- 1School of Agricultural Biotechnology, Punjab Agricultural University, Ludhiana, India
- 2Department of Soil Science, Punjab Agricultural University, Ludhiana, India
- 3Department of Plant Breeding and Genetics, Punjab Agricultural University, Ludhiana, India
- 4Genetics and Plant Breeding, National Institute of Agricultural Botany, Cambridge, United Kingdom
- 5International Wheat and Maize Improvement Center (CIMMYT), Texcoco, Mexico
Nitrogen is one of the most important macronutrients for crop growth and metabolism. To identify marker-trait associations for complex nitrogen use efficiency (NUE)-related agronomic traits, field experiments were conducted on nested synthetic wheat introgression libraries at three nitrogen input levels across two seasons. The introgression libraries were genotyped using the 35K Axiom® Wheat Breeder's Array and genetic diversity and population structure were examined. Significant phenotypic variation was observed across genotypes, treatments, and their interactions across seasons for all the 22 traits measured. Significant positive correlations were observed among grain yield and yield-attributing traits and root traits. Across seasons, a total of 233 marker-trait associations (MTAs) associated with fifteen traits of interest at different levels of nitrogen (N0, N60, and N120) were detected using 9,474 genome-wide single nucleotide polymorphism (SNP) markers. Of these, 45 MTAs for 10 traits in the N0 treatment, 100 MTAs for 11 traits in the N60 treatment, and 88 MTAs for 11 traits in the N120 treatment were detected. We identified putative candidate genes underlying the significant MTAs which were associated directly or indirectly with various biological processes, cellular component organization, and molecular functions involving improved plant growth and grain yield. In addition, the top 10 lines based on N response and grain yield across seasons and treatments were identified. The identification and introgression of superior alleles/donors improving the NUE while maintaining grain yield may open new avenues in designing next generation nitrogen-efficient high-yielding wheat varieties.
Introduction
The global demand for nitrogen currently stands at about 117 million metric tons with a projected annual increase of approximately 1.5% expected in the near future (FAO, 2019). Farmers generally apply high doses of nitrogenous fertilizers to ensure good yields. The high input of commercially available fertilizers has led to the degradation of air, soil, and water quality (Hickman et al., 2014; Russo et al., 2017). In addition, when the supply of nitrogen (N) is in excess of crop N demand, it increases the susceptibility of plants to various diseases and insect pests (Reddy, 2017). Therefore, it is necessary to optimize and improve the nitrogen use efficiency (NUE) of cereal crops to maximize yield in addition to minimizing the negative impact of increase in N use on the environments and natural resources. Identification of marker-trait associations (MTAs) can be used to make effective targeted introgressions and is one possible genetic method to address the challenge of developing N-efficient wheat varieties with stable yield under N-limited environments.
Wheat varieties that maintain yield under moderate or intense N deficiency can adapt to low input systems. To breed such varieties, genetic variation for adaptation traits to N deficiency is required. To date, limited quantitative trait loci (QTL) for both yield and its response to N deficiency in wheat under field conditions have been documented. Detection of genotypes and underlying QTLs for maintaining yields at low N levels are of value in wheat breeding programs designed to increase N-deficiency tolerance. Some QTLs influencing N uptake have been genetically mapped in wheat under different doses of fertilizer application using biparental populations (An et al., 2006; Laperche et al., 2008; Xu et al., 2014; Mahjourimajd et al., 2016; Deng et al., 2017). A number of genetic loci for agronomic traits related to N use and grain yield have also been mapped to the chromosomal regions containing the GS2 gene in wheat and rice (Prasad et al., 1999; Yamaya et al., 2002; Obara et al., 2004; Habash et al., 2007; Laperche et al., 2008; Fontaine et al., 2009). This suggests the role of the genomic region surrounding GS2 (Pritchard and Wen, 2004) is favorable in breeding wheat and rice varieties with improved agronomic performance and nitrogen use efficiency (NUE). Other genetic regions associated with N uptake have also been detected in rice (Wissuwa et al., 1998; Ming et al., 2000), wheat (Su et al., 2006, 2009); maize (Zhu et al., 2005), common bean (Liao et al., 2004; Yan et al., 2004), and soybean (Li et al., 2005; Liang et al., 2010). The NRT2.1, NRT2.2, and NAR2.1 genes have been reported to be the important contributors to the high-affinity transport system in Arabidopsis roots (Orsel et al., 2006). Sixteen genes were identified in wheat homologous to characterize the low-affinity nitrate transporter NPF genes in Arabidopsis, suggesting a complex wheat NPF gene family (Buchner and Hawkesford, 2014). The regulation of wheat NPF genes by plant N-status indicated the involvement of these transporters in the substrate transport in relation to N-metabolism.
