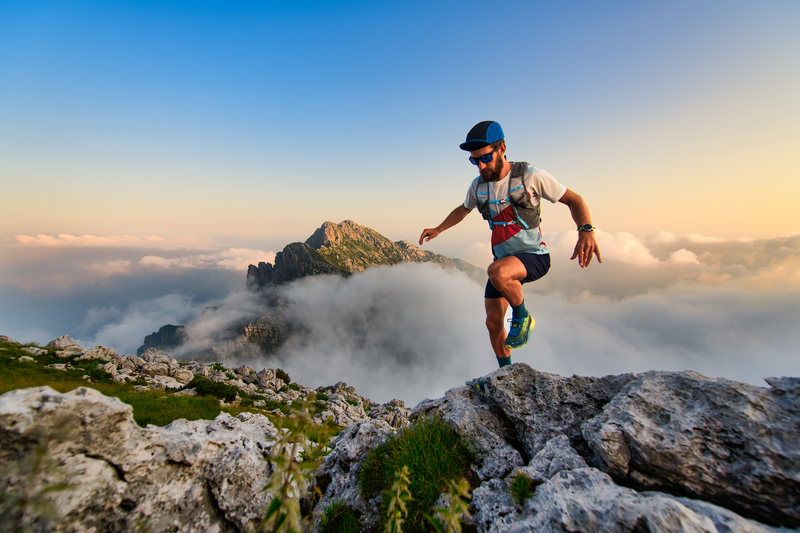
94% of researchers rate our articles as excellent or good
Learn more about the work of our research integrity team to safeguard the quality of each article we publish.
Find out more
ORIGINAL RESEARCH article
Front. Plant Sci. , 23 November 2021
Sec. Plant Breeding
Volume 12 - 2021 | https://doi.org/10.3389/fpls.2021.758221
In forest tree breeding, assisted migration has been proposed to accelerate the adaptive response to climate change. Response functions are currently fitted across multiple populations and environments, enabling selections of the most appropriate seed sources for a specific reforestation site. So far, the approach has been limited to capturing adaptive variation among populations, neglecting tree-to-tree variation residing within a population. Here, we combined the response function methodology with the in-situ breeding approach, utilizing progeny trials of European larch (Larix decidua) across 21 test sites in Austria ranging from Alpine to lowland regions. We quantified intra-population genetic variance and predicted individual genetic performance along a climatic gradient. This approach can be adopted in most breeding and conservation programs, boosting the speed of adaptation under climate change.
Global temperature is likely to increase up to 1.5–2°C by the end of the century (Lindner et al., 2014; Pachauri et al., 2014) along with an increased frequency and intensity of extreme events (Dai, 2011; Trenberth et al., 2014; Seidl et al., 2017; Senf and Seidl, 2021). Due to global warming and the increased atmospheric CO2, there is a positive trend in overall forest productivity, mainly when water restriction is not a limiting factor (Boisvenue and Running, 2006). While the above boost in productivity is anticipated in Northern and Western European regions, the Southern counterparts seem more threatened by intensified drought events that may decrease survival and productivity (Lindner et al., 2010). Climate is one of the primary factors influencing local adaptation (Howe et al., 2003; Savolainen et al., 2007). Faced with unfavorable changes in environmental conditions, tree populations can either persist, migrate or go extinct (Aitken et al., 2008). Both persistence and natural migration rates may not sufficiently cope with the predicted rate of climate change (CC) (Davis and Shaw, 2001; Malcolm et al., 2002; McLachlan and Clark, 2004; Richter et al., 2012; Dyderski et al., 2018). Therefore, to reduce the impact of CC on forests, there is an urgent need to understand and secure genetic variation to support future adaptation.
