- 1Rice Research Institute, Shenyang Agricultural University, Shenyang, China
- 2State Key Laboratory of Rice Biology, China National Rice Research Institute, Hangzhou, China
- 3Shenzhen Branch, Guangdong Laboratory of Lingnan Modern Agriculture, Genome Analysis Laboratory of the Ministry of Agriculture and Rural Affairs, Agricultural Genomics Institute at Shenzhen, Chinese Academy of Agricultural Sciences, Shenzhen, China
Nitrogen is essential for plant growth and yield, and it is, therefore, crucial to increase the nitrogen-use efficiency (NUE) of crop plants in fields. In this study, we measured four major low-nitrogen-induced growth response (LNGR) agronomic traits (i.e., plant height, tiller number, chlorophyll content, and leaf length) of the 225-rice-variety natural population from the Rice 3K Sequencing Project across normal nitrogen (NN) and low nitrogen (LN) environments. The LNGR phenotypic difference between NN and LN levels was used for gene analysis using a genome-wide association study (GWAS) combined with 111,205 single-nucleotide polymorphisms (SNPs) from the available sequenced data from the 3K project. We obtained a total of 56 significantly associated SNPs and 4 candidate genes for 4 LNGR traits. Some loci were located in the candidate regions, such as MYB61, OsOAT, and MOC2. To further study the role of candidate genes, we conducted haplotype analyses to identify the elite germplasms. Moreover, several other plausible candidate genes encoding LN-related or NUE proteins were worthy of mining. Our study provides novel insight into the genetic control of LNGR and further reveals some related novel haplotypes and potential genes with phenotypic variation in rice.
Introduction
Nitrogen (N) is an essential macronutrient element for plant growth. Plants take up N from the soil mainly in the form of nitrate (NO3–) and/or ammonium (NH4+) (Wang et al., 2012; Fan et al., 2016). In crops, the demand for nitrogen is in accordance with its importance as the basic constituent of nucleotides, amino acids, and chlorophyll (Zhang M. et al., 2021). Nitrogen utilization involves multiple limiting factors, mainly including the varieties and the amount of nitrogen fertilizer, the method of nitrogen fertilizer application, and the expression of nitrogen genes (Ju et al., 2009; Yang et al., 2015). However, some elite rice germplasm can be harvested and produce the same biomass and grain yield under low N (LN) fertilizers, as compared with high N levels. This phenomenon is called low nitrogen-use efficiency (NUE), and thus, it mitigates detrimental effects on ecosystems (Good et al., 2004; Zhang X. et al., 2015). It is critically important to understand the molecular mechanisms of low-N-induced growth response (LNGR) and to develop crop varieties with improved NUE (Fan et al., 2017; Du et al., 2021; Yu et al., 2021).
The NUE is a complex trait controlled by multiple essential genes and quantitative trait loci (QTLs). Nitrogen-use efficiency is closely related to agronomic traits, such as plant height, tiller number, chlorophyll content, and yield, which can be markedly affected by N application in rice. In recent years, several NUE-related genes and loci have been identified by mutants and QTL mapping, targeting various components of nitrogen metabolism and potential regulators of NUE. DENSE AND ERECT PANICLES 1 (DEP1) is a major typical QTL that controls rice yield traits, and its allele, DEP1-1, affects rice NUE. The dominant allele is a nitrogen-insensitive vegetative growth mutation that increases nitrogen uptake and assimilation, resulting in improved harvest index and grain yield at moderate levels of nitrogen fertilization (Sun et al., 2014, 2018).
Allelic variants for the nitrate-transporter gene NRT1.1B display distinctive divergence between two subspecies of rice, namely, indica and japonica, which show the difference in NO3– utilization (Hu et al., 2015). Notably, ARE1 (for abc1 PEPRESSOR1) is prominent due to its enhanced NUE under nitrogen-limiting conditions (Wang et al., 2018a). Subsequently, grain number, plant height, and heading date7 (Ghd7) confer tolerance to LN, in order to positively regulate nitrogen utilization (Good et al., 2004; Saito et al., 2019; Wang et al., 2021). Additionally, the allelic variation in OsNR2 (Gao Z. et al., 2019) caused differences in the NO3– assimilation capacity and NUE of the indica and japonica subspecies. Moreover, it has been reported that other transcription factors, such as OsGRF4 (Li et al., 2018), OsTCP19 (Liu Y. et al., 2021), OsNLP4 (Yu et al., 2021), OsCCA1 (Zhang S. et al., 2021), MYB61 (Gao Y. et al., 2020), and OsNAC42 (Tang et al., 2019), participate in nitrogen utilization regulation. The molecular mechanisms of NUE between diverse rice varieties are still worthy of intensive exploration.
Increasing evidence has revealed that N supply has a significant impact on plant growth and development (McAllister et al., 2012; Chen et al., 2016). However, the conventional approach of linkage mapping has been used to identify potential genes with phenotypic variation, and even when this approach was limited to a biparental experimental population, it was time-consuming (Liu Z. et al., 2016; Wei et al., 2021).
Genome-wide association study (GWAS) is a high-resolution method for analyzing complex genetic traits in plants (Liu N. et al., 2016; Gao H. et al., 2020). Association mapping simultaneously reveals multiple loci of candidate genes with important agronomic traits. In this study, based on the genomic database and bioinformatics analysis, we carried out GWAS with 225 natural varieties of rice from the Rice 3K Sequencing Project with 4 LNGR agronomic phenotypes and analyzed their differences between normal nitrogen (NN) and LN conditions. We subsequently identified 56 LN-induced loci and 4 candidate genes for further analysis.
Materials and Methods
Plant Materials
A set of 225 natural varieties of rice were collected and selected from the Rice 3K Project (Supplementary Table 1), most of which belonged to the four genetic groups. The subpopulations present in this study were estimated using PowerMarker software. All varieties were stored at the China National Rice Research Institute (CNRRI, Hangzhou City, Zhejiang Province, China). All the materials were grown in the experimental field of the Agricultural Genomics Institute at Shenzhen (AGIS, Guangdong Province, China) over the cropping seasons (2020) and with a randomized complete block design.
Statistical Analysis of Phenotypic Variation
We measured the LN-related agronomic traits of the soil and plant analyzer development value (SV), leaf length (LL), plant height (PH), and tiller number (TN) of the 225 rice varieties planted where there were NN levels (180 kg⋅hm–2) and LN levels (90 kg⋅hm–2). There were 20 and 18 cm between rows and individuals, respectively. Additionally, 10 plants were randomly selected from each variety at maturity. For field measurement, the flag leaf became the target of the SV and LL. We calculated the four-trait difference between NN and LN conditions as follows: (the NN phenotype value) – (the LN phenotype value of the same material).
The descriptive statistical analysis was performed in R 4.0.3 software. Based on the phenotypic data, four traits were validated for the analysis of correlation by GraphPad Prism.9 software.
Association Analysis
The genotype data information released from the Rice 3K Sequencing Project (Wang et al., 2018c)1 was downloaded, and the association analysis was determined using EMMAX software (Segura et al., 2013). A total of 111,205 single-nucleotide polymorphisms (SNPs) were filtered with a missing rate < 0.4 and a minimum allele frequency (MAF) > 0.05 (Kang et al., 2010). A population structure correction was made by Admixture_linux-1.3.0 (Alexander and Lange, 2011) for the GWAS analysis.
