province are the major section of the Tibetan Plateau, separated by the Tanggula Mountains (Figure 1).
We downloaded Normalized Difference Vegetation Index (NDVI) from NASA’s moderateresolution imaging spectroradiometer (MODIS) (MOD13A3) from 2000 to 2017. This dataset was produced at a spatial resolution of 1,000 m and a time step of one month. The noises in time-series NDVI data, such as atmospheric effects and cloud contamination, were calibrated based on the Savitzky–Golay method (Chen et al., 2004). Previous studies suggest that the low quality of NDVI data for sparsely vegetated lands on the Tibetan Plateau may affect further analyses of ecosystem processes (Zu et al., 2018; Hua et al., 2022a). Thus, any pixel with annual NDVI lower than 0.1 was eliminated to reduce the potential effects of noisy data. The daily meteorological data, including temperature, precipitation and sunshine hours, were collected from the National Meteorological Information Center of China Meteorological Administration. We first aggregated daily meteorological data to monthly level to match the temporal resolution of the NDVI data. Then, we used ANUSPLIN 4.3 to interpolate monthly climatic records into raster surfaces with a 1,000 m resolution (Hutchinson, 2004). Solar radiation was calculated based on sunshine hours with the FAO procedure (Allen et al., 1998). The quality of grid climatic surfaces has been demonstrated by the high correlations to field observation records (Chen et al., 2014; Tao et al., 2015). Information on the distribution of grasslands was obtained from the vegetation atlas of China with a scale of 1:1000 000 (Editorial Committee for Vegetation Map of China, 2001). Livestock records for each county on the Tibetan Plateau, including sheep, goat, yak, donkey, and horse, were downloaded from statistical yearbooks (https://kns.cnki.net/kns8?dbcode=CYFD).
In this study, we measured the grazing intensity (GI) for each county on the Tibetan Plateau. We first converted livestock numbers to standard sheep units (SHU) to ensure comparability between different counties. As suggested by previous studies, one sheep or one goat equals one SHU, and one yak, one donkey, or one horse equals four SHUs (Li et al., 2021a; Yu et al., 2021). Then, we extracted the area of available natural grassland (ha) in each county from the information of grassland distribution. Finally, we calcluated the GI as the ratio of livestock number to natural grassland areas.
In this study, we calculated three stability components, including temporal invariability, resistance, and resilience, based on the temporal NDVI and climatic variables. Prior to analysis, all-time series of NDVI and the three climate variables were detrended using a linear regression model. Temporal invariability was computed as the ratio of long-term mean of a NDVI value to its standard deviation (Deléglise et al., 2011; Ma et al., 2017). Resistance and resilience were quantified through the relationships between NDVI and climate variables, as did in previous studies (De Keersmaecker et al., 2015; Seddon et al., 2016; Li et al., 2018). A linear autoregressive model was used to build the relationship between NDVI anomaly and climate anomaly (temperature, precipitation and solar radiation). In this model, all variables were transformed to z-score anomalies using variables’ means and standard deviations. The coefficients of the linear model were used to measure ecosystem resistance and resilience.
where NDVIt is the NDVI anomaly at time t; Tt, Pt and Rt are the corresponding temperature, precipitation and radiation anomaly, respectively; NDVIt-1 is the NDVI anomaly at time t-1, and ϵ is the residual error. α, β and γ are temperature resistance metric, drought resistance metric and radiation resistance metric, which can be used to calculate the ecosystem’s resistance to climate change. δ indicates the similarity between the current state and the previous state, which can be considered a resilience metric. A higher absolute δ value represents lower resilience (De Keersmaecker et al., 2015; Li et al., 2018). More detailed information for calculating ecosystem resistance and resilience can be found in Li et al. (2018). Finally, temporal invariability, resistance and resilience of grassland ecosystems were normalized between 0 and 100 using the minimum and maximum values.
We used the space-for-time substitution to calculate the correlations among stability components. Firstly, we coarsened gridding cells of alpine grassland to the administration unit based on the boundary of 122 counties (districts) on the Tibetan Plateau. Then, we divided grassland cells in each county(district) into different grassland types. We finally generated 206 spatial datasets to analyze the correlations among invariability, resistance and resilience.
For each spatial dataset, we calculated and tested Pearson correlations between pairs of the three stability variables and constructed covariance matrices with these Pearson correlations to measure the dimensionality of stability. The dimensionality of stability represents the strength of the relationship among various stability components (Donohue et al., 2013). The magnitude of dimensionality is equal to the volume of the ellipsoid (V), which is constructed by covariance matrices (Donohue et al., 2013):
where ai is the semi-axis length of the ellipsoid, V is the volume of the ellipsoid, n is the dimensionality of the covariance matrix, λi is the ith eigenvalue of the covariance matrix. For each covariance matrix, semi-axis lengths are normalized by dividing the maximum length among all the semi-axis lengths so that the maximum standardized length equaled 1. Thus, proportional volume can be normalized by dividing the actual ellipsoid volume by the theoretical maximum between 0 (low dimensionality, a ‘cigar’-like shape of ellipsoids) and 1 (high dimensionality, a perfect sphere). If the dimensionality is high, the stability components are independent (the relationship among various stability components is poor), and vice versa.
