- 1Molecular Biology and Genetic Engineering Center (CBMEG), University of Campinas (UNICAMP), Campinas, Brazil
- 2Center of Rubber Tree and Agroforestry Systems, Agronomic Institute (IAC), Votuporanga, Brazil
- 3Centre de Coopération Internationale en Recherche Agronomique pour le Développement (CIRAD), UMR AGAP, Montpellier, France
- 4AGAP, Univ Montpellier, CIRAD, INRAE, Institut Agro, Montpellier, France
- 5Department of Genetics, Luiz de Queiroz College of Agriculture (ESALQ), University of São Paulo (USP), Piracicaba, Brazil
- 6São Francisco University (USF), Itatiba, Brazil
- 7Department of Plant Biology, Biology Institute, University of Campinas (UNICAMP), Campinas, Brazil
Hevea brasiliensis (rubber tree) is a large tree species of the Euphorbiaceae family with inestimable economic importance. Rubber tree breeding programs currently aim to improve growth and production, and the use of early genotype selection technologies can accelerate such processes, mainly with the incorporation of genomic tools, such as marker-assisted selection (MAS). However, few quantitative trait loci (QTLs) have been used successfully in MAS for complex characteristics. Recent research shows the efficiency of genome-wide association studies (GWAS) for locating QTL regions in different populations. In this way, the integration of GWAS, RNA-sequencing (RNA-Seq) methodologies, coexpression networks and enzyme networks can provide a better understanding of the molecular relationships involved in the definition of the phenotypes of interest, supplying research support for the development of appropriate genomic based strategies for breeding. In this context, this work presents the potential of using combined multiomics to decipher the mechanisms of genotype and phenotype associations involved in the growth of rubber trees. Using GWAS from a genotyping-by-sequencing (GBS) Hevea population, we were able to identify molecular markers in QTL regions with a main effect on rubber tree plant growth under constant water stress. The underlying genes were evaluated and incorporated into a gene coexpression network modelled with an assembled RNA-Seq-based transcriptome of the species, where novel gene relationships were estimated and evaluated through in silico methodologies, including an estimated enzymatic network. From all these analyses, we were able to estimate not only the main genes involved in defining the phenotype but also the interactions between a core of genes related to rubber tree growth at the transcriptional and translational levels. This work was the first to integrate multiomics analysis into the in-depth investigation of rubber tree plant growth, producing useful data for future genetic studies in the species and enhancing the efficiency of the species improvement programs.
Introduction
Hevea brasiliensis (rubber tree) is an outbreeding forest species belonging to the Euphorbiaceae family with an inestimable importance in the world economy because it is the only crop capable of producing natural rubber with quantity and quality levels able to meet global demand (De Faÿ and Jacob, 1989). Possessing unique characteristics such as resistance, elasticity and heat dissipation, Hevea rubber is used as a feedstock for more than 40,000 products (Pootakham et al., 2017; Mantello et al., 2019). Although it is very important, H. brasiliensis is still in an early domestication stage due to its long breeding cycle (25–30 years), the large areas required for planting and its recent cultivation (Priyadarshan and Clément-Demange, 2004; Gonçalves et al., 2006). In this context, Hevea breeding programs aim to improve important agronomic traits for rubber fabrication, mainly those related to latex growth and production (Priyadarshan, 2003). The use of early genotype selection technologies has been proposed as a breeding alternative for accelerating this process, e.g., incorporating genomic tools for marker-assisted selection (MAS; Pootakham et al., 2017; Priyadarshan, 2017). Although the discovery of quantitative trait loci (QTLs) can benefit Hevea breeding programs (Souza et al., 2019), this characterization is hindered by the large number of genes and molecular interactions controlling such characteristics (Pootakham et al., 2020). To date, few QTLs have been successfully used for rubber tree MAS for complex quantitative traits due to the insufficient quantity of linked markers in the QTLs, small QTL effects on the phenotype, or strong environmental influences (Nguyen et al., 2019).
Several studies have been carried out in the last decade to identify QTLs in H. brasiliensis through genetic linkage maps (Souza et al., 2013; Pootakham et al., 2015; Conson et al., 2018; Rosa et al., 2018; Xia et al., 2018) and association mapping (Chanroj et al., 2017). Genome-wide association studies (GWAS) are important tools for the identification of candidate genetic variants underlying QTLs, with great potential to be incorporated into MAS. Compared to linkage maps, the use of GWAS methodologies has advantages such as using genetically diverse populations with different rates of recombination and linkage disequilibrium (LD; Myles et al., 2009). Despite the observed GWAS efficiency in several crops (Warraich et al., 2020; Zhang et al., 2020; Verzegnazzi et al., 2021), this methodology still presents limitations related to the low proportion of phenotypic variance explained by the identified genomic regions (Manolio et al., 2009). As an alternative, the combination of GWAS results with other molecular methodologies, such as transcriptomics and proteomics analyses, can contribute to better knowledge of the genetic mechanisms involved in the definition of a trait (Tam et al., 2019), overcoming the statistical limitations on the characterization of a broad set of causal genomic regions.
Although the identification of genes with a great phenotypic effect is consolidated with GWAS methodologies (Nebel et al., 2011), there are no established methods for investigating the complete set of genes controlling complex traits through multiomics approaches, and such characterization is an open scientific challenge, especially in crops with complex genomes such as rubber trees (Schaefer et al., 2018). Different initiatives have associated GWAS results with RNA-Seq data (Yan et al., 2020), linking causal genes relevant to the observed phenotypic variation with cell transcription activity profiles (Schaefer et al., 2018; Nguyen et al., 2019). In Hevea, however, RNA-Seq-based studies have been mainly performed to investigate differentially expressed genes (DEGs) under different environmental or stress conditions and profiling rubber tree samples (Hurtado Páez et al., 2015; Sathik et al., 2018; Mantello et al., 2019; Ding et al., 2020). Although the integration of GWAS with RNA-Seq methodologies has proven to provide a deeper comprehension of the genetic relationships involved in trait definition, there is no study, to date, aggregating such data in Hevea.
We are currently undergoing a major revolution in omics sciences (genomics, transcriptomics, proteomics, and phenomics) with different methods for data integration enabling important advances in all phases of genetic improvement, ranging from the discovery of new variants to the understanding of important metabolic pathways (Scossa et al., 2021). The integration of data derived from multiomics can be combined to reveal, in a profound way, the relationships that represent the true biological meaning of the studied elements (Jamil et al., 2020; Wu et al., 2020b). This approach has become increasingly common in humans (Wu et al., 2018), animals (Fonseca et al., 2018), microorganisms (Wang et al., 2019), and combinations of species (Pinu et al., 2019). However, for plants, such integrated methodologies are still a great challenge, especially for nonmodel species with elevated genetic diversity and complex genomes (Jamil et al., 2020), which is the case for H. brasiliensis (Tang et al., 2016; Liu et al., 2020c, Wu et al., 2020a). Despite its economic importance, no study incorporating multiomics has been carried out on H. brasiliensis. With the wide availability of omics data, coexpression networks have become a tool with great potential for inferring gene interactions, mainly based on regulatory and structural relationships, allowing for a broader understanding of unknown molecular mechanisms (Rao and Dixon, 2019). The identification of these genes also allows us to indirectly assess, through their enzymes, the global metabolic relationships involved in defining the evaluated characteristic (Pérez-Bercoff et al., 2011). In this way, we can make use of GWAS to select genes of great importance for the phenotype of interest. Such genes can be used as a guide to select modules of coexpressed genes and their enzymes, which may have minor effects on the phenotype but may be important to maintaining heritability.
In this context, this work presents for the first time a combination of omics data to determine the molecular mechanisms involved in rubber tree growth. For this task, we used a breeding population to infer QTLs using a GWAS approach. These results were incorporated into network analyzes based on RNA-Seq and enzymatic networks. By using this multiomics framework, our study supplies important cues on the interconnection of the metabolic mechanisms of rubber tree growth, providing novel growth-associated genes for future research on increasing Hevea production.
Materials and Methods
According to the analysis workflow performed, different molecular layers were investigated in this work (Figure 1). The study started with the identification of the SNPs with the greatest effect on stem diameter (SD) through a GWAS. After selecting these markers, the markers that presented a significant correlation were selected. This entire set of markers was annotated using a transcriptome assembled on the basis of two commercial genotypes that have been widely used in the genetic improvement of the species. Additionally, a weighted gene coexpression network was constructed, from which it is possible to select the functional modules containing the genes identified by the GWAS. An enzymatic network was also built based on the annotation of genes present in the functional modules selected, which supplied insights into the interaction of these enzymes with the studied phenotype.
Plant Material
For this work, we employed a population composed of four test clones (GT1, PB235, RRIM701, and RRIM600) and individuals from crosses between PR255 x PB217 (251 samples), GT1 x RRIM701 (143 samples) and GT1 x PB235 (40 samples; Souza et al., 2013, 2019; Conson et al., 2018; Rosa et al., 2018). The PR255 genotype was selected because of its early growth and high yield, as well as for being vigorous with stable latex production throughout life (Souza et al., 2013). In contrast, the PB217 genotype presents slow growth but has a rapid increase in latex production in its early years and great potential for long-term performance and yield (Souza et al., 2013; Rosa et al., 2018). These genotypes were planted in random blocks, with four replications of the same genotype grafted on the same plot. This plantation is located in Itiquira, Mato Grosso (MT), Brazil (17° 24′03″ S and 54° 44′53″ W). The GT1 genotype was selected because it is a sterile male and is classified as a primary clone that is tolerant to wind and cold (Shearman et al., 2014). The RRIM701 clone shows vigorous growth and a SD increase after the initial cut (Romain and Thierry, 2011). PB235 has been shown to be a high-yield genotype but is susceptible to panel dryness (Sivakumaran et al., 1988). These two populations (GT1 x RRIM 701 and GT1 x PB 235) were planted in an augmented block design that was repeated in four blocks containing two plants of the same genotype per plot with 4 meters of spacing between them. These populations were planted at the Center for Rubber and Agroforestry Systems/Instituto Agronômico (IAC; 20° 25′00″ S and 49° 59′00″ W) in the northwest region of the state of São Paulo (SP), Brazil. All of these genotypes are widely employed in commercial production and used in Brazilian breeding programs, representing the main rubber tree genetic sources in Latin America. Crossing was carried out via open pollination, and paternity was confirmed using microsatellite markers (SSRs; Souza et al., 2013, Conson et al., 2018).
Phenotypic Analyses
As the main characteristic evaluated in rubber tree genetic breeding (Rao and Kole, 2016), SD was measured in the selected population during the first 4 years of genotype development. Each plant was individually phenotyped (in centimeters) at a height of 50 cm from the soil in two seasons with contrasting average rainfall (low precipitation and high precipitation), which are considered in Hevea studies as contrasting environments (Chanroj et al., 2017; Souza et al., 2019). The variance caused by the genotypic effects was estimated using the best linear unbiased predictor (BLUP) with the breedR package in R (Munõz and Sanchez, 2017). The linear mixed model was as follows:
where y is the vector of the phenotypic measures; is the trait mean; and , , , and are the incidence matrices for the fixed effects of blocks (b), replicates (r), water levels (w) and month of the measurement (m), respectively. and are the incidence matrices of random effects for genotypic effects (g) and genotype x environment interactions (gw), respectively, and is the residual variance. The significance of random effects was estimated by a likelihood ratio test (LRT) with a significance level of 0.05. We estimated the broad heritability ( ) for genotypic means using the following equation:
where is the genotypic variance, is the variance caused by the environment x genotype interaction, s is the number of environments analyzed, is the residual variance and a is the number of blocks.
Genotypic Analyses
The extraction of genomic DNA was performed according to Souza et al. (2013) and Conson et al. (2018). Genotyping-by-sequencing (GBS) libraries were prepared from genomic DNA using the method proposed by Elshire et al. (2011). Initially, the genomic DNA of each sample was digested using the methylation-sensitive enzyme EcoT22I to reduce the genomic complexity. The resulting fragments of each sample were linked to specific barcodes and combined in pools. These fragments were amplified by PCR and sequenced. Sequencing of the PR255 x RRIM217 population was performed using the Illumina HiSeq platform, and sequencing of the GT1 x RRIM701 and GT1 x PB235 populations was performed with the GAIIx platform (Illumina Inc., San Diego, CA, United States). Processing of the GBS data from both experiments was carried out at the same time. SNPs were identified with TASSEL GBS 5 software (Glaubitz et al., 2014) using the following parameters: (i) k-mer size of 64 bp; (ii) minimum read quality (Q) score of 20; and (iii) minimum locus depth of six reads. Reads were aligned with the rubber tree reference genome proposed by Liu et al. (2020a) using Bowtie2 version 2.1 software (Langmead and Salzberg, 2012) with the very sensitive option. We only kept the biallelic markers selected with the VCFtools program (Danecek et al., 2011). Using snpReady software (Granato and Fritsche-Neto, 2018), SNPs with more than 20% missing data and minimum allele frequency (MAF) <0.05 were filtered out. Imputation was performed using the k-nearest neighbor imputation (kNNI) algorithm (Hastie et al., 2001). LD estimations were calculated with the ldsep R package (Gerard, 2020) based on the squared Pearson correlation (R2). For linkage decay investigation, we created a scatter plot of R2 against the chromosomal distances, considering an exponential decay (Tenesa et al., 2004) created with a nonlinear least squares regression model using R software, calculated according to the following equation.
where is the linkage disequilibrium, is the physical distance in bp, is the mean level of disequilibrium for loci at the same location, and is the exponential term.
Genome-Wide Association Studies
GWAS were performed using the Fixed and random model Circulating Probability Unification (FarmCPU) method implemented in the FarmCPU R package (Liu et al., 2016). This method tests the association of markers as fixed and random effects in a mixed linear model in separate steps (Liu et al., 2016). The kinship matrix and the first two principal components (PC1 and PC2) from a principal component analysis (PCA) were used as covariables in the mixed linear model to control the effects caused by the population structure (Challa and Neelapu, 2018). The significance threshold used for the association mapping was calculated based on 30 SD permutations and a 95% quantile value. Additionally, we expanded the set of putatively associated markers through LD. Considering a minimum R2 of 0.7, we created a set of GWAS LD-associated markers (snpsLD), which was used for modeling an LD network with the igraph R package (Csardi and Nepusz, 2006).
