- College of Agronomy and Biotechnology, Southwest University, Chongqing, China
Fiber quality and yield-related traits are important agronomic traits in cotton breeding. To detect the genetic basis of fiber quality and yield related traits, a recombinant inbred line (RIL) population consisting of 182 lines was established from a cross between Gossypium hirsutum cultivar CCRI35 and G. hirsutum race palmeri accession TX-832. The RIL population was deeply genotyped using SLAF-seq and was phenotyped in six environments. A high-density genetic linkage map with 15,765 SNP markers and 153 SSR markers was constructed, with an average distance of 0.30 cM between adjacent markers. A total of 210 fiber quality quantitative trait loci (QTLs) and 73 yield-related QTLs were identified. Of the detected QTLs, 62 fiber quality QTLs and 10 yield-related QTLs were stable across multiple environments. Twelve and twenty QTL clusters were detected on the At and Dt subgenome, respectively. Twenty-three major QTL clusters were further validated through associated analysis and five candidate genes of four stable fiber quality QTLs were identified. This study revealed elite loci influencing fiber quality and yield and significant phenotypic selection regions during G. hirsutum domestication, and set a stage for future utilization of molecular marker assisted breeding in cotton breeding programs.
Introduction
Cotton, with more than 7,000 years of cultivation history, is the most significant natural fiber crop and an important resource of plant protein and oil (Yuan et al., 2015; Wang M. et al., 2017). Besides textile fiber, cotton seeds could be processed into foodstuff, feed, and biofuel (Coppock et al., 1987; Liu et al., 2002, 2017). The economic impact of cotton is estimated to be approximately 500 billion dollars per year worldwide (Chen et al., 2007). With the rapid development of spinning industry and the increasing human demand, enhancing the fiber quality and yield has been a crucial goal of cotton breeding (Fang X. et al., 2017).
Gossypium hirsutum (upland cotton), which has experienced a long period of domestication for its high yield traits (Zhang et al., 2005; Hu et al., 2019), provides more than 90% of global cotton output. However, the genetic diversity of G. hirsutum cultivars are narrow and thus decreased the opportunities for identifying favorable alleles and breeding new cotton cultivars with more eminent yield and quality (Zhang et al., 2013; Okubazghi et al., 2017; Li et al., 2019). G. hirsutum races, also known as semiwild accessions, are primitive type of cultivated upland cotton (Hutchinson, 1951; Lacape et al., 2006; Feng et al., 2019). G. hirsutum races are classified into seven races (yucatanense, marie-galante, morrlli, richmondii, punctatum, palmeri and latifolium) according to their geographical distribution (Brubaker et al., 1999; Lacape et al., 2006). They were confirmed with promising phenotypes, including resistance to biotic stress and abiotic stress, and agronomically important traits such as variable fiber quality and yield traits (Okubazghi et al., 2017; Zhang et al., 2019). G. hirsutum races could be crossed with cultivars directly and therefore were gradually utilized to broaden the genetic basis of modern cotton cultivars and improve fiber quality and yield-related traits (Wang Y. et al., 2016; Okubazghi et al., 2017; Feng et al., 2019).
Yield-related and fiber quality traits are complex quantitative traits that were controlled by multiple genes and were affected by environments (Paterson et al., 2003; Said et al., 2013). The negative relation between fiber quality and yield traits limited the efficiency of conventional breeding methods (Yu et al., 2012; Zhang et al., 2014). A majority of previous quantitative trait locus (QTL) studies for fiber quality and yield-related traits were based on upland cotton intraspecific population and interspecific, whereas a few of them were conducted with G. hirsutum race accessions (Zhang et al., 2016; Feng et al., 2019). In this work, an accession TX-832 from G. hirsutum race palmeri, which is distributed in the Mexican States of Guerrero and Oaxaca and is characterized with distinctive laciniate leaves and small bolls, was utilized to detect elite loci influencing fiber quality and yield-related traits (Brubaker et al., 1999). A recombinant inbred line (RIL) population containing 182 lines from a cross between G. hirsutum cultivar CCRI35 and G. hirsutum race palmeri accession TX-832 were established. Single nucleotide polymorphism (SNP) markers obtained from Specific-Locus Amplified Fragment Sequencing (SLAF-seq) were applied to construct a high-density genetic linkage map. Polymorphic simple sequence repeats (SSR) markers were utilized to verify the accuracy of SNP markers and enrich the genetic map. Environment-stable QTLs and QTL clusters for fiber quality and yield-related traits were detected based on this genetic map. Major QTL clusters were further validated by SNP associated analysis, and five candidate genes related to fiber quality were identified. This work provided elite loci influencing fiber quality, yield-related traits, and valuable insights into the phenotypic selection regions during G. hirsutum domestication. The results built the basis for breeding cotton cultivars with high fiber quality and yield.
Materials and Methods
Mapping Population
The G. hirsutum cultivar CCRI35 and the G. hirsutum race palmeri accession TX-832 were crossed at Southwest University, Chongqing, China, in the summer of 2016. CCRI35 has fine performance in yield and was widely planted in China in the 2000s. TX-832, which was provided by Chinese cotton research academy, is characterized with features such as okra leaf and small boll. The F1 seeds were planted at Hainan, China, in the winter of 2016. One hundred and eighty-two F2 individuals were randomly selected at Chongqing in the subsequent summer, and then single-seed descent was conducted from the F2:3 generation to the F2:6 generation to complete a RIL population in the summer of 2019. The RIL population, as well as the two parents, were planted in totally six environments in China, including Beibei in Chongqing province (19CQ), Changsha in Hunan province (19CS), Sanya in Hainan province (19SY), and Kuitun in Xinjiang province (19KT) in 2019, Kuitun (20KT) and Kuerle (20KL) in Xinjiang province in 2020.
Phenotyping and Analysis
All the mature bolls of the 182 lines and the two parents were hand-harvested in each environment. Their fiber samples were evaluated for five fiber quality traits, including fiber length (FL, mm), fiber micronaire (FM), fiber uniformity ratio (FU,%), fiber strength (FS, cN/tex), and fiber elongation (FE,%), using the high volume instrument (HIV) system at the Supervision Inspection and Testing Cotton Quality Center, Anyang, China. Three yield-related traits, including boll weight (BW, g), seed index (g), and lint percentage (LP,%) were collected from all environments. Besides, the yield-related traits lint index (LI, g) was also tested in the three environments. Microsoft Excel 2019 was applied to analysis the variance of each trait. SPSS 20.0 was used to analyze the correlations among traits. The R package “ggplot2” was applied to draw the visualized plots of traits performance and correlations in each environment.
DNA Extraction and Specific-Locus Amplified Fragment Library Construction
Total genomic DNA of the two parents and the RIL population were extracted using modified CTAB method as described by Zhang et al. (2005). For SLAF-Seq library construction, two endonucleases HaeIII and Hpy166II were finally chosen, based on the in silico analysis of G. hirsutum reference genome (TM-1, V2.1) (Hu et al., 2019), to digest the genomic DNA. SLAF labels with length of 414–464 bp were chosen for library construction and sequencing. The procedures of quality control, reads filter and trim, reads mapping and SNPs detection were carried out, as described by Wang et al. (2019).
Marker Filtration and Map Construction
Single nucleotide polymorphisms in each SLAF locus were filtered as described by Wang et al. (2019). SSR markers were obtained previously in our laboratory by polymorphism screening between the two parents. The genetic map was constructed by Joinmap 4.0 using both SLAF markers and SSR markers (Van Ooijen, 2006). Chip-squared tests were applied to detected markers that deviated significantly from the expected Mendelian 1:1 segregation ratio with their p-value < 0.05. Regions that contained more than three adjacent significant segregation distorted loci were determined as segregation distortion region (SDR) (Liu et al., 2017).
