- 1State Key Laboratory for Rice Biology, China National Rice Research Institute, Chinese Academy of Agricultural Sciences, Hangzhou, China
- 2The Key Laboratory for Quality Improvement of Agricultural Products of Zhejiang Province, College of Advanced Agricultural Sciences, Zhejiang A & F University, Hangzhou, China
Highly seed vigor (SV) is essential for rice direct seeding (DS). Understanding the genetic mechanism of SV-related traits could contribute to increasing the efficiency of DS. However, only a few genes responsible for SV have been determined in rice, and the regulatory network of SV remains obscure. In this study, the seed germination rate (GR), seedling shoot length (SL), and shoot fresh weight (FW) related to SV traits were measured, and a genome-wide association study (GWAS) was conducted to detect high-quality loci responsible for SV using a panel of 346 diverse accessions. A total of 51 significant SNPs were identified and arranged into six quantitative trait locus (QTL) regions, including one (qGR1-1), two (qSL1-1, qSL1-2), and three (qFW1-1, qFW4-1, and qFW7-1) QTLs associated with GR, SL, and FW respectively, which were further validated using chromosome segment substitution lines (CSSLs). Integrating gene expression, gene annotation, and haplotype analysis, we found 21 strong candidate genes significantly associated with SV. In addition, the SV-related functions of LOC_Os01g11270 and LOC_Os01g55240 were further verified by corresponding CRISPR/Cas9 gene-edited mutants. Thus, these results provide clues for elucidating the genetic basis of SV control. The candidate genes or QTLs would be helpful for improving DS by molecular marker-assisted selection (MAS) breeding in rice.
Introduction
Rice direct seeding (DS) technology, in which seeds are directly sown in the field without seedling raising or transplanting, provides an advanced and simplified cultivation technology for mechanized rice production. With the decrease in the rural labor force, the rise in labor cost, and the development of mechanized rice planting technology, rice planting in Asia, the main rice-producing area, has a trend of developing from traditional or mechanical transplanting to mechanical direct planting due to its labor- and cost-saving. However, DS requires the strong seed vigor (SV) of cultivated rice varieties, including high and uniform seed germination rate, strong seedling establishment and growth capacity, and enhanced stress tolerances. Most transplanting rice varieties on the market, including conventional rice, hybrid rice, and super rice, cannot be used for DS due to their poor SV. Thus, it is urgent to develop high SV varieties suitable for rice mechanized direct seeding through molecular-assisted selective breeding and biotechnology breeding, which depends on understanding the regulatory mechanism of SV-related traits and identifying the essential genes underlying these traits.
SV is a comprehensive trait affected by genetic and environmental factors during seed development and maturation. At present, a large number of QTLs for SV-related characteristics, such as seed germination rate (Wang et al., 2010; Jiang et al., 2020; Najeeb et al., 2020), coleoptile/seedling length (Dang et al., 2014; Xie et al., 2014; Jin et al., 2018), and seedling weight (Zhang et al., 2017; Najeeb et al., 2020), have been found in rice. Most of these QTLs were identified by bi-parental mapping with traditional markers. However, due to the low density of traditional markers, only a few QTLs or genes for SV have been fine-mapped. For instance, Fujino et al. isolated qLTG3-1, a QTL associated with low-temperature germinability. It may accelerate vacuolization and weaken the tissues covering the embryo during seed germination, thereby reducing the mechanical resistance to the growth potential of coleoptile (Fujino et al., 2008). In addition, qSE3, a major QTL for rice seed germination and seedling establishment under salinity stress, was identified as a K+ transporter OsHAK21 (He et al., 2019a). Physiological analysis revealed that qSE3 improved seed germination and seedling establishment against salinity stress through mediating seed physiological states, including increasing K+ and Na+ uptake, promoting abscisic acid (ABA) biosynthesis, and reducing reactive oxygen species (ROS) accumulation. Very recently, OsHIPL1, which encodes a hedgehog-interacting protein-like 1 protein, was cloned as a causal gene for the major QTL qSV3 associated with rice SV (He et al., 2022). And OsHIPL1 may play its role by modulating ABA levels and OsABIs expression during rice seed germination.
Genome-wide association study (GWAS) has become an effective way to dissect complex traits and identify corresponding loci or candidate genes. Several QTLs or genes for SV have been detected via GWAS in rice. For example, four germination rate (GR)-related QTLs in rice were revealed by GWAS, and OsOMT, encoding the 2-oxoglutarate/malate translocator, was further validated as a causal gene for qGR11. OsOMT played an essential role in GR by modulating amino acid levels, and the processes of glycolysis and tricarboxylic acid cycle (Li et al., 2021a). Yang et al. identified 19 QTLs associated with rice seed germination by GWAS. They further found that OsPK5 was a positive regulator of rice seed germination by encoding a pyruvate kinase and thereby modulating glycolytic metabolism and abscisic (ABA)/gibberellins (GA) balance (Yang et al., 2022). In addition, OsCDP3.10, encoding a cupin domain protein, was identified from qSP3, a significant QTL for rice seedling percentage by GWAS (Peng et al., 2022). Functional analysis revealed that OsCDP3.10 affected rice SV mainly by modulating the levels of amino acids and H2O2 in the germinating seeds. These studies demonstrated that GWAS is an effective way to identify QTLs/genes for SV in rice.
However, so far, almost all studies on GWAS related to SV have been carried out in Petri dishes and under constant temperature conditions, which cannot fully simulate the natural environment of the field. Therefore, the present study intends to apply GWAS to identify QTLs/genes for rice SV in the field. We surveyed the GR, seedling shoot length (SL), and shoot fresh weight (FW) of 346 rice accessions in the field, and performed GWAS analysis to identify reliable loci responsible for rice SV. In total, six QTLs were detected, including one for GR, two for SL, and three for FW. Combining gene expression, gene annotation, and haplotype analysis, we screened 21 candidate genes significantly associated with SV from these QTLs, among which LOC_Os01g11270 and LOC_Os01g55240 were further validated by corresponding CRISPR/Cas9 gene-edited mutants. The detected QTLs and candidate genes will enhance our understanding of the genetic and molecular basis for SV and provide valuable resources for applying these potential elite loci in rice molecular breeding.
Materials and methods
Plant material and phenotyping
The 346 representative accessions distributed worldwide were selected for the GWAS panel, including 213 indica and 133 japonica rice accessions (Supplementary Table 1). All seeds of these accessions were harvested at their maturity stage in September and October 2020, dried, and stored in a refrigeration house. To break seed dormancy, these seeds were dried at 42 °C for seven days before sowing (He et al., 2019b; He et al., 2022). When seeds are sown, the waterlogged paddy field is silty and sticky without standing water. In May 2021, 50 well-filled seeds of each accession were evenly sown in a circular box (6.4 cm with a diameter) on the surface of the paddy field at China National Rice Research Institute (Hangzhou, Zhejiang, China), following a randomized complete block experimental design with three replications. One week after sowing, we began to slowly saturate the paddy field, maintaining a thin layer of water with a depth of 1-2 cm on the soil’s surface. After direct sowing for two weeks, the data of GR, SL, and FW were measured. The temperature during this period was 17 °C ~ 26 °C. The seed was regarded as germination when the coleoptile or radicle length was longer than 1 cm, and GR was defined as the ratio of the number of germinated seeds to the total number of seeds. Five plants for each accession were randomly selected to measure the SL and FW (shoot fresh weight of all five plants). The mean data of GR, SL, and FW were presented and obtained from the three replicates, including 344, 314, and 313 mean values for GR, SL, and FW, respectively (Supplementary Table 1). The lack of mean GR data for two accessions was due to the large SD of three replicates, which was considered inaccurate and consequently discarded. Similarly, the mean FW value of D188 accession, and SL and FW values of D299 accession were also discarded. In addition, to eliminate the influence of germination time on SL and FW, the SL and FW of 31 accessions with low GR (<38%) and shallow emergence rate one week after sowing were not recorded. These missing GR, SL, and FW data were marked as NA in Supplementary Table 1.
Chromosome segment substitution lines (CSSLs) used for qGR1-1, qSL1-1, qSL1-2, qFW1-1, qFW4-1, and qFW7-1 validation were constructed by a combination of backcross and molecular maker-assisted selection (Dai et al., 2020). After backcrossing four times, each CSSL has a genetic background with recurrent receptor parent 9311 and only carries a target chromosomal segment from the donor parent Nipponbare (NPB). After drying at 42 °C for seven days, 50 well-filled seeds per replicate of 9311 and CSSLs were directly sown in ddH2O at 30 °C for four days, then transplanted in a conventional Kimura B hydroponic medium at 30 °C for six days (maintain culture in ddH2O for GR). The GR, SL, and FW data for each CSSL and its recurrent parent 9311 were collected ten days after cultivation. Three biological replicates were performed for GR and FW, and ten replicates were used for SL.
The CRISPR/Cas9-mediated gene editing mutants for LOC_Os01g11270 and LOC_Os01g55240 were obtained from Weimi Biotechnology Co., Ltd. (Lu et al., 2017). And the cultivation and measurement methods are the same as those of the CSSLs.
Genome resequencing and SNP genotyping
The genomic DNA of each accession for the GWAS population was extracted by the cetyltrimethylammonium bromide (CTAB) method (Murray and Thompson, 1980). The resequencing library was constructed according to the manufacturer’s instructions (Illumina, San Diego, CA, USA). The paired-end reads in each library were generated by Illumina NovaSeq 6000 platform at Berry Genomics Company (http://www.berrygenomics.com/,Beijing,China). The clean reads were aligned to the NPB reference genome (IRGSP-1.0) with Burrows-Wheeler Aligner (BWA) (Li and Durbin, 2009). Genome Analysis Toolkit software (GATK) (Van der Auwera et al., 2013) was used for single nucleotide polymorphism (SNP) calling. The SNP annotations were analyzed by SnpEff (Cingolani et al., 2012) based on the GFF3 files of the NPB reference genome. A total of 2,748,212 high-quality SNPs with minor allele frequency (MAF) greater than 5% and a missing rate less than 20% were screened for further GWAS.