The phenotypic traits reported to be associated with NUE in cereal crops so far include root number, length, density, and branching (Morita et al., 1988; Yang et al., 2012; Steffens and Rasmussen, 2016), dense and erect panicle (Sun et al., 2014), plant height (Gaju et al., 2011), and leaf width (Zhu et al., 2020). The collocation of QTLs for N-uptake and root architecture traits have suggested that breeding for better and efficient root systems is a way to improve NUE (Coque et al., 2008; Sandhu et al., 2015).
Diverse accessions, landraces, breeding populations, and next-generation mapping populations, including nested-association mapping (NAM) and multi-parent advanced generation inter-cross (MAGIC) populations have shown a potential for mining novel genetic variation in rice (Zhao et al., 2011; Sandhu et al., 2019; Subedi et al., 2019), wheat (Mackay et al., 2014), maize (Yu et al., 2008), and soybean (Xavier et al., 2015). The NAM and MAGIC populations have proven advantageous over biparental populations as they capture additional recombination breakpoints, thus increasing the allelic diversity and improving the power of QTL detection (Yu et al., 2008; Scott et al., 2020). Further, the availability of high throughput genotyping platforms to generate uniformly distributed genome-wide molecular markers is critical for the high-resolution genetic dissection of polygenic traits, and the tracking of favorable alleles in breeding populations (Pandey et al., 2012, 2016; Varshney et al., 2013). To date, a series of high-density wheat single nucleotide polymorphism (SNP) arrays, such as the Illumina 9K iSelect SNP array (Cavanagh et al., 2013), Illumina 90K iSelect SNP genotyping array (Wang et al., 2014a), 15K SNP array (Boeven et al., 2016), Axiom® 660K SNP array, 55K SNP array, Axiom® HD 820K genotyping array (Winfield et al., 2016), 35K Axiom array (Allen et al., 2017), and 50K Triticum TraitBreed array (Rasheed and Xia, 2019) have been developed and their utility has been demonstrated across a range of applications.
In the present study, we developed nested synthetic wheat introgression libraries capturing novel genetic variation. The libraries were genotyped using a high-density SNP array and phenotypically assessed for root traits and agronomic performance under three N input conditions in the field. Genome-wide association mapping was used to identify MTAs for the root and agronomic traits, and lines carrying favorable genetic combinations were also identified for use in future breeding for improved N use.
Materials and Methods
Plant Material
A total of 31 cultivated and 12 synthetic wheats were evaluated at 6 nitrogen (N) levels (N0, N40, N80, N120, N160, and N200) in 3 replications in 2015 and 2016 during the rabi seasons at Punjab Agricultural University, Ludhiana, India. The synthetic wheats, PDW233/Ae. tauschii acc. pau 14135 and PBW114/Ae. tauschii acc. pau 14170 produced high-grain yields as well as high agronomic efficiency at low fertilizer N doses (unpublished data). Nested introgression line libraries developed from the synthetic wheats, PDW233/Ae. tauschii acc. pau 14135 and PBW114/Ae. tauschii acc. pau 14170 (N-SHW) constituting a set of 352 lines were used in the present study. The N-SHW library was made up of subsets from four populations (Pop1: 75 lines from PDW233/Ae. tauschii acc. pau 14135 amphiploid//2*BWL4444; Pop2: 106 lines from PDW233/Ae. tauschii acc. pau 14135 amphiploid//2*BWL3531; Pop3: 88 lines from PBW114/Ae. tauschii acc. pau 14170 amphiploid//2*BWL4444; Pop4: 83 lines from PBW114/Ae. tauschii acc. pau 14170 amphiploid//2*BWL3531 along with the two common parents (BWL3531, BWL4444) and other unique parents (PDW233, PBW114, Ae. tauschii acc. pau 14135 amphiploid, and Ae. tauschii acc. pau 14170 amphiploid). The breeding scheme that was used to develop the N-SHW introgression library is summarized in Figure 1.