Transcriptome
To estimate rubber tree gene expression, RNA-Seq data from RRIM600 and GT1 clones (Mantello et al., 2019) were used. From 6 months of age, these plants were transferred to a growth chamber at a temperature of 28°C with a 12-h photoperiod and were irrigated every 2 days for a period of 10 days. After this period, the plants were subjected to cold stress by changing the chamber temperature to 10°C for 24 h, with the leaf tissues being sampled at 0 h (control), 90 min, 12 and 24 h after exposure to the stress. RNA was extracted from the leaves of three biological replicates using the lithium chloride protocol (Dusotoit-Coucaud et al., 2009). From the total RNA, a cDNA library was built using the TruSeq RNA Sample Preparation Kit (Illumina Inc., San Diego, CA, United States). The 24 samples (three replicates per sample at each time) were randomly pooled (four samples per pool) and grouped using the TruSeq Paired-End Reads Cluster Kit on the cBot platform (Illumina Inc., San Diego, CA, United States). The cDNA libraries were posteriorly sequenced on the Illumina Genome platform Analyzer IIx with a TruSeq kit with 36 cycles (Illumina, San Diego, CA, United States) for 72 bp paired-end reads.
RNA-Seq barcodes were removed from FastQ files using Fastx-Tookit,1 and raw reads were filtered using the program NGS QC Toolkit 2.3 (Trivedi et al., 2014), keeping only sequences with a minimum Q-score of 20 across at least 70% of the sequence length. The filtered sequences were combined with bark reads (Mantello et al., 2014) and mapped to the reference genome of H. brasiliensis (Tang et al., 2016) using the HISAT2 aligner (Kim et al., 2015). The alignment was ordered and assembled using SAMtools (Li et al., 2009) and Trinity (Grabherr et al., 2011) software, respectively. Hevea brasiliensis scaffolds (Tang et al., 2016) were submitted for ab initio annotation using the Maker-P (Campbell et al., 2014) tool. The Trinity assembled transcripts and the Maker-P annotations were combined with nonredundant H. brasiliensis ESTs in the NCBI database (August 2016) and used as a database for aligning assemblies against the H. brasiliensis genome (Tang et al., 2016) with the PASA v2.0 pipeline (Haas et al., 2003) after removing redundant alternate splicing data. The obtained transcripts were filtered with a minimum size of 500 bp and evidence of transcription; we excluded sequences that were only predicted by ab initio genome annotation and with high identity for nonplant transcripts. To estimate the physical position of these sequences across Hevea chromosomes, we performed comparative alignments of these transcripts against the H. brasiliensis genome proposed by Liu et al. (2020a) using BLASTn (Johnson et al., 2008). The annotation of these transcripts was performed using the Trinotate v3.2.1 program (Haas, 2015) and SwissProt database (downloaded in February 2021; Boeckmann et al., 2003).
Gene-Associated Markers
The analysis of candidate genes in QTL regions was performed based on transcript annotations. Candidate genes for the phenotypic variation of GWAS-discovered SNPs were considered by using the first transcripts positioned in the upstream and downstream regions of these markers. In addition to the SNPs significantly associated with the phenotype discovered by the GWAS, which we will call snpsGWAS here, we also searched for candidate genes in the neighboring snpsLD. The GO terms associated with these annotations (snpsGWAS and snpsLD) were investigated using REVIGO (Supek et al., 2011). The genomic regions of the phenotypically associated SNPs discovered in this work were compared with the QTLs discovered by Conson et al. (2018) from the mapping population GT1 x RRIM701. For this analysis, the sequences underlying the QTLs (Conson et al., 2018) were aligned to the reference genome of Liu et al. (2020a) using BLASTn. Alignments with identity above 90% and with the largest coverage area were selected (minimum e-value of e-10). Based on the position of this alignment in relation to the reference genome of Liu et al. (2020a), a representation of the 18 chromosomes of H. brasiliensis was made using snpsGWAS, snpsLD and QTLs (Conson et al., 2018) using the MapChart program v.2.2 (Voorrips, 2002).
Coexpression Networks
For modeling coexpression networks, we used RNA-Seq count data grouped into transcript clusters through PASA v2.0 software (Haas et al., 2003). Only transcripts with at least 10 counts per million (CPM) were retained and normalized with a quantile-based approach implemented in the edgeR package in R (Robinson et al., 2010). Weighted gene correlation analysis (WGCNA) was performed using the WGCNA R package (Langfelder and Horvath, 2008) together with Pearson correlation coefficients. A soft thresholding power β-value was estimated for fitting the network into a scale-free topology, and a topological overlap measure (TOM) for each gene pair was used for building a dissimilarity matrix and for performing unweighted pair group method with arithmetic mean (UPGMA) hierarchical clustering. The best clustering scheme was defined using a variable height pruning technique implemented in the Dynamic Tree Cut R package (Langfelder et al., 2008). The groups containing genes associated with snpsGWAS were used to model a specific coexpression network using the igraph R package (Csardi and Nepusz, 2006) with Pearson correlation coefficients (minimum R value of 0.5), where we calculated the hub scores for each gene considering Kleinberg’s hub centrality scores (Kleinberg, 1999).
Metabolic Network Modeling
From the annotations performed for genes surrounding the snpsGWAS and the snpsLD, we retrieved the enzyme commission (EC) numbers and investigated the related metabolic pathways using the Kyoto Encyclopedia of Genes and Genomes (KEGG) database (Kanehisa and Goto, 2000). All the H. brasiliensis metabolic pathways with enzymes related to snpsGWAS and snpsLD were retrieved and used to model a metabolic network using BioPython v.1.78 (Cock et al., 2009). From the created network, we evaluate the following topological properties: (i) degree (Barabási and Oltvai, 2004), (ii) betweenness centrality (Brandes, 2001), (iii) stress (Brandes, 2001), (iv) short path length value (Watts and Strogatz, 1998), and (v) neighborhood connectivity (Maslov and Sneppen, 2002), using Cytoscape v3.8.2 (Shannon et al., 2003). The network was also categorized regarding its community structure, with the enzymes organized into modules using the HiDeF algorithm (Zheng et al., 2021).
Results
Phenotypic and Genotypic Analyses
The SD values were adjusted according to the mixed model from which the BLUPs were extracted for further analysis (Supplementary Table 1). All fixed and random effects showed significant effects under the LRT test (p < 0.01). The estimated variances were 4.56, 0.0001 and 26.69 for the genotype ( ), genotype x environment interaction ( ) and residual ( ) effects, respectively. The experimental design was confirmed to show normality of the residual variance based on the quantile-quantile graph (Q-Q plot; Supplementary Figure 1). The estimated heritability ( ) in the entire population was 0.55, which is close to those values found in previous studies on the species (Gonçalves et al., 1999; Chanroj et al., 2017).
The identification of SNPs was carried out using all 437 individuals. By employing the TASSEL pipeline, we produced 363,641 tags, which were aligned with the Hevea reference genome, producing an alignment rate of ~84.78%. We identified a total of 107,466 SNPs, which were filtered, resulting in a total of 30,266 high-quality markers (~28.16%), with an imputation rate of ~6.74%. This filtered SNP dataset was used for PCA, with 18.33 and 2.61% of the variance explained by the first two main components, respectively (Supplementary Figure 2). Although high LD decay was observed (Supplementary Figure 3A), we also assessed the LD decay rate only in the regions containing transposable elements (TEs; Supplementary Figure 3B), which was higher.
RNA-Seq Analyses
A total of ~530 million and ~633 million paired-end (PE) reads were obtained for the RRIM600 and GT1 genotypes, respectively. After quality filtering, we obtained ~933 million PE reads for assembling the transcripts through Trinity software. We identified 104,738 transcripts ranging from 500 to 22,333 bp (average transcript size of 1,874 bp and N50 of 2,369 bp) that were related to 49,304 genes. In total, 82,629 transcripts (78.89%) could be annotated using the Swiss-Prot database. We were able to associate Gene Ontology (GO) categories with 81,095 transcripts (77.42%) and metabolic pathways from the KEGG database with 74,668 transcripts (71.29%). A total of 11,150 different proteins could be associated with the estimated set of genes for rubber trees, with a high incidence of TEs; the retrovirus-related Pol polyprotein from transposon RE1 (RE1) (4.45%) and the retrovirus-related Pol polyprotein from transposon TNT 1-94 (TNT 1-94) (2.80%) were the most pronounced categories.
Genome Wide Association Study
With the FarmCPU method and the selected covariates, we were able to observe satisfactory adherence to the association mapping results (Figure 2A). Four snpsGWAS were identified on chromosomes 2, 5, 8, and 15 (Figure 2B). The MAFs of the snpsGWAS ranged from 10 to 45%, with the proportion of phenotype variance explained (PVE) ranging from 2 to 9% and additive effects ranging from −1 to 0.84 cm (Table 1). To assess all markers associated with SD, we expanded the set of significantly associated markers by means of LD tests on the total set of SNPs. A total of 181 snpsLD were found and showed a correlation greater than 0.7 with the snpsGWAS (Supplementary Figure 4). snpsLD are distributed on the 18 chromosomes of the rubber tree (Figure 3), flanking previously described QTLs (Conson et al., 2018). We were able to identify SNPs with distances of approximately 40 bp in the QTL regions (Figure 3).
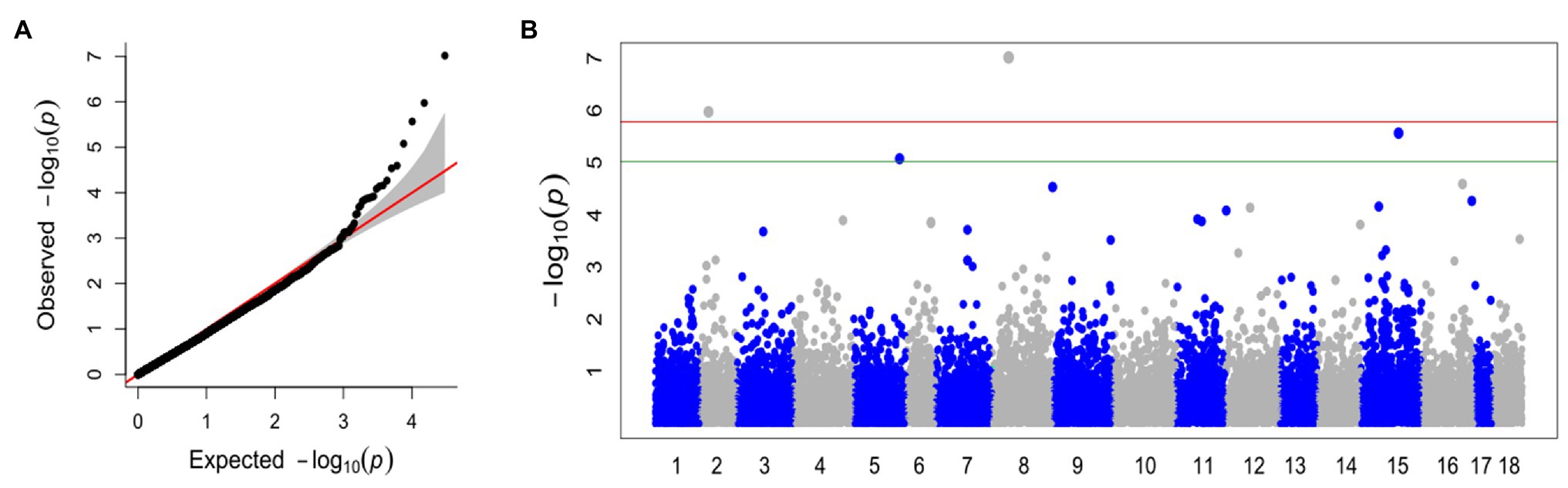
Figure 2. (A) Quantile-quantile plot for the broad genomic association model (GWAS), with the inclusion of the first main component (PC1 and PC2) as a covariate. (B) Manhattan plot for the GWAS. The x axis shows the chromosomes containing the discovered markers in their respective positions. The y axis shows the log (value of p) of the association. The green line represents the threshold obtained based on the data, and the red line represents the Bonferroni-corrected threshold of 0.05.
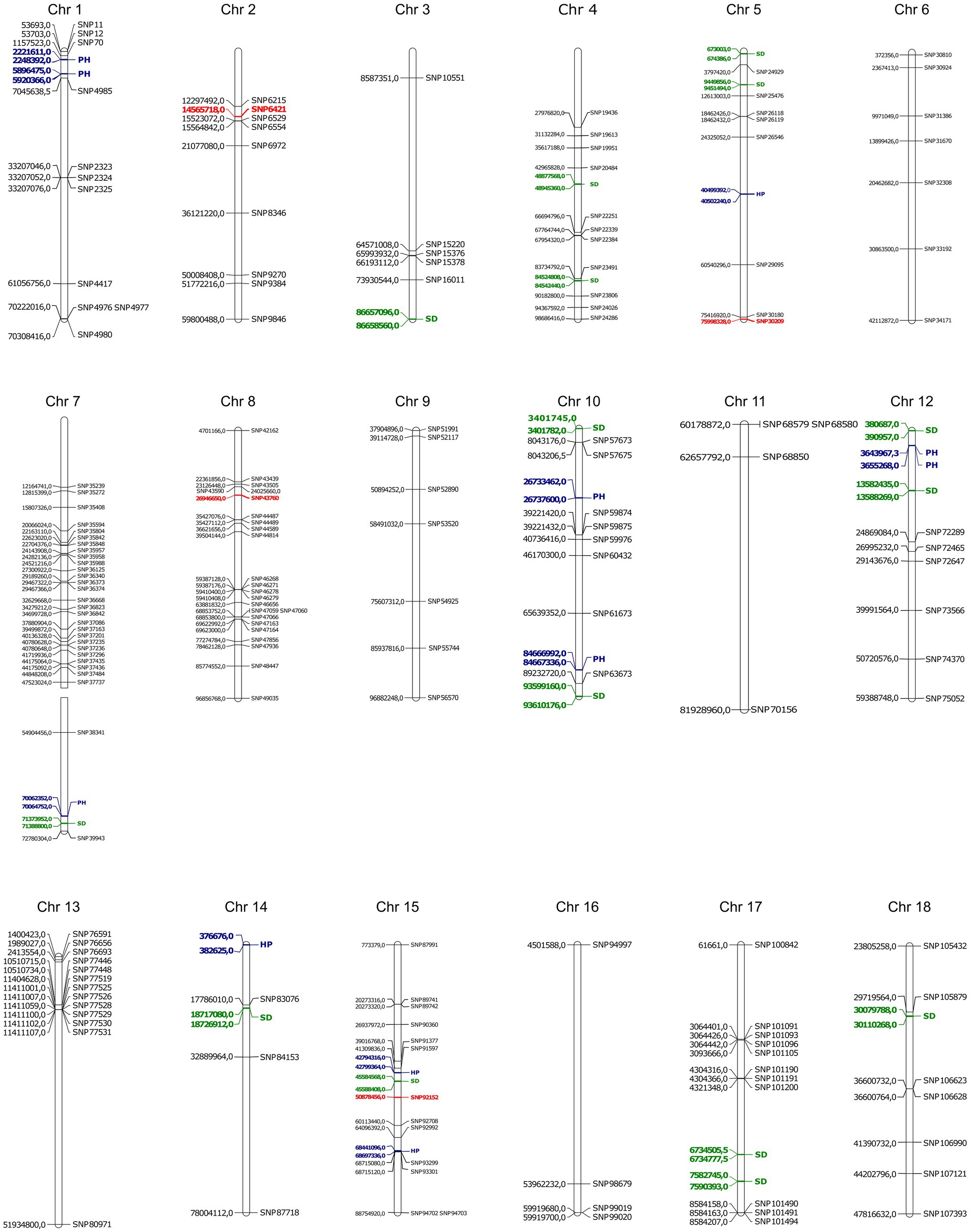
Figure 3. Physical position of snpsGWAS in red, snpsLD in black and QTLs discovered by Conson et al. (2018). The QTLs for plant height (PH) are in blue and those for stem diameter (SD) are in green.
