- 1State Key Laboratory of North China Crop Improvement and Regulation, Hebei Agricultural University, Baoding, China
- 2Department of Crop Genetics and Breeding, College of Agronomy, Hebei Agricultural University, Baoding, China
- 3Department of Crop Science, Faculty of Agriculture and Environmental Science, Bindura University of Science Education, Bindura, Zimbabwe
- 4Academy of Agriculture and Forestry Sciences, Hebei North University, Zhangjiakou, China
Novel crop improvement approaches, including those that facilitate for the exploitation of crop wild relatives and underutilized species harboring the much-needed natural allelic variation are indispensable if we are to develop climate-smart crops with enhanced abiotic and biotic stress tolerance, higher nutritive value, and superior traits of agronomic importance. Top among these approaches are the “omics” technologies, including genomics, transcriptomics, proteomics, metabolomics, phenomics, and their integration, whose deployment has been vital in revealing several key genes, proteins and metabolic pathways underlying numerous traits of agronomic importance, and aiding marker-assisted breeding in major crop species. Here, citing several relevant examples, we appraise our understanding on the recent developments in omics technologies and how they are driving our quest to breed climate resilient crops. Large-scale genome resequencing, pan-genomes and genome-wide association studies are aiding the identification and analysis of species-level genome variations, whilst RNA-sequencing driven transcriptomics has provided unprecedented opportunities for conducting crop abiotic and biotic stress response studies. Meanwhile, single cell transcriptomics is slowly becoming an indispensable tool for decoding cell-specific stress responses, although several technical and experimental design challenges still need to be resolved. Additionally, the refinement of the conventional techniques and advent of modern, high-resolution proteomics technologies necessitated a gradual shift from the general descriptive studies of plant protein abundances to large scale analysis of protein-metabolite interactions. Especially, metabolomics is currently receiving special attention, owing to the role metabolites play as metabolic intermediates and close links to the phenotypic expression. Further, high throughput phenomics applications are driving the targeting of new research domains such as root system architecture analysis, and exploration of plant root-associated microbes for improved crop health and climate resilience. Overall, coupling these multi-omics technologies to modern plant breeding and genetic engineering methods ensures an all-encompassing approach to developing nutritionally-rich and climate-smart crops whose productivity can sustainably and sufficiently meet the current and future food, nutrition and energy demands.
Introduction
Optimizing climate-change adaptation, agricultural productivity, food security and environmental protection is the grand challenge confronting scientists in this 21st century. The unequivocal change in climate, manifested in form of elevated average temperatures, global warming, sporadic and unreliable rainfalls, and enlargement of affected terrestrial regions under flood or water deficit is contributing to the expansion of drought or salinity-prone regions that are characterized by diminished plant growth and crop productivity (Lamaoui et al., 2018). Additionally, climate related changes will likely boost up the severity of both sole and combined abiotic stresses, especially drought, heat, salinity, cold, and submergence (Pandey et al., 2017; Anwar et al., 2021). Moreover, these climate change scenarios harshen the biotic stresses by boosting up the insect, pests or pathogen numbers and disease severity, stimulating weed species proliferation, dwindling soil beneficial microbes, and threatening vital plant pollinators (Kole et al., 2015; Raza A. et al., 2019; Shahzad et al., 2021). These effects have far-reaching implications for global food security, by significantly impacting plant growth, development and productivity, and consequently, global agricultural production (Dhankher and Foyer, 2018; Nhamo et al., 2019). This is occurring against the backdrop of a continued spiraling of world human population, spurred by relatively high levels of fertility in developing countries (UN, 2017), with modest projections pointing to 9.15 billion people by the year 2050 (Alexandratos and Bruinsma, 2012). This is exacerbating pressure on the agricultural production and food supply systems, since 56% more food will need to be produced to feed additional 3 billion mouths using the same or less quantity of resources as compared to the year 2010 (Ranganathan et al., 2018). More worryingly, around 800 million and 2 billion people are already facing acute food shortages and malnutrition problems, respectively, as access to nutritious foods is out of reach of many (FAO, 2019; Fiaz et al., 2021). Further, the edaphic environment, upon which our agricultural system relies for sustenance and provision of food to humans, is facing serious challenges related to natural resource degradation and decline as well as biodiversity erosion (Wassie, 2020; Zandalinas et al., 2021).
Given the scenario highlighted above, innovative sustainable crop production efforts are required to ensure optimized resilience under climate change conditions (Vaughan et al., 2018). Developing climate resilient crops, increasing efficiency of natural resource use, linking agricultural intensification with natural ecosystem protection, and diversification of agricultural systems have been widely proposed as sustainable solutions to address these challenges (Gil et al., 2017; Dhankher and Foyer, 2018; Evans and Lawson, 2020). These strategies will facilitate the closing of three main types of gaps, viz., the food gap, land gap, and greenhouse gases (GHG) mitigation gap (for detailed explanations, see The World Resources Institute, 2019). In particular, development of climate resilient crop cultivars with desired agronomic traits has been advocated as the most plausible, economical, sustainable and efficient way to adapt our agricultural system to climate change (Mba et al., 2012; Kumari et al., 2020; Kim J.H. et al., 2021). Breeding for climate smart crop cultivars will entail exploring crop wild relatives and revisiting neglected and underutilized species for the untapped novel allelic variation harbored by those species, thereby broadening the genetic variation available for crop breeders’ use (Brozynska et al., 2016; Gupta et al., 2017; Ananda et al., 2020; Kilian et al., 2020; Kamenya et al., 2021). Additionally, there will be need to employ advanced crop breeding techniques and methodologies, integrated with conventional and improved data analysis pipelines (Ahmar et al., 2020; Bohra et al., 2020; Pourkheirandish et al., 2020; Qaim, 2020; Steinwand and Ronald, 2020).
Fortunately, the flourishing developments in omics technologies have revolutionized our crop improvement endeavors, by fortifying crop breeders’ toolboxes and galvanizing omics-assisted breeding programs targeting various agronomic traits (Langridge and Fleury, 2011; Li and Yan, 2020). Omics technology is a modern molecular tool useful in understanding functional genomic systems in an organism (Hu et al., 2018; Banerjee et al., 2019), and involves DNA sequencing and profiling of the expressed transcripts and translated proteins (Missanga et al., 2021). With the term “omics” being a derivative of the Greek word “-ome” meaning “whole,” omics refer to scientific disciplines that study different types of biological molecules constituting complete biological systems (SETAC, 2019). These disciplines encompass genomics, transcriptomics, proteomics, metabolomics, and phenomics (Hasin et al., 2017; Khalid et al., 2019).
Specifically, recent advances in genome sequencing techniques, coupled with omics-platforms generated data, have facilitated the availability of enormous genomic and transcriptomic data for various crop species, and have significantly improved gene discovery, gene expression profiling, marker-assisted selection, domestication of underutilized species, and introgression of unique and key traits into desired crops (Pathak et al., 2018; Muthamilarasan et al., 2019; Cortés and López-Hernández, 2021). This is now permitting us to routinely delineate the molecular and genetic underpinnings to the several phenotypic traits of agricultural importance (Scossa et al., 2021). Integrated with other modern crop improvement strategies such as speed breeding and gene editing technologies, omics approaches now facilitate rapid creation of elite climate smart cultivars with desired traits such as enhanced productivity, abiotic and biotic tolerance, and nutritive quality (Gao, 2021; Kumar R. et al., 2021; Singh R. K. et al., 2021).
Here, citing some relevant examples, we appraise our knowledge on the recent progress in omics approaches and how these developments, integrated with other modern plant breeding, data analysis, and gene editing technologies, are altering the crop improvement landscape related to abiotic and biotic stress tolerance, higher nutritional quality and other key agronomic traits, thereby facilitating global food and nutrition security.
Omics Approaches for Crop Improvement: an Overview
In modern molecular biology, the suffix “-omics” specially refers to a collection of technologies applied to the analysis of a huge and complete data set of a particular class or type of biological molecule in a cell, tissue, organ, or whole organism (Zaitlin, 2020). In other words, plant molecular biology revolves around investigating cellular processes, their genetic determinants, and interactions with environmental alterations, and such a multi-dimensional and comprehensive inquiry involves large-scale experiments targeting entire genetic, structural, or functional components. These large scale studies are what are known as “omics” (Deshmukh et al., 2014). The omics sub-disciplines at the forefront of fundamental systems biology studies and contemporary crop improvement interventions are genomics, transcriptomics, proteomics, metabolomics, and phenomics (Hasin et al., 2017; Dubey et al., 2019a); which chiefly involve comprehensive investigation of the genome, transcriptome, proteome, metabolome, and phenotypes, respectively (Table 1). All of these omics branches are closely linked to bioinformatics (Zaitlin, 2020).
In general, the analyses of the -omics fields are modeled along the structure of Francis Crick‘s (1954) classical central dogma of molecular biology (through targeted investigation of each molecule at a particular level). Put simply, the genome, transcriptome, proteome, metabolome, and phenome constitute different layers of the omics cascade, each of which defines a biosystem or an organism at different biomolecular levels (Jendoubi, 2021; Figure 1A). However, the complexity of biological systems means that dynamic environmental and spatio-temporal molecular interactions do not actually follow this simple path of reductionism and cannot be studied from the static topology point of view (Franklin and Vondriska, 2011; Wolkenhauer and Muir, 2011). Hence, a systems biology approach provides a holistic way for dissecting the underlying genetic and molecular mechanisms governing specific traits of economic importance (Pazhamala et al., 2021). The advent of omics strategies, coupled with other technological inventions such as gene sequencing and mutagenesis, has offered new dimensions in crop improvement programs, by facilitating improved gene function prediction, and better dissection of molecular mechanisms underlying important agronomic traits (Kumar R. et al., 2021). This is essential for the development of superior crop cultivars enhanced with greater yield, stability, abiotic and biotic stress tolerance, and nutritional composition, through introgressing genes or QTL from identified donor genotypes, either via forward genetics or reverse genetic approaches (Figure 1B; Bahuguna et al., 2018).
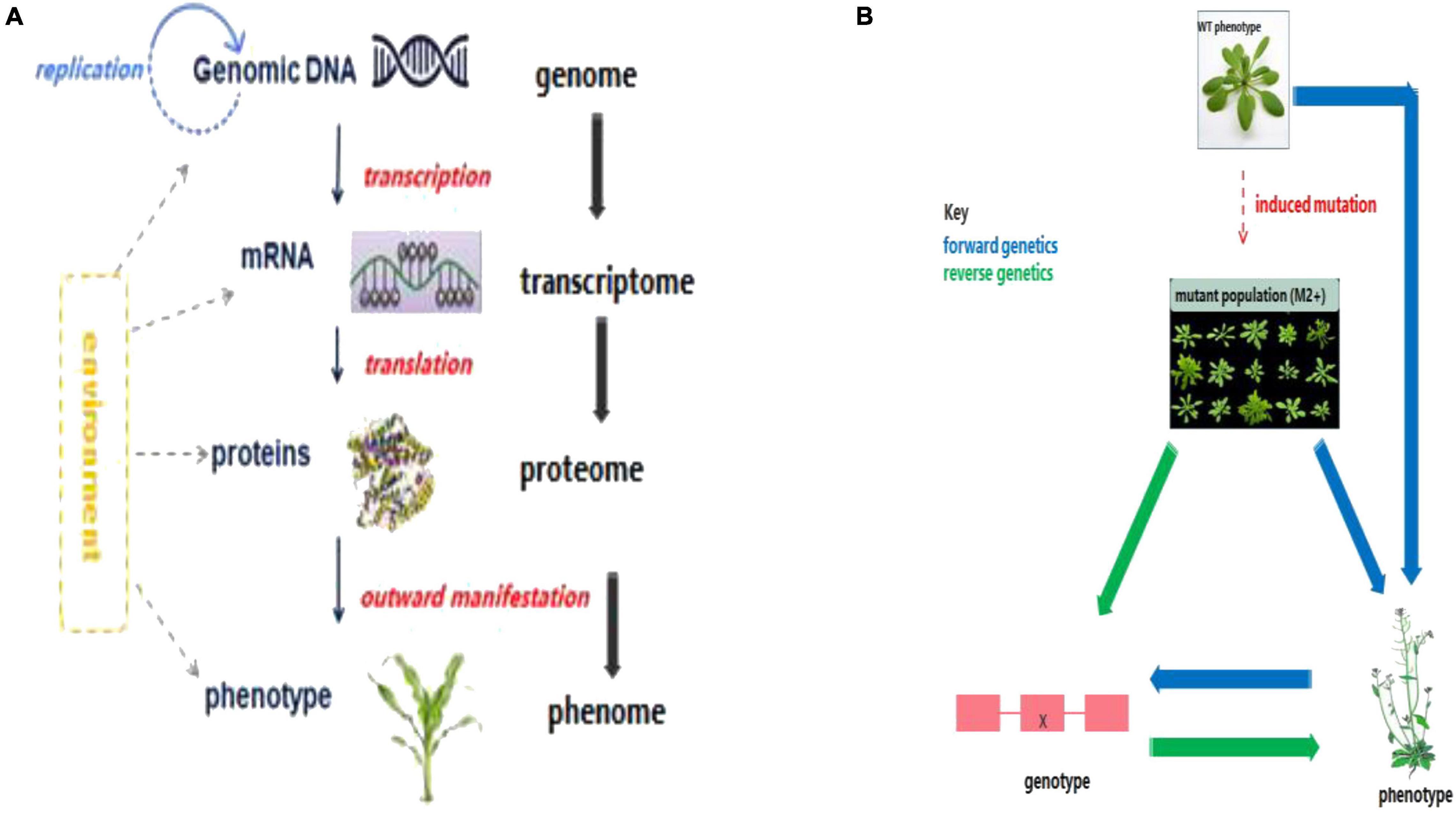
Figure 1. Link among the major biological molecules and genetic approaches for crop improvement. (A) A cascade of interactions among the major biological molecules constituting the central dogma of molecular biology. Owing to the complexity of biological systems and molecular interactions, the simplistic arrows shown here offer only a general scheme of cascading influence. Dotted lines imply that the environment affects the biomolecules at different levels. (B) Genetic approaches for crop improvement. The canonical forward genetic approach involves creating variation (either naturally or via induced mutations) in a population; identifying interesting and novel phenotypes; and then cloning the gene/s responsible for the identified phenotypic variation. Reverse genetic approach involves first carrying out genotypic screening of the mutant population to identify novel induced mutations in candidate genes, and then perform phenotypic evaluation of those individuals harboring putative mutations (Jankowicz-Cieslak and Till, 2015; SETAC, 2019).
Genomics and Pan-Genomics
High Quality Reference Genomes as Vital Resources for Accurate Annotation of Gene Structure, Content and Variation
Recent cost reductions in high throughput (HTP) sequencing and rapid improvements in sequence assembly algorithms and surveying platforms have facilitated for the readily availability of genomic tools and resources for several crops (Bohra, 2013; Jayakodi et al., 2021). These tools include high quality reference genomes, DNA markers, and genetic maps, which are essential for functional and comparative genomic studies, as well as molecular crop improvement (Zhang and Hao, 2020). Especially, the availability of reference genomes for several major crops and the ability to perform HTP resequencing have enabled us to demarcate genes and other regulatory sequences, map genomic variations, refine gene models and better understand gene functions (Morrell et al., 2012; Schreiber et al., 2018; Zhang Q. et al., 2020). Researchers can now routinely perform genome-wide scans for genes controlling key traits of agronomic importance in crops (Zhang et al., 2019; Nakano and Kobayashi, 2020).
Genome sequencing technologies have evolved from the classical Senger method (first generation), through next generation sequencing (NGS), to third generation sequencing (TGS) approaches. For detailed reviews on these sequencing approaches, we refer you to previous papers (Li et al., 2018; Cui et al., 2020). Through these technologies, especially NGS and TGS, several crop genomes have been sequenced (Purugganan and Jackson, 2021), including those for soybean (Glycine max L., Shen et al., 2018), lablab (Lablab purpureus L. Sweet) and other major grain legumes (see Varshney et al., 2015; Missanga et al., 2021), ten top most world food crops (see Varshney et al., 2021), major and minor millets (see Vetriventhan et al., 2020; Singh R. K. et al., 2021), several cereal crops including orphaned species (see Table 1 of our most recent paper, Zenda et al., 2021), diverse crop species (Michael and Jackson, 2013; see Bevan and Uauy, 2013; Bevan et al., 2017; Mohanta et al., 2017; Schreiber et al., 2018), and fruit crops (Li et al., 2019). Among these sequenced crop species are crop wild relatives and underutilized species (Chang et al., 2019; Vetriventhan et al., 2020), which have been recognized as excellent sources of novel genetic diversity for future crop improvements (Schreiber et al., 2018; Singh R. K. et al., 2021). Thus, complete genome assemblies for hundreds of crop species are now available in public repositories [Kersey, 2019; Pazhamala et al., 2021; Sequenced plant genomes – CoGepedia (genomevolution.org)] and several genome databases and tools have been created (for extensive review, see Bohra, 2013; Chen et al., 2018; Varshney et al., 2021). Additionally, progress in genome sequencing and HTP genotyping has opened a window for increased de novo domestication of crop wild relatives and orphan species for accelerated crop improvement for abiotic stress and higher nutritive value (Morrell et al., 2012; Bevan and Uauy, 2013; Bohra et al., 2014; Schreiber et al., 2018; Gasparini et al., 2021). Taken together, the recent fast-paced developments in genome sequencing and assembly are enabling easy decoding of intricate crop genomes for genes and alleles controlling key agronomic traits.
Large-Scale Resequencing and Pan-Genomes Facilitating Identification and Analysis of Species-Level Genomic Variations
Genetic diversity among species and within populations is the mainstay of crop improvement and genetic dissection of complex traits (Gao, 2021). In plant genomes, natural variations emanate from single nucleotide polymorphisms (SNPs), small insertions and deletitions (InDels, <50 nucleotides), and structural variants (SVs, >50 nucleotides) (Vishwakarma et al., 2017). Large polymorphisms, encompassing large-scale duplications, presence/absence variants (PAVs), copy number variants (CNVs), deletions and rearrangements constitute the SVs (Saxena et al., 2014; Ho et al., 2020; Zhao et al., 2020). Particularly, SVs have been recognized as important sources of functionally consequential genetic variations within species (Tao et al., 2019), and have significantly contributed to crop domestication, evolution and improvement (Qin et al., 2021). Owing to developments in high quality genome sequencing and resequencing, an increasing number of crop genomic studies based on high quality assemblies have resolved SVs and facilitated the accurate annotation of functional gene variants among selected accessions (Table 2; Alonge et al., 2020; Qin et al., 2021).
Genome SVs can be detected using any of the three approaches, viz., de novo domestication, resequencing, and pan-genome (Saxena et al., 2014). Particularly, de novo assembly of multiple high-quality reference genome sequences and their subsequent comparison by pair-wise sequence alignment has proved a very powerful and accurate method of detecting all types of SVs at base-level resolution (Jayakodi et al., 2021). For example Li et al. (2014) constructed a de novo assembly-based pan-genome of Glycine soja, the wild relative of cultivated soybean Glycine max, by sequencing seven phylogenetically linked accessions and observed lineage-specific genes and CNV-possessing genes by intergenomic comparisons, with some CNV-containing genes exhibiting evidence of positive selection and linked to variation of key agronomic traits such as anthesis and maturity time, seed composition, final biomass, and biotic resistance. Additionally, they identified that 80% of the Glycine soja pan-genome constituted the core genome, whereas 20% (the dispensable genome) showed greater variation than the core genome, probably reflecting the dispensable genome‘s role in acclimation to diverse environments (Li et al., 2014).
Large-scale resequencing of diverse crop germplasm and genome-wide association studies (GWAS) are laying bare the extent of genome variation, the genetic architecture, and link between the phenotype and genotype, which are gateways in deciphering the genes underpinning several agronomically important traits in various crops (Huang and Han, 2014; Xu and Bai, 2015; Zhang Q. et al., 2020; Ye et al., 2021). Some of the major crops that have been resequenced include sorghum (McCormick et al., 2018; Cooper et al., 2019), maize (Lai et al., 2010; Xu et al., 2014), soybean (Zhou et al., 2015), tomato (Solanum lycopersicum L., Roohanitaziani et al., 2020; Ye et al., 2021), eggplant (Solanum melongena L.) and its wild relative (Solanum incanum L.) (Gramazio et al., 2019), rice and its wild progenitors (Oryza rufipogon L. and Oryza nivara L.) (Xu et al., 2012), Brassica rapa L. and Brassica. oleracea L. (Cheng et al., 2016), and several crop species (reviewed in Varshney et al., 2021). The TGS approaches such as PacBio Single Molecule Real Time, Illumina Tru-seq Synthetic Long-Read and Oxford Nanopore technologies employ the use of single molecule reads (see Li et al., 2018; Cui et al., 2020 for extensive review), which can exceed megabases in length, thereby providing unprecedented opportunities to resolve SVs missed by short read approaches (Schreiber et al., 2018; Michael and VanBuren, 2020). For example, Zhou et al. (2015) resequenced 302 soybean accessions (comprising wild, landraces, and improved cultivars) at > 11 × depth and then performed GWAS analysis of these accessions‘ sequences, which identified 13 previously uncharacterized loci for key agronomic traits including plant height and oil content, among others. As the costs for DNA sequencing continue to decline and new innovations in gene editing, machine learning and data algorithms gather pace, whole genome resequencing approaches will not only help in better understanding of the genetic basis of complex traits, but will increasingly play important roles in QTL mapping and gene identification, consequently accelerating crop improvement for climate resilience and higher nutritive value via genomics assisted breeding (GAB).
The concept of pan-genomes has been propelled by the realization that a single reference genome sequence is insufficient to represent the full spectrum of genetic variation occurring within a species (Golicz et al., 2016a; Bayer et al., 2020). Pan-genome involves the non-redundant assemblage of genes and/or DNA sequences in a clade or a species (Lei et al., 2021), and encompasses core genome (containing genes present all accessions) and variable genome (comprising partially shared and accession specific genes) (Saxena et al., 2014; Tahir ul Qamar et al., 2020). Since a pan-genome provides an entire complement of genomic diversity repertoire of a genus, pan-genome analysis is a more robust, comprehensive and indispensable approach to identify gene content variation and perform a whole-species genetic diversity analysis (Tao et al., 2019; Khan et al., 2020).
Crucially, pan-genomes usually contain within-species CNVs and PAVs (Scheben et al., 2016), and such SVs have been observed to influence traits of agronomic importance in crops (Zuo et al., 2015; Tao et al., 2019; Khan et al., 2020). Notably, variable gene annotations often exhibit similarities across plant species, with genes for biotic and abiotic stress tolerance frequently enriched within variable gene clusters (Bayer et al., 2020). It is no surprising that pan-genomics is a hot topic at the present moment, with pan-genomic studies facilitating the dissection of the genetic variation, which is critical for linking the desirable phenotypes to major agronomical traits (Danilevicz et al., 2020; Coletta et al., 2021). Ever since the concept of pan-genomes was first established in 2005 by Tettelin et al. (2005), several crop pan-genomes have been developed, including for maize, soybean and wheat among others (Table 2).
Since pan-genomes can reveal the extent of novel alleles and genes in crop wild relatives, the novel candidate genes that may be linked to adaptation to numerous biotic and abiotic stresses can be introgressed into cultivated crops to increase their resilience to climate variability. Essentially, genes harboring SVs and large-effect mutations showing association with important agronomic phenotypes (as inferred by mapped QTLs) can be harnessed to develop molecular markers for the SV containing regions and test new allelic combinations (Li et al., 2014), thereby providing new resources for designing new crop cultivars (Khan et al., 2020).
Already (Zenda et al., 2021), we have highlighted that transposable elements (TEs), which are ubiquitous mobile DNA sequences with the propensity to transverse along the genome (Makalowski et al., 2019), are becoming a new research avenue for crop genome analysis and helping us better understand crop abiotic and biotic stress responses. TE transposition has been shown to modulate transcriptional activity of contiguous genes through regulation of epigenomic profile of the region (Ariel and Manavella, 2021). Additionally, TEs largely contribute to genome size variation (Dubin et al., 2018; Anderson et al., 2019) and SVs among different crop species (Tao et al., 2019; Coletta et al., 2021). Particularly, TEs have been shown to activate important gene allelic or regulatory variation in abiotic stress responses (Makarevitch et al., 2015). As the omics technology develop, new methodologies for comprehensive TE annotation and analysis will also need to keep pace with these developments, in order to help us better decipher how TEs regulate plant phenotypic responses to abiotic stresses (for a detailed review, see Zenda et al., 2021).
Genetic Diversity Analysis and Mapping of Quantitative Traits
Dissecting the genetic basis of important agronomic traits, such as grain yield, grain size, flowering time, fiber quality and disease resistance is essential for manipulating and precise introgression of these traits in breeding programs (Würschum et al., 2012; Noble et al., 2018; Mérida-García et al., 2019; Shi Y. et al., 2019; Goddard et al., 2020). In other words, GAB is facilitated by the identification of molecular genomic markers linked to QTLs or genes underlying agronomic traits of interest, which are then utilized as useful tools for molecular breeding (Singh R.K. et al., 2020; Sinha et al., 2021). To that end, several GAB approaches have been deployed in various crop improvement programs, including marker-assisted backcrossing (MABC) to enhance β-carotene content in maize (Qutub et al., 2021); marker-assisted recurrent selection (MARS) to improve crown rot (Fusarium pseudograminearum) resistance in bread wheat (Rahman et al., 2020) and pod shattering resistance in soybean (Kim et al., 2020); as well as genomic selection (GS) to improve rice blast (Magnaporthe oryzae) resistance (Huang et al., 2019) and maize drought tolerance (Shikha et al., 2017). Meanwhile, molecular marker based applications such as gene linkage and quantitative trait loci (QTL) mapping have become more feasible owing to the recent advances in genotyping platforms and statistical genomics (Kulwal, 2018). More significantly, cost-effective NGS technologies have accelerated the development of molecular markers and their deployment in genetic diversity and phylogenic relationship analyses in various species. Molecular markers have been widely used to ascertain the magnitude of genetic diversity in cultivated and wild crop gene pools (see Kumar J. et al., 2021). Additionally, numerous studies have been performed to identify several QTLs for diverse traits of agronomic value in different crop species (see Nepolean et al., 2018; Choudhary et al., 2019; Kumar J. et al., 2019; Singh R.K. et al., 2020; Liu and Qin, 2021). For example, nine QTLs for grain yield under low soil nitrogen environments in maize (Ribeiro et al., 2018), major QTLs controlling grain yield under drought in pearl millet (Bidinger et al., 2007; Debieu et al., 2018), QTLs for plant height and flowering time in soybean (Cao et al., 2017), QTLs and candidate genes for root-knot nematode resistance in cowpea (Vigna unguiculata L.) (Santos et al., 2018), QTLs for Fusarium head blight resistance in barley (Huang et al., 2018), novel QTLs for salinity tolerance in rice (Pundir et al., 2021), QTLs controlling protein and oil contents and oil quality in groundnut (Sarvamangala et al., 2011), and QTLs for seed Fe and Zn content in chickpea (Sab et al., 2020) were identified, among others.
Especially, sequence-based and genome-wide distributed high-density SNP markers have been successfully used to characterize cultivated varieties and landraces based on their geographical origin, and have been efficient in the identification of varied levels of genetic diversity among diverse genotypes in gene pools (Kumar J. et al., 2021). Additionally, SNP markers have been used to map QTLs/genes controlling the target traits of agronomic importance in different crops such as maize (Cui et al., 2015), lentil (Kumar J. et al., 2021), soybean (Lee et al., 2015), cotton (Sun et al., 2017, 2018; Majeed et al., 2019), groundnut (Liang et al., 2017; Han et al., 2018) and several crops (Mammadov et al., 2012). Notably, SNPs have greatly supported GWAS in delineating the slightest possible genome variations linked to plant phenotypic variations (Bohra et al., 2020). Thus, GWAS improves the mapping resolution for accurate location of allele/QTL/genes underlying key agronomic traits (Huang and Han, 2014; Pang et al., 2020). Unsurprising, large-scale GWAS has become a powerful tool for performing efficient genome-phenotype association analysis and identification of causative QTL/genes for key agronomic traits in diverse crop species (Sun et al., 2017; Jha et al., 2020; Berhe et al., 2021; Kaur et al., 2021; Sinha et al., 2021). For instance, using a natural population comprising 713 upland cotton accessions, Sun et al. (2018) discovered a total of 10 and 15 SNPs that were significantly associated with relative survival rate and salt tolerance level, respectively, among which two SNPs (i46598Gh and i47388Gh) on genomic region D09 were simultaneously linked with the two traits. A GWAS using a diverse panel of 206 genotypes identified genetic loci associated with Striga (Striga hermonthica) resistance genes in sorghum (Kavuluko et al., 2021). The study detected secondary cell wall modification genes for lignin biosynthesis genes, including PMT2 Methyltransferase at position S2_59157949, secondary wall NAC TF 4 at S6_60968111 and early nodulin 93 at S10_2576197. Additionally, they identified the Fasciclin-like arabinogalactan protein 11 that regulates plasticity and integrity of cell walls at position S9_5732771, as well as revealing the association of Striga resistance with the Ethylene-responsive transcription factor ERF113 at S4_50512606. ERF113 is a key regulator of both jasmonic acid (JA) and salicylic acid (SA) mediated defense pathways in plants (Kavuluko et al., 2021). GWAS to understand the genetic architecture of grain yield (GY) and flowering time under drought and heat stresses in a collection of 300 tropical and subtropical maize inbred lines using 381 165 genotyping-by-sequencing (GBS) SNPs revealed that 1549 SNPs were significantly associated with all the 12 trait-environment combinations, with 193, 95, and 405 candidate genes associated with GY, anthesis-silking interval (ASI), and anthesis date (AD), respectively (Yuan et al., 2019). In the haplotype-based association mapping analysis, 19 candidate genes were identified for the 12 trait-environment combinations, and 156 SNPs were in the genic region of these candidate genes. Notably, four candidate genes (GRMZM2G329229, GRMZM2G313009, GRMZM2G043764, and GRMZM2G10 9651) overlapped in both the GBS SNP-based and the haplotype-based association mapping analyses, with three of these genes being associated with AD evaluated under different conditions (Yuan et al., 2019).