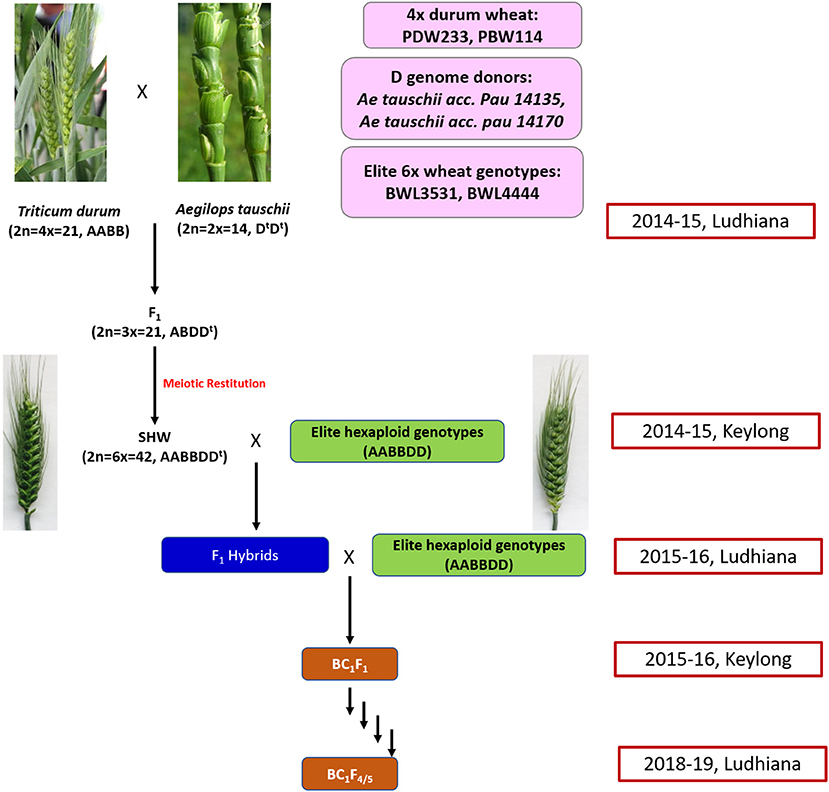
Figure 1. Schematic representation of the breeding strategy that is used to develop the nested synthetic wheat introgression libraries.
Agronomic Practices and Management of Experiments
The N-SHW library, six parents, and two synthetic hexaploid wheats were assessed under field conditions at the experimental farms of School of Agricultural Biotechnology, PAU Ludhiana (30 54' N latitude, 75 48' E longitude, and 247 m above sea level) over 2 years in 3N levels (6-year x N combinations). The soil analysis of experimental plots showed soil pH: 7.3; EC: 0.157 ds/m; organic content: 0.29%; N: 99 Kg ha−1, P: 69 Kg ha−1; K: 116 Kg ha−1, Zn: 4.77 parts per million (ppm), Cu: 1.73 ppm, and Fe: 7.30 ppm. The N-SHW libraries were evaluated in rabi seasons of 2018 and 2019. Details of the number of lines tested and experimental design is provided in Table 1. The breeding material was sown on 21st of November and 18th of November in 2018 and 2019, respectively. In both the years, the experiments were conducted at three N levels [i.e. zero N (0 Kg ha−1), half N (60 Kg ha−1), and full N (recommended, 120 Kg ha−1),] referred to as N0, N1, and N2, respectively. The recommended dose of phosphorus, potassium, and manganese was applied at the time of sowing. Half of N was applied at the time of sowing while the other half was applied in two equal splits, the first at crown root initiation stage and the remaining at the maximum tillering stage in both the N1 and N2 experiments. N0 was treated as a control. Recommended fungicides and insecticides were applied to control stripe rusts, brown rusts, and aphids at jointing, booting, and 10 days after anthesis to prevent diseases and pests. Weeds were controlled manually.
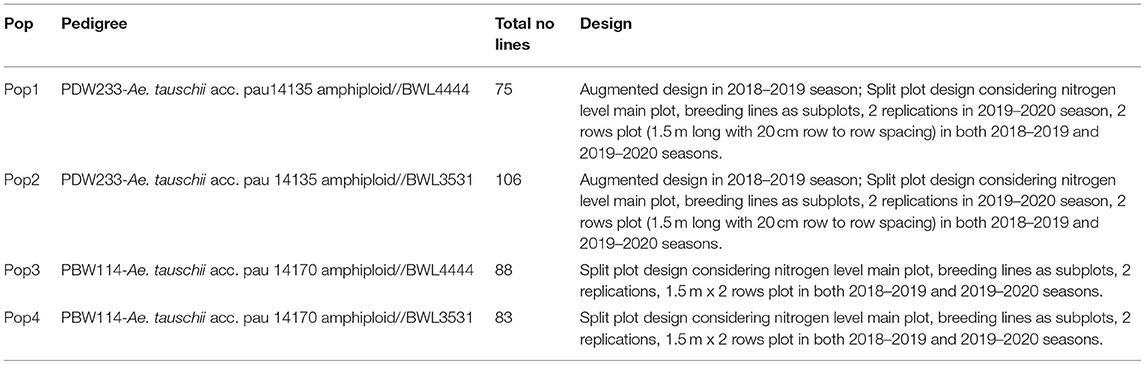
Table 1. Details on experimental material evaluated for nitrogen use efficiency related traits and design used across two crop seasons (2018–2019 and 2019–2020).