Quantitative Trait Loci Identification
Identification of QTLs and evaluation of their effects were carried out by Interval Mapping methods in MapQTL 6.0 (Van Ooijen, 2009). The threshold of odds ratio (LOD) to determine QTL was set to 3.0. QTLs identified in at least two (LI) or at least three (BW, SI, LP, FL, FS, FM, FU, and FE) environments were considered to be stable QTLs. A region contained more than three QTLs was determined as QTL cluster, and clusters that contained QTLs with their PVE value larger than 10% and LOD value larger than 5.0 were determined as major QTL clusters.
Candidate Gene Identification
The physical regions of QTLs within the G. hirsutum reference genome (TM-1, V2.1) were determined by physical locations of flanking markers of their genetical confidence interval.
Transcriptome sequencing data from G. hirsutum TM-1 ovules including 0 days post-anthesis (DPA) and 5 DPA, and fibers at 10 DPA, 15 DPA and 25 DPA were acquired from the Cotton Omics Database1.
DNA libraries for whole genome re-sequencing were created for the CCRI35 and TX-832 and sequenced in an Illumina HiSeq platform. The procedures of reads mapping and SNP/Indel calling were carried out as described by Liu et al. (2020). Variations annotation was performed according the G. hirsutum reference genome (TM-1, V2.1) with the ANNOVAR package (Wang et al., 2010).
Associated Analysis
The association analysis was conduct with public data released by Ma Z. et al. (2018). SNP Markers in major QTL clusters were firstly converted to their corresponding position of the reference genome (TM-1, V1.1) (Zhang et al., 2015). Genotype of common SNPs (SNPs sharing the same genome position with the present study) and phenotype data of 419 G. hirsutum accessions from study of Ma Z. et al. (2018) were analyzed. The software PLINK 2.0 (Chang et al., 2015) was applied to carry out association analysis.
Results
Phenotypic Variation and Correlation of Traits
The descriptive statistics of all traits for the two parents and the RIL population were summarized in Supplementary Table 1 and displayed in Figure 1A. CCRI35 performed more superiorly than TX-832 in all yield related traits and most fiber quality traits including fiber length, fiber strength and fiber uniformity. The fiber micronaire performance of TX-832 was better than CCRI35, whereas the fiber elongation traits were comparable between the two parents. All the traits were continuous and approximately normal distributed, indicating that they are typical quantitative traits. Furthermore, transgressive segregation was observed for all fiber quality and yield related traits.
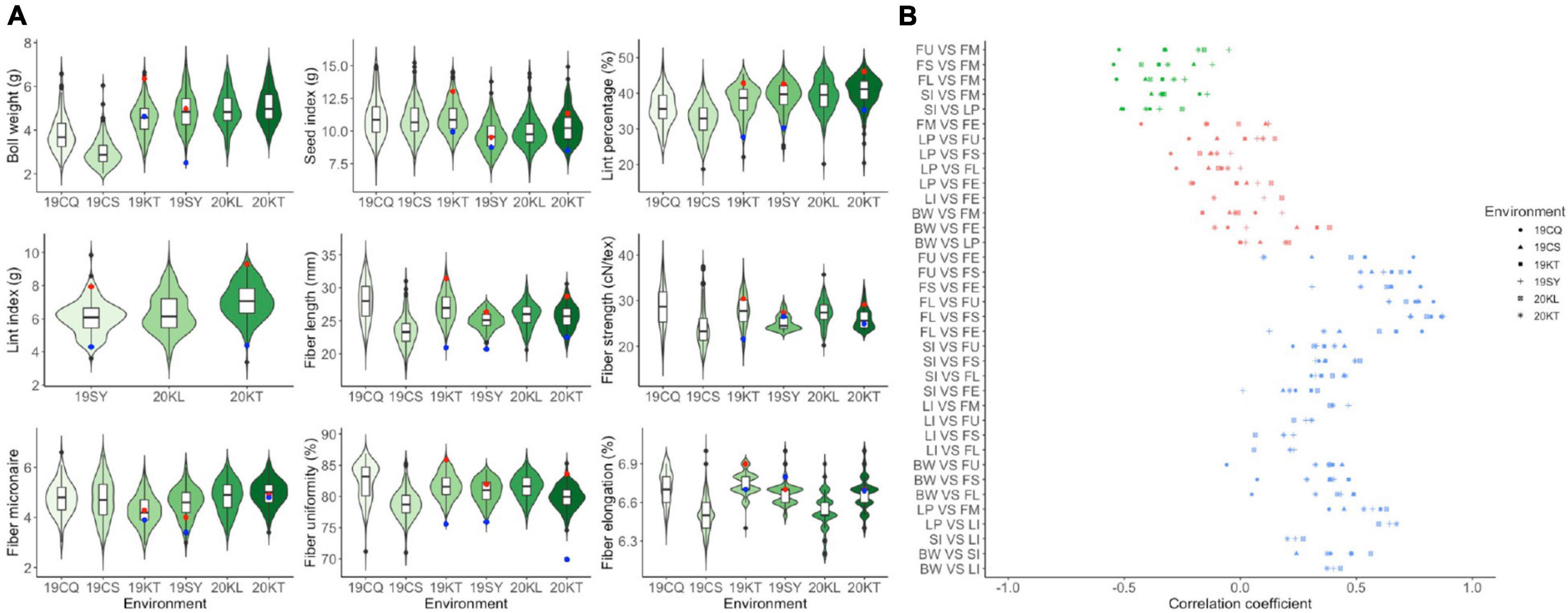
Figure 1. The phenotype performance (A) and the correlation analysis (B) among fiber quality and yield-related traits. Red and blue point in figure a suggesting the trait performance of CCRI35 and TX-832, respectively.
All the four yield related traits and the five fiber quality traits varied with environments (Figure 1A). The result of ANOVA also showed that all traits were significantly affected by both genetic and environmental factors (Supplementary Table 2).
Correlation analysis between traits pairs across all the environments was carried out separately (Supplementary Table 3). Three categories of correlativity were observed including significantly positive related, significantly negatively related and weakly related (Figure 1B). Among them, BW, SI, FL, FS were positively correlated with most traits except for LP and FM. FM was significantly positive correlated with LP and LI, whereas was negatively correlated with the other traits. LI was almost positively correlated with all the other traits except for FE.
Analysis of Specific-Locus Amplified Fragment-Seq Data and Specific-Locus Amplified Fragment Labels
A total of 2,060.13 M paired-end reads (including 18.50 M reads for CCRI35, 166.97 M reads for TX-832 and the others for the RIL population) were generated from the SLAF library, with 95.50% of the bases were of high quality with Q30 and the average GC content was 37.81%. Totally, among the 400,313 SLAFs, 260,123 SLAFs located in the At subgenome and 129,614 SLAFs located in the Dt subgenome. The number of SLAFs of CCRI35 and TX-832 were 397,774 and 397,252, with average sequencing depth were 31.29-fold and 351.25-fold, respectively. For individuals in the RIL population, the SLAF numbers ranged from 302,115 to 398,465, the average sequencing depth ranged from 9.93-fold to 95.92-fold. Among the developed SLAFs on 26 chromosomes, 195,449 were polymorphic with a polymorphism rate of 50.15% (Supplementary Table 4 and Supplementary Figure 1). Chromosome A01, A06, A10 and D03 harbored the more than 60% polymorphic SLAFs, while the polymorphism rate of chromosome A04, with a number of 23.60%, was the least.
Genotyping and Construction of the Genetic Map
A total of 2,962,929 SNP markers were generated from these SLAFs, and 82,920 SNPs with genotype aa × bb were remained after filtering. These SNPs were further filtered with criteria including minimum read depth of parents less than 10, minimum read depth of offspring less than two and more than 25% missing data. Finally, the offspring lines were genotyped with aa, ab and bb using 15,765 filtered SNP markers to constructed the genetic linkage map. The density of these SNPs on the G. hirsutum genome was displayed in Figure 2A.