Pearson correlation analysis was performed to examine the pairwise correlations among stability components. One-way analysis of variance (ANOVA) was used to examine the differences in stability between different types. We employed random forest algorithm with the randomForest package in R 4.1.2 to evaluate the relative contribution of climate variables and grazing intensity to variation in grassland stability. Random forest algorithm is a high accuracy and robust machine learning algorithm, which is suitable for processing high-dimensional and -correlated datasets (Tin Kam, 1998; Breiman, 2001; Xia et al., 2018). In the random forest model, we selected the trends in MAT, AP, AR, and GI (TrendMAT, TrendAP, TrendAR and TrendGI, respectively) as predictors because they are closely correlated with ecosystem stability and are widely used in determining the driving mechanisms of vegetation change (Wessels et al., 2004; Mariano et al., 2018; Li et al., 2021b). The mean square error (%IncMSE) of each predictor in the random forest model represents variable importance, and higher %IncMSE values indicate higher variable importance.
The spatial patterns of temporal invariability, resistance and resilience of alpine grassland ecosystems were consistent in the two periods: 2000–2008 and 2009–2017 (Figure 2). Temporal invariability and resistance showed a clear spatial heterogeneity pattern, but the resilience showed a relative homogeneity pattern across the plateau. Especially, grasslands with high temporal invariability (> 80) occupying less than 10% of grassland areas on the Tibetan Plateau, mainly distributed in the northwest areas. The spatial pattern of resistance was partly consistent with invariability, indicating that productivity in northwestern grassland tended to be more resistant to disturbances than in other regions. Grasslands with low resilience (< 20) only accounted for less than 3% of the total grassland area, and mainly distributed in the northeast of the plateau.
Figure 2 Spatial patterns of grassland standardized temporal invariability (A for 2000-2008, B for 2009-2017), resistance (C for 2000-2008, D for 2009-2017), and resilience (E for 2000-2008, F for 2009-2017) on the Tibetan Plateau.
Regarding different grassland types on the Tibetan Plateau, alpine steppe had the highest temporal invariability, followed by the alpine meadow and desert steppe. Resistance in alpine steppe was significantly higher than the other two grassland types in the second period (P< 0.05) but was similar to that in alpine meadow in the first period (P > 0.05). The resilience was the highest in the alpine meadow, and lowest in the desert steppe (Table 1).
Table 1 Comparisons of means ( ± standard deviation) of grassland invariability, resistance, and resilience among different grassland types on the Tibetan Plateau.
Temporal invariability and resistance showed a positive correlation for more than 90% of grassland areas on the Tibetan Plateau (RInv-Rst > 0), especially for southern areas where grasslands had a value of RInv-Rst higher than 0.4. Again, the correlation between invariability and resilience was positive (RInv-Rsl > 0) for most grassland areas. However, the correlation between resistance and resilience (RRst-Rsl) showed a heterogeneous pattern, that is, RRst-Rsl was negative in eastern grasslands but positive in western grasslands (Figure 3).
Figure 3 Spatial patterns of pairwise correlations among stability properties during (A-C) 2000-2008, (D-F) 2009-2017 and (G-I) the difference in the corresponding correlation coefficients (ΔR) between the two periods on the Tibetan Plateau. RInv-Rst, RInv-Rsl and RRst-Rsl respersent the correlation coefficients between temporal invariability and resistance, temporal invariability and resilience, and resistance and resilience, respectively.
Although there are no significant changes in the spatial patterns of RInv-Rst, RInv-Rsl and RRst-Rsl between the two periods, the magnitude of correlations among stability components has changed in the second period, compared to the first period. Especially, the average RInv-Rst and RInv-Rsl increased from 0.28 and 0.09 of the first period to 0.36 and 0.14 of the second period, respectively. However, the RRst-Rsl transformed from positive (0.01) of the first period to negative (-0.01) of the second period. For different grassland types, RInv-Rst increased in alpine meadow, alpine steppe and desert steppe, and RInv-Rsl increased in all grassland types with the exception of the desert steppe. Besides, compared with that in the first period, RRst-Rsl in all three grassland types decreased slightly in the second period (Figure 4).
Figure 4 The pairwise correlation among stability properties for each grassland type in 2000-2008 and 2009-2017. TP represents the Tibetan Plateau; AM, AS and DS represent alpine meadow, alpine steppe and alpine desert steppe, respectively. RInv-Rst, RInv-Rsl and RRst-Rsl represent the correlation coefficients between temporal invariability and resistance, temporal invariability and resilience, and resistance and resilience, respectively.
The spatial pattern of dimensionality of stability was heterogeneous. The value of dimensionality, either in the first or second period, was high in the two sides of the Tibetan Plateau and low in the middle of this plateau (Figures 5A, B). For the entire plateau, the dimensionality of alpine grasslands decreased by almost 10%, from 0.61 in the first period to 0.55 in the second period. This decrease in dimensionality mostly occurred in the southwest of the Xizang Autonomous Region and the center of the Qinghai province, where the dimensionality decreased by more than 20% (Figure 5C). Meanwhile, we also observed a slight increase in the dimensionality in the northern Xizang Autonomous Region and northeastern Qinghai province. Statistically, the dimensionality of stability decreased in 72.7% of the study area, 26.3% of which decreased with a magnitude of more than 20%. In terms of different grassland types, the dimensionality in the alpine meadow was the highest, followed by alpine steppe and desert steppe. Comparing the two periods, we found that the dimensionality in the alpine meadow decreased from 0.64 in the first period to 0.57 in the second period. Besides, the dimensionality in the alpine steppe decreased from the first period (0.59) to the second period (0.53). In contrast, the dimensionality in desert steppe showed little increase between the two periods (Figure 5D).