In another study, a GWAS analysis using 195 peanut accessions subjected to GBS approach produced a total of 13 435 high-quality SNPs, including 93 non-overlapping peak SNPs that were significantly associated with four (yield per plant, hundred-pod weight, hundred-seed weight, and pod branch number per plant) of the studied yield-related traits (Wang J. et al., 2019). Among the 93 yield-related-trait-associated SNP peaks, 12 were found to be co-localized with the QTLs identified in earlier related QTL mapping studies and these 12 SNP peaks were only related to three traits and were almost all positioned on chromosomes Arahy.05 and Arahy.16. Remarkably, gene annotation of the 12 co-localized SNP peaks identified 36 candidate genes, among which one interesting gene arahy.RI9HIF was picked as prime target for further evaluation. The rice homolog of arahy.RI9HIF produces a protein that has been shown to improve rice yield when over-expressed. Therefore, further validation of the arahy.RI9HIF gene, and other candidate genes particularly harbored within the more confident co-localized genomic regions, may hold much promise for considerably enhancing peanut yield (Wang J. et al., 2019). Besides these examples, several recent papers have highlighted how GWAS, supported by SNPs, have been successfully deployed to detect genomic regions and candidate genes for various crop agronomic traits (Mammadov et al., 2012; Mousavi-Derazmahalleh et al., 2019; Alqudah et al., 2020; Pang et al., 2020).
In recent years, the increased use of GS in GAB has facilitated for quick crop improvement (Shamshad and Sharma, 2018). In GS, genome-wide high throughput markers (such as SNPs) that are in LD with QTLs are used to estimate their effects through optimum statistical models, before genomic estimated breeding values (GEBVs) are computed for each individual to select potential elite lines (Shamshad and Sharma, 2018; Mérida-García et al., 2019; Voss-Fels et al., 2019). Two population types are a pre-requisite in GS, viz., a training/reference population comprised of a cohort of individuals with both genotypic and phenotypic data and a testing/breeding population consisting of candidate breeding lines with genotypic data only (Dwivedi et al., 2020; Xu et al., 2020). The predicted GEBVs are then used for selection, excluding the need for further phenotyping (Srivastava et al., 2020; Zenda et al., 2021). Therefore, GS remarkably shortens the breeding cycle as compared to traditional breeding strategies (Bhat et al., 2016; Sinha et al., 2021). Thus, GS is an economical and viable alternative to MAS and phenotypic selection of quantitative traits (Shikha et al., 2017; Mérida-García et al., 2019). It enables crop breeders to explore and increase genetic gain per selection per unit breeding cycle, consequently enhancing speed and efficiency of breeding programs, thus, enabling the faster development of improved crop cultivars to cope with the climate change induced challenges (Spindel et al., 2015; Bhat et al., 2016; Voss-Fels et al., 2019). Moreover, GS is more superior to traditional MAS approach because it addresses the effect of small genes which cannot be captured by the traditional MAS (Heffner et al., 2009). Already, GS has shown great promise for predicting genotype performance and selection of complex traits such as disease resistance (Arruda et al., 2015; Huang et al., 2019) and drought tolerance (Shikha et al., 2017; Cerrudo et al., 2018).
In order to resolve some difficulties surrounding the use of QTL information in marker assisted breeding and gene candidate identification, especially regarding complex abiotic stress related traits, meta-QTL analysis approach has been advanced. Meta-QTL analysis compiles QTL data from diverse studies together on the same genetic linkage map for identification of precise QTL region (Deshmukh et al., 2014). For instance, using 34 different mapping populations encompassing 53 different parental accessions, Soriano et al. (2021) conducted a meta-QTL analysis on 45 traits in durum wheat, including quality and abiotic and biotic stress-related traits. A total of 368 QTL distributed on all 14 chromosomes of the genomes A and B were projected, among which 171 QTLs were related to quality-related traits, 127 to abiotic stress and 71 to biotic stress. Resultantly, 318 QTLs were grouped in 85 meta-QTL (mQTL), of which 15 mQTL were selected as the most promising for candidate gene selection (Soriano et al., 2021). These 15 most promising mQTLs were located on nine different chromosomes and showed co-localized QTLs for several grain traits. Interestingly, five mQTLs (2B.7, 4A.1, 7A.1, 7A.2 and 7A3) harbored genes associated grain weight and size (TaGS2-B1, TaCwi-A1, TaTEF-7A, TaGASR7-A1 and TaTGW-7A), and two genes affecting grain yield and quality (TaSdr-A1 and TaALP-4A – involved in preharvest sprouting tolerance) and were located in mQTL2A.4 and mQTL4A.5, respectively (Soriano et al., 2021). In another study, meta-QTL analysis was applied for a large set of phenotypic data obtained from nine inter-connected biparental RIL populations and seven environments in order to reveal the genetic control of yield-related traits and seed protein content in pea (Klein et al., 2020). A total of 89 QTL explaining a part of phenotypic variation were detected across the seven pea chromosomes. The meta-analysis of these QTL revealed 27 consensus or mQTLs, with each mQTL corresponding to one to 15 initial QTLs. Notably, most mQTLs were consistently detected in different environments, regardless of significant environmental and GxE effects (Klein et al., 2020). The study pinpointed several robust mQTLs of seed yield and seed protein content in pea and proposed some candidate genes, including Psat5g299400, a gene belonging to the AUX/IAA family putatively involved in early response to auxin (found located on mQTL1.5 region), and Psat2g005160, a gene encoding ADP-glucose pyrophosphorylase (found located on the locus AGPS2 on mQTL1.1 region) (Klein et al., 2020) and previously shown to be associated with seed size QTL in pea (Smith et al., 1989). Other meta-QTL studies carried out to identify mQTLs for various quantitative traits of agronomic importance in crops are available for soybean (Deshmukh et al., 2014), maize (Chen et al., 2017; Guo et al., 2018), barley (Zhang X. et al., 2017), wheat (Safdar et al., 2020), rice (Raza Q. et al., 2019; Selamat and Nadarajah, 2021), and cotton (Said et al., 2013), among others. The useful information generated from these mQTL studies facilitates the cloning and pyramiding of QTLs to create new crop cultivars with specific quantitative traits and speed up breeding programs via MAS.
Linkage mapping using artificially created segregating populations has been the most conventional method used to dissect the genetic basis of crop traits (Kulwal, 2018; Noble et al., 2018). Different genetic populations have been exploited to identify thousands of QTLs for several agronomic traits, especially recombinant inbred lines, because of their simple development, balanced parental mixture, repeated phenotyping, and relatively high mapping power (Liang et al., 2021a). Other mapping population types include introgression lines, advanced backcross populations, F2 populations, double-haploid populations, and backcross populations (reviewed in Kaur et al., 2021; Zenda et al., 2021).
However, association mapping (AM), based on linkage dis-equilibrium (LD) in natural population is a powerful and highly desirable approach in quickly and efficiently dissecting important traits in plants (Nachimuthu et al., 2015; Zhao et al., 2017). AM is a strategy that accounts for thousands of polymorphisms to evaluate the effects of QTL, and has more advantages than linkage analysis as it offers comparatively high-resolution power (which is based on the structure of LD) (Ibrahim et al., 2020) and provides the possibility to study various genomic regions simultaneously without construction of mapping populations (Saba Rahim et al., 2018). The size and diversity of the population for AM is critical to successful identification of key traits to previously known chromosomal regions with greater precision. The AM population must have sufficient variation for the traits of interest at both DNA sequence and phenotype levels. The greater is the size and extent of DNA sequence variation, the greater is the chance of discovering polymorphic markers (Liu et al., 2015). For instance, in one AM study, 104 peanut accessions were utilized to identify molecular markers associated with seed-related traits using 554 single locus simple sequence repeat (SSR) markers. Most of the accessions had weak or no relationship in the peanut panel, and large phenotypic variation was observed for four seed-related traits (seed length, seed weight, ratio of seed length to width, and hundred-seed weight) in the association panel (Zhao et al., 2017). AM detected a total of 30 significant SSR markers associated with four seed-related traits in different environments, which explained 11.22–32.30% of the phenotypic variation for each trait. The marker AHGA44686 was simultaneously and repeatedly associated with seed length and hundred-seed weight in multiple environments with large phenotypic variance (26.23∼32.30%), suggesting that AHGA44686 is a promising genetic marker which can enhance hundred-seed weight through seed length (Zhao et al., 2017). In soybean, Bao et al. (2015) used a set of 282 breeding lines (composed of ancestral lines, advanced breeding lines, released cultivars and landraces from the University of Minnesota Soybean Breeding Program) genotyped by using a genome-wide panel of 1536 SNP markers, to perform AM for four sudden death syndrome (SDS) (caused by Fusarium virguliforme) resistance traits (root lesion severity, foliar symptom severity, root retention, and dry matter reduction). AM approach identified significant peaks in genomic regions of known SDS resistance. Eight and two SNP markers in significant association with root retention and dry matter reduction were identified, respectively, exhibiting a total of five loci underlying SDS resistance, including three known SDS resistance QTL, viz., cqSDS001 (on linkage group D2, chr 17), cqRfs4 (at position 80.28 cM on linkage group C2, chr 6), and SDS11-2, as well as two novel loci, SDS14-1 (on chr 3) and SDS14-2 (on chr 18). Interestingly, among the five loci identified, cqSDS001 and cqRfs4 had been previously identified and confirmed in multiple bi-parental populations, thereby strengthening the accuracy of the overall AM analysis (Bao et al., 2015). AM has also proved convenient in the identification of major-effect QTLs for grain yield under drought in rice (Swamy et al., 2017), heat tolerance in maize (Seetharam et al., 2021), and flowering time in rapeseed (Xu et al., 2015) among other important traits. Thus, aided by the recent developments in genome sequencing and computational tools, AM provides huge potential to enhance crop genetic improvement.
Meanwhile, multiparental, or next-generation mapping populations (NGMPs), possess greater utility as compared to biparental populations since they yield additional recombination break points and increase the allelic diversity and QTL detection power (Gangurde et al., 2020). Examples of NGMPs include nested-association mapping (NAM) (see Gangurde et al., 2020), Multi-parent Advanced Generation Inter-Cross (MAGIC) (Huang et al., 2015) and random-openparent association mapping (ROAM) (Xiao et al., 2016) (for extensive review, see Liang et al., 2021a; Sinha et al., 2021). These NGMPs can be effectively used to identify rare alleles in joint linkage association mapping studies to circumvent the limitations of natural mapping populations and GWAS. The recent genome sequenced and re-sequenced assemblies for various crop species are valuable resources for sequence based trait mapping and candidate gene discovery (Gangurde et al., 2020). Going forward, our focus is increasingly shifting from QTL identification to quantitative trait nucleotides (QTNs) and positional (or map-based) cloning. It is envisaged that in the near future fine mapping of QTLs and pinpointing of QTNs will become more efficient, consequently enhancing our capacity to perform precision breeding of crops that can withstand the emerging climatic shifts (Liang et al., 2021a; Varshney et al., 2021).
Epigenomics as an Emerging Research Avenue for Abiotic and Biotic Stress Tolerance Breeding
Recently, epigenetics, which refers to the heritable and stable alterations in gene expression not attributable to DNA sequence changes or variation (Peschansky and Wahlestedt, 2014), has emerged as a potential research avenue for exploitation in our endeavor to develop climate smart crops (Crisp et al., 2021; Gogolev et al., 2021; Kakoulidou et al., 2021; Samantara et al., 2021). Such epigenetic modifications include DNA methylation, histone proteins/variants rearrangements, micro-RNA (mRNA) induced chromatin remodeling, histone acetylation, ATP-dependent nucleosome remodeling, among others (McCoy et al., 2021; Singh and Prasad, 2021). These epigenetic modifications are instituted to modulate spatio-temporal gene expressions in response to external stimuli or specific developmental requirements (Yuan et al., 2013; Singh and Prasad, 2021). More crucially, these epigenetic alterations involve the development of internal memory marks which assist plants to adapt to several abiotic and biotic stresses via physiological regulation directed by plants‘ epigenetic history (reviewed in Samantara et al., 2021; Sun et al., 2021). The molecular mechanisms underpinning plant environmental stress responses often rely on these epigenetic modifications (for extensive reviews, see Kim et al., 2010; Kim et al., 2015; Banerjee et al., 2017; Chang et al., 2020). A collection of examples of epigenetic studies for crop improvement are tabled in a more recent review by Kakoulidou et al., 2021. Therefore enhancing our understanding of the epigenetic regulation induced gene expressions related to abiotic and biotic stress responses will create more avenues for crop improvement for climate resilience via molecular breeding and/or biotechnological approaches (Chinnusamy et al., 2013; Singh and Prasad, 2021). Essentially, with the support of new genome analysis tools, epigenomics can be integrated with the investigation of non-coding RNA, cis-regulatory elements, and other non-genic variations controlling plant abiotic and biotic stress responses (Crisp et al., 2021; Zenda et al., 2021), to facilitate epigenetics-assisted breeding of crops (Gogolev et al., 2021).
Omics Facilitated Crop Improvement for Abiotic and Biotic Stress Resistances
In this section, we shall briefly highlight, with several relevant examples, how the omics approaches and technologies have been successfully used in many studies focusing on abiotic and biotic stress responses in diverse crop species.
Transcriptomics
Transcriptome profiling offers a global snapshot of the entire RNA molecules, including mRNA, tRNA, rRNA, sRNA, and other non-coding RNA within a cell, tissue, organ, or whole organism at any given time point, which is not possible to be investigated at the genomic level (Weckwerth et al., 2020; Chaturvedi et al., 2021). Understanding the transcriptome is crucial for deducing the genome‘s functional elements and revealing the molecular components of cells or tissues, understanding cells‘ responses to developmental and environmental stimuli triggered changes (Wang et al., 2009). Unlike the genome which is stable, the transcriptome is variable under different conditions (developmental stage, type of tissue, environmental stimuli, etc.), and is therefore a promising molecular level for exploring an organism’s stress responses (Kukurba and Montgomery, 2015; Escandón et al., 2021). Different technologies for deducing and quantifying the transcriptome have been established, including hybridization-or sequence-based methods (Wang et al., 2009). Such techniques are categorized as either targeted (microarray or reverse transcription-quantitative PCR (RT-qPCR) based) or untargeted (RNA-sequencing based) transcriptomic approaches (Escandón et al., 2021). Whereas hybridization-based methods usually encompass incubating fluorescently labeled cDNA with microarrays, sequence-based methods directly determine the cDNA sequence (for extensive review, see Wang et al., 2009).
These genome sequencing techniques have evolved over decades (see Section “High Quality Reference Genomes as Vital Resources for Accurate Annotation of Gene Structure, Content and Variation” above). Notably, the recent progress in high-throughput genome sequencing approaches and sequencing costs reduction has revolutionized the genomics research field. Particularly, this has brought about RNA-seq, a modern technique for both transcriptome mapping and quantification (Wang et al., 2009). Compared to other approaches, RNA-seq based method possesses several advantages of lower costs, a wider dynamic range, higher sensitivity, ability to provide whole-genome coverage, and applicability to non-model species (Kircher and Kelso, 2010; Chaturvedi et al., 2021), and has since provided unprecedented opportunities for conducting abiotic and biotic stress response studies in various crop species (Table 3). In particular, comparative transcriptomic approach has been widely applied in gene differential expression analysis in plants exposed to with- and without stress treatments in several crop species. For example, in a maize salinity stress response study, the tolerant genotype exhibited specific functional genes involved in salt tolerance, particularly CBL-interacting kinase (Zm00001d044642), salt stress induced protein (Zm00001d023516), thioredoxins (Zm00001d018238, Zm00001d041804 and Zm00001d018461), defense genes such as leucine-rich repeat protein (Zm00001d035756) and pathogenesis-related protein (Zm00001d018324), and TF genes belonging to MYB (Zm00001d053220), WRKY (Zm00001d005622) and bZIP (Zm00001d043992) families, most of which were involved in the ABA signaling pathway (Zhang et al., 2021) and have been previously implicated in salt (Chen et al., 2013, 2014, Zhao C. et al., 2018) and drought (Zenda et al., 2019) stress tolerances. Besides, B73 maize plants grown under heat and control conditions revealed that several TF gene families including AP2-EREBP (GRMZM2G010555, etc.), b-ZIP (GRMZM2G479760, etc.), bHLH (GRMZM2G001930, etc.), and WRKY (GRMZM2G324999, GRMZM2G071907, etc.), and HSPs (GRMZM2G069651, GRMZM2G366532, GRMZM2G149647, etc.) were significantly enriched in the protein processing in endoplasmic reticulum (PPER) pathway, which played a key role in maize heat stress response (Qian et al., 2019). Moreover, Tifleaf 3 pearl millet genotype plants grown under heat and drought stress conditions showed that out of the nine ROS production related DEGs (two amine oxidases and seven polyamine oxidases), only two DEGs (i2_LQ_LWC_c7872/f15p2/2851 and i1_LQ_LWC_c34699/f1p0/1833) were up-regulated in response to heat stress, suggesting the inhibition of ROS production after 48 hr of heat stress (Sun et al., 2020). Additionally, they identified five ROS scavenging enzymes, including SOD (i0_LQ_LWC_c2218/f1p0/833), CAT (i2_HQ_LWC_c41068/f2p7/2070), APX (i1_LQ_LWC_c18498/f1p3/1627, i3_LQ_LWC_c37944/f1p0/3280, etc.), and thirty HSPs (including i2_HQ_LWC_c49563/f2p1/2825, i2_HQ_LWC_ c43630/f6p12/2432, sHSP i0_LQ_LWC_c967/f1p0/765, etc.) that were up-regulated in response to heat stress (Sun et al., 2020). Under drought stress conditions, two Asr genes (i1_LQ_LWC_c40079/f7p0/1159 and i0_HQ_LWC_c31/f2p0/781) were up-regulated, suggesting the critical role of these LEA proteins in drought stress tolerance. Most of the genes were involved in photosynthesis, starch and sucrose metabolism, circadian rhythm, phenylpropanoid, and glycerophospholipid metabolic pathways (Sun et al., 2020).
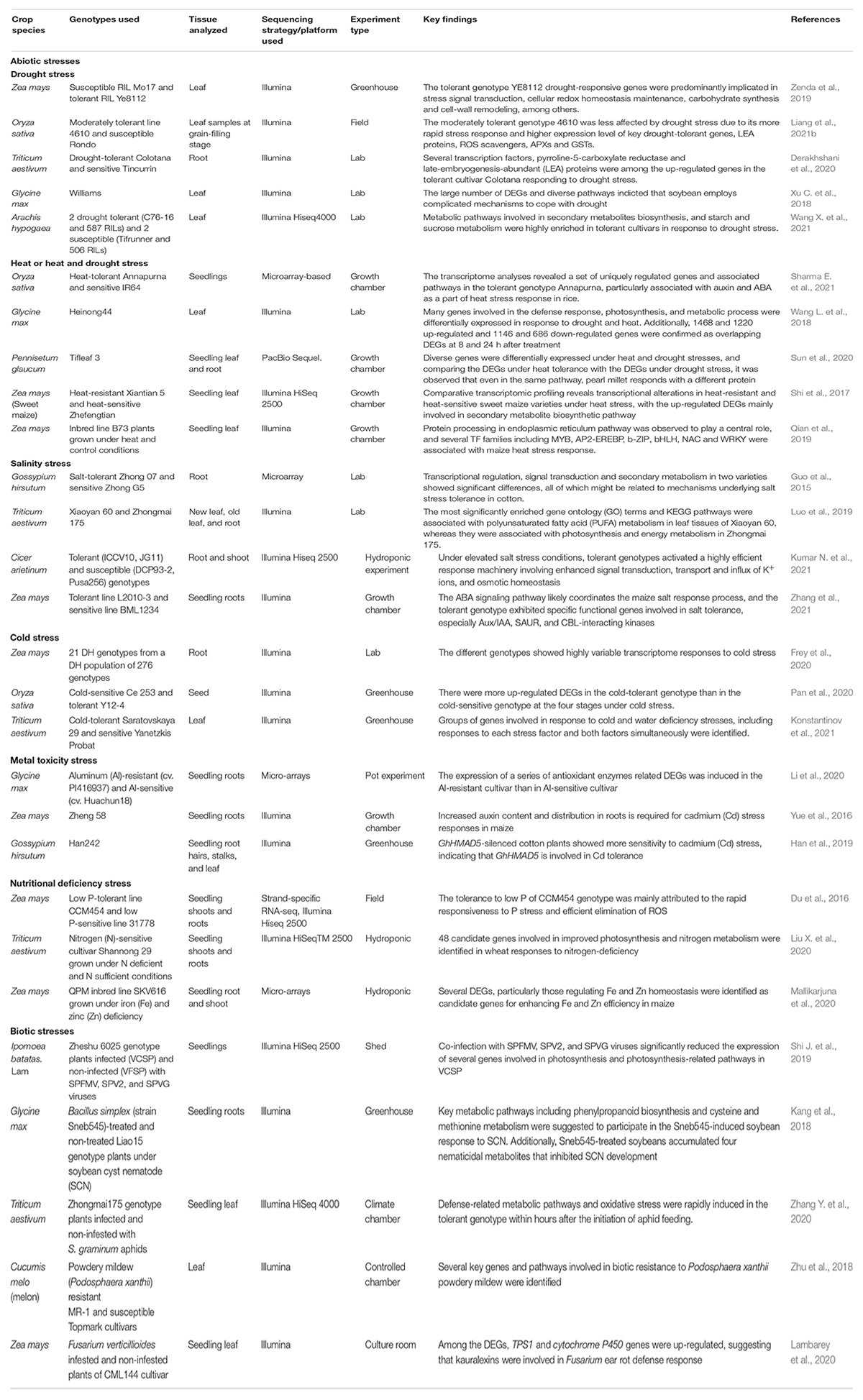
Table 3. Selected examples of transcriptomic studies for abiotic and biotic stress tolerance in different crop species.
In cotton, GhHMAD5-silenced cotton plants exhibited more sensitivity to cadmium (Cd) stress, demonstrating that GhHMAD5 gene is involved in Cd tolerance (Han et al., 2019). In rice, the relatively tolerant genotype 4610 got less affected by drought stress than the susceptible genotype Rondo due to its more rapid stress response and higher expression of key drought-tolerance genes at the grain filling stage, including dehydrin rab (responsive to ABA) 16C (Os11g0454000) and Rab21 (Os11g454300), one bZIP TF (Os01g0658900), some known LEA proteins (Os01g0705200, Os11g0454200), ascorbate peroxidase (APX) (Os04g0434800), RIC2 family protein (Os03g0286900), drought and salt stress response 1 (Os09g0109600), and two HSP (Os02g0232000, Os03g0277300) genes (Liang et al., 2021b). In wheat, aphids (Schizaphis graminum) attack significantly increased the expression levels of several genes related to the salicyclic acid (SA) and jasmonic acid (JA) signaling pathways, including lipoxygenase (LOX, TraesCS4B01G037700, etc.), FAD (TraesCS4A01G109300, etc.), phenylalanine ammonia-lyase (PAL, TraesCS2A01G196700, etc.), and PR1 (TraesCS7D01G161200, TraesCS5A01G183300, etc.) genes (Zhang Y. et al., 2020). Additionally, several ROS scavenging enzymes such as POD (TraesCS2B01G125200, TraesCS2A01G107500, etc.), SOD (TraesCS2D01G123300) and CAT (TraesCS6A01G041700), as well as mitogen-activated protein kinases (Novel11623, TraesCS4D01G198600, etc.) and WRKY TF genes (Novel00700, Novel01914, etc.) were up-regulated in response to aphid attack (Zhang Y. et al., 2020). These results suggest that the SA, JA, protein phosphatases and MAPK-WRKY signaling pathways are the central metabolic pathways activated in response to aphid attack and can be targeted for aphid tolerance breeding. Thus, transcriptomic analysis has become central in abiotic and biotic stress tolerance studies (Li et al., 2019; Kaur et al., 2021; Table 3), and genes and metabolic pathways identified in these studies can be used as targets in marker assisted breeding programs.
With the large amount of data that has been generated and deposited into various public repositories, it is now possible to conduct meta-analysis of transcriptomic responses to abiotic and biotic stresses. It is now possible to acquire more reliable results by integrating information from multiple sources, and we can now study the expression and co-expression patterns of several genes under different abiotic stresses (Cohen and Leach, 2019; Tahmasebi et al., 2019). For instance, a meta-analysis of biotic and abiotic stress responses in tomato was performed by analyzing 391 microarray samples from 23 different experiments and 2,336 DEGs involved in multiple stresses were identified, including 1,862 DEGs responding to biotic and 835 DEGs responding to abiotic stresses, of which 4.2% of those DEGs belonged to various TF families (Ashrafi-Dehkordi et al., 2018). Among these TF genes, Jasmonate Ethylene Response Factor 1 (JERF1), MYB48, EIL2, EIL3, protein LATE ELONGATED HYPOCOTYL (LHY), and SlGRAS6 played critical roles in biotic and abiotic stress responses (Ashrafi-Dehkordi et al., 2018). Therefore, meta-analysis can be used for characterization and identification of candidate genes for both biotic and abiotic stress tolerance and the identified genes pinpointed as potential targets for the genetic engineering of improved stress tolerance crops.
Meanwhile, single cell transcriptomics (SCT) is slowly becoming the major omics approach for plant biology studies. Since its first assessment attempt in 2013, single cell transcriptome profiling has become an indispensable tool for decoding cell type, transcriptomic signatures, and performing single-cell transcriptomics of ncRNAs (Pratik, 2018). Although the greatest technical hurdles to adopting single-cell protocols to plants are related to dissociating cells from the appropriate tissues, obtaining sufficiently high numbers of cells for high-throughput analysis, the technical noise associated with single-cell assays, and the lack of true biological replicates (Efroni and Birnbaum, 2016), matching SCT analysis tools and algorithms are being developed to facilitate the use of SCT approach in molecular biology research (Gogolev et al., 2021). Recently, some researchers have used isolated protoplast or nuclei to successfully establish Arabidopsis roots and stomatal cells (Jean-Baptiste et al., 2019; Liu Z. et al., 2020), as well as maize anther cell transcriptomes (Nelms and Walbot, 2019; Xu et al., 2021) at the single-cell level (Thibivilliers and Libault, 2021). Further, single-cell ATAC-seq (Assay for Transposase Accessible Chromatin-sequencing) has been applied on nuclei isolated from Arabidopsis roots and different maize organs to divulge the differential chromatin accessibility between plant cell types (Thibivilliers and Libault, 2021). For instance, single cell RNA-seq has been applied to Arabidopsis root cells to capture gene expressions in 3121 root cells and hundreds of genes with cell-type–specific expressions were identified, revealing both known and novel genes that are expressed along the developmental trajectories of cell lineages (Jean-Baptiste et al., 2019). Additionally, single-nuclei RNA-seq has been integrated with ATAC-seq datasets to reveal how chromatin accessibility controls gene expression and the differential organization of the Arabidopsis genome between cell types (Farmer et al., 2021). As a result, these studies have shown the significant virtues of single-cell RNA-seq to detect rare cell types and resolve developmental trajectories in complex tissues, and have offered rare insights into the processes of cell differentiation, tissue-specific abiotic stress responses, cell-type-specific responses to genetic perturbations, and cell-cycle interactions (Denyer et al., 2019; Jean-Baptiste et al., 2019; Denyer and Timmermans, 2021). Thus, SCT approach is improving the spatiotemporal resolution of our analyses to the individual cell level, and is quickly expanding the portfolio of available tools and applications for plant molecular biology research (Rich-Griffin et al., 2020; Giacomello, 2021; Seyfferth et al., 2021). However, to harness the potential benefits of the SCT and to popularize its use in plant biology research, a lot of issues still need to be resolved, among which include the optimization of cell-isolation protocols, discerning the number of cells and sequencing reads required, and accommodating abiotic/biotic stress responses (Denyer and Timmermans, 2021).
Proteomics
The proteomics domain involves the large-scale analysis of the proteome profile within an organism, tissue or cell, during normal organismal growth and development or in response to the fluctuations in environmental conditions. It aims to reveal the protein diversity, abundance, isoforms, localization, interactions with other proteins and post-translational modifications (PTMs) (Hashiguchi et al., 2010; Kosova et al., 2018; Labuschagne, 2018). It has been well acknowledged that the mRNA expressed at the transcriptional level is not directly linked with the plant phenotype; hence, it poorly correlates with the phenotype. However, the proteins are the direct effectors of the plant responses to developmental or environmental changes. Therefore, proteomics is a crucial link between transcriptomics and metabolomics (Tan et al., 2017; Labuschagne, 2018). Moreover, the proteome, unlike the genome which is static, is dynamic and the evaluation of proteins takes into account the effects of PTMs, thereby providing more information in understanding biological functions (Wu et al., 2016; Wu and Wang, 2016).
Several proteomics approaches have been deployed in molecular biology studies, and they are generally categorized into gel-based and gel-free-based techniques, coupled with mass spectrometry (MS) for protein identification, fractionation and analysis, as well as data processing techniques (reviewed in Mustafa and Komatsu, 2021; Sinha and Verma, 2021). On one hand, gel based proteomic approaches encompass initial protein separation by way of gel electrophoresis, followed by quantification, digestion and identification through MS. Examples of gel-based techniques include one or two dimensional polyacrylamide gel electrophoresis (1- or 2- DE) and differential in-gel electrophoresis (DIGE) (Tan et al., 2017; Labuschagne, 2018). On the other hand, gel-free technologies, which involve the digestion of intact proteins (via protease degradation) into peptides prior to liquid chromatographic (LC) separation and MS identification, include the isobaric tags for relative and absolute quantitation (iTRAQ), isotope-coded affinity tags (ICAT), and targeted mass tags (TMT), among others (extensively discussed in Chandramouli and Qian, 2009; Hu et al., 2015; Ghatak et al., 2017; Tan et al., 2017; Vo et al., 2021).