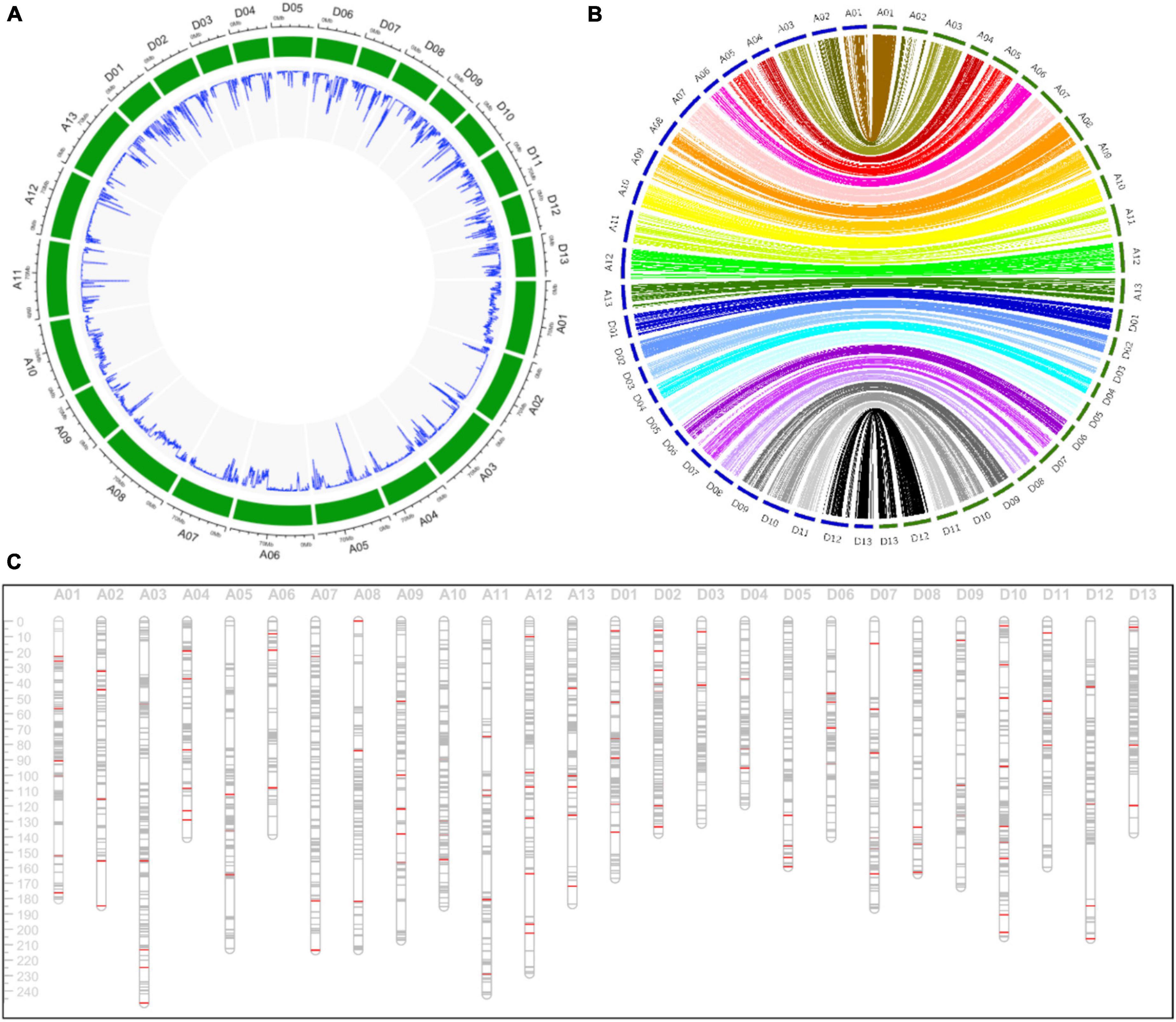
Figure 2. Detailed information about the genetic linkage map. (A) The density of SNP markers in the G. hirsutum genome in a 500 Kb window. (B) The collinearity analysis of markers between the genetic map and the physical map. The blue circle and the green circle indicating the genetic map and the physical map, respectively. (C) The distribution of markers in the genetic map. The gray lines and the red lines indicating SNP and SSR markers, respectively.
Simple sequence repeat primers were screened with polymorphism between CCRI35 and TX-832 to constructed a genetic linkage map using (CCRI35 × TX-832) F2 population previously in our laboratory. In this study, 153 polymorphic SSR markers distributed on all the 26 chromosomes were chosen to obtain the genotype of the RIL population and further verified the genotype of SNP markers. Names of these SSR markers were listed in Supplementary Table 5.
A high-density consensus genetic map including 15,765 SNP markers and 153 SSR markers were constructed, with a total length of 4,665.59 cM and an average distance of 0.30 cM between adjacent markers (Table 1). The At subgenome spanned 2,578.69 cM with 8,829 markers (8,750 SNP markers and 79 SSR markers), and the Dt subgenome spanned 2,086.90 cM with 7,015 SNP markers and 74 SSR markers. Chromosome A01, spanning 180.44 cM, contained the maximum markers including 1,327 SNP and 8 SSR markers. While chromosome D05 harbored only 94 SNP and 5 SSR markers with genetic length of 159.35 cM. Chromosome A03 and D04 was the longest and the shortest chromosome, respectively.
Collinearity analysis indicating that most of markers on the genetic map were in accordance with the physical map (Figure 2B). The density of markers in the physical and genetic map indicated the high-coverage and uneven distribution of markers throughout the whole genome (Figures 2A,C).
Analysis of Segregation Distortion Regions
A total of 80 SDRs distributed on 26 chromosomes were detected, and only five of these SDRs (locating on chromosome A01, A08, A09, D08 and D10) favored TX-832 alleles, whereas the remaining favored CCRI35 alleles (Supplementary Figure 2 and Supplementary Table 6). Remarkably, the whole chromosome of A10 (SDRA10.1) and D02 (SDRD02.1), and 95.03% of chromosome A03 (SDRA03.1) were significantly skewed toward CCRI35. In addition, the interval of other SDRs that skewed toward CCRI35 ranged from 1.16 to 114.94 cM, and their chromosome coverage ranged from 0.54 to 61.61%. As for SDRs skewed toward TX-832, their interval ranged from 5.40 to 16.19 cM, and their chromosome coverage ranged from 2.63 to 8.73%. This result suggests that fragments from the cultivar CCRI35 are more predominant than the race accession TX-832.
Quantitative Trait Loci Identification for Fiber Quality and Yield-Related Traits
Totally, 61 FL QTLs, 62 FS QTLs, 12 FM QTLs, 50 FU QTLs, and 25 FE QTLs were identified based on six environments (Supplementary Table 7). Among them, 96 QTLs were located in the At subgenome and 114 QTLs were located in the Dt subgenome. The additive effect of five FL QTLs, three FS QTLs, seven FM QTLs, and five FE QTLs were conferred by TX-832, while the other fiber quality QTLs were contributed by CCRI35. Twenty-three FL QTLs, 12 FS QTLs, two FM QTLs, 13 FU QTLs, and two FE QTLs were detected in at least three environments. Except for qFM_D12.1, the additive effect origin of the other stable fiber quality QTLs were all derived from CCRI35.
A total of 73 QTLs (43 on the At subgenome and 30 on the Dt subgenome) for yield-related traits were identified, including 37 QTLs for BW, 15 QTLs for SI, 12 QTLs for LP, and 9 QTLs for LI (Supplementary Table 8). Three BW QTLs and three LP QTLs were detected in three or more environments, and four LI QTLs were identified in two environments. Especially, qLP_D03.1 were detected in all the tested environments. The favorable alleles of all these stable yield-related QTLs were contributed by CCRI35.
The distribution of all identified QTLs are shown in Figure 3. To summarize, QTLs unevenly distributed on 26 chromosomes, with Chr.D10 harbored the maximum QTL (20 QTLs), followed by Chr.A08 and Chr.A12 (18 QTLs), while Chr.A04, Chr.A06, and Chr.D06 possessed only two QTLs, respectively.