To infer the associations between the set of SNPs (snpsGWAS and snpsLD) and expressed genomic regions, we performed comparative alignments of the transcripts assembled to the rubber tree chromosomes. SNPs were assigned to the first genes that were downstream and upstream of their location with an average distance of 7 kbp (Supplementary Table 2). Among the snpsLD, genes related to the transcription of important proteins involved in different stresses were found, such as TNT 1-94, receptor-like protein EIX2, integrin-linked protein kinase 1, U1 small nuclear ribonucleoprotein 70 kDa, histidine-containing phosphotransfer protein 2, rhomboid-like protein 14, and mitochondrial and threonine-protein kinase STN7. The annotation of the set of SNPs putatively associated with SD showed major biological processes related to DNA integration, response to water deprivation, regulation of intracellular pH, proton transmembrane transport, stomatal opening, flavonoid biosynthetic process, pollen sperm cell differentiation, oxidation–reduction process, circadian rhythm, carbohydrate metabolic process, multidimensional cell growth and chromatin organization (Figure 4).
Gene Coexpression Network
Of the 104,738 transcripts, 30,407 were selected for modeling a gene coexpression network using the WGCNA methodology (Zhang and Horvath, 2005). In such a network, pairwise gene interactions are modeled through a similarity measure, such as the Pearson correlation coefficient employed here. For fitting the network into a scale-free topology, we selected a power of 9 (scale-free topology model fit with and mean connectivity of ~183.47) and calculated the corresponding dissimilarity matrix through the WGCNA R package. With the network modeled, we combined UPGMA clustering with a variable height pruning technique, enabling the identification of 174 groups, with sizes ranging from 52 to 3,823 genes. The five groups containing the genes potentially related to the snpsGWAS were selected (Supplementary Table 3), and a new coexpression network was built including the genes associated with the snpsLD (Figure 5). All these genes formed a unique interaction network with weaker interactions connecting the found groups, which putatively represents the direct and indirect molecular associations with the SD phenotype. For the analysis of all reactions triggered by the genomic regions associated with GWAS, we evaluated this set of 1,528 genes for related GO terms (Figure 6). From the biological process category, we found new GO terms not associated with the genes related to snpsGWAS and snpsLD. These GO terms included defense response, positive regulation of transcription, cell wall organization, photosynthesis, cell division, mitotic cell cycle phase transition, carbon fixation, cell population proliferation, asymmetric cell division, and stomatal closure.
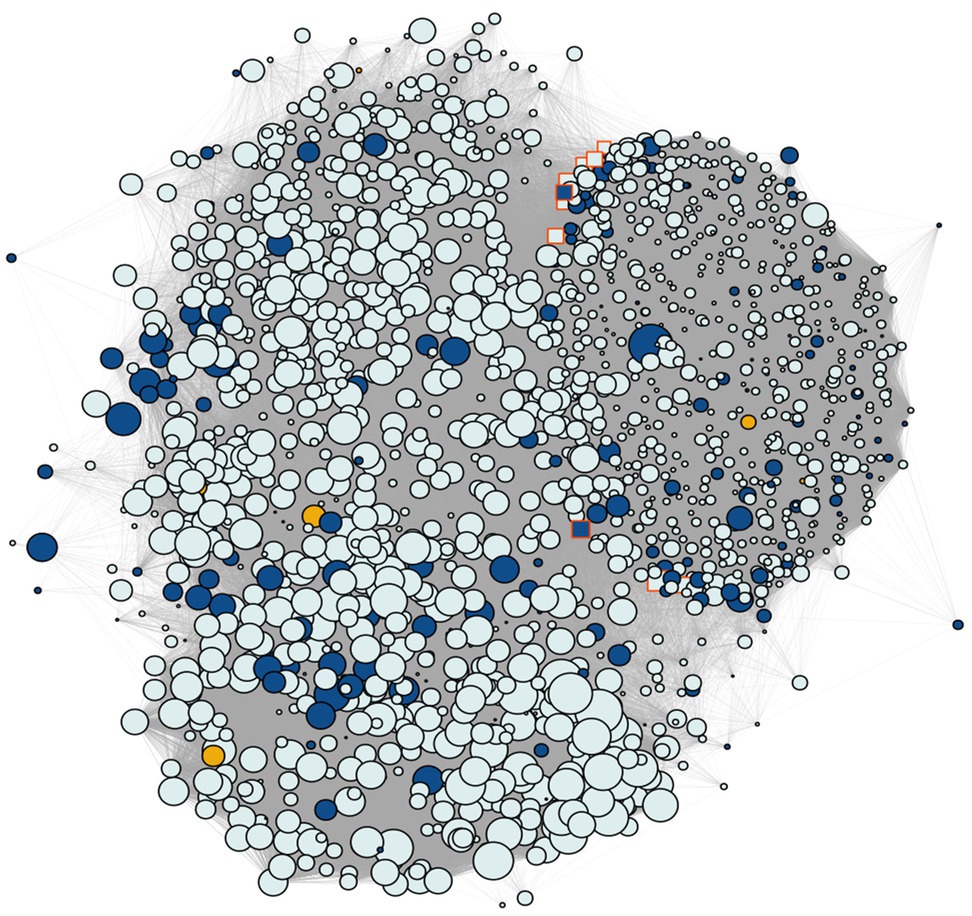
Figure 5. Coexpression network containing the SNP gene modules discovered by GWAS. Yellow shows the genes annotated for the snpsGWAS, blue shows the genes annotated for the snpsLD and gray shows the genes identified in the modules. The highlighted genes with a red border represent the 10 hubs with the most connectivity, while the size of the nodes shows the number of connected genes.
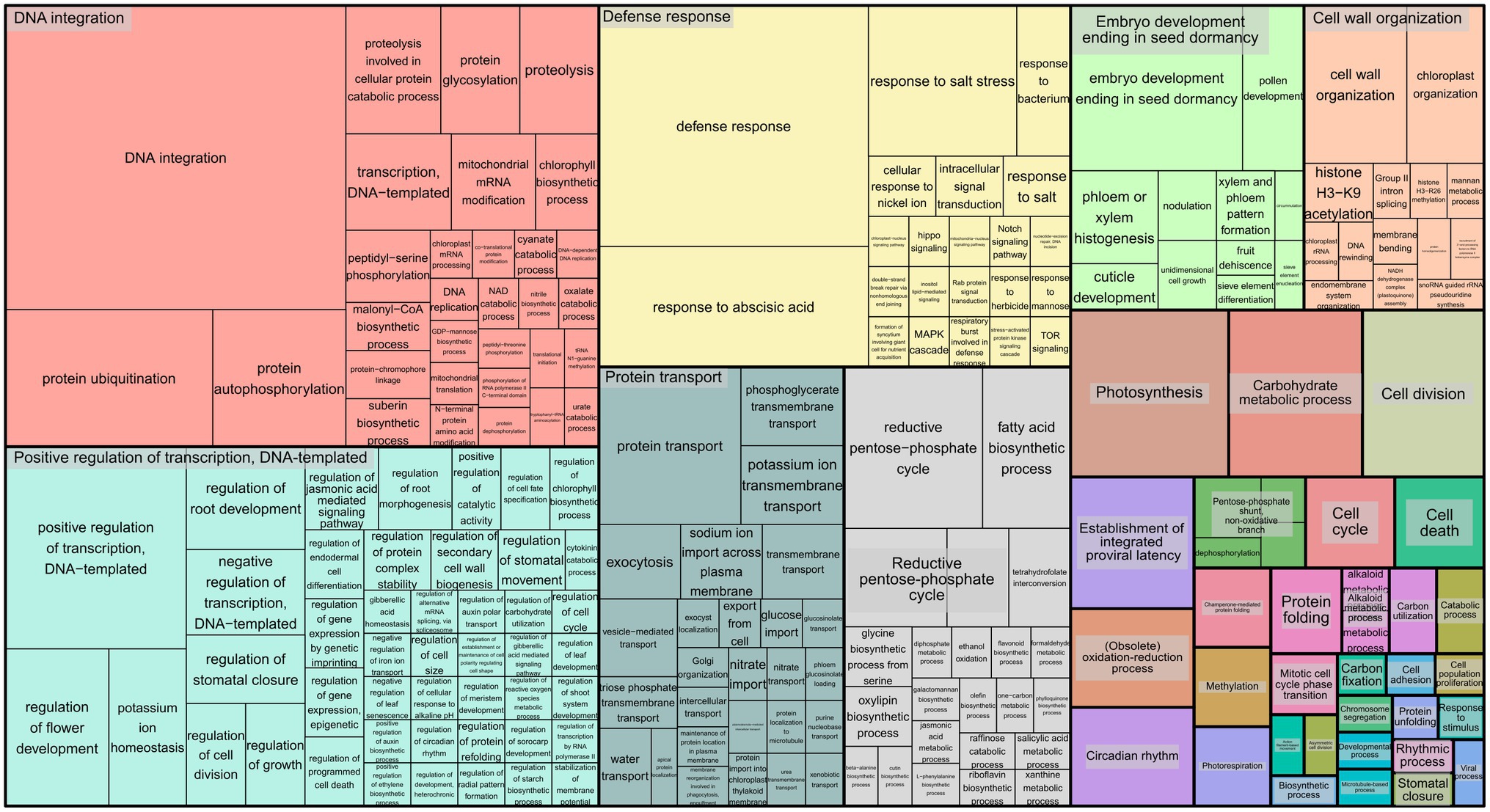
Figure 6. Treemap representing the biological processes for the GO terms of the annotated functional modules.
Regarding the genes found in these modules, as also observed in the general transcriptome profile, we observed a predominance of genes related to the protein retrovirus-related Pol polyprotein from transposon 17.6 (TE 17.6) (2.36%) and TNT 1-94 (1.23%). We also found several genes related to proteins involved in (Supplementary Table 3): (i) plant growth (e.g., MEI2-like 4 and threonine-protein kinase GSO1); (ii) the response to biotic and abiotic stress (e.g., abscisic acid-insensitive 5-like protein 6, transcription factor ICE1, abscisic acid receptor PYL4, transcription factor jungbrunnen 1, transcription factor MYB44, and galactinol synthase 2); (iii) root growth (e.g., alkaline/neutral invertase CINV2, threonine protein kinase IREH1, phospholipase D zeta 1, protein arabidillo 1, regulatory-associated protein of TOR 1, agamous-like MADS-box protein AGL12, and omega-hydroxypalmitate O-feruloyl transferase); (iv) the hormone abscisic acid (ABA) pathway; and (v) the light acclimatization process (e.g., GATA transcription factor 7 and malate dehydrogenase [NADP]). However, the great majority of these identified genes were not overexpressed, with a few exceptions (Supplementary Figure 5). To assess the most influential nodes within the network structure, we evaluated the hub scores of each gene within the network. The first hub gene in this network (PASSA_cluster_140395) was among the snpsLD genes, and the 10 first hubs had many known annotations. The first three hubs that had a known annotation were PASA_cluster_160224, PASA_cluster_87395, and PASA_cluster_140392, showing associations with TEs (Supplementary Table 3).
Metabolic Networks
Due to the clear absence of functional annotations, all the genes identified in the coexpressed modules with a known enzymatic activity relatedness were used for modeling a metabolic network using the KEGG database. In this structure, each enzyme corresponds to a node, and their connections are based on metabolic interactions. Nineteen genes were related to 19 different enzymes present in 28 metabolic pathways (Supplementary Table 4). All these reactions were joined into a unique network structure containing 405 nodes (enzymes) and 1,311 edges (average number of 5.338 neighbors and diameter of 22 nodes; Figure 7A; Supplementary Figure 6), representing a diverse cascade of mechanisms with putative associations with plant growth. Network topology measurements were performed to identify the most important enzymes in the modeled mechanisms.
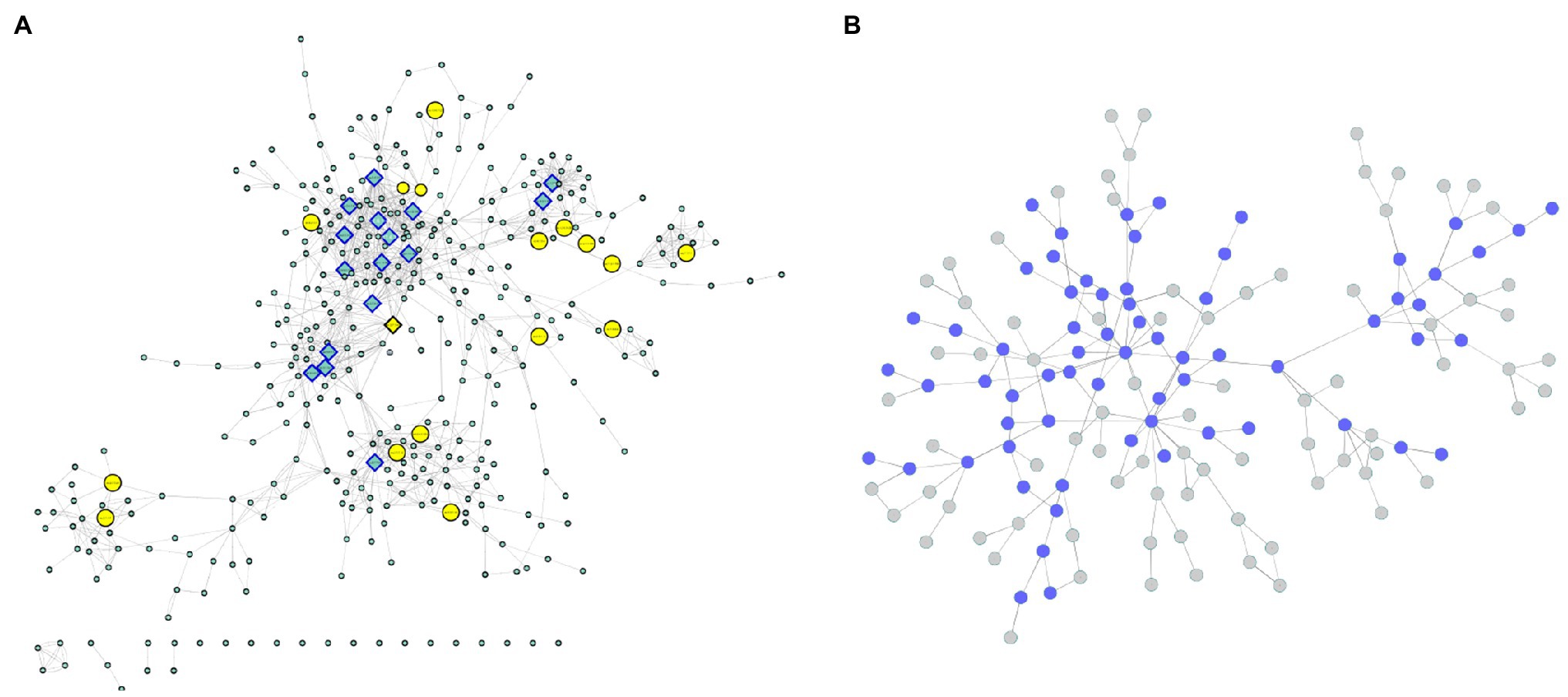
Figure 7. (A) Enzyme network. The yellow nodes represent the enzymes discovered in the coexpression modules, and the rectangular nodes indicate the enzymes with the highest centrality values. (B) Communities. The blue nodes are represented by communities containing enzymes discovered in the coexpression modules.