During the past two decades, the scientific community has witnessed tremendous advances in plant proteomics, largely characterized by the refinement of the conventional techniques and advent of modern, high throughput, and high-resolution approaches related to samples preparation and protein extraction, fractionation, quantification and analysis; proteomics data processing and analysis, among other areas (Ross et al., 2004; Matros et al., 2011; Agrawal et al., 2013; Tan et al., 2017). For instance, the proteomics field has seen a gradual shift from the general descriptive studies of plant protein abundances and covalent modifications to large scale analysis of protein-metabolite interactions (PMIs) and protein-protein interactions (PPIs) (Ramalingam et al., 2015; Scossa et al., 2021). These advances have been necessitated largely by the recent developments in LC-tandem MS systems, which have significantly improved their resolution and scanning rates. Particularly, the PMI field has been given special attention due to the role metabolites play, not only as metabolic intermediates, but also as co-factors or ligands with the capacity to alter protein confirmations and functions (Scossa et al., 2021). Detailed discussions on the advances made in plant proteomics can be accessed in numerous previous reviews (Agrawal et al., 2013; Ghatak et al., 2017; Kosova et al., 2018; Labuschagne, 2018; Raza et al., 2021a; Sinha and Verma, 2021).
Several next generation quantitative proteomic techniques have been widely employed in descriptive and comparative plant abiotic and biotic stress response studies (Ahmad et al., 2016; Mustafa and Komatsu, 2021). For instance, an iTRAQ-based comparative proteomics study to investigate the salinity-responsive proteins and related metabolic pathways in two contrasting rice genotypes at the maximum tillering stage identified 368 and 491 proteins that were up-regulated in the tolerant genotype LYP9 under moderate salinity and high salinity stress, respectively (Hussain et al., 2019). Among the highly expressed proteins were those involved in redox reactions, including peroxidases (gi| 125525683), glutathione -S- transferase (gi| 115459582) and SOD (gi| 125604340); salt stress-responsive proteins including malate dehydrogenase (gi| 115482534), methyltransferase (gi| 115477769), glucanase (gi| 13249140), pyruvate dehydrogenase (gi| 125564321), glutathione peroxidase (gi| 125540587), fructose-bisphosphate aldolase (gi| 218196772), and triosephosphate isomerase (gi| 125528336); photosynthesis related proteins including psbP-like protein 1 (gi| 38636895), thylakoid lumenal protein (gi| 115477166), ferredoxin-thioredoxin reductase (gi| 115447507), psbP domain-containing protein 6 (gi| 115440559), and photosystem II oxygen-evolving complex protein 2 (gi| 164375543); and carbohydrate metabolism related proteins such as xyloglucan endotransglycosylase/hydrolase protein (gi| 115475445), polygalacturonase (gi| 115479865) and β-glucosidase (gi| 115454825) (Hussain et al., 2019). In another comparative study, 2-DE proteomics analysis complemented with MALDI TOF mass spectrometry revealed 39 key proteins that mediate soybean response to heat stress, water stress and combined stresses, especially those involved in metabolism [alanine aminotransferase 2 (A8IKE5), glutamine synthetase (O82560), serine hydroxy methyl transferase 5 (C6ZJZ0), translation elongation factor (O23963), pyruvate dehydrogenase (E5RPJ6), etc.], response to heat [HSP70 (P26413), HSP22 (mitochondrial) (Q39818), HSP 17.6 kda class 1(P04795), 17.7 kda class 1 HSP (B4 × 941)], and photosynthesis [Rubisco activase (D4N5G3), oxygen-evolving enhancer protein 2 (I1JJ05), glyceraldehyde 3-phosphate dehydrogenase (Q2IOH4), chlorophyll A/B-binding protein (Q39831), etc.] showing significant cross-tolerance mechanisms in the tolerant genotype PI-471938 (Katam et al., 2020).
Further, a comparative iTRAQ proteomics analysis for wheat stripe rust (Puccinia striiformis f. sp. tritici) resistance in wheat cultivar Suwon11 revealed a set of ROS metabolism-related proteins, peptidyl–prolyl cis–trans isomerases (PPIases), RNA-binding proteins (RBPs), and chaperonins that were involved in the response to Pst infection (Yang Y. et al., 2016). Among the 42 ROS metabolism-related proteins (encompassing GPXs, CATs, and peroxiredoxins), 11 peroxidases were strongly induced at both 24 and 48 hpi. Twelve PPIases (including AEGTA05000, AEGTA08970, AEGTA26095, AEGTA06390, etc.) were strongly up-regulated at 24 hpi. Moreover, thirteen RBPs, including one alternative splicing regulator (AEGTA28251), one arginine/serine-rich splicing factor (TRAES3BF080700020CFD_c1) and two predicted glycine-rich RBPs (AEGTA28395 and TRAES3BF152900030CFD_c1) were significantly altered (by exhibiting up-regulation) during the incompatible interaction, particularly at 24 hpi. Further, six chaperonins were also up-regulated at 24 hpi (Yang Y. et al., 2016). Besides, a comparative label-free quantitative proteomic analysis of three sorghum genotypes with variable resistance to spotted stem borer (Chilo partellus) insect pest identified putative leaf C. partellus responsive proteins. Among a total of 967 C. partellus-responsive proteins, those involved in stress and defense, photosynthesis, small molecule biosynthesis, amino acid metabolism, catalytic and translation regulation activities were significantly up-regulated in resistant sorghum genotypes upon pest infestation (Tamhane et al., 2021). Especially, known defense proteins such as pathogenesis related protein 5 (PR-5), thaumatin like pathogenesis related protein 1, chitin-binding type-1 domaincontaining protein, osmotin, calmodulin, peroxidases, glutathione S-transferase, expansin-like EG45 domaincontaining protein, non-specific lipid transfer protein, abscisic acid stress ripening 3, and alpha-amylase/trypsin inhibitor were amongst the candidate proteins identified (Tamhane et al., 2021), strengthening their role in plant defense against insect pest and pathogen attack (War et al., 2012; Zhang et al., 2018). Other proteomics studies aimed at identifying key proteins associated with responses to several abiotic and biotic stresses are available (Hu et al., 2015; Luo et al., 2018; Table 4). Overall, the information generated from these proteomic studies can be an invaluable resource for crop breeding programs, as it facilitates for potential markers identification, candidate proteins isolation and incorporation into breeding pipelines via proteomics-driven-marker assisted selection and protein-marker-centered gene pyramiding (Agrawal et al., 2012; Labuschagne, 2018).
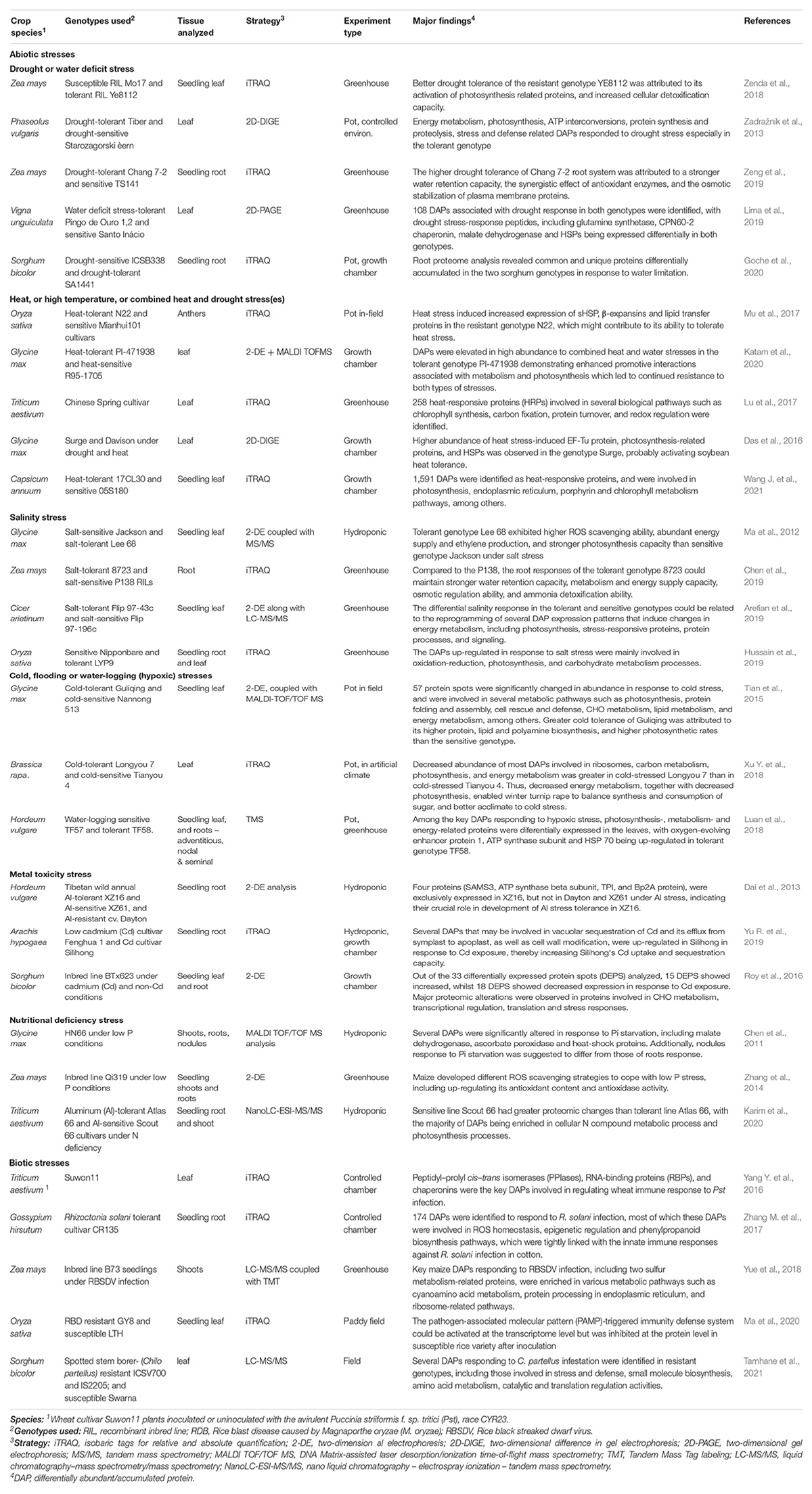
Table 4. Examples of proteomic studies for abiotic and biotic stress tolerance in different crop species.
Meanwhile, protein PTMs such as phosphorylation, nitrosylation and ubiquitination are central in the modulation of several cellular functions in plants, including metabolism, signaling transduction, gene expression, protein stability and interactions, and enzyme kinetics, as well as plant-environmental interactions (Kaufmann et al., 2011; Hashiguchi and Komatsu, 2017; Tan et al., 2017). Therefore, systematic investigations of these PTMs is critical for gaining insights into several regulatory mechanisms underpinning biological processes, including plant stress responses (Tan et al., 2017). Fortunately, the study of protein PTMs is increasingly gaining attention in plant science, particularly on their role in abiotic stresses (Wu et al., 2016; Haak et al., 2017; Stone, 2019; Martí et al., 2020) and plant immunity (De Vega et al., 2018; Zhang and Zeng, 2020). This is being driven by MS-based identification and analytical approaches in targeted proteomics (extensively reviewed in Arsova et al., 2018), as well as new innovations to study complex PTMs and integrate them with other domains such as epigenetics (Wu et al., 2016). For instance, MS-based analysis of chromatin has emerged as an indispensible tool for the identification of proteins linked to gene regulation, as it facilitates studying of protein functions and protein complex formation in their in vivo chromatin-bound context (van Mierlo and Vermeulen, 2021). Going forward, our ability to identify and quantify PTMs, supported by robust, efficient and high-throughput analytical and computational tools, will facilitate for large-scale comprehensive protein functional characterization that will enhance our knowledge of the crop stress acclimation and tolerance acquisition (Wu et al., 2016; Arsova et al., 2018).
Metabolomics
In response to various environmental and pathogenic stresses, plants institute sophisticated physiological, biochemical and molecular mechanisms, including biosynthesis of a diverse range of metabolites, antioxidant enzymes activation, ions uptake and transport, osmoprotectants (especially proline) accumulation, and phytohormones release, among others (Pandey et al., 2015; Singhal R.K. et al., 2021). Metabolites encompass hundreds or thousands of primary or secondary compounds such as organic acids, sugar alcohols, hormones, allelochemicals, ketones, amino acids, steroids, etc. (Razzaq et al., 2019; Singhal R.K. et al., 2021). More crucially, plants have been observed to undergo metabolic adjustments in order to acclimate to predominant stress conditions by synthesizing anti-stress components including antioxidants, compatible solutes and stress-responsive proteins (Ramalingam et al., 2015). Therefore, metabolomics is aimed at qualitatively and quantitatively detecting, quantifying and analyzing all low molecular weight metabolites (called metabolome) within a cell, tissue, or an organism synthesized via cellular metabolism at a specific developmental stage, and/or in response to certain environmental stimuli (Fiehn, 2002; Arbona et al., 2013).
Owing to their close link to the phenotypic expression more than the mRNA transcripts and proteins, metabolites more precisely reflect the connection between gene expressions, protein interactions and diverse regulatory processes, as well as offering a direct functional readout of the physiological state of the cell (Arbona et al., 2013; Ramalingam et al., 2015; Pinu et al., 2019). Therefore, metabolomics, integrated with mass spectrometric and bioinformatics analyses, is an indispensable tool to study plant molecular responses to abiotic and biotic stresses, since alterations in the flux of both primary and secondary metabolites can be observed and analyzed against several stress conditions (Singh N. et al., 2021). Thus, in a bottom-up approach of omics integration, metabolomics data can be used to target subsequent up-stream proteomics or transcriptomics analyses to uncover mechanistic genes or proteins driving the processes of plant responses to stresses (Saito and Matsuda, 2010; Pinu et al., 2019). In other words, metabolomics is a more appropriate foundation for developing plant phenotype biomarkers and cross-omics biomarkers since it integrates genetic and non-genetic factors (Jendoubi, 2021).
Major plant metabolomics methods comprise metabolite profiling (focusing on metabolites with similar and specific chemical properties, and requires separation techniques), metabolic fingerprinting (without the need for separation technique, and uses different kinds of analyzers to compare sets of spectra and hence the samples from which the spectra were derived), and targeted analysis (identification and quantitative analysis of targeted metabolic compounds) (Krishnan et al., 2005; Arbona et al., 2013; Ramalingam et al., 2015). These approaches can be applied individually or in integration depending on the objective of the study (Ramalingam et al., 2015).
Most notably, the post-genomics period has seen massive improvements in the traditional (separation and MS based) methods to cutting-edge technologies that are facilitating for cost-efficient and high-throughput ways for molecular detection, quantification and analysis of a diverse range of metabolites (Kumar et al., 2017; Scossa et al., 2021). It is not surprising that the metabolomics domain is fastly receiving attention in both basic and applied plant research. More specifically, the advent of “hyphenated” separation methods and several detection systems has facilitated for systematic detection, quantification and analysis of a vast array of plant metabolites (Fraire-Velázquez and Balderas-Hernández, 2013). Liquid chromatography (LC), gas chromatography (GC) and capillary electrophoresis (CE) comprise the separation methods, whereas different types of MS, including MS, LC-MS, flow injection analysis coupled to MS (FIA/MS), ultraviolet light spectroscopy (UV/VIS), nuclear magnetic resonance (NMR), and high resolution mass spectrometry (HRMS) technologies are used for detection (Arbona et al., 2013; Fraire-Velázquez and Balderas-Hernández, 2013; Li et al., 2019). Direct infusion mass spectrometry (DIMS) and Fourier transform ion cyclotron resonance mass spectrometry (FT-ICR-MS) are specialized techniques normally used in direct infusion mode for metabolomics analyses since their high mass accuracy permits for separation to be achieved entirely based on this parameter (Fraire-Velázquez and Balderas-Hernández, 2013; Villate et al., 2021). Applicability and limitations of these metabolomics methods and techniques have been extensively discussed in previous articles (Allwood et al., 2011; Razzaq et al., 2019; Hamany Djande et al., 2020; Kaur et al., 2021).
Crucially, over the past decade, metabolomics approaches have facilitated for data mining and interpretation for structural elucidation of complex biological networks underpinning plants‘ responses to abiotic and biotic stresses (Saito and Matsuda, 2010; Resham et al., 2014; Barupal et al., 2018; Sharma V. et al., 2021). For instance, a comparative metabolic investigation of drought stress tolerance in contrasting groundnut genotypes using GC-MS, HPLC and UPLC-MS/MS analyses identified 46 key drought responsive metabolites (including pentitol, phytol, xylonic acid, d-xylopyranose, stearic acid, and d-ribose, agmatine, cadaverine, etc.). Among these, agmatine and cadaverine were accumulated in both roots and leaves, and were suggested as potential polyamines for drought tolerance (Gundaraniya et al., 2020). Additionally, seven metabolic pathways (including galactose metabolism, starch and sucrose metabolism, pentose and glucuronate interconversion, etc.) were revealed as critical in groundnut response to drought stress (Gundaraniya et al., 2020). These findings can augment transcriptomic and proteomic inquiries aimed at improving drought tolerance in groundnut. Besides, metabolomic profiling of soybean leaf tissues by GC-MS and LC-MS analyses revealed the role of phytochemical metabolism, as well as sugar and nitrogen metabolism in conferring tolerance to combined drought and heat stress conditions (Das et al., 2017). Integrated metabolomic, transcriptomic and gene regulatory network analyses of common rust (Puccinia sorghi) resistance in maize identified a number of Rp1-D-mediated defense response metabolites (including chlorogenic acid, caffeic acid, ferulic acid, flavonoids, terpenoids, kauralexins and zealexins) and genes involved in SA biosynthesis (especially, calmodulin-binding protein 60G and systemic acquired resistance deficient 1, SARD 1; and several TFs such as WRKY53, BZIP84, NKD1, BHLH124 and MYB100) as potentially critical regulators of P. sorghi resistance in maize (Kim S.B. et al., 2021). Additionally, they revealed a number of secondary metabolite biosynthesis (especially “phenylpropanoid and phenolics” and “terpenoid biosynthesis”) pathways as key in modulating common rust defense response in maize (Kim S.B. et al., 2021). Further, metabolic profiling of root lesion nematode (Pratylenchus thornei) resistant and susceptible wheat genotypes using UHPLC-QTOF analysis revealed that metabolites belonging to the fatty acids, flavonoid, glycerolipid, alkaloids, and steroid glycoside classes were constitutively expressed in the resistant wheat genotype (QT16258) roots (Rahaman et al., 2021), suggesting that the induction of these compounds in roots is a part of the inducible chemical arsenal that wheat employs to counteract root lesion nematode infection. Besides these few examples highlighted here, several other metabolic studies for crop improvement are listed (Table 5) and reviewed (Kumar et al., 2017; Kaur et al., 2021; Singhal R.K. et al., 2021; Vo et al., 2021). Taken together, metabolic profiles identified from these comparative studies can fortify transcriptomics and proteomics findings or can be utilized as signatures for evaluating the genetic diversity among different cultivars or species of the same genotype at different crop growth phases and environments and could guide tailoring of genotypes for desired or targeted performance under specific growth conditions, i.e., designing and creating crop varieties best suited to specific agricultural environments (Fraire-Velázquez and Balderas-Hernández, 2013).
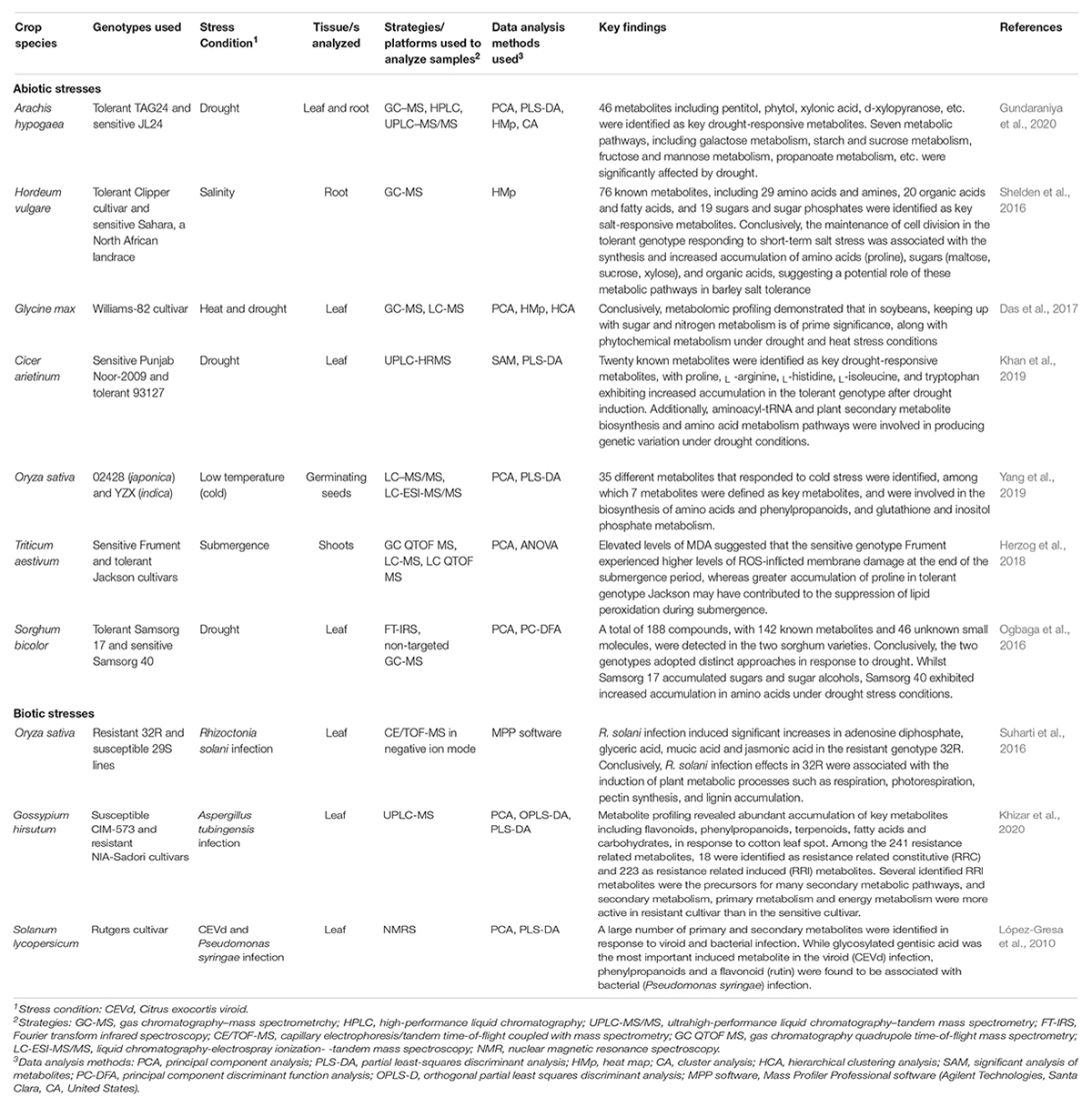
Table 5. Selected examples of metabolomics studies to help understand abiotic and biotic stress tolerance mechanisms in different crop species.
Large-scale metabolite profiling is offering convenience in accessing the global metabolites data sets and their corresponding metabolic pathways in an unparalleled way (Kumar et al., 2017). Thus, plant metabolomics has provided gateways in the discovery of new metabolic pathways and its integration with other omics has improved existing genome annotations. Moreover, metabolic-based quantitative trait loci (mbQTL) mapping is fastly proving to be an effective approach for identifying stress-responsive trait pathways (reviewed in Sharma V. et al., 2021). Complementary to genetic QTLs, proteomic QTLs and epigenetic QTLs, mbQTLs are also employed for quantitative traits mapping and identification of genetic variations at the metabolic level. Consequently, GWASs based on mbQTLs and metabolomics GWAS (mbGWAS) have become key in detecting genetic variations associated with metabolic traits in plants, thereby facilitating metabolomics-assisted breeding of crops (reviewed in Razzaq et al., 2019; Kumar R. et al., 2021). For instance, a metabolic profiling of barley flag leaves under drought stress conditions identified 57 mbQTLs for metabolites linked to primary carbon and nitrogen metabolism, as well as antioxidant metabolism pathways. Interestingly, mbQTLs for flag leaf γ-tocopherol, glutathione and succinate content were observed (by association mapping) to co-localize with the genes encoding enzymes of the pathways synthesizing these antioxidant metabolites (Templer et al., 2017).
Looking ahead, embracing the current trends in new technologies and approaches in crop biotechnology, the metabolite investigation of mutants and transgenic lines holds much promise in elucidating the metabolic networks and pinpointing the candidate genes underpinning crop stress responses. Additionally, an integrated omics approach encompassing inferences from genomics, transcriptomics, proteomics, and metabolomics will facilitate for cataloging and focusing on key genes for improving key traits of agronomic importance in crops (Kumar et al., 2017).
Omics Facilitated Crop Improvement for Nutritive Traits
Global climate changes such as increased temperature and elevated CO2 levels are associated with decreased nutrient density of some staple crops, ultimately worsening the serious human health challenges suffered by billions of malnourished people in low-income countries (Myers et al., 2014; Macdiarmid and Whybrow, 2019). Moreover, the projected changes could cause reductions in yields of both staple cereal and non-staple legume and vegetable crops, potentially affecting their global availability, affordability and consumption (Scheelbeek et al., 2018; Wang J. et al., 2018; Ray et al., 2019). Since crops are the primary sources of essential nutrients including vitamins, iron (Fe), zinc (Zn), folate, fiber, etc., limited access and consumption of plant-based diets could have serious health implications such as increased risk of non-communicable diseases, and increased nutritional deficiencies that may be difficult to rectify through substitution with other foods (Scheelbeek et al., 2018). In the wake of such climate change scenarios and the need to address human health challenges, improving crop nutritional quality through breeding, agronomic interventions or transgenic approaches become critical. Particularly, enhancing crop micronutrient (particularly Zn, Fe, and vitamins) densities by genetic biofortification through breeding has emerged as a promising, cost-effective and sustainable way to ensure healthy diets to millions of people (Qamar-uz et al., 2017; Garg et al., 2018; Wakeel et al., 2018; Kumar S. et al., 2019).
In order to achieve successful crop nutritional quality improvement, precise identification of major QTLs, genes and metabolic pathways that help interpret the genetic architecture related to plant nutrient acquisition is essential. To this end, several genomic and other omics techniques have been employed to target these nutritive traits, information of which has guided GAB programs (Singh R.K. et al., 2020; Roorkiwal et al., 2021). Major QTLs for nutrition-related traits have been identified in major cereals (reviewed in Singh R.K. et al., 2020) and legumes (reviewed in Roorkiwal et al., 2021). For instance, 14 rice QTLs for cooking and eating quality of grain (including qTV9 on chr 9) (Park et al., 2019), and 23 rice QTLs for Fe and Zn concentration in grain harboring several candidate genes (including OsZIP6 on QTL qZn5.1.) (Calayugan et al., 2020) were detected. In wheat, five QTLs for gluten strength (including QGlu.spa-1A and QGlu.spa-1B.1 on chr 1A and 1B, respectively) were identified (Ruan et al., 2020). Additionally, 16 wheat QTLs for grain Fe, Zn and protein contents, and 1000-kernel weight were identified, encompassing four Fe QTLs (QGFe.iari-2A, QGFe.iari-5A, QGFe.iari-7A and QGFe.iari-7B), five Zn QTLs (QGZn.iari-2A, QGZn.iari-4A, QGZn.iari-5A, QGZn.iari-7A and QGZn.iari-7B), two protein content QTLs (QGpc.iari-2A and QGpc.iari-3A), and five 1000-kernel weight QTLs (QTkw.iari-1A, QTkw.iari-2A, QTkw.iari-2B, QTkw.iari-5B and QTkw.iari-7A) (Krishnappa et al., 2017). Besides, 21 QTLs for kernel oil and protein content (including qOIL08-01, qOIL10-01, qOIL05-01 and qOIL06-1 for oil content, and qPRO01-01, qPRO05-01 and qPRO06- 1 for protein content) were identified in maize (Yang Z. et al., 2016). In legumes, QTLs for seed Fe and Zn concentrations in chickpea (Upadhyaya et al., 2016); QTLs affecting seed hardiness in common bean (Sandhu et al., 2018), 8 stable QTLs controlling oil and protein content in soybean (Huang J. et al., 2020), and several QTLs governing oil content, protein content, and fatty acids (linoleic and oleic acids) in groundnut (Sarvamangala et al., 2011; Shasidhar et al., 2017; Roorkiwal et al., 2021) were identified, among others.