Figure 5 The spatial pattern of dimensionality of stability on the Tibetan Plateau: (A, B) dimensionality during 2000-2008 and 2009-2017, (C) the difference in dimensionality between the two periods, and (D) the boxplot of dimensionality for different grassland types.
Figure 6 presents the relationships of difference in dimensionality (Δdimensionality) with differences in three climatic variables trend (ΔTrendMAT, ΔTrendAP, and ΔTrendAR) and grazing intensity trend (ΔTrendGI) between the two time periods. Δdimensionality significantly increased with increasing ΔTrendMAT, ΔTrendAR and ΔTrendGI, but decreased with increasing ΔTrendAP.
Figure 6 Relationships of the difference in dimensionality of stability with corresponding mean-differences in (A) mean annual temperature (MAT), (B) annual precipitation (AP), (C) annual radiation (AR), and (D) grazing intensity (GI).
RF model was conducted to measure the relative contribution of ΔTrendMAT, ΔTrendAP, and ΔTrendAR, and ΔTrendGI to the differences of alpine grassland dimensionality. Results of the RF model show that the simulated Δdimensionality could explain 89.6% variation in observed Δdimensionality (Figure 7A). Among climate variables and grazing intensity, ΔTrendMAT contributed the most variations of dimensionality (%IncMSE=21.5%), followed by ΔTrendAR (%IncMSE=11.1%). ΔTrendAP (%IncMSE=6.9%) and ΔTrendGI (%IncMSE=6.4%) were the third and last important variables to Δdimensionality (Figure 7B).
Figure 7 The performances of the RF model: (A) the relationship of observed differences dimensionality with simulated differences dimensionality during 2000–2008 and 2009–2017 (the grey line is the 1:1 line; the blue line is the regression line), and (B) relative contribution of predicators in RF model denoted by the percentage increase of mean squared error (%IncMSE).
Temporal invariability, resistance and resilience describe different aspects of ecosystem stability in response to perturbations. Radchuk et al. (2019) suggest that the positive correlations among stability components are more beneficial to maintain a stable state of an ecosystem. For alpine grassland on the Tibetan Plateau, we find that some stability components were strongly correlated while others were uncorrelated. Especially, temporal invariability was positively correlated with resistance and resilience, while resistance was independent of resilience, indicating that ecological stability of alpine grasslands appeared to be complex. This result was in line with the findings of Donohue et al. (2013) and Radchuk et al. (2019). They suggest that one single stability component cannot capture the overall stability of an ecosystem. The high correlation between temporal invariability and resistance can be explained by the fact that both the two stability components reflect the ability of an ecosystem to maintain its initial state (Hoover et al., 2014; Zhong et al., 2019). The weak correlation between resistance and resilience did not agree with theoretical research that resistance may negatively correlate with resilience (Donohue et al., 2013), and also did not agree with Isbell et al. (2015) who find that biodiversity increase resistance but decrease resilience to climate extremes in grassland ecosystems. However, some experimental studies point out that ecosystems tend to optimize resistance-related functional traits rather than resilience-related functional traits to stabilize their functions, leading to the weak connection between resistance and resilience (Hoover et al., 2014; Baert et al., 2016). The lack of correlations between resistance and resilience suggest that it is necessary to measure the two stability components simultaneously on the Tibetan Plateau to enrich understanding of the overall ecosystem stability.
The dimensionality of stability captures multi aspects of stability independently. High dimensionality indicates weak connections among stability components, and vice versa (Donohue et al., 2013). If the dimensionality is high, policymakers should maximize one stability component without affecting other stability’s aspects (Polazzo and Rico, 2021). The research of Li et al. (2020) and Chen et al. (2021) confirm that the dimensionality of stability varies among biomes due to the differences in community characteristics and environmental conditions. In this study, we find alpine meadow had a higher dimensionality than alpine steppe and desert steppe on the Tibetan Plateau, implying that complex processes and regulatory mechanisms underlie the stability components of alpine meadow. The potential explanation is that alpine meadow has higher species richness and more complex community structure, leading to a complicated response of ecosystem stability to perturbations. According to the relationship between species richness and the dimensionality of stability, we find that alpine grasslands with higher species richness tended to shape lower dimensionality of stability (Figure 8). Although the large local species pool benefits grassland self-restoration on the Tibetan Plateau (Wu et al., 2017), strong competitive interactions among species usually result in weaker connection among stability components, thus generating higher dimensionality (Radchuk et al., 2019). Therefore, more stability components should be measured simultaneously to monitor and manage grassland dynamics in alpine meadows on the Tibetan Plateau.
Figure 8 Relationships between native species richness and the dimensionality of stability at the two periods on the Tibetan Plateau. The species richness data of this plateau was extracted from the global data of vascular plant species richness (https://doi.org/10.1371/journal.pone.0030535) (Moen et al., 2012).