Tree species are known for high levels of genetic variation across vast geographical ranges; genetic differences are observed at different hierarchical levels (among species, populations, to individual trees). Additive genetic variance () is a product of respective allelic frequencies and their direct biochemical effects on individual phenotypes (Falconer and Mackay, 1996). The genetic rate of adaptive response to natural selection (i.e., the directional change in fitness) is directly attributable to the current (Fisher, 1930). Genetic variation in forest trees has been substantially investigated in line with evolutionary forces, human intervention and environmental change, and explored in tree breeding programs for over a half-century, even though selection in these programs has primarily focused on economically important traits (e.g., height, straightness, or disease resistance) (White et al., 2007). CC may imposes a severe constraint to the directional natural (and artificial) selection. Assisted migration could speed up the selection response in adaptive traits (Gougherty et al., 2021; Sáenz-Romero et al., 2021; St-Laurent et al., 2021).
Since the 90s, apart from conventional tree breeding, the correlation of quantitative traits with climatic variables has been studied in several economically important tree species using pre-existing provenance trials (Mátyás, 1994; Schmidtling, 1994; Rehfeldt et al., 1999). Typical provenance trials are composed of specific test sites where several populations (provenances) originating throughout the species range are planted. Response functions have been applied on provenance trial data to investigate the intraspecific genetic adaptation to climatic conditions. Two types of functions have been used: (i) the Transfer Function (Mátyás, 1994; Thomson and Parker, 2008; O'Neill et al., 2014), and (ii) the Response Function (Rehfeldt et al., 1999; Wang et al., 2006; Kapeller et al., 2012). The former is used to analyze the performance of several provenances in a specific environment. The latter tests the performance of a particular provenance across a range of planting conditions across different sites. Thus, transfer functions are based on the genetic drivers, and response functions are based on the environmental drivers of variation in fitness-related traits. Combined with future climatic scenarios, these functions can provide estimates of the impact of CC on forests. They can be used as a decision support tool for seed delineation zones and assisted migration (Leites et al., 2012).
As outlined above, response functions have been proposed to capture adaptive variation at the population (provenance) level, unlike the conventional breeding focusing mainly on intra-population . We combine genetic evaluation and the response function methodology to capture intrapopulation adaptive response across environmental gradients. We demonstrate the methodology using progeny trials of European larch (Larix decidua) from 21 test sites in Austria ranging from Alpine to lowland regions. We utilized height and wood density measured directly in forest stands on individual mature trees with reconstructed pedigree. Using the response function methodology combined with mixed-model genetic evaluation, we quantified the intra-population matching specific genetically adapted trees to specific climatic variables. This approach can be adopted in most forest tree species, boosting the speed of adaptation under CC while overcoming the practical limitations of traditional breeding and conservation programs.
The European larch data used in this study were previously sampled and genetically analyzed by Lstibůrek et al. (2020). We will briefly outline their methodology related to the current investigation. Originating from a local European Larch provenance, a clonal seed orchard was established in 1954 in the Northern Alpine region by grafting 53 phenotypically superior parental trees. The orchard has served as a major seed source for afforestation activities in the region. Newly established forest stands comprise individual half-siblings, i.e., offspring from random mating (open-pollination) among parental trees in the orchard. One-half of the respective parentage (i.e., paternal gametic contributions) has originated from unknown trees within the orchard. In 2018, 21 of these forest stands (Figure 1, OpenStreetMap contributors, 2021) were selected for phenotyping and genotyping, yielding potential breeding candidates (25 to 37 years old). All sites were situated at altitudes ranging from 280 to 760 m. Over 4,000 individuals were measured for height and wood density, and 1,253 of them, plus the 53 parental trees, had their DNA extracted for microsatellite analysis. A pedigree was then reconstructed using the likelihood-based method implemented in the Cervus software (Marshall et al., 1998). The investigation revealed a marginal 8.4% of parental contributions outside the orchard (i.e., pollen contamination). In total, 491 full-sib families were assembled, representing 35% of the possible 53-parent half-diallel mating scheme. This assembled pedigree (Lstibůrek et al., 2020) constitutes the basis for our subsequent analyses.
Figure 1. Map of the 21 testing sites (blue dots) spread accross North-Eastern Austria. A darker blue color means that sites are overlaping at this scale.