From the degree measures for each node (considering in and out connections), we identified 17 outliers (Figure 7A; Supplementary Figure 6), which were considered network hubs. We found enzymes with diverse roles (Supplementary Table 5), such as UDP-sugar pyrophosphorylase (ec: 2.7.7.64) (34 connections), ureidoglycolate amidohydrolase (ec:3.5.1.116) (26 connections) and alanine-glyoxylate transaminase (ec:2.6.1.44) (25 connections). Interestingly, these enzymes were also the ones with the highest values of outdegree, stress and betweenness. Considering only the indegree connections, the top four enzymes (also identified among the network hubs) were UDP-sugar pyrophosphorylase (ec: 2.7.7.64) (34 connections), glutamate dehydrogenase (NAD (P) +) (ec: 1.4.1.3) (19 connections), glutamate dehydrogenase (NADP+) (ec: 1.4.1.4) (18 connections) and malate dehydrogenase (oxaloacetate-decarboxylating) (NADP+) (ec: 1.1.1.40) (15 connections). Among the 17 hubs, pyruvate kinase (ec: 2.7.1.40) also presented high values for other centrality measures (betweenness and stress). Additionally, the enzyme threonine synthase (ec: 4.2.3.1) showed the highest short path length value (14.30) and the highest eccentricity value (22), and the glucuronokinase enzyme (ec: 2.7.1.43) showed the highest value for neighborhood connectivity (24).
In addition to these evaluations, the modeled network was also categorized into condensed modules regarding the community structure and enzyme organization (Figure 7B; Supplementary Figure 7; Supplementary Table 6). Using the HiDeF (Zheng et al., 2021) algorithm, 149 communities were identified, containing 4 to 389 enzymes. The community with the highest eccentricity (7) was c1337, which also contained the highest number of enzymes. The community with the highest stress value (255) and betweenness (0.37) was c13340 (with 68 enzymes), and it was among the top three communities with the highest eccentricity value (5; Supplementary Table 6).
Discussion
The genetic improvement of rubber trees requires a long period of time, with more than 30 years estimated for developing an improved genotype (Gonçalves and Fontes, 2012). Despite the specialized labor required for Hevea phenotyping, its plantation is only possible in vast areas, making the selection process laborious and financially expensive. In this context, the use of MAS can drastically reduce the time and the cost of genetic improvement, especially if implemented in the first years after obtaining the seeds by selecting the target characteristics indirectly through phenotypically associated markers (Xu and Crouch, 2008). As a way of assisting such initiatives, in this work, we identified SNPs associated with SD, and this set of markers can be used as high-priority candidates for MAS, with a high potential of providing greater precision and requiring less time in the selection of superior genotypes.
The main abiotic limitations for the productivity of cultivated plants are excessive salinity, adverse temperatures, and water deficit (Zhu, 2016), and for rubber tree production, water stress and cold are widely described as the most impactful limitations (Ding et al., 2020). Although several studies have investigated the molecular mechanisms of Hevea in cold resistance for its improvement (Cheng et al., 2018; Deng et al., 2018; Mantello et al., 2019), one of the main characteristics evaluated in Hevea breeding programs is SD (Priyadarshan, 2003) due to its versatility in assessing rubber tree productive efficiency (Dijkman, 1951; Goncalves et al., 1984; Chanroj et al., 2017; Conson et al., 2018; Khan et al., 2018; Chen et al., 2020). The use of SD measures can provide insights into phenotypes that can only be measured in specific climate conditions, such as drought resistance (Ohashi et al., 2006; Zhang et al., 2019a), which impacts rubber tree growth (Chandrashekar et al., 1998). Additionally, traits that can only be measured after a certain age of the plant, such as the production of latex and vigor (Dijkman, 1951; Goncalves et al., 1984), can be estimated by SD.
As SD is a quantitative characteristic, the study of the genetic architecture related to this trait is quite complex, considering the high amount of genes and metabolic pathways involved in its definition (Pootakham et al., 2020). Furthermore, the genome of rubber trees encompasses a large number of repetitive regions, reaching approximately 71% of rubber tree genomic content (Tang et al., 2016). The first Hevea reference genome at the chromosome level was only recently published in 2020 (Liu et al., 2020a), and most genomic approaches in the species have been based on highly fragmented sequences and biocomputational estimations (Pootakham et al., 2015; Chanroj et al., 2017; Conson et al., 2018; de Souza et al., 2018; Souza et al., 2019). Only with the advent of molecular biology techniques for reducing genomic complexities during sequencing procedures, such as GBS (Elshire et al., 2011; Poland and Rife, 2012), has it been feasible to generate thousands of SNP markers with high frequency in complex plant genomes (Pootakham et al., 2015). By using a GBS approach combined with a rubber tree chromosome-level reference genome, we characterized a large number of high-quality markers regarding their genomic distribution and LD relatedness, which enabled us to compare our findings with the locations of several QTLs for this characteristic, embracing novel possible causal genes explaining this phenotypic variation.
The large number of SNP markers discovered in this work allowed us to assess the LD throughout the genome of the entire population in a very representative way. As in other studies using arboreal and allogamous species (Peláez et al., 2020), our results showed high LD, which is consistent with previous H. brasiliensis results (Chanroj et al., 2017; De Souza et al., 2018). Interestingly, such elevated decay is not constant, and regions with a high density of TEs present a lower level of LD compared to the overall genomic LD. TEs are known as mobile elements due to their ability to change positions along the genome and produce copies of themselves (Singh et al., 2019), mainly in genomic regions with low LD (Stuart et al., 2016; Choudhury et al., 2019), as was observed in this work (Supplementary Figure 3). As stated by Choudhury et al. (2019), we also believe that there are two main reasons for this observation: (i) TEs alter the genetic architecture of the chromosome by decreasing the recombination rate in its vicinity and (ii) TEs accumulate in these regions due to the low recombination rate that occurs in these locations.
Several studies have been developed to characterize SD QTLs (Souza et al., 2013; Conson et al., 2018; Rosa et al., 2018); however, these studies are limited to the biparental populations employed (Myles et al., 2009). With the use of genetically diverse populations, GWAS approaches use the historical links between different genotypes, capturing more genetic diversity through a broader set of markers that would be neglected in association maps (Kulwal, 2018). When we are unable to identify the expected segregation ratios in markers from biparental progenies, these regions, even those close to important QTLs, are often discarded along with their associated QTLs (Kulwal, 2018). In this context, GWAS approaches have been suggested as a powerful tool for overcoming such limitations, which are intensified in species such as H. brasiliensis, in which there are great difficulties in obtaining mapping populations.
Genome-Wide Association Studies
To date, only one study employing GWAS has been described in the literature for H. brasiliensis. Using a population of 170 individuals genotyped with 14,155 SNP markers by capture probes (Shearman et al., 2014), Chanroj et al. (2017) tested four association models. The authors could associate two SNP markers with latex production (one for the rainy season and the other one for the drought season) and two others with SD (also separated by rainy and drought seasons). According to Conson et al. (2018), the rubber tree populations planted in the escape areas are under water stress at all times, despite the differences in water regime across seasons. Due to such observations and the Brazilian climate, we performed our analyses without making this distinction. In this way, we identified four SNPs associated with SD, which were annotated following an RNA-Seq-based approach. Even though SD is a quantitative characteristic, the discovery of a relatively small number of markers by GWAS is possibly related to the limitation of the technique (Yang et al., 2010; Korte and Farlow, 2013; Tam et al., 2019). To overcome the large number of false negatives caused by the restrictive threshold employed (Tam et al., 2019) and the limitations related to the discovery of SNPs by GBS, we investigated the set of snpsLD. Although the GBS methodology avoids repeated regions of the genome (Elshire et al., 2011), these duplications represent about 70% of the rubber tree genome (Tang et al., 2016; Liu et al., 2020c), and the linkage disequilibrium tests represent an indirect way of assessing putative functional associations with GWAS results, including those ones caused by duplication events (Lee et al., 2012). Additionally, for going further in the establishment of putative genes related to QTLs, we integrated these results with co-expression network analyses based on RNA-Seq data. This approach has been shown to be effective in several species (Calabrese et al., 2017; Schaefer et al., 2018; Yan et al., 2020), but with a restricted use in non-model plant species.
Different from establishing a genomic window surrounding these markers and performing comparative alignments against plant databases (Chanroj et al., 2017; García-Fernández et al., 2021), we used an assembled transcriptome for the association of the snpsGWAS. This step was performed mainly because of the absence of available data for several neglected species in public databases (Schaefer et al., 2018), such as H. brasiliensis. Moreover, transcriptome assemblies are a way of categorizing a broader range of important genes found under stress conditions (Valdés et al., 2013; Wei et al., 2021), as already reported by other Hevea studies (Ahn et al., 2017; Mantello et al., 2019). Additionally, because of the recent availability of the Hevea genome (Liu et al., 2020a), more studies are required for complete and accurate gene categorization. By coupling the transcriptome assembly with GWAS, we could associate the three candidate genes identified by the snpsGWAS, which were annotated and had their expression profile estimated in two different genotypes, including in the population used here, and in specific stages of the plant development and physiology. As pointed out by Schaefer et al. (2018), this type of strategy provides associations not only with growth but also with resistance to abiotic stress. The genes identified flanking the snpsGWAS were interpreted according to their biological function and their metabolic context (Watanabe et al., 2017), suggesting their potential relationships in defining the phenotype.
The SBT4.6 gene (Table 1), identified based on the snpsGWAS, belongs to the subtilisin-like protease family, whose members are involved in general protein turnover and regulatory processes and in mechanisms of resistance to biotic and abiotic stresses (Tian et al., 2005; Budič et al., 2013; Figueiredo et al., 2018). Under normal conditions, mutants for this gene do not show obvious changes in the normal growth of the plant, so there is still a need for further investigations regarding this gene in the development of the plant (Rautengarten et al., 2005). Although this gene is not clearly involved in plant growth under normal conditions, we suggest that it may be indirectly related to this characteristic. Two other genes associated with snpsGWAS show evidence of a relationship with abiotic stresses. In addition to showing an increasing additive effect of 0.54 cm in the SD for a specific genotypic class (Table 1), SNP30209 was in the vicinity of a genomic region containing a candidate gene for GK1. In experiments carried out with Arabidopsis thaliana, GK1 showed a behavior of D-aminoacyl-tRNA deacylase, which is important for protecting the plant against the toxicity of D-amino acids (Wydau et al., 2007), which, when present in the soil, can have effects on plant growth in different ecosystems, whether managed or not. These compounds can act in different ways on root and stem growth, with D-serine, D-alanine and D-tyrosine being the strongest growth inhibitors, while others, such as D-lysine, D-isoleucine, D-valine, D-asparagine, and D-glutamine, act as milder inhibitors (Vranova et al., 2012). Another associated gene was IQM2, which contains a domain for the IQM2 protein. Such a protein belongs to a calmodulin-binding family protein and has strict involvement in the response to biotic and abiotic stress (Wan et al., 2012).
Despite the unquestionable importance of GWAS methods, the practical application of these findings in MAS for the selection of several complex characteristics is limited due to the low heritability associated with these markers (Bogardus, 2009). Considering this fact, we also investigated associated genomic regions, which may be jointly involved in phenotype definition (Yuan et al., 2012). Several statistical methods are used to identify genomic associations, such as multifactor dimensionality reduction (Ritchie et al., 2001), LD (Wu et al., 2008) and entropy-based statistics (Dong et al., 2008). In this work, we employed SNP correlations, which led us to already establish QTL positions (Conson et al., 2018), showing the robustness of this method. These newly identified markers may reveal genes that would be overlooked by conventional GWAS approaches.
In addition to MAS, other important tools for the genetic improvement of various plant species have been developed, such as iRNA (Zhang et al., 2017) and CRISPR (Jaganathan et al., 2018), which have shown enormous potential for breeding strategies in recent years (Kalunke et al., 2020; Liu et al., 2020c). However, these approaches require the definition of target genes and their interactions, which might be estimated through coexpression and metabolic networks. In this way, to provide a deeper investigation into the metabolic activities of the genes associated with the snpsGWAS and snpsLD, we modeled complex networks to investigate their interactions and provide insights into the definition of the SD quantitative trait (Kosová et al., 2015; Tam et al., 2019), decreasing the variability of the indirectly selected phenotype and accessing other omics layers. The multiomics approaches employed here can contribute to a better understanding of the molecular mechanisms that are important to the vegetative growth of rubber trees, opening new perspectives for deeper genomic studies.
Multiomics
Quantitative traits are strongly affected by environment x genotype interactions (Nguyen et al., 2019). Genotypes with a greater capacity to resist these abiotic factors have a greater capacity to grow and develop under these stresses (Mantello et al., 2019). Understanding all the molecular biological levels that confer such a resistance to these specific genotypes requires the integration of multiple omics approaches, such as genomics, transcriptomics, proteomics and metabolomics. Multiomics approaches have as their main objective the integration of data analysis of different biological levels for a better understanding of their relationships and the functioning of a biological system as a whole (Joyce and Palsson, 2006). The use of joint approaches benefits from including all relevant parts that integrate the analyzed biological system (Zhang et al., 2010). Studies that integrate the discovery of QTLs with other omics have used genetically well-studied agricultural crops such as corn (Jiang et al., 2019) and, more recently, tree species such as citrus (Mou et al., 2021).