Population genetics analysis
We randomly selected 100,000 SNPs with a MAF > 5% and a missing rate < 20% to build a phylogenetic tree using SNPhylo (Lee et al., 2014; Li et al., 2021b; Feng et al., 2022), and plotted it with the R package of ‘ggtree’ (Yu et al., 2017). The Principal component analysis (PCA) and population structure analysis for all accessions were calculated by Plink (Purcell et al., 2007) and ADMIXTURE (Alexander et al., 2009).
Genome-wide association study
GWAS was conducted using vcf2gwas with a linear mixed model (Vogt et al., 2022). GR, SL, and FW values for 346 rice accessions were used as input data. Based on the Bonferroni-corrected method and previous reports (Yang et al., 2014; Li et al., 2021b; Feng et al., 2022), the P-value of the whole-genome significance cutoff was set as 1/n (n = the number of SNPs used in association analysis); that is, the threshold of -log10 (P) was about 6.4. Manhattan and quantile-quantile (Q-Q) plots were generated using the R package of Cmplot (https://github.com/YinLiLin/CMplot). Based on the previous report, the 200-kb upstream and downstream significant SNP site was regarded as a QTL region, and adjacent overlapped regions were merged into the same QTL (Li et al., 2022).
Haplotype analysis
The SNPs in the regions of 2-kb upstream, CDS, and 1-kb downstream sequence of each gene were selected for haplotype analysis. The rare haplotype with a frequency lower than ten were discarded. The top two or three haplotypes with the highest frequency were chosen for further phenotypic association analysis.
Result
Phenotypic variation of SV-related traits in 346 rice accessions
We observed significant variations of three SV-related traits (GR, SL, and FW) among 346 rice accessions. In detail, the GR ranged from 6.00 to 100.00%, with an average of 69.74% and a variation coefficient of 28.69% (Table 1). The SL changed from 8.40 to 26.20 cm, with a mean value of 15.42 cm and a variation coefficient of 22.65%. The FW varied from 0.1325 to 0.4800 g, with an average of 0.2592 g and a variation coefficient of 23.01%. These results indicate an extensive phenotypic variation (PV) in our experimental population. Furthermore, according to the kurtosis and skewness values, all traits showed skewed distribution, especially GR (Figures 1A, C, E; Table 1). We further analyzed the SV-related values in indica and japonica subpopulations. It revealed that the GR, SL, and FW of indica and japonica were 69.94% and 69.42%, 16.4 cm and 14.0 cm, 0.2722 g and 0.2391 g, respectively. Except for GR, a significant difference was observed for SL and FW between indica and japonica (Figures 1B, D, F). In sum, the GR, SL, and FW traits were suitable for subsequent GWAS mapping.
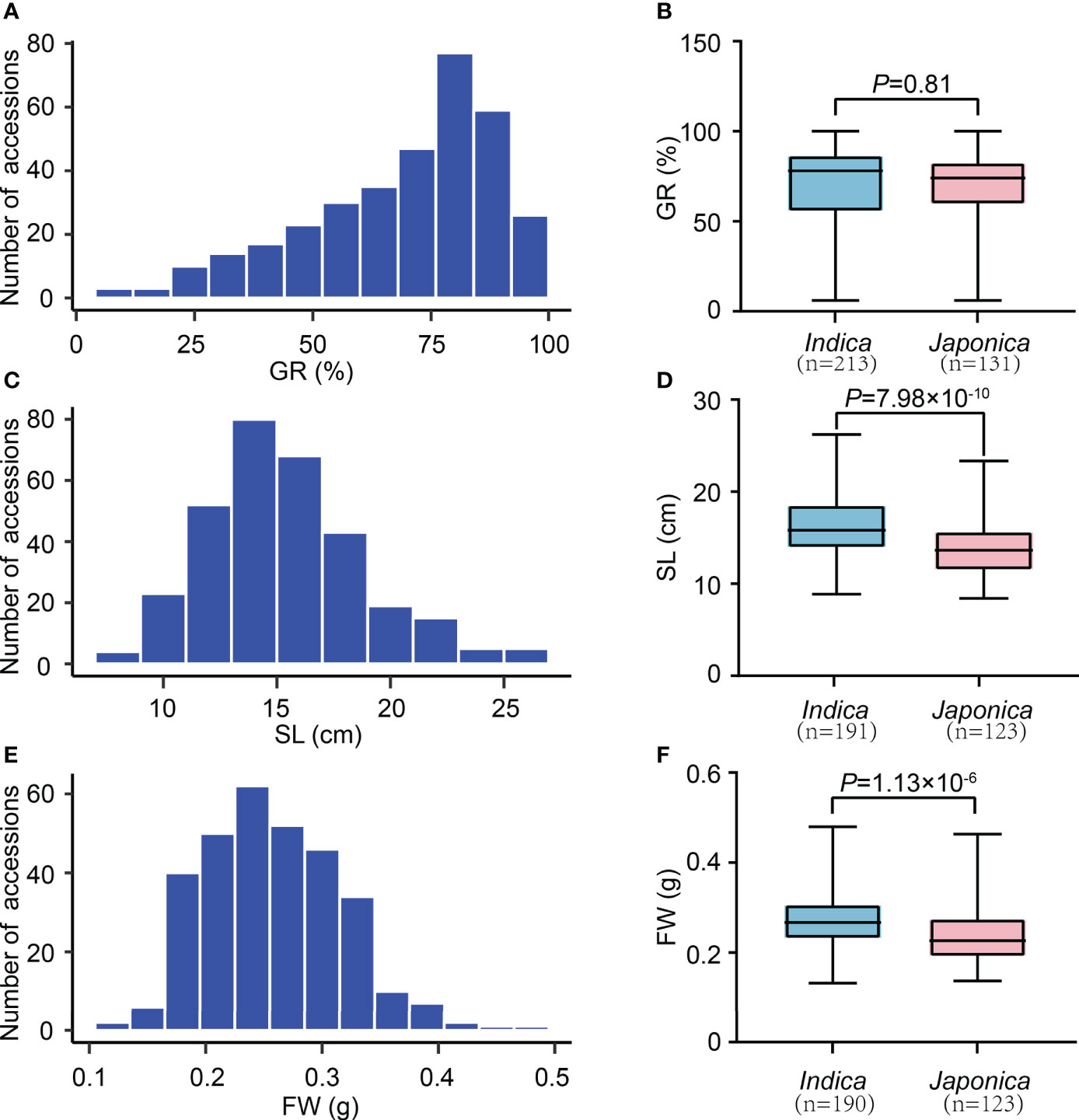
Figure 1 The variation of SV-related traits in 346 rice accessions. (A) Distribution of GR values. (C) Distribution of SL values. (E) Distribution of FW values. (B) Comparison of GR values between indica and japonica. (D) Comparison of SL values between indica and japonica. (F) Comparison of FW values between indica and japonica. n indicates the number of accessions. The p-value is obtained from the T-test.
Population structure of 346 rice accessions
The 346 rice accessions were classified into two subgroups based on the phylogenetic tree, roughly corresponding to the classification of indica and japonica (Figure 2A). Similarly, PCA and the population structure base on the possible clusters (k) method also showed that these rice accessions could be divided into two subgroups as the phylogenetic tree classification (Figures 2B, C). Taken together, the category of population structure has a similar result compared to a previous study (Shi et al., 2021; Li et al., 2022).
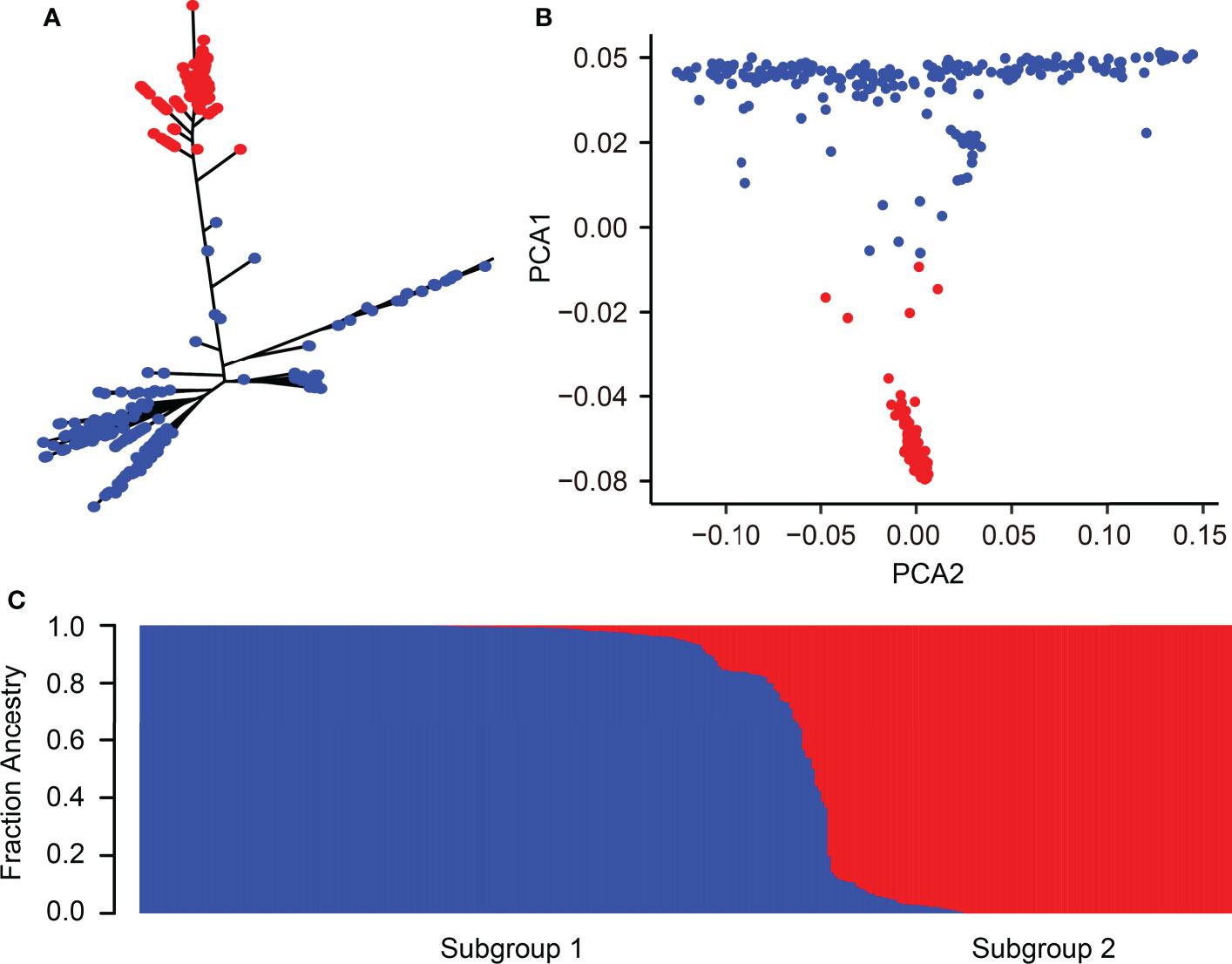
Figure 2 Population structure of 346 rice accessions. (A) Phylogenetic tree of 346 rice accessions. (B) PCA of 346 rice accessions. The first and second principal components are represented by PCA1 and PCA2, respectively. (C) Cluster analysis of 346 rice accessions. Blue and red colors correspond to indica and japonica accessions, respectively.