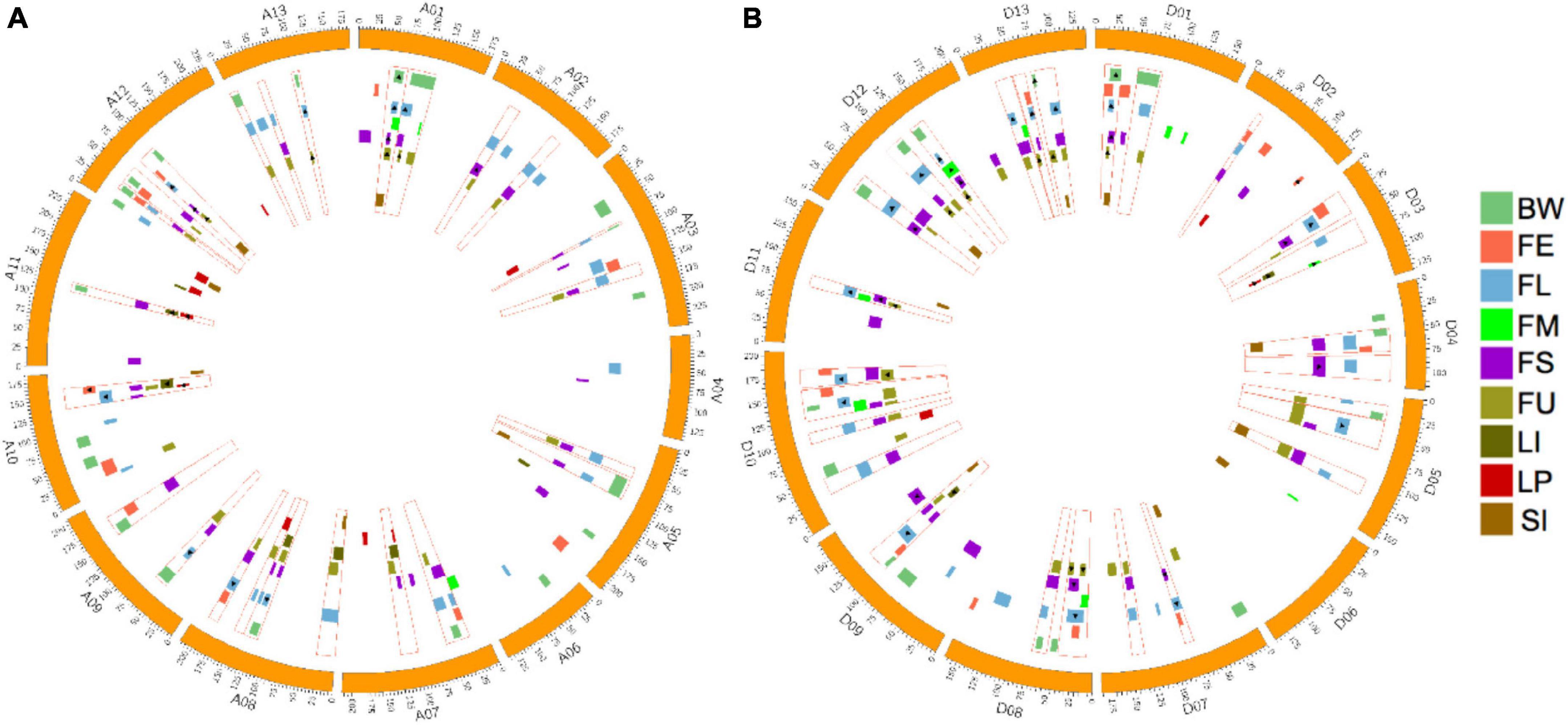
Figure 3. Distribution of QTLs for fiber quality and yield related traits and QTL clusters in the genetic map. (A) Distribution of QTLs and QTL clusters in the At subgenome. (B) Distribution of QTLs and QTL clusters in the Dt subgenome. The red rectangular frame indicating QTL clusters. The highlight rectangle indicating the confidence interval of each QTL, and the triangle indicating that the QTL is stable across environments.
Quantitative Trait Loci Clusters Analysis
In this work, regions that contained three and more overlapped QTLs were determined to be QTL clusters. As a result, 24 QTL clusters in the At subgenome and 26 QTL clusters in the Dt subgenome were identified (Figure 3 and Supplementary Table 9). The number of clusters distributed on chromosomes ranged from one to four, while no cluster was located on Chr.A04, Chr.A06, and Chr.D06. The number of QTLs located in each cluster ranged from three to seven. A total of 213 QTLs, accounting for 75.5% of all QTLs, were contained in clusters. Twenty-seven QTL clusters, with 10 located on the At subgenome and 17 located on the Dt subgenome, contained at least one stable QTL. Totally, 59 stable QTLs were contained in QTL clusters. More detailed information about QTL clusters are summarized in Figure 3 and Supplementary Table 9.
Validation of Major Quantitative Trait Loci Clusters
Clusters that contained QTLs, of which PVE values larger than 10 and LOD values larger than five, were determined as major QTL clusters in this study. Totally 23 major QTL clusters were identified and analyzed (Supplementary Table 10). Eight major QTL clusters were located in the At subgenome and the other 15 were located in the Dt subgenome. SNP markers in these major QTL clusters that shared the same genome position with published SNPs data from the study of Ma Z. et al. (2018) were chosen to validate their association with target traits from 419 G. hirsutum accessions. Validation results of each cluster are displayed in Supplementary Table 10 and Figure 4. As a result, common SNPs are significantly associated with one or more target traits. Specially, SNPs that are located in the stable QTL regions showed extremely significant difference between alleles (Figure 4). For example, two environment-stable QTLs for yield-related traits (qLP_D03.1 and qLI_D03.1) were contained in Cluster_D03.2, and accessions with different SNP (D03_34508261) alleles showed distinctively phenotype performance of these two traits. Besides, in the Cluster_D11.1, which contained three stable QTL for fiber quality (qFL_D11.1, qFS_D11.2, and qFU_D11.1), different SNP (D11_23867554) alleles also performed distinctively phenotype in fiber length, fiber strength, and fiber uniformity. The validation result indicates that these major QTL clusters are important phenotype selection regions during cotton domestication, and some of them accounted for large percentage of the phenotypic variation.
Prediction of Candidate Genes in Stable Quantitative Trait Loci
In this study, four stable QTLs, with relatively narrow confidence interval, related to fiber length (qFL_A08.2, qFL_A12.3), micronaire (qFM_D03.1), and strength (qFS_D08.2) were carried out candidate gene prediction analysis. The confidence intervals of these stable QTLs were aligned to the physical genome to identify the corresponding genes. The public released RNA-seq data were analyzed. The expression levels of cell elongation stage (0–15 DPA) were focused for fiber length and secondary wall synthesis stage (15–25 DPA) were focused for fiber strength and micronaire, respectively. Together with the genome resequencing data of TX-832 and CCRI35 and the functional annotation of genes, candidate genes for the above stable QTLs were finally identified.
qFL_A08.2 was positioned at 103.7–104.6 Mb on chromosome A08, where 35 genes were located, and 18 of them were expressed in developing fibers. Among the expressed genes, only GH_A08G1613, encoding a Leucine-rich repeat protein kinase family protein (LRR-RLK), showed non-synonymous variations (a frameshift deletion and two single nucleotide variations) between TX-832 and CCRI35.
qFL_A12.3 was mapped to 77.9–79.4 Mb on chromosome A12, where 56 genes were located in this region with 30 of them expressed in the early stage of developing fibers. Eleven out of the 30 expressed genes harbored non-synonymous sequence variations between TX-832 and CCRI35. Among them, GH_A12G1155 (TGD4), which participates lipid transport process, was terminated early at the 153rd amino acid in TX-832.
For the qFM_D03.1, it was located in at an ∼1.6 Mb region with 63 annotated genes and 23 genes expressed in the secondary wall synthesis stage. Non-synonymous sequence variations were identified in two genes that participated in activities and processes including oxidoreductase activity (GH_D03G1329) and microtubule-based process (GH_D03G1338).
qFS_D08.2 was positioned at 5.2–5.7 Mb on chromosome D08, where 27 out of the total 47 genes were expressed in fibers of secondary wall synthesis stage. Among the 27 genes, eight genes harbored non-synonymous sequence variations between the two parents, and a gene that was involved in the brassinosteroid mediated signaling pathway (GH_D08G0503/BSL1) was identified.