To provide deeper insights into the molecular basis of the evaluated phenotype, we extended the selected set of SD-associated SNPs with data from transcriptomics using complex network methodologies. These methodologies have revolutionized research in molecular biology because of their capability to simulate complex biological systems (D’haeseleer et al., 2000; Liu et al., 2020b) and infer novel biological associations, such as regulatory relationships, metabolic pathway inferences and annotation transference (Rao and Dixon, 2019). In H. brasiliensis, coexpression network methods have already revealed genes involved in different environmental or stress conditions and are a powerful tool for profiling rubber tree samples (Hurtado Páez et al., 2015; Sathik et al., 2018; Mantello et al., 2019; Deng et al., 2018; Ding et al., 2020). Such studies in rubber trees are still incipient and have not yet been coupled with breeding strategies for the genetic improvement of the species. Starting from RNA-Seq-based data, we could associate our GWAS results with expression profiles from important Hevea genotypes, incorporating our results into a complete set of molecular interactions estimated through the WGCNA approach. Using this strategy, we can infer biological functions for genes present in the same network module, as these genes probably exert correlated functions (Childs et al., 2011). This is the first initiative that proposes the integration of GWAS and coexpression networks in rubber trees to identify genes with great potential to be used in MAS.
The transcriptome used for annotation and construction of the coexpression network showed a large number of TEs, which are indeed present in large amounts in plant genomes (Matsunaga et al., 2015). In addition, these TEs were also found to be abundant in the selected functional modules, with TE 17.6 and TNT 1-94 being the most prominent. These TEs have already been described as being involved in gene expression, responses to external stimuli and plant development (Kashkush et al., 2003; Matsunaga et al., 2015; Traylor-Knowles et al., 2017; Tran and Choi, 2020). In rubber trees, TEs may be related to the differential expression observed in some commercial clones, affecting important processes such as rubber production (Wu et al., 2020a). As pointed out by Wang et al. (2020), the identification of TEs associated with functional genes related to important characteristics suggest that they can be used as molecular markers in MAS, contributing significantly to the genetic improvement of woody trees. In this sense, our findings supply a wide range of genomic resources for breeding. In the selected coexpression network, the most abundant elements were also TE 17.6 and TNT 1-94.
In the coexpression module with the largest number of genes, we were able to identify many genes related to plant growth, such as the protein MEI2-like 4 (ML4), which is a substrate for putative TOR, the main regulator of cell growth in eukaryotes (Anderson et al., 2005), representing an extremely important molecule in meiotic signaling (Watanabe et al., 1988). In this module, we also identified the proteins alkaline/neutral invertase CINV2 (CINV2) and LRR receptor-like serine/threonine-protein kinase GSO1 (GSO1), which are related to root growth and endoderm. The invertase enzyme (INV) is one of only two enzymes capable of catabolizing physiological carbon, together with the sucrose synthase enzyme (SUS); thus, most of the plant biomass is indispensable for normal growth, and the loss of these genes slows plant growth (Barratt et al., 2009). According to Racolta et al. (2014), the GSO1 protein works together with GSO2 for the intracellular signaling of the plant, positively regulating cell proliferation, the differentiation of root cells and the identity of stem cells.
In the other functional modules, we identified several proteins involved in abiotic stress, such as transcription factor ICE1 (SCRM), an upstream transcription factor that regulates cold CBF gene transcription, improving plant tolerance to freezing (Chinnusamy et al., 2003). The regulatory-associated protein of TOR 1 (RAPTOR1) presents itself as a TOR regulator in response to osmotic stress (Mahfouz et al., 2006). The transcription factor jumgbrunnen 1 (JUB1), which delays senescence, also confers resistance to abiotic stress, such as heat shock, and resistance to high levels of intracellular H2O2 (Wu et al., 2012). Protein galactinol synthase 2 (GOLS2) plays an important role in the response against drought and cold stresses (Taji et al., 2002). The protein E3 ubiquitin-protein ligase PUB23 (PUB23), which responds quickly to water stress (Cho et al., 2008) and biotic stress, and the protein glucan endo-1,3-beta-glucosidase (HGN1) have been reported in H. brasiliensis and participate in a defense response against fungi (Galicia et al., 2015). In addition to these proteins produced in response to a given stress, genes involved in the maintenance and development of vegetative parts important for the development of the plant under a given stressful condition, such as constant drought, were identified, including arabidillo 1 protein (FBX5), which is related to the development of the roots (Coates et al., 2006), and Agamous-like MADS-box protein AGL12 (AGL12; Tapia-López et al., 2008). These results confirm the involvement of genes identified by GWAS and other genes identified in functional modules in the investigated characteristic definition. We can also relate the region of the SNP43760 marker, which has no known annotation, to QTLs involved in resistance to environmental factors, since the functional module containing these genes is related to this process.
Finally, a metabolic network for the enzymes found in this data set was constructed to identify the main metabolic pathways involved in the growth process of the rubber tree. The metabolites produced in cells can be understood as a bridge between the genotype and the phenotype. A clearer understanding of the relationship between these enzymes, such as by identifying the main enzymes present in the network, is essential for maintaining the properties of this network and thus preserving these relationships. The network built in this work shows some disconnected enzymes because the reactions that connect them with the other enzymes in the network have not yet been elucidated.
We identified UDP-sugar pyrophosphorylase (USP) as the hub of this enzyme network; this enzyme indicated to be an enzyme of great importance in the network, as it presented the highest degree value (Barabási and Oltvai, 2004). It also showed the highest out-degree value, which represents the number of connections directed from this node to the other nodes in the network. This enzyme is very conserved in plants (Geserick and Tenhaken, 2013). Evidence indicates a high affinity of USP for acid-1-phosphate (UDP-GlcA-1-P), a substrate of the myo-inositol oxygenase (MIOX) pathway for UDP-GlcA (Geserick and Tenhaken, 2013). USP can also convert different types of sugar-1-phosphatates into the UDP sugars that make up polymers and glycerols in plant cell walls (Geserick and Tenhaken, 2013). USP is found in a single copy in Arabidopsis, and mutants for this gene are lethal (Geserick and Tenhaken, 2013), as the pollen that carries this mutation does not develop normally (Schnurr et al., 2006; Geserick and Tenhaken, 2013). Knock-down mutants also show impaired vegetative growth due to deficiency in sugar recycling (Geserick and Tenhaken, 2013). The enzyme glutamate dehydrogenase (NAD (P) +) (GDH) appeared in the enzymatic network containing a high degree of indegree. GDH catalyzes the deamination of glutamate using NAD as a coenzyme and releases 2-oxoglutarate and ammonia when there is little carbon (Fontaine et al., 2006). Participating in the response to various stresses, including drought and the presence of pathogens, their expression levels are regulated according to the intensity of the stress (Restivo, 2004), increasing the capacity of resistance to stress and the acquisition of biomass by the plant (Qiu et al., 2009; Tercé-Laforgue et al., 2015). The pyruvate kinase enzyme was shown to be central in the integration of its components, presenting a higher value of betweenness centrality (Brandes, 2001), indicating an important control function of this enzyme in the network, since this measure indicates elements in the network that join communities. In addition to this enzyme being important for the integration of the components in the metabolic network, this enzyme also presents itself as important in the dissemination of information among the elements present in the metabolic network, since it presented a higher stress value (Brandes, 2001), which indicates the shortest path between two random nodes in the network. This enzyme is a key element in the regulation and adjustment of the glucose metabolic pathway (Ambasht and Kayastha, 2002; Cai et al., 2018). Pyruvate kinase catalyzes the irreversible transfer of the high-energy phosphate group from phosphoenopyruvate to ADP, synthesizing ATP (Ambasht and Kayastha, 2002). Another important enzyme for the dissemination of information within the network was threonine synthase (thrC), which showed a higher value for the short path length (Watts and Strogatz, 1998) and eccentricity, which indicates the maximum number of nodes necessary for the information to reach all nodes present in the network (Hage and Harary, 1995). Theonine (Thr) enzymes play important roles in the stress response to abiotic factors such as salinity, cold and drought (Rudrabhatla and Rajasekharan, 2002; Diédhiou et al., 2008), in addition to the different processes related to plant growth, such as cell division and the regulation of several phytohormones (Rudrabhatla and Rajasekharan, 2002) and carbon flux (Zeh et al., 2001).
In this work, we identified many genes involved in the response to drought, showing the importance of this element for the development of rubber trees, as already reported by Conforto (2008). Conson et al. (2018) and Souza et al. (2019) showed that the environments in which the populations used in this work are grown are environments with constant water deficit, which was expected because they are escape areas, which presents different climate of their natural habitat but where the rubber tree has adapted well. In the context of climate change, the discovery of genes involved in responding to water stress is of great value since forecasts show that in the near future areas suitable for planting today may become unsuitable (Ray et al., 2016). Most likely, these changes will occur mainly in the water regime, which can lead to the death of many woody plants (Adams et al., 2009).
Despite the limitations of the GWAS in identifying genes related to quantitative traits, the multiomics strategy employed in this study allowed us to explore the main genes that putatively define this phenotype from a holistic perspective, expanding this investigation and supplying a large reservoir of data. Using the integration of GWAS with coexpression networks and enzyme networks, we were able to elucidate the main relationships of these major genes and their products in a more complete way, mainly considering the limitations of GWAS in the identification of regions of QTLs with small effects. With the functional modules defined, we can gain insight into the genes that work together. In addition to the understanding that the definition of SD is based on the interaction of several processes, we have identified six functional modules. Even with more than one process, all these interactions work together, as we can see in the network shown in Figure 6. In addition, we can see the robustness of these results, which show correlations with previously published QTL maps (Conson et al., 2018). Posttranslational inferences were made regarding the relationships identified in the enzymatic network, which allowed us to identify new and important gene products that were previously unidentified. All these results show the importance of these integrative studies that correct the limitations of each individual technique.
This work is the first initiative that integrates multiomics in the study of QTLs in H. brasiliensis. Using this approach, we were able to access all important molecular levels for the definition of SD. Despite the great economic importance of the species, as it is the only one capable of producing natural rubber in sufficient quantity and quality to supply the world market for this product (Ding et al., 2020), its genetic studies are still quite limited due to the complexity of its genome (Tang et al., 2016), its great genetic variability (De Souza et al., 2018) and the large areas needed for its plantation. Despite all these limitations, this work overcomes these difficulties, producing data, results and new methodological perspectives for future genomic studies in this species and identifying markers and genes useful for genetic improvement.
Data Availability Statement
The original contributions presented in the study are publicly available. This data can be found here: National Center for Biotechnology Information (NCBI) BioProject database under accession numbers SRP155829, PRJNA540286 and PRJNA541308.
Author Contributions
FF and AA performed all the analyses and wrote the manuscript. CS assisted in the genotypic data analyses. PG, VG, and ES conducted the field experiments. AS, LS, and RF-N conceived the project. All authors contributed to the article and approved the submitted version.
Funding
The authors gratefully acknowledge the Fundação de Amparo à Pesquisa do Estado de São Paulo (FAPESP) for Ph.D. fellowship to FF (18/18985-7) and AA (2019/03232-6); the Coordenação de Aperfeiçoamento do Pessoal de Nível Superior (CAPES) for financial support (Computational Biology Program and CAPES-Agropolis Program); and the Conselho Nacional de Desenvolvimento Científico e Tecnológico (CNPq) for research fellowships to AS and PG.
Conflict of Interest
The authors declare that the research was conducted in the absence of any commercial or financial relationships that could be construed as a potential conflict of interest.
Publisher’s Note
All claims expressed in this article are solely those of the authors and do not necessarily represent those of their affiliated organizations, or those of the publisher, the editors and the reviewers. Any product that may be evaluated in this article, or claim that may be made by its manufacturer, is not guaranteed or endorsed by the publisher.
Supplementary Material
The Supplementary Material for this article can be found online at: https://www.frontiersin.org/articles/10.3389/fpls.2021.768589/full#supplementary-material
Footnotes
References
Adams, H. D., Guardiola-Claramonte, M., Barron-Gafford, G. A., Villegas, J. C., Breshears, D. D., Zou, C. B., et al. (2009). Temperature sensitivity of drought-induced tree mortality portends increased regional die-off under global-change-type drought. Proc. Natl. Acad. Sci. U. S. A. 106, 7063–7066. doi: 10.1073/pnas.0901438106
Ahn, H., Jung, I., Shin, S. J., Park, J., Rhee, S., Kim, J. K., et al. (2017). Transcriptional network analysis reveals drought resistance mechanisms of AP2/ERF transgenic rice. Front. Plant Sci. 8:1044. doi: 10.3389/fpls.2017.01044
Ambasht, P. K., and Kayastha, A. M. (2002). Plant pyruvate kinase. Biol. Plant. 45, 1–10. doi: 10.1023/A:1015173724712
Anderson, G. H., Veit, B., and Hanson, M. R. (2005). The Arabidopsis AtRaptor genes are essential for post-embryonic plant growth. BMC Biol. 3:12. doi: 10.1186/1741-7007-3-12
Barabási, A. L., and Oltvai, Z. N. (2004). Network biology: understanding the cell’s functional organization. Nat. Rev. Genet. 5, 101–113. doi: 10.1038/nrg1272
Barratt, D. H., Derbyshire, P., Findlay, K., Pike, M., Wellner, N., Lunn, J., et al. (2009). Normal growth of Arabidopsis requires cytosolic invertase but not sucrose synthase. Proc. Natl. Acad. Sci. U. S. A. 106, 13124–13129. doi: 10.1073/pnas.0900689106
Boeckmann, B., Bairoch, A., Apweiler, R., Blatter, M. C., Estreicher, A., Gasteiger, E., et al. (2003). The SWISS-PROT protein knowledgebase and its supplement TrEMBL in 2003. Nucleic Acids Res. 31, 365–370. doi: 10.1093/nar/gkg095
Bogardus, C. (2009). Missing heritability and GWAS utility. Obesity 17:209. doi: 10.1038/oby.2008.613
Brandes, U. (2001). A faster algorithm for betweenness centrality. J. Math. Sociol. 25, 163–177. doi: 10.1080/0022250X.2001.9990249
Budič, M., Sabotič, J., Meglič, V., Kos, J., and Kidrič, M. (2013). Characterization of two novel subtilases from common bean (Phaseolus vulgaris L.) and their responses to drought. Plant Physiol. Biochem. 62, 79–87. doi: 10.1016/j.plaphy.2012.10.022
Cai, Y., Li, S., Jiao, G., Sheng, Z., Wu, Y., Shao, G., et al. (2018). OsPK2 encodes a plastidic pyruvate kinase involved in rice endosperm starch synthesis, compound granule formation and grain filling. Plant Biotechnol. J. 16, 1878–1891. doi: 10.1111/pbi.12923
Calabrese, G. M., Mesner, L. D., Stains, J. P., Tommasini, S. M., Horowitz, M. C., Rosen, C. J., et al. (2017). Integrating GWAS and co-expression network data identifies bone mineral density genes SPTBN1 and MARK3 and an osteoblast functional module. Cell Syst. 4, 46–59. doi: 10.1016/j.cels.2016.10.014
Campbell, M. S., Holt, C., Moore, B., and Yandell, M. (2014). Genome annotation and curation using MAKER and MAKER-P. Curr. Protoc. Bioinformatics 48, 4.11.1–4.11.39. doi: 10.1002/0471250953.bi0411s48
Challa, S., and Neelapu, N. R. R. (2018). “Genome-wide association studies (GWAS) for abiotic stress tolerance in plants,” in Biochemical, Physiological and Molecular Avenues for Combating Abiotic Stress in Plants. ed. S. H. Wani (London, UK: Academic Press), 125–150.