In a recent study, using a population of 190 genotypes, Puranik et al. (2020) applied an integration of GBS and GWAS mapping to perform comparative genomics related to identification of genomic regions controlling grain nutrient content (for Fe, Zn, Ca, Mg, K, Na, and protein) in finger millet (Eleusine coracana L. Gaertn.). By comparative mapping, they identified several marker-trait associations (MTAs) and predicted associated putative candidate genes underlying significant associations, including S1_30253617 and probable mitochondrial 3-hydroxyisobutyrate dehydrogenase-like 1 (LOC101754224) which were associated with iron content, and SNP S1_5982733 encoding a SEUSS-like transcriptional corepressor which was associated with calcium content (Puranik et al., 2020). Besides, Singhal T. et al. (2021) performed a multi-environment QTL mapping for grain iron and zinc content using bi-parental recombinant inbred lines in pearl millet and identified several QTLs for Fe and Zn, and putative candidate genes within those QTLs involved in Fe and Zn content enhancement. Among the genes identified were ferritin 1 – chloroplastic, potassium transporter 3, and aluminum-activated malate transporter 5 (Singhal T. et al., 2021). Considering that pearl millet and other small grains are richly endowed with micro-nutrients and climate-resilience related traits, these candidate QTL regions or genes identified to be linked to such nutritive traits can be targeted for introgression into elite cultivars via GAB (such as marker-assisted backcrossing) or transgenic approaches (Puranik et al., 2020; Roorkiwal et al., 2021). Besides, using multi-omics technologies, cis-regulatory elements (CREs; which are the non-coding DNA containing binding sites for transcriptional factors or other regulatory molecules that influence transcription, Wu et al., 2021) can be precisely identified, analyzed, and targeted for the creation of allelic variation and enhancement of grain quality traits (including grain appearance, milling properties, nutritional value and cooking quality) in crops such as rice via genome editing approaches (Swinnen et al., 2016; Huang L. et al., 2020; Ding et al., 2021).
Meanwhile, maximizing bioavailability of nutrients requires full understanding of the processes involved in crop nutrient uptake, transport, and assimilation into seeds, since multiple genes and complex metabolic pathways are involved. Omics approaches can be applied to help understand the genes and metabolic pathways, including rate limiting steps, involved in nutrient acquisition or biosynthesis, uptake, transport, assimilation and storage processes (Roorkiwal et al., 2021). In particular, manipulating genes and metabolic pathways involved in uptake and transport of Fe, Zn and phosphorus in legumes holds the key for the success of crop nutritional quality improvement. Pathways that can be targeted include beta-carotene biosynthesis, folate biosynthesis, vitamin E biosynthesis and lysine biosynthesis (Kumar A. et al., 2021; Roorkiwal et al., 2021). For instance, metabolomics approaches have been used to target carotenoid biosynthesis pathways (since carotenoids and β-carotene are the primary precursors of vitamin A) and to perform metabolic engineering aimed at increasing β-carotene levels in crops such as rice, maize and potato (reviewed in Sharma V. et al., 2021). Besides, nutritional quality has been improved in maize landraces by enhancing β-carotene content via MABC (Qutub et al., 2021).
Aflatoxin, produced by mostly the fungus Aspergillus flavus and Aspergillus parasiticus, is a harmful mycotoxin whose contamination is common in several agricultural crops including groundnut, maize, cotton seed and tree nuts, both pre- and post-harvest (Klich, 2007; Frisvad et al., 2019). Aflatoxin contamination poses serious human and animal health consequences since aflatoxin is carcinogenic, immune-suppressive, cause liver toxicities and abnormalities of physiological development (Kowalska et al., 2017). Fortunately, in groundnut improvement programs, for instance, genomic advances such as sequencing of groundnut diploid progenitors and the cultivated tetraploid groundnut have presented an unparalleled opportunity for enhancing A. flavus resistance by helping the decoding of genes and genomic regions underlying host resistance to A. flavus. Additionally, metabolomics approaches can be employed to decipher the key metabolic pathways aflatoxin metabolite biosynthesis (reviewed in Ojiewo et al., 2020).
High oleic acid content is a vital quality trait which determines the flavor, stability, shelf-life, and nutritional quality of groundnut and groundnut products. Therefore, the genetic control of this trait is important for high oleic groundnut breeding programs (Amoah et al., 2020). Genetic approaches such as QTL analysis, the use of genetic markers, gene knock-downs and mutants have been successively used to develop high oleic acid (and low linoleic acid) groundnut cultivars, possessing mutated form of FAD (fatty acid dehydrogenase) gene (see Ojiewo et al., 2020). Two homologous sequences of the FAD gene exist as FAD2A and FAD2B, owing to the allotetraploid nature of groundnut. These gene homologs are thought to emanate from the two groundnut species genomes, viz., Arachis ipaensis and Arachis duranensis (Chu et al., 2009; Pandey et al., 2014). The identification of linked allele-specific genetic markers for these two gene homologs has facilitated for breeders to use marker assisted selection and MABC breeding to enhance oleic acid content of elite groundnut varieties (Bera et al., 2018; Desmae et al., 2019). Further, these genomic tools are aiding pyramiding of multiple agronomic traits into a single cultivar (Ojiewo et al., 2020). Going forward, advances in genome sequencing and the availability of diploid and tetraploid genome sequences, as well as the accelerated use of MARS and GS, are envisaged to simplify detection of useful genetic variation, identification of key genes underlying priority traits (such as oleic acid content and low aflatoxin accumulation in groundnut), and introgression of those priority traits into elite cultivars, thereby improving their nutritive value (Desmae et al., 2019).
Phenomics Facilitated Improvement of Crop Agronomic Traits
Since we have already discussed the recent developments in crop phenotyping methods and tools/technologies in our most recent review (Zenda et al., 2021), here, in this current paper, we will only focus on the application of phenomics to target newly emerging research domains for crop improvement.
Phenomics Analysis of Root Traits as a New Avenue for Crop Improvement
Root system architecture (RSA) and anatomical traits have important effects on plant function, including acquisition of soil nutrients and water, and subsequent transportation to the aboveground parts (Meister et al., 2014; Paez-Garcia et al., 2015; Zhao et al., 2019). In the past, the lack of information on the measurable genetic or physiological traits has prompted plant breeders to largely focus on optimizing the crop above-ground parts, neglecting the roots. However, the search for new alternative ways to create climate-resilient future crops is making the optimization of both the below-ground and areal plant parts a priority (González and Manavella, 2021). The RSA acts as a major interface between plants and numerous abiotic and biotic stress factors, and helps plants to adapt to these environmental instabilities by sensing and responding to them (Pandey et al., 2017). Such adaptive mechanism or “developmental plasticity” in root growth and development has presented an opportunity for crop breeders to develop climate resilient crops possessing customized RSA that can better adapt to scavenging for diverse supplies of nutrients under specific soil environments (Hodge, 2004; Reynolds et al., 2021).
Several key root structural traits such as primary root length, lateral root length and density, root angle (gravitropism), root tip diameter, crown root number, root hairs, and anatomical root traits (such as root cortical aerenchyma and cell wall modification) can be targeted for QTL mapping and identification of genes underlying these traits under specific abiotic stress conditions (Paez-Garcia et al., 2015; Wasaya et al., 2018). Then, the identified genes can be manipulated via GAB, reverse or forward genetics approaches, or gene editing techniques to develop crops with customized RSA (reviewed in Paez-Garcia et al., 2015). For example, Guo et al. (2020) combined functional phenomics and root economics space analysis approach in winter wheat and identified some root traits, viz., specific root respiration (SRR) and specific root length (SRL), and genomic regions underlying these traits. In particular, they discovered significant variation in SRR and SRL, which were the key aspects of root metabolic and structural costs, respectively. GWASs for the univariate traits identified numerous underlying genetic regions whereas multivariate and PCA-based GWASs offered an enhanced ability to identify the genetics of the root economics space. Moreover, they identified several SNPs linked to these traits that could be used as vital tools for marker-assisted breeding (Guo et al., 2020).
Besides, greater primary root length density enhanced drought tolerance in winter wheat (Djanaguiraman et al., 2019), whilst reduced lateral root branching density but extended length have also improved drought tolerance in maize by enabling access to water available at greater soil depths (Zhan et al., 2015). As an example, we can target such key RSA traits to identify and manipulate genes underlying these traits. Fortunately, the past few years has witnessed massive development of some novel micro-image acquisition techniques and computer based technologies, coupled with several emerging algorithms and softwares that can handle the microscopic images (see Wasaya et al., 2018; Zhao et al., 2019; Demidchik et al., 2020), as well as high-throughput plant phenotyping (HT3P) approaches (reviewed in Li et al., 2021). We can now leverage on these techniques to phenotype the key root traits at cellular, tissue, or organ levels, and these traits can now be estimated from the lab to the field (Tardieu et al., 2017; Li et al., 2021). Ultimately, harnessing and incorporation of these key root traits into crop breeding programs will facilitate for the development of more climate-resilient and efficient crops for the future (Meister et al., 2014; Wasaya et al., 2018).
Phenomics Applied in Improving Photosynthetic Efficiency and Source-Sink Balance
Photosynthesis process is the basis of plant biomass synthesis or productivity, and the plant photosynthetic machinery is adversely affected by various environmental stressors (reviewed in Muhammad et al., 2021). Therefore, manipulating the photosynthetic processes under environmental fluctuations can be a target for crop improvement (Batista-Silva et al., 2020; González and Manavella, 2021). Phenomics can significantly play a role in accurately detecting plant photosynthetic damages and adaptive response mechanisms under diverse abiotic stress factors, as well predicting the fluctuations in plant biomass or productivity under such environmental conditions (Flood et al., 2016).
Although several bottlenecks in phenotypic evaluation of photosynthesis-related traits have been identified (see Flood et al., 2011), recent advances (and integration) in plant genomics and phenomics technologies have the capability to circumvent these challenges (Furbank and Tester, 2011). Consequently, studying of natural variation (by GWAS analyses) in photosynthesis related traits (including chlorophyll content, chlorophyll reflectance, non-photochemical quenching, photosystem II efficiency, etc.) in diverse crop species under different abiotic stress factors has been made possible (reviewed in van Bezouw et al., 2019). Moving forward, particularly, the investigation of natural variation in photosynthetic efficiency and molecular mechanisms regulating the acclimation of the photosynthetic machinery to these abiotic stresses may be vital in the discovery of novel functional allelic variations, traits and genes that can be targeted for incorporation into current crop improvement programs or used in forward genetic approaches to bio-engineer future crops with enhanced crop photosynthesis efficiency (van Bezouw et al., 2019; Furbank et al., 2020).
It has been long established that photosynthesis flux (source activity) is also dependent on the sink strength (such as grain number and weight in wheat, soybean, rice, etc.). Where an imbalance between source and sink at the whole plant level exists, this can result in reduced expression of photosynthetic genes and accelerated leaf senescence (Paul and Foyer, 2001; Smith et al., 2018). Therefore, the modification of photo-assimilates distribution and accumulation in sink-constrained crops can greatly enhance productivity (Araus et al., 2021). Thus, crop breeders can target increasing mapping and identification of QTLs and genomic regions linked to the rate of grain setting per unit of spike growth at flowering, grain number and grain weight in order to enlarge the sink capacities of crops such as wheat, ultimately improving their photosynthetic efficiencies (Furbank et al., 2020; González and Manavella, 2021; Pretini et al., 2021).
Fortunately, HT3P technologies can facilitate for the analysis of CO2 assimilation from the canopy and leaf level (Furbank et al., 2019, 2020). Especially, HT3P data from chlorophyll fluorescence imaging can provide accurate phenotypic dissection of photosynthesis related traits (Dong et al., 2020), and can help to estimate how much biomass (carbon) crops should devote to their root systems in order to fully and efficiently maximize nutrient acquisition with minimal loss of plant fitness and yield (reviewed in Reynolds et al., 2021). Moreover, root anatomical traits such as cell wall remodeling and cortical aerenchyma can also be targeted for phenotyping and genetics analyses since they have shown to significantly limit root respiration, thereby allowing plants to reallocate their biomass in roots or other above-ground plant parts (reviewed in Reynolds et al., 2021). Taken collectively, improving crop photosynthetic efficiency and sink capacity can be targeted for improvement of crop productivity and resilience under future climate conditions, necessitated by improved phenomic and genomics approaches, coupled with gene-editing or bio-engineering technologies.
Phenomics (Integrated With Multi-Omic Approaches) for Revealing and Exploiting Plant Root-Associated Microbiomes for Improved Crop Health and Climate Resilience
Plant root-associated microbiomes (collection of microbes living inside and around the roots) provide diverse functions that directly influence several plant traits and metabolites are the primary tools plants employ to actively shape their microbiome (De Coninck et al., 2015; Pascale et al., 2020; Chen et al., 2021; Chouhan et al., 2021; Pang et al., 2021). Mechanistically, plant roots exude a cocktail of primary and secondary metabolites which work as growth substrates for some microbial families, exert toxic and antagonistic effects on others, or serve as signals that modulate the plant microbe interactions (Lareen et al., 2016; Chen et al., 2021). Whilst some soil rhizosphere microbial species benefit the plant by acting as growth promoting rhizobacteria or symbionts in enhancing plant pathogen defense and nutrition, some microbes may be commensal or parasitic (reviewed in Lareen et al., 2016; Pascale et al., 2020; Chen et al., 2021). Therefore, dissecting these complex plant - soil rhizosphere - microbiome interactions is critical for designing new approaches for crop resilience to pathogenic and environmental stresses.
Fortunately, the emerging technologies are advancing our understanding of the plant-microbe responses to climate change, as researchers can now investigate host-microbe interactions at a much greater resolution and significance (Dubey et al., 2019b; Pang et al., 2021). In particular, integrated omics approaches, coupled with developments in HTP culturing, synthetic and computational biology, are offering greater insights into the structure and functions of diverse natural microbiomes, and opening a window for creating artificially engineered microbial assemblages aimed at improving crop growth, fitness and resilience to pathogens and numerous abiotic stresses (Trivedi et al., 2021). Combined multi-omics methods are quantifying and revealing the microbiomes features (via HTP amplicon sequencing and metagenomics), microbiomes functions (via metagenomics, metatranscriptomics and metaproteomics), and microbiomes connections with plants and the environment (via metabolomics) (reviewed in Clouse and Wagner, 2021; Trivedi et al., 2021). This has offered new mechanistic insights into how individual or collective microbes underpin plant-microbe interactions for plant health and resilience to climate change (Trivedi et al., 2021). Additionally, plant rhizosphere microbial richness analyses have effectively revealed genotypic and morphological trait variation in crops. For instance, Phaseolus vulgaris wild accessions exhibited high relative richness of Bacteroidetes, whilst their counterparts (elite or modern accessions) showed higher abundance of Actinobacteria and Proteobacteria, with the variation being attributed to the plant genotypic and specific root morphological traits (Pérez-Jaramillo et al., 2017; Pascale et al., 2020). Besides, phenomics integrated with bioinformatics, genomic and deep learning approaches are being applied for the diagnosis of crop diseases (reviewed in Adeniji and Babalola, 2020; Marsh et al., 2021).
Moving ahead, plant root-associated microbiomes can be targeted as a source of variation in crop breeding and engineering microbial inoculants to support plant growth and suppress diseases (reviewed in Pascale et al., 2020). Especially, advanced and HTP techniques, such as stable isotope probing, amplicon sequencing, whole-genome shotgun sequencing and metabolomics, coupled with sophisticated bioinformatics softwares and tools (including QIIME, MEGAN, MOTHUR, etc., reviewed in Dubey et al., 2019b; Pang et al., 2021), will become more routinely applied in unlocking the metabolite dialogs between plants and the microbes, and linking those metabolic footprints to key plant genes and phenotypic traits modulating microbiome recruitment or regulation (Chen et al., 2021; Clouse and Wagner, 2021; Trivedi et al., 2021). Taken together, integrating phenomics with other multi-omics approaches provides an invaluable strategy to develop new disease- and climate resilient cultivars via the identification, characterization, manipulation and recruitment of plant rhizospheric microbes into crop breeding and bioengineering programs aimed at improving host plant‘s pathogen resistance and overall fitness and functionality under environmentally challenged conditions.
Omics Technologies Integrated With Modern Plant Breeding Methods in a Systems Biology Approach for Crop Improvement
Momentous advances in the omics technologies, coupled with reduction in costs for genome sequencing and analysis, as well as developments in bioinformatics tools and databases, have enabled rapid accumulation of huge volumes of omics data that is being routinely used to identify novel alleles and molecular elements underlying key agronomic traits in different crop species. Moreover, these large omics datasets are becoming easily accessible (Chaudhary et al., 2019a). Despite this progress, however, more often, these datasets have been studied independently until recently, and the actual integration of several omics approaches remains tedious due to individualized experimental designs and analytical tools not fit for integrative omics models (Pinu et al., 2019; Pazhamala et al., 2021). Consequently, results from studies employing dis-integrated omics approaches could not provide much insight into the molecular mechanisms regulating key biological systems and complex traits.
Fortunately, integration of multi-omics techniques has emerged as a promising way to address these shortcomings through what is now commonly known as systems biology approach, which is an interdisciplinary research discipline that integrates multi-omics datasets, biological concepts, mathematical models, and machine learning tools to decipher complex biological networks or systems (Pinu et al., 2019). It is premised on multi-omics integration in order to develop a meaningful interpretation of how the genotype is linked to phenotype and subsequent plant responses to environmental stresses (Mohanta et al., 2017). Combining different omics approaches has proven expedient for identifying key candidate genes/proteins and metabolic pathways/networks for functional analysis and/or elucidation of complex molecular underpinnings to several important agronomic traits or plant abiotic and biotic stress responses. For instance, integrated transcriptomics, proteomics and metabolomics analyses of the mechanisms regulating low tiller production in low-tillering wheat identified 474, 166, and 28 tillering-associated differentially expressed genes, proteins, and 28 metabolites, respectively (Wang Z. et al., 2019). Comprehensive metabolic pathway enrichment analyses of these genes, proteins and metabolites pinpointed to three TF families (GRAS, GRF, and REV) and lignin biosynthesis pathway as responsible for the inhibition of tiller development in low-tillering wheat cultivars (Wang Z. et al., 2019). Besides, conjoint analysis (coupling comparative cytology with transcriptomic and metabolomic approaches) to understand the mechanisms underlying Solanum nigrum L. response to cadmium toxicity revealed key differentially expressed genes and metabolites, including laccase, peroxidase, D-fructose, and cellobiose, that were associated with cell wall biosynthesis, implying that the cell wall biosynthesis pathway plays a central role in Cd detoxification in Solanum nigrum (Wang et al., 2022). Combined transcriptomic and metabolomic approaches applied in maize to analyze gene regulatory networks modulating Rp1-D21 mutant-mediated hypersensitive pathogen defense response revealed that four uridinediphosphate-dependent glycosyltransferase (UGT) (ZmUGTs) genes were highly expressed, whilst the SA biosynthesis and phenylpropanoid biosynthesis pathways were induced at both the transcriptional and metabolic levels, suggesting that ZmUGT genes may be involved in maize defense response by regulating SA homeostasis (Ge et al., 2021). Earlier, the epigenetic-based amalgamation of multi-omics approaches elucidated the critical role DNA methylation and play in lipid biosynthesis regulation and spatio-temporal modulation of ROS during cotton fiber development (Wang et al., 2016). Thus, integrated omics approaches facilitate for in-depth understanding of complex physiological and molecular mechanisms underpinning several key traits of agronomic importance (Singh N. et al., 2020), as well as formulation of predictive models of those key traits using large molecular datasets (Scossa et al., 2021). This all-encompassing approach is crucial and a very promising strategy for creating climate-smart crop cultivars (Chaudhary et al., 2019a, b; Jha et al., 2020; Pazhamala et al., 2021; Raza et al., 2021b).
Meanwhile, these multi-omics generated data will need to be integrated with modern plant breeding and gene editing technologies in order to provide a comprehensive, time- and cost-effective strategy for targeting candidate genes regulating key agronomic and nutrition-related traits essential for developing climate-ready crops (Liu H.J. et al., 2020; Gogolev et al., 2021; Kumar R. et al., 2021). Such modern plant breeding technologies include double-haploid (DH) breeding (Yan et al., 2017), induced mutagenesis (Kharkwal and Shu, 2009), CRISPR-Cas based gene editing technologies (see Ahmar et al., 2020; Steinwand and Ronald, 2020; Fiaz et al., 2021; Gao, 2021; Kumar A. et al., 2021; Marsh et al., 2021; Sinha et al., 2021), and the single seed chipping (SSC) facilitated marker-based early generation selection (MEGS) technique (Parmar et al., 2021), among others. For instance, the SSC facilitated MEGS protocol could be used to successfully advance 3.5 breeding generations in groundnuts, and could significantly cut the time required to complete the entire breeding cycle by approximately 6-8 months. Additionally, the SSC technique did not significantly affect germination percentage (as it remained high, 95-99%) (Parmar et al., 2021). Therefore, this technique could be an indispensible tool to promote high-throughput genotyping and speed breeding of climate-smart groundnut (and possibly other legume) crop cultivars. Further, improved crop management practices that help maintain stabilized yields under resource constrained environments, including conservation agriculture and the use of melatonin to enhance crop stress tolerance will remain more relevant (Fahad et al., 2017; Zenda et al., 2020). This holistic approach to crop improvement for resilience to climate change and higher nutritive value is summarized in Figure 2.
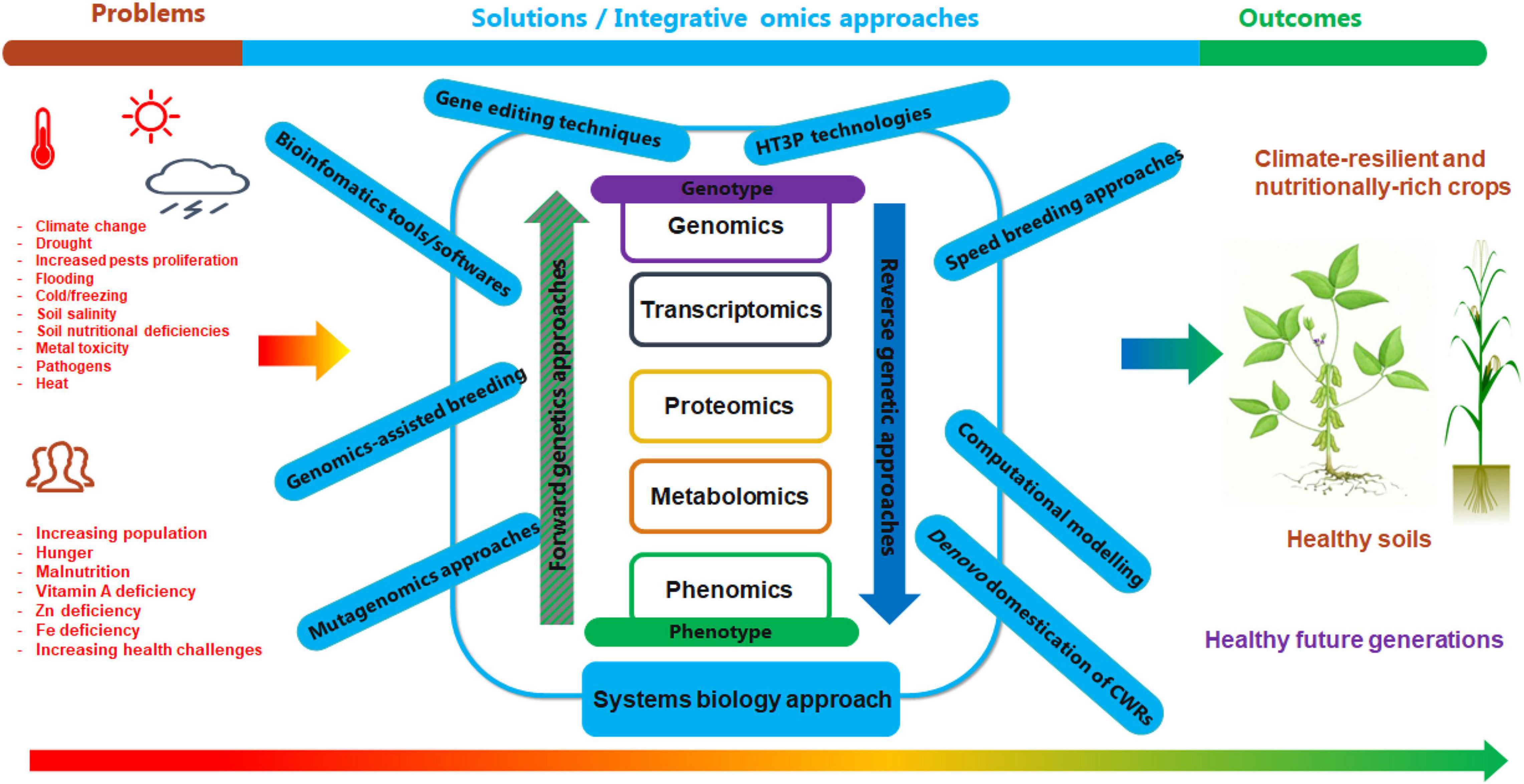
Figure 2. Abstract illustration of the role of integrated omics approaches in anchoring the development of climate-smart future crops. Integrated multi-omics strategies coupled with forward and reverse genetics methods, as well as advanced plant breeding, gene editing, mutagenomics and computational modeling techniques in a systems biology approach facilitate for the creation of climate-resilient and nutrition-rich crops. HT3P, high-throughput plant phenotyping platforms; CWRs, crop wild relatives.
Conclusion and Future Prospects
Here, we have cited several relevant examples to highlight how various omics approaches have anchored the crop improvement programs. Deployment of these omics techniques, particularly genomics, transcriptomics, proteomics, metabolomics and phenomics to study plant responses to numerous abiotic and biotic stresses has been vital in revealing several key genes, proteins and metabolic pathways underlying several quantitative and quality traits of agronomic importance in major crop species. Some of the identified candidate genes and metabolic pathways have been deployed in genomics-assisted or marker assisted breeding programs via molecular breeding approaches or genetic-engineering methodologies. Moreover, the recent advances in metabolomics and high-throughput phenotyping platforms have fortified the utility of genomics, transcriptomics and proteomics. Particularly, metabolomics is currently receiving special attention, owing to the role metabolites play as metabolic intermediates and close links to the phenotypic expression. Additionally, high throughput phenomics applications are driving the targeting of new research domains such as root system architecture analysis, and exploration of plant root-associated microbes for improved crop health and climate resilience. Further, single-cell transcriptomics and ionomics have emerged as the new “kids on the block” showing great promise for effective use in solving complex biological questions in the near future, although several technical and experimental design related challenges still need to be resolved. Fortunately other areas such as gene editing, bioinformatics analysis tools and softwares, and machine learning have also witnessed significant progress to support the advances in omics techniques. Leveraging on these developments, we envisage that combining multi-omics methods with modern plant breeding techniques, HTP experimental techniques, advanced bioinformatics, and computational modeling tools in a systems biology approach will facilitate for the development of sustainably higher yielding and nutritionally rich climate-resilient crops for the future.
Author Contributions
TZ and HD conceived the idea. TZ, SL, AD, JL, YW, XL, and NW performed the literature search. TZ prepared and wrote the original draft manuscript, and designed the figures. TZ, SL, AD, JL, YW, XL, NW, and HD reviewed and edited the manuscript. TZ and SL prepared the tables. HD involved in funding acquisition. All authors have read and agreed to the published version of the manuscript.
Funding
This research was funded by Identification, Evaluation, and Innovative Application of Maize Germplasm Resources Project, Grant No. 21326328D.
Conflict of Interest
The authors declare that the research was conducted in the absence of any commercial or financial relationships that could be construed as a potential conflict of interest.
Publisher’s Note
All claims expressed in this article are solely those of the authors and do not necessarily represent those of their affiliated organizations, or those of the publisher, the editors and the reviewers. Any product that may be evaluated in this article, or claim that may be made by its manufacturer, is not guaranteed or endorsed by the publisher.
Acknowledgments
We are grateful to several colleagues with whom we had personal exchanges via several interactive platforms and whose articles and insights we have incorporated in this paper and some which could not be included because of space limitations.
References
Adeniji, A. A., and Babalola, O. O. (2020). Metabolomic applications for understanding complex tripartite plant-microbes interactions: Strategies and perspectives. Biotechnol. Rep. 25:e00425. doi: 10.1016/j.btre.2020.e00425
Agrawal, G. K., Pedreschi, R., Barkla, B. J., Bindschedler, L. V., Cramer, R., Sarkar, A., et al. (2012). Translational plant proteomics: a perspective. J. Proteomics 75, 4588–4601. doi: 10.1016/j.jprot.2012.03.055
Agrawal, G. K., Sarkar, A., Righetti, P. G., Pedreschi, R., Carpentier, S., Wang, T., et al. (2013). A decade of plant proteomics and mass spectrometry: Translation of technical advancements to food security and safety issues. Mass Spectrom. Rev. 32, 335–365.
Ahmad, P., Abdel Latef, A. A., Rasool, S., Akram, N. A., Ashraf, M., and Gucel, S. (2016). Role of proteomics in crop stress tolerance. Front. Plant Sci. 7:1336. doi: 10.3389/fpls.2016.01336
Ahmar, S., Gill, R. A., Jung, K.-H., Faheem, A., Qasim, M. U., Mubeen, M., et al. (2020). Conventional and Molecular Techniques from Simple Breeding to Speed Breeding in Crop Plants: Recent Advances and Future Outlook. Int. J. Mol. Sci. 21:2590. doi: 10.3390/ijms21072590
Alexandratos, N., and Bruinsma, J. (2012). “World agriculture towards 2030/2050: the 2012 revision,” in ESA Working paper No. 12-03, (Rome: FAO).
Allwood, J. W., De Vos, R. C., Moing, A., Deborde, C., Erban, A., Kopka, J., et al. (2011). Plant metabolomics and its potential for systems biology research back- ground concepts, technology, and methodology. Methods Enzymol. 500, 299–336.
Alonge, M., Shumate, A., Puiu, D., Zimin, A. V., and Salzberg, S. L. (2020). Chromosome-Scale Assembly of the Bread Wheat Genome Reveals Thousands of Additional Gene Copies. Genetics 216, 599–608. doi: 10.1534/genetics.120.303501
Alqudah, A. M., Sallam, A., Baenziger, P. S., and Börner, A. (2020). GWAS: Fast-forwarding gene identification and characterization in temperate Cereals: Lessons from Barley–A review. J. Adv. Res. 22, 119–135.