Mining loci associated with SV by GWAS
To explore the novel loci related to SV, we performed GWAS on GR, SL, and FW in 346 rice accessions, respectively. The Manhattan plots of GWAS showed 18, 30, and 3 significant SNPs were associated with GR, SL, and FW, respectively, and assigned as six QTLs (qGR1-1, qSL1-1, qSL1-2, qFW1-1, qFW4-1, and qFW7-1) according to their physical position in the genome (Figure 3; Table 2 and Supplementary Table 2). For GR and SL, 18, 2, and 28 significant SNPs were detected in qGR1-1, qSL1-1, and qSL1-2 on chromosome 1, contributing 7.76 ~ 10.53%, 7.92%, and 7.78 ~ 9.33% of the phenotypic variation explanation (PVE), respectively (Table 2, Supplementary Table 2). Whereas FW, qFW1-1, qFW4-1, and qFW7-1 located on chromosomes 1, 4, and 7 respectively, all contain only one associated SNP that explains 7.98 ~ 9.28% of the PVE (Table 2, Supplementary Table 2).
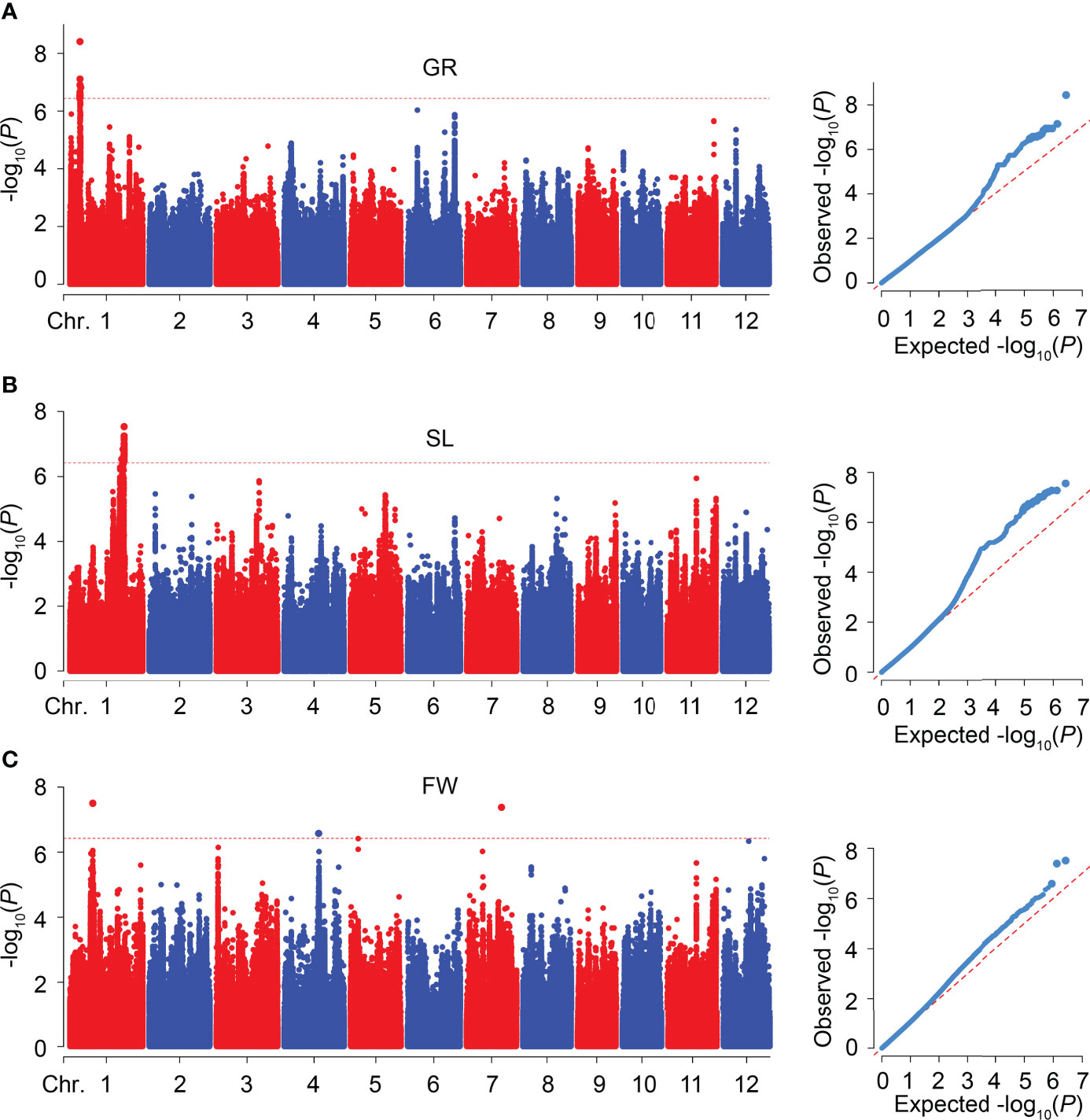
Figure 3 GWAS for SV-related traits in 346 rice accessions. (A-C) Manhattan and quantile-quantile (Q-Q) plots of GWAS for GR (A), SL (B), and FW (C), respectively.
Validation of GWAS-associated loci using CSSLs
To verify the GWAS-associated QTLs, we screened five CSSLs from the CSSL library with 9311 as recurrent parent and NPB as donor parent. We named them CSSL14 (containing qGR1-1), CSSL16 (containing qSL1-1 and qSL1-2), CSSL3 (containing qFW1-1), CSSL18 (containing qFW4-1), and CSSL15 (containing qFW7-1), respectively (Figure 4A). A comparison of SV-related traits between 9311 and corresponding CSSLs revealed significant differences in GR, SL, and FW, respectively. In detail, the GR of CSSL14 increased by 7.34% compared with that of 9311 (Figure 4B), while the SL of CSSL16 and FW of CSSL3, CSSL18, and CSSL15 decreased by 12.86%, 13.27%, 29.50%, and 22.08% compared with that of 9311, respectively (Figures 4C, D). These data suggest that the above GWAS results are reliable and imply that the NPB allele is the dominant allele of qGR1-1, whereas the allele from 9311 is the superior allele for qSL1-1, qSL1-2, qFW1-1, qFW4-1, and qFW7-1.
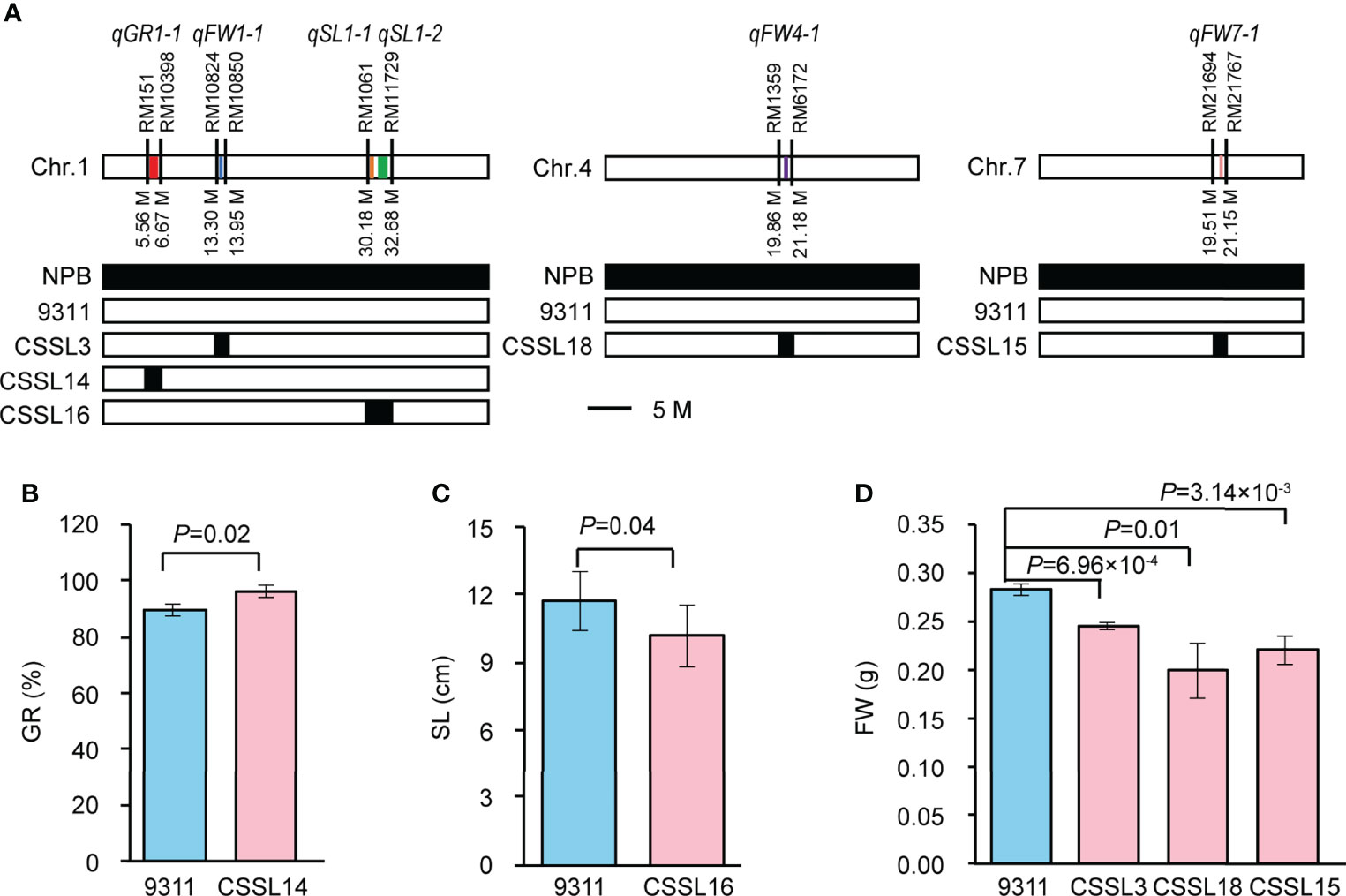
Figure 4 Validation of six GWAS-associated QTLs using CSSLs. (A) The genotype diagrams of CSSLs. qGR1-1, qSL1-1, qSL1-2, qFW1-1, qFW4-1, qFW7-1 are represented by red, orange, green, blue, purple, and yellow lines, respectively. Line width indicates the size of QTL interval. (B) Comparison of GR between CSSL14 and 9311. Data represent mean ± SD (n=3). (C) Comparison of SL between CSSL16 and 9311. Data represent mean ± SD (n=10). (D) Comparison of FW among CSSL3, CSSL18, CSSL15, and 9311. Data represent mean ± SD (n=3). n indicates the number of replicates. The p-value is obtained from the T-test.