We extracted climatic variables for the test sites from the WORDCLIM dataset (Hijmans et al., 2005). The dataset covers a period from 1950 to 2000 with a 1km spatial resolution of 1 km. It includes monthly temperature means, minima, maxima, monthly precipitation sums, seasonal and annual temperatures, and precipitation variables. Secondly, we used the random forest model (Breiman, 2001) to identify the most important variables. The random forest provides two types of importance measures, the mean decrease in accuracy and the mean decrease in node impurity (Liaw and Wiener, 2002). We selected the most recurring variables over several runs of the model. Afterward, we checked these variables for collinearity, and we plotted preliminary response functions (as explained in section 2.4) to single out the most important variable.
We conducted all statistical analyses in R (R Core Team, 2020) and Rstudio (RStudio Team, 2020). We utilized the mixed-model genetic evaluation protocol implemented within the ASReml-R (Butler et al., 2017). Individual tree height was divided by the respective stand age to obtain mean annual increment (MAI) values comparable across all sites. MAI phenotypic values were jointly analyzed with wood density in the bivariate animal genetic model following the original protocol by Henderson (1984).
where y is the vector of bivariate phenotypic observations; X is the incidence matrix for the fixed effect b (trait and site means); Z is the genetic relationship matrix; a is the vector of additive genetic (breeding) values, , and the random residual effects are distributed as . The covariance matrix for the random additive genetic effects was modeled using the heterogeneous covariance structure as
where A is the average numerator relationship matrix, σa1a2 is the additive genetic covariance between traits 1 and 2, and ⊗ is the Kronecker product operator. The random residual error effect was modeled using an unstructured covariance matrix structure as
where σe1e2 is the residual covariance between the two traits. Random effects were assumed to be independent.
We utilized the above predictions of the fixed site effects and calculated the respective all-pairwise differences. Next, we trimmed the dataset so that each half-sib family was represented in at least six sites to achieve even representation of families while maximizing their distribution across multiples sites (see Kapeller et al., 2012; Foff et al., 2014; Suvanto et al., 2016 for a similar number of test sites). For each individual, we calculated the predicted phenotypic performance for the MAI (further denoted as PMAI) as a sum of the overall mean, respective site effect, and the individual additive genetic (breeding) value (BV) from the bivariate additive genetic model.
We developed individual- and population-level univariate response functions (RF) to describe the within-population genetic variation following major climatic gradients. We tested the linear, quadratic, and Gaussian models as they have been predominantly used in previous studies (Wang et al., 2006; O'Neill et al., 2007, 2014; Leites et al., 2012; Sáenz-Romero et al., 2017). The linear model did not yield a significant fit. Contrastingly, both the quadratic and Gaussian models showed significant fit. The shape of the response function was almost identical, and so was Akaike Information Criterion (AIC). Below, we present the quadratic model that we selected.
where v is the estimated mean height at the site j for parent k; β0, β1, and β2 are regression coefficients; c is the climatic variable at planting site j.
Using the bivariate animal genetic model, we obtained significant narrow-sense heritability for height (h2 = 0.27, SE = 0.07) and wood density (h2 = 0.30, SE = 0.07), respectively. We observed negligible additive genetic correlation between the respective traits (ra = 0.09, SE = 0.20). Our data did not show a significant genotype by environment interaction (GxE), as shown earlier by Lstibůrek et al. (2020). Summary of the model fit statistics (full and reduced model) are provided in Supplementary Table S2.
Experimental site effects (considered fixed) were found statistically significant (p < 0.01). 87% of all pairwise differences between sites were statistically significant (p < 0.05). Details are provided in Supplementary Table S1. In Figure 2, BVs for MAI are plotted with their respective confidence intervals showing the extent of additive genetic variation present within a random set of 31 parents. The BVs are ranging from –5 to 6.4 cm (average BV is zero).
Figure 2. Parental trees are sorted by additive genetic values. The BVs (dots) are expressed in the units of measurement (cm/year). 95% approximate confidence intervals (dashed line) were calculated as two times the standard errors.