Chandrashekar, T. R., Nazeer, M. A., Marattukalam, J. G., Prakash, G. P., Annamalainathan, K., and Thomas, J. (1998). An analysis of growth and drought tolerance in rubber during the immature phase in a dry subhumid climate. Exp. Agric. 34, 287–300. doi: 10.1017/S0014479798343045
Chanroj, V., Rattanawong, R., Phumichai, T., Tangphatsornruang, S., and Ukoskit, K. (2017). Genome-wide association mapping of latex yield and girth in Amazonian accessions of Hevea brasiliensis grown in a suboptimal climate zone. Genomics 109, 475–484. doi: 10.1016/j.ygeno.2017.07.005
Chen, L., Fang, Y., Li, X., Zeng, K., Chen, H., Zhang, H., et al. (2020). Identification of soybean drought-tolerant genotypes and loci correlated with agronomic traits contributes new candidate genes for breeding. Plant Mol. Biol. 102, 109–122. doi: 10.1007/s11103-019-00934-7
Cheng, H., Chen, X., Fang, J., An, Z., Hu, Y., and Huang, H. (2018). Comparative transcriptome analysis reveals an early gene expression profile that contributes to cold resistance in Hevea brasiliensis (the Para rubber tree). Tree Physiol. 38, 1409–1423. doi: 10.1093/treephys/tpy014
Childs, K. L., Davidson, R. M., and Buell, C. R. (2011). Gene coexpression network analysis as a source of functional annotation for rice genes. PLoS One 6:e22196. doi: 10.1371/journal.pone.0022196
Chinnusamy, V., Ohta, M., Kanrar, S., Lee, B. H., Hong, X., Agarwal, M., et al. (2003). ICE1: a regulator of cold-induced transcriptome and freezing tolerance in Arabidopsis. Genes Dev. 17, 1043–1054. doi: 10.1101/gad.1077503
Cho, S. K., Ryu, M. Y., Song, C., Kwak, J. M., and Kim, W. T. (2008). Arabidopsis PUB22 and PUB23 are homologous U-box E3 ubiquitin ligases that play combinatory roles in response to drought stress. Plant Cell 20, 1899–1914. doi: 10.1105/tpc.108.060699
Choudhury, R. R., Rogivue, A., Gugerli, F., and Parisod, C. (2019). Impact of polymorphic transposable elements on linkage disequilibrium along chromosomes. Mol. Ecol. 28, 1550–1562. doi: 10.1111/mec.15014
Coates, J. C., Laplaze, L., and Haseloff, J. (2006). Armadillo-related proteins promote lateral root development in Arabidopsis. Proc. Natl. Acad. Sci. 103, 1621–1626. doi: 10.1073/pnas.0507575103
Cock, P. J., Antao, T., Chang, J. T., Chapman, B. A., Cox, C. J., Dalke, A., et al. (2009). Biopython: freely available python tools for computational molecular biology and bioinformatics. Bioinformatics 25, 1422–1423. doi: 10.1093/bioinformatics/btp163
Conforto, E. D. (2008). Respostas fisiológicas ao déficit hídrico em duas cultivares enxertadas de seringueira (“RRIM 600” e “GT 1”) crescidas em campo. Cienc. Rural. 38, 679–684. doi: 10.1590/S0103-84782008000300013
Conson, A. R. O., Taniguti, C. H., Amadeu, R. R., Andreotti, I. A. A., De Souza, L. M., Dos Santos, L. H. B., et al. (2018). High-resolution genetic map and QTL analysis of growth-related traits of Hevea brasiliensis cultivated under suboptimal temperature and humidity conditions. Front. Plant Sci. 9:1255. doi: 10.3389/fpls.2018.01255
Csardi, G., and Nepusz, T. (2006). The igraph software package for complex network research. Int. J. Complex Syst. 1695, 1–9. doi: 10.1016/B978-0-12-813066-7.00009-7
D’haeseleer, P., Liang, S., and Somogyi, R. (2000). Genetic network inference: from co-expression clustering to reverse engineering. Bioinformatics 16, 707–726. doi: 10.1093/bioinformatics/16.8.707
Danecek, P., Auton, A., Abecasis, G., Albers, C. A., Banks, E., DePristo, M. A., et al. (2011). The variant call format and VCFtools. Bioinformatics 27, 2156–2158. doi: 10.1093/bioinformatics/btr330
De Faÿ, E., and Jacob, J. L. (1989). “Anatomical organization of the laticiferous system in the bark,” in Physiology of Rubber Tree Latex. eds. J. D’Auzac, J. Jacob, and H. Chrestin (Boca Raton, FL: CRC Press), 3–14.
De Souza, L. M., Dos Santos, L. H. B., Rosa, J., Da Silva, C. C., Mantello, C. C., Conson, A. R. O., et al. (2018). Linkage disequilibrium and population structure in wild and cultivated populations of rubber tree (Hevea brasiliensis). Front. Plant Sci. 9:815. doi: 10.3389/fpls.2018.00815
Deng, X., Wang, J., Li, Y., Wu, S., Yang, S., Chao, J., et al. (2018). Comparative transcriptome analysis reveals phytohormone signalings, heat shock module and ROS scavenger mediate the cold-tolerance of rubber tree. Sci. Rep. 8:4931. doi: 10.1038/s41598-018-23094-y
Diédhiou, C. J., Popova, O. V., Dietz, K. J., and Golldack, D. (2008). The SNF1-type serine-threonine protein kinase SAPK4 regulates stress-responsive gene expression in rice. BMC Plant Biol. 8:49. doi: 10.1186/1471-2229-8-49
Dijkman, M. (1951). Hevea. Thiry Years of Research in the Far East. Waltham, MA: University of Miami Press.
Ding, Z., Fu, L., Tan, D., Sun, X., and Zhang, J. (2020). An integrative transcriptomic and genomic analysis reveals novel insights into the hub genes and regulatory networks associated with rubber synthesis in H. brasiliensis. Ind. Crop. Prod. 153:112562. doi: 10.1016/j.indcrop.2020.112562
Dong, C., Chu, X., Wang, Y., Wang, Y., Jin, L., Shi, T., et al. (2008). Exploration of gene-gene interaction effects using entropy-based methods. Eur. J. Hum. Genet. 16, 229–235. doi: 10.1038/sj.ejhg.5201921
Dusotoit-Coucaud, A., Brunel, N., Kongsawadworakul, P., Viboonjun, U., Lacointe, A., Julien, J. L., et al. (2009). Sucrose importation into laticifers of Hevea brasiliensis, in relation to ethylene stimulation of latex production. Ann. Bot. 104, 635–647. doi: 10.1093/aob/mcp150
Elshire, R. J., Glaubitz, J. C., Sun, Q., Poland, J. A., Kawamoto, K., Buckler, E. S., et al. (2011). A robust, simple genotyping-by-sequencing (GBS) approach for high diversity species. PLoS One 6:e19379. doi: 10.1371/journal.pone.0019379
Figueiredo, J., Sousa Silva, M., and Figueiredo, A. (2018). Subtilisin-like proteases in plant defence: the past, the present and beyond. Mol. Plant Pathol. 19, 1017–1028. doi: 10.1111/mpp.12567
Fonseca, P. A. D. S., Id-Lahoucine, S., Reverter, A., Medrano, J. F., Fortes, M. S., Casellas, J., et al. (2018). Combining multi-OMICs information to identify key-regulator genes for pleiotropic effect on fertility and production traits in beef cattle. PLoS One 13:e0205295. doi: 10.1371/journal.pone.0205295
Fontaine, J. X., Saladino, F., Agrimonti, C., Bedu, M., Tercé-Laforgue, T., Tétu, T., et al. (2006). Control of the synthesis and subcellular targeting of the two GDH genes products in leaves and stems of Nicotiana plumbaginifolia and Arabidopsis thaliana. Plant Cell Physiol. 47, 410–418. doi: 10.1093/pcp/pcj008
Galicia, C., Mendoza-Hernández, G., and Rodríguez-Romero, A. (2015). Impact of the vulcanization process on the structural characteristics and IgE recognition of two allergens, Hev b 2 and Hev b 6.02, extracted from latex surgical gloves. Mol. Immunol. 65, 250–258. doi: 10.1016/j.molimm.2015.01.018
García-Fernández, C., Campa, A., Garzón, A. S., Miklas, P., and Ferreira, J. J. (2021). GWAS of pod morphological and color characters in common bean. BMC Plant Biol. 21:184. doi: 10.1186/s12870-021-02967-x
Gerard, D. (2020). Pairwise linkage disequilibrium estimation for polyploids. Mol. Ecol. Resour. 21, 1230–1242. doi: 10.1111/1755-0998.13349
Geserick, C., and Tenhaken, R. (2013). UDP-sugar pyrophosphorylase is essential for arabinose and xylose recycling, and is required during vegetative and reproductive growth in Arabidopsis. Plant J. 74, 239–247. doi: 10.1111/tpj.12116
Glaubitz, J. C., Casstevens, T. M., Lu, F., Harriman, J., Elshire, R. J., Sun, Q., et al. (2014). TASSEL-GBS: a high capacity genotyping by sequencing analysis pipeline. PLoS One 9:e90346. doi: 10.1371/journal.pone.0090346
Gonçalves, P. D. S., Bortoletto, N., Ortolani, A. A., Belletti, G. O., and Santos, W. R. D. (1999). Desempenho de novos clones de seringueira: III. seleções promissoras para a região de Votuporanga, Estado de São Paulo. Pesquisa Agropecuária
Gonçalves, P. S., and Fontes, J. R. A. (2012). “Domestication and breeding of rubber tree,” in Domestication and Breeding – Amazonian Species. eds. A. Borém, M. T. G. Lopes, C. R. C. Clement, and H. Noda (Viçosa, Brazil: Suprema Editora Ltda), 393–441.
Goncalves, P. D. S., Rossetti, A. G., Valois, A. C. C., and Viegas, I. D. J. (1984). Genetic and phenotypic correlations between some quantitative traits in juvenile clonal rubber trees (Hevea Spp.). Rev. Bras. Genet. 7, 95–107.
Gonçalves, P. D. S., Silva, M. D. A., Gouvêa, L. R. L., and Scaloppi Junior, E. J. (2006). Genetic variability for girth growth and rubber yield in Hevea brasiliensis. Sci. Agric. 63, 246–254. doi: 10.1590/S0103-90162006000300006
Grabherr, M. G., Haas, B. J., Yassour, M., Levin, J. Z., Thompson, D. A., Amit, I., et al. (2011). Full-length transcriptome assembly from RNA-Seq data without a reference genome. Nat. Biotechnol. 29, 644–652. doi: 10.1038/nbt.1883
Granato, I., and Fritsche-Neto, R. (2018). snpReady: Preparing Genotypic Datasets in Order to Run Genomic; Analysis. R Package Version 0.9.6. Available at: https://CRAN.R-project.org/package=snpReady. (Acessed July 24, 2020).
Haas, B. J. (2015). Trinotate: Transcriptome Functional Annotation and Analysis. Available at: https://github.com/Trinotate/Trinotate.github.io/wiki. (Acessed July 24, 2020).
Haas, B. J., Delcher, A. L., Mount, S. M., Wortman, J. R., Smith, R. K. Jr., Hannick, L. I., et al. (2003). Improving the Arabidopsis genome annotation using maximal transcript alignment assemblies. Nucleic Acids Res. 31, 5654–5666. doi: 10.1093/nar/gkg770
Hage, P., and Harary, F. (1995). Eccentricity and centrality in networks. Soc. Networks 17, 57–63. doi: 10.1016/0378-8733(94)00248-9
Hastie, T., Tibshirani, R., Balasubramanian, N., and Chu, G. (2001). Impute: Imputation for Microarray Data. Bioinform. 17, 520–525.