Discussion
Gossypium hirsutum Race palmeri Accessions Contribute to Broaden the Genetic Diversity and Improve Fiber Micronaire of Cultivars
In this work, a total of 73 yield-related QTLs (10 stable QTLs) and 210 fiber quality QTLs (52 stable QTLs) were identified across six environments. In a previous work, Liu et al. (2017) established a G. hirsutum intraspecific RIL population and detected only 32 yield-related QTLs and 73 QTLs across six environments. Similarly, Jamshed et al. (2016) identified 165 fiber quality QTLs (47 stable QTLs) across 11 environments also using a G. hirsutum intraspecific RIL population. Overall, the number of total QTLs and stable QTLs in this work were more than previous analogous studies. Modern G. hirsutum cultivars had been domesticated in many traits including the grown habit, high yield, and superior fiber quality (Fang L. et al., 2017), causing narrow genetic basis among cultivars but rich genetic diversity between races and cultivars (Lacape et al., 2006). Besides, the difference in trait performance between mapping parents is also a causing reason of total QTL numbers. In this work, CCRI35 performed much better than TX-832 in almost all traits, except for fiber micronaire and fiber elongation. The great genetic diversity and significantly different phenotype between CCRI35 and TX-832 resulted in large amount of QTL alleles detected in this work. Therefore, G. hirsutum race accessions, which are both genetically and phenotypically diverse, could be utilized as resources to broaden the genetic diversity of cultivars, and further contributed to identify more favorable alleles related to important agronomic traits.
As an index to reflect the fitness and maturity of cotton fiber, fiber micronaire is one of the most significant characteristics of the textile industry. However, the complexity of fiber micronaire trait hindered its improvement in cotton breeding. On the one hand, fiber micronaire tends to variate widely across environments, and thus was excluded from many studies that focus on improving fiber quality (Wang B. et al., 2017; Ma J. et al., 2018; He et al., 2021). Compared with other fiber quality traits such as length and strength, the opportunity of detecting favorable alleles for fiber micronaire was decreased. On the other hand, the G. hirsutum cultivars have experienced a long period of domestication for high yield related traits, such as high lint percentage, which usually resulted in coarse fibers with high micronaire values (Song et al., 2021). In this work, the race accession TX-832 performed better than the cultivar CCRI35 in fiber micronaire in all environments. Different from other fiber quality traits, that most of their favorable alleles conferred by the cultivar CCRI35, five out of 12 FM QTLs were favorable in the race accession TX-832. TX-832 provides valuable genomic resources for the improvement of fiber micronaire. Among these QTLs, FM_D03.1 were identified in five environments and explained 10.2–33.8% of the phenotypic variation. This QTL allele from palmeri accession could be preferentially utilized to improve fiber micronaire of cultivars.
Major Quantitative Trait Loci Clusters and Common Quantitative Trait Loci Reveal Footprints of Cotton Domestication
In this work, 23 major QTL clusters were detected, and favorable alleles of QTLs (except for qFM_D03.1) in these clusters were all contributed by the cultivar parent CCRI35. This result suggests that these clusters are major domesticated regions during the evolution from G. hirsutum races to modern G. hirsutum cultivars. Eight major QTL clusters were located at the At subgenome whereas the other 15 were located in the Dt subgenome, suggesting that the Dt subgenome might have experienced stronger domestication and selection than the At subgenome, which was consistent with previous studies (Wang M. et al., 2017; Ma Z. et al., 2018). Associated studies of common SNPs in these regions verified major QTL clusters, but also suggested that unfavorable allele still exist in part of modern cultivars. These SNP markers could be applied as an effective molecular tool to select varieties with high yield and superior fiber quality in future cotton breeding.
A total of 72 stable QTLs were identified, and many of them had been reported in previous studies (Shen et al., 2005, 2019; Zhang et al., 2011; Su et al., 2013; Yu et al., 2013; Shang et al., 2015, 2016; Guo et al., 2018; Deng et al., 2019; Ma et al., 2019; Chen et al., 2020). By comparing the physical confidence interval of QTLs in different studies, 35 stable QTLs in this work were found sharing overlapped interval with previous reported QTL (Supplementary Table 11), and 15 of them were detected in more than one previous work. Among them, qFL_A10.3 was found to be the same with QTLs in other five works (Liang et al., 2013; Shao et al., 2014; Wang et al., 2015; Wang H. et al., 2016; Shi et al., 2019), and qFL_D11.1 and qLP_D03.1 were found to be the same with QTLs in other four works, respectively (Wang et al., 2013, 2020; Ning et al., 2014; Shi et al., 2015; Jamshed et al., 2016; Diouf et al., 2018; Ma J. et al., 2018). Six QTLs (qFE_D02.3, qFL_A08.5, qFL_D07.1, qFL_D13.3, qFS_D01.1, qFS_D07.1) were identified in other three previous works (Shen et al., 2006; Feng et al., 2015; Tang et al., 2015; Wang F. et al., 2016; Zhang et al., 2016). These common QTLs identified across different populations and environments suggest that they are important domesticated alleles with stable genetic effects, and will provide valuable reference for future marker-assisted breeding of cotton varieties with high-yield and eminent fiber quality (Shi et al., 2020).
Candidate Genes for Stable Quantitative Trait Loci
In this work, we focused on four stable QTLs for fiber length, strength, and micronaire, and five candidate genes were identified. In the confidence interval of qFL_A08.2, only GH_A08G1613, which encodes a leucine-rich repeat protein kinase family protein (LRR-RLK), was detected as the candidate gene. A few LRR-RLK genes have been suggested to be functional during fiber development in cotton researches (Islam et al., 2016; Fang X. et al., 2017; Sun et al., 2018). LRR–RLKs were previously reported to be involved in pathways that regulate cellulose deposition (Xu S. et al., 2008) and cell expansion (Dai et al., 2013). The regulation of GH_A08G1613 on fiber length might also occur through these two pathways. GH_A12G1155 was identified for qFL_A12.3, and it is a homolog of AT2G44640 (TRIGALACTOSYLDIACYLGLYCEROL-like protein, TGD4) in Arabidopsis. AtTGD4 was reported to be required for lipid transport (Xu C. et al., 2008). It has been demonstrated that during fiber elongation, continuous synthesis and transport of lipids and proteins are crucial for supporting the enlargement of vacuoles and plasma membrane (Wanjie et al., 2005). The length of fiber was significantly reduced in the lipid transfer protein GhLTPG1-knockdown cotton plants (Deng et al., 2016). In the accession TX-832, a single nucleotide variation resulted in the early termination of GH_A12G1155 protein, suggesting that it might affect the lipid transport pathway and further influence fiber length.
For qFM_D03.1, two genes were identified with non-synonymous sequence variations between TX-832 and CCRI35, including the probable NAD(P)H dehydrogenase (quinone) FQR1-like 3 (GH_D03G1329) and tubulin beta chain 3 (GH_D03G1338). NAD(P)H dehydrogenase was involved in the oxidoreductase activity that was associated with reactive oxygen species (ROS) metabolism, and ROS levels were known to play vital roles in fiber elongation and secondary cell wall synthesis (Wang M. et al., 2017). Tubulin proteins are the major structural components of microtubules which undergo several phases of reorganization during cotton fiber development (Seagull, 1992). Microtubules are core elements of the cytoskeleton and are closely related to cellulose deposition and secondary cell wall development in fibers (Deng et al., 2016; Chen et al., 2021).