For the response function modeling, the seven variables with the highest importance were selected (Supplementary Table S3): altitude, minimum temperature of January and December, mean temperature of the coldest month (MTMC), mean temperature of December, and maximum temperature of January and December. All of them were highly collinear, with pairwise correlations > 0.85. The preliminary response functions showed similar results for each variable. Finally, we decided to retain only the MTCM, as it explains over 69% of the variability in our data according to the Random Forest model. Following the regression analysis, the adjusted coefficient of determination in the quadratic model for the RF at the population level was 0.32% with p < 0.001 (Figure 3). The PMAI culminates at 65 cm/year for a MTCM of -2.2°C. The 21 boxplots represent the range of families' PMAIs at each testing site. For example, we can see the boxplot of site B16 with both the lowest MTCM and PMAIs values in the lower-left corner.
Figure 3. Population RF with of 0.32. PMAIs are plotted against the sites' MTCM. Boxplot were plotted for each site. The black dots represent the outliers. The gray band represents the curve's 95% confidence interval. Sites are from the left to right: B16, B18, B11, B12/B20, B6/B7/B5/B13, W3, B4/B3, A/B9/N, W4, B2/B1/H2, T1/T2 (sites separated by a “/” have the same MTCM but are plotted next to each other for a clearer plot).
In Table 1, the number of individuals per half-sib family (Nb) varies from 35 to 120. This uneven number is explained because it is a product of natural crosses among parents; hence the families' sizes were only revealed at the pedigree reconstruction stage. In Supplementary Figure S1, we report no statistical association between Nb and the mean model's ; however, the model's variability is higher for families with a smaller number of offspring. At the half-sib family level, the ranged from 0.06 to 0.64 with a median value of 0.27 (Table 1, Figure 4). All p-values of the RFs curve fitting were significant (p < 0.05), except one (genotype L2).
Figure 4. RF at the family level (solid and dashed lines). PMAIs are plotted against the MTCM of the testing sites.
In Figure 4, we plotted the response curves of all families to provide an overview of the variation in PMAIs found in the data. Some families showed substantial variation in PMAIs. For example, the genotype S23 rises above all the others in PMAI, but only for a narrow range of MTCM. With lower or higher MTCM, this genotype performs poorly compared to the other families. Some families showed less steeped curves. For example, genotype S7 does not rise as high as genotype S23 but surpasses most genotypes along the whole studied MTCM gradient with a more rounded curve. Half-sib families culminate at different PMAIs, ranging from 62 to 70 cm/year, with genotypes L2/S11 and S23, respectively. Similarly, there was a difference among the MTCM optimums of the families with a range of −2.4 to −1.6°C for the genotypes S23 and S12, respectively (Figure 4).
Phenotypic data were regressed onto random genetic and fixed site factors using the mixed linear animal genetic model. The genetic variation observed in this study resembles typical values for height and wood density in conifers (White et al., 2007), thus app. one-third of phenotypic variation is attributable to direct allelic effects. This, along with the presence of climatic gradients, is a prerequisite to efficient response function fitting, as shown in Figure 3. The choice of MTCM as our climatic gradient is supported by Foff et al. (2014), who found that cold temperature is an important limiting factor of growth in European Larch. As the GxE interactions were not significant in the present study, there is a general tendency of the genotypes to keep the same ranking across all environments (see Figure 4). However, our results showed some response functions with a clear change in rank and/or variance across the MTCM. Therefore, one may select a set of genotypes that are performing well across all sites and combine them with those, that are performing best only in specific environments. In the case of significant GxE, one may start with calculating first-order partial derivatives with respect to climate variables of planting location and provenance origin (Wang et al., 2010; Chakraborty et al., 2015).
Compared to traditional breeding trials, the proposed methodology minimizes resources for establishing the actual experiments because all activities (phenotyping, genotyping) take place in operational afforestation sites with a designated seed source. Further, uneven gametic contributions within and among the respective sites are optimally accounted for within the combined genetic evaluation protocol, i.e., multi-site animal genetic model. Unlike the traditional breeding programs relying on transfer within and among fixed-seed zones, the current approach is flexible. Seed transfer delineation is dynamic in line with particular CC development.