Identification of SV-related candidate genes in GWAS-associated loci
To search for the candidate genes in six QTLs, we excavated all 540 annotated genes, including 135, 60, 169, 59, 58, and 59 genes in qGR1-1, qSL1-1, qSL1-2, qFW1-1, qFW4-1, and qFW7-1, respectively (Supplementary Table 3). We used three strategies to narrow down the candidate genes: Firstly, 76 genes not expressed in seeds (for GR) or seeds and leaves (for SL and FW) were deleted based on the expression profile in Rice Expression Database (http://expression.ic4r.org/) (Supplementary Table 3). Secondly, 65 genes encoding retrotransposon or transposon protein were excluded based on their function annotations (Supplementary Table 3). Meanwhile, 28 genes considered highly related to the SV phenotype were screened from the remaining genes (Supplementary Table 3). The common feature of these 28 genes is that they and their homologs are at least associated with SV-related traits or involved in hormone metabolism or signaling pathways such as ABA and GA. Lastly, haplotype analysis was carried out using the SNPs in 346 accessions, and 21 candidate genes associated with significant SV-related phenotypic differences between their top two or three haplotypes were screened (Supplementary Tables 1, 3). Their distances from the nearest significant SNPs are shown in Supplementary Table 4. However, it is important to note that the phenotypic differences between haplotypes of some candidate genes may result from the phenotypic differences between indica and japonica subpopulations, since SL and FW differences exist in the two subspecies.
Among them, LOC_Os01g11270 located in qGR1-1 was annotated as a cytochrome P450 (CYP450) (Supplementary Table 3), and its homologs played a crucial role in hormone metabolisms, such as gibberellin (GA) (Zhu et al., 2005; Wu et al., 2014) and abscisic acid (ABA) (Zhu et al., 2009; Zhang et al., 2020). GA and ABA are two antagonistic phytohormones with positive and negative regulatory effects on seed germination and seedling establishment, respectively. Therefore, LOC_Os01g11270 is a strong candidate associated with GR. Haplotype analysis revealed that LOC_Os01g11270 has two major haplotypes according to an SNP located in its promoter (Figure 5A). Haplotype 1 (Hap1, reference sequence) was mainly found in japonica accessions, while Hap2 only occurred in indica accessions (Figure 5A; Supplementary Table 1). Comparatively, Hap1 had a lower GR than Hap2 (Figure 5B). To further validate the role of LOC_Os01g11270 in rice seed germination, we compared the GR of the LOC_Os01g11270 gene-edited mutant (KO#270) and its wild-type Zhonghua 11 (ZH11). In KO#270, an 8-bp deletion at base 799 to 806 in the LOC_Os01g11270 CDS caused a frameshift mutation (Figure 5C). The statistical result showed that the GR of KO#270 decreased by 15% compared to that of ZH11 (Figures 5C, D), suggesting a positive regulation effect of LOC_Os01g11270.
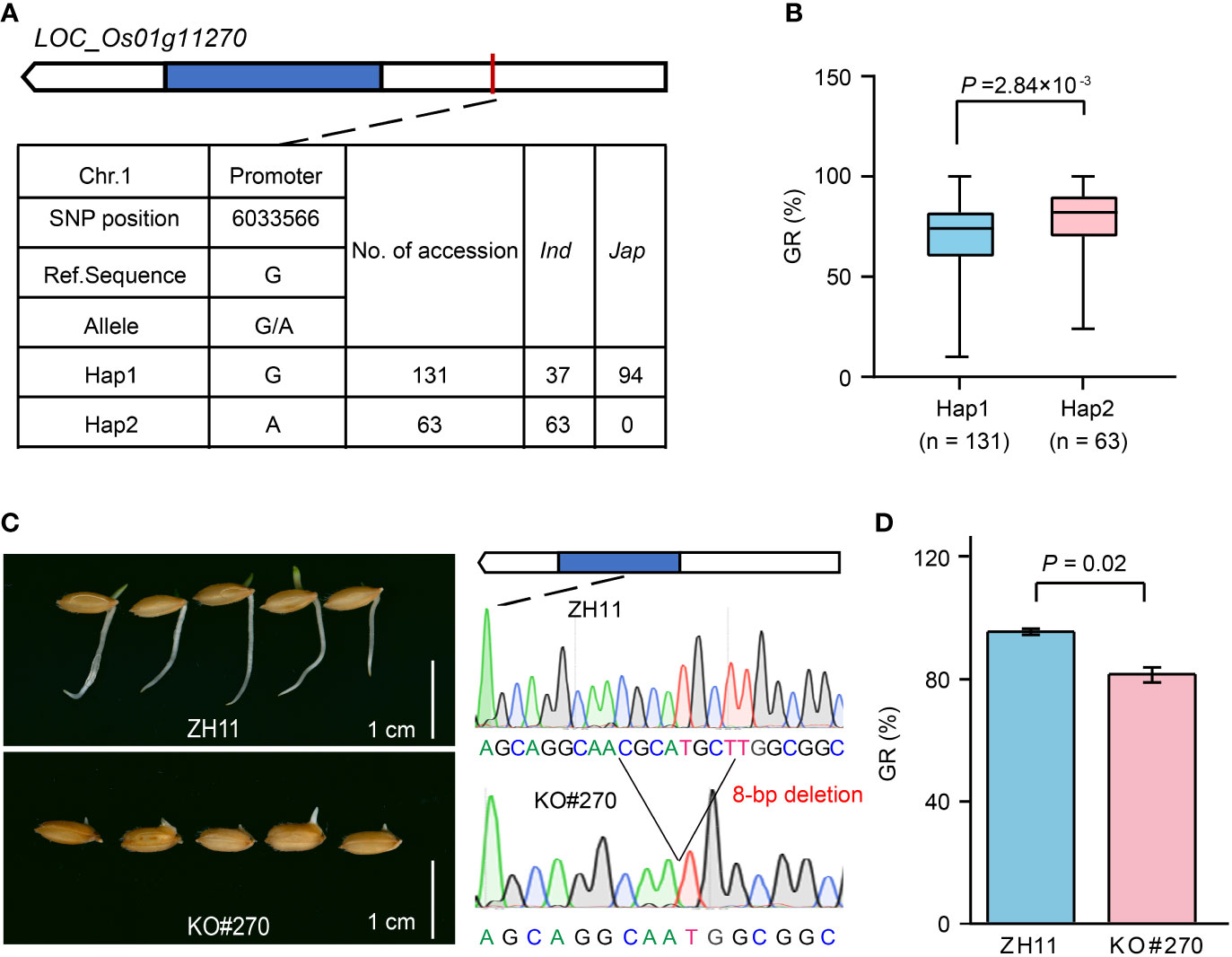
Figure 5 Effect of LOC_Os01g11270 on rice seed germination. (A) The top two haplotypes of LOC_Os01g11270 in 346 rice accessions. The blue box represents the exon, the white box indicates 2-kb upstream region of the start codon or 1-kb downstream region of the stop codon, and the black line represents the intron. (B) Comparison of GR between accessions containing Hap1 and Hap2. (C) Comparison of seed germination status after imbibition for five days between LOC_Os01g11270 gene-edited mutant (KO#270) and its wild-type ZH11. (D) Comparison of GR between KO#270 and ZH11. Data represent mean ± SD from three replicates. The p-value is obtained from the T-test.