Characterization of Phenotypic Traits
Under field conditions, a total of 22 traits were assessed in all experiments across both seasons except the maximum root length and root angle which were measured in 2018 only. The details of the nitrogen use efficiency (NUE)-related traits, root and plant morphological traits, grain yield, and yield attributing traits are presented in Supplementary Figure 1. Destructive sampling of six plants per plot was done at 60 days after sowing (DAS) to evaluate early root and shoot traits (Supplementary Figure 2). Shoots were separated from the roots, and the fresh root weight (FRW; g) and fresh shoot weight (FSW; g) were measured. The root and shoot samples were dried at 70°C in an oven until constant dry root weight (DRW; g) and dry shoot weight (DSW; g) were observed, while the roots were cleaned thoroughly and stored in 70% alcohol at 4°C for root trait evaluation. The maximum root length (MRL) and root angle (RA) were measured using ImageJ software. total root length (TRL), total root surface area (RSA), total root diameter (RD), total root volume (RV), number of forks (NF), and number of tips (Ntips) were recorded using WinRhizo STD4800 (Supplementary Figure 2). The roots were then dried at 70°C in the oven until constant DRW was observed. The data on N-uptake related traits were recorded using a chlorophyll meter, Soil Plant Analysis Development Meter (SPAD502) and leaf color chart (LCC). The LCC provides a decision-support system to the farmers for sustaining the high yields with optimum N dose in the field crops. It measures the leaf color variations of 6 SPAD units comprising 3, 3.5, 4.0, 4.5, 5.0, and 6.0 and provides N recommendation in the field crops. Flag leaf length (FLL) and flag leaf width (FLW) were recorded using a centimeter scale. Days to flowering (DTF) rate of about 50% was recorded when 50% of the plants in a plot exerted their panicles. Spikelets per spike (SPS) were counted manually from five random plants. The number of productive tillers (NPT) was counted manually in 0.5 m row length and shoot biomass (SB) at harvesting was measured from 0.5 m row length. The plant height (PHT) in cm was measured as the mean height of five random plants for each entry measured from the base of the plant to the tip of the panicle at the maturity stage. The plants were harvested at physiological maturity or when 80–85% of the panicles turned to golden yellow and the panicles at the base were already at the hard dough stage; harvested grains were threshed, dried, and weighed to determine the grain yield (GY). The shoot biomass (SB) was recorded at harvesting.
Phenotypic Data Analysis
Analysis of variance, and experiment-wise mean for each season was calculated using a mixed model analysis in PBTools V 1.4.0. for augmented design and in STAR Version: 2.0.1 for the split plot design. In the split plot design, the N levels were considered as the main plot and the breeding lines as subplot. Fisher's t-test was used to determine the significant difference among the breeding lines, treatments, and to estimate the interactions. The correlation analysis among traits was performed in R. v.1.1.423.
To evaluate the phenotypic stability and GY adaptability of the breeding lines across seasons and treatments, the genotype and genotype × environment (GGE) biplot analysis was performed, considering the effects of genotype (G) and genotype by environment (GE) as random. The best linear unbiased prediction (BLUP) values of the G and GE effects were calculated. The multiplicative model in PB tool version 1.3 (http://bbi.irri.org/) was used to explain the relationship between G and the seasons.
Genotypic Data
High-density genotyping was performed using the 35K Axiom® Wheat Breeder's Array (Affymetrix UK Ltd., United Kingdom). The quality pre-processing of 35,143 markers obtained from the 35K chip was done using PLINK software (Purcell et al., 2007). A total of 9,474 single nucleotide polymorphisms (SNPs) with minor allele frequency (MAF) of >5%, maximum heterozygote proportion of 0.1 and missing rates <0.1 were used to estimate the genetic relationships and for the mapping of MTAs for different traits associated with plant growth, yield, and yield-related traits. Principal component analysis (PCA) was carried out to detect and correct for population structure.
Population Structure and Association Analysis
The model-based STRUCTURE V. 2.3.4 software was used to test K values from 1 to 10, with a burn-in period of 10,000 and 1,000,000 Markov chain Monte Carlo (MCMC) reps after burn-in in order to assess the population structure in the 352 breeding lines using a total of 9,474 SNPs. The consistency and accuracy of the results were validated across 10 runs for each K. The K value with maximum likelihood over the 10 runs was used to estimate the most appropriate number of clusters (Pritchard and Wen, 2004). The population structure was determined by plotting the proposed number of subpopulations against the delta k (Earl, 2012). The PCA was performed in Genome Association and Prediction Integrated Tool (R/GAPIT) and added iteratively to the fixed model, ranging from PC1 to PC10.
Significant marker-trait associations were identified using a compressed mixed linear model (CMLM)/P3D (population parameters previously defined) in GAPIT executed in R. Identity by state (IBS) values and a relatedness matrix were used to estimate the random effect and genetic similarity of the accessions, respectively. The statistical power of the association studies was further improved by considering the population structure (Q value) and kinship matrix (K) estimated from the genotyping data. The Bonferroni correction method was used to correct for false positives in the analysis, using the stringent p-value benchmark. The Bonferroni multiple test correction was performed (0.05/9474; significance level of 5%/total number of markers used in analysis) and the calculated threshold value was found to be 5.28 × 10−6. The allelic effect of all the significant markers associated with the measured traits was determined by comparing the mean phenotypic values and the significant allelic variants for the trait/s using a Kruskal–Wallis test in R.