Candidate Gene
To present clearer association analyses and subsequent results, the significance level of SNPs was set at P ≤ 10–5. Simultaneously, in accordance with linkage disequilibrium (LD) decay values, we examined the candidate genes from ± 100 kb (i.e., 100 kb upstream and 100 kb downstream) of the significant site (Zhang C. et al., 2018). In this study, gene functional annotations and haplotype analysis were performed by the Rice Genome Annotation Project Database.2
Phylogenetic Analyses
We used the PowerMarker (Liu and Muse, 2005) software to first estimated genetic diversity and distance measures of 225 natural rice varieties. We identified the varieties of structure and infer the classification of subpopulations among the individual varieties by using the model-based program POPGENE (Liu N. et al., 2016). We finally used R 4.0.3 software to statistically analyze the genetic relationship between subpopulations.
Results
Phenotyping of Four Low-N-Induced Agronomic Traits
The four LN-related agronomic traits of SV, LL, PH, and TN of the 225 rice varieties with the representative LN-related phenotypes exhibited obvious differences in NN level and LN level (Figure 1). By evaluating the statistics data, we found a broad phenotypic variation of the NUE-related agronomic traits of the natural rice population, indicating that the LN-induced phenotypic data were reliable for further genetic analyses. The correlations of the mean amount for four NUE-related agronomic traits of the 225 rice varieties were separately analyzed (Table 1). The results showed that the correlations between the two nitrogen levels were different.
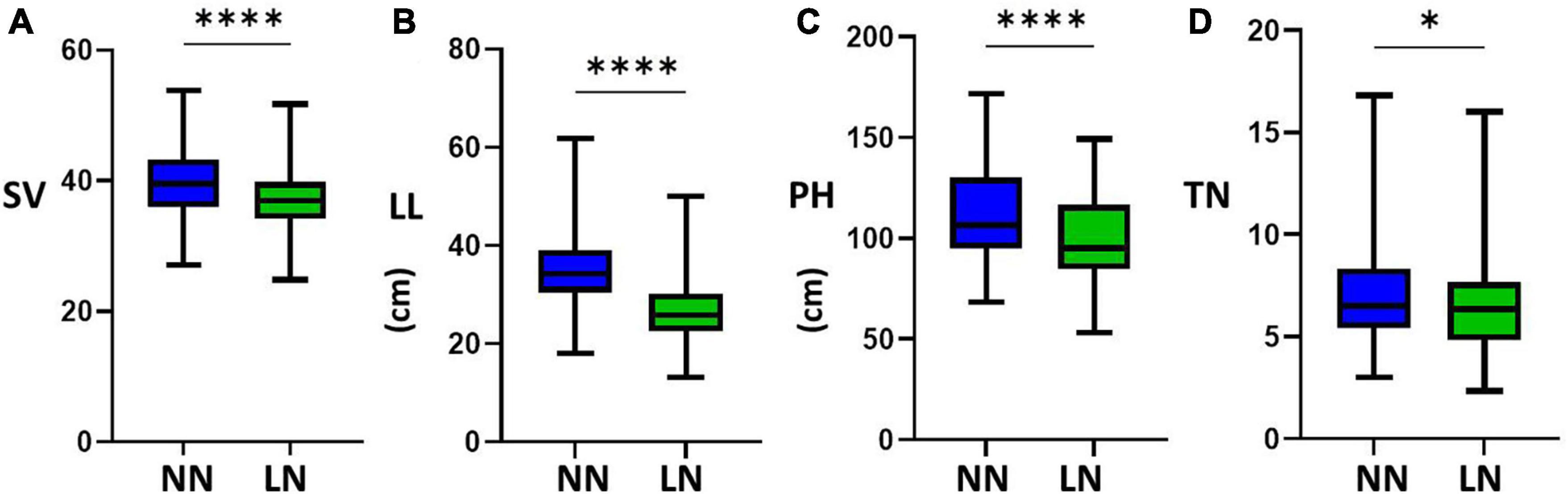
Figure 1. Boxplots of the mean amounts of four nitrogen-use efficiency (NUE)-related agronomic traits of different accessions under the normal nitrogen (NN) level and the low nitrogen (LN) level. Phenotypic traits of (A) soil and plant analyzer development value (SV), (B) leaf length (LL), (C) plant height (PH), and (D) tiller number (TN) (****P < 0.0001; *P < 0.05).
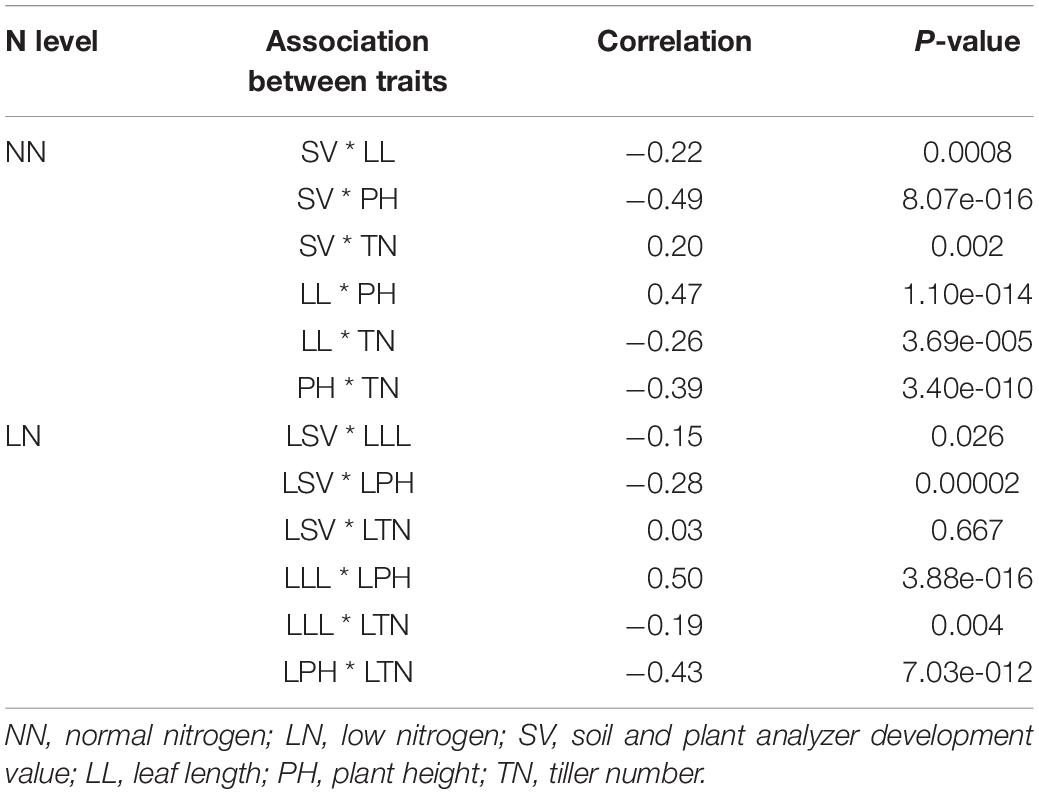
Table 1. Correlation analysis of the mean amount on low-nitrogen-induced growth response (LNGR) agronomic traits.
To confirm whether the phenotypic changes were related to nitrogen, we found that the mean values for the four traits under NN were higher than the mean values for the phenotypic data under LN (P△ SV, △ LL, △ PH < 0.0001, P△ TN < 0.05). The results were consistent with the frequency distribution of phenotypic data, and thus, the N treatment was proven to be effective (Figure 2). By contrast, not all phenotypic values were significantly reduced under LN conditions. To reflect LNGR, we focused on the difference (△NN-LN) between the phenotypic value of NN and LN (Table 2 and Supplementary Table 1). We divided the 225 rice varieties into hyposensitive and sensitive varieties with significantly different values. Notably, the phenotypic values of 45.16%, 13.82%, 23.04%, and 47.93% varieties were insignificantly reduced for SV at LN level (LSV), LL at LN level (LLL), PH at LN level (LPH), and TN at LN level (LTN), respectively, under LN. These varieties might be a target of selection for rice breeding and cultivation under LN conditions, especially Geng_7623 (HY124) and Huhui_91269 (HY206).