There are possible pitfalls of this proposed strategy that should be addressed here. Although used in many studies, the quadratic model fitting is a simplistic representation of the trait response to the environment. It assumes a physiological response that increases to a maximum value, then drops immediately (Leites et al., 2012). In reality, the curvature results from a multidimensional space of adaptive topography reflecting a specific genetic architecture of quantitative traits. The actual underlying function is likely non-linear and non-parametric. An additional limitation is related to the future adaption of the new plantations established from the offspring of the selected parents. While these would be better adapted to new climatic conditions in the short term, evaluating the long-term selection response across multiple generations is more complicated. Repeated cycles of selection would affect the environmental sensitivity depending on the functional characteristics of the reaction norms (Kolmodin et al., 2003). Optimizing the long-term methodology across multiple selection cycles should be the subject of future research.
The particular finding of our investigation can be seen as a case study demonstrating that combining in-situ large-scale genetic evaluation with response function methodology works. Compared to the current methodology of response functions, we are adding the opportunity to utilize the intra-population genetic variation that can further boost the adaptive response to CC. Ultimately, one would be interested in combining the provenance-based response function methodology with the presented intra-population approach. We are suggesting to optimize gene contributions from the two genetic hierarchical levels utilizing methods that were initially developed in forest tree breeding to optimize artificial selection (Lindgren et al., 1993). Assisted migration would then follow optimum contributions, thus maximizing overall adaptive response across a range of environmental conditions while maintaining sufficient levels of genetic diversity (Sáenz-Romero et al., 2021).
The suggested methodology could be practically implemented as follows. (1) identification of a common seed source representing a specific population, i.e., a provenance, (2) phenotypic evaluation followed by pedigree reconstruction (Lstibůrek et al., 2015), (3) phenotypic measurements across multiple sites combined with the pedigree in multivariate statistical analysis to predict the genetic merit of individual trees, (4) selection of principal environmental gradients influencing the studied traits, and (5) development of the individual- and population-level RFs to describe the genetic variation along prevalent environmental gradients, (6) selection of the best-adapted reforestation material accounting for genetic diversity (Funda et al., 2009), and (7) transfer of the adapted forest reproductive material to the target location.
The datasets presented in this study can be found in online repositories. The names of the repository/repositories and accession number(s) can be found at: https://github.com/mlstiburek/indiv_tree_response_functions.
ML and SS conceived the project. VP and DC conducted statistical analyses. VP and ML wrote the manuscript. SS, DC, JS, and HK contributed to discussions. All authors contributed to the article and approved the submitted version.
VP was financed by the Internal Grant Agency of the Faculty of Forestry and Wood Sciences, Czech University of Life Sciences Prague. DC, HK, and SS were supported by the Austrian Research Promotion Agency (FFG) and the Cooperation Platform Forst Holz Papier (FHP) and LIECO nurseries and the Austrian Federal Forests (ÖBf). ML was supported by grant “EXTEMIT – K”, no. CZ.02.1.01/0.0/0.0/15_003/0000433 financed by OP RDE. ML and JS were supported by the Ministry of Education, Youth, and Sports program INTER-EXCELLENCE, subprogram INTER-ACTION [grant number LTAUSA19113].
The authors declare that the research was conducted in the absence of any commercial or financial relationships that could be construed as a potential conflict of interest.
All claims expressed in this article are solely those of the authors and do not necessarily represent those of their affiliated organizations, or those of the publisher, the editors and the reviewers. Any product that may be evaluated in this article, or claim that may be made by its manufacturer, is not guaranteed or endorsed by the publisher.
Map data copyrighted OpenStreetMap contributors and available from https://www.openstreetmap.org.
The Supplementary Material for this article can be found online at: https://www.frontiersin.org/articles/10.3389/fpls.2021.758221/full#supplementary-material
Aitken, S. N., Yeaman, S., Holliday, J. A., Wang, T., and Curtis-McLane, S. (2008). Adaptation, migration or extirpation: climate change outcomes for tree populations. Evol. Appl. 1, 95–111. doi: 10.1111/j.1752-4571.2007.00013.x
Boisvenue, C., and Running, S. W. (2006). Impacts of climate change on natural forest productivity–evidence since the middle of the 20th century. Glob. Change Biol. 12, 862–882. doi: 10.1111/j.1365-2486.2006.01134.x
Butler, D. G., Cullis, B. R., Gilmour, A. R., Gogel, B. J., and Thompson, R. (2017). Asreml-R Reference Manual, version 4. Hemel Hempstead: VSN International Ltd.