Candidate Gene Analysis and Functional Annotation of Putative Candidate Genes
Single nucleotide polymorphisms that exhibited a false discovery rate (FDR) corrected p < 0.05 for a particular trait of interest were evaluated as markers for the potential putative candidate genes. A window of 1 Mb adjacent to each significant SNP was examined for candidate genes and annotations were identified through the Ensembplants database (http://plants.ensembl.org/index.html).
The functional annotation and gene ontology (GO) of the identified putative candidate genes were performed using OMIX box software. Blast (E ≤ 1 0−5) was performed using the CloudBlast tool against Triticum (nr_subset) [monocots_triticum, taxa:4564] and NCBI non-redundant database (http://www.ncbi.nlm.nih.gov), followed by the InterPro using CloudIPS, followed by GO mapping, and annotation configuration. The GO terms were then used to generate the semantic similarity-based scatterplots/interactive graphs/tag clouds by using REVIGO (http://revigo.irb.hr/).
Defining N-Irresponsive and N-Responsive Lines
The genotypes that showed more or equal/stable GY with the minimal application of nitrogen fertilizer when compared to the recommended or standard N fertilizer application were considered as the N irresponsive (NIR) genotypes or the top grain yielders across seasons and treatments. On the other way around, the genotypes that were low yielding or not able to maintain the GY with the minimal application of N fertilizer when compared to the recommended or standard N fertilizer application, were considered as the N responsive (NR) genotypes or the poor grain yielders across seasons and treatments.
Results
Significant Phenotypic Trait Variation and Correlations Detected Across Nitrogen Treatments
The 352 N-SHW lines, six parents, and two synthetic hexaploid wheat donors were screened for 22 traits in six growing conditions (2 years x 3 nitrogen levels). The ANOVA revealed significant genetic variation for the root, plant morphological, and agronomic traits among genotypes, treatments, seasons, and their interactions (genotype x treatment, genotype x season, treatment x season and genotype x treatment x season) (Table 2). The detailed information on trial means, least significance difference (LSD), and heritability for all the traits measured are presented in Supplementary Table 1. The results revealed significant genetic variations across genotypes, treatments, and their interactions in 2018–2019 and 2019–2020 seasons for all the traits measured (Supplementary Table 2). The phenotypic data of the traits measured in the present study were averaged across two seasons and are presented as mean values in Supplementary Table 3.
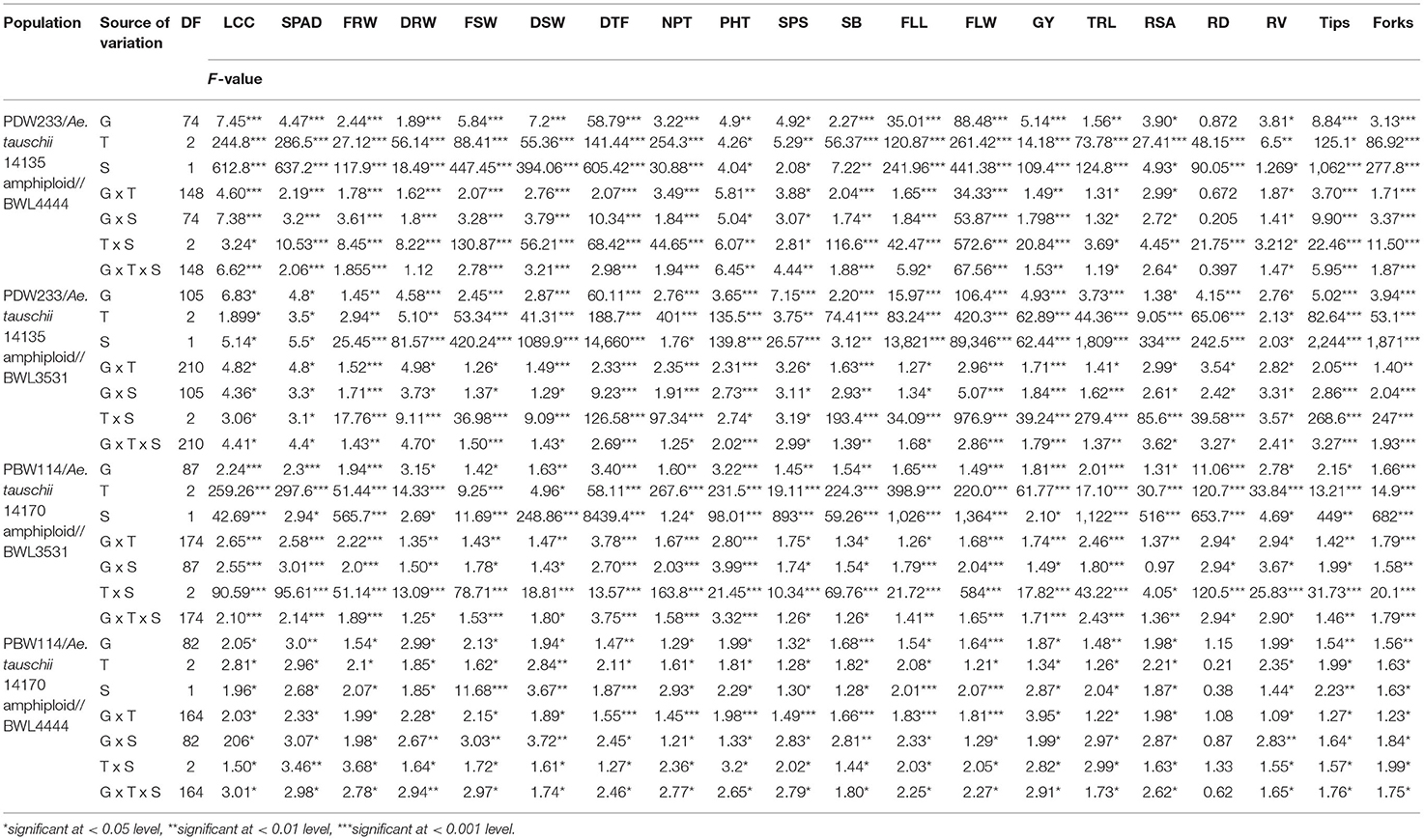