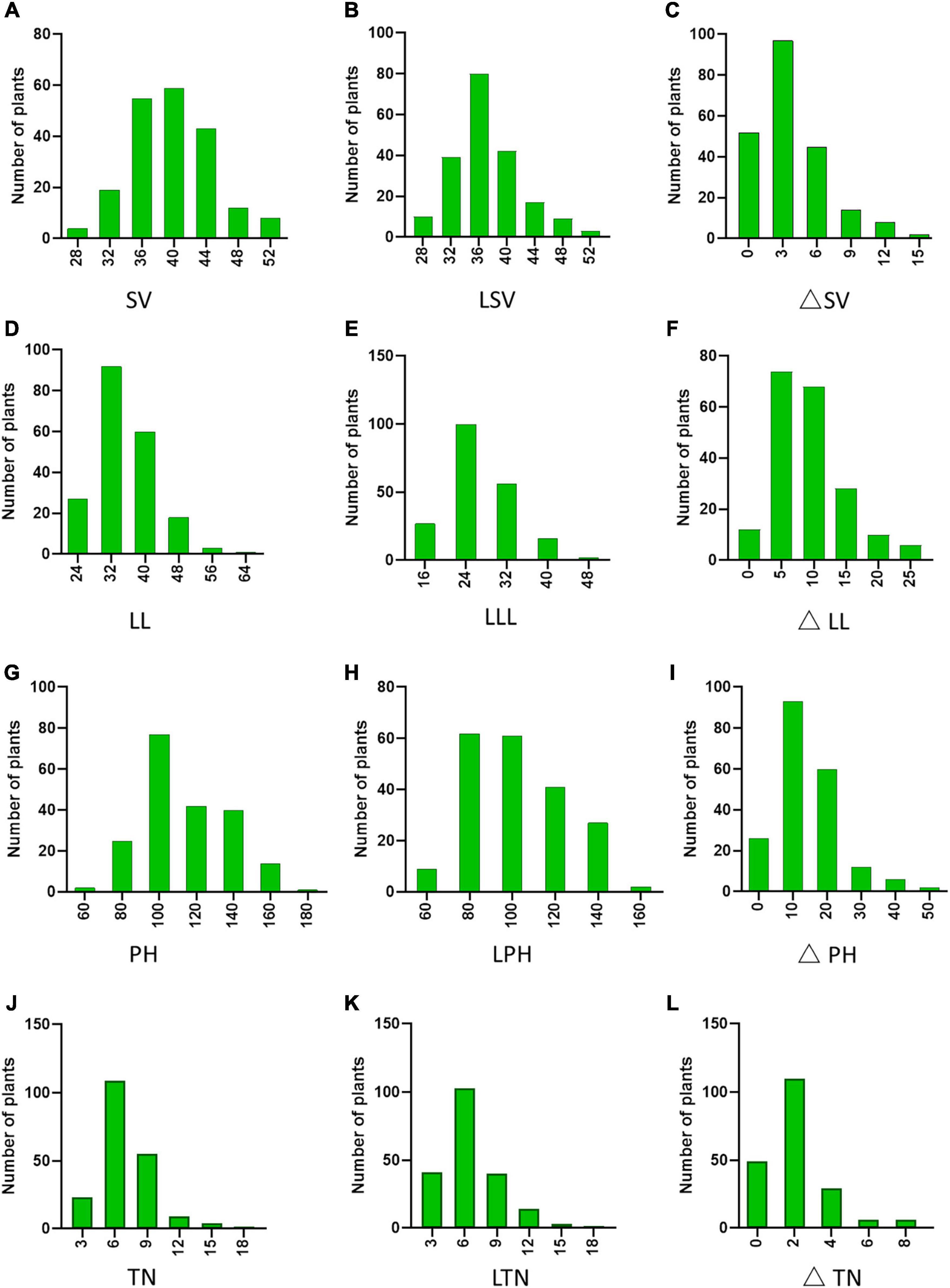
Figure 2. Phenotypic diversity of 225 rice accessions: (A) SV, (D) LL, (G) PH, and (J) TN at NN level. (B) SV, (E) LL, (H) PH, and (K) TN at LN level. Phenotypic difference (△NN-LN) of (C) SV, (F) LL, (I) PH, and (L) TN.
Phylogenetic Analyses and Single-Nucleotide Polymorphisms
We planted 225 natural rice varieties in NN (180 kg⋅hm–2) and LN (90 kg⋅hm–2) fields at CNRRI. Based on the genetic distance, we further identified neighbor-joining clustering and divided 225 varieties into 4 main rice subpopulations (Figure 3). The structural analysis showed that there are indica, japonica, Aus, and Intermediate-type varieties distributed in each subpopulation. The 225 varieties with NUE-related phenotypes originated from 5 geographic regions (Supplementary Figure 1). Varieties in Asia accounted for 85.71%, followed by America 4.15%, Europe 3.69%, Africa 3.69%, and Oceania 2.76% (Supplementary Figure 2).
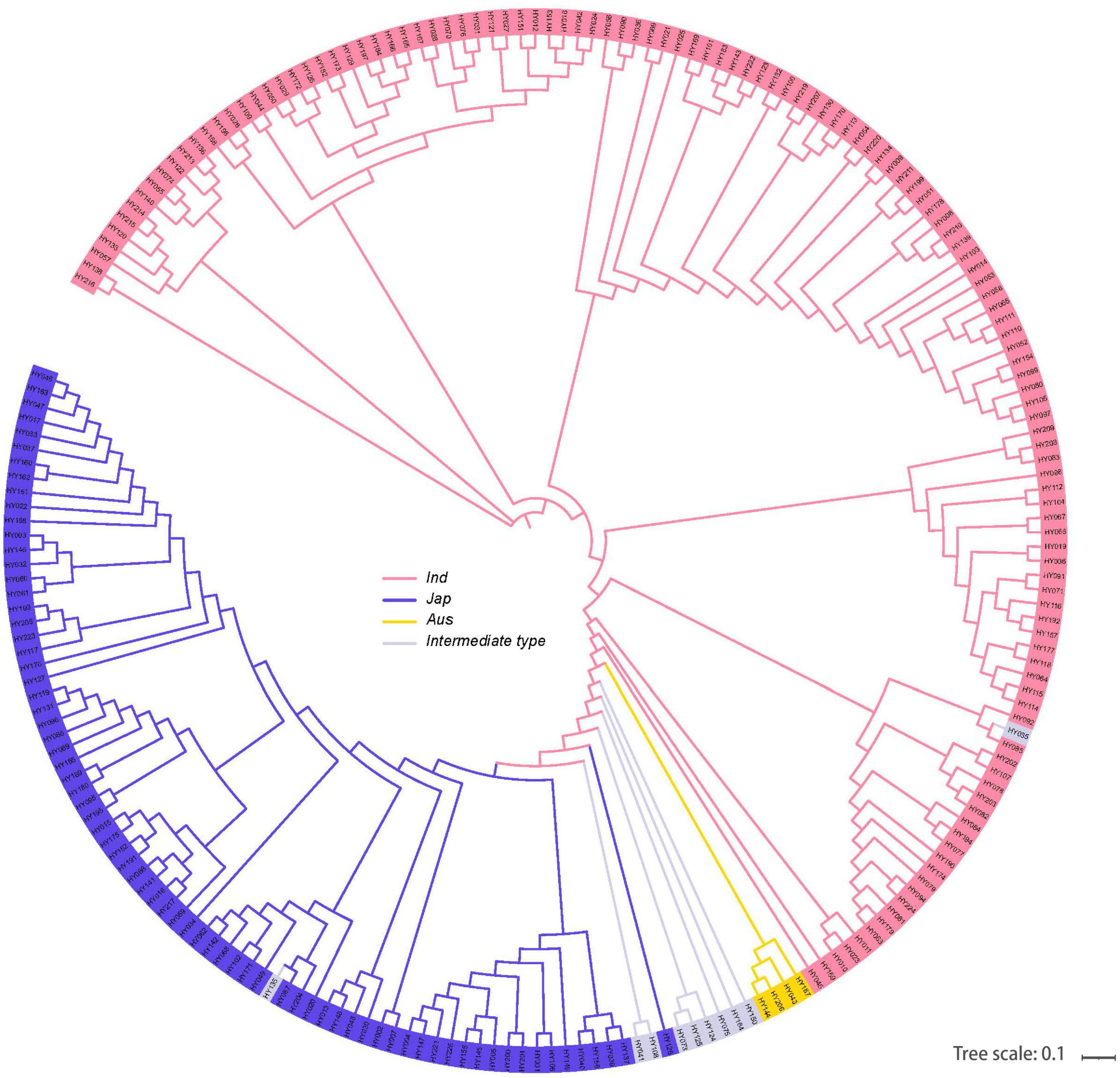
Figure 3. Phylogram of 225 natural varieties showing the divergence. Four main rice subpopulations indicated with different colors: O. indica, O. japonica, Aus, and Intermediate types.