Chakraborty, D., Wang, T., Andre, K., Konnert, M., Lexer, M. J., Matulla, C., et al. (2015). Selecting populations for non-analogous climate conditions using universal response functions: the case of Douglas-fir in central Europe. PLoS ONE 10:e0136357. doi: 10.1371/journal.pone.0136357
Dai, A. (2011). Drought under global warming: a review. Wiley Interdiscipl. Rev. Clim. Change 2, 45–65. doi: 10.1002/wcc.81
Davis, M. B., and Shaw, R. G. (2001). Range shifts and adaptive responses to Quaternary climate change. Science 292, 673–679. doi: 10.1126/science.292.5517.673
Dyderski, M. K., Paź, S., Frelich, L. E., and Jagodziński, A. M. (2018). How much does climate change threaten european forest tree species distributions? Glob. Change Biol. 24, 1150–1163. doi: 10.1111/gcb.13925
Falconer, D., and Mackay, T. F. C. (1996). Introduction to Quantitative Genetics. Harlow; Essex: Longman Group Ltd.
Foff, V., Weiser, F., Foffova, E., Gömöry, D., et al. (2014). Growth response of european larch (Larix decidua mill.) populations to climatic transfer. Silvae Genet. 63, 67–75. doi: 10.1515/sg-2014-0010
Funda, T., Lstibůrek, M., Lachout, P., Klápště, J., and El-Kassaby, Y. (2009). Optimization of combined genetic gain and diversity for collection and deployment of seed orchard crops. Tree Genet. Genomes 5, 583–593. doi: 10.1007/s11295-009-0211-3
Gougherty, A. V., Keller, S. R., and Fitzpatrick, M. C. (2021). Maladaptation, migration and extirpation fuel climate change risk in a forest tree species. Nat. Clim. Change 11, 166–171. doi: 10.1038/s41558-020-00968-6
Henderson, C. (1984). Applications of Linear Models in Animal Breeding. Guelph, ON: University of Guelph, Canada.
Hijmans, R. J., Cameron, S. E., Parra, J. L., Jones, P. G., and Jarvis, A. (2005). Very high resolution interpolated climate surfaces for global land areas. Int. J. Climatol. 25, 1965–1978. doi: 10.1002/joc.1276
Howe, G. T., Aitken, S. N., Neale, D. B., Jermstad, K. D., Wheeler, N. C., and Chen, T. H. (2003). From genotype to phenotype: unraveling the complexities of cold adaptation in forest trees. Can. J. Bot. 81, 1247–1266. doi: 10.1139/b03-141
Kapeller, S., Lexer, M. J., Geburek, T., Hiebl, J., and Schueler, S. (2012). Intraspecific variation in climate response of Norway spruce in the eastern Alpine range: selecting appropriate provenances for future climate. For. Ecol. Manage. 271, 46–57. doi: 10.1016/j.foreco.2012.01.039
Kolmodin, R., Strandberg, E., Jorjani, H., and Danell, B. (2003). Selection in the presence of a genotype by environment interaction: response in environmental sensitivity. Anim. Sci. 76, 375–385. doi: 10.1017/S1357729800058604
Leites, L. P., Rehfeldt, G. E., Robinson, A. P., Crookston, N. L., and Jaquish, B. (2012). Possibilities and limitations of using historic provenance tests to infer forest species growth responses to climate change. Nat. Resour. Model. 25, 409–433. doi: 10.1111/j.1939-7445.2012.00129.x
Lindgren, D., Wei, R., and Bondesson, L. (1993). Optimal selection from families. Heredity 70, 619–621. doi: 10.1038/hdy.1993.88
Lindner, M., Fitzgerald, J. B., Zimmermann, N. E., Reyer, C., Delzon, S., van der Maaten, E., et al. (2014). Climate change and European forests: what do we know, what are the uncertainties, and what are the implications for forest management? J. Environ. Manage. 146, 69–83. doi: 10.1016/j.jenvman.2014.07.030