LOC_Os01g55240 in qSL1-2 was identified as a GA inactivation gene (Supplementary Table 3), encoding a gibberellin 2-beta-dioxygenase (OsGA2ox3) (Tong et al., 2014), and thus may control seedling growth. Haplotype analysis of OsGA2ox3 revealed two major haplotypes, Hap1 (reference sequence) and Hap2, that existed in 346 rice accessions (Figure 6A). The Hap1 associated with short SL was mainly found in japonica accessions, whereas Hap2 related to long SL only occurred in indica accessions (Figures 6A, B). To know whether the differential gene expression levels cause the SL variation in different haplotypes, we compared the transcripts of OsGA2ox3 between Hap1 and Hap2 accessions using the leaf tissues (the uppermost complete leaves from four 3-week-old plants) RNA-seq dataset from our laboratory. The result showed that there was no significant difference between the average gene expression levels of Hap1 and Hap2 (Figure 6C; Supplementary Table 1), suggesting that the SL difference between Hap1 and Hap2 was not caused by their differential gene expression levels. To further validate the effect of OsGA2ox3 in rice seedling growth, we compared the SL between the OsGA2ox3 gene-edited mutant (KO#240) and its wild-type ZH11. In KO#240, one bp insertion between base 104 to 105 in the OsGA2ox3 CDS resulted in a frameshift mutation (Figure 6D). It showed that the SL of KO#240 was 15% longer than that of the wild type (Figures 6D, E), suggesting a negative regulation effect of OsGA2ox3.
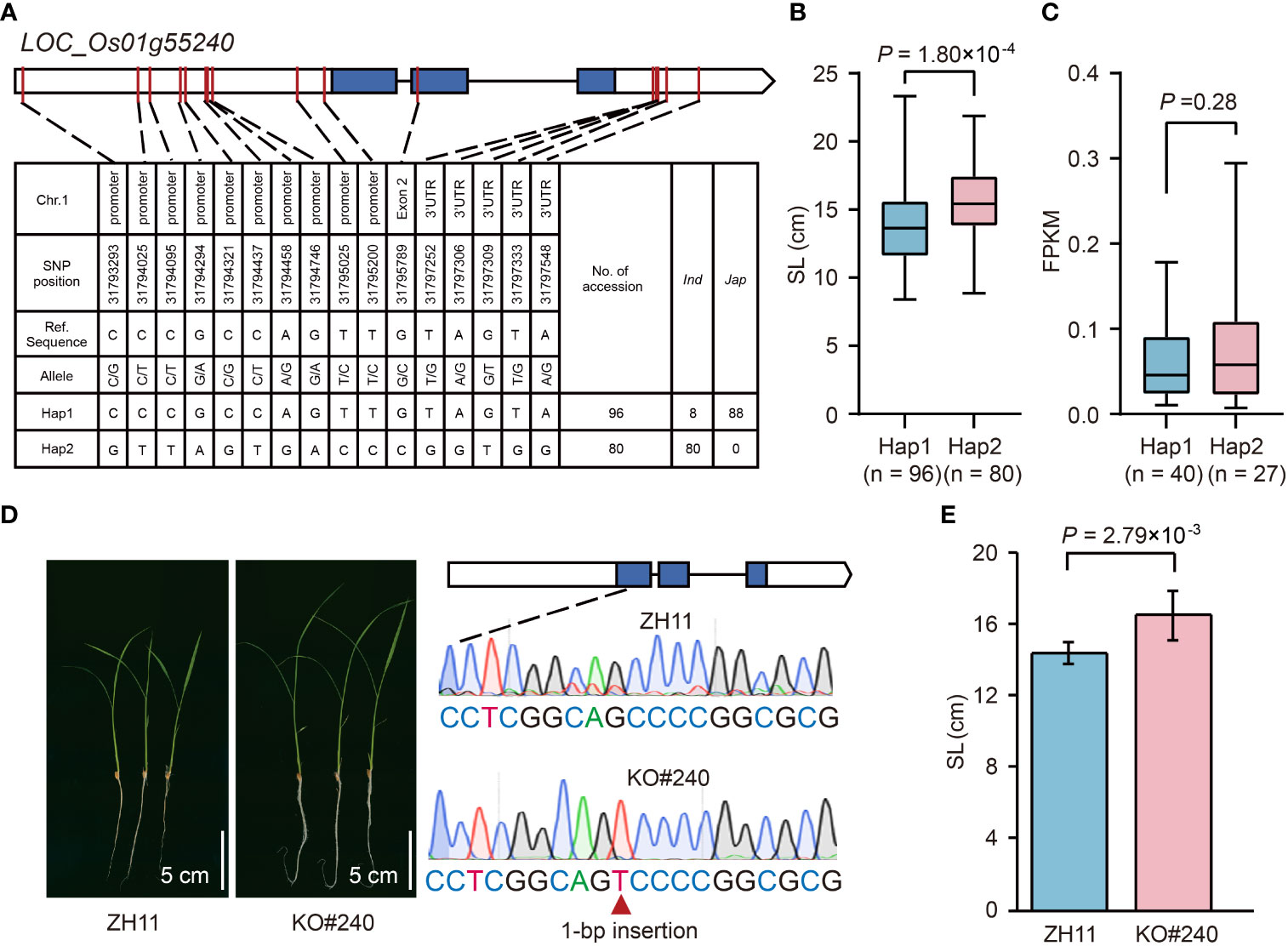
Figure 6 Effects of LOC_Os01g55240 on rice seedling growth. (A) The top two haplotypes of LOC_Os01g55240 in 346 rice accessions. The blue box represents the exon, the white box indicates 2-kb upstream region of the start codon or 1-kb downstream region of the stop codon, and the black line represents the intron. (B) Comparison of SL between accessions with Hap1 and Hap2. (C) Comparison of gene expression in leaves between accessions with Hap1 and Hap2. The mean FPKM value of three replicates is represented as the gene expression value for each accession. (D) Comparison of seedling growth status after imbibition for ten days between LOC_Os01g55240 gene-edited mutant (KO#240) and its wild-type ZH11. (E) Comparison of SL between KO#240 and ZH11. Data represent mean ± SD from ten replicate. The p-value is obtained from the T-test.
Discussion
Seed vigor is crucial in determining the yield of direct seeding rice. Good SV contains strong and uniform seed germination, rapid seedling growth, and increased stress tolerance. In contrast, apart from without these advantages, the poor SV also leads to weed bloom due to the weak seedling competitiveness, which further reduces rice production (Rajjou et al., 2012). Therefore, deciphering the molecular regulatory mechanisms of SV, and identifying the causative genes underlying SV have been the focus of rice biology. In the past decade, GWAS has been widely used to detect the potential loci or alleles underlying complex agronomic traits in rice. This study used three indicators, GR, SL, and FW, closely related to seed germination and seedling growth, to evaluate SV and perform GWAS. The results revealed a considerable variation and nearly normal distribution of GR, SL, and FW in 346 accessions, respectively (Figures 1A, C, E; Table 1). In addition, the indica accessions may be more suitable for DS because they had larger SL and FW than japonica accessions (Figures 1D, F). A total of six QTLs (qGR1-1 for GR, qSL1-1 and qSL1-2 for SL, qFW1-1, qFW4-1, and qFW7-1 for FW) were identified through GWAS using the whole population (Figure 3; Table 2). Among them, we found two QTLs co-located with previously reported SV-related QTLs using the traditional mapping method, suggesting that our GWAS results are pretty reliable (Table 2). For instance, qGR1-1 corresponds to QAlGC1.2, a QTL for germination capacity under aluminum stress and mapped between markers RM14 and RM243 (Jahan et al., 2021). qFW1-1 shares the same location with qSWW1b, a major QTL for the wet seedling weight (Zhang et al., 2017). In addition, we also found qSL1-2 co-located with previously reported qASRI1c, a QTL for anaerobic salt response index (ASRI) of coleoptile identified by GWAS (Islam et al., 2022). However, other GWAS signals do not overlap with the previous GWAS results for SV-related traits (Li et al., 2021a; Peng et al., 2022; Yang et al., 2022). Besides the different rice accessions we used, one possible reason for the different results of GWAS is that phenotypic statistics of previous GWAS were mainly performed in Petri dishes and under constant temperature conditions. In contrast, we conducted phenotypic statistics in field conditions. In this study, we further used CSSLs to validate the GWAS-associated loci, and this strategy is relatively rare in GWAS studies.
By scanning the 540 annotated genes in six QTLs, we screened 21 strong candidate genes associated with SV (Supplementary Table 3). Based on functional annotations and literature review, these genes can be broadly classified into hormone homeostasis and signal-related, CYP450s, calcium signal-related, transcription factors, and so on. Here, we chose some representative ones for discussion. For example, LOC_Os01g11150 (OsGA2ox7), LOC_Os01g55240 (OsGA2ox3), LOC_Os07g34240, and LOC_Os07g34370 were annotated as gibberellin 2-beta-dioxygenase or putative gibberellin receptor (Supplementary Table 3). Therefore, they may influence SV by regulating GA activity or signaling pathway. In fact, OsGA2ox3 is mainly expressed in seedlings, and the OsGA2ox3-activated mutant or overexpression transgenic plant was severely dwarfed and maintained a vegetative growth (Lo et al., 2008; Takehara et al., 2020; Hsieh et al., 2021). In contrast, OsGA2ox3 CRISPR/Cas9 knockout mutant showed a taller plant height due to elongated internodes and leaves (Takehara et al., 2020; Hsieh et al., 2021). These results suggest that OsGA2ox3 is a negative regulator of plant height. Similarly, we also confirmed that OsGA2ox3 down-regulated SL using OsGA2ox3 CRISPR/Cas9 knockout mutant (Figures 6D, E). Furthermore, we found that the Hap2 in indica accessions was the dominant haplotype compared to Hap1 in japonica accessions (Figures 6A, B), implicating a great potential for improving SV-related traits in japonica accessions by OsGA2ox3. Compared with OsGA2ox3, OsGA2ox7 in qGR1-1 is an attenuated functional gene since its overexpression transgenic plants exhibited a semi-dwarf phenotype, whereas no phenotypic change on plant height was observed in osga2ox7 knockout mutant (Hsieh et al., 2021). Here, we found apparent differences in GR and SL between the two main haplotypes of OsGA2ox7 (Supplementary Figures 1A, B). Similarly, the SL and FW of the two main haplotypes of LOC_Os07g34240 and LOC_Os07g34370 in qFW7-1 also differ significantly (Supplementary Figures 1C–F). These results suggest that GA is a vital hormone for regulating SV, and there is a great potential to improve SV by targeting genes related to the GA pathway.