Table 2. Analysis of variance (ANOVA) for the NUE-related, root, plant morphological, yield, and yield-related traits among genotype (G), treatments (T), seasons (S), and their interactions (G x T, genotype x treatment; G x S, genotype x season; T x S, treatment x season; and G x T x S, genotype x treatment x season).
Grain yield increased with applied N level. In the N0 treatment, the average GY of the tested breeding lines across seasons was 2,022 kg ha−1 and it ranged from 564 to 4,092 kg ha−1 (Supplementary Table S3). In the N60 treatment, the GY varied from 882 to 4,685 kg ha−1 with an average GY of 2,357 kg ha−1 and, while in N120 treatment, the GY varied from 1,332 to 4,270 with an average of 2,579 kg ha−1 (Supplementary Table 3). Across seasons, N in the limited conditions (N0) resulted in the 14% and 22% GY reduction compared to N60 and N120 treatments, respectively. The N application also significantly increased the shoot biomass (SB) by 8% in N60 and 52% in N120 treatment across seasons. The average NPT across experiments was higher in N120 (28) compared to N60 (24) and N0 (22) (Supplementary Table 3). Under the N0 treatment, the average value of the leaf color chart (LCC) varied from 3.3 to 4.8, from 3.5 to 5.1 in the N60 treatment, and from 4.1 to 5.3 in the N120 treatment (Supplementary Table 3). The response of lines in terms of average dry shoot weight (DSW) across seasons increased from 3.28 in N0 to 3.62 in N60 to 3.75 in N120 treatment (Supplementary Table 3). The minimum and maximum values of dry root weight (DRW) under N0 were 0.187 g and 2.425 g; 0.298 g and 2.001 g under N60, and 0.338 g and 2.333 g under N120, respectively (Supplementary Table 3). The average root diameter was the highest under N60 (0.610 g) compared to N0 (0.560 g) and N120 (0.409 g) (Supplementary Table 3). Across seasons, the average flowering was delayed by 2 days under the N0 treatment compared to the N60 and N120 treatments. Average plant height (PHT) was lower (92 cm) in N0 compared to N60 (95 cm) and N120 (99 cm).
We calculated the Pearson's correlation coefficients between all the traits measured in N0 (Figure 2A), N60 (Figure 2B), and N120 (Figure 2C) treatments. The Pearson's correlation coefficients across all treatments considering pooled mean data for all the traits measured in the present study is presented in Supplementary Figure 3. The strongest and most significant positive correlation among grain yield and yield-attributing traits and root traits were observed in N60 treatment. The grain yield was significantly and positively correlated with shoot biomass (SB) (r = 0.23, p < 0.001), NPT (r = 0.18, p <0.01), fresh root weight (FRW) (r = 0.16, p < 0.01), fresh shoot weight (FSW) (r = 0.24, p <0.001), dry shoot weight (DSW) (r = 0.23, p < 0.001), and with root surface area (RSA) = (r = 0.23, p < 0.001). Across treatments, the GY showed a negative correlation with days to flowering (DTF), and shoot biomass (SB) showed a positive correlation with GY.