Lindner, M., Maroschek, M., Netherer, S., Kremer, A., Barbati, A., Garcia-Gonzalo, J., et al. (2010). Climate change impacts, adaptive capacity, and vulnerability of European forest ecosystems. For. Ecol. Manage. 259, 698–709. doi: 10.1016/j.foreco.2009.09.023
Lstibůrek, M., Hodge, G. R., and Lachout, P. (2015). Uncovering genetic information from commercial forest plantations-making up for lost time using “breeding without breeding”. Tree Genet. Genomes 11:55. doi: 10.1007/s11295-015-0881-y
Lstibůrek, M., Schueler, S., El-Kassaby, Y. A., Hodge, G. R., Stejskal, J., Koreckỳ, J., et al. (2020). In Situ genetic evaluation of European larch across climatic regions using marker-based pedigree reconstruction. Front. Genet. 11:28. doi: 10.3389/fgene.2020.00028
Malcolm, J. R., Markham, A., Neilson, R. P., and Garaci, M. (2002). Estimated migration rates under scenarios of global climate change. J. Biogeogr. 29, 835–849. doi: 10.1046/j.1365-2699.2002.00702.x
Marshall, T., Slate, J., Kruuk, L., and Pemberton, J. (1998). Statistical confidence for likelihood-based paternity inference in natural populations. Mol. Ecol. 7, 639–655. doi: 10.1046/j.1365-294x.1998.00374.x
Mátyás, C. (1994). Modeling climate change effects with provenance test data. Tree Physiol. 14, 797–804.
McLachlan, J. S., and Clark, J. S. (2004). Reconstructing historical ranges with fossil data at continental scales. For. Ecol. Manage. 197, 139–147. doi: 10.1016/j.foreco.2004.05.026
O'Neill, G. A., Nigh, G., Wang, T., and Ott, P. K. (2007). Growth response functions improved by accounting for nonclimatic site effects. Can. J. For. Res. 37, 2724–2730. doi: 10.1139/X07-100
O'Neill, G. A., Stoehr, M., and Jaquish, B. (2014). Quantifying safe seed transfer distance and impacts of tree breeding on adaptation. For. Ecol. Manage. 328, 122–130. doi: 10.1016/j.foreco.2014.05.039
OpenStreetMap contributors (2021). Map Data Retrieved From Leaflet, the Open-Source JavaScript Library, Basemaps: Stamen.TerrainLabels and Stamen.TerrainBackground Basemaps. Available online at: http://leaflet-extras.github.io/leaflet-providers/preview/index.html; https://www.openstreetmap.org.
Pachauri, R. K., Allen, M. R., Barros, V. R., Broome, J., Cramer, W., Christ, R., et al. (2014). Climate Change 2014: Synthesis Report. Contribution of Working Groups I, II and III to the Fifth Assessment Report of the Intergovernmental Panel on Climate Change. IPCC.
R Core Team (2020). R: A Language and Environment for Statistical Computing, version 4.0.0. Vienna: R Foundation for Statistical Computing.
Rehfeldt, G. E., Ying, C. C., Spittlehouse, D. L., and Hamilton, D. A. Jr (1999). Genetic responses to climate in Pinus contorta: niche breadth, climate change, and reforestation. Ecol. Monogr. 69, 375–407.
Richter, S., Kipfer, T., Wohlgemuth, T., Guerrero, C. C., Ghazoul, J., and Moser, B. (2012). Phenotypic plasticity facilitates resistance to climate change in a highly variable environment. Oecologia 169, 269–279. doi: 10.1007/s00442-011-2191-x
RStudio Team (2020). RStudio: Integrated Development Environment for R, version 1.2.5042. Boston, MA: RStudio, PBC.