Genome-Wide Association Study and Candidate Analysis
We further performed GWAS on the difference of four LNGR agronomic traits under NN and LN levels to identify significantly associated genes (Figure 4). We selected the SNPs from the Rice 3K Sequencing Project that filtered a missing rate < 0.4 and a MAF > 0.05. We finally obtained 111,205 SNPs and performed an association analysis covering the entire genome with population structure corrections. It is generally considered that the SNP significant threshold is P ≤ 10–5, and linkage disequilibrium is 100 kb, as the literature queries showed (Lv et al., 2019).
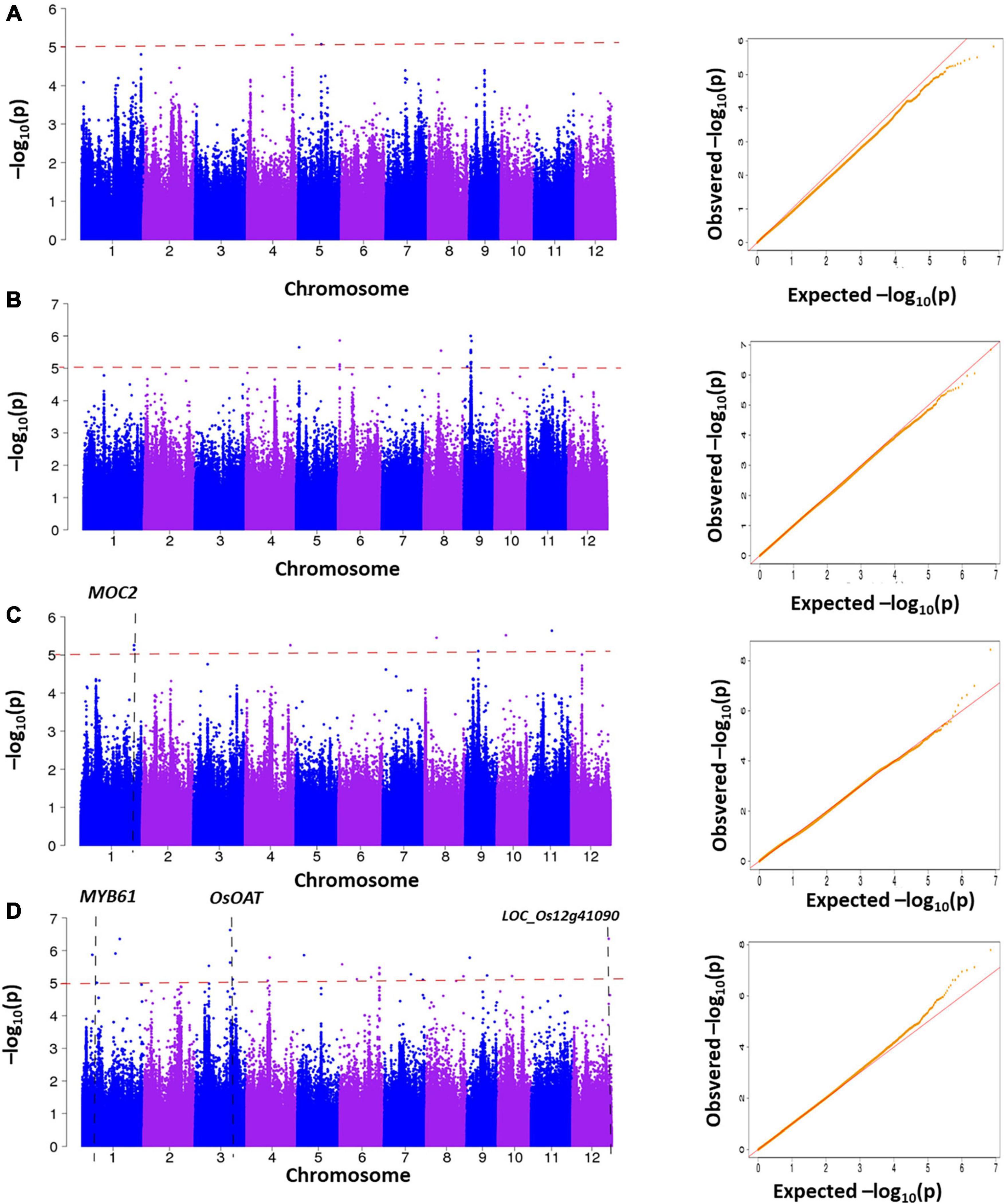
Figure 4. Manhattan plots generated from the genome-wide association study (GWAS) analysis were derived from the trait differences (△NN-LN) of NUE-related agronomic traits. (A) △SV, (B) △LL, (C) △PH, and (D) △TN.
The genomic positions of known NUE-related functional genes with the GWAS intervals obtained in this study were compared. A total of 4 NUE-related candidate genes were detected after estimating 56 significantly associated SNPs distributed on the whole genome in 225 rice varieties. Notably, 2, 8, 20, and 26 significantly associated SNPs were obtained for △SV, △PH, △LL, and △TN, respectively, and each candidate region of the significant SNPs spanned 200 kb (i.e., 100 kb upstream and 100 kb downstream) (Figure 5 and Supplementary Table 2).