Amoah, R. A., Akromah, R., Asibuo, J. Y., Wireko-Kena, A., Asare, K. B., Lamptey, M., et al. (2020). Mode of inheritance and combining ability of oleic acid content in groundnut (Arachis hypogaea L.). Ecol. Genet. Genom. 17:100064. doi: 10.1016/j.egg.2020.100064
Ananda, G. K., Myrans, H., Norton, S. L., Gleadow, R., Furtado, A., and Henry, R. J. (2020). Wild Sorghum as a Promising Resource for Crop Improvement. Front. Plant Sci. 11:1108. doi: 10.3389/fpls.2020.01108
Anderson, S. N., Stitzer, M. C., Brohammer, A. B., Zhou, P., Noshay, J. M., O’Connor, C. H., et al. (2019). Transposable Elements Contribute to Dynamic Genome Content in Maize. Plant J. 2019, 1052–1065. doi: 10.1111/tpj.14489
Anwar, K., Joshi, R., Dhankher, O. P., Singla-Pareek, S. L., and Pareek, A. (2021). Elucidating the Response of Crop Plants towards Individual, Combined and Sequentially Occurring Abiotic Stresses. Int. J. Mol. Sci. 22:6119. doi: 10.3390/ijms221161
Araus, J. L., Sanchez-Bragado, R., and Vicente, R. (2021). Improving crop yield and resilience through optimization of photosynthesis: panacea or pipe dream? J. Exp. Bot. 72, 3936–3955.
Arbona, V., Manzi, M., Ollas, C. D., and Gómez-Cadenas, A. (2013). Metabolomics as a Tool to Investigate Abiotic Stress Tolerance in Plants. Int. J. Mol. Sci. 14, 4885–4911. doi: 10.3390/ijms14034885
Arefian, M., Vessal, S., Malekzadeh-Shafaroudi, S., Siddique, K. H., and Bagheri, A. (2019). Comparative proteomics and gene expression analyses revealed responsive proteins and mechanisms for salt tolerance in chickpea genotypes. BMC Plant Biol. 2019:1–26. doi: 10.1186/s12870-019-1793-z
Ariel, F. D., and Manavella, P. A. (2021). When junk DNA turns functional: Transposon-derived noncoding RNAs in plants. J. Exp. Bot. 2021:erab073. doi: 10.1093/jxb/erab073
Arruda, M. P., Brown, P. J., Lipka, A. E., Krill, A. M., Thurber, C., and Kolb, F. L. (2015). Genomic selection for predicting Fusarium head blight resistance in a wheat breeding program. Plant Genome 8, lantgenome2015–lantgenome2011. doi: 10.3835/plantgenome2015.01.0003
Arsova, B., Watt, M., and Usadel, B. (2018). Monitoring of plant protein post-translational modifications using targeted proteomics. Front. Plant Sci. 9:1168. doi: 10.3389/fpls.2018.01168
Ashrafi-Dehkordi, E., Alemzadeh, A., Tanaka, N., and Razi, H. (2018). Meta-analysis of transcriptomic responses to biotic and abiotic stress in tomato. PeerJ. 6, e4631. doi: 10.7717/peerj.4631
Bahuguna, R. N., Gupta, P., Bagri, J., Singh, D., Dewi, A. K., Tao, L., et al. (2018). Forward and reverse genetics approaches for combined stress tolerance in rice. Ind. J. Plant Physiol. 23, 630–646. doi: 10.1007/s40502-018-0418-0
Banerjee, A., Wani, S. H., and Roychoudhury, A. (2017). Epigenetic Control of Plant Cold Responses. Front. Plant Sci. 8:1643. doi: 10.3389/fpls.2017.01643
Banerjee, R., Kumar, G. V., and Kumar, S. P. J. (eds) (2019). OMICS-based approaches in plant biotechnology. Hoboken, NJ: John Wiley & Sons.
Bao, Y., Kurle, J. E., Anderson, G., and Young, N. D. (2015). Association mapping and genomic prediction for resistance to sudden death syndrome in early maturing soybean germplasm. Mol. Breed. 35:128. doi: 10.1007/s11032-015-0324-3
Barupal, D. K., Fan, S., and Fiehn, O. (2018). Integrating bioinformatics approaches for a comprehensive interpretation of metabolomics datasets. Curr. Opin. Biotechnol. 54, 1–9. doi: 10.1016/j.copbio.2018.01.010
Batista-Silva, W., da Fonseca-Pereira, P., Martins, A. O., Zsögön, A., Nunes-Nesi, A., and Araújo, W. L. (2020). Engineering improved photosynthesis in the era of synthetic biology. Plant Commun. 1:100032. doi: 10.1016/j.xplc.2020.100032
Bayer, P. E., Golicz, A. A., Scheben, A., Batley, J., and Edwards, D. (2020). Plant pan-genomes are the new reference. Nat. Plants 6, 914–920. doi: 10.1038/s41477-020-0733-0
Bera, S. K., Kamdar, J. H., Kasundra, S. V., Dash, P., Maurya, A. K., and Jasani, M. D. (2018). XXX Improving oil quality by altering levels of fatty acids through marker-assisted selection of ahfad2 alleles in groundnut (Arachis hypogaea L.). Euphutica 214:162. doi: 10.1007/s10681-018-2241-0
Berhe, M., Dossa, K., You, J., Mboup, P. A., Diallo, I. N., Diouf, D., et al. (2021). Genome-wide association study and its applications in the non-model crop Sesamum indicum. BMC Plant Biol. 21:1–19. doi: 10.1186/s12870-021-03046-x
Bevan, M. W., and Uauy, C. (2013). Genomics reveals new landscapes for crop improvement. Genome biology 14, 1–11.
Bevan, M. W., Uauy, C., Wulff, B. B., Zhou, J., Krasileva, K., and Clark, M. D. (2017). Genomic innovation for crop improvement. Nature 543, 346–354. doi: 10.1038/nature22011
Bhat, J. A., Ali, S., Salgotra, R. K., Mir, Z. A., Dutta, S., Jadon, V., et al. (2016). Genomic selection in the era of next generation sequencing for complex traits in plant breeding. Front. Genet. 7:221. doi: 10.3389/fgene.2016.00221
Bidinger, F. R., Nepolean, T., Hash, C. T., Yadav, R. S., and Howarth, C. J. (2007). Quantitative trait loci for grain yield in pearl millet under variable post flowering moisture conditions. Crop Sci. 47, 969–980. doi: 10.2135/cropsci2006.07.0465
Bohra, A. (2013). Emerging paradigms in genomics-based crop improvement. Sci. World J. 585467, 1–17. doi: 10.1155/2013/585467
Bohra, A., Jha, U. C., Godwin, I. D., and Kumar Varshney, R. (2020). Genomic interventions for sustainable agriculture. Plant Biotechnol. J. 18, 2388–2405. doi: 10.1111/pbi.13472
Bohra, A., Jha, U. C., Kishor, P. K., Pandey, S., and Singh, N. P. (2014). Genomics and molecular breeding in lesser explored pulse crops: current trends and future opportunities. Biotechnol. Adv. 32, 1410–1428.
Brozynska, M., Furtado, A., and Henry, R. J. (2016). Genomics of crop wild relatives: expanding the gene pool for crop improvement. Plant Biotechnol. J. 14, 1070–1085. doi: 10.1111/pbi.12454
Calayugan, M. I. C., Formantes, A. K., Amparado, A., Descalsota-Empleo, G. I., Nha, C. T., et al. (2020). Genetic analysis of agronomic traits and grain iron and zinc concentrations in a doubled haploid population of rice (Oryza sativa L.). Sci. Rep. 10:2283.
Cao, Y., Li, S., He, X., Chang, F., Kong, J., Gai, J., et al. (2017). Mapping QTLs for plant height and flowering time in a Chinese summer planting soybean RIL population. Euphytica 213:39. doi: 10.1007/s10681-016-1834-8
Cerrudo, D., Cao, S., Yuan, Y., Martinez, C., Suarez, E. A., Babu, R., et al. (2018). Genomic Selection Outperforms Marker Assisted Selection for Grain Yield and Physiological Traits in a Maize Doubled Haploid Population Across Water Treatments. Front. Plant Sci. 9:366. doi: 10.3389/fpls.2018.00366
Chandramouli, K., and Qian, P. Y. (2009). Proteomics: challenges, techniques and possibilities to overcome biological sample complexity. HGP 2009:239204. doi: 10.4061/2009/239204
Chang, Y. N., Zhu, C., Jiang, J., Zhang, H., Zhu, J. K., and Duan, C. G. (2020). Epigenetic regulation in plant abiotic stress responses. J. Integr. Plant Biol. 62, 563–580. doi: 10.1111/jipb.12901
Chang, Y., Liu, H., Liu, M., Liao, X., Sahu, S. K., Fu, Y., et al. (2019). The draft genomes of five agriculturally important African orphan crops. GigaScience 8:giy152.
Chaturvedi, P., Wiese, A. J., Ghatak, A., Záveská Drábková, L., Weckwerth, W., and Honys, D. (2021). Heat stress response mechanisms in pollen development. New Phytologist. 231, 571–585. doi: 10.1111/nph.17380
Chaudhary, J., Khatri, P., Singla, P., Kumawat, S., Kumari, A., Vikram, A., et al. (2019a). Advances in Omics Approaches for Abiotic Stress Tolerance in Tomato. Biology 8:90. doi: 10.3390/biology8040090
Chaudhary, J., Shivaraj, S., Khatri, P., Ye, H., Zhou, L., Klepadlo, M., et al. (2019b). “Approaches, Applicability, and Challenges for Development of Climate-Smart Soybean,” in Genomic Designing of Climate-Smart Oilseed Crops, (Berlin: Springer Science and Business Media LLC), 1–74.
Chen, F., Dong, W., Zhang, J., Chen, J., Wang, Z., Lin, Z., et al. (2018). The Sequenced Angiosperm Genomes and Genome Databases. Front. Plant Sci. 9:418. doi: 10.3389/fpls.2018.00418
Chen, F., Fang, P., Peng, Y., Zeng, W., Zhao, X., Ding, Y., et al. (2019). Comparative Proteomics of Salt-Tolerant and Salt-Sensitive Maize Inbred Lines to Reveal the Molecular Mechanism of Salt Tolerance. Int. J. Mol. Sci. 20:4725. doi: 10.3390/ijms20194725
Chen, L., An, Y., Li, Y. X., Li, C., Shi, Y., Song, Y., et al. (2017). Candidate loci for yield-related traits in maize revealed by a combination of metaQTL analysis and regional association mapping. Front. Plant Sci. 8:2190. doi: 10.3389/fpls.2017.02190
Chen, L., Schwier, M., Krumbach, J., Kopriva, S., and Jacoby, R. P. (2021). Metabolomics in plant-microbe interactions in the roots. Adv. Bootanical Res. 98, 133–161. doi: 10.1016/bs.abr.2020.09.018
Chen, L., Wang, Q. Q., Zhou, L., Ren, F., Li, D. D., and Li, X. B. (2013). Arabidopsis CBL-interacting protein kinase (CIPK6) is involved in plant response to salt/osmotic stress and ABA. Mol. Biol. Rep. 40, 4759–4767.
Chen, X., Huang, Q., Zhang, F., Wang, B., Wang, J., and Zheng, J. (2014). ZmCIPK21, A Maize CBL-Interacting Kinase, Enhances Salt Stress Tolerance in Arabidopsis thaliana. Int. J. Mol. Sci. 15, 14819–14834. doi: 10.3390/ijms150814819
Chen, Z., Cui, Q., Liang, C., Sun, L., Tian, J., and Liao, H. (2011). Identification of differentially expressed proteins in soybean nodules under phosphorus deficiency through proteomic analysis. Proteomics 11, 4648–4659. doi: 10.1002/pmic.201100231
Cheng, F., Wu, J., Cai, C., Fu, L., Liang, J., Borm, T., et al. (2016). Genome resequencing and comparative variome analysis in a Brassica rapa and Brassica oleracea collection. Sci. Data 3:160119. doi: 10.1038/sdata.2016.119
Chinnusamy, V., Dalal, M., and Zhu, J. K. (2013). “Epigenetic regulation of abiotic stress responses in plants,” in Plant Abiotic Stress, 2nd Edn, eds M. A. Jenks and P. M. Hasegawa (Hoboken, NJ: John Wiley & Sons), 203–229. doi: 10.1002/9781118764374.ch8
Choudhary, M., Wani, S. H., Kumar, P., Bagaria, P. K., Rakshit, S., Roorkiwal, M., et al. (2019). QTLian breeding for climate resilience in cereals: progress and prospects. Funct. Integr. Genomics 19, 685–701. doi: 10.1007/s10142-019-00684-1
Chouhan, G. K., Verma, J. P., Jaiswal, D. K., Mukherjee, A., Singh, S., de Araujo Pereira, A. P., et al. (2021). Phytomicrobiome for promoting sustainable agriculture and food security: Opportunities, challenges, and solutions. Microbiol. Res. 248:126763. doi: 10.1016/j.micres.2021.126763
Chu, Y., Holbrook, C. C., and Ozias-Akins, P. (2009). Two alleles of control the high oleic acid trait in cultivated peanut. Crop Sci. 49, 2029–2036. doi: 10.2135/cropsci2009.01.0021
Clouse, K. M., and Wagner, M. R. (2021). Plant Genetics as a Tool for Manipulating Crop Microbiomes: Opportunities and Challenges. Front. Bioeng. Biotechnol. 9:567548. doi: 10.3389/fbioe.2021.567548
Cohen, S. P., and Leach, J. E. (2019). Abiotic and biotic stresses induce a core transcriptome response in rice. Sci. Rep. 9:6273. doi: 10.1038/s41598-019-42731-8
Coletta, R. D., Qiu, Y., Ou, S., Hufford, M. B., and Hirsch, C. N. (2021). How the pan-genome is changing crop genomics and improvement. Genome Biol. 22, 1–19. doi: 10.1186/s13059-020-02224-8
Contreras-Moreira, B., Cantalapiedra, C. P., García-Pereira, M. J., Gordon, S. P., Vogel, J. P., Igartua, E., et al. (2017). Analysis of plant pangenomes and transcriptomes with GET_HOMOLOGUES-EST, a clustering solution for sequences of the same species. Front. Plant Sci. 8:184. doi: 10.3389/fpls.2017.00184
Cooper, E. A., Brenton, Z. W., Flinn, B. S., Jenkins, J., Shu, S., Flowers, D., et al. (2019). A new reference genome for Sorghum bicolor reveals high levels of sequence similarity between sweet and grain genotypes: Implications for the genetics of sugar metabolism. BMC Genom. 20:1–13. doi: 10.1186/s12864-019-5734-x
Cortés, A. J., and López-Hernández, F. (2021). Harnessing Crop Wild Diversity for Climate Change Adaptation. Genes 12:783.
Crisp, P. A., Bhatnagar-Mathur, P., Hundleby, P., Godwin, I. D., Waterhouse, P. M., and Hickey, L. T. (2021). Beyond the gene: epigenetic and cis-regulatory targets offer new breeding potential for the future. Curr. Opin. Biotechnol. 73, 88–94. doi: 10.1016/j.copbio.2021.07.008
Cui, D., Wu, D., Somarathna, Y., Xu, C., Li, S., Li, P., et al. (2015). QTL mapping for salt tolerance based on snp markers at the seedling stage in maize (Zea mays L.). Euphytica 203, 273–283.
Cui, J., Lu, Z., Xu, G., Wang, Y., and Jin, B. (2020). Analysis and comprehensive comparison of PacBio and nanopore-based RNA sequencing of the Arabidopsis transcriptome. Plant Methods 16, 1–3. doi: 10.1186/s13007-020-00629-x
Dai, H., Cao, F., Chen, X., Zhang, M., Ahmed, I. M., Chen, Z. H., et al. (2013). Comparative proteomic analysis of aluminum tolerance in Tibetan wild and cultivated barleys. PLoS One 8:e63428. doi: 10.1371/journal.pone.0063428
Danilevicz, M. F., Fernandez, C. G. T., Marsh, J. I., Bayer, P. E., and Edwards, D. (2020). Plant pangenomics: approaches, applications and advancements. Curr. Opin. Plant Biol. 54, 18–25. doi: 10.1016/j.pbi.2019.12.005
Das, A., Eldakak, M., Paudel, B., Kim, D. W., Hemmati, H., Basu, C., et al. (2016). Leaf proteome analysis reveals prospective drought and heat stress response mechanisms in soybean. BioMed Res. Int. 6021047:23. doi: 10.1155/2016/6021047
Das, A., Rushton, P. J., and Rohila, J. S. (2017). Metabolomic Profiling of Soybeans (Glycine max L.) Reveals the Importance of Sugar and Nitrogen Metabolism under Drought and Heat Stress. Plants 6:21. doi: 10.3390/plants6020021
De Coninck, B., Timmermans, P., Vos, C., Cammue, B. P., and Kazan, K. (2015). What lies beneath: belowground defense strategies in plants. Trends Plant Sci. 20, 91–101. doi: 10.1016/j.tplants.2014.09.007
De Vega, D., Newton, A. C., and Sadanandom, A. (2018). Post-translational modifications in priming the plant immune system: ripe for exploitation? FEBS Lett. 592, 1929–1936. doi: 10.1002/1873-3468.13076
Debieu, M., Sine, B., Passot, S., Grondin, A., Akata, E., Gangashetty, P., et al. (2018). Response to early drought stress and identification of QTLs controlling biomass production under drought in pearl millet. PLoS One 13:e0201635. doi: 10.1371/journal.pone.0201635
Demidchik, V. V., Shashko, A. Y., Bandarenka, U. Y., Smolikova, G. N., Przhevalskaya, D. A., Charnysh, M. A., et al. (2020). Plant Phenomics: Fundamental Bases, Software and Hardware Platforms, and Machine Learning. Russ. J. Plant Physiol. 67, 397–412. doi: 10.1134/S1021443720030061
Denyer, T., and Timmermans, M. C. (2021). Crafting a blueprint for single-cell RNA sequencing. Trends Plant Sci. 2021:016. doi: 10.1016/j.tplants.2021.08.016
Denyer, T., Ma, X., Klesen, S., Scacchi, E., Nieselt, K., and Timmermans, M. C. (2019). Spatiotemporal developmental trajectories in the Arabidopsis root revealed using high-throughput single-cell RNA sequencing. Dev. Cell 48, 840–852. doi: 10.1016/j.devcel.2019.02.022
Derakhshani, B., Ayalew, H., Mishina, K., Tanaka, T., Kawahara, Y., Jafary, H., et al. (2020). Comparative Analysis of Root Transcriptome Reveals Candidate Genes and Expression Divergence of Homoeologous Genes in Response to Water Stress in Wheat. Plants 9:596. doi: 10.3390/plants9050596
Deshmukh, R., Sonah, H., Patil, G., Chen, W., Prince, S., Mutava, R., et al. (2014). Integrating omic approaches for abiotic stress tolerance in soybean. Front. Plant Sci. 2014:244. doi: 10.3389/fpls.2014.00244
Desmae, H., Janila, P., Okori, P., Pandey, M. K., Motagi, B. N., Monyo, E., et al. (2019). Genetics, genomics and breeding of groundnut (Arachis hypogaea L.). Plant Breed. 138, 425–444. doi: 10.1111/pbr.12645
Dhankher, O. P., and Foyer, C. H. (2018). Climate resilient crops for improving global food security and safety. Plant Cell Environ. 41, 877–884. doi: 10.1111/pce.13207
Ding, Y., Zhu, J., Zhao, D., Liu, Q., Yang, Q., and Zhang, T. (2021). Targeting cis-regulatory elements for rice grain quality improvement. Front. Plant Sci. 12:1597. doi: 10.3389/fpls.2021.705834
Djanaguiraman, M., Prasad, P. V. V., Kumari, J., and Rengel, Z. (2019). Root length and root lipid composition contribute to drought tolerance of winter and spring wheat. Plant Soil 439, 57–73. doi: 10.1007/s11104-018-3794-3
Dong, Z., Men, Y., Liu, Z., Li, J., and Ji, J. (2020). Application of chlorophyll fluorescence imaging technique in analysis and detection of chilling injury of tomato seedlings. Comput. Electron. Agricult. 168:105109.
Du, Q., Wang, K., Xu, C., Zou, C., Xie, C., Xu, Y., et al. (2016). Strand-specific RNA-Seq transcriptome analysis of genotypes with and without low-phosphorus tolerance provides novel insights into phosphorus-use efficiency in maize. BMC Plant Biol. 16:222. doi: 10.1186/s12870-016-0903-4
Dubey, A., Kumar, A., Abd Allah, E. F., Hashem, A., and Khan, M. L. (2019a). Growing more with less: breeding and developing drought resilient soybean to improve food security. Ecol. Indicat. 105, 425–437.
Dubey, A., Malla, M. A., Khan, F., Chowdhary, K., Yadav, S., Kumar, A., et al. (2019b). Soil microbiome: a key player for conservation of soil health under changing climate. Biodivers. Conserv. 28, 2405–2429. doi: 10.1007/s10531-019-01760-5
Dubin, M. J., Scheid, O. M., and Becker, C. (2018). Transposons: A blessing curse. Curr. Opin. Plant Biol. 2018, 23–29.
Dwivedi, S. L., Goldman, I., Ceccarelli, S., and Ortiz, R. (2020). Advanced analytics, phenomics and biotechnology approaches to enhance genetic gains in plant breeding. Adv. Agronomy 162, 89–142. doi: 10.1016/bs.agron.2020.02.002
Efroni, I., and Birnbaum, K. D. (2016). The potential of single-cell profiling in plants. Genome Biol. 17:65.
Escandón, M., Castillejo, M. Á, Jorrín-Novo, J. V., and Rey, M.-D. (2021). Molecular Research on Stress Responses in Quercus spp.: From Classical Biochemistry to Systems Biology through Omics Analysis. Forests 12:364. doi: 10.3390/f12030364
Evans, J. R., and Lawson, T. (2020). From green to gold: Agricultural revolution for food security. J. Exp. Bot. 71, 2211–2215.
Fahad, S., Bajwa, A. A., Nazir, U., Anjum, S. A., Farooq, A., Zohaib, A., et al. (2017). Crop production under drought and heat stress: Plant responses and management options. Front. Plant Sci. 8:1147. doi: 10.3389/fpls.2017.01147
FAO (2019). The State of Food Security and Nutrition in the World: Safeguarding against Economic Slowdowns and Downturns. Quebec City, QC: Food and Agriculture Organization.
Farmer, A., Thibivilliers, S., Ryu, K. H., Schiefelbein, J., and Libault, M. (2021). Single-nucleus RNA and ATAC sequencing reveals the impact of chromatin accessibility on gene expression in Arabidopsis roots at the single-cell level. Mol. Plant 14, 372–383. doi: 10.1016/j.molp.2021.01.001
Fiaz, S., Ahmar, S., Saeed, S., Riaz, A., Mora-Poblete, F., and Jung, K.-H. (2021). Evolution and Application of Genome Editing Techniques for Achieving Food and Nutritional Security. Int. J. Mol. Sci. 22:5585. doi: 10.3390/ijms22115585
Fiehn, O. (2002). Metabolomics-the link between genotypes and phenotypes. Plant Mol. Biol. 48, 155–171.
Flood, P. J., Harbinson, J., and Aarts, M. G. M. (2011). Natural genetic variation in plant photosynthesis. Trends Plant Sci. 16, 327–335.
Flood, P. J., Kruijer, W., Schnabel, S. K., van der Schoor, R., Jalink, H., Snel, J. F., et al. (2016). Phenomics for photosynthesis, growth and reflectance in Arabidopsis thaliana reveals circadian and long-term fluctuations in heritability. Plant Methods 12:14. doi: 10.1186/s13007-016-0113-y
Fraire-Velázquez, S., and Balderas-Hernández, V. E. (2013). Abiotic Stress in Plants and Metabolic Responses. Abiotic Stress—Plant Responses and Applications in Agriculture. Rijeka: InTech, 25–48.
Franklin, S., and Vondriska, T. M. (2011). Genomes, proteomes, and the central dogma. Circulat. Cardiovascul. Genet. 4, 576–576.
Frey, F. P., Pitz, M., Schön, C. C., and Hochholdinger, F. (2020). Transcriptomic diversity in seedling roots of European flint maize in response to cold. BMC Genomics 21:1–15. doi: 10.1186/s12864-020-6682-1
Frisvad, J. C., Hubka, V., Ezekiel, C. N., Hong, S. B., Nováková, A., Chen, A. J., et al. (2019). Taxonomy of Aspergillus section Flavi and their production of aflatoxins, ochratoxins and other mycotoxins. Stud. Mycol. 93, 1–63. doi: 10.1016/j.simyco.2018.06.001
Furbank, R. T., and Tester, M. (2011). Phenomics – technologies to relieve the phenotyping bottleneck. Trends Plant Sci. 16, 635–644.
Furbank, R. T., Jimenez –Berni, J. A., George –Jaeggli, B., Potgieter, A. B., and Deery, D. M. (2019). Field crop phenomics: enabling breeding for radiation use efficiency and biomass in cereal crops. New Phytol. 223, 1714–1727.
Furbank, R. T., Sharwood, R., Estavillo, G. M., Silva-Perez, V., and Condon, A. G. (2020). Photons to food: genetic improvement of cereal crop photosynthesis. J. Exp. Bot. 71, 2226–2238. doi: 10.1093/jxb/eraa077
Gangurde, S. S., Wang, H., Yaduru, S., Pandey, M. K., Fountain, J. C., Chu, Y., et al. (2020). Nested-association mapping (NAM)-based genetic dissection uncovers candidate genes for seed and pod weights in peanut (Arachis hypogaea). Plant Biotechnol. J. 18, 1457–1471.
Gao, C. (2021). Genome engineering for crop improvement and future agriculture. Cell 184, 1621–1635.
Gao, L., Gonda, I., Sun, H., Ma, Q., Bao, K., Tieman, D. M., et al. (2019). The tomato pan-genome uncovers new genes and a rare allele regulating fruit flavor. Nat. Genet. 51, 1044–1051.
Garg, M., Sarma, N., Sharma, S., Kapoor, P., Kumar, A., Chunduri, V., et al. (2018). Biofortified crops generated by breeding, agronomy, and transgenic approaches are improving lives of millions of people around the world. Front. Nutr. 5:12. doi: 10.3389/fnut.2018.00012
Gasparini, K., dos Reis, Moreira, J., Peres, L. E. P., and Zsögön, A. (2021). De novo domestication of wild species to create crops with increased resilience and nutritional value. Curr. Opin. Plant Biol. 60:102006.
Ge, C., Wang, Y.-G., Lu, S., Zhao, X. Y., Hou, B.-K., Balint-Kurti, P. J., et al. (2021). Multi-Omics Analyses Reveal the Regulatory Network and the Function of ZmUGTs in Maize Defense Response. Front. Plant Sci. 12:738261. doi: 10.3389/fpls.2021.738261
Ghatak, A., Chaturvedi, P., and Weckwerth, W. (2017). Cereal crop proteomics: Systemic analysis of crop drought stress responses towards marker-assisted selection breeding. Front. Plant Sci. 8:757. doi: 10.3389/fpls.2017.00757
Giacomello, S. (2021). A new era for plant science: spatial single-cell transcriptomics. Curr. Opin. Plant Biol. 60:102041. doi: 10.1016/j.pbi.2021.102041
Gil, J. D., Cohn, A. S., Duncan, J., Newton, P., and Vermeulen, S. (2017). The resilience of integrated agricultural systems to climate change. Wiley Interdiscipl. Rev. Clim. Change 8:e461.
Goche, T., Shargie, N. G., Cummins, I., Brown, A. P., Chivasa, S., and Ngara, R. (2020). Comparative physiological and root proteome analyses of two sorghum varieties responding to water limitation. Sci. Rep. 10:11835. doi: 10.1038/s41598-020-68735-3
Goddard, R., Steed, A., Chinoy, C., Ferreira, J. R., Scheeren, P. L., Maciel, J. L. N., et al. (2020). Dissecting the genetic basis of wheat blast resistance in the Brazilian wheat cultivar BR 18-Terena. BMC Plant Biol. 20:398. doi: 10.1186/s12870-020-02592-0
Gogolev, Y. V., Ahmar, S., Akpinar, B. A., Budak, H., Kiryushkin, A. S., Gorshkov, V. Y., et al. (2021). OMICs, Epigenetics, and Genome Editing Techniques for Food and Nutritional Security. Plants 10:1423. doi: 10.3390/plants10071423
Golicz, A. A., Batley, J., and Edwards, D. (2016a). Towards plant pangenomics. Plant Biotechnol. J. 14, 1099–1105. doi: 10.1111/pbi.12499
Golicz, A. A., Bayer, P. E., Barker, G. C., Edger, P. P., Kim, H., Martinez, P. A., et al. (2016b). The pangenome of an agronomically important crop plant Brassica oleracea. Nat. Commun. 7, 1–8. doi: 10.1038/ncomms13390
González, F. G., and Manavella, P. A. (2021). Prospects for plant productivity: from the canopy to the nucleus. J. Exp. Bot. 72, 3931–3935. doi: 10.1093/jxb/erab147
Gramazio, P., Yan, H., Hasing, T., Vilanova, S., Prohens, J., and Bombarely, A. (2019). Whole-Genome Resequencing of Seven Eggplant (Solanum melongena) and One Wild Relative (S. incanum) Accessions Provides New Insights and Breeding Tools for Eggplant Enhancement. Front. Plant Sci. 10:1220. doi: 10.3389/fpls.2019.01220
Gundaraniya, S. A., Ambalam, P. S., and Tomar, R. S. (2020). Metabolomic Profiling of Drought-Tolerant and Susceptible Peanut (Arachis hypogaea L.) Genotypes in Response to Drought Stress. ACS Omega 5, 31209–31219.