Hurtado Páez, U. A., García Romero, I. A., Restrepo Restrepo, S., Aristizábal Gutiérrez, F. A., and Montoya Castaño, D. (2015). Assembly and analysis of differential transcriptome responses of Hevea brasiliensis on interaction with Microcyclus ulei. PLoS One 10:e0134837. doi: 10.1371/journal.pone.0134837
Jaganathan, D., Ramasamy, K., Sellamuthu, G., Jayabalan, S., and Venkataraman, G. (2018). CRISPR for crop improvement: an update review. Front. Plant Sci. 9:985. doi: 10.3389/fpls.2018.00985
Jamil, I. N., Remali, J., Azizan, K. A., Nor Muhammad, N. A., Arita, M., Goh, H. H., et al. (2020). Systematic multi-omics integration (MOI) approach in plant systems biology. Front. Plant Sci. 11:944. doi: 10.3389/fpls.2020.00944
Jiang, J., Xing, F., Wang, C., Zeng, X., and Zou, Q. (2019). Investigation and development of maize fused network analysis with multi-omics. Plant Physiol. Biochem. 141, 380–387. doi: 10.1016/j.plaphy.2019.06.016
Johnson, M., Zaretskaya, I., Raytselis, Y., Merezhuk, Y., McGinnis, S., and Madden, T. L. (2008). NCBI BLAST: a better web interface. Nucleic Acids Res. 36, W5–W9. doi: 10.1093/nar/gkn201
Joyce, A. R., and Palsson, B. (2006). The model organism as a system: integrating ‘omics’ data sets. Nat. Rev. Mol. Cell Biol. 7, 198–210. doi: 10.1038/nrm1857
Kalunke, R. M., Tundo, S., Sestili, F., Camerlengo, F., Lafiandra, D., Lupi, R., et al. (2020). Reduction of allergenic potential in bread wheat RNAi transgenic lines silenced for CM3, CM16 and 0.28 ATI genes. Int. J. Mol. Sci. 21:5817. doi: 10.3390/ijms21165817
Kanehisa, M., and Goto, S. (2000). KEGG: Kyoto encyclopedia of genes and genomes. Nucleic Acids Res. 28, 27–30. doi: 10.1093/nar/28.1.27
Kashkush, K., Feldman, M., and Levy, A. A. (2003). Transcriptional activation of retrotransposons alters the expression of adjacent genes in wheat. Nat. Genet. 33, 102–106. doi: 10.1038/ng1063
Khan, M. A., Tong, F., Wang, W., He, J., Zhao, T., and Gai, J. (2018). Analysis of QTL-allele system conferring drought tolerance at seedling stage in a nested association mapping population of soybean [Glycine max (L.) Merr.] using a novel GWAS procedure. Planta 248, 947–962. doi: 10.1007/s00425-018-2952-4
Kim, D., Langmead, B., and Salzberg, S. L. (2015). HISAT: a fast spliced aligner with low memory requirements. Nat. Methods 12, 357–360. doi: 10.1038/nmeth.3317
Kleinberg, J. M. (1999). Hubs, authorities, and communities. ACM Comput. Surv. 31:5–es. doi: 10.1145/345966.345982
Korte, A., and Farlow, A. (2013). The advantages and limitations of trait analysis with GWAS: a review. Plant Methods 9, 1–9. doi: 10.1186/1746-4811-9-29
Kosová, K., Vítámvás, P., Urban, M. O., Klíma, M., Roy, A., and Prášil, I. T. (2015). Biological networks underlying abiotic stress tolerance in temperate crops: a proteomic perspective. Int. J. Mol. Sci. 16, 20913–20942. doi: 10.3390/ijms160920913
Kulwal, P. L. (2018). Trait mapping approaches through linkage mapping in plants. Adv. Biochem. Eng. Biotechnol. 164, 53–82. doi: 10.1007/10_2017_49
Langfelder, P., and Horvath, S. (2008). WGCNA: an R package for weighted correlation network analysis. BMC Bioinform. 9:559. doi: 10.1186/1471-2105-9-559
Langfelder, P., Zhang, B., and Horvath, S. (2008). Defining clusters from a hierarchical cluster tree: the dynamic tree cut package for R. Bioinformatics 24, 719–720. doi: 10.1093/bioinformatics/btm563
Langmead, B., and Salzberg, S. L. (2012). Fast gapped-read alignment with bowtie 2. Nat. Methods 9, 357–359. doi: 10.1038/nmeth.1923
Lee, K. W., San Woon, P., Teo, Y. Y., and Sim, K. (2012). Genome wide association studies (GWAS) and copy number variation (CNV) studies of the major psychoses: what have we learnt? Neurosci. Biobehav. Rev. 36, 556–571. doi: 10.1016/j.neubiorev.2011.09.001
Li, H., Handsaker, B., Wysoker, A., Fennell, T., Ruan, J., Homer, N., et al. (2009). The sequence alignment/map format and SAMtools. Bioinformatics 25, 2078–2079. doi: 10.1093/bioinformatics/btp352
Liu, X., Huang, M., Fan, B., Buckler, E. S., and Zhang, Z. (2016). Iterative usage of fixed and random effect models for powerful and efficient genome-wide association studies. PLoS Genet. 12:e1005767. doi: 10.1371/journal.pgen.1005767
Liu, H. J., Jian, L., Xu, J., Zhang, Q., Zhang, M., Jin, M., et al. (2020a). High-throughput CRISPR/Cas9 mutagenesis streamlines trait gene identification in maize. Plant Cell 32, 1397–1413. doi: 10.1105/tpc.19.00934
Liu, C., Ma, Y., Zhao, J., Nussinov, R., Zhang, Y. C., Cheng, F., et al. (2020b). Computational network biology: data, models, and applications. Phys. Rep. 846, 1–66. doi: 10.1016/j.physrep.2019.12.004
Liu, J., Shi, C., Shi, C. C., Li, W., Zhang, Q. J., Zhang, Y., et al. (2020c). The chromosome-based rubber tree genome provides new insights into spurge genome evolution and rubber biosynthesis. Mol. Plant 13, 336–350. doi: 10.1016/j.molp.2019.10.017
Mahfouz, M. M., Kim, S., Delauney, A. J., and Verma, D. P. (2006). Arabidopsis TARGET OF RAPAMYCIN interacts with RAPTOR, which regulates the activity of S6 kinase in response to osmotic stress signals. Plant Cell 18, 477–490. doi: 10.1105/tpc.105.035931
Manolio, T. A., Collins, F. S., Cox, N. J., Goldstein, D. B., Hindorff, L. A., Hunter, D. J., et al. (2009). Finding the missing heritability of complex diseases. Nature 461, 747–753. doi: 10.1038/nature08494
Mantello, C. C., Boatwright, L., da Silva, C. C., Scaloppi, E. J., de Souza Goncalves, P., Barbazuk, W. B., et al. (2019). Deep expression analysis reveals distinct cold-response strategies in rubber tree (Hevea brasiliensis). BMC Genomics 20:455. doi: 10.1186/s12864-019-5852-5
Mantello, C. C., Cardoso-Silva, C. B., Da Silva, C. C., De Souza, L. M., Scaloppi Junior, E. J., De Souza Gonçalves, P., et al. (2014). De novo assembly and transcriptome analysis of the rubber tree (Hevea brasiliensis) and SNP markers development for rubber biosynthesis pathways. PLoS One 9:e102665. doi: 10.1371/journal.pone.0102665
Maslov, S., and Sneppen, K. (2002). Specificity and stability in topology of protein networks. Science 296, 910–913. doi: 10.1126/science.1065103
Matsunaga, W., Ohama, N., Tanabe, N., Masuta, Y., Masuda, S., Mitani, N., et al. (2015). A small RNA mediated regulation of a stress-activated retrotransposon and the tissue specific transposition during the reproductive period in Arabidopsis. Front. Plant Sci. 6:48. doi: 10.3389/fpls.2015.00048
Mou, J., Zhang, Z., Qiu, H., Lu, Y., Zhu, X., Fan, Z., et al. (2021). Multiomics-based dissection of citrus flavonoid metabolism using a Citrus reticulata × Poncirus trifoliata population. Hortic. Res. 8:56. doi: 10.1038/s41438-021-00472-8
Munõz, F., and Sanchez, L. (2017). breedR: Statistical Methods for Forest Genetic; Resources Analysts. R Package Version 0.12–2. Available at: https://github.com/famuvie/breedR (Accessed November 2021).
Myles, S., Peiffer, J., Brown, P. J., Ersoz, E. S., Zhang, Z., Costich, D. E., et al. (2009). Association mapping: critical considerations shift from genotyping to experimental design. Plant Cell 21, 2194–2202. doi: 10.1105/tpc.109.068437
Nebel, A., Kleindorp, R., Caliebe, A., Nothnagel, M., Blanché, H., Junge, O., et al. (2011). A genome-wide association study confirms APOE as the major gene influencing survival in long-lived individuals. Mech. Ageing Dev. 132, 324–330. doi: 10.1016/j.mad.2011.06.008
Nguyen, K. L., Grondin, A., Courtois, B., and Gantet, P. (2019). Next-generation sequencing accelerates crop gene discovery. Trends Plant Sci. 24, 263–274. doi: 10.1016/j.tplants.2018.11.008
Ohashi, Y., Nakayama, N., Saneoka, H., and Fujita, K. (2006). Effects of drought stress on photosynthetic gas exchange, chlorophyll fluorescence and stem diameter of soybean plants. Biol. Plant. 50, 138–141. doi: 10.1007/s10535-005-0089-3
Peláez, P., Ortiz-Martínez, A., Figueroa-Corona, L., Montes, J. R., and Gernandt, D. S. (2020). Population structure, diversifying selection, and local adaptation in Pinus patula. Am. J. Bot. 107, 1555–1566. doi: 10.1002/ajb2.1566
Pérez-Bercoff, Å., McLysaght, A., and Conant, G. C. (2011). Patterns of indirect protein interactions suggest a spatial organization to metabolism. Mol. BioSyst. 7, 3056–3064. doi: 10.1039/c1mb05168g
Pinu, F. R., Beale, D. J., Paten, A. M., Kouremenos, K., Swarup, S., Schirra, H. J., et al. (2019). Systems biology and multi-omics integration: viewpoints from the metabolomics research community. Meta 9:76. doi: 10.3390/metabo9040076
Poland, J. A., and Rife, T. W. (2012). Genotyping-by-sequencing for plant breeding and genetics. Plant Genome 5, 92–102. doi: 10.3835/plantgenome2012.05.0005
Pootakham, W., Ruang-Areerate, P., Jomchai, N., Sonthirod, C., Sangsrakru, D., Yoocha, T., et al. (2015). Construction of a high-density integrated genetic linkage map of rubber tree (Hevea brasiliensis) using genotyping-by-sequencing (GBS). Front. Plant Sci. 6:367. doi: 10.3389/fpls.2015.00367
Pootakham, W., Shearman, J. R., and Tangphatsornruang, S. (2020). “Development of molecular markers in Hevea brasiliensis for marker-assisted breeding,” in The Rubber Tree Genome. eds. M. Matsui and K. S. Chow (Cham: Springer International Publishing), 67–79.
Pootakham, W., Sonthirod, C., Naktang, C., Ruang-Areerate, P., Yoocha, T., Sangsrakru, D., et al. (2017). De novo hybrid assembly of the rubber tree genome reveals evidence of paleotetraploidy in Hevea species. Sci. Rep. 7:41457. doi: 10.1038/srep41457
Priyadarshan, P. (2003). Contributions of weather variables for specific adaptation of rubber tree (Hevea brasiliensis Muell.-Arg) clones. Genet. Mol. Biol. 26, 435–440. doi: 10.1590/S1415-47572003000400006
Priyadarshan, P. M. (2017). Refinements to Hevea rubber breeding. Tree Genet. Genomes 13:20. doi: 10.1007/s11295-017-1101-8
Priyadarshan, P. M., and Clément-Demange, A. (2004). Breeding Hevea rubber: formal and molecular genetics. Adv. Genet. 52, 51–115. doi: 10.1016/s0065-2660(04)52003-5
Qiu, X., Xie, W., Lian, X., and Zhang, Q. (2009). Molecular analyses of the rice glutamate dehydrogenase gene family and their response to nitrogen and phosphorous deprivation. Plant Cell Rep. 28, 1115–1126. doi: 10.1007/s00299-009-0709-z
Racolta, A., Bryan, A. C., and Tax, F. E. (2014). The receptor-like kinases GSO1 and GSO2 together regulate root growth in Arabidopsis through control of cell division and cell fate specification. Dev. Dyn. 243, 257–278. doi: 10.1002/dvdy.24066
Rao, X., and Dixon, R. A. (2019). Co-expression networks for plant biology: why and how. Acta Biochim. Biophys. Sin. 51, 981–988. doi: 10.1093/abbs/gmz080
Rao, G. P., and Kole, P. C. (2016). Evaluation of Brazilian wild Hevea germplasm for cold tolerance: genetic variability in the early mature growth. J. For. Res. 27, 755–765. doi: 10.1007/s11676-015-0188-8
Rautengarten, C., Steinhauser, D., Büssis, D., Stintzi, A., Schaller, A., Kopka, J., et al. (2005). Inferring hypotheses on functional relationships of genes: analysis of the Arabidopsis thaliana subtilase gene family. PLoS Comput. Biol. 1:e40. doi: 10.1371/journal.pcbi.0010040
Ray, D., Behera, M. D., and Jacob, J. (2016). Predicting the distribution of rubber trees (Hevea brasiliensis) through ecological niche modelling with climate, soil, topography and socioeconomic factors. Ecol. Res. 31, 75–91. doi: 10.1007/s11284-015-1318-7
Restivo, F. M. (2004). Molecular cloning of glutamate dehydrogenase genes of Nicotiana plumbaginifolia: structure analysis and regulation of their expression by physiological and stress conditions. Plant Sci. 166, 971–982. doi: 10.1016/j.plantsci.2003.12.011
Ritchie, M. D., Hahn, L. W., Roodi, N., Bailey, L. R., Dupont, W. D., Parl, F. F., et al. (2001). Multifactor-dimensionality reduction reveals high-order interactions among estrogen-metabolism genes in sporadic breast cancer. Am. J. Hum. Genet. 69, 138–147. doi: 10.1086/321276
Robinson, M. D., McCarthy, D. J., and Smyth, G. K. (2010). edgeR: a bioconductor package for differential expression analysis of digital gene expression data. Bioinformatics 26, 139–140. doi: 10.1093/bioinformatics/btp616.
Romain, B., and Thierry, C. (2011). Rubber Clones (Hevea Clonal Descriptions). Available at: http://rubberclones.cirad.fr (Acessed July 24, 2020).
Rosa, J., Mantello, C. C., Garcia, D., De Souza, L. M., Da Silva, C. C., Gazaffi, R., et al. (2018). QTL detection for growth and latex production in a full-sib rubber tree population cultivated under suboptimal climate conditions. BMC Plant Biol. 18:223. doi: 10.1186/s12870-018-1450-y
Rudrabhatla, P., and Rajasekharan, R. (2002). Developmentally regulated dual-specificity kinase from peanut that is induced by abiotic stresses. Plant Physiol. 130, 380–390. doi: 10.1104/pp.005173
Sathik, M. B. M., Luke, L. P., Rajamani, A., Kuruvilla, L., Sumesh, K. V., and Thomas, M. (2018). De novo transcriptome analysis of abiotic stress-responsive transcripts of Hevea brasiliensis. Mol. Breed. 38:32. doi: 10.1007/s11032-018-0782-5
Schaefer, R. J., Michno, J. M., Jeffers, J., Hoekenga, O., Dilkes, B., Baxter, I., et al. (2018). Integrating coexpression networks with GWAS to prioritize causal genes in maize. Plant Cell 30, 2922–2942. doi: 10.1105/tpc.18.00299
Schnurr, J. A., Storey, K. K., Jung, H. J. G., Somers, D. A., and Gronwald, J. W. (2006). UDP-sugar pyrophosphorylase is essential for pollen development in Arabidopsis. Planta 224, 520–532. doi: 10.1007/s00425-006-0240-1
Scossa, F., Alseekh, S., and Fernie, A. R. (2021). Integrating multi-omics data for crop improvement. J. Plant Physiol. 257:153352. doi: 10.1016/j.jplph.2020.153352
Shannon, P., Markiel, A., Ozier, O., Baliga, N. S., Wang, J. T., Ramage, D., et al. (2003). Cytoscape: a software environment for integrated models of biomolecular interaction networks. Genome Res. 13, 2498–2504. doi: 10.1101/gr.1239303
Shearman, J. R., Sangsrakru, D., Ruang-Areerate, P., Sonthirod, C., Uthaipaisanwong, P., Yoocha, T., et al. (2014). Assembly and analysis of a male sterile rubber tree mitochondrial genome reveals DNA rearrangement events and a novel transcript. BMC Plant Biol. 14:45. doi: 10.1186/1471-2229-14-45
Singh, G., Ratnaparkhe, M., and Kumar, A. (2019). Comparative analysis of transposable elements from Glycine max, Cajanus cajan and Phaseolus vulgaris. J. Exp. Biol. Agric. Sci. 7, 167–177. doi: 10.18006/2019.7(2).167.177
Sivakumaran, S., Haridas, G., and Abraham, P. D. (1988). Problem of tree dryness with high yielding precocious clones and methods to exploit such clones. Proc. Coll. Hevea 88, 253–267.