Auxin is a universal coordinator for plant growth and development and stress responses. It is well known to promote coleoptile elongation and seedling growth (Gallei et al., 2020). Of 21 strong candidates, LOC_Os01g54990 (OsARF3), LOC_Os01g10970, and LOC_Os01g55940 (OsGH3.2) were regarded as auxin response factor (ARF) or indole-3-acetic acid (IAA)-amido synthetase (conversion of active IAA to an inactive form) (Supplementary Table 3), and thus may control SV through modulating auxin homeostasis or signaling pathway. Actually, OsGH3.2 overexpression not only resulted in remarkable morphological changes in transgenic lines, such as dwarfism, shortened leaf length, and small panicles and internodes, but also showed increased cold stress tolerance and drought hypersensitivity through the modulation of IAA and ABA homeostasis. In contrast, no significant change in these phenotypes was detected in OsGH3.2-suppressed plants (Du et al., 2012). Recently, OsGH3.2 was demonstrated as a negative regulator of seed longevity by regulating the accumulation of ABA (Yuan et al., 2021). In this study, we observed significant differences in SV-related traits between the two main haplotypes of OsGH3.2 and OsARF3 in qSL1-2 and LOC_Os01g10970 in qGR1-1 (Supplementary Figures 2A–C). These data suggest that auxin acts as an essential factor in modulating SV.
LOC_Os01g11270, LOC_Os01g11280, and LOC_Os01g11340 in qGR1-1 were predicted as CYP450s (Supplementary Table 3), which are the biggest enzymatic protein family involved in NADPH- and oxygen-dependent hydroxylation reactions in plants (Pandian et al., 2020). As versatile catalysts, CYP450s play vital roles in the biosynthesis of primary and secondary metabolites, antioxidants, and phytohormones, thereby regulating plants’ growth and development, and protecting them from stresses (Xu et al., 2015; Pandian et al., 2020). CYP714D1, CYP714B1, and CYP714B2 act as the GA deactivating enzymes in rice, and their mutants and overexpressing transgenic plants showed tall and dwarfed phenotypes, respectively (Zhu et al., 2006; Magome et al., 2013). CYP90D2 encodes a brassinosteroid (BR) biosynthetic enzyme whose mutants were dwarfed (Hong et al., 2003; Fang et al., 2016). CYP96B4 modulates rice growth and stress tolerance by finetuning the balance of GA and ABA. cyp96b4 mutant showed pleiotropic abnormal phenotypes, including dwarfism, delayed germination and early growth, and enhanced tolerance to drought (Tamiru et al., 2015). In addition, CYP71D8L is a crucial regulator for GA and cytokinin (CK) homeostasis, thereby controlling multiple agronomic traits and stress responses (Zhou et al., 2020). These results imply that LOC_Os01g11270, LOC_Os01g11280, and LOC_Os01g11340 could influence SV by mediating phytohormones’ homeostasis. Here, significant variations in GR between the two main haplotypes of these three genes were detected (Figures 5A, B, Supplementary Figures 3A, B), which support the above speculation. And the effect of LOC_Os01g11270 on GR was further confirmed using its CRISPR/Cas9 knockout mutant (Figures 5C, D). In addition, the Hap2 with a higher GR may be an elite allele available for SV improvement.
Ca2+ acts as an essential nutrient and a universal second messenger for plants’ growth and development in normal and stressful conditions. The Ca2+ signals are decoded through the interactions between a unique set of Ca2+ sensors named calcineurin B-like proteins (CBLs) and the major downstream signaling components called CBL-interacting protein kinases (CIPKs). These CBLs-CIPKs interactions define the complexity of the Ca2+ signaling networks for the perception and transduction of stress signals under various environmental stresses (Tang et al., 2020). In rice, OsCIPK3, OsCIPK9, and OsCIPK23 are involved in cold, salinity, and drought stress responses, respectively (Xiang et al., 2007; Yang et al., 2008; Shabala et al., 2021). OsCIPK31 functions in seed germination and seedling growth under abiotic stress conditions (Piao et al., 2010). OsCIPK15 is related to rice germination and subflooding tolerance under oxygen deprivation (Lee et al., 2009; Kudahettige et al., 2011). Of 21 strong candidates, two CIPK genes, LOC_Os01g55450 (OsCIPK12) in qSL1-2 and LOC Os01g10870 (OsCIPK13) in qGR1-1 were observed (Supplementary Table 3). We found apparent variations in SL and GR between the two main haplotypes of OsCIPK12 and OsCIPK13, respectively (Supplementary Figures 4A, C). In addition, the longer SL in Hap2 of OsCIPK12 corresponds to the higher gene expression level, indicating that OsCIPK12 is a positive regulator of SL (Supplementary Figure 4B). Our results, combined with the overexpression of OsCIPK12 resulted in increased drought tolerance in seedlings (Xiang et al., 2007), suggesting that OsCIPK12 is a promising SV improvement gene.
As critical regulatory elements of gene expression, we identified three transcription factors (TFs) in qSL1-1 and qSL1-2, including one bZIP family TF (LOC_Os01g55150) and two OVATE family TFs (LOC_Os01g53160 and LOC_Os01g54570) (Supplementary Table 3). The bZIP family proteins have been shown to regulate a set of plant growth and development processes, such as photomorphogenesis, floral induction and development, and seed maturation and germination. They also involve stress responses (Nijhawan et al., 2008). Here, we observed that the Hap2 of LOC_Os01g55150 had longer SL, heavier FW, and higher gene expression than those of Hap1 (reference sequence) (Supplementary Figures 5A-C), implicating that LOC_Os01g55150 acts as a positive regulator. Compared with the bZIP family, the OVATE family with a conserved DUF623 domain is relatively small and poorly understood. In rice, several OVATE family proteins (OsOFPs) have been cloned and shown to regulate multiple aspects of plant growth and development, including seedling growth, plant architecture, leaf morphology, grain shape, and abiotic stresses (Schmitz et al., 2015; Yang et al., 2016; Ma et al., 2017; Xiao et al., 2017; Yang et al., 2018; Xiao et al., 2020). Among them, LOC_Os01g53160, also named OsOFP3, acts as a repressor of both BR biosynthesis and signal and incorporates into a TF complex to regulate BR signaling, thereby controlling the proper development of plants. In this study, we found apparent variations in SL and FW between the top two haplotypes of LOC_Os01g53160 and LOC_Os01g54570 (Supplementary Figures 5D–G). Furthermore, the larger values of SL and FW in Hap2 of LOC_Os01g54570 were associated with its higher gene expression level (Supplementary Figure 5H), implicating a positive role of LOC_Os01g54570 on SV.
In sum, we detected six QTLs for GR, SL, and FW traits by GWAS within 346 rice accessions, and screened 21 strong candidate genes with differential SV-related features between their top two haplotypes. Two genes, LOC_Os01g11270 and LOC_Os01g55240, were further validated. The identification of those candidate genes and their elite haplotypes provides a promising source for molecular breeding for high vigor seeds in rice.
Data availability statement
The original SNP data presented in the study are publicly available. This data can be found here: https://github.com/LipingDai/GWAS-for-rice-vigor/. The raw sequencing data presented in this article are not readily available for ownership reasons. Requests to access the datasets should be directed to the corresponding authors.
Author contributions
DZ, QQ, and QL designed and supervised the study. LD and XL performed the experiments. LD conducted bioinformatics analysis and interpretation of the data. LS, LG, GZ, ZG, LZ, JH, DR, QZ, DZ, QQ, and QL participated in rice material collection and provided resequencing resources. LD and QL drafted the manuscript. DZ revised the manuscript. LD and QL responded to review comments. All authors contributed to the article and approved the submitted version.
Funding
This work was financially supported by the Hainan Yazhou Bay Seed Laboratory Project (B21HJ0220), the National Natural Science Foundation of China (32101755), the China Postdoctoral Science Foundation (2020M680778), and the Key Research and Development Program of Zhejiang Province (2021C02056).
Acknowledgments
We thank Biogle Genome Editing Centre (BGEC) for providing the rice gene editing seeds.
Conflict of interest
The authors declare that the research was conducted in the absence of any commercial or financial relationships that could be construed as a potential conflict of interest.
Publisher’s note
All claims expressed in this article are solely those of the authors and do not necessarily represent those of their affiliated organizations, or those of the publisher, the editors and the reviewers. Any product that may be evaluated in this article, or claim that may be made by its manufacturer, is not guaranteed or endorsed by the publisher.