Guo, H., Ayalew, H., Seethepalli, A., Dhakal, K., Griffiths, M., Ma, X. F., et al. (2020). Functional phenomics and genetics of the root economics space in winter wheat using high-throughput phenotyping of respiration and architecture. New Phytol. 2020:15. doi: 10.1111/nph.17329
Guo, J., Chen, L., Li, Y., Shi, Y., Song, Y., Zhang, D., et al. (2018). Meta-QTL analysis and identification of candidate genes related to root traits in maize. Euphytica 214, 1–15. doi: 10.1007/s10681-018-2283-3
Guo, J., Shi, G., Guo, X., Zhang, L., Xu, W., Wang, Y., et al. (2015). Transcriptome analysis reveals that distinct metabolic pathways operate in salt-tolerant and salt-sensitive upland cotton varieties subjected to salinity stress. Plant Sci. 238, 33–45.
Gupta, S. M., Arora, S., Mirza, N., Pande, A., Lata, C., Puranik, S., et al. (2017). Finger Millet: A “Certain” Crop for an “Uncertain” Future and a Solution to Food Insecurity and Hidden Hunger under Stressful Environments. Front. Plant Sci. 8:643. doi: 10.3389/fpls.2017.00643
Haak, D. C., Fukao, T., Grene, R., Hua, Z., Ivanov, R., Perrella, G., et al. (2017). Multilevel regulation of abiotic stress responses in plants. Front. Plant Sci. 8:1564. doi: 10.3389/fpls.2017.01564
Hamany Djande, C. Y., Pretorius, C., Tugizimana, F., Piater, L. A., and Dubery, I. A. (2020). Metabolomics: A Tool for Cultivar Phenotyping and Investigation of Grain Crops. Agronomy 10:831. doi: 10.3390/agronomy10060831
Han, M., Lu, X., Yu, J., Chen, X., Wang, X., Malik, W. A., et al. (2019). Transcriptome Analysis Reveals Cotton (Gossypium hirsutum) Genes That Are Differentially Expressed in Cadmium Stress Tolerance. Int. J. Mol. Sci. 20:1479. doi: 10.3390/ijms20061479
Han, S., Yuan, M., Clevenger, J. P., Li, C., Hagan, A., Zhang, X., et al. (2018). A SNP-based linkage map revealed QTLs for resistance to early and late leaf spot diseases in peanut (Arachis hypogaea L.). Front. Plant Sci. 9:1012. doi: 10.3389/fpls.2018.01012
Hashiguchi, A., Ahsan, N., and Komatsu, S. (2010). Proteomics application of crops in the context of climatic changes. Food Res. Int. 43, 1803–1813.
Hashiguchi, A., and Komatsu, S. (2017). Posttranslational modifications and plant–Environment interaction. Methods Enzymol. 586, 97–113. doi: 10.1016/bs.mie.2016.09.030
Hasin, Y., Seldin, M., and Lusis, A. (2017). Multi-omics approaches to disease. Genome Biol. 18, 1–15.
Heffner, E. L., Sorrells, M. E., and Jannink, J. (2009). Genomic selection for crop improvement. Crop Sci. 49, 1–12. doi: 10.2135/cropsci2008.08.0512
Herzog, M., Fukao, T., Winkel, A., Konnerup, D., Lamichhane, S., Alpuerto, J. B., et al. (2018). Physiology, gene expression, and metabolome of two wheat cultivars with contrasting submergence tolerance. Plant Cell Environ. 41, 1632–1644. doi: 10.1111/pce.13211
Hirsch, C. N., Foerster, J. M., Johnson, J. M., Sekhon, R. S., Muttoni, G., Vaillancourt, B., et al. (2014). Insights into the maize pan-genome and pan-transcriptome. Plant Cell 2014, 121–135. doi: 10.1105/tpc.113.119982
Ho, S. S., Urban, A. E., and Mills, R. E. (2020). Structural variation in the sequencing era. Nat. Rev. Genet. 21, 171–189.
Hodge, A. (2004). The plastic plant: root responses to heterogeneous supplies of nutrients. New Phytol. 162, 9–24. doi: 10.1111/j.1469-8137.2004.01015.x
Hrdlickova, R., Toloue, M., and Tian, B. (2017). RNA-Seq methods for transcriptome analysis. Wiley Interdiscip. Rev. RNA 8:1364.
Hu, H., Scheben, A., and Edwards, D. (2018). Advances in integrating genomics and bioinformatics in the plant breeding pipeline. Agriculture 8:75. doi: 10.3390/agriculture8060075
Hu, X., Wu, L., Zhao, F., Zhang, D., Li, N., Zhu, G., et al. (2015). Phosphoproteomic analysis of the response of maize leaves to drought, heat and their combination stress. Front. Plant Sci. 6:298. doi: 10.3389/flps.2015.00298
Huang, B. E., Verbyla, K. L., Verbyla, A. P., Raghavan, C., Singh, V. K., et al. (2015). MAGIC populations in crops: current status and future prospects. Theor. Appl. Genet. 128, 999–1017.
Huang, J., Ma, Q., Cai, Z., Xia, Q., Li, S., Jia, J., et al. (2020). Identification and Mapping of Stable QTLs for Seed Oil and Protein Content in Soybean [Glycine max (L.) Merr.]. J. Agric. Food Chem. 68, 6448–6460. doi: 10.1021/acs.jafc.0c01271
Huang, L., Li, Q., Zhang, C., Chu, R., Gu, Z., Tan, H., et al. (2020). Creating novel Wx alleles with fine-tuned amylose levels and improved grain quality in rice by promoter editing using CRISPR/Cas9 system. Plant Biotechnol. J. 18:2164. doi: 10.1111/pbi.13391
Huang, M., Balimponya, E. G., Mgonja, E. M., McHale, L. K., Luzi-Kihupi, A., Wang, G. L., et al. (2019). Use of genomic selection in breeding rice (Oryza sativa L.) for resistance to rice blast (Magnaporthe oryzae). Mol. Breed. 39:114. doi: 10.1007/s11032-019-1023-2
Huang, X., and Han, B. (2014). Natural variations and genome-wide association studies in crop plants. Annu. Rev. Plant Biol. 65, 531–551.
Huang, Y., Haas, M., Heinen, S., Steffenson, B. J., Smith, K. P., and Muehlbauer, G. J. (2018). QTL Mapping of Fusarium Head Blight and Correlated Agromorphological Traits in an Elite Barley Cultivar Rasmusson. Front. Plant Sci. 9:1260. doi: 10.3389/fpls.2018.01260
Hübner, S., Bercovich, N., Todesco, M., Mandel, J. R., Odenheimer, J., Ziegler, E., et al. (2019). Sunflower pan-genome analysis shows that hybridization altered gene content and disease resistance. Nat. Plants 5, 54–62.
Hussain, S., Zhu, C., Bai, Z., Huang, J., Zhu, L., Cao, X., et al. (2019). iTRAQ-Based Protein Profiling and Biochemical Analysis of Two Contrasting Rice Genotypes Revealed Their Differential Responses to Salt Stress. Int. J. Mol. Sci. 20:547. doi: 10.3390/ijms20030547
Ibrahim, A. K., Zhang, L., Niyitanga, S., Afzal, M. Z., Xu, Y., Zhang, L., et al. (2020). Principles and approaches of association mapping in plant breeding. Tropical Plant Biol. 13, 212–224. doi: 10.1007/s12042-020-09261-4
Jankowicz-Cieslak, J., and Till, B. J. (2015). Forward and reverse genetics in crop breeding. Adv. Plant Breed. Strateg. Breed. Biotechnol. Mol. Tools 2015, 215–240.
Jayakodi, M., Padmarasu, M., Haberer, G., Bonthala, V. S., Gundlach, H., Monat, C., et al. (2020). 2020 The barley pan-genome reveals the hidden legacy of mutation breeding. Nature 588, 285–292. doi: 10.1038/s41586-020-2947-8
Jayakodi, M., Schreiber, M., Stein, N., and Mascher, M. (2021). Building pan-genome infrastructures for crop plants and their use in association genetics. DNA Res. 28:dsaa030. doi: 10.1093/dnares/dsaa030
Jean-Baptiste, K., McFaline-Figueroa, J. L., Alexandre, C. M., Dorrity, M. W., Saunders, L., Bubb, K. L., et al. (2019). Dynamics of gene expression in single root cells of A. thaliana. Plant Cell 31, 993–1011. doi: 10.1105/tpc.18.00785
Jendoubi, T. (2021). Approaches to Integrating Metabolomics and Multi-Omics Data: A Primer. Metabolites 11:184. doi: 10.3390/metabo11030184
Jha, U. C., Bohra, A., and Nayyar, H. (2020). Advances in “omics” approaches to tackle drought stress in grain legumes. Plant Breed. 139, 1–27. doi: 10.1111/pbr.12761
Kakoulidou, I., Avramidou, E. V., Baránek, M., Brunel-Muguet, S., Farrona, S., Johannes, F., et al. (2021). Epigenetics for Crop Improvement in Times of Global Change. Biology 10:766. doi: 10.3390/biology10080766
Kamenya, S. N., Mikwa, E. O., Song, B., and Odeny, D. A. (2021). Genetics and breeding for climate change in Orphan crops. Theoret. Appl. Genet. 2021, 1–29. doi: 10.1007/s00122-020-03755-1
Kang, W., Zhu, X., Wang, Y., Chen, L., and Duan, Y. (2018). Transcriptomic and metabolomic analyses reveal that bacteria promote plant defense during infection of soybean cyst nematode in soybean. BMC Plant Biol. 18:86. doi: 10.1186/s12870-018-1302-9
Karim, M. R., Wang, R., Zheng, L., Dong, X., Shen, R., and Lan, P. (2020). Physiological and Proteomic Dissection of the Responses of Two Contrasting Wheat Genotypes to Nitrogen Deficiency. Int. J. Mol. Sci. 21:2119. doi: 10.3390/ijms21062119
Katam, R., Shokri, S., Murthy, N., Singh, S. K., Suravajhala, P., Khan, M. N., et al. (2020). Proteomics, physiological, and biochemical analysis of cross tolerance mechamnisms in response to heat and water stresses in soybean. PLoS One 15:e0233905. doi: 10.1371/journal.pone.0233905
Kaufmann, K., Smaczniak, C., de Vries, S., Angenent, G. C., and Karlova, R. (2011). Proteomics insights into plant signaling and development. Proteomics 11, 744–755. doi: 10.1002/pmic.201000418
Kaur, B., Sandhu, K. S., Kamal, R., Kaur, K., Singh, J., Röder, M. S., et al. (2021). Omics in Major Cereals: Applications, Challenges, and Prospects. [Preprint]. doi: 10.20944/preprints202104.0531.v1
Kavuluko, J., Kibe, M., Sugut, I., Kibet, W., Masanga, J., Mutinda, S., et al. (2021). GWAS provides biological insights into mechanisms of the parasitic plant (Striga) resistance in sorghum. BMC Plant Biol. 21:392. doi: 10.1186/s12870-021-03155-7
Kersey, P. J. (2019). Plant genome sequences: Past, present, future. Curr. Opin. Plant Biol. 48, 1–8. doi: 10.1016/j.pbi.2018.11.001
Khalid, N., Aqeel, M., and Noman, A. (2019). “System Biology of Metal Tolerance in Plants: An Integrated View of Genomics, Transcriptomics, Metabolomics, and Phenomics,” in Plant Metallomics and Functional Omics, ed. G. Sablok (Cham: Springer), doi: 10.1007/978-3-030-19103-0_6
Khan, A. W., Garg, V., Roorkiwal, M., Golicz, A. A., Edwards, D., and Varshney, R. K. (2020). Super-pangenome by integrating the wild side of a species for accelerated crop improvement. Trends Plant Sci. 25, 148–158. doi: 10.1016/j.tplants.2019.10.012
Khan, N., Bano, A., Rahman, M. A., Rathinasabapathi, B., and Babar, M. A. U. P. L. C. (2019). -HRMS-based untargeted metabolic profiling reveals changes in chickpea (Cicer arietinum) metabolome following long-term drought stress. Plant Cell Environ. 42, 115–132. doi: 10.1111/pce.13195
Kharkwal, M. C., and Shu, Q. Y. (2009). The role of induced mutations in world food security. Induced plant mutations in the genomics era. Food Agric. Organ. 2009, 33–38.
Khizar, M., Shi, J., Saleem, S., Liaquat, F., Ashraf, M., Latif, S., et al. (2020). Resistance associated metabolite profiling of Aspergillus leaf spot in cotton through non-targeted metabolomics. PLoS One 15:e0228675. doi: 10.1371/journal.pone.0228675
Kilian, B., Dempewolf, H., Guarino, L., Werner, P., Coyne, C., and Warburton, M. L. (2020). Crop Science special issue: Adapting agriculture to climate change: A walk on the wild side. Crop Sci. 61, 32–36. doi: 10.1002/csc2.20418
Kim, J. H., Hilleary, R., Seroka, A., and He, S. Y. (2021). Crops of the future: building a climate-resilient plant immune system. Curr. Opin. Plant Biol. 60:101997.
Kim, J. M., Kim, K. H., Jung, J., Kang, B. K., Lee, J., Ha, B. K., et al. (2020). Validation of marker-assisted selection in soybean breeding program for pod shattering resistance. Euphytica 216:166. doi: 10.1007/s10681-020-02703-w
Kim, J. M., Sasaki, T., Ueda, M., Sako, K., and Seki, M. (2015). Chromatin changes in response to drought, salinity, heat, and cold stresses in plants. Front. Sci. 6:114. doi: 10.3389/fpls.2015.00114
Kim, J. M., To, T. K., Nishioka, T., and Seki, M. (2010). Chromatin regulation functions in plant abiotic stress responses. Plant Cell Environ. 33, 604–611.
Kim, S. B., Van den Broeck, L., Karre, S., Choi, H., Christensen, S. A., Wang, G.-F., et al. (2021). Analysis of the transcriptomic, metabolomic, and gene regulatory responses to Puccinia sorghi in maize. Mol. Plant Pathol. 22, 465–479. doi: 10.1111/mpp.13040
Kircher, M., and Kelso, J. (2010). High-throughput DNA sequencing–concepts and limitations. Bioessays 32, 524–536. doi: 10.1002/bies.200900181
Klein, A., Houtin, H., Rond-Coissieux, C., Naudet-Huart, M., Touratier, M., Marget, P., et al. (2020). Meta-analysis of QTL reveals the genetic control of yield-related traits and seed protein content in pea. Sci. Rep. 10, 1–11. doi: 10.1038/s41598-020-72548-9
Klich, M. A. (2007). Aspergillus flavus: the major producer of aflatoxin. Mol. Plant Pathol. 8, 713–722. doi: 10.1111/j.1364-3703.2007.00436.x
Kole, C., Muthamilarasan, M., Henry, R., Edwards, D., Sharma, R., Abberton, M., et al. (2015). Application of genomics-assisted breeding for generation of climate resilient crops: progress and prospects. Front. Plant Sci. 6:563. doi: 10.3389/fpls.2015.00563
Konstantinov, D. K., Zubairova, U. S., Ermakov, A. A., and Doroshkov, A. V. (2021). Comparative transcriptome profiling of aresistant vs susceptible bread wheat (Triticum aestivum L.) cultivar in response to water deficit and cold stress. PeerJ 9:e11428. doi: 10.7717/peerj.11428
Kosova, K., Vitamvas, P., Urban, M. O., Prasil, I. T., and Renaut, J. (2018). Plant abiotic stress proteomics: The major factors determining alterations in cellular proteome. Front. Plant Sci. 9:122. doi: 10.3389/fpls.2018.00122
Kowalska, A., Walkiewicz, K., Kozieł, P., and Muc-Wierzgoñ, M. (2017). Aflatoxins: characteristics and impact on human health. Postepy. Hig. Med. Dosw. 71, 315–327. doi: 10.5604/01.3001.0010.3816
Krishnan, P., Kruger, N. J., and Ratcliffe, R. G. (2005). Metabolite fingerprinting and profiling in plants using NMR. J. Exp. Bot. 56, 255–265. doi: 10.1093/jxb/eri010
Krishnappa, G., Singh, A. M., Chaudhary, S., Ahlawat, A. K., Singh, S. K., Shukla, R. B., et al. (2017). Molecular mapping of the grain iron and zinc concentration, protein content and thousand kernel weight in wheat (Triticum aestivum L.). PLoS One 12:e0174972. doi: 10.1371/journal.pone.0174972
Kukurba, K. R., and Montgomery, S. B. (2015). RNA sequencing and analysis. Cold Spring Harb. Protoc. 2015, 951–969.
Kulwal, P. L. (2018). “Trait Mapping Approaches Through Linkage Mapping in Plants,” in Plant Genetics and Molecular Biology. Advances in Biochemical Engineering/Biotechnology, eds R. Varshney, M. Pandey, and A. Chitikineni (Cham: Springer), 164. doi: 10.1007/10_2017_49
Kumar, A., Anju, T., Kumar, S., Chhapekar, S. S., Sreedharan, S., Singh, S., et al. (2021). Integrating Omics and Gene Editing Tools for Rapid Improvement of Traditional Food Plants for Diversified and Sustainable Food Security. Int. J. Mol. Sci. 22:8093. doi: 10.3390/ijms22158093
Kumar, J., Choudhary, A. K., Gupta, D. S., and Kumar, S. (2019). Towards Exploitation of Adaptive Traits for Climate-Resilient Smart Pulses. Int. J. Mol. Sci. 20:2971. doi: 10.3390/ijms20122971
Kumar, J., Gupta, D. S., Baum, M., Varshney, R. K., and Kumar, S. (2021). Genomics-assisted lentil breeding: Current status and future strategies. Legume Sci. 2021:e71. doi: 10.1002/leg3.71
Kumar, N., Soren, K. R., Bharadwaj, C., Pr, S. P., Shrivastava, A. K., Pal, M., et al. (2021). Genome-wide transcriptome analysis and physiological variation modulates gene regulatory networks acclimating salinity tolerance in chickpea. Environ. Exp. Bot. 187:104478. doi: 10.1016/j.envexpbot.2021.104478
Kumar, R., Bohra, A., Pandey, A. K., Pandey, M. K., and Kumar, A. (2017). Metabolomics for plant improvement: status and prospects. Front. Plant Sci. 8:1302. doi: 10.3389/fpls.2017.01302
Kumar, R., Sharma, V., Suresh, S., Ramrao, D. P., Veershetty, A., Kumar, S., et al. (2021). Understanding Omics Driven Plant Improvement and de novo Crop Domestication: Some Examples. Front. Genet. 12:415. doi: 10.3389/fgene.2021.637141
Kumar, S., Palve, A., Joshi, C., and Srivastava, R. K. (2019). Crop biofortification for iron (Fe), zinc (Zn) and vitamin A with transgenic approaches. Heliyon 5:e01914. doi: 10.1016/j.heliyon.2019.e01914
Kumari, P., Rastogi, A., and Yadav, S. (2020). Effects of Heat stress and molecular mitigation approaches in orphan legume, Chickpea. Mol. Biol. Rep. 47, 4659–4670.
Labuschagne, M. T. (2018). A review of cereal grain proteomics and its potential for sorghum improvement. J. Cereal Sci. 84, 151–158. doi: 10.1016/j.jcs.2018.10.010
Lai, J., Li, R., Xu, X., Jin, W., Xu, M., Zhao, H., et al. (2010). Genome-wide patterns of genetic variation among elite maize inbred lines. Nat. Genet. 42, 1027–1030. doi: 10.1038/ng.684
Lamaoui, M., Jemo, M., Datla, R., and Bekkaoui, F. (2018). Heat and Drought Stresses in Crops and Approaches for Their Mitigation. Front. Chem. 6:26. doi: 10.3389/fchem.2018.00026
Lambarey, H., Moola, N., Veenstra, A., Murray, S., and Suhail Rafudeen, M. (2020). Transcriptomic Analysis of a Susceptible African Maize Line to Fusarium verticillioides Infection. Plants 9:1112. doi: 10.3390/plants9091112
Langridge, P., and Fleury, D. (2011). Making the most of ‘omics’ for crop breeding. Trends Biotechnol. 29, 33–40.
Lareen, A., Burton, F., and Schäfer, P. (2016). Plant root-microbe communication in shaping root microbiomes. Plant Mol. Biol. 90, 575–587.
Lee, S., Jun, T. H., Michel, A. P., et al. (2015). SNP markers linked to QTL conditioning plant height, lodging, and maturity in soybean. Euphytica 203, 521–532. doi: 10.1007/s10681-014-1252-8
Lei, L., Goltsman, E., Goodstein, D., Wu, G. A., Rokhsar, D. S., and Vogel, J. P. (2021). Plant Pan-Genomics Comes of Age. Annu. Rev. Plant Biol. 72, 411–435.
Li, C., Lin, F., An, D., Wang, W., and Huang, R. (2018). Genome Sequencing and Assembly by Long Reads in Plants. Genes 9:6. doi: 10.3390/genes9010006
Li, D., Quan, C., Song, Z., Li, X., Yu, G., Li, C., et al. (2021). High-Throughput Plant Phenotyping Platform (HT3P) as a Novel Tool for Estimating Agronomic Traits From the Lab to the Field. Front. Bioeng. Biotechnol. 8:1533. doi: 10.3389/fbioe.2020.623705
Li, Q., and Yan, J. (2020). Sustainable agriculture in the era of omics: knowledge-driven crop breeding. Genome Biol. 21, 1–5. doi: 10.1186/s13059-020-02073-5
Li, T., Wang, Y. H., Liu, J. X., Feng, K., Xu, Z. S., and Xiong, A. S. (2019). Advances in genomic, transcriptomic, proteomic, and metabolomic approaches to study biotic stress in fruit crops. Crit. Rev. Biotechnol. 39, 680–692. doi: 10.1080/07388551.2019.1608153
Li, W., Sun, Y., Wang, B., Xie, H., Wang, J., and Nan, Z. (2020). Transcriptome analysis of two soybean cultivars identifies an aluminum respon-sive antioxidant enzyme GmCAT1. Biosci. Biotechnol. Biochem. 84, 1394–1400. doi: 10.1080/09168451.2020.1740970
Li, Y. H., Zhou, G., Ma, J., Jiang, W., Jin, L. G., Zhang, Z., et al. (2014). De novo assembly of soybean wild relatives for pan-genome analysis of diversity and agronomic traits. Nat. Biotechnol. 32, 1045–1052.
Liang, Y., Baring, M., Wang, S., and Septiningsih, E. M. (2017). Mapping QTLs for leafspot resistance in peanut using SNP-based next-generation sequencing markers. Plant Breed. Biotechnol. 5, 115–122. doi: 10.9787/PBB.2017.5.2.115
Liang, Y., Liu, H. J., Yan, J., and Tian, F. (2021a). Natural variation in crops: realized understanding, continuing promise. Annu. Rev. Plant Biol. 72:090632. doi: 10.1146/annurev-arplant-080720-090632
Liang, Y., Tabien, R. E., Tarpley, L., Mohammed, A. R., and Septiningsih, E. M. (2021b). Transcriptome profiling of two rice genotypes under mild field drought stress during grain-filling stage. AoB Plants 13:lab043. doi: 10.1093/aobpla/plab043
Lima, E. N., Silva, M. D. S., de Abreu, C. E. B., Mesquita, R. O., Lobo, M. D. P., Monteiro-Moreira, A. D. O., et al. (2019). Differential proteomics in contrasting cowpea genotypes submitted to different water regimes. Genet. Mol. Res. 18:GMR18396. doi: 10.4238/gmr18396
Liu, H. J., Jian, L., Xu, J., Zhang, Q., Zhang, M., Jin, M., et al. (2020). High-throughput CRISPR/Cas9 mutagenesis streamlines trait gene identification in maize. Plant Cell 32, 1397–1413. doi: 10.1105/tpc.19.00934
Liu, S., and Qin, F. (2021). Genetic dissection of maize drought tolerance for trait improvement. Mol. Breed. 41, 1–3.
Liu, X., Yin, C., Xiang, L., Jiang, W., Xu, S., and Mao, Z. (2020). Transcription strategies related to photosynthesis and nitrogen metabolism of wheat in response to nitrogen deficiency. BMC Plant Biol. 20:448. doi: 10.1186/s12870-020-02662-3
Liu, Y., Du, H., Li, P., Shen, Y., Peng, H., Liu, S., et al. (2020). Pan-genome of wild and cultivated soybeans. Cell 182, 162–176. doi: 10.1016/j.cell.2020.05.023
Liu, Z., El-Basyoni, I., Kariyawasam, G., Zhang, G., Fritz, A., Hansen, J., et al. (2015). Evaluation and association mapping of resistance to tan spot and Stagonospora nodorum blotch in adapted winter wheat germplasm. Plant Dis. 99, 1333–1341. doi: 10.1094/PDIS-11-14-1131-RE
Liu, Z., Zhou, Y., Guo, J., Li, J., Tian, Z., Zhu, Z., et al. (2020). Global dynamic molecular profiling of stomatal lineage cell development by single-cell RNA sequencing. Mol. Plant 13, 1178–1193. doi: 10.1016/j.molp.2020.06.010
López-Gresa, M. P., Maltese, F., Bellés, J. M., Conejero, V., Kim, H. K., Choi, Y. H., et al. (2010). Metabolic response of tomato leaves upon different plant–pathogen interactions. Phytochem. Anal. Int. J. Plant Chem. Biochem. Techniq. 21, 89–94. doi: 10.1002/pca.1179
Lu, Y., Li, R., Wang, R., Wang, X., Zheng, W., Sun, Q., et al. (2017). Comparative proteomic analysis of flag leaves reveals new insight into wheat heat adaptation. Front. Plant Sci. 8:1086. doi: 10.3389/fpls.2017.01086
Luan, H., Shen, H., Pan, Y., Guo, B., Lv, C., and Xu, R. (2018). Elucidating the hypoxic stress response in barley (Hordeum vulgare L.) during waterlogging: A proteomics approach. Sci. Rep. 8:9655.
Luo, M., Zhao, Y., Wang, Y., Shi, Z., Zhang, P., Zhang, Y., et al. (2018). Comparative proteomics of contrasting maize genotypes provides insights into salt-stress tolerance mechanisms. J. Proteome Res. 17, 141–153. doi: 10.1021/acs.jproteome.7b00455
Luo, Q., Teng, W., Fang, S., Li, H., Li, B., Chu, J., et al. (2019). Transcriptome analysis of salt-stress response in three seedling tissues of common wheat. Crop J. 7, 378–392.
Ma, H., Song, L., Shu, Y., Wang, S., Niu, J., Wang, Z., et al. (2012). Comparative proteomic analysis of seedling leaves of different salt tolerant soybean genotypes. J. Proteom. 75, 1529–1546. doi: 10.1016/j.jprot.2011.11.026
Ma, Z., Wang, L., Zhao, M., Gu, S., Wang, C., Zhao, J., et al. (2020). iTRAQ proteomics reveals the regulatory response to Magnaporthe oryzae in durable resistant vs. susceptible rice genotypes. PLoS One 15:e0227470. doi: 10.1371/journal.pone.0227470
Macdiarmid, J. I., and Whybrow, S. (2019). Nutrition from a climate change perspective. Proc. Nutrit. Soc. 78, 380–387. doi: 10.1017/S0029665118002896
Majeed, S., Rana, I. A., Atif, R. M., Zulfiqar, A. L. I., Hinze, L., and Azhar, M. T. (2019). Role of SNPs in determining QTLs for major traits in cotton. J. Cotton Res. 2, 1–13.
Makalowski, W., Gotea, V., Pande, A., and Makalowski, I. (2019). Transposable elements: Classification, identification, and their use as a tool for comparative genomics. Evol. Genom. Methods Mol. Biol. 1910, 177–207. doi: 10.1007/978-1-4939-9074-0_6
Makarevitch, I., Waters, A. J., West, P. T., Stitzer, M., Hirsch, C. N., Ross-Ibarra, J., et al. (2015). Transposable elements contribute to activation of maize genes in response to abiotic stress. PLoS Genet. 11:e1004915. doi: 10.1371/journal.pgen.1004915
Mallikarjuna, M. G., Thirunavukkarasu, N., Sharma, R., Shiriga, K., Hossain, F., Bhat, J. S., et al. (2020). Comparative Transcriptome Analysis of Iron and Zinc Deficiency in Maize (Zea mays L.). Plants 9:1812. doi: 10.3390/plants9121812
Mammadov, J., Aggarwal, R., Buyyarapu, R., and Kumpatla, S. (2012). SNP markers and their impact on plant breeding. Int. J. Plant Genom. 12:728398. doi: 10.1155/2012/728398
Marsh, J. I., Hu, H., Gill, M., Batley, J., and Edwards, D. (2021). Crop breeding for a changing climate: integrating phenomics and genomics with bioinformatics. Theor. Appl. Genet. 134, 1677–1690. doi: 10.1007/s00122-021-03820-3
Martí, M. C., Jiménez, A., and Sevilla, F. (2020). Thioredoxin network in plant mitochondria: cysteine S-posttranslational modifications and stress conditions. Front. Plant Sci. 11:1476. doi: 10.3389/fpls.2020.571288
Matros, A., Kaspar, S., Witzel, K., and Mock, H. P. (2011). Recent progress in liquid chromatography-based separation and label-free quantitative plant proteomics. Phytochemistry 72, 963–974.
Mba, C., Guimaraes, E. P., and Ghosh, K. (2012). Re-orienting crop improvement for the changing climatic conditions of the 21st century. Agric. Food Secur. 1, 1–17. doi: 10.1186/2048-7010-1-7
McCormick, R. F., Truong, S. K., Sreedasyam, A., Jenkins, J., Shu, S., Sims, D., et al. (2018). The Sorghum bicolor reference genome: Improved assembly, gene annotations, a transcriptome atlas, and signatures of genome organization. Plant J. 93, 338–354.
McCoy, R. M., Julian, R., Kumar, S. R. V., Ranjan, R., Varala, K., and Li, Y. (2021). A Systems Biology Approach to Identify Essential Epigenetic Regulators for Specific Biological Processes in Plants. Plants 10:364.