Sáenz-Romero, C., Lamy, J.-B., Ducousso, A., Musch, B., Ehrenmann, F., Delzon, S., et al. (2017). Adaptive and plastic responses of Quercus petraea populations to climate across Europe. Glob. Change Biol. 23, 2831–2847. doi: 10.1111/gcb.13576
Sáenz-Romero, C., O'Neill, G., Aitken, S. N., and Lindig-Cisneros, R. (2021). Assisted migration field tests in canada and mexico: lessons, limitations, and challenges. Forests 12:9. doi: 10.3390/f12010009
Savolainen, O., Pyhäjärvi, T., and Knürr, T. (2007). Gene flow and local adaptation in trees. Annu. Rev. Ecol. Evol. Syst. 38, 595–619. doi: 10.1146/annurev.ecolsys.38.091206.095646
Schmidtling, R. (1994). Use of provenance tests to predict response to climate change: loblolly pine and Norway spruce. Tree Physiol. 14, 805–817.
Seidl, R., Thom, D., Kautz, M., Martin-Benito, D., Peltoniemi, M., Vacchiano, G., et al. (2017). Forest disturbances under climate change. Nat. Clim. Change 7, 395–402. doi: 10.1038/nclimate3303
Senf, C., and Seidl, R. (2021). Mapping the forest disturbance regimes of Europe. Nat. Sustain. 4, 63–70. doi: 10.1038/s41893-020-00609-y
St-Laurent, G. P., Kozak, R., and Hagerman, S. (2021). Cross-jurisdictional insights from forest practitioners on novel climate-adaptive options for Canada's forests. Reg. Environ. Change 21, 1–14. doi: 10.1007/s10113-020-01733-3
Suvanto, S., Nöjd, P., Henttonen, H. M., Beuker, E., and Mäkinen, H. (2016). Geographical patterns in the radial growth response of Norway spruce provenances to climatic variation. Agric. For. Meteorol. 222, 10–20. doi: 10.1016/j.agrformet.2016.03.003
Thomson, A. M., and Parker, W. H. (2008). Boreal forest provenance tests used to predict optimal growth and response to climate change. 1. Jack pine. Can. J. For. Res. 38, 157–170. doi: 10.1139/X07-122
Trenberth, K. E., Dai, A., Van Der Schrier, G., Jones, P. D., Barichivich, J., Briffa, K. R., et al. (2014). Global warming and changes in drought. Nat. Clim. Change 4, 17–22. doi: 10.1038/nclimate2067
Wang, T., Hamann, A., Yanchuk, A., O'neill, G., and Aitken, S. (2006). Use of response functions in selecting lodgepole pine populations for future climates. Glob. Change Biol. 12, 2404–2416. doi: 10.1111/j.1365-2486.2006.01271.x
Wang, T., O'Neill, G. A., and Aitken, S. N. (2010). Integrating environmental and genetic effects to predict responses of tree populations to climate. Ecol. Appl. 20, 153–163. doi: 10.1890/08-2257.1
Keywords: assisted migration, genetic diversity, intraspecific variation, provenance trials, European larch
Citation: Poupon V, Chakraborty D, Stejskal J, Konrad H, Schueler S and Lstibůrek M (2021) Accelerating Adaptation of Forest Trees to Climate Change Using Individual Tree Response Functions. Front. Plant Sci. 12:758221. doi: 10.3389/fpls.2021.758221
Received: 13 August 2021; Accepted: 27 October 2021;
Published: 23 November 2021.
Edited by:
Alma Balestrazzi, University of Pavia, ItalyReviewed by:
Eugene Vaganov, Siberian Federal University, RussiaCopyright © 2021 Poupon, Chakraborty, Stejskal, Konrad, Schueler and Lstibůrek. This is an open-access article distributed under the terms of the Creative Commons Attribution License (CC BY). The use, distribution or reproduction in other forums is permitted, provided the original author(s) and the copyright owner(s) are credited and that the original publication in this journal is cited, in accordance with accepted academic practice. No use, distribution or reproduction is permitted which does not comply with these terms.
*Correspondence: Milan Lstibůrek, bHN0aWJ1cmVrQGZsZC5jenUuY3o=
†These authors share senior authorship
Disclaimer: All claims expressed in this article are solely those of the authors and do not necessarily represent those of their affiliated organizations, or those of the publisher, the editors and the reviewers. Any product that may be evaluated in this article or claim that may be made by its manufacturer is not guaranteed or endorsed by the publisher.
Research integrity at Frontiers
Learn more about the work of our research integrity team to safeguard the quality of each article we publish.