Phytohormones play vital roles in regulating cotton fiber development (Xiao et al., 2019). Brassinosteroid (BR) signaling has been reported to affect the deposition of cellulose into secondary cell wall of cotton fibers, and increasing the expression of the a brassinosteroid receptor BRI1 produced cotton fibers with thicker secondary cell walls (Sun et al., 2015). GH_D08G0503 in the interval of qFS_D08.2 was annotated to encode a BR1 SUPPRESSOR 1 (BSU1)-like protein (BSL1). BSU1 are required for signal transduction from BR1 to downstream proteins (Kim et al., 2011). Therefore, BSL1 might play the same role as BSU1, and it affects fiber strength through participating in the BR signal pathway and further regulating the deposition of cellulose into secondary cell wall.
Based on the above analysis, five candidate genes related to fiber quality were identified. But further functional analysis, such as generating genetic modified cotton plants, is needed to be conducted to verify these candidate genes.
Data Availability Statement
The datasets presented in this study can be found in online repositories. The names of the repository/repositories and accession number(s) can be found below: https://www.ncbi.nlm.nih.gov/, PRJNA781434.
Author Contributions
ZZ designed and supervised the research. XL and LY performed the data analysis and wrote the manuscript. JW, ZG, ZT, and DJL established the population and participated in the field experiment. YQW, QL, and JY extracted the DNA for sequencing library construction. YLW, LC, DXL, and KG collected the phenotype data. All authors have read and approved the manuscript.
Funding
This work was supported financially by the National Natural Science Foundation of China (31871670 and 32001590).
Conflict of Interest
The authors declare that the research was conducted in the absence of any commercial or financial relationships that could be construed as a potential conflict of interest.
Publisher’s Note
All claims expressed in this article are solely those of the authors and do not necessarily represent those of their affiliated organizations, or those of the publisher, the editors and the reviewers. Any product that may be evaluated in this article, or claim that may be made by its manufacturer, is not guaranteed or endorsed by the publisher.
Supplementary Material
The Supplementary Material for this article can be found online at: https://www.frontiersin.org/articles/10.3389/fpls.2021.817748/full#supplementary-material
Footnotes
References
Brubaker, C. L., Bourland, F. M., and Wendel, J. F. (1999). “The origin and domestication of cotton,” in Cotton: Origin, History, Technology, and Production, eds C. W. Smith and J. T. Cothren (New York, NY: John Wiley & Sons, Inc), 22–27.
Chang, C. C., Chow, C. C., Tellier, L. C., Vattikuti, S., Purcell, S. M., and Lee, J. J. (2015). Second-generation PLINK: rising to the challenge of larger and richer datasets. Gigascience 4:7. doi: 10.1186/s13742-015-0047-8
Chen, B., Zhao, J., Fu, G., Pei, X., Pan, Z., Li, H., et al. (2021). Identification and expression analysis of Tubulin gene family in upland cotton. J. Cotton Res. 4:20.
Chen, Q., Wang, W., Wang, C., Zhang, M., Yu, J., Zhang, Y., et al. (2020). Validation of QTLs for fiber quality introgressed from gossypium mustelinum by selective genotyping. G3 10, 2377–2384. doi: 10.1534/g3.120.401125
Chen, Z., Scheffler, B. E., Dennis, E., Triplett, B. A., Zhang, T., Guo, W., et al. (2007). Toward sequencing cotton (Gossypium) genomes. Plant Physiol. 145, 1303–1310. doi: 10.1104/pp.107.107672
Coppock, C. E., Lanham, J. K., and Horner, J. I. (1987). A review of the nutritive-value and utilization of whole cottonseed, cottonseed meal and associated by-products by dairy-cattle. Anim. Feed. Sci. Tech. 18, 89–129.
Dai, N., Wang, W., Patterson, S. E., and Bleecker, A. B. (2013). The TMK subfamily of receptor-like kinases in Arabidopsis display an essential role in growth and a reduced sensitivity to auxin. PLoS One 8:e60990. doi: 10.1371/journal.pone.0060990
Deng, T., Yao, H., Wang, J., Wang, J., Xue, H., and Zuo, K. (2016). GhLTPG1, a cotton GPI-anchored lipid transfer protein, regulates the transport of phosphatidylinositol monophosphates and cotton fiber elongation. Sci. Rep. 6:26829. doi: 10.1038/srep26829
Deng, X., Gong, J., Liu, A., Shi, Y., Gong, W., Ge, Q., et al. (2019). QTL mapping for fiber quality and yield-related traits across multiple generations in segregating population of CCRI 70. J. Cotton Res. 2:13.
Diouf, L., Magwanga, R. O., Gong, W., He, S., Pan, Z., Jia, Y., et al. (2018). QTL mapping of fiber quality and yield-related traits in an intra-specific upland cotton using genotype by sequencing (GBS). Int. J. Mol. Sci. 19:441. doi: 10.3390/ijms19020441
Fang, L., Wang, Q., Hu, Y., Jia, Y., Chen, J., Liu, B., et al. (2017). Genomic analyses in cotton identify signatures of selection and loci associated with fiber quality and yield traits. Nat. Genet. 49, 1089–1098. doi: 10.1038/ng.3887
Fang, X., Liu, X., Wang, X., Wang, W., Liu, D., Zhang, J., et al. (2017). Fine-mapping qFS07.1 controlling fiber strength in upland cotton (Gossypium hirsutum L.). Theor. Appl. Genet. 130, 795–806. doi: 10.1007/s00122-017-2852-1
Feng, H., Guo, L., Wang, G., Sun, J., Pan, Z., He, S., et al. (2015). The negative correlation between fiber color and quality traits revealed by QTL analysis. PLoS One 10:e0129490. doi: 10.1371/journal.pone.0129490
Feng, L., Zhang, S., Xing, L., Yang, B., Gao, X., Xie, X., et al. (2019). QTL analysis for yield and fibre quality traits using three sets of introgression lines developed from three Gossypium hirsutum race stocks. Mol. Genet. Genomics 294, 789–810. doi: 10.1007/s00438-019-01548-w
Guo, L., Shi, Y., Gong, J., Liu, A., Tan, Y., Gong, W., et al. (2018). Genetic analysis of the fiber quality and yield traits in G. hirsutum background using chromosome segments substitution lines (CSSLs) from Gossypium barbadense. Euphytica 214:82.
He, S., Sun, G., Geng, X., Gong, W., Dai, P., Jia, Y., et al. (2021). The genomic basis of geographic differentiation and fiber improvement in cultivated cotton. Nat. Genet. 53, 916–924. doi: 10.1038/s41588-021-00844-9
Hu, Y., Chen, J., Fang, L., Zhang, Z., Ma, W., Niu, Y., et al. (2019). Gossypium barbadense and Gossypium hirsutum genomes provide insights into the origin and evolution of allotetraploid cotton. Nat. Genet. 51, 739–748. doi: 10.1038/s41588-019-0371-5
Hutchinson, J. B. (1951). Intra-specific differentiation in Gossypium-hirsutum. Heredity 5, 161–193.
Islam, M. S., Fang, D. D., Thyssen, G. N., Delhom, C. D., Liu, Y., and Kim, H. J. (2016). Comparative fiber property and transcriptome analyses reveal key genes potentially related to high fiber strength in cotton (Gossypium hirsutum L.) line MD52ne. BMC Plant Biol. 16:36. doi: 10.1186/s12870-016-0727-2
Jamshed, M., Jia, F., Gong, J., Palanga, K. K., Shi, Y., Li, J., et al. (2016). Identification of stable quantitative trait loci (QTLs) for fiber quality traits across multiple environments in Gossypium hirsutum recombinant inbred line population. BMC Genomics 17:197. doi: 10.1186/s12864-016-2560-2
Kim, T. W., Guan, S., Burlingame, A. L., and Wang, Z. (2011). The CDG1 kinase mediates brassinosteroid signal transduction from BRI1 receptor kinase to BSU1 phosphatase and GSK3-like kinase BIN2. Mol. Cell 43, 561–571. doi: 10.1016/j.molcel.2011.05.037
Lacape, J. M., Dessauw, D., Rajab, M., Noyer, J. L., and Hau, B. (2006). Microsatellite diversity in tetraploid Gossypium germplasm: assembling a highly informative genotyping set of cotton SSRs. Mol. Breed. 19, 45–58.