Souza, L. M., Francisco, F. R., Gonçalves, P. S., Scaloppi Junior, E. J., Le Guen, V., Fritsche-Neto, R., et al. (2019). Genomic selection in rubber tree breeding: a comparison of models and methods for managing G×E interactions. Front. Plant Sci. 10:1353. doi: 10.3389/fpls.2019.01353
Souza, L. M., Gazaffi, R., Mantello, C. C., Silva, C. C., Garcia, D., Le Guen, V., et al. (2013). QTL mapping of growth-related traits in a full-sib family of rubber tree (Hevea brasiliensis) evaluated in a sub-tropical climate. PLoS One 8:e61238. doi: 10.1371/journal.pone.0061238
Stuart, T., Eichten, S. R., Cahn, J., Karpievitch, Y. V., Borevitz, J. O., and Lister, R. (2016). Population scale mapping of transposable element diversity reveals links to gene regulation and epigenomic variation. elife 5:e20777. doi: 10.7554/eLife.20777
Supek, F., Bošnjak, M., Škunca, N., and Šmuc, T. (2011). REVIGO summarizes and visualizes long lists of gene ontology terms. PLoS One 6:e21800. doi: 10.1371/journal.pone.0021800
Taji, T., Ohsumi, C., Iuchi, S., Seki, M., Kasuga, M., Kobayashi, M., et al. (2002). Important roles of drought- and cold-inducible genes for galactinol synthase in stress tolerance in Arabidopsis thaliana. Plant J. 29, 417–426. doi: 10.1046/j.0960-7412.2001.01227.x
Tam, V., Patel, N., Turcotte, M., Bossé, Y., Paré, G., and Meyre, D. (2019). Benefits and limitations of genome-wide association studies. Nat. Rev. Genet. 20, 467–484. doi: 10.1038/s41576-019-0127-1
Tang, C., Yang, M., Fang, Y., Luo, Y., Gao, S., Xiao, X., et al. (2016). The rubber tree genome reveals new insights into rubber production and species adaptation. Nat. Plants 2:16073. doi: 10.1038/nplants.2016.73
Tapia-López, R., García-Ponce, B., Dubrovsky, J. G., Garay-Arroyo, A., Pérez-Ruíz, R. V., Kim, S. H., et al. (2008). An AGAMOUS-related MADS-box gene, XAL1 (AGL12), regulates root meristem cell proliferation and flowering transition in Arabidopsis. Plant Physiol. 146, 1182–1192. doi: 10.1104/pp.107.108647
Tenesa, A., Wright, A. F., Knott, S. A., Carothers, A. D., Hayward, C., Angius, A., et al. (2004). Extent of linkage disequilibrium in a Sardinian sub-isolate: sampling and methodological considerations. Hum. Mol. Genet. 13, 25–33. doi: 10.1093/hmg/ddh001
Tercé-Laforgue, T., Clément, G., Marchi, L., Restivo, F. M., Lea, P. J., and Hirel, B. (2015). Resolving the role of plant NAD-glutamate dehydrogenase: III. Overexpressing individually or simultaneously the two enzyme subunits under salt stress induces changes in the leaf metabolic profile and increases plant biomass production. Plant Cell Physiol. 56, 1918–1929. doi: 10.1093/pcp/pcv114
Tian, M., Benedetti, B., and Kamoun, S. (2005). A second kazal-like protease inhibitor from Phytophthora infestans inhibits and interacts with the apoplastic pathogenesis-related protease P69B of tomato. Plant Physiol. 138, 1785–1793. doi: 10.1104/pp.105.061226
Tran, K. N., and Choi, J. I. (2020). Comparative transcriptome analysis of high-growth and wild-type strains of Pyropia yezoensis. Acta Bot. Croat. 79, 148–156. doi: 10.37427/botcro-2020-020
Traylor-Knowles, N., Rose, N. H., Sheets, E. A., and Palumbi, S. R. (2017). Early transcriptional responses during heat stress in the coral Acropora hyacinthus. Biol. Bull. 232, 91–100. doi: 10.1086/692717
Trivedi, U. H., Cézard, T., Bridgett, S., Montazam, A., Nichols, J., Blaxter, M., et al. (2014). Quality control of next-generation sequencing data without a reference. Front. Genet. 5:111. doi: 10.3389/fgene.2014.00111
Valdés, A., Ibáñez, C., Simó, C., and García-Cañas, V. (2013). Recent transcriptomics advances and emerging applications in food science. TrAC Trends Anal. Chem. 52, 142–154. doi: 10.1016/j.trac.2013.06.014
Verzegnazzi, A. L., Dos Santos, I. G., Krause, M. D., Hufford, M., Frei, U. K., Campbell, J., et al. (2021). Major locus for spontaneous haploid genome doubling detected by a case-control GWAS in exotic maize germplasm. Theor. Appl. Genet. 134, 1423–1434. doi: 10.1007/s00122-021-03780-8
Voorrips, R. E. (2002). MapChart: software for the graphical presentation of linkage maps and QTLs. J. Hered. 93, 77–78. doi: 10.1093/jhered/93.1.77
Vranova, V., Zahradnickova, H., Janous, D., Skene, K. R., Matharu, A. S., Rejsek, K., et al. (2012). The significance of D-amino acids in soil, fate and utilization by microbes and plants: review and identification of knowledge gaps. Plant Soil 354, 21–39. doi: 10.1007/s11104-011-1059-5
Wan, D., Li, R., Zou, B., Zhang, X., Cong, J., Wang, R., et al. (2012). Calmodulin-binding protein CBP60g is a positive regulator of both disease resistance and drought tolerance in Arabidopsis. Plant Cell Rep. 31, 1269–1281. doi: 10.1007/s00299-012-1247-7
Wang, J., Lu, N., Yi, F., and Xiao, Y. (2020). Identification of transposable elements in conifer and their potential application in breeding. Evol. Bioinforma. 16:1176934320930263. doi: 10.1177/1176934320930263
Wang, Q., Wang, K., Wu, W., Giannoulatou, E., Ho, J. W. K., and Li, L. (2019). Host and microbiome multi-omics integration: applications and methodologies. Biophys. Rev. 11, 55–65. doi: 10.1007/s12551-018-0491-7
Warraich, A. S., Krishnamurthy, S. L., Sooch, B. S., Vinaykumar, N. M., Dushyanthkumar, B. M., Bose, J., et al. (2020). Rice GWAS reveals key genomic regions essential for salinity tolerance at reproductive stage. Acta Physiol. Plant. 42:134. doi: 10.1007/s11738-020-03123-y
Watanabe, Y., Lino, Y., Furuhata, K., Shimoda, C., and Yamamoto, M. (1988). The S.pombe mei2 gene encoding a crucial molecule for commitment to meiosis is under the regulation of cAMP. EMBO J. 7, 761–767. doi: 10.1002/j.1460-2075.1988.tb02873.x
Watanabe, K., Taskesen, E., Van Bochoven, A., and Posthuma, D. (2017). Functional mapping and annotation of genetic associations with FUMA. Nat. Commun. 8:1826. doi: 10.1038/s41467-017-01261-5
Watts, D. J., and Strogatz, S. H. (1998). Collective dynamics of ‘small-world’ networks. Nature 393, 440–442. doi: 10.1038/30918
Wei, Z., Yuan, Q., Lin, H., Li, X., Zhang, C., Gao, H., et al. (2021). Linkage analysis, GWAS, transcriptome analysis to identify candidate genes for rice seedlings in response to high temperature stress. BMC Plant Biol. 21:85. doi: 10.1186/s12870-021-02857-2
Wu, A., Allu, A. D., Garapati, P., Siddiqui, H., Dortay, H., Zanor, M. I., et al. (2012). JUNGBRUNNEN1, a reactive oxygen species-responsive NAC transcription factor, regulates longevity in Arabidopsis. Plant Cell 24, 482–506. doi: 10.1105/tpc.111.090894
Wu, S., Guyot, R., Bocs, S., Droc, G., Oktavia, F., Hu, S., et al. (2020a). Structural and functional annotation of transposable elements revealed a potential regulation of genes involved in rubber biosynthesis by TE-derived siRNA interference in Hevea brasiliensis. Int. J. Mol. Sci. 21:4220. doi: 10.3390/ijms21249440
Wu, L., Han, L., Li, Q., Wang, G., Zhang, H., and Li, L. (2020b). Using interactome big data to crack genetic mysteries and enhance future crop breeding. Mol. Plant 14, 77–94. doi: 10.1016/j.molp.2020.12.012
Wu, X., Jin, L., and Xiong, M. (2008). Composite measure of linkage disequilibrium for testing interaction between unlinked loci. Eur. J. Hum. Genet. 16, 644–651. doi: 10.1038/sj.ejhg.5202004
Wu, S., Zhang, M., Yang, X., Peng, F., Zhang, J., Tan, J., et al. (2018). Genome-wide association studies and CRISPR/Cas9-mediated gene editing identify regulatory variants influencing eyebrow thickness in humans. PLoS Genet. 14:e1007640. doi: 10.1371/journal.pgen.1007640
Wydau, S., Ferri-Fioni, M. L., Blanquet, S., and Plateau, P. (2007). GEK1, a gene product of Arabidopsis thaliana involved in ethanol tolerance, is a D-aminoacyl-tRNA deacylase. Nucleic Acids Res. 35, 930–938. doi: 10.1093/nar/gkl1145
Xia, Z., Liu, K., Zhang, S., Yu, W., Zou, M., He, L., et al. (2018). An ultra-high density map allowed for mapping QTL and candidate genes controlling dry latex yield in rubber tree. Ind. Crop. Prod. 120, 351–356. doi: 10.1016/j.indcrop.2018.04.057
Xu, Y., and Crouch, J. H. (2008). Marker-assisted selection in plant breeding: from publications to practice. Crop Sci. 48, 391–407. doi: 10.2135/cropsci2007.04.0191
Yan, Z., Huang, H., Freebern, E., Santos, D. J., Dai, D., Si, J., et al. (2020). Integrating RNA-Seq with GWAS reveals novel insights into the molecular mechanism underpinning ketosis in cattle. BMC Genomics 21:489. doi: 10.1186/s12864-020-06909-z
Yang, J., Benyamin, B., McEvoy, B. P., Gordon, S., Henders, A. K., Nyholt, D. R., et al. (2010). Common SNPs explain a large proportion of the heritability for human height. Nat. Genet. 42, 565–569. doi: 10.1038/ng.608
Yuan, Z., Gao, Q., He, Y., Zhang, X., Li, F., Zhao, J., et al. (2012). Detection for gene-gene co-association via kernel canonical correlation analysis. BMC Genet. 13:83. doi: 10.1186/1471-2156-13-83
Zeh, M., Casazza, A. P., Kreft, O., Roessner, U., Bieberich, K., Willmitzer, L., et al. (2001). Antisense inhibition of threonine synthase leads to high methionine content in transgenic potato plants. Plant Physiol. 127, 792–802.
Zhang, H., Chu, Y., Dang, P., Tang, Y., Jiang, T., Clevenger, J. P., et al. (2020). Identification of QTLs for resistance to leaf spots in cultivated peanut (Arachis hypogaea L.) through GWAS analysis. Theor. Appl. Genet. 133, 2051–2061. doi: 10.1007/s00122-020-03576-2
Zhang, B., and Horvath, S. (2005). A general framework for weighted gene co-expression network analysis. Stat. Appl. Genet. Mol. Biol. 4:7. doi: 10.2202/1544-6115.1128
Zhang, J., Khan, S. A., Heckel, D. G., and Bock, R. (2017). Next-generation insect-resistant plants: RNAi-mediated crop protection. Trends Biotechnol. 35, 871–882. doi: 10.1016/j.tibtech.2017.04.009
Zhang, W., Li, F., and Nie, L. (2010). Integrating multiple ‘omics’ analysis for microbial biology: application and methodologies. Microbiology 156, 287–301. doi: 10.1099/mic.0.034793-0
Zhang, C., Stratopoulos, L. M. F., Pretzsch, H., and Rötzer, T. (2019a). How do Tilia cordata Greenspire trees cope with drought stress regarding their biomass allocation and ecosystem services? Forests 10:676.
Zheng, F., Zhang, S., Churas, C., Pratt, D., Bahar, I., and Ideker, T. (2021). HiDeF: identifying persistent structures in multiscale ‘omics data. Genome Biol. 22:21. doi: 10.1186/s13059-020-02228-4
Zhu, J. K. (2016). Abiotic stress signaling and responses in plants. Cell 167, 313–324. doi: 10.1016/j.cell.2016.08.029
Glossary
Keywords: GBS, GWAS, Hevea brasiliensis, linkage disequilibrium, metabolic networks, QTL, RNA-Seq, WGCNA
Citation: Francisco FR, Aono AH, da Silva CC, Gonçalves PS, Scaloppi Junior EJ, Le Guen V, Fritsche-Neto R, Souza LM and Souza AP (2021) Unravelling Rubber Tree Growth by Integrating GWAS and Biological Network-Based Approaches. Front. Plant Sci. 12:768589. doi: 10.3389/fpls.2021.768589
Edited by:
Kun Lu, Southwest University, ChinaReviewed by:
Zitong Li, Commonwealth Scientific and Industrial Research Organisation (CSIRO), AustraliaRodrigo Iván Contreras-Soto, Universidad de O'Higgins, Chile
Bindu Roy, Rubber Research Institute of India, India
Copyright © 2021 Francisco, Aono, da Silva, Gonçalves, Scaloppi Junior, Le Guen, Fritsche-Neto, Souza and de Souza. This is an open-access article distributed under the terms of the Creative Commons Attribution License (CC BY). The use, distribution or reproduction in other forums is permitted, provided the original author(s) and the copyright owner(s) are credited and that the original publication in this journal is cited, in accordance with accepted academic practice. No use, distribution or reproduction is permitted which does not comply with these terms.
*Correspondence: Anete Pereira de Souza, anete@unicamp.br
†These authors have contributed equally to this work