Meister, R., Rajani, M. S., Ruzicka, D., and Schachtman, D. P. (2014). Challenges of modifying root traits in crops for agriculture. Trends Plant Sci. 19, 779–788. doi: 10.1016/j.tplants.2014.08.005
Mérida-García, R., Liu, G., He, S., Gonzalez-Dugo, V., Dorado, G., Gálvez, S., et al. (2019). Genetic dissection of agronomic and quality traits based on association mapping and genomic selection approaches in durum wheat grown in Southern Spain. PLoS One 14:e0211718. doi: 10.1371/journal.pone.0211718
Michael, T. P., and Jackson, S. (2013). The first 50 plant genomes. Plant Genome 2013, 547–562. doi: 10.3835/plantgenome2013.03.0001in
Michael, T. P., and VanBuren, R. (2020). Building near-complete plant genomes. Curr. Opin. Plant Biol. 54, 26–33.
Missanga, J. S., Venkataramana, P. B., and Ndakidemi, P. A. (2021). Recent developments in Lablab purpureus genomics. A focus on drought stress tolerance and use of genomic resources to develop stress-resilient varieties. Legume Sci. 2021:e99. doi: 10.1002/leg3.99
Mohanta, T. K., Bashir, T., Hashem, A., and Abd_Allah, E. F. (2017). Systems biology approach in plant abiotic stresses. Plant Physiol. Biochem. 121, 58–73. doi: 10.1016/j.plaphy.2017.10.019
Montenegro, J. D. (2017). The pangenome of hexaploid bread wheat. Plant J. 90, 1007–1013. doi: 10.1111/tpj.13515
Morrell, P., Buckler, E., and Ross-Ibarra, J. (2012). Crop genomics: advances and applications. Nat. Rev. Genet. 13, 85–96. doi: 10.1038/nrg3097
Mousavi-Derazmahalleh, M., Bayer, P. E., Hane, J. K., Valliyodan, B., Nguyen, H. T., Nelson, M. N., et al. (2019). Adapting legume crops to climate change using genomic approaches. Plant Cell Environ. 42, 6–19. doi: 10.1111/pce.13203
Mu, Q., Zhang, W., Zhang, Y., Yan, H., Liu, K., Matsui, T., et al. (2017). iTRAQ-Based Quantitative Proteomics Analysis on Rice Anther Responding to High Temperature. Int. J. Mol. Sci. 18:1811. doi: 10.3390/ijms18091811
Muhammad, I., Shalmani, A., Ali, M., Yang, Q. H., Ahmad, H., and Li, F. B. (2021). Mechanisms regulating the dynamics of photosynthesis under abiotic stresses. Front. Plant Sci. 11:2310. doi: 10.3389/fpls.2020.615942
Mustafa, G., and Komatsu, S. (2021). Plant proteomic research for improvement of food crops under stresses: a review. Mol. Omics. 2021, 1–21. doi: 10.1039/d1mo00151e
Muthamilarasan, M., Singh, N. K., and Prasad, M. (2019). Multi-omics approaches for strategic improvement of stress tolerance in underutilized crop species: a climate change perspective. Adv. Genet. 103, 1–38.
Myers, S. S., Zanobetti, A., Kloog, I., Huybers, P., Leakey, A. D., Bloom, A. J., et al. (2014). Increasing CO2 threatens human nutrition. Nature 510, 139–142. doi: 10.1038/nature13179
Nachimuthu, V. V., Muthurajan, R., Duraialaguraja, S., et al. (2015). Analysis of Population Structure and Genetic Diversity in Rice Germplasm Using SSR Markers: An Initiative Towards Association Mapping of Agronomic Traits in Oryza Sativa. Rice 8:30. doi: 10.1186/s12284-015-0062-5
Nakano, Y., and Kobayashi, Y. (2020). Genome-wide Association Studies of Agronomic Traits Consisting of Field- and Molecular-based Phenotypes. Rev. Agricult. Sci. 8, 28–45.
Nelms, B., and Walbot, V. (2019). Defining the developmental program leading to meiosis in maize. Science 364, 52–56. doi: 10.1126/science.aav6428
Nepolean, T., Kaul, J., Mukri, G., and Mittal, S. (2018). Genomics-Enabled Next-Generation Breeding Approaches for Developing System-Specific Drought Tolerant Hybrids in Maize. Front. Plant Sci. 9:361. doi: 10.3389/fpls.2018.00361
Nhamo, L., Matchaya, G., Mabhaudhi, T., Nhlengethwa, S., Nhemachena, C., and Mpandeli, S. (2019). Cereal Production Trends under Climate Change: Impacts and Adaptation Strategies in Southern Africa. Agriculture 9:30. doi: 10.3390/agriculture9020030
Noble, T. J., Tao, Y., Mace, E. S., Williams, B., Jordan, D. R., Douglas, C. A., et al. (2018). Characterization of linkage disequilibrium and population structure in a mungbean diversity panel. Front. Plant Sci. 8:2102. doi: 10.3389/fpls.2017.02102
Ogbaga, C. C., Stepien, P., Dyson, B. C., Rattray, N. J., Ellis, D. I., Goodacre, R., et al. (2016). Biochemical analyses of sorghum varieties reveal differential responses to drought. PLoS One 11:e0154423. doi: 10.1371/journal.pone.0154423
Ojiewo, C. O., Janila, P., Bhatnagar-Mathur, P., Pandey, M. K., Desmae, H., Okori, P., et al. (2020). Advances in crop improvement and delivery research for nutritional quality and health benefits of groundnut (Arachis hypogaea L.). Front. Plant Sci. 11:29. doi: 10.3389/fpls.2020.00029
Paez-Garcia, A., Motes, C. M., Scheible, W.-R., Chen, R., Blancaflor, E. B., and Monteros, M. J. (2015). Root Traits and Phenotyping Strategies for Plant Improvement. Plants 4, 334–355. doi: 10.3390/plants4020334
Pan, Y., Liang, H., Gao, L., Dai, G., Chen, W., Yang, X., et al. (2020). Transcriptomic profiling of germinating seeds under cold stress and characterization of the cold-tolerant gene LTG5 in rice. BMC Plant Biol. 20:1–17. doi: 10.1186/s12870-020-02569-z
Pandey, M. K., Wang, M. L., Qiao, L., Feng, S., Khera, P., Wang, H., et al. (2014). Identification of QTLs associated with peanut oil contents in RIL populations and mapping FAD2 genes and their relative contribution towards oil quality. BMC Genetics 15:133. doi: 10.1186/s12863-014-0133-4
Pandey, P., Irulappan, V., Bagavathiannan, M. V., and Senthil-Kumar, M. (2017). Impact of combined abiotic and biotic stresses on plant growth and avenues for crop improvement by exploiting physio-morphological traits. Front. Plant Sci. 8:537. doi: 10.3389/flps.2017.00537
Pandey, P., Ramegowda, V., and Senthil-Kumar, M. (2015). Shared and unique responses of plants to multiple individual stresses and stress combinations: physiological and molecular mechanisms. Front. Plant Sci. 6:723. doi: 10.3389/fpls.2015.00723
Pang, Y., Liu, C., Wang, D., Amand, P. S., Bernardo, A., Li, W., et al. (2020). High-resolution genome-wide association study identifies genomic regions and candidate genes for important agronomic traits in wheat. Mol. Plant 13, 1311–1327.
Pang, Z., Chen, J., Wang, T., Gao, C., Li, Z., Guo, L., et al. (2021). Linking Plant Secondary Metabolites and Plant Microbiomes: A Review. Front. Plant Sci 12:621276. doi: 10.3389/fpls.2021.621276
Park, S. G., Park, H. S., Baek, M. K., Jeong, J. M., Cho, Y. C., Lee, G. M., et al. (2019). Improving the glossiness of cooked rice, an important component of visual rice grain quality. Rice 12:87.
Parmar, S., Deshmukh, D. B., Kumar, R., Manohar, S. S., Joshi, P., Sharma, V., et al. (2021). Single Seed-Based High-Throughput Genotyping and Rapid Generation Advancement for Accelerated Groundnut Genetics and Breeding Research. Agronomy 11:1226. doi: 10.3390/agronomy11061226
Pascale, A., Proietti, S., Pantelides, I. S., and Stringlis, I. A. (2020). Modulation of the root microbiome by plant molecules: the basis for targeted disease suppression and plant growth promotion. Front. Plant Sci. 2020:1741. doi: 10.3389/fpls.2019.01741
Pathak, R. K., Baunthiyal, M., Pandey, D., et al. (2018). Augmentation of crop productivity through interventions of omics technologies in India: challenges and opportunities. 3 Biotech 8:454. doi: 10.1007/s13205-018-1473-y
Paul, M. J., and Foyer, C. H. (2001). Sink regulation of photosynthesis. J. Exp. Bot. 52, 1383–1400.
Pazhamala, L. T., Kudapa, H., Weckwerth, W., Millar, A. H., and Varshney, R. K. (2021). Systems biology for crop improvement. Plant Genome 2021:e20098. doi: 10.1002/tpg2.20098
Pérez-Jaramillo, J. E., Carrion, V. J., Bosse, M., Ferrao, L. F. V., De Hollander, M., Garcia, A. A. F., et al. (2017). Linking rhizosphere microbiome composition of wild and domesticated Phaseolus vulgaris to genotypic and root phenotypic traits. ISME J. 11, 2244–2257. doi: 10.1038/ismej.2017.85
Peschansky, V. J., and Wahlestedt, C. (2014). Non-coding RNAs as direct and indirect modulators of epigenetic regulation. Epigenetics 9, 3–12. doi: 10.4161/epi.27473
Pinu, F. R., Beale, D. J., Paten, A. M., Kouremenos, K., Swarup, S., Schirra, H. J., et al. (2019). Systems Biology and Multi-Omics Integration: Viewpoints from the Metabolomics Research Community. Metabolites 9:76. doi: 10.3390/metabo9040076
Pourkheirandish, M., Golicz, A. A., Bhalla, P. L., and Singh, M. B. (2020). Global role of crop genomics in the face of climate change. Front. Plant Sci. 11:922. doi: 10.3389/fpls.2020.00922
Pratik, K. (2018). Transcriptomics of Single Cell: New Discoveries to Become Basis for Future Studies. Transcriptomics 6:143. doi: 10.4172/2329-8936.100014
Pretini, N., Alonso, M. P., Vanzetti, L., Pontaroli, A. C., and González, F. G. (2021). The physiology and genetics behind fruiting efficiency: a promising spike trait to improve wheat yield potential. J. Exp. Bot. 72, 3987–4004.
Pundir, P., Devi, A., Krishnamurthy, S. L., Sharma, P. C., and Vinaykumar, N. M. (2021). QTLs in salt rice variety CSR10 reveals salinity tolerance at reproductive stage. Acta Physiol. Plant. 43:35. doi: 10.1007/s11738-020-03183-0
Puranik, S., Sahu, P. P., Beynon, S., Srivastava, R. K., Sehgal, D., Ojulong, H., et al. (2020). Genome-wide association mapping and comparative genomics identifies genomic regions governing grain nutritional traits in finger millet (Eleusine coracana L. Gaertn.). Plants People Planet 2, 649–662. doi: 10.1002/ppp3.10120
Purugganan, M. D., and Jackson, S. A. (2021). Advancing crop genomics from lab to field. Nat. Genet. 53, 595–601. doi: 10.1038/s41588-021-00866-3
Qaim, M. (2020). Role of new plant breeding technologies for food security and sustainable agricultural development. Appl. Econom. Perspect. Policy 42, 129–150. doi: 10.1002/aepp.13044
Qamar-uz, Z., Zubair, A., Muhammad, Y., Muhammad, Z. I., Abdul, K., Fahad, S., et al. (2017). Zinc biofortification in rice: leveraging agriculture to moderate hidden hunger in developing countries. Arch. Agron. Soil Sci. 64, 147–161. doi: 10.1080/03650340.2017.1338343
Qian, Y., Ren, Q., Zhang, J., and Chen, L. (2019). Transcriptomic analysis of the maize (Zea mays L.) inbred line B73 response to heat stress at the seedling stage. Gene 692, 68–78. doi: 10.1016/j.gene.2018.12.062
Qin, P., Lu, H., Du, H., Wang, H., Chen, W., Chen, Z., et al. (2021). Pan-genome analysis of 33 genetically diverse rice accessions reveals hidden genomic variations. Cell 184, 3542.e–3558.e.
Qu, L., Li, D., Lv, J., Chen, W., Zhang, Z., Li, X., et al. (2018). Pan-genome of cultivated pepper (Capsicum) and its use in gene presence–absence variation analyses. New Phytol. 220, 360–363. doi: 10.1111/nph.15413
Qutub, M., Chandran, S., Rathinavel, K., Sampathrajan, V., Rajasekaran, R., Manickam, S., et al. (2021). Improvement of a Yairipok Chujak Maize Landrace from North Eastern Himalayan Region for β-Carotene Content through Molecular Marker-Assisted Backcross Breeding. Genes 12:762. doi: 10.3390/genes12050762
Rahaman, M. M., Zwart, R. S., Rupasinghe, T. W., Hayden, H. L., and Thompson, J. P. (2021). Metabolomic profiling of wheat genotypes resistant and susceptible to root-lesion nematode Pratylenchus thornei. Plant Mol. Biol. 106, 381–406. doi: 10.1007/s11103-021-01156-6
Rahman, M., Davies, P., Bansal, U., Pasam, R., Hayden, M., and Trethowan, R. (2020). Marker-assisted recurrent selection improves the crown rot resistance of bread wheat. Mol. Breeding 40:28. doi: 10.1007/s11032-020-1105-1
Ramalingam, A., Kudapa, H., Pazhamala, L. T., Weckwerth, W., and Varshney, R. K. (2015). Proteomics and metabolomics: two emerging areas for legume improvement. Front. Plant Sci. 6:1116. doi: 10.3389/fpls.2015.01116
Ranganathan, J., Waite, R., Searchinger, T., and Hanson, C. (2018). How to sustainably feed 10 billion people by 2050, in 21 charts. Washington, D.C: World Resource Institute.
Ray, D. K., West, P. C., Clark, M., Gerber, J. S., Prishchepov, A. V., and Chatterjee, S. (2019). Climate change has likely already affected global food production. PLoS One 14:e0217148. doi: 10.1371/journal.pone.0217148
Raza, A., Razzaq, A., Mehmood, S. S., Hussain, M. A., Wei, S., He, H., et al. (2021a). Omics: The way forward to enhance abiotic stress tolerance in Brassica napus L. GM Crops Food 12, 251–281. doi: 10.1080/21645698.2020.1859898
Raza, A., Razzaq, A., Mehmood, S. S., Zou, X., Zhang, X., Lv, Y., et al. (2019). Impact of climate change on crops adaptation and strategies to tackle its outcome: a review. Plants 8:34. doi: 10.3390/plants8020034
Raza, A., Tabassum, J., Kudapa, H., and Varshney, R. K. (2021b). Can omics deliver temperature resilient ready-to-grow crops? Crit. Rev. Biotechnol. 2021, 1–24. doi: 10.1080/07388551.2021.1898332
Raza, Q., Riaz, A., Sabar, M., Atif, R. M., and Bashir, K. (2019). Meta-analysis of grain iron and zinc associated QTLs identified hotspot chromosomal regions and positional candidate genes for breeding biofortified rice. Plant Sci. 288:110214.
Razzaq, A., Sadia, B., Raza, A., Khalid Hameed, M., and Saleem, F. (2019). Metabolomics: A Way Forward for Crop Improvement. Metabolites 9:303. doi: 10.3390/metabo9120303
Resham, S., Akhter, F., Ashraf, M., and Kazi, A. G. (2014). Metabolomics role in crop improvement. Emerg. Technol. Manage. Crop Stress Toler. 1, 39–55. doi: 10.1016/B978-0-12-800876-8.00002-3
Reynolds, M., Atkin, O. K., Bennett, M., Cooper, M., Dodd, I. C., Foulkes, M. J., et al. (2021). Addressing research bottlenecks to crop productivity. Trends Plant Sci. 26, 607–630.
Ribeiro, P. F., Badu-Apraku, B., Gracen, V. E., Danquah, E. Y., Garcia-Oliveira, A. L., Asante, M. D., et al. (2018). Identification of quantitative trait loci for grain yield and other traits in tropical maize under high and low soil-nitrogen environments. Crop Sci. 58, 321–331. doi: 10.2135/cropsci2017.02.0117
Rich-Griffin, C., Stechemesser, A., Finch, J., Lucas, E., Ott, S., and Schäfer, P. (2020). Single-Cell Transcriptomics: A High-Resolution Avenue for Plant Functional Genomics. Trends Plant Sci. 25, 186–197. doi: 10.1016/j.tplants.2019.10.008
Roohanitaziani, R., de Maagd, R. A., Lammers, M., Molthoff, J., Meijer-Dekens, F., van Kaauwen, M. P. W., et al. (2020). Exploration of a Resequenced Tomato Core Collection for Phenotypic and Genotypic Variation in Plant Growth and Fruit Quality Traits. Genes 11:1278. doi: 10.3390/genes11111278
Roorkiwal, M., Pandey, S., Thavarajah, D., Hemalatha, R., and Varshney, R. K. (2021). Molecular mechanisms and biochemical pathways for micronutrient acquisition and storage in legumes to support biofortification for nutritional security. Fronts. Plant Sci. 12:682842. doi: 10.3389/fpls.2021.682842
Ross, P. L., Huang, Y. N., Marchese, J. N., Williamson, B., Parker, K., Hattan, S., et al. (2004). Multiplexed protein quantitation in Saccharomyces cerevisiae using amine-reactive isobaric tagging reagents. Mol. Cell. Proteom. 3, 1154–1169. doi: 10.1074/mcp.M400129-MCP200
Roy, S. K., Cho, S. W., Kwon, S. J., Kamal, A. H., Kim, S. W., Oh, M. W., et al. (2016). Morpho-Physiological and Proteome Level Responses to Cadmium Stress in Sorghum. PLoS One 11:e0150431. doi: 10.1371/journal.pone.0150431
Ruan, Y., Yu, B., Knox, R. E., Singh, A. K., DePauw, R., Cuthbert, R., et al. (2020). High density mapping of quantitative trait loci conferring gluten strength in Canadian durum wheat. Front. Plant Sci. 11:170. doi: 10.3389/fpls.2020.00170
Ruperao, P., Thirunavukkarasu, N., Gandham, P., Selvanayagam, S., Govindaraj, M., Nebie, B., et al. (2021). Sorghum Pan-Genome Explores the Functional Utility for Genomic-Assisted Breeding to Accelerate the Genetic Gain. Front. Plant Sci. 12:666342. doi: 10.3389/fpls.2021.666342
Sab, S., Lokesha, R., Mannur, D. M., Somasekhar, Jadhav, K., Mallikarjuna, B. P., et al. (2020). Genome-Wide SNP Discovery and Mapping QTLs for Seed Iron and Zinc Concentrations in Chickpea (Cicer arietinum L.). Front. Nutr. 7:559120. doi: 10.3389/fnut.2020.559120
Saba Rahim, M., Sharma, H., Parveen, A., and Roy, J. K. (2018). “Trait Mapping Approaches Through Association Analysis in Plants,” in Plant Genetics and Molecular Biology. Advances in Biochemical Engineering/Biotechnology, Vol. 164, eds R. Varshney, M. Pandey, and A. Chitikineni (Cham: Springer), 83–108. doi: 10.1007/10_2017_50
Safdar, L. B., Andleeb, T., Latif, S., Umer, M. J., Tang, M., Li, X., et al. (2020). Genome-wide association study and QTL meta-analysis identified novel genomic loci controlling potassium use efficiency and agronomic traits in bread wheat. Front. Plant Sci. 11:70. doi: 10.3389/fpls.2020.00070
Said, J. I., Lin, Z., Zhang, X., Song, M., and Zhang, J. (2013). A comprehensive meta QTL analysis for fiber quality, yield, yield related and morphological traits, drought tolerance, and disease resistance in tetraploid cotton. BMC Genom. 14:1–22. doi: 10.1186/1471-2164-14-776
Saito, K., and Matsuda, F. (2010). Metabolomics for functional genomics, systems biology, and biotechnology. Annu. Rev. Plant Biol. 61, 463–489.
Samantara, K., Shiv, A., de Sousa, L. L., Sandhu, K. S., Priyadarshini, P., and Mohapatra, S. R. (2021). A Comprehensive Review on Epigenetic Mechanisms and Application of Epigenetic Modifications for Crop Improvement. Environ. Exp. Bot. 188:104479. doi: 10.1016/j.envexpbot.2021.104479
Sandhu, K. S., You, F. M., Conner, R. L., Balasubramanian, P. M., and Hou, A. (2018). Genetic analysis and QTL mapping of the seed hardness trait in a black common bean (Phaseolus vulgaris) recombinant inbred line (RIL) population. Mol. Breeding 38, 1–13. doi: 10.1007/s11032-018-0789-y
Santos, J. R., Ndeve, A. D., Huynh, B. L., Matthews, W. C., and Roberts, P. A. (2018). QTL mapping and transcriptome analysis of cowpea reveals candidate genes for root-knot nematode resistance. PLoS One 13:e0189185. doi: 10.1371/journal.pone.0189185
Sarvamangala, C., Gowda, M. V. C., and Varshney, R. K. (2011). Identification of quantitative trait loci for protein content, oil content and oil quality for groundnut (Arachis hypogaea L.). Field Crops Res. 122, 49–59. doi: 10.1016/j.fcr.2011.02.010
Saxena, R. K., Edwards, D., and Varshney, R. K. (2014). Structural variations in plant genomes. Briefings Funct. Genom. 13, 296–307.
Schatz, M. C., Maron, L. G., Stein, J. C., Wences, A. H., Gurtowski, J., Biggers, E., et al. (2014). Whole genome de novo assemblies of three divergent strains of rice, Oryza sativa, document novel gene space of aus and indica. Genome Biol. 2014, 1–16. doi: 10.1186/s13059-014-0506-z
Scheben, A., Yuan, Y., and Edwards, D. (2016). Advances in genomics for adapting crops to climate change. Curr. Plant Biol. 6, 2–10. doi: 10.1016/j.cpb.2016.09.001
Scheelbeek, P. F., Bird, F. A., Tuomisto, H. L., Green, R., Harris, F. B., Joy, E. J., et al. (2018). Effect of environmental changes on vegetable and legume yields and nutritional quality. Proc. Natl. Acad. Sci. 115, 6804–6809. doi: 10.1073/pnas.1800442115
Schreiber, M., Stein, N., and Mascher, M. (2018). Genomic approaches for studying crop evolution. Genome Biol. 19, 1–15.
Scossa, F., Alseekh, S., and Fernie, A. R. (2021). Integrating multi-omics data for crop improvement. J. Plant Physiol. 257:153352.
Seetharam, K., Kuchanur, P. H., Koirala, K. B., Tripathi, M. P., Patil, A., Sudarsanam, V., et al. (2021). Genomic regions associated with heat stress tolerance in tropical maize (Zea mays L.) (2021). Sci. Rep. 11:13730.
Selamat, N., and Nadarajah, K. K. (2021). Meta-Analysis of Quantitative Traits Loci (QTL) Identified in Drought Response in Rice (Oryza sativa L.). Plants 10:716. doi: 10.3390/plants10040716
Seyfferth, C., Renema, J., Wendrich, J. R., Eekhout, T., Seurinck, R., Vandamme, N., et al. (2021). Advances and Opportunities in Single-Cell Transcriptomics for Plant Research. Annu. Rev. Plant Biol. 72, 847–866. doi: 10.1146/annurev-arplant-081720-010120
Shahzad, A., Ullah, S., Dar, A. A., Sardar, M. F., Mehmood, T., Tufail, M. A., et al. (2021). Nexus on climate change: agriculture and possible solution to cope future climate change stresses. Environ. Sci. Pollut. Res. 2021, 1–22.
Shamshad, M., and Sharma, A. (2018). The usage of genomic selection strategy in plant breeding. Next Gener. Plant Breed. 26:93.
Sharma, E., Borah, P., Kaur, A., Bhatnagar, A., Mohapatra, T., Kapoor, S., et al. (2021). A comprehensive transcriptome analysis of contrasting rice cultivars highlights the role of auxin and ABA responsive genes in heat stress response. Genomics 113, 1247–1261.
Sharma, V., Gupta, P., Priscilla, K., SharanKumar, H. B., Veershetty, A., Ramrao, D. P., et al. (2021). Metabolomics Intervention Towards Better Understanding of Plant Traits. Cells 10:346. doi: 10.3390/cells10020346
Shasidhar, Y., Vishwakarma, M. K., Pandey, M. K., Janila, P., Variath, M. T., Manohar, S. S., et al. (2017). Molecular Mapping of Oil Content and Fatty Acids Using Dense Genetic Maps in Groundnut (Arachis hypogaea L.). Front. Plant Sci. 8:794. doi: 10.3389/fpls.2017.00794
Shelden, M. C., Dias, D. A., Jayasinghe, N. S., Bacic, A., and Roessner, U. (2016). Root spatial metabolite profiling of two genotypes of barley (Hordeum vulgare L.) reveals differences in response to short-term salt stress. J. Exp. Bot. 67, 3731–3745. doi: 10.1093/jxb/erw059
Shen, Y., Liu, J., Geng, H., et al. (2018). De novo assembly of a Chinese soybean genome. Sci. China Life Sci. 61, 871–884. doi: 10.1007/s11427-018-9360-0
Shi, J., Yan, B., Lou, X., Ma, H., and Ruan, S. (2017). Comparative transcriptome analysis reveals the transcriptional alterations in heat-resistant and heat-sensitive sweet maize (Zea mays L.) varieties under heat stress. BMC Plant Biol. 17:26. doi: 10.1186/s12870-017-0973-y
Shi, J., Zhao, L., Yan, B., Zhu, Y., Ma, H., Chen, W., et al. (2019). Comparative Transcriptome Analysis Reveals the Transcriptional Alterations in Growth- and Development-Related Genes in Sweet Potato Plants Infected and Non-Infected by SPFMV, SPV2, and SPVG. Int. J. Mol. Sci. 20:1012. doi: 10.3390/ijms20051012
Shi, Y., Liu, A., Li, J., Zhang, J., Zhang, B., Ge, Q., et al. (2019). Dissecting the genetic basis of fiber quality and yield traits in interspecific backcross populations of Gossypium hirsutum× Gossypium barbadense. Mol. Genet. Genomics 294, 1385–1402. doi: 10.1007/s00438-019-01582-8
Shikha, M., Kanika, A., Rao, A. R., Mallikarjuna, M. G., Gupta, H. S., and Nepolean, T. (2017). Genomic selection for drought tolerance using genome-wide SNPs in maize. Front. Plant Sci. 8:550. doi: 10.3389/fpls.2017.00550
Singh, N., Mansoori, A., Dey, D., Kumar, R., and Kumar, A. (2021). “Potential of Metabolomics in Plant Abiotic Stress Management,” in Omics Technologies for Sustainable Agriculture and Global Food Security, Vol. II, eds A. Kumar, R. Kumar, P. Shukla, and H. K. Patel (Singapore: Springer), 193–214. doi: 10.1007/978-981-16-2956-3_7
Singh, N., Rai, V., and Singh, N. K. (2020). Multi-omics strategies and prospects to enhance seed quality and nutritional traits in pigeonpea. Nucleus 63, 249–256. doi: 10.1007/s13237-020-00341-0
Singh, R. K., and Prasad, M. (2021). Delineating the epigenetic regulation of heat and drought response in plants. Crit. Rev. Biotechnol. 2021, 1–14. doi: 10.1080/07388551.2021.1946004
Singh, R. K., Muthamilarasan, M., and Prasad, M. (2021). Biotechnological approaches to dissect climate-resilient traits in millets and their application in crop improvement. J. Biotechnol. 327, 64–73. doi: 10.1016/j.jbiotec.2021.01.002
Singh, R. K., Prasad, A., Muthamilarasan, M., Parida, S. K., and Prasad, M. (2020). Breeding and biotechnological interventions for trait improvement: status and prospects. Planta 252:54. doi: 10.1007/s00425-020-03465-4
Singhal, R. K., Saha, D., Skalicky, M., Mishra, U. N., Chauhan, J., Behera, L. P., et al. (2021). Crucial cell signaling compounds cross-talk and integrative multi-omics techniques for salinity stress tolerance in plants. Front. Plant Sci. 2021:1227. doi: 10.3389/fpls.2021.670369
Singhal, T., Satyavathi, C. T., Singh, S. P., Kumar, A., Sankar, S. M., Bhardwaj, C., et al. (2021). Multi-Environment Quantitative Trait Loci Mapping for Grain Iron and Zinc Content Using Bi-parental Recombinant Inbred Line Mapping Population in Pearl Millet. Front. Plant Sci. 12:744. doi: 10.3389/fpls.2021.659789
Sinha, P., Singh, V. K., Bohra, A., Kumar, A., Reif, J. C., and Varshney, R. K. (2021). Genomics and breeding innovations for enhancing genetic gain for climate resilience and nutrition traits. Theoret. Appl. Genet. 2021:15. doi: 10.1007/s00122-021-03847-6
Sinha, R. K., and Verma, S. S. (2021). “Proteomics approach in horticultural crops for abiotic-stress tolerance,” in Stress Tolerance in Horticultural Crops, eds A. Kumar, A. C. Rai, A. Rai, K. K. Rai, and V. P. Rai (Sawston: Woodhead Publishing), 371–385.