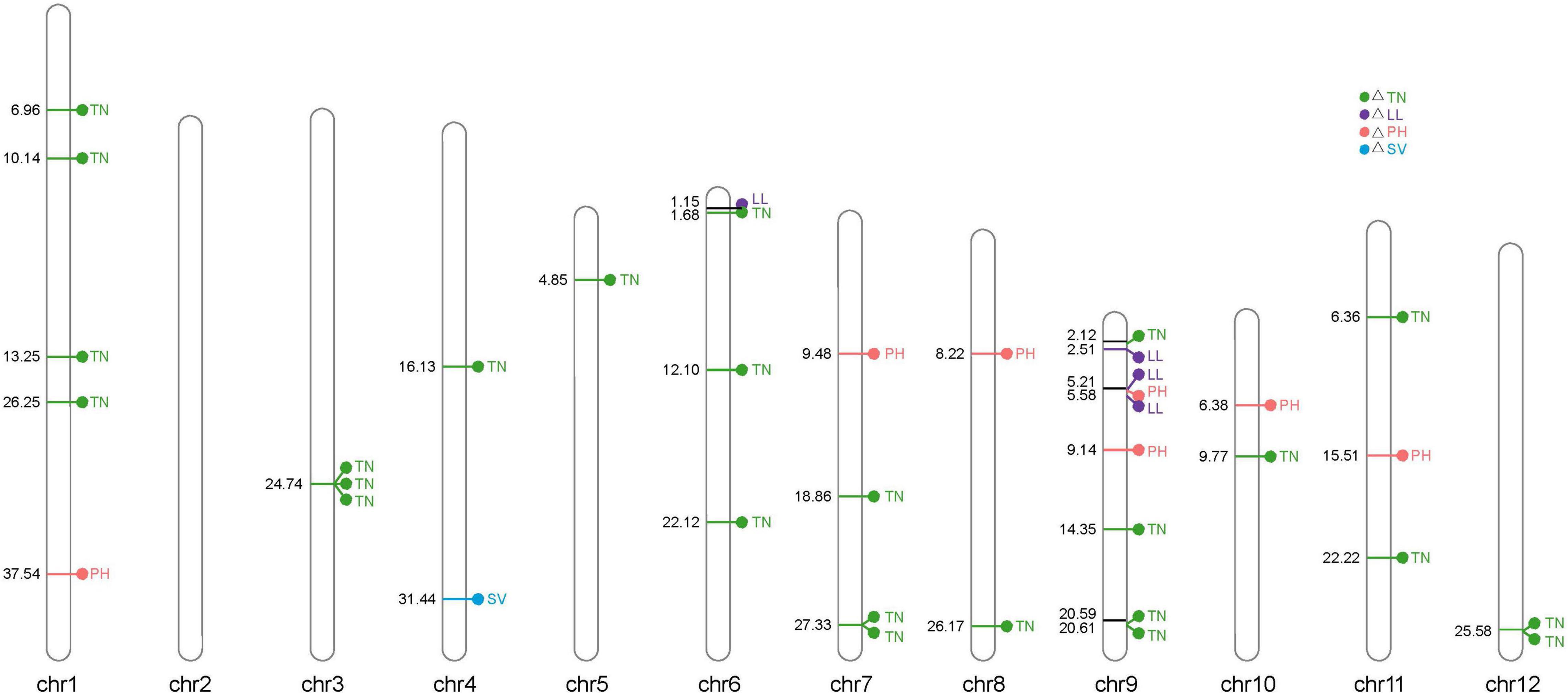
Figure 5. Distribution of 56 significantly associated single-nucleotide polymorphisms (SNPs) on 12 chromosomes based on the physical distance from the four NUE-related agronomic trait differences. The numbers on the left side of each column represent the physical location (Mb) of each lead SNP. Letters to the right of each column represent the corresponding traits of △SV, △LL, △PH, and △TN.
Using the previously reported genes and published studies regarding nitrogen as references, altogether, three LN-associated candidate genes corresponding to the significantly associated SNPs were commonly detected in our study (Table 3). In this study, MYB61 was detected in the candidate interval of Chr01_10141302, LOC_Os01g18240, which is a well-known gene-modulating N use and C metabolism between rice indica and japonica subspecies. As a transcriptional factor, MYB61 is directly regulated by a known NUE regulator, GRF4, which coordinates cellulosic biomass production and N utilization (Gao Y. et al., 2020).
The candidate gene of OsOAT, LOC_Os03g44150, was located at the candidate interval of Chr03_24739537. OsOAT (i.e., ornithine δ-aminotransferase) plays a critical role in connecting arginine cycling and proline cycling and in contributing to floret development and seed setting in rice (Liu C. et al., 2018). On chromosome 1, we identified a candidate gene, MOC2, at the candidate interval of Chr01_37543388, whose mutants display significantly reduced TN, a reduced growth rate, and a consequent dwarf phenotype (Koumoto et al., 2013). We found that the typical N-related phenotypes changed, and therefore, we considered it to be a candidate gene for the subsequent haplotype analysis.
Haplotype Analysis and Gene Mining
To further study the role of candidate genes, we conducted the haplotype analyses on the 225 rice varieties to identify the elite haplotypes. We first analyzed the sequences of MYB61 in the 225 varieties. Based on the haplotype analysis and comparative analysis of the corresponding phenotypic data, all varieties possessed five types of haplotypes that play important roles in response to LN stress (Figures 6A,D). The varieties of MYB61Hap⋅5 contained CTT sequences, especially Menjiagao_1 (HY192) and Xiangaizao_10hao (HY197), and the phenotypic differences in the four traits were significantly or extremely significantly lower than others. We presumed that base differences affected the gene expression of MYB61, and MYB61Hap⋅5 performed more optimally in increasing the nitrogen utilization efficiency as an elite haplotype.
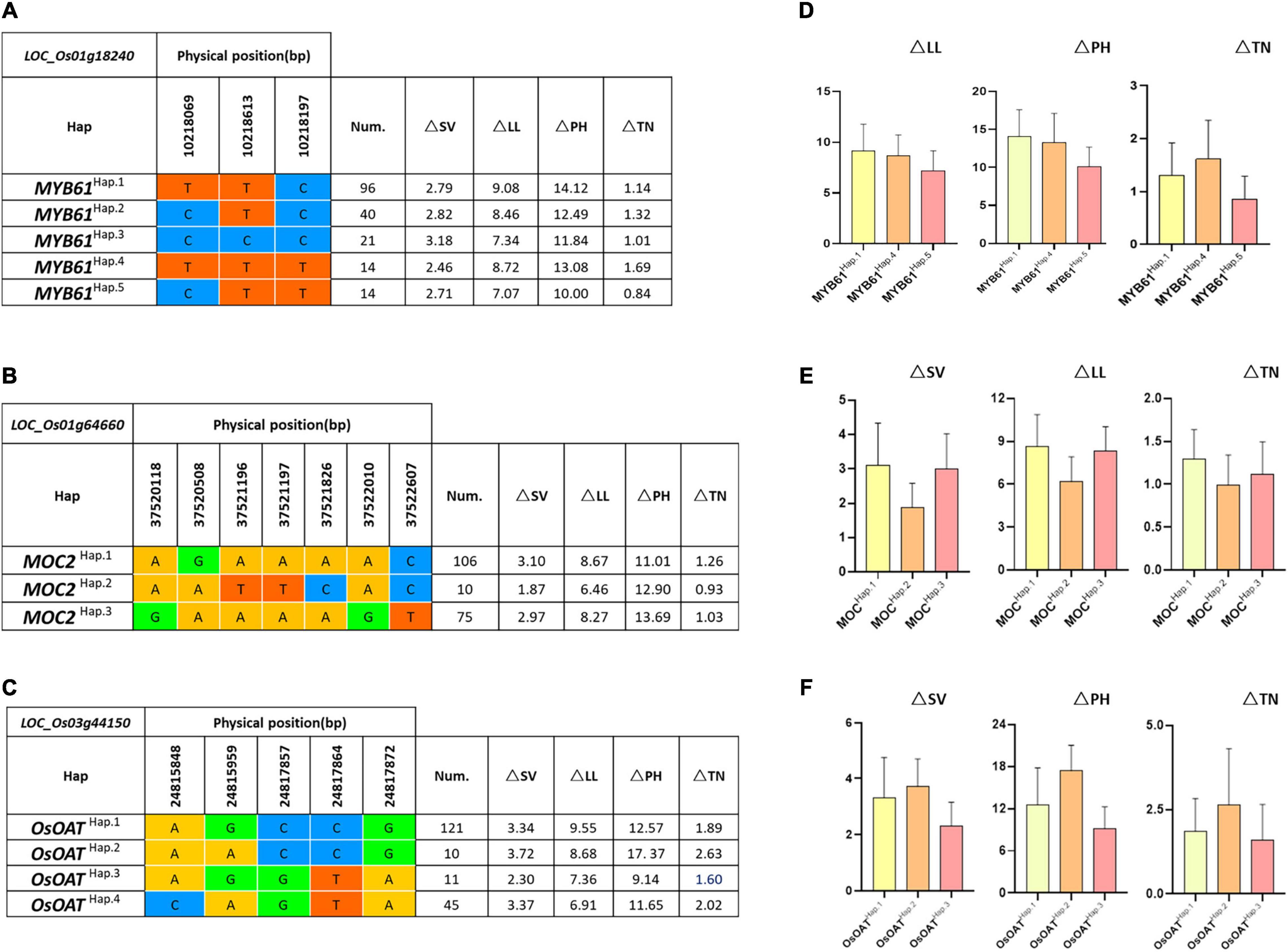
Figure 6. Haplotype analysis of known genes MYB61, MOC2, and OsOAT. Gene structures (left) and the mean amount on △SV, △LL, △PH, and △TN of different haplotypes (right) of (A,D) MYB61 (LOC_Os01g18240), (B,E) MOC2 (LOC_Os01g64660), and (C,F) OsOAT (LOC_Os03g44150). Num, number of accessions.