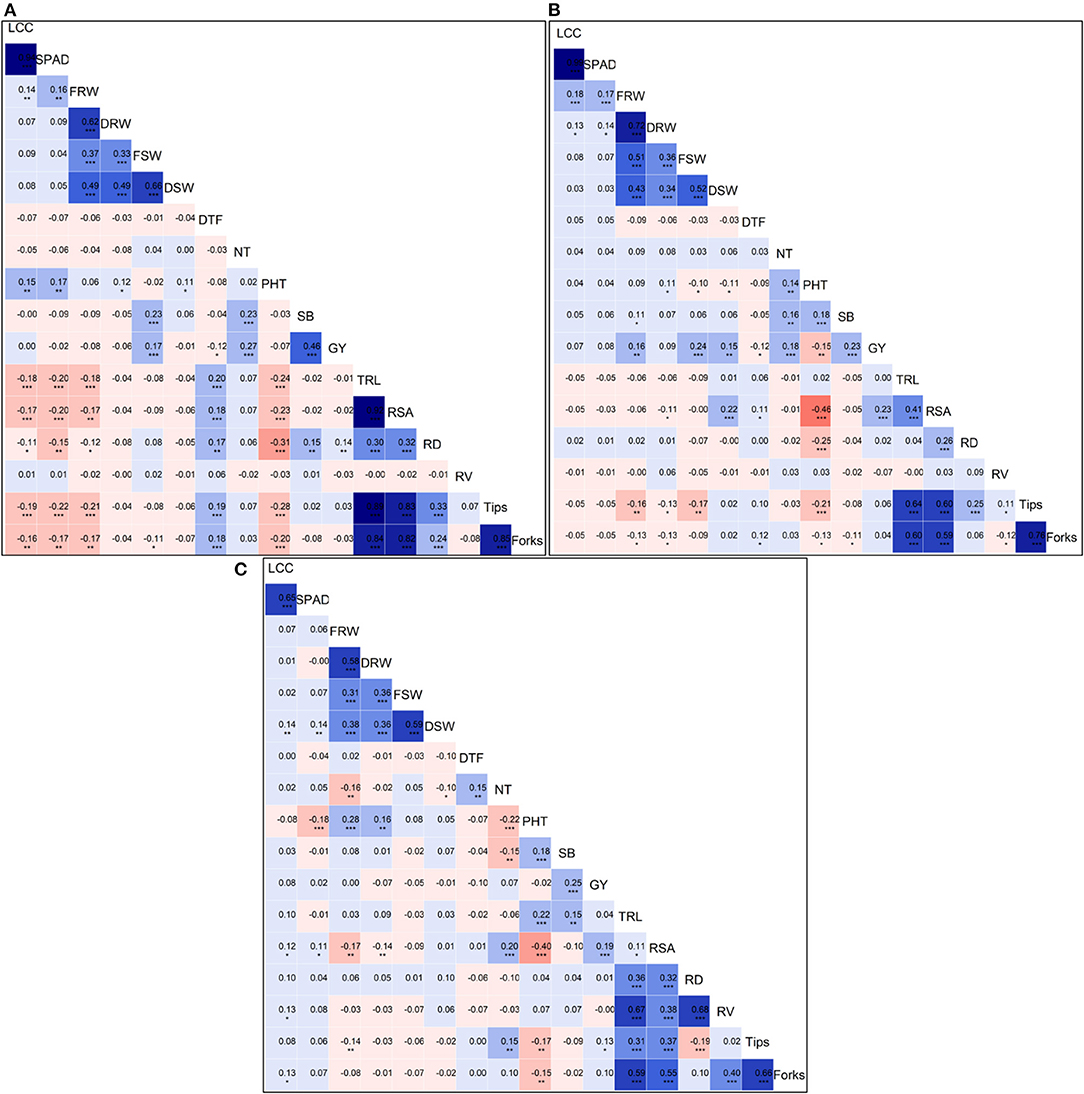
Figure 2. Plots of Pearson's r-values showing the correlation among traits measured (A) measured at N0, (B) N60, and (C) N120 levels. The blue color indicates positive correlation and red color indicates the negative correlation among different traits, the variation in color intensity represents the strength of the correlation among the traits. *Significance at <5% level, **significance at <1% level, ***significance at <0.1% level.
Population Structure Analysis Detected Three Genetic Subpopulations
The population structure of the N-SHW lines was assessed to understand the genetic structure of the 352 lines based on 9,474 single nucleotide polymorphisms (SNPs) distributed across all 21 wheat chromosomes. The most appropriate K explaining the population structure was K = 3 at minor allele frequency (MAF) ≥ 5% (Figure 3A). The kinship heatmap indicated a weak relatedness in the panel (Figure 3B). The first three principal components (PCs) were the most informative and gradually decreasing (Figures 3C,D) until the tenth PC. The kinship and PCs were considered during the genome-wide association study (GWAS) to correct for population structure. The appropriate number of subpopulations was determined from the largest delta K value of 3 (Figure 3E).