Supplementary material
The Supplementary Material for this article can be found online at: https://www.frontiersin.org/articles/10.3389/fpls.2022.1005203/full#supplementary-material
References
Alexander, D. H., Novembre, J., Lange, K. (2009). Fast model-based estimation of ancestry in unrelated individuals. Genome Res. 19 (9), 1655–1664. doi: 10.1101/gr.094052.109
Cingolani, P., Platts, A., Wang, L. L., Coon, M., Nguyen, T., Wang, L., et al. (2012). A program for annotating and predicting the effects of single nucleotide polymorphisms, SnpEff: SNPs in the genome of Drosophila melanogaster strain w1118; iso-2; iso-3. Fly 6 (2), 80–92. doi: 10.4161/fly.19695
Dai, L. P., Lu, X. L., Zou, W. W., Wang, C. J., Shen, L., Hu, J., et al. (2020). Mapping of QTLs for source and sink associated traits under elevated CO2 in rice (Oryza sativa L.). Plant Growth Regul. 90 (2), 359–367. doi: 10.1007/s10725-019-00564-5
Dang, X., Thi, T. G. T., Dong, G., Wang, H., Edzesi, W. M., Hong, D. (2014). Genetic diversity and association mapping of seed vigor in rice (Oryza sativa L.). Planta 239 (6), 1309–1319. doi: 10.1007/s00425-014-2060-z
Du, H., Wu, N., Fu, J., Wang, S., Li, X., Xiao, J., et al. (2012). A GH3 family member, OsGH3-2, modulates auxin and abscisic acid levels and differentially affects drought and cold tolerance in rice. J. Exp. Bot. 63 (18), 6467–6480. doi: 10.1093/jxb/ers300
Fang, N., Xu, R., Huang, L., Zhang, B., Duan, P., Li, N., et al. (2016). SMALL GRAIN 11 controls grain size, grain number and grain yield in rice. Rice 9 (1), 64. doi: 10.1186/s12284-016-0136-z
Feng, Z., Li, L. B., Tang, M. Q., Liu, Q. B., Ji, Z. H., Sun, D. L., et al. (2022). Detection of stable elite haplotypes and potential candidate genes of boll weight across multiple encironments via GWAS in upland cotton. Front. Plant Sci. 13, 929168. doi: 10.3389/fpls.2022.929168
Fujino, K., Sekiguchi, H., Matsuda, Y., Sugimoto, K., Ono, K., Yano, M. (2008). Molecular identification of a major quantitative trait locus, qLTG3–1, controlling low-temperature germinability in rice. Proc. Natl. Acad. Sci. 105 (34), 12623–12628. doi: 10.1073/pnas.0805303105
Gallei, M., Luschnig, C., Friml, J. (2020). Auxin signalling in growth: Schrödinger’s cat out of the bag. Curr. Opin. Plant Bio. 53, 43–49. doi: 10.1016/j.pbi.2019.10.003
He, Y. Q., Cheng, J. P., He, Y., Yang, B., Cheng, Y. H., Yang, C., et al. (2019b). Influence of isopropylmalate synthase OsIPMS1 on seed vigour associated with amino acid and energy metabolism in rice. Plant Biotechnol. J. 17 (2), 322–337. doi: 10.1111/pbi.12979
He, Y., Chen, S., Liu, K., Chen, Y., Cheng, Y., Zeng, P., et al. (2022). OsHIPL1, a hedgehog-interacting protein-like 1 protein, increases seed vigour in rice. Plant Biotechnol. J. 20 (7), 1346–1362. doi: 10.1111/pbi.13812
He, Y., Yang, B., He, Y., Zhan, C., Cheng, Y., Zhang, J., et al. (2019a). A quantitative trait locus, qSE 3, promotes seed germination and seedling establishment under salinity stress in rice. Plant J. 97 (6), 1089–1104. doi: 10.1111/tpj.14181
Hong, Z., Ueguchi-Tanaka, M., Umemura, K., Uozu, S., Fujioka, S., Takatsuto, S., et al. (2003). A rice brassinosteroid-deficient mutant, ebisu dwarf (d2), is caused by a loss of function of a new member of cytochrome P450. Plant Cell 15 (12), 2900–2910. doi: 10.1105/tpc.014712
Hsieh, K. T., Chen, Y. T., Hu, T. J., Lin, S. M., Hsieh, C. H., Liu, S. H., et al. (2021). Comparisons within the rice GA 2-oxidase gene family revealed three dominant paralogs and a functional attenuated gene that led to the identification of four amino acid variants associated with GA deactivation capability. Rice 14 (1), 70. doi: 10.1186/s12284-021-00499-4
Islam, M. R., Naveed, S. A., Zhang, Y., Li, Z., Zhao, X., Fiaz, S., et al. (2022). Identification of candidate genes for salinity and anaerobic tolerance at the germination stage in rice by genome-wide association analyses. Front. Genet. 13, 822516. doi: 10.3389/fgene.2022.822516
Jahan, N., Javed, M. A., Khan, A., Manan, F. A., Tabassum, B. (2021). Genetic architecture of Al3+ toxicity tolerance in rice F2:3 populations determined through QTL mapping. Ecotoxicology 30 (5), 794–805. doi: 10.1007/s10646-021-02413-6
Jiang, S., Yang, C., Xu, Q., Wang, L., Yang, X., Song, X., et al. (2020). Genetic dissection of germinability under low temperature by building a resequencing linkage map in japonica rice. Int. J. Mol. Sci. 21 (4), 1284. doi: 10.3390/ijms21041284
Jin, J., Long, W., Wang, L., Liu, X., Pan, G., Xiang, W., et al. (2018). QTL mapping of seed vigor of backcross inbred lines derived from Oryza longistaminata under artificial aging. Front. Plant Sci. 9, 1909. doi: 10.3389/fpls.2018.01909
Kudahettige, N., Pucciariello, C., Parlanti, S., Alpi, A., Perata, P. (2011). Regulatory interplay of the Sub1A and CIPK15 pathways in the regulation of α-amylase production in flooded rice plants. Plant Biol. 13 (4), 611–619. doi: 10.1111/j.1438-8677.2010.00415.x
Lee, K. W., Chen, P. W., Lu, C. A., Chen, S., Ho, T. H. D., Yu, S. M. (2009). Coordinated responses to oxygen and sugar deficiency allow rice seedlings to tolerate flooding. Sci. Signal. 2 (91), ra61. doi: 10.1126/scisignal.2000333
Lee, T. H., Guo, H., Wang, X., Kim, C., Paterson, A. H. (2014). SNPhylo: a pipeline to construct a phylogenetic tree from huge SNP data. BMC Genom. 15, 162. doi: 10.1186/1471-2164-15-162
Li, H., Durbin, R. (2009). Fast and accurate short read alignment with burrows-wheeler transform. Bioinformatics 25 (14), 1754–1760. doi: 10.1093/bioinformatics/btp324
Li, Q., Lu, X., Wang, C., Shen, L., Dai, L., He, J., et al. (2022). Genome-wide association study and transcriptome analysis reveal new QTL and candidate genes for nitrogen-deficiency tolerance in rice. Crop J. 10 (4), 942–951. doi: 10.1016/j.cj.2021.12.006
Li, W., Yang, B., Xu, J., Peng, L., Sun, S., Huang, Z., et al. (2021a). A genome-wide association study reveals that the 2-oxoglutarate/malate translocator mediates seed vigor in rice. Plant J. 108 (2), 478–491. doi: 10.1111/tpj.15455
Li, L., Zhang, C., Huang, J., Liu, Q., Wei, H., Wang, H., et al. (2021b). Genomic analyses reveal the genetic basis of early maturity and identification of loci and candidate genes in upland cotton (Gossypium hirsutum l.). Plant Biotechnol. J. 19 (1), 109–123. doi: 10.1111/pbi.13446
Lo, S. F., Yang, S. Y., Chen, K. T., Hsing, Y. I., Zeevaart, J. A., Chen, L. J., et al. (2008). A novel class of gibberellin 2-oxidases control semidwarfism, tillering, and root development in rice. Plant Cell 20 (10), 2603–2618. doi: 10.1105/tpc.108.060913
Lu, Y., Ye, X., Guo, R., Huang, J., Wang, W., Tang, J., et al. (2017). Genome-wide targeted mutagenesis in rice using the CRISPR/Cas9 system. Mol. Plant 10 (9), 1242–1245. doi: 10.1016/j.molp.2017.06.007
Magome, H., Nomura, T., Hanada, A., Takeda-Kamiya, N., Ohnishi, T., Shinma, Y., et al. (2013). CYP714B1 and CYP714B2 encode gibberellin 13-oxidases that reduce gibberellin activity in rice. Proc. Natl. Acad. Sci. 110 (5), 1947–1952. doi: 10.1073/pnas.1215788110
Ma, Y., Yang, C., He, Y., Tian, Z., Li, J., Sunkar, R. (2017). Rice OVATE family protein 6 regulates plant development and confers resistance to drought and cold stresses. J. Exp. Bot. 68 (17), 4885–4898. doi: 10.1093/jxb/erx309
Murray, M., Thompson, W. (1980). Rapid isolation of high molecular weight plant DNA. Nucleic Acids Res. 8 (19), 4321–4326. doi: 10.1093/nar/8.19.4321
Najeeb, S., Ali, J., Mahender, A., Pang, Y., Zilhas, J., Murugaiyan, V., et al. (2020). Identification of main-effect quantitative trait loci (QTLs) for low-temperature stress tolerance germination-and early seedling vigor-related traits in rice (Oryza sativa L.). Mol. Breed. 40 (1), 1–25. doi: 10.1007/s11032-019-1090-4
Nijhawan, A., Jain, M., Tyagi, A. K., Khurana, J. P. (2008). Genomic survey and gene expression analysis of the basic leucine zipper transcription factor family in rice. Plant Physiol. 146 (2), 333–350. doi: 10.1104/pp.107.112821
Pandian, B. A., Sathishraj, R., Djanaguiraman, M., Prasad, P. V., Jugulam, M. (2020). Role of cytochrome P450 enzymes in plant stress response. Antioxidants 9 (5), 454. doi: 10.3390/antiox9050454
Peng, L., Sun, S., Yang, B., Zhao, J., Li, W., Huang, Z., et al. (2022). Genome-wide association study reveals that the cupin domain protein OsCDP3.10 regulates seed vigour in rice. Plant Biotechnol. J. 20 (3), 485–498. doi: 10.1111/pbi.13731
Piao, H. L., Xuan, Y. H., Park, S. H., Je, B. I., Park, S. J., Park, S. H., et al. (2010). OsCIPK31, a CBL-interacting protein kinase is involved in germination and seedling growth under abiotic stress conditions in rice plants. Mol. Cells 30 (1), 19–27. doi: 10.1007/s10059-010-0084-1