Although previous studies have explored the effect of perturbations on each single stability component (Fussmann et al., 2014; Oliver et al., 2015; Ma et al., 2017), it is still not clear how perturbation affects the relationships among stability components. In this study, we show that climate variables (temperature, precipitation and radiation) and grazing have changed the magnitude of grassland stability components and their relationships. The dimensionality of stability decreased from 0.61 during 2000-2008 to 0.55 during 2009-2017, suggesting the increasing connections among temporal invariability, resistance and resilience of alpine grasslands. Previous results find that the dimensionality of stability depends on perturbation type (Radchuk et al., 2019). Hence, quantifying the relative importance of multiple perturbations on the dimensionality of stability is of great significance in diagnosing regional environmental problems and protecting critical regional ecosystems.
Both climate change and grazing activity, the major perturbations for the dynamic of grassland ecosystems on the Tibetan Plateau, significantly affected the dimensionality of stability. Although some studies disentangle the relative contributions of different perturbations to the dynamics of alpine grassland, the primary driver is still controversial. For example, Zeng et al. (2019) report that temperature has the highest contribution to the aboveground biomass variation. Zhang et al. (2016) also find that alpine grassland growth is strongly correlated with temperature. However, some studies also find that precipitation rather than warming is the primary determinant factor of plant productivity on the Tibetan Plateau (Fu et al., 2018; Yu et al., 2021). In our study, we find that temperature was the controlling factor for decreasing dimensionality on this plateau. This result is in line with the findings of a meta-analysis, in which Valencia et al. (2020) detect a significant effect of temperature on plant community stability worldwide. Climate warming on the Tibetan Plateau was faster than the global average over the past decades. However, the current air temperature is still lower than the optimum physiological temperature of leaf-level photosynthetic capacity (Huang et al., 2019). Thus, warming affects photosynthesis, community structure and species interactions of alpine grasslands significantly, and further regulates grassland stability (Zhu et al., 2016). Consequently, temperature become one of the critical explanatory climatic variables to the changes in the dimensionality of stability.
Previous studies suggest that the herbivores may decouple the relationships among temporal invariability, resistance and resilience, and thus lead to the high dimensionality of stability (Donohue et al., 2013; Chen et al., 2022). For example, Saruul et al. (2019) demonstrate that continuous grazing changes the interrelationships among stability components and further alter the dimensionality of stability. However, in our study, random forest modeling confirmed that grazing intensity explained the lowest variation of dimensionality. This finding is largely consistent with the results of Li et al. (2021a) that climate change rather than grazing activities dominate changes in grassland productivity on the Tibetan Plateau, and with the current prevailing views that climate plays a crucial role in ecosystem stability (Chen et al., 2021; Wang et al., 2022). Overgrazing or unsustainable livestock management might damage the structure and function of alpine grasslands, but such negative effects are only evident in hotspots directly adjacent to pastoralist camps (Wang et al., 2017). Thus, for the whole plateau, grazing activities might not change the dominant role of climate change for plant growth on the Tibetan Plateau.
We note that this study has two limitations for further improvement. Firstly, the calculation of resistance and resilience was based on the linear relationships between NDVI and climate variables (temperature, precipitation and radiation). This method does not incorporate human activity into consideration. Since 2000, various ecological protection policies have been renewed and implemented on the Tibetan Plateau, which have driven vegetation restoration (Hua et al., 2022b). Although climate change is the dominant factor in controlling the inter-annual dynamic of grassland productivity, ecological engineering projects may have more or less impact on the changes in vegetation productivity (Li et al., 2021a; Wang et al., 2022). In this case, we might have overestimated or underestimated the contributions of climate variables to the dynamics of NDVI. Thus, in the future, we call for more complementary studies to disentangle climatic and anthropogenic contributions to the variations of vegetation productivity. Secondly, the grazing intensity was quantified at coarse spatial resolution due to insufficient data on the spatial distribution of livestock. Recently, Sun et al. (2022) have rasterized the county-scale grazing data based on the NPP data and the statistical yearbook on the Tibetan Plateau. However, this dataset only covers four years of grazing intensity, which cannot meet the requirements of trend analysis. Therefore, it is essential to produce a dataset of grazing intensity with high quality and fine spatiotemporal resolution to improve the accuracy of spatial data analysis.
In this study, we use NDVI records and corresponding temperature, precipitation, and solar radiation to quantify three stability components (temporal invariability, resistance and resilience) of alpine grasslands and their relationships on the Tibetan Plateau. The findings confirm that the dimensionality of stability in the period of low human activity intensity is lower than that in the period of high human activity intensity, indicating the increasing connections among temporal invariability, resistance and resilience. Furthermore, we find that climate variability rather than grazing intensity regulates the changes in grassland stability.
The raw data supporting the conclusions of this article will be made available by the authors, without undue reservation. Requests to access the datasets should be directed to ML.
ML and FH conceptualized this study. YY collected and analyzed the data. ML and YY led the writing. BN, YS, YF, and YY interpreted the results and revised the text. All authors contributed to this work and approved the final manuscript before submission.
The study was supported by the Second Tibetan Plateau Scientific Expedition and Research (STEP) program (2019QZKK1002); the construction of the fixed Observation and Experimental Station of the first Support System for Agricultural Green Development in Zhongba County; the study on the path of agricultural green development and carbon reduction and sequestration in typical counties of Yarlung Zangbo River Basin; and National Natural Science Foundation of China (42207129).