Li, S., Liu, A., Kong, L., Gong, J., Li, J., Gong, W., et al. (2019). QTL mapping and genetic effect of chromosome segment substitution lines with excellent fiber quality from Gossypium hirsutum × Gossypium barbadense. Mol. Genet. Genomics 294, 1123–1136. doi: 10.1007/s00438-019-01566-8
Liang, Q., Hu, C., Hua, H., Li, Z., and Hua, J. (2013). Construction of a linkage map and QTL mapping for fiber quality traits in upland cotton (Gossypium hirsutum L.). Chin. Sci. Bull. 58, 3233–3243.
Liu, Q., Singh, S. P., and Green, A. G. (2002). High-stearic and High-oleic cottonseed oils produced by hairpin RNA-mediated post-transcriptional gene silencing. Plant Physiol. 129, 1732–1743. doi: 10.1104/pp.001933
Liu, X., Moncuquet, P., Zhu, Q.-H., Stiller, W., Zhang, Z., and Wilson, I. (2020). Genetic identification and transcriptome analysis of lintless and fuzzless traits in Gossypium arboreum L. Int. J. Mol. Sci. 21:1675. doi: 10.3390/ijms21051675
Liu, X., Teng, Z., Wang, J., Wu, T., Zhang, Z., Deng, X., et al. (2017). Enriching an intraspecific genetic map and identifying QTL for fiber quality and yield component traits across multiple environments in upland cotton (Gossypium hirsutum L.). Mol. Genet. Genomics 292, 1281–1306. doi: 10.1007/s00438-017-1347-8
Ma, J., Geng, Y., Pei, W., Wu, M., Li, X., Liu, G., et al. (2018). Genetic variation of dynamic fiber elongation and developmental quantitative trait locus mapping of fiber length in upland cotton (Gossypium hirsutum L.). BMC Genomics 19:882. doi: 10.1186/s12864-018-5309-2
Ma, L., Su, Y., Wang, Y., Nie, H., Cui, Y., Cheng, C., et al. (2019). QTL and genetic analysis controlling fiber quality traits using paternal backcross population in Upland Cotton. J. Cotton Res. 3:22.
Ma, Z., He, S., Wang, X., Sun, J., Zhang, Y., Zhang, G., et al. (2018). Resequencing a core collection of upland cotton identifies genomic variation and loci influencing fiber quality and yield. Nat. Genet. 50, 803–813. doi: 10.1038/s41588-018-0119-7
Ning, Z., Chen, H., Mei, H., and Zhang, T. (2014). Molecular tagging of QTLs for fiber quality and yield in the upland cotton cultivar Acala-Prema. Euphytica 195, 143–156.
Okubazghi, K. W., Li, X., Cai, X., Wang, X., Chen, H., Zhou, Z., et al. (2017). Genome-wide assessment of genetic diversity and fiber quality traits characterization in Gossypium hirsutum races. J. Integr. Agric. 16, 2402–2412.
Paterson, A. H., Saranga, Y., Menz, M., Jiang, C., and Wright, R. J. (2003). QTL analysis of genotype x environment interactions affecting cotton fiber quality. Theor. Appl. Genet. 106, 384–396. doi: 10.1007/s00122-002-1025-y
Said, J. I., Lin, Z., Zhang, X., Song, M., and Zhang, J. (2013). A comprehensive meta QTL analysis for fiber quality, yield, yield related and morphological traits, drought tolerance, and disease resistance in tetraploid cotton. BMC Genomics 14:776. doi: 10.1186/1471-2164-14-776
Seagull, R. W. (1992). A quantitative electron-microscopic study of changes in microtubule arrays and wall microfibril orientation during invitro cotton fiber development. J. Cell Sci. 101, 561–577.
Shang, L., Liang, Q., Wang, Y., Wang, X., Wang, K., Abduweli, A., et al. (2015). Identification of stable QTLs controlling fiber traits properties in multi-environment using recombinant inbred lines in Upland cotton (Gossypium hirsutum L.). Euphytica 205, 877–888.
Shang, L., Liang, Q., Wang, Y., Zhao, Y., Wang, K., and Hua, J. (2016). Epistasis together with partial dominance, over-dominance and QTL by environment interactions contribute to yield heterosis in upland cotton. Theor. Appl. Genet. 129, 1429–1446. doi: 10.1007/s00122-016-2714-2
Shao, Q., Zhang, F., Tang, S., Liu, Y., Fang, X., Liu, D., et al. (2014). Identifying QTL for fiber quality traits with three upland cotton (Gossypium hirsutum L.) populations. Euphytica 198, 43–58.
Shen, C., Wang, N., Huang, C., Wang, M., Zhang, X., and Lin, Z. (2019). Population genomics reveals a fine-scale recombination landscape for genetic improvement of cotton. Plant J. 99, 494–505. doi: 10.1111/tpj.14339
Shen, X., Guo, W., Zhu, X., Yuan, Y., Yu, J. Z., Kohel, R. J., et al. (2005). Molecular mapping of QTLs for fiber qualities in three diverse lines in Upland cotton using SSR markers. Mol. Breed. 15, 169–181.
Shen, X., Zhang, T., Guo, W., Zhu, X., and Zhang, X. (2006). Mapping fiber and yield QTLs with main, epistatic, and QTL × environment interaction effects in recombinant inbred lines of Upland Cotton. Crop Sci. 46:61.
Shi, Y., Li, W., Li, A., Ge, R., Zhang, B., Li, J., et al. (2015). Constructing a high-density linkage map for Gossypium hirsutum x Gossypium barbadense and identifying QTLs for lint percentage. J. Integr. Plant Biol. 57, 450–467. doi: 10.1111/jipb.12288
Shi, Y., Liu, A., Li, J., Zhang, J., Li, S., Zhang, J., et al. (2020). Examining two sets of introgression lines across multiple environments reveals background-independent and stably expressed quantitative trait loci of fiber quality in cotton. Theor. Appl. Genet. 133, 2075–2093. doi: 10.1007/s00122-020-03578-0
Shi, Y., Liu, A., Li, J., Zhang, J., Zhang, B., Ge, Q., et al. (2019). Dissecting the genetic basis of fiber quality and yield traits in interspecific backcross populations of Gossypium hirsutum × Gossypium barbadense. Mol. Genet. Genomics 294, 1385–1402. doi: 10.1007/s00438-019-01582-8
Song, J., Pei, W., Ma, J., Yang, S., Jia, B., Bian, Y., et al. (2021). Genome-wide association study of micronaire using a natural population of representative upland cotton (Gossypium hirsutum L.). J. Cotton Res. 4:14.
Su, C., Wang, W., Qiu, X., Yang, L., Li, S., Wang, M., et al. (2013). Fine-mapping a fibre strength QTLQFS-D11-1 on cotton chromosome 21 using introgressed lines. Plant Breed. 132, 725–730.
Sun, R., Wang, S., Ma, D., and Liu, C. (2018). Genome-wide analysis of LRR-RLK gene family in four Gossypium species and expression analysis during cotton development and stress responses. Genes 9:592. doi: 10.3390/genes9120592
Sun, Y., Veerabomma, S., Fokar, M., Abidi, N., Hequet, E., Payton, P., et al. (2015). Brassinosteroid signaling affects secondary cell wall deposition in cotton fibers. Industr. Crops Prod. 65, 334–342.
Tang, S., Teng, Z., Zhai, T., Fang, X., Liu, F., Liu, D., et al. (2015). Construction of genetic map and QTL analysis of fiber quality traits for Upland cotton (Gossypium hirsutum L.). Euphytica 201, 195–213.
Van Ooijen, J. W. (2006). JoinMap 4.0, Software for the Calculation of Genetic Linkage Maps in Experimental Populations. Wageningen: Kyazma B.V.
Van Ooijen, J. W. (2009). MapQTL 6.0, SOFTWARE for the Mapping of Quantitative Trait Loci in Experimental Populations. Wageningen: Kyazma B.V.