Purcell, S., Neale, B., Todd-Brown, K., Thomas, L., Ferreira, M. A., Bender, D., et al. (2007). PLINK: a tool set for whole-genome association and population-based linkage analyses. Am. J. Hum. Genet. 81 (3), 559–575. doi: 10.1086/519795
Rajjou, L., Duval, M., Gallardo, K., Catusse, J., Bally, J., Job, C., et al. (2012). Seed germination and vigor. Annu. Rev. Plant Biol. 63, 507–533. doi: 10.1146/annurev-arplant-042811-105550
Schmitz, A. J., Begcy, K., Sarath, G., Walia, H. (2015). Rice ovate family protein 2 (OFP2) alters hormonal homeostasis and vasculature development. Plant Sci. 241, 177–188. doi: 10.1016/j.plantsci.2015.10.011
Shabala, S., Alnayef, M., Bose, J., Chen, Z. H., Venkataraman, G., Zhou, M., et al. (2021). Revealing the role of the calcineurin b-like protein-interacting protein kinase 9 (CIPK9) in rice adaptive responses to salinity, osmotic stress, and k+ deficiency. Plants 10 (8), 1513. doi: 10.3390/plants10081513
Shi, J., Shi, J., Liang, W., Zhang, D. (2021). Integrating GWAS and transcriptomics to identify genes involved in seed dormancy in rice. Theor. Appl. Genet. 134 (11), 3553–3562. doi: 10.1007/s00122-021-03911-1
Takehara, S., Sakuraba, S., Mikami, B., Yoshida, H., Yoshimura, H., Itoh, A., et al. (2020). A common allosteric mechanism regulates homeostatic inactivation of auxin and gibberellin. Nat. Commun. 11, 2143. doi: 10.1038/s41467-020-16068-0
Tamiru, M., Undan, J. R., Takagi, H., Abe, A., Yoshida, K., Undan, J. Q., et al. (2015). A cytochrome P450, OsDSS1, is involved in growth and drought stress responses in rice (Oryza sativa L.). Plant Mol. Biol. 88 (1), 85–99. doi: 10.1007/s11103-015-0310-5
Tang, R. J., Wang, C., Li, K., Luan, S. (2020). The CBL-CIPK calcium signaling network: unified paradigm from 20 years of discoveries. Trends Plant Sci. 25 (6), 604–617. doi: 10.1016/j.tplants.2020.01.009
Tong, H., Xiao, Y., Liu, D., Gao, S., Liu, L., Yin, Y., et al. (2014). Brassinosteroid regulates cell elongation by modulating gibberellin metabolism in rice. Plant Cell 26 (11), 4376–4393. doi: 10.1105/tpc.114.132092
Van der Auwera, G. A., Carneiro, M. O., Hartl, C., Poplin, R., Del Angel, G., Levy-Moonshine, A., et al. (2013). From FastQ data to high-confidence variant calls: the genome analysis toolkit best practices pipeline. Curr. Proto. Bioinf. 43 (1), 11.10. 1–11.10. 33. doi: 10.1002/0471250953.bi1110s43
Vogt, F., Shirsekar, G., Weigel, D. (2022). vcf2gwas: Python API for comprehensive GWAS analysis using GEMMA. Bioinformatics 38 (3), 839–840. doi: 10.1093/bioinformatics/btab710
Wang, Z. F., Wang, J. F., Bao, Y. M., Wang, F. H., Zhang, H. S. (2010). Quantitative trait loci analysis for rice seed vigor during the germination stage. J. Zhejiang Univ. Sci. B. 11 (12), 958–964. doi: 10.1631/jzus.B1000238
Wu, J., Zhu, C., Pang, J., Zhang, X., Yang, C., Xia, G., et al. (2014). OsLOL1, a C2C2-type zinc finger protein, interacts with OsbZIP58 to promote seed germination through the modulation of gibberellin biosynthesis in Oryza sativa. Plant J. 80 (6), 1118–1130. doi: 10.1111/tpj.12714
Xiang, Y., Huang, Y., Xiong, L. (2007). Characterization of stress-responsive CIPK genes in rice for stress tolerance improvement. Plant Physiol. 144 (3), 1416–1428. doi: 10.1104/pp.107.101295
Xiao, Y., Liu, D., Zhang, G., Tong, H., Chu, C. (2017). Brassinosteroids regulate OFP1, a DLT interacting protein, to modulate plant architecture and grain morphology in rice. Front. Plant Sci. 8, 1698. doi: 10.3389/fpls.2017.01698
Xiao, Y., Zhang, G., Liu, D., Niu, M., Tong, H., Chu, C. (2020). GSK2 stabilizes OFP3 to suppress brassinosteroid responses in rice. Plant J. 102 (6), 1187–1201. doi: 10.1111/tpj.14692
Xie, L., Tan, Z., Zhou, Y., Xu, R., Feng, L., Xing, Y., et al. (2014). Identification and fine mapping of quantitative trait loci for seed vigor in germination and seedling establishment in rice. J. Integr. Plant Biol. 56 (8), 749–759. doi: 10.1111/jipb.12190
Xu, J., Wang, X. Y., Guo, W. Z. (2015). The cytochrome P450 superfamily: Key players in plant development and defense. J. Integr. Agr. 14 (9), 1673–1686. doi: 10.1016/S2095-3119(14)60980-1
Yang, B., Chen, M., Zhan, C., Liu, K., Cheng, Y., Xie, T., et al. (2022). Identification of OsPK5 involved in rice glycolytic metabolism and GA/ABA balance for improving seed germination via GWAS. J. Exp. Bot. 11 (2), 3446–3461. doi: 10.1093/jxb/erac071
Yang, W., Guo, Z., Huang, C., Duan, L., Chen, G., Jiang, N., et al. (2014). Combining high-throughput phenotyping and genome-wide association studies to reveal natural genetic variation in rice. Nat. Commun. 5, 5087. doi: 10.1038/ncomms6087
Yang, W., Kong, Z., Omo-Ikerodah, E., Xu, W., Li, Q., Xue, Y. (2008). Calcineurin b-like interacting protein kinase OsCIPK23 functions in pollination and drought stress responses in rice (Oryza sativa L.). J. Genet. Genomics 35 (9), 531–543. doi: 10.1016/S1673-8527(08)60073-9
Yang, C., Ma, Y., He, Y., Tian, Z., Li, J. (2018). OsOFP19 modulates plant architecture by integrating the cell division pattern and brassinosteroid signaling. Plant J. 93 (3), 489–501. doi: 10.1111/tpj.13793
Yang, C., Shen, W., He, Y., Tian, Z., Li, J. (2016). OVATE family protein 8 positively mediates brassinosteroid signaling through interacting with the GSK3-like kinase in rice. PloS Genet. 12 (6), e1006118. doi: 10.1371/journal.pgen.1006118
Yuan, Z., Fan, K., Wang, Y., Tian, L., Zhang, C., Sun, W., et al. (2021). OsGRETCHENHAGEN3-2 modulates rice seed storability via accumulation of abscisic acid and protective substances. Plant Physiol. 186 (1), 469–482. doi: 10.1093/plphys/kiab059
Yu, G., Smith, D. K., Zhu, H., Guan, Y., Lam, T. T. Y. (2017). Ggtree: an r package for visualization and annotation of phylogenetic trees with their covariates and other associated data. Methods Ecol. Evol. 8 (1), 28–36. doi: 10.1111/2041-210X.12628
Zhang, A., Liu, C., Chen, G., Hong, K., Gao, Y., Tian, P., et al. (2017). Genetic analysis for rice seedling vigor and fine mapping of a major QTL qSSL1b for seedling shoot length. Breed. Sci. 67 (3), 307–315. doi: 10.1270/jsbbs.16195
Zhang, Y., Wang, X., Luo, Y., Zhang, L., Yao, Y., Han, L., et al. (2020). OsABA8ox2, an ABA catabolic gene, suppresses root elongation of rice seedlings and contributes to drought response. Crop J. 8 (3), 480–491. doi: 10.1016/j.cj.2019.08.006
Zhou, J., Li, Z., Xiao, G., Zhai, M., Pan, X., Huang, R., et al. (2020). CYP71D8L is a key regulator involved in growth and stress responses by mediating gibberellin homeostasis in rice. J. Exp. Bot. 71 (3), 1160–1170. doi: 10.1093/jxb/erz491
Zhu, S., Gao, F., Cao, X., Chen, M., Ye, G., Wei, C., et al. (2005). The rice dwarf virus P2 protein interacts with ent-kaurene oxidases in vivo, leading to reduced biosynthesis of gibberellins and rice dwarf symptoms. Plant Physiol. 139 (4), 1935–1945. doi: 10.1104/pp.105.072306
Zhu, Y., Nomura, T., Xu, Y., Zhang, Y., Peng, Y., Mao, B., et al. (2006). ELONGATED UPPERMOST INTERNODE encodes a cytochrome P450 monooxygenase that epoxidizes gibberellins in a novel deactivation reaction in rice. Plant Cell 18 (2), 442–456. doi: 10.1105/tpc.105.038455
Keywords: rice, GWAS, seed vigor, direct seeding, haplotype
Citation: Dai L, Lu X, Shen L, Guo L, Zhang G, Gao Z, Zhu L, Hu J, Dong G, Ren D, Zhang Q, Zeng D, Qian Q and Li Q (2022) Genome-wide association study reveals novel QTLs and candidate genes for seed vigor in rice. Front. Plant Sci. 13:1005203. doi: 10.3389/fpls.2022.1005203
Received: 28 July 2022; Accepted: 13 October 2022;
Published: 26 October 2022.
Edited by:
Ryuji Ishikawa, Hirosaki University, JapanReviewed by:
Tian Qing Zheng, Institute of Crop Sciences (CAAS) ChinaWei Zong, Indiana University, United States
Jianmin Bian, Jiangxi Agricultural University, China
Xin-Yuan Huang, Nanjing Agricultural University, China
Yoshiaki Ueda, Japan International Research Center for Agricultural Sciences (JIRCAS), Japan
Jiang Shukun, Heilongjiang Academy of Agricultural Sciences, China
Copyright © 2022 Dai, Lu, Shen, Guo, Zhang, Gao, Zhu, Hu, Dong, Ren, Zhang, Zeng, Qian and Li. This is an open-access article distributed under the terms of the Creative Commons Attribution License (CC BY). The use, distribution or reproduction in other forums is permitted, provided the original author(s) and the copyright owner(s) are credited and that the original publication in this journal is cited, in accordance with accepted academic practice. No use, distribution or reproduction is permitted which does not comply with these terms.
*Correspondence: Dali Zeng, ZGFsaXplbmdAMTI2LmNvbQ==; Qian Qian, cWlhbnFpYW4xODhAaG90bWFpbC5jb20=; Qing Li, bGlxaW5nMTk4NjEwMkAxNjMuY29t
†These authors have contributed equally to this work