The authors declare that the research was conducted in the absence of any commercial or financial relationships that could be construed as a potential conflict of interest.
The handling editor declared a past collaboration with the authors ML and YS.
All claims expressed in this article are solely those of the authors and do not necessarily represent those of their affiliated organizations, or those of the publisher, the editors and the reviewers. Any product that may be evaluated in this article, or claim that may be made by its manufacturer, is not guaranteed or endorsed by the publisher.
Allen, R., Pereira, L., Smith, M. (1998). Crop evapotranspiration-guidelines for computing crop water requirements. FAO irrigation and drainage Vol. 56 (Rome: FAO).
Antão, L. H., Weigel, B., Strona, G., Hällfors, M., Kaarlejärvi, E., Dallas, T., et al. (2022). Climate change reshuffles northern species within their niches. Nat. Climate Change 12, 587–592. doi: 10.1038/s41558-022-01381-x
Baert, J. M., De Laender, F., Sabbe, K., Janssen, C. R. (2016). Biodiversity increases functional and compositional resistance, but decreases resilience in phytoplankton communities. Ecology 97, 3433–3440. doi: 10.1002/ecy.1601
Bai, Y., Han, X., Wu, J., Chen, Z., Li, L. (2004). Ecosystem stability and compensatory effects in the inner Mongolia grassland. Nature 431, 181–184. doi: 10.1038/nature02850
Cao, Y., Wu, J., Zhang, X., Niu, B., Li, M., Zhang, Y., et al. (2019). Dynamic forage-livestock balance analysis in alpine grasslands on the northern Tibetan plateau. J. Environ. Manage. 238, 352–359. doi: 10.1016/j.jenvman.2019.03.010
Chen, J., Chi, Y., Zhou, W., Wang, Y., Zhuang, J., Zhao, N., et al. (2021). Quantifying the dimensionalities and drivers of ecosystem stability at global scale. J. Geophys. Res.: Biogeosci. 126, e2020JG006041. doi: 10.1029/2020JG006041
Chen, J., Jönsson, P., Tamura, M., Gu, Z., Matsushita, B., Eklundh, L. (2004). A simple method for reconstructing a high-quality NDVI time-series data set based on the savitzky–golay filter. Remote Sens. Environ. 91, 332–344. doi: 10.1016/j.rse.2004.03.014
Chen, Q. Q., Wang, S. P., Seabloom, E. W., MacDougall, A. S., Borer, E. T., Bakker, J. D., et al. (2022). Nutrients and herbivores impact grassland stability across spatial scales through different pathways. Global Change Biol. 28, 2678–2688. doi: 10.1111/gcb.16086
Chen, B., Zhang, X., Tao, J., Wu, J., Wang, J., Shi, P., et al. (2014). The impact of climate change and anthropogenic activities on alpine grassland over the qinghai-Tibet plateau. Agric. For. Meteorol. 189-190, 11–18. doi: 10.1016/j.agrformet.2014.01.002
Chen, H., Zhu, Q., Peng, C., Wu, N., Wang, Y., Fang, X., et al. (2013). The impacts of climate change and human activities on biogeochemical cycles on the qinghai-Tibetan plateau. Global Change Biol. 19, 2940–2955. doi: 10.1111/gcb.12277
Crossman, N. D., Bryan, B. A., Summers, D. M. (2012). Identifying priority areas for reducing species vulnerability to climate change. Diversity Distrib. 18, 60–72. doi: 10.1111/j.1472-4642.2011.00851.x
De Keersmaecker, W., Lhermitte, S., Tits, L., Honnay, O., Somers, B., Coppin, P. (2015). A model quantifying global vegetation resistance and resilience to short-term climate anomalies and their relationship with vegetation cover. Global Ecol. Biogeogr. 24, 539–548. doi: 10.1111/geb.12279
Deléglise, C., Loucougaray, G., Alard, D. (2011). Effects of grazing exclusion on the spatial variability of subalpine plant communities: A multiscale approach. Basic Appl. Ecol. 12, 609–619. doi: 10.1016/j.baae.2011.08.006
Del Grosso, S. J., Parton, W. J., Derner, J. D., Chen, M., Tucker, C. J. (2018). Simple models to predict grassland ecosystem c exchange and actual evapotranspiration using NDVI and environmental variables. Agric. For. Meteorol. 249, 1–10. doi: 10.1016/j.agrformet.2017.11.007
Donohue, I., Petchey, O. L., Montoya, J. M., Jackson, A. L., McNally, L., Viana, M., et al. (2013). On the dimensionality of ecological stability. Ecol. Lett. 16, 421–429. doi: 10.1111/ele.12086
Editorial Committee for Vegetation Map of China, 2001. Vegetation atlas of China.science press, Beijing (in Chinese).
Fu, G., Shen, Z.-X., Zhang, X.-Z. (2018). Increased precipitation has stronger effects on plant production of an alpine meadow than does experimental warming in the northern Tibetan plateau. Agric. For. Meteorol. 249, 11–21. doi: 10.1016/j.agrformet.2017.11.017
Fussmann, K. E., Schwarzmüller, F., Brose, U., Jousset, A., Rall, B. C. (2014). Ecological stability in response to warming. Nat. Climate Change 4, 206–210. doi: 10.1038/nclimate2134
Grice, A. C., Campbell, S., Breaden., R., Bebawi, F., Vogler, W. (2008). Rangelands: ecological principles for the strategic management of weeds in rangeland habitats (Adelaide, SA, Australia: CRC for Australian Weed Management).