As the literature queries showed, MOC2 is involved in N-related agronomic trait regulation. Importantly, MOC2 has not been reported participating in N assimilation or utilization. The haplotype analysis (Figures 6B,E) indicates that out of 225 varieties, there are a total of 3 haplotypes at the MOC2 locus. Accessions with MOC2Hap⋅2 showed minimal differences in SV, LL, and TN under NN or LN (i.e., △SV, △LL, and △TN). Thus, we speculated that MOC2Hap⋅2 may be associated with the NUE regulation.
Moreover, on chromosome 3, the candidate gene of OsOAT was predicted to encode ornithine δ-aminotransferase. Through published studies, we found that OsOAT regulates the N-related function of rice. Our results revealed that the accessions with OsOATHap⋅3 exhibit a lower difference of SV, PH, and TN (i.e., △SV, △PH, and △TN). The haplotype analysis suggests that OsOATHap⋅3 is strongly associated with NUE in rice (Figures 6C,F).
As expected, in addition to the published genes described in the sections earlier, several other genes encoding N-related protein were worthy of mining in our study. In this report, it was described that OsCPK12 encodes calcium-dependent protein kinase and also participates in the signal transduction pathway in the LN stress response. Thus, it may be useful to include it when engineering crop plants with improved tolerance to LN levels (Takayuki et al., 2010). In our association panel, LOC_Os12g41090 was located at the candidate interval of Chr12_25582237. It was annotated to associate with calcium/calmodulin-dependent protein kinases. Based on the gene annotation and the publicly available literature, we deduced that LOC_Os12g41090 was a candidate gene involved in the NUE regulation.
Discussion
Nitrogen (N) is an indispensable element for crop production. Excessive chemical nitrogen fertilizer is applied to obtain high crop yields (Krapp, 2015; Chen et al., 2016). The sequence-based GWAS is a highly efficient approach for analyzing rice agronomic traits, and it can identify the elite genes and can evaluate rice core accessions to assist in specifically improving the usage of nitrogen. In this study, we collected 225 worldwide rice germplasms and performed GWAS on the trait differences (△NN-LN) of NUE-related agronomic traits. More importantly, compared with the hydroponic or seedling stage phenotyping experiment, our study was completed in the field under two different N levels (NN, 180 kg⋅hm–2; LN, 90 kg⋅hm–2) until maturity. The results obtained exhibited meaning and persuasiveness.
Plant NUE is an inherently complex trait (Masclaux-Daubresse et al., 2010; Gao Z. et al., 2019; Gao H. et al., 2020). At present, some studies exist that focus on NUE in rice (Hu et al., 2015; Wang et al., 2018b; Zhang S. et al., 2021). However, the studies on NUE by the GWAS analysis are limited, and to the best of our knowledge, few studies have been previously performed. In rice, a NIN-like protein, OsNLP4, was associated with NUE and was identified through GWAS in rice core populations. The previous studies showed that OsNLP4 imparts nitrogen assimilation efficiency and promotes the number of tillers and yield of rice (Yu et al., 2021). Additionally, OsNPF6.1HapB is an elite haplotype derived from the GWAS analysis of extreme nitrogen-related phenotypes, such as PH, effective panicle number (EPN), and yield per plant (YPP) (Tang et al., 2019).
We measured the LN-related agronomic traits of SV, LL, PH, and TN of the 225 rice varieties under a NN level and an LN level. There was a correlation between different LN-related traits under NN and LN levels, suggesting that there were close physiological bases among different traits (Xu et al., 2012). We, therefore, focused on the difference (△NN-LN) between the phenotypic value of NN and LN and divided the varieties into hyposensitive and sensitive varieties. The critical first step before initiating association mapping is to collect a wide range of germplasm resources with different genetic backgrounds (Zheng M. et al., 2017). Our selection of 225 rice germplasms covered 5 continents around the world, which indicated that the results for genetic diversity were reliable for a feasible GWAS. Furthermore, we selected high-quality SNPs from the Rice 3K Sequencing Project and performed the association analysis on the phenotypic value difference (△NN-LN) covering the entire genome.
Numerous studies have supported the idea that N regulation is involved in plant development (Zhang S. et al., 2020; Zhang M. et al., 2021). Based on the previously reported genes and published studies, we conducted candidate gene identification. More importantly, the reliability of our GWAS for association mapping of NUE was previously confirmed by Gao (Gao Y. et al., 2020), who found that the indica allele of MYB61 exhibited superiority in governing nitrogen use and biomass production. Encouragingly, we also identified the NUE-associated genes OsOAT and MOC2 from our GWAS, and both of them play a critical role in modulating N use. Additionally, we also identified several other genes encoding N-related proteins worthy of mining in our study.
As expected, the gene LOC_Os12g41090, which encodes calcium/calmodulin-dependent protein kinases, displays a signal close to OsCPK12. OsCPK12 itself can participate in the signal transduction pathway in the LN stress response and can potentially improve the NUE of rice. One concern with studying this candidate gene is that the gene function must be verified. We, therefore, have constructed various vectors for transgenic verification. There are multiple haplotypes of genes in different varieties, which cause differences in phenotypic value. MYB61 was classified into five haplotypes based on three missense SNPs in its coding region. MYB61Hap⋅5 was found to be an elite haplotype for its lower phenotypic difference (△NN-LN). MOC2 regulates the N-related function of rice, and further haplotype analysis revealed that MOC2 Hap⋅2 is the elite haplotype that enhances NUE. A previous study suggested that OsOAT has an important role in regulating NUE, and OsOATHap⋅3 was significantly associated with the NUE of rice compared with other haplotypes. Moreover, Menjiagao_1 and Xiangaizao_10hao with MYB61Hap⋅5 were identified as promising candidate rice accessions in NUE under LN-induced phenotypic data, which have shown an insignificant phenotypic difference (△NN-LN).
Different varieties responded differently to N levels, and the physiological differences may be caused by genotype and N interactions (Watanabe and Inubushl, 1986; He et al., 2020). The analysis of agronomic traits for two different N levels suggests a critical role for NUE. We successfully deduced that four candidate genes are involved in N regulation, and these were consistent with what currently appears in the available literature. Importantly, our research provides a theoretical basis for the selection and breeding of NUE genes and materials.