Smith, A. M., Bettey, M., and Bedford, I. D. (1989). Evidence that the rb locus alters the starch content of developing pea embryos through an effect on ADP glucose pyrophosphorylase. Plant Physiol. 89, 1279–1284. doi: 10.1104/pp.89.4.1279
Smith, M. R., Rao, I. M., and Merchant, A. (2018). Source-sink relationships in crop plants and their influence on yield development and nutritional quality. Front. Plant Sci. 9:1889. doi: 10.3389/fpls.2018.01889
SETAC (2019). Technical Issue Paper: OMICS: Complete Systems and Complete Analyses. Pensacola, FL: SETAC, 4.
Song, J. M., Guan, Z., Hu, J., Guo, C., Yang, Z., Wang, S., et al. (2020). Eight high-quality genomes reveal pan-genome architecture and ecotype differentiation of Brassica napus. Nat. Plants 2020, 34–45. doi: 10.1038/s41477-019-0577-7
Soriano, J. M., Colasuonno, P., Marcotuli, I., and Gadaleta, A. (2021). Meta-QTL analysis and identification of candidate genes for quality, abiotic and biotic stress in durum wheat. Sci. Rep. 11:11877. doi: 10.1038/s41598-021-91446-2
Spindel, J., Begum, H., Virk, P., Collard, B., Redoña, E., Atlin, G., et al. (2015). Genomic selection and association mapping in rice (Oryza sativa): Effect of trait genetic architecture, training population composition, marker number and statistical model on accuracy of rice genomic selection in elite, tropical rice breeding lines. PLoS Genet. 11:1–25. doi: 10.1371/journal.pgen.1004982
Srivastava, R. K., Singh, R. B., Pujarula, V. L., Bollam, S., Pusuluri, M., Chellapilla, T. S., et al. (2020). Genome-Wide Association Studies and Genomic Selection in Pearl Millet: Advances and Prospects. Front. Genet. 10:1389. doi: 10.3389/fgene.2019.01389
Steinwand, M. A., and Ronald, P. C. (2020). Crop biotechnology and the future of food. Nat. Food 1, 273–283. doi: 10.1038/s43016-020-0072-3
Stone, S. L. (2019). Role of the ubiquitin proteasome system in plant response to abiotic stress. Int. Rev. Cell. Mol. Biol. 343, 65–110. doi: 10.1016/bs.ircmb.2018.05.012
Suharti, W. S., Nose, A., and Zheng, S. H. (2016). Metabolomic study of two rice lines infected by Rhizoctonia solani in negative ion mode by CE/TOF-MS. J. Plant Physiol. 206, 13–24. doi: 10.1016/j.jplph.2016.09.004
Sun, C., Ali, K., Yan, K., Fiaz, S., Dormatey, R., Bi, Z., et al. (2021). Exploration of Epigenetics for Improvement of Drought and Other Stress Resistance in Crops: A Review. Plants 10:1226. doi: 10.3390/plants10061226
Sun, M., Huang, D., Zhang, A., Khan, I., Yan, H., Wang, X., et al. (2020). Transcriptome analysis of heat stress and drought stress in pearl millet based on Pacbio full-length transcriptome sequencing. BMC Plant Biol. 20:323. doi: 10.1186/s12870-020-02530-0
Sun, Z., Li, H., Zhang, Y., Li, Z., Ke, H., Wu, L., et al. (2018). Identification of SNPs and Candidate Genes Associated With Salt Tolerance at the Seedling Stage in Cotton (Gossypium hirsutum L.). Front. Plant Sci. 9:1011. doi: 10.3389/fpls.2018.01011
Sun, Z., Wang, X., Liu, Z., Gu, Q., Zhang, Y., Li, Z., et al. (2017). Genome-wide association study discovered genetic variation and candidate genes of fibre quality traits in Gossypium hirsutum L. Plant Biotechnol. J. 15, 982–996.
Swamy, B. M., Shamsudin, N. A. A., Abd Rahman, S. N., Mauleon, R., Ratnam, W., Cruz, M. T. S., et al. (2017). Association mapping of yield and yield-related traits under reproductive stage drought stress in rice (Oryza sativa L.). Rice 10:21. doi: 10.1186/s12284-017-0161-6
Swinnen, G., Goossens, A., and Pauwels, L. (2016). Lessons from domestication: targeting cis-regulatory elements for crop improvement. Trends Plant Sci. 21, 506–515. doi: 10.1016/j.tplants.2016.01.014
Tahir ul Qamar, M., Zhu, X., Khan, M. S., Xing, F., and Chen, L. L. (2020). Pan-genome: A promising resource for noncoding RNA discovery in plants. Plant Genome 13:e20046. doi: 10.1002/tpg2.20046
Tahmasebi, A., Ashrafi-Dehkordi, E., Shahriari, A. G., Mazloomi, S. M., and Ebrahimie, E. (2019). Integrative meta-analysis of transcriptomic responses to abiotic stress in cotton. Prog. Biophys. Mol. Biol. 146, 112–122. doi: 10.1016/j.pbiomolbio.2019.02.005
Tamhane, V. A., Sant, S. S., Jadhav, A. R., War, A. R., Sharma, H. C., Jaleel, A., et al. (2021). Label-free quantitative proteomics of Sorghum bicolor reveals the proteins strengthening plant defense against insect pest Chilo partellus. Proteome Sci. 19:6. doi: 10.1186/s12953-021-00173-z
Tan, C. T., Lim, Y. S., and Lau, S. E. (2017). Proteomics in commercial crops: An overview. J. Protozool. 169, 176–188.
Tao, Y., Luo, H., Xu, J., Cruickshank, A., Zhao, X., Teng, F., et al. (2021). Extensive variation within the pan-genome of cultivated and wild sorghum. Nat. Plants 7, 766–773.
Tao, Y., Zhao, X., Mace, E., Henry, R., and Jordan, D. (2019). Exploring and Exploiting Pan-genomics for Crop Improvement. Mol. Plant. 12, 156–169. doi: 10.1016/j.molp.2018.12.016
Tardieu, F., Cabrera-Bosquet, L., Pridmore, T., and Bennett, M. (2017). Plant phenomics, from sensors to knowledge. Curr. Biol. 27, R770–R783. doi: 10.1016/j.cub.2017.05.055
Templer, S. E., Ammon, A., Pscheidt, D., Ciobotea, O., Schuy, C., McCollum, C., et al. (2017). Metabolite profiling of barley flag leaves under drought and combined heat anddrought stress reveals metabolic QTLs for metabolites associated with antioxidant defense. J. Exp. Bot. 68, 1697–1713. doi: 10.1093/jxb/erx038
Tettelin, H., Vega, M., Michael, J. C., Claudio, D., Duccio, M., Naomi, L. W., et al. (2005). Genome analysis of multiple pathogenic isolates of Streptococcus agalactiae: Implications for the microbial “pan-genome”. Proc. Natl. Acad. Sci. U S A 2005, 13950–13955. doi: 10.1073/pnas.0506758102
The World Resources Institute (2019). Creating a sustainable food future: Final Report: A menu of solutions to feed nearly 10 billion people by 2050. Washington D.C: WRI, 558.
Thibivilliers, S., and Libault, M. (2021). Enhancing Our Understanding of Plant Cell-to-Cell Interactions Using Single-Cell Omics. Front. Plant Sci. 12:696811. doi: 10.3389/fpls.2021.696811
Tian, X., Liu, Y., Huang, Z., Duan, H., Tong, J., He, X., et al. (2015). Comparative proteomic analysis of seedling leaves of cold-tolerant and-sensitive spring soybean cultivars. Mol. Biol. Rep. 42, 581–601. doi: 10.1007/s11033-014-3803-4
Trivedi, P., Mattupalli, C., Eversole, K., and Leach, J. E. (2021). Enabling sustainable agriculture through understanding and enhancement of microbiomes. New Phytol. 230, 2129–2147. doi: 10.1111/nph.17319
UN (2017). “World Population Prospects: The 2017 Revision, Key Findings and Advance Tables,” in Working Paper No. ESA/P/WP/248, (New York, NY: United Nations), 46.
Upadhyaya, H. D., Bajaj, D., Das, S., Kumar, V., Gowda, C. L. L., Sharma, S., et al. (2016). Genetic dissection of seed-iron and zinc concentrations in chickpea. Sci. Rep. 6:24050. doi: 10.1038/srep24050
van Bezouw, R. F., Keurentjes, J. J., Harbinson, J., and Aarts, M. G. (2019). Converging phenomics and genomics to study natural variation in plant photosynthetic efficiency. Plant J. 97, 112–133. doi: 10.1111/tpj.14190
van Mierlo, G., and Vermeulen, M. (2021). Chromatin Proteomics to Study Epigenetics - Challenges and Opportunities. Mol. Cell. Proteom. 20:100056. doi: 10.1074/mcp.R120.002208
Varshney, R. K., Bohra, A., Yu, J., Graner, A., Zhang, Q., and Sorrells, M. E. (2021). Designing future crops: genomics-assisted breeding comes of age. Trends Plant Sci. 26, 631–649.
Varshney, R. K., Kudapa, H., Pazhamala, L., Chitikineni, A., Thudi, M., Bohra, A., et al. (2015). Translational genomics in agriculture: Some examples in grain legumes. Crit. Rev. Plant Sci. 34, 169–194. doi: 10.1080/07352689.2014.897909
Vaughan, M. M., Block, A., Christensen, S. A., Allen, L. H., and Schmelz, E. A. (2018). The effects of climate change associated abiotic stresses on maize phytochemical defenses. Phytochem. Rev. 17, 37–49.
Vetriventhan, M., Azevedo, V. C. R., Upadhyaya, H. D., et al. (2020). Genetic and genomic resources, and breeding for accelerating improvement of small millets: current status and future interventions. Nucleus 63, 217–239. doi: 10.1007/s13237-020-00322-3
Villate, A., San Nicolas, M., Gallastegi, M., Aulas, P. A., Olivares, M., Usobiaga, A., et al. (2021). Metabolomics as a prediction tool for plants performance under environmental stress. Plant Sci. 303:110789. doi: 10.1016/j.plantsci.2020.110789
Vishwakarma, M. K., Kale, S. M., Sriswathi, M., Naresh, T., Shasidhar, Y., Garg, V., et al. (2017). Genome-Wide Discovery and Deployment of Insertions and Deletions Markers Provided Greater Insights on Species, Genomes, and Sections Relationships in the Genus Arachis. Front. Plant Sci. 8:2064. doi: 10.3389/fpls.2017.02064
Vo, K. T. X., Rahman, M. M., Rahman, M. M., Trinh, K. T. T., Kim, S. T., and Jeon, J. S. (2021). Proteomics and Metabolomics Studies on the Biotic Stress Responses of Rice: an Update. Rice 14, 1–16. doi: 10.1186/s12284-021-00461-4
Voss-Fels, K. P., Cooper, M., and Hayes, B. J. (2019). Accelerating crop genetic gains with genomic selection. Theor. Appl. Genet. 132, 669–686. doi: 10.1007/s00122-018-3270-8
Wakeel, A., Farooq, M., Bashir, K., and Ozturk, L. (2018). “Micronutrient Malnutrition and Biofortification: Recent advances and future perspectives,” in Plant Micronutrient Use Efficiency: Molecular and Genomic Perspectives in Crop Plants, eds M. A. Hossain, T. Kamiya, D. J. Burritt, L. S. P. Tran, and T. Fujiwara (Cambridge, MA: Academic Press), 225–243. doi: 10.1016/B978-0-12-812104-7.00017-4
Wang, J., Chen, X., Chu, S., You, Y., Chi, Y., Wang, R., et al. (2022). Comparative cytology combined with transcriptomic and metabolomic analyses of Solanum nigrum L. in response to Cd toxicity. J. Hazard. Mater. 423:127168. doi: 10.1016/j.jhazmat.2021.127168
Wang, J., Liang, C., Yang, S., Song, J., Li, X., Dai, X., et al. (2021). iTRAQ-based quantitative proteomic analysis of heat stress-induced mechanisms in pepper seedlings. PeerJ 9:e11509. doi: 10.7717/peerj.11509
Wang, J., Vanga, S. K., Saxena, R., Orsat, V., and Raghavan, V. (2018). Effect of Climate Change on the Yield of Cereal Crops: A Review. Climate 6:41. doi: 10.3390/cli6020041
Wang, J., Yan, C., Li, Y., Li, C., Zhao, X., Yuan, C., et al. (2019). GWAS Discovery of Candidate Genes for Yield-Related Traits in Peanut and Support from Earlier QTL Mapping Studies. Genes 10:803. doi: 10.3390/genes10100803
Wang, L., Liu, L., Ma, Y., Li, S., Dong, S., and Zu, W. (2018). Transcriptome profiling reveals PEG-simulated drought, heat and combined stress response mechanisms in soybean[J]. Computat. Biol. Chem. 77, 413–429. doi: 10.1016/j.compbiolchem.2018.09.012
Wang, M., Wang, P., Tu, L., Zhu, S., Zhang, L., Li, Z., et al. (2016). Multi-omics maps of cotton fibre reveal epigenetic basis for staged single-cell differentiation. Nucleic Acids Res. 44, 4067–4079. doi: 10.1093/nar/gkw238
Wang, W., Mauleon, R., Hu, Z., Chebotarov, D., Tai, S., Wu, Z., et al. (2018). Genomic variation in 3,010 diverse accessions of Asian cultivated rice. Nature 557, 43–49. doi: 10.1038/s41586-018-0063-9
Wang, X., Yang, X., Feng, Y., Dang, P., Wang, W., Graze, R., et al. (2021). Transcriptome Profile Reveals Drought-Induced Genes Preferentially Expressed in Response to Water Deficit in Cultivated Peanut (Arachis hypogaea L.). Front. Plant Sci. 12:645291. doi: 10.3389/fpls.2021.645291
Wang, Z., Gerstein, M., and Snyder, M. (2009). RNA-Seq: a revolutionary tool for transcriptomics. Nat. Rev. Genet. 10, 57–63. doi: 10.1038/nrg2484
Wang, Z., Shi, H., Yu, S., Zhou, W., Li, J., Liu, S., et al. (2019). Comprehensive transcriptomics, proteomics, and metabolomics analyses of the mechanisms regulating tiller production in low-tillering wheat. Theoret. Appl. Genet. 132, 2181–2193.
War, A. R., Paulraj, M. G., Ahmad, T., Buhroo, A. A., Hussain, B., Ignacimuthu, S., et al. (2012). Mechanisms of plant defense against insect herbivores. Plant Signal Behav. 7, 1306–1320. doi: 10.4161/psb.21663
Wasaya, A., Zhang, X., Fang, Q., and Yan, Z. (2018). Root Phenotyping for Drought Tolerance: A Review. Agronomy 8:241. doi: 10.3390/agronomy8110241
Wassie, S. B. (2020). Natural resource degradation tendencies in Ethiopia: a review. Environ. Syst. Res. 9, 1–29.
Weckwerth, W., Ghatak, A., Bellaire, A., Chaturvedi, P., and Varshney, R. K. (2020). PANOMICS meets germplasm. Plant Biotechnol. J. 18, 1507–1525. doi: 10.1111/pbi.13372
Wolkenhauer, O., and Muir, A. (2011). “The complexity of cell-biological systems,” in Philosophy of complex systems, eds C. A. Hooker, D. M. Gabbay, P. Thagard, and J. Woods (Amsterdam: North-Holland), 355–385.
Wu, X., and Wang, W. (2016). Increasing confidence of proteomics data regarding the identification of stress-responsive proteins in crop plants. Front. Plant Sci. 7:702. doi: 10.3389/fpls.2016.00702
Wu, X., Gong, F., Cao, D., Hu, X., and Wang, W. (2016). Advances in crop proteomics: PTMs of proteins under abiotic stress. Proteomics 16, 847–865. doi: 10.1002/pmic.201500301
Wu, X., Liang, Y., Gao, H., Wang, J., Zhao, Y., Hua, L., et al. (2021). Enhancing rice grain production by manipulating the naturally evolved cis-regulatory element-containing inverted repeat sequence of OsREM20. Mol. Plant. 14, 997–1011. doi: 10.1016/j.molp.2021.03.016
Würschum, T., Liu, W., Maurer, H. P., Abel, S., and Reif, J. C. (2012). Dissecting the genetic architecture of agronomic traits in multiple segregating populations in rapeseed (Brassica napus L.). Theor. Appl. Genet. 124, 153–161. doi: 10.1007/s00122-011-1694-5
Xiao, Y., Tong, H., Yang, X., Xu, S., Pan, Q., et al. (2016). Genome-wide dissection of the maize ear genetic architecture using multiple populations. New Phytol. 210, 1095–1106.
Xu, C., Xia, C., Xia, Z., Zhou, X., Huang, J., Huang, Z., et al. (2018). Physiological and transcriptomic responses of reproductive stage soybean to drought stress. Plant cell Rep. 37, 1611–1624.
Xu, J., Yuan, Y., Xu, Y., Zhang, G., Guo, X., Wu, F., et al. (2014). Identification of candidate genes for drought tolerance by whole-genome resequencing in maize. BMC Plant Biol. 14:1–15. doi: 10.1186/1471-2229-14-83
Xu, L., Hu, K., Zhang, Z., Guan, C., Chen, S., Hua, W., et al. (2015). Genome-wide association study reveals the genetic architecture of flowering time in rapeseed (Brassica napus L.). DNA Res. 23, 43–52. doi: 10.1093/dnares/dsv035
Xu, X., and Bai, G. (2015). Whole-genome resequencing: changing the paradigms of SNP detection, molecular mapping and gene discovery. Mol. Breed. 35, 1–11.
Xu, X., Crow, M., Rice, B. R., Li, F., Harris, B., Liu, L., et al. (2021). Single-cell RNA sequencing of developing maize ears facilitates functional analysis and trait candidate gene discovery. Dev. Cell 56, 557–568.
Xu, X., Liu, X., Ge, S., Jensen, J. D., Hu, F., Li, X., et al. (2012). Resequencing 50 accessions of cultivated and wild rice yields markers for identifying agronomically important genes. Nat. Biotechnol. 30, 105–111. doi: 10.1038/nbt.2050
Xu, Y., Liu, X., Fu, J., Wang, H., Wang, J., Huang, C., et al. (2020). Enhancing genetic gain through genomic selection: From livestock to plants. Plant Commun. 1:100005. doi: 10.1016/j.xplc.2019.100005
Xu, Y., Zeng, X., Wu, J., Zhang, F., Li, C., Jiang, J., et al. (2018). iTRAQ-Based Quantitative Proteome Revealed Metabolic Changes in Winter Turnip Rape (Brassica rapa L.) under Cold Stress. Int. J. Mol. Sci. 19:3346. doi: 10.3390/ijms19113346
Yan, G., Liu, H., Wang, H., Lu, Z., Wang, Y., Mullan, D., et al. (2017). Accelerated generation of selfed pure line plants for gene identification and crop breeding. Front. Plant Sci. 8:1786. doi: 10.3389/fpls.2017.01786
Yang, M., Yang, J., Su, L., Sun, K., Li, D., Liu, Y., et al. (2019). Metabolic profile analysis and identification of key metabolites during rice seed germination under low-temperature stress. Plant Sci. 289:110282. doi: 10.1016/j.plantsci.2019.110282
Yang, Y., Yu, Y., Bi, C., and Kang, Z. (2016). Quantitative Proteomics Reveals the Defense Response of Wheat against Puccinia striiformis f. sp. tritici. Sci. Rep. 6:34261. doi: 10.1038/srep34261
Yang, Z., Li, X., Zhang, N., Zhang, Y. N., Jiang, H. W., Gao, J., et al. (2016). Detection of quantitative trait loci for kernel oil and protein concentration in a B73 and Zheng58 maize cross. Genet. Mol. Res. 15:10. doi: 10.4238/gmr.15038951
Ye, J., Wang, X., Wang, W., Yu, H., Ai, G., Li, C., et al. (2021). Genome-wide association study reveals the genetic architecture of 27 agronomic traits in tomato. Plant Physiol. 00, 1–15. doi: 10.1093/plphys/kiab230
Yu, J., Golicz, A. A., Lu, K., Dossa, K., Zhang, Y., Chen, J., et al. (2019). Insight into the evolution and functional characteristics of the pan-genome assembly from sesame landraces and modern cultivars. Plant Biotechnol. J. 17, 881–892.
Yu, R., Jiang, Q., Xv, C., Li, L., Bu, S., and Shi, G. (2019). Comparative proteomics analysis of peanut roots reveals differential mechanisms of cadmium detoxification and translocation between two cultivars differing in cadmium accumulation. BMC Plant Biol. 19:137. doi: 10.1186/s12870-019-1739-5
Yuan, L., Liu, X., Luo, M., Yang, S., and Wu, K. (2013). Involvement of histone modifications in plant abiotic stress responses. J. Integrat. Plant Biol. 55, 892–901. doi: 10.1111/jipb.12060
Yuan, Y., Cairns, J. E., Babu, R., Gowda, M., Makumbi, D., Magorokosho, C., et al. (2019). Genome-wide association mapping and genomic prediction analyses reveal the genetic architecture of grain yield and flowering time under drought and heat stress conditions in maize. Front. Plant Sci. 2019:1919. doi: 10.3389/fpls.2018.01919
Yue, R., Lu, C., Han, X., Guo, S., Yan, S., Liu, L., et al. (2018). Comparative proteomic analysis of maize (Zea mays L.) seedlings under rice black-streaked dwarf virus infection. BMC Plant Biol. 18:191. doi: 10.1186/s12870-018-1419-x
Yue, R., Lu, C., Qi, J., Han, X., Yan, S., Guo, S., et al. (2016). Transcriptome Analysis of Cadmium-Treated Roots in Maize (Zea mays L.). Front. Plant Sci. 7:1298. doi: 10.3389/fpls.2016.01298
Zadražnik, T., Hollung, K., Egge-Jacobsen, W., Meglič, V., and Šuštar-Vozlič, J. (2013). Differential proteomic analysis of drought stress response in leaves of common bean (Phaseolus vulgaris L.). J. Proteom. 78, 254–272. doi: 10.1016/j.jprot.2012.09.021
Zaitlin, D. (2020). “Tobacco Biotechnology and Omics Taskforce Technical Report,” in Literature Review on the Use of Biotechnology and Omics, (Lexington: Kentucky Tobacco Research and Development Center), 64.
Zandalinas, S. I., Fritschi, F. B., and Mittler, R. (2021). Globl warming, climate change, and environmental pollution: Recipe for a multifactorial stress combination disaster. Trends Plant Sci. 26, 588–599. doi: 10.1016/j.tplants.2021.02.011
Zenda, T., Liu, S., and Duan, H. (2020). “Adapting Cereal Grain Crops to Drought Stress: 2020 and Beyond,” in Abiotic Stress in Plants, eds S. Fahad, S. Saud, Y. Chen, C. Wu, and D. Wang (London: IntechOpen), 1–30. doi: 10.5772/intechopen.93845
Zenda, T., Liu, S., Dong, A., and Duan, H. (2021). Advances in Cereal Crop Genomics for Resilience under Climate Change. Life 11:502. doi: 10.3390/life11060502
Zenda, T., Liu, S., Wang, X., et al. (2019). Key Maize Drought-Responsive Genes and Pathways Revealed by Comparative Transcriptome and Physiological Analyses of Contrasting Inbred Lines. Int. J. Mol. Sci. 20:1268. doi: 10.3390/ijms20061268
Zenda, T., Liu, S., Wang, X., Jin, H., Liu, G., and Duan, H. (2018). Comparative Proteomic and Physiological Analyses of Two Divergent Maize Inbred Lines Provide More Insights into Drought-Stress Tolerance Mechanisms. Int. J. Mol. Sci. 19:3225. doi: 10.3390/ijms19103225
Zeng, W., Peng, Y., Zhao, X., Wu, B., Chen, F., Ren, B., et al. (2019). Comparative Proteomics Analysis of the Seedling Root Response of Drought-sensitive and Drought-tolerant Maize Varieties to Drought Stress. Int. J. Mol. Sci. 20:2793. doi: 10.3390/ijms20112793
Zhan, A., Schneider, H., and Lynch, J. P. (2015). Reduced lateral root branching density improves drought tolerance in maize. Plant Physiol. 168, 1603–1615.
Zhang, C., and Hao, Y. J. (2020). Advances in Genomic, Transcriptomic, and Metabolomic Analyses of Fruit Quality in Fruit Crops. Horticult. Plant J. 6, 361–371. doi: 10.1016/j.hpj.2020.11.001
Zhang, J., Wang, F., Liang, F., Zhang, Y., Ma, L., Wang, H., et al. (2018). Functional analysis of a pathogenesis-related thaumatin-like protein gene TaLr35PR5 from wheat induced by leaf rust fungus. BMC Plant Biol. 18:76. doi: 10.1186/s12870-018-1297-2
Zhang, K., Liu, H., Tao, P., and Chen, H. (2014). Comparative Proteomic Analyses Provide New Insights into Low Phosphorus Stress Responses in Maize Leaves. PLoS One 9:e98215. doi: 10.1371/journal.pone.0098215
Zhang, M., Cheng, S. T., Wang, H. Y., Wu, J. H., Luo, Y. M., Wang, Q., et al. (2017). iTRAQ-based proteomic analysis of defence responses triggered by the necrotrophic pathogen Rhizoctonia solani in cotton. J. Proteomics 152, 226–235. doi: 10.1016/j.jprot.2016.11.011
Zhang, P., Zhong, K., Zhong, Z., et al. (2019). Genome-wide association study of important agronomic traits within a core collection of rice (Oryza sativa L.). BMC Plant Biol. 19:259. doi: 10.1186/s12870-019-1842-7
Zhang, Q., Xu, M., Xia, X., Komatsuda, T., Varshney, R. K., and Shi, K. (2020). Crop genetics research in Asia: improving food security and nutrition. Theoret. Appl. Genet. 133, 1339–1344. doi: 10.1007/s00122-020-03597-x
Zhang, X., Liu, P., Qing, C., Yang, C., Shen, Y., and Ma, L. (2021). Comparative transcriptome analyses of maize seedling root responses to salt stress. PeerJ 9:e10765.
Zhang, X., Shabala, S., Koutoulis, A., Shabala, L., and Zhou, M. (2017). Meta-analysis of major QTL for abiotic stress tolerance in barley and implications for barley breeding. Planta 245, 283–295.
Zhang, Y., and Zeng, L. (2020). Crosstalk between ubiquitination and other post-translational protein modifications in plant immunity. Plant Commun. 1:100041. doi: 10.1016/j.xplc.2020.100041
Zhang, Y., Fu, Y., Wang, Q., Liu, X., Li, Q., and Chen, J. (2020). Transcriptome analysis reveals rapid defence responses in wheat induced by phytotoxic aphid Schizaphis graminum feeding. BMC Genomics 21:339. doi: 10.1186/s12864-020-6743-5
Zhao, C., Zayed, O., Yu, Z., Jiang, W., Zhu, P., Hsu, C. C., et al. (2018). Leucine-rich repeat extensin proteins regulate plant salt tolerance in Arabidopsis. Proc. Natl. Acad. Sci. U S A. 115, 13123–13128. doi: 10.1073/pnas.1816991115
Zhao, C., Zhang, Y., Du, J., Guo, X., Wen, W., Gu, S., et al. (2019). Crop phenomics: current status and perspectives. Front. Plant Sci. 10:714.
Zhao, F., Wang, Y., Zheng, J., et al. (2020). A genome-wide survey of copy number variations reveals an asymmetric evolution of duplicated genes in rice. BMC Biol. 18:73. doi: 10.1186/s12915-020-00798-0
Zhao, J., Huang, L., Ren, X., Pandey, M. K., Wu, B., Chen, Y., et al. (2017). Genetic Variation and Association Mapping of Seed-Related Traits in Cultivated Peanut (Arachis hypogaea L.) Using Single-Locus Simple Sequence Repeat Markers. Front. Plant Sci. 8:2105. doi: 10.3389/fpls.2017.02105
Zhao, Q., Feng, Q., Lu, H., Li, Y., Wang, A., Tian, Q., et al. (2018). Pan-genome analysis highlights the extent of genomic variation in cultivated and wild rice. Nat. Genet. 50, 278–284. doi: 10.1038/s41588-018-0041-z
Zhou, Z., Jiang, Y., Wang, Z., Gou, Z., Lyu, J., Li, W., et al. (2015). Resequencing 302 wild and cultivated accessions identifies genes related to domestication and improvement in soybean. Nat. Biotechnol. 33, 408–414.
Zhu, Q., Gao, P., Wan, Y., Cui, H., Fan, C., Liu, S., et al. (2018). Comparative transcriptome profiling of genes and pathways related to resistance against powdery mildew in two contrasting melon genotypes. Sci. Horticult. 227, 169–180. doi: 10.1016/j.scienta.2017.09.033
Keywords: abiotic stress, biotic stress, pan-genomes, nutritive traits, multi-omics technologies, systems biology approach, genomics assisted breeding (GAB), single cell transcriptomics
Citation: Zenda T, Liu S, Dong A, Li J, Wang Y, Liu X, Wang N and Duan H (2021) Omics-Facilitated Crop Improvement for Climate Resilience and Superior Nutritive Value. Front. Plant Sci. 12:774994. doi: 10.3389/fpls.2021.774994
Received: 13 September 2021; Accepted: 08 November 2021;
Published: 01 December 2021.
Edited by:
Varodom Charoensawan, Mahidol University, ThailandReviewed by:
Gonzalo Gajardo, University of Los Lagos, ChileWirulda Pootakham, National Center for Genetic Engineering and Biotechnology (BIOTEC), Thailand
Copyright © 2021 Zenda, Liu, Dong, Li, Wang, Liu, Wang and Duan. This is an open-access article distributed under the terms of the Creative Commons Attribution License (CC BY). The use, distribution or reproduction in other forums is permitted, provided the original author(s) and the copyright owner(s) are credited and that the original publication in this journal is cited, in accordance with accepted academic practice. No use, distribution or reproduction is permitted which does not comply with these terms.
*Correspondence: Huijun Duan, hjduan@hebau.edu.cn; Tinashe Zenda, zenda@hebau.edu.cn