Grman, E., Lau, J. A., Schoolmaster, J. D. R., Gross, K. L. (2010). Mechanisms contributing to stability in ecosystem function depend on the environmental context. Ecol. Lett. 13, 1400–1410. doi: 10.1111/j.1461-0248.2010.01533.x
Haddad, N. M., Brudvig, L. A., Clobert, J., Davies, K. F., Gonzalez, A., Holt, R. D., et al. (2015). Habitat fragmentation and its lasting impact on earth's ecosystems. Sci. Adv. 1, e1500052. doi: 10.1126/sciadv.1500052
Harris, R. B. (2010). Rangeland degradation on the qinghai-Tibetan plateau: A review of the evidence of its magnitude and causes. J. Arid Environ. 74, 1–12. doi: 10.1016/j.jaridenv.2009.06.014
Hooper, D. U., Adair, E. C., Cardinale, B. J., Byrnes, J. E. K., Hungate, B. A., Matulich, K. L., et al. (2012). A global synthesis reveals biodiversity loss as a major driver of ecosystem change. Nature 486, 105–108. doi: 10.1038/nature11118
Hoover, D. L., Knapp, A. K., Smith, M. D. (2014). Resistance and resilience of a grassland ecosystem to climate extremes. Ecology 95, 2646–2656. doi: 10.1890/13-2186.1
Huang, M. T., Piao, S. L., Ciais, P., Penuelas, J., Wang, X. H., Keenan, T. F., et al. (2019). Air temperature optima of vegetation productivity across global biomes. Nat. Ecol. Evol. 3, 772–779. doi: 10.1038/s41559-019-0838-x
Hua, T., Zhao, W., Cherubini, F., Hu, X., Pereira, P. (2022a). Effectiveness of protected areas edges on vegetation greenness, cover and productivity on the Tibetan plateau, China. Landscape Urban Plann. 224, 104421. doi: 10.1016/j.landurbplan.2022.104421
Hua, T., Zhao, W., Pereira, P. (2022b). Opinionated views on grassland restoration programs on the qinghai-Tibetan plateau. Front. Plant Sci. 13. doi: 10.3389/fpls.2022.861200
Hutchinson, M. (2004). Anusplin (Canberra, Australia: Centre for Resource and Environmental Studies. The Australian National University).
IPCC (2014). “Climate change 2014: Impacts, adaptation, and vulnerability. part a: Global and sectoral aspects,” in Contribution of working group II to the fifth assessment report of the international panel on climate change (Cambridge, United Kingdom and New York, NY, USA: Cambridge University Press).
Isbell, F., Craven, D., Connolly, J., Loreau, M., Schmid, B., Beierkuhnlein, C., et al. (2015). Biodiversity increases the resistance of ecosystem productivity to climate extremes. Nature 526, 574–577. doi: 10.1038/nature15374
Kefi, S., Dominguez-Garcia, V., Donohue, I., Fontaine, C., Thebault, E., Dakos, V. (2019). Advancing our understanding of ecological stability. Ecol. Lett. 22, 1349–1356. doi: 10.1111/ele.13340
Li, M., Wu, J., Feng, Y., Niu, B., He, Y., Zhang, X. (2021a). Climate variability rather than livestock grazing dominates changes in alpine grassland productivity across Tibet. Front. Ecol. Evol. 9. doi: 10.3389/fevo.2021.631024
Li, M., Wu, J., He, Y., Wu, L., Niu, B., Song, M., et al. (2020). Dimensionality of grassland stability shifts along with altitudes on the Tibetan plateau. Agric. For. Meteorol. 291, 108080. doi: 10.1016/j.agrformet.2020.108080
Li, D., Wu, S., Liu, L., Zhang, Y., Li, S. (2018). Vulnerability of the global terrestrial ecosystems to climate change. Global Change Biol. 24, 4095–4106. doi: 10.1111/gcb.14327
Li, M., Zhang, X., Wu, J., Ding, Q., Niu, B., He, Y. (2021b). Declining human activity intensity on alpine grasslands of the Tibetan plateau. J. Environ. Manage. 296, 113198. doi: 10.1016/j.jenvman.2021.113198
Long, R. J., Apori, S. O., Castro, F. B., Ørskov, E. R. (1999). Feed value of native forages of the Tibetan plateau of China. Anim. Feed Sci. Technol. 80, 101–113. doi: 10.1016/S0377-8401(99)00057-7
Lu, Q., Zhao, D. S., Wu, S. H., Dai, E. F., Gao, J. B. (2019). Using the NDVI to analyze trends and stability of grassland vegetation cover in inner Mongolia. Theor. Appl. Climatol. 135, 1629–1640. doi: 10.1007/s00704-018-2614-2
Ma, Z. Y., Liu, H. Y., Mi, Z. R., Zhang, Z. H., Wang, Y. H., Xu, W., et al. (2017). Climate warming reduces the temporal stability of plant community biomass production. Nat. Commun. 8, 15378. doi: 10.1038/Ncomms15378
Mariano, D. A., Santos, C., Wardlow, B. D., Anderson, M. C., Schiltmeyer, A. V., Tadesse, T., et al. (2018). Use of remote sensing indicators to assess effects of drought and human-induced land degradation on ecosystem health in northeastern Brazil. Remote Sens. Environ. 213, 129–143. doi: 10.1016/j.rse.2018.04.048