Data Availability Statement
The original contributions presented in the study are included in the article/Supplementary Material, further inquiries can be directed to the corresponding author/s.
Author Contributions
YL, LG, QQ, and LS conceived and designed the study. LS and QW performed GWAS. YW and XL provided advice for the experimental design. YL, JM, HH, and YW performed the phenotyping measurement. YL, JM, and YW performed the data analysis. YL wrote the manuscript. All authors have read the manuscript and approved it for publication.
Funding
This study was supported by the Shenzhen Science and Technology Program (Grant No. KQTD2016113010482651) and the Guangdong Basic and Applied Basic Research Foundation (Grant Nos. 2019A1515110557 and 2020B1515120086).
Conflict of Interest
The authors declare that the research was conducted in the absence of any commercial or financial relationships that could be construed as a potential conflict of interest.
Publisher’s Note
All claims expressed in this article are solely those of the authors and do not necessarily represent those of their affiliated organizations, or those of the publisher, the editors and the reviewers. Any product that may be evaluated in this article, or claim that may be made by its manufacturer, is not guaranteed or endorsed by the publisher.
Supplementary Material
The Supplementary Material for this article can be found online at: https://www.frontiersin.org/articles/10.3389/fpls.2021.770736/full#supplementary-material
Footnotes
References
Alexander, D., and Lange, K. (2011). Enhancements to the ADMIXTURE algorithm for individual ancestry estimation. BMC Bioinformatics 12:246. doi: 10.1186/1471-2105-12-246
Chen, J., Zhang, Y., Tan, Y., Zhang, M., Zhu, L., Xu, G., et al. (2016). Agronomic nitrogen-use efficiency of rice can be increased by driving OsNRT2.1 expression with the OsNAR2.1 promoter. Plant Biotechnol. J. 14, 1705–1715. doi: 10.1111/pbi.12531
Du, Q.-G., Yang, J., Syed Muhammad Sadiq, S., Yang, R.-X., Yu, J.-J., and Li, W.-X. (2021). Comparative transcriptome analysis of different nitrogen responses in low-nitrogen sensitive and tolerant maize genotypes. J. Integr. Agric. 20, 2043–2055. doi: 10.1016/S2095-3119(20)63220-8
Fan, X., Naz, M., Fan, X., Xuan, W., Miller, A. J., and Xu, G. (2017). Plant nitrate transporters: from gene function to application. J. Exp. Bot. 68, 2463–2475. doi: 10.1093/jxb/erx011
Fan, X. R., Feng, H. M., Tan, Y. W., Xu, Y. L., Miao, Q. S., and Xu, G. H. (2016). A putative 6-transmembrane nitrate transporter OsNRT1.1b plays a key role in rice under low nitrogen. J. Integr. Plant Biol. 58, 590–599. doi: 10.1111/jipb.12382
Gao, H., Zhang, C., He, H., Liu, T., Zhang, B., Lin, H., et al. (2020). Loci and alleles for submergence responses revealed by GWAS and transcriptional analysis in rice. Mol. Breed. 40:75. doi: 10.1007/s11032-020-01160-6
Gao, Y., Xu, Z., Zhang, L., Li, S., Wang, S., Yang, H., et al. (2020). MYB61 is regulated by GRF4 and promotes nitrogen utilization and biomass production in rice. Nat. Commun. 11:5219. doi: 10.1038/s41467-020-19019-x
Gao, Z., Wang, Y., Chen, G., Zhang, A., Yang, S., Shang, L., et al. (2019). The indica nitrate reductase gene OsNR2 allele enhances rice yield potential and nitrogen use efficiency. Nat. Commun. 10:5207. doi: 10.1038/s41467-019-13110-8
Good, A. G., Shrawat, A. K., and Muench, D. G. (2004). Can less yield more? Is reducing nutrient input into the environment compatible with maintaining crop production? Trends Plant Sci. 9, 597–605. doi: 10.1016/j.tplants.2004.10.008
He, K., Xu, S., Zhang, X., Li, Y., and Xue, J. (2020). Mining of candidate genes for nitrogen use efficiency in maize based on genome-wide association study. Mol. Breed. 40:83. doi: 10.1007/s11032-020-01163-3
Hu, B., Wang, W., Ou, S., Tang, J., Li, H., Che, R., et al. (2015). Variation in NRT1.1B contributes to nitrate-use divergence between rice subspecies. Nat. Genet. 47, 834–838. doi: 10.1038/ng.3337
Ju, X. T., Xing, G. X., Chen, X. P., Zhang, S. L., Zhang, L. J., Liu, X. J., et al. (2009). Reducing environmental risk by improving N management in intensive Chinese agricultural systems. Proc. Natl. Acad. Sci. U.S.A. 106, 3041–3046. doi: 10.1073/pnas.0813417106
Kang, H. M., Sul, J. H., Service, S. K., Zaitlen, N. A., Kong, S. Y., Freimer, N. B., et al. (2010). Variance component model to account for sample structure in genome-wide association studies. Nat. Genet. 42, 348–354. doi: 10.1038/ng.548
Koumoto, T., Shimada, H., Kusano, H., She, K.-C., Iwamoto, M., and Takano, M. (2013). Rice monoculm mutation moc2, which inhibits outgrowth of the second tillers, is ascribed to lack of a fructose-1,6-bisphosphatase. Plant Biotechnol. 30, 47–56. doi: 10.5511/plantbiotechnology.12.1210a
Krapp, A. (2015). Plant nitrogen assimilation and its regulation: a complex puzzle with missing pieces. Curr. Opin. Plant Biol. 25, 115–122. doi: 10.1016/j.pbi.2015.05.010
Li, S., Tian, Y., Wu, K., Ye, Y., Yu, J., Zhang, J., et al. (2018). Modulating plant growth–metabolism coordination for sustainable agriculture. Nature 560, 595–600. doi: 10.1038/s41586-018-0415-5
Liu, C., Xue, Z., Tang, D., Shen, Y., Shi, W., Ren, L., et al. (2018). Ornithine delta-aminotransferase is critical for floret development and seed setting through mediating nitrogen reutilization in rice. Plant J. 96, 842–854. doi: 10.1111/tpj.14072
Liu, K., and Muse, S. V. (2005). PowerMarker: an integrated analysis envirosnment for genetic marker analysis. Bioinformatics 21, 2128–2129. doi: 10.1093/bioinformatics/bti282
Liu, N., Xue, Y., Guo, Z., Li, W., and Tang, J. (2016). Genome-wide association study identifies candidate genes for starch content regulation in maize kernels. Front. Plant Sci. 7:1046. doi: 10.3389/fpls.2016.01046
Liu, Y., Wang, H., Jiang, Z., Wang, W., Xu, R., Wang, Q., et al. (2021). Genomic basis of geographical adaptation to soil nitrogen in rice. Nature 590, 600–605. doi: 10.1038/s41586-020-03091-w
Liu, Z., Zhu, C., Jiang, Y., Tian, Y., Yu, J., An, H., et al. (2016). Association mapping and genetic dissection of nitrogen use efficiency-related traits in rice (Oryza sativa L.). Function. Integr. Genom. 16, 323–333. doi: 10.1007/s10142-016-0486-z
Lv, Y., Wang, Y., Noushin, J., Hu, H., Chen, P., Shang, L., et al. (2019). Genome-wide association analysis and allelic mining of grain shape-related traits in rice. Rice Sci. 26, 384–392. doi: 10.1016/j.rsci.2018.09.002