Wang, B., Draye, X., Zhuang, Z., Zhang, Z., Liu, M., Lubbers, E. L., et al. (2017). QTL analysis of cotton fiber length in advanced backcross populations derived from a cross between Gossypium hirsutum and G. mustelinum. Theor. Appl. Genet. 130, 1297–1308. doi: 10.1007/s00122-017-2889-1
Wang, F., Xu, Z., Sun, R., Gong, Y., Liu, G., Zhang, J., et al. (2013). Genetic dissection of the introgressive genomic components from Gossypium barbadense L. that contribute to improved fiber quality in Gossypium hirsutum L. Mol. Breed. 32, 547–562.
Wang, F., Zhang, C., Liu, G., Chen, Y., Zhang, J., Qiao, Q., et al. (2016). Phenotypic variation analysis and QTL mapping for cotton (Gossypium hirsutum L.) fiber quality grown in different cotton-producing regions. Euphytica 211, 169–183.
Wang, F., Zhang, J., Chen, Y., Zhang, C., Gong, J., Song, Z., et al. (2020). Identification of candidate genes for key fibre-related QTLs and derivation of favourable alleles in Gossypium hirsutum recombinant inbred lines with G. barbadense introgressions. Plant Biotechnol. J. 18, 707–720. doi: 10.1111/pbi.13237
Wang, H., Huang, C., Guo, H., Li, X., Zhao, W., Dai, B., et al. (2015). QTL mapping for fiber and yield traits in Upland Cotton under multiple environments. PLoS One 10:e0130742. doi: 10.1371/journal.pone.0130742
Wang, H., Huang, C., Zhao, W., Dai, B., Shen, C., Zhang, B., et al. (2016). Identification of QTL for fiber quality and yield traits using two immortalized backcross populations in Upland Cotton. PLoS One 11:e0166970. doi: 10.1371/journal.pone.0166970
Wang, K., Li, M., and Hakonarson, H. (2010). ANNOVAR: functional annotation of genetic variants from high-throughput sequencing data. Nucleic Acids Res. 38:e164. doi: 10.1093/nar/gkq603
Wang, M., Tu, L., Lin, M., Lin, Z., Wang, P., Yang, Q., et al. (2017). Asymmetric subgenome selection and cis-regulatory divergence during cotton domestication. Nat. Genet. 49, 579–587. doi: 10.1038/ng.3807
Wang, W., Sun, Y., Yang, P., Cai, X., Yang, L., Ma, J., et al. (2019). A high density SLAF-seq SNP genetic map and QTL for seed size, oil and protein content in upland cotton. BMC Genomics 20:599. doi: 10.1186/s12864-019-5819-6
Wang, Y., Zhou, Z., Wang, X., Cai, X., Li, X., Wang, C., et al. (2016). Genome-wide association mapping of glyphosate-resistance in Gossypium hirsutum races. Euphytica 209, 209–221.
Wanjie, S. W., Welti, R., Moreau, R. A., and Chapman, K. D. (2005). Identification and quantification of glycerolipids in cotton fibers: reconciliation with metabolic pathway predictions from DNA databases. Lipids 40, 773–785. doi: 10.1007/s11745-005-1439-4
Xiao, G., Zhao, P., and Zhang, Y. (2019). A pivotal role of hormones in regulating cotton fiber development. Front. Plant Sci. 10:87. doi: 10.3389/fpls.2019.00087
Xu, C., Fan, J., Cornish, A. J., and Benning, C. (2008). Lipid trafficking between the endoplasmic reticulum and the plastid in Arabidopsis requires the extraplastidic TGD4 protein. Plant Cell 20, 2190–2204. doi: 10.1105/tpc.108.061176
Xu, S., Rahman, A., Baskin, T., and Kieber, J. (2008). Two leucine-rich repeat receptor kinases mediate signaling, linking cell wall biosynthesis and ACC synthase in Arabidopsis. Plant Cell 20, 3065–3079. doi: 10.1105/tpc.108.063354
Yu, J., Yu, S., Wu, M., Zhai, H., Song, M., Fan, S., et al. (2013). Identification of quantitative trait loci across interspecific F2, F2:3 and testcross populations for agronomic and fiber traits in tetraploid cotton. Euphytica 191, 375–389.
Yu, J., Zhang, K., Li, S., Yu, S., Zhai, H., Wu, M., et al. (2012). Mapping quantitative trait loci for lint yield and fiber quality across environments in a Gossypium hirsutum × Gossypium barbadense backcross inbred line population. Theor. Appl. Genet. 126, 275–287. doi: 10.1007/s00122-012-1980-x
Yuan, D., Tang, Z., Wang, M., Gao, W., Tu, L., Jin, X., et al. (2015). The genome sequence of Sea-Island cotton (Gossypium barbadense) provides insights into the allopolyploidization and development of superior spinnable fibres. Sci. Rep. 5:17662. doi: 10.1038/srep17662
Zhang, J., Zhang, T., Qian, N., Zhu, X., Chen, H., Wang, S., et al. (2013). Variations and transmission of QTL alleles for yield and fiber qualities in Upland Cotton cultivars developed in China. PLoS One 8:e57220.
Zhang, K., Zhang, J., Ma, J., Tang, S., Liu, D., Teng, Z., et al. (2011). Genetic mapping and quantitative trait locus analysis of fiber quality traits using a three-parent composite population in upland cotton (Gossypium hirsutum L.). Mol. Breed. 29, 335–348.
Zhang, S., Cai, Y., Guo, J., Li, K., Peng, R., Liu, F., et al. (2019). Genotyping-by-sequencing of Gossypium hirsutum races and cultivars uncovers novel patterns of genetic relationships and domestication footprints. Evol. Bioinform. Online 15:1176934319889948. doi: 10.1177/1176934319889948
Zhang, S., Wang, T., Liu, Q., Gao, X., Zhu, X., Zhang, T., et al. (2014). Quantitative trait locus analysis of boll-related traits in an intraspecific population of Gossypium hirsutum. Euphytica 203, 121–144.
Zhang, S., Zhu, X., Feng, L., Gao, X., Yang, B., Zhang, T., et al. (2016). Mapping of fiber quality QTLs reveals useful variation and footprints of cotton domestication using introgression lines. Sci. Rep. 6:31954. doi: 10.1038/srep31954
Zhang, T., Hu, Y., Jiang, W., Fang, L., Guan, X., Chen, J., et al. (2015). Sequencing of allotetraploid cotton (Gossypium hirsutum L. acc. TM-1) provides a resource for fiber improvement. Nat. Biotechnol. 33, 531–537. doi: 10.1038/nbt.3207
Keywords: genetic map, fiber quality, yield related traits, QTL, Gossypium hirsutum race palmeri
Citation: Liu X, Yang L, Wang J, Wang Y, Guo Z, Li Q, Yang J, Wu Y, Chen L, Teng Z, Liu D, Liu D, Guo K and Zhang Z (2022) Analyzing Quantitative Trait Loci for Fiber Quality and Yield-Related Traits From a Recombinant Inbred Line Population With Gossypium hirsutum Race palmeri as One Parent. Front. Plant Sci. 12:817748. doi: 10.3389/fpls.2021.817748
Received: 18 November 2021; Accepted: 01 December 2021;
Published: 03 January 2022.
Edited by:
Baohua Wang, Nantong University, ChinaReviewed by:
Xinlian Shen, Jiangsu Academy of Agricultural Sciences (JAAS), ChinaJun Zhang, Shandong Academy of Agricultural Sciences, China
Copyright © 2022 Liu, Yang, Wang, Wang, Guo, Li, Yang, Wu, Chen, Teng, Liu, Liu, Guo and Zhang. This is an open-access article distributed under the terms of the Creative Commons Attribution License (CC BY). The use, distribution or reproduction in other forums is permitted, provided the original author(s) and the copyright owner(s) are credited and that the original publication in this journal is cited, in accordance with accepted academic practice. No use, distribution or reproduction is permitted which does not comply with these terms.
*Correspondence: Zhengsheng Zhang, emhhbmd6c0Bzd3UuZWR1LmNu