Moen, J., Ellis, E. C., Antill, E. C., Kreft, H. (2012). All is not loss: Plant biodiversity in the anthropocene. PloS One 7, e30535. doi: 10.1371/journal.pone.0030535
Oliver, T. H., Isaac, N. J. B., August, T. A., Woodcock, B. A., Roy, D. B., Bullock, J. M. (2015). Declining resilience of ecosystem functions under biodiversity loss. Nat. Commun. 6, 10122. doi: 10.1038/ncomms10122
Pennekamp, F., Pontarp, M., Tabi, A., Altermatt, F., Alther, R., Choffat, Y., et al. (2018). Biodiversity increases and decreases ecosystem stability. Nature 563, 109–112. doi: 10.1038/s41586-018-0627-8
Pimm, S. L. (1984). The complexity and stability of ecosystems. Nature 307, 321–326. doi: 10.1038/307321a0
Polazzo, F., Rico, A. (2021). Effects of multiple stressors on the dimensionality of ecological stability. Ecol. Lett. 24, 1594–1606. doi: 10.1111/ele.13770
Qin, Y., Yi, S., Ding, Y., Zhang, W., Qin, Y., Chen, J., et al. (2019). Effect of plateau pika disturbance and patchiness on ecosystem carbon emissions in alpine meadow in the northeastern part of qinghai–Tibetan plateau. Biogeosciences 16, 1097–1109. doi: 10.5194/bg-16-1097-2019
Radchuk, V., Laender, F. D., Cabral, J. S., Boulangeat, I., Crawford, M., Bohn, F., et al. (2019). The dimensionality of stability depends on disturbance type. Ecol. Lett. 22, 674–684. doi: 10.1111/ele.13226
Saruul, K., Jiangwen, L., Jianming, N., Qing, Z., Xuefeng, Z., Guodong, H., et al. (2019). Typical steppe ecosystems maintain high stability by decreasing the connections among recovery, resistance, and variability under high grazing pressure. Sci. Total Environ. 659, 1146–1157. doi: 10.1016/j.scitotenv.2018.12.447
Seddon, A. W., Macias-Fauria, M., Long, P. R., Benz, D., Willis, K. J. (2016). Sensitivity of global terrestrial ecosystems to climate variability. Nature 531, 229–232. doi: 10.1038/nature16986
Song, M. H., Cornelissen, J. H. C., Li, Y. K., Xu, X. L., Zhou, H. K., Cui, X. Y., et al. (2020). Small-scale switch in cover-perimeter relationships of patches indicates shift of dominant species during grassland degradation. J. Plant Ecol. 13, 704–712. doi: 10.1093/jpe/rtaa057
Sun, Y. X., Liu, S. L., Liu, Y. X., Dong, Y. H., Li, M. Q., An, Y., et al. (2022). Grazing intensity and human activity intensity data sets on the qinghai-Tibetan plateau during 1990-2015. Geosci. Data J. 9, 140–153. doi: 10.1002/gdj3.127
Tao, J., Zhang, Y., Dong, J., Fu, Y., Zhu, J., Zhang, G., et al. (2015). Elevation-dependent relationships between climate change and grassland vegetation variation across the qinghai-xizang plateau. Int. J. Climatol. 35, 1638–1647. doi: 10.1002/joc.4082
Tilman, D., Lehman, C. (2001). Human-caused environmental change: Impacts on plant diversity and evolution. Proc. Natl. Acad. Sci. United States America 98, 5433–5440. doi: 10.1073/pnas.091093198
Tin Kam, H. (1998). The random subspace method for constructing decision forests. IEEE Trans. Pattern Anal. Mach. Intell. 20, 832–844. doi: 10.1109/34.709601
Valencia, E., de Bello, F., Galland, T., Adler, P. B., Leps, J., E-Vojtko, A., et al. (2020). Synchrony matters more than species richness in plant community stability at a global scale. Proc. Natl. Acad. Sci. United States America 117, 24345–24351. doi: 10.1073/pnas.1920405117
Venter, O., Sanderson, E. W., Magrach, A., Allan, J. R., Beher, J., Jones, K. R., et al. (2016). Sixteen years of change in the global terrestrial human footprint and implications for biodiversity conservation. Nat. Commun. 7, 12558. doi: 10.1038/ncomms12558
Wang, Y., Heberling, G., Gorzen, E., Miehe, G., Seeber, E., Wesche, K. (2017). Combined effects of livestock grazing and abiotic environment on vegetation and soils of grasslands across Tibet. Appl. Veg. Sci. 20, 327–339. doi: 10.1111/avsc.12312
Wang, Y., Lv, W., Xue, K., Wang, S., Zhang, L., Hu, R., et al. (2022). Grassland changes and adaptive management on the qinghai–Tibetan plateau. Nat. Rev. Earth Environ. 3, 668–683. doi: 10.1038/s43017-022-00330-8
Wessels, K. J., Prince, S. D., Frost, P. E., van Zyl, D. (2004). Assessing the effects of human-induced land degradation in the former homelands of northern south Africa with a 1 km AVHRR NDVI time-series. Remote Sens. Environ. 91, 47–67. doi: 10.1016/j.rse.2004.02.005