Masclaux-Daubresse, C., Daniel-Vedele, F., Dechorgnat, J., Chardon, F., Gaufichon, L., and Suzuki, A. (2010). Nitrogen uptake, assimilation, and remobilization in plants: challenges for sustainable and productive agriculture. Ann. Bot. 105, 1141–1157. doi: 10.1093/aob/mcq028
McAllister, C. H., Beatty, P. H., and Good, A. G. (2012). Engineering nitrogen use efficient crop plants: the current status. Plant Biotechnol. J. 10, 1011–1025. doi: 10.1111/j.1467-7652.2012.00700.x
Saito, H., Okumoto, Y., Tsukiyama, T., Xu, C., Teraishi, M., and Tanisaka, T. (2019). Allelic differentiation at the E1/Ghd7 locus has allowed expansion of rice cultivation area. Plants 8:550. doi: 10.3390/plants8120550
Segura, V., Vilhjálmsson, B., Platt, A., Korte, A., Seren, U., Long, Q., et al. (2013). An efficient multi-locus mixed-model approach for genome-wide association studies in structured populations. Nat. Genet. 44, 825–830. doi: 10.1038/ng.2314
Sun, H., Qian, Q., Wu, K., Luo, J., Wang, S., Zhang, C., et al. (2014). Heterotrimeric G proteins regulate nitrogen-use efficiency in rice. Nat. Genet. 46, 652–656. doi: 10.1038/ng.2958
Sun, S., Wang, L., Mao, H., Shao, L., Li, X., Xiao, J., et al. (2018). A G-protein pathway determines grain size in rice. Nat. Commun. 9:851. doi: 10.1038/s41467-018-03141-y
Takayuki, A., Masataka, W., Naohiro, A., Setsuko, K., Hiroaki, I., Hirohiko, Hi., et al. (2010). Overexpression of a calcium-dependent protein kinase gene enhances growth of rice under low-nitrogen conditions. Plant Biotechnol. 27, 369–373. doi: 10.5511/plantbiotechnology.27.369
Tang, W., Ye, J., Yao, X., Zhao, P., Xuan, W., Tian, Y., et al. (2019). Genome-wide associated study identifies NAC42-activated nitrate transporter conferring high nitrogen use efficiency in rice. Nat. Commun. 10:5279. doi: 10.1038/s41467-019-13187-1
Wang, Q., Nian, J., Xie, X., Yu, H., Zhang, J., Bai, J., et al. (2018a). Genetic variations in ARE1 mediate grain yield by modulating nitrogen utilization in rice. Nat. Commun. 9:735. doi: 10.1038/s41467-017-02781-w
Wang, W., Mauleon, R., Hu, Z., Chebotarov, D., Tai, S., Wu, Z., et al. (2018c). Genomic variation in 3,010 diverse accessions of Asian cultivated rice. Nature 557, 43–49. doi: 10.1038/s41586-018-0063-9
Wang, W., Hu, B., Yuan, D., Liu, Y., Che, R., Hu, Y., et al. (2018b). Expression of the nitrate transporter gene OsNRT1.1A/OsNPF6.3 confers high yield and early maturation in rice. Plant Cell 30, 638–651. doi: 10.1105/tpc.17.00809
Wang, Q., Su, Q., Nian, J., Zhang, J., Guo, M., Dong, G., et al. (2021). The Ghd7 transcription factor represses ARE1 expression to enhance nitrogen utilization and grain yield in rice. Mol. Plant 14, 1012–1023. doi: 10.1016/j.molp.2021.04.012
Wang, Y. Y., Hsu, P. K., and Tsay, Y. F. (2012). Uptake, allocation and signaling of nitrate. Trends Plant Sci. 17, 458–467. doi: 10.1016/j.tplants.2012.04.006
Watanabe, I., and Inubushl, K. (1986). Dynamics of available nitrogen in paddy soils: I. changes in available n during rice cultivation and origin of N. Soil Sci. Plant Nutr. 32, 37–50. doi: 10.1080/00380768.1986.10557479
Wei, Z., Yuan, Q., Lin, H., Li, X., Zhang, C., Gao, H., et al. (2021). Linkage analysis, GWAS, transcriptome analysis to identify candidate genes for rice seedlings in response to high temperature stress. BMC Plant Biol. 21:85. doi: 10.1186/s12870-021-02857-2
Xu, G., Fan, X., and Miller, A. J. (2012). Plant nitrogen assimilation and use efficiency. Annu. Rev. Plant Biol. 63, 153–182. doi: 10.1146/annurev-arplant-042811-105532
Yang, J., Jiang, H., Yeh, C. T., Yu, J., Jeddeloh, J. A., Nettleton, D., et al. (2015). Extreme-phenotype genome-wide association study (XP-GWAS): a method for identifying trait-associated variants by sequencing pools of individuals selected from a diversity panel. Plant J. 84, 587–596. doi: 10.1111/tpj.13029
Yu, J., Xuan, W., Tian, Y., Fan, L., Sun, J., Tang, W., et al. (2021). Enhanced OsNLP4-OsNiR cascade confers nitrogen use efficiency by promoting tiller number in rice. Plant Biotechnol. J. 19, 167–176. doi: 10.1111/pbi.13450
Zhang, C., Dong, S., Xu, J., He, W., and Yang, T. (2018). PopLDdecay: a fast and effective tool for linkage disequilibrium decay analysis based on variant call format files. Bioinformatics 10, 1786–1788. doi: 10.1093/bioinformatics/bty875
Zhang, M., Wang, Y., Chen, X., Xu, F., Ding, M., Ye, W., et al. (2021). ATPase overexpression increases rice yield via simultaneous enhancement of nutrient uptake and photosynthesis. Nat. Commun. 12:735. doi: 10.1038/s41467-021-20964-4
Zhang, S., Zhang, Y., Li, K., Yan, M., Zhang, J., Yu, M., et al. (2021). Nitrogen mediates flowering time and nitrogen use efficiency via floral regulators in rice. Curr. Biol. 31, 671.e5–683.e5. doi: 10.1016/j.cub.2020.10.095
Zhang, S., Zhu, L., Shen, C., Ji, Z., and Li, S. (2020). Natural allelic variation in a modulator of auxin homeostasis improves grain yield and nitrogen use efficiency in rice. Plant Cell 33, 566–580. doi: 10.1093/plcell/koaa037
Zhang, X., Davidson, E. A., Mauzerall, D. L., Searchinger, T. D., Dumas, P., and Shen, Y. (2015). Managing nitrogen for sustainable development. Nature 528, 51–59. doi: 10.1038/nature15743
Keywords: rice, GWAS, low-nitrogen-induced growth response, haplotype analysis, gene mining
Citation: Lv Y, Ma J, Wang Y, Wang Q, Lu X, Hu H, Qian Q, Guo L and Shang L (2021) Loci and Natural Alleles for Low-Nitrogen-Induced Growth Response Revealed by the Genome-Wide Association Study Analysis in Rice (Oryza sativa L.). Front. Plant Sci. 12:770736. doi: 10.3389/fpls.2021.770736
Received: 04 September 2021; Accepted: 30 September 2021;
Published: 05 November 2021.
Edited by:
Meixue Zhou, University of Tasmania, AustraliaReviewed by:
Zhong-Hua Chen, Western Sydney University, AustraliaJie Qiu, Shanghai Normal University, China
Copyright © 2021 Lv, Ma, Wang, Wang, Lu, Hu, Qian, Guo and Shang. This is an open-access article distributed under the terms of the Creative Commons Attribution License (CC BY). The use, distribution or reproduction in other forums is permitted, provided the original author(s) and the copyright owner(s) are credited and that the original publication in this journal is cited, in accordance with accepted academic practice. No use, distribution or reproduction is permitted which does not comply with these terms.
*Correspondence: Longbiao Guo, guolongbiao@caas.cn; Lianguang Shang, shanglianguang@163.com
†These authors have contributed equally to this work