White, H. J., Gaul, W., Sadykova, D., León-Sánchez, L., Caplat, P., Emmerson, M. C., et al. (2020). Quantifying large-scale ecosystem stability with remote sensing data. Remote Sens. Ecol. Conserv. 6, 354–365. doi: 10.1002/rse2.148
Wu, J., Feng, Y., Zhang, X., Wurst, S., Tietjen, B., Tarolli, P., et al. (2017). Grazing exclusion by fencing non-linearly restored the degraded alpine grasslands on the Tibetan plateau. Sci. Rep. 7, 15202. doi: 10.1038/s41598-017-15530-2
Wu, J., Zhang, X., Shen, Z., Shi, P., Xu, X., Li, X. (2013). Grazing-exclusion effects on aboveground biomass and water-use efficiency of alpine grasslands on the northern Tibetan plateau. Rangeland Ecol. Manage. 66, 454–461. doi: 10.2111/Rem-D-12-00051.1
Xia, J., Ma, M., Liang, T., Wu, C., Yang, Y., Zhang, L., et al. (2018). Estimates of grassland biomass and turnover time on the Tibetan plateau. Environ. Res. Lett. 13, 014020. doi: 10.1088/1748-9326/aa9997
Yang, G., Peng, C. H., Chen, H., Dong, F. Q., Wu, N., Yang, Y. Z., et al. (2017a). Qinghai–Tibetan plateau peatland sustainable utilization under anthropogenic disturbances and climate change. Ecosyst. Health Sustain. 3, e01263. doi: 10.1002/ehs2.1263
Yang, Z., Zhang, Q., Su, F., Zhang, C., Pu, Z., Xia, J., et al. (2017b). Daytime warming lowers community temporal stability by reducing the abundance of dominant, stable species. Global Change Biol. 23, 154–163. doi: 10.1111/gcb.13391
Yao, T., Thompson, L. G., Mosbrugger, V., Zhang, F., Ma, Y., Luo, T., et al. (2012). Third pole environment (TPE). Environ. Dev. 3, 52–64. doi: 10.1016/j.envdev.2012.04.002
Yu, H., Ding, Q., Meng, B., Lv, Y., Liu, C., Zhang, X., et al. (2021). The relative contributions of climate and grazing on the dynamics of grassland NPP and PUE on the qinghai-Tibet plateau. Remote Sens. 13, 3424. doi: 10.3390/rs13173424
Zeng, N., Ren, X. L., He, H. L., Zhang, L., Zhao, D., Ge, R., et al. (2019). Estimating grassland aboveground biomass on the Tibetan plateau using a random forest algorithm. Ecol. Indic. 102, 479–487. doi: 10.1016/j.ecolind.2019.02.023
Zhang, L., Zhou, G., Ji, Y., Bai, Y. (2016). Spatiotemporal dynamic simulation of grassland carbon storage in China. Sci. China Earth Sci. 59, 1946–1958. doi: 10.1007/s11430-015-5599-4
Zhong, Q., Ma, J., Zhao, B., Wang, X., Zong, J., Xiao, X. (2019). Assessing spatial-temporal dynamics of urban expansion, vegetation greenness and photosynthesis in megacity shanghai, China during 2000-2016. Remote Sens. Environ. 233, 111374. doi: 10.1016/j.rse.2019.111374
Zhu, J., Zhang, Y., Wang, W. (2016). Interactions between warming and soil moisture increase overlap in reproductive phenology among species in an alpine meadow. Biol. Lett. 12, 20150749. doi: 10.1098/rsbl.2015.0749
Keywords: alpine grassland, grazing, climate change, stability, dimensionality
Citation: Yang Y, Sun Y, Niu B, Feng Y, Han F and Li M (2022) Increasing connections among temporal invariability, resistance and resilience of alpine grasslands on the Tibetan Plateau. Front. Plant Sci. 13:1026731. doi: 10.3389/fpls.2022.1026731
Received: 24 August 2022; Accepted: 21 October 2022;
Published: 09 November 2022.
Edited by:
Xiong Zhao He, Massey University, New ZealandReviewed by:
Ting Hua, Beijing Normal University, ChinaCopyright © 2022 Yang, Sun, Niu, Feng, Han and Li. This is an open-access article distributed under the terms of the Creative Commons Attribution License (CC BY). The use, distribution or reproduction in other forums is permitted, provided the original author(s) and the copyright owner(s) are credited and that the original publication in this journal is cited, in accordance with accepted academic practice. No use, distribution or reproduction is permitted which does not comply with these terms.
*Correspondence: Fusong Han, aGFuZnMuMTdiQGlnc25yci5hYy5jbg==; Meng Li, bGltZW5nQG50dS5lZHUuY24=
Disclaimer: All claims expressed in this article are solely those of the authors and do not necessarily represent those of their affiliated organizations, or those of the publisher, the editors and the reviewers. Any product that may be evaluated in this article or claim that may be made by its manufacturer is not guaranteed or endorsed by the publisher.
Research integrity at Frontiers
Learn more about the work of our research integrity team to safeguard the quality of each article we publish.