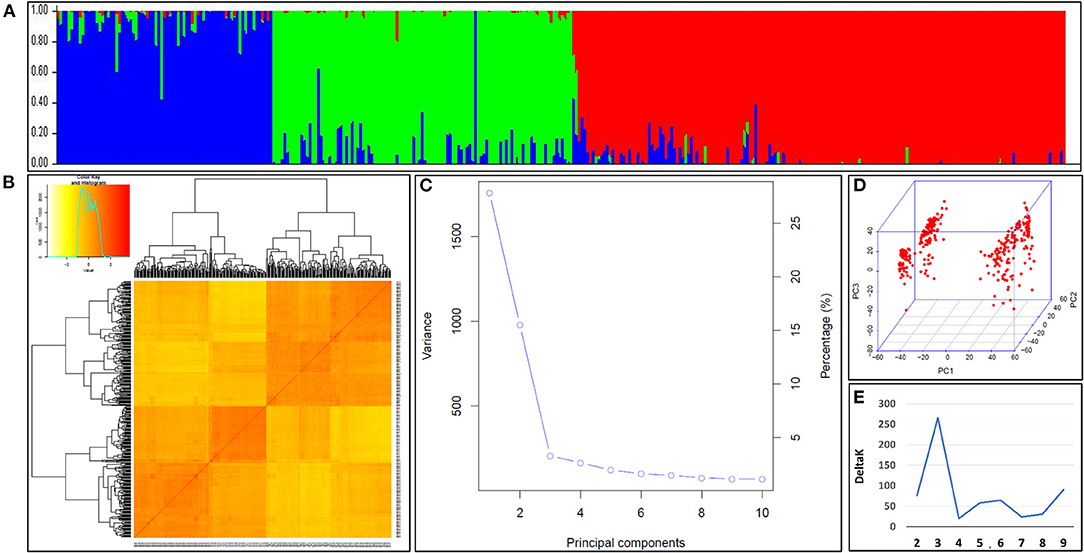
Figure 3. (A) Population structure within the nested synthetic wheat introgression libraries. The population structure plots with each vertical bar representing a breeding line colored according to the particular group to which the breeding line has been assigned. The breeding lines assigned to more than one of group represents the degree of their admixed set of the alleles (B) The Kinship matrix displayed as the heat map, where the red indicates the highest correlation between the pairs of breeding lines and yellow indicates the lowest correlation (C) The Scree plot indicating the most of the variabilities explained by the first three principal components (PCs) for association study (D) The three-dimensional view of the PCs explaining the genotypic variation among breeding lines constituting the introgression libraries. (E) The appropriate number of the subpopulations determined from the largest delta, K = 3.
Mapping Reveals Significant Marker-Trait Associations for All Traits
Genome-wide association study was performed exploiting the phenotypic variability in the 352 N-SHW lines using 9,474 SNPs from the 35K Axiom® Wheat Breeder's Array. Using the -log(P) ≥0.001 at 5% significance level, a total of 233 marker-trait associations (MTAs) were detected across seasons associated with fifteen traits of interest at different N levels (N0, N60, and N120; Table 3). Of these, 45 MTAs were associated with 10 traits in the N0 treatment, 100 MTAs were associated with 11 traits in the N60 treatment, and 88 MTAs were associated with 11 traits in the N120 treatment (Table 3). Across seasons and N treatments, a total of 53 MTAs associated with more than one trait/treatment were detected (Table 3). In addition to these 53 MTAs, another 41 MTAs associated with a single trait only were detected across seasons (Supplementary Table 4). All MTAs detected in the present study either in one season, both seasons, each treatment, or across treatments are compiled in Supplementary Table 5. Mapping detected MTAs on all subgenomes (A: 42, B: 18, and D: 34) across seasons and treatments. The highest number of MTAs were detected on chromosome 6A (26) followed by 2D (25), 3B (12), 4A (9), 6D (8), 2A (6), and 2B (4) with 1 MTA on each of 1B, 4B, 5A, and 7D. Considering all assessed traits, significant MTAs were reported for fresh root weight (FRW) (2A, 2D), fresh shoot weight (FSW) (2A, 2B, 2D, 5A, 7A), dry root weight (DRW) (2A, 2B, 2D, 7A), dry shoot weight (DSW) (2A, 2B, 2D), flag leaf width (FLW) (4A, 4B), number of tillers (NT) (3A), spikelets per spike (SPS) (1B), days to flowering (DTF) (3A, 3B, 6A), shoot biomass (SB) (6A), total root length (TRL) (6D), root surface area (RSA) (6A, 6D), root volume (RV) (6D), tips (6A, 6D) and forks (6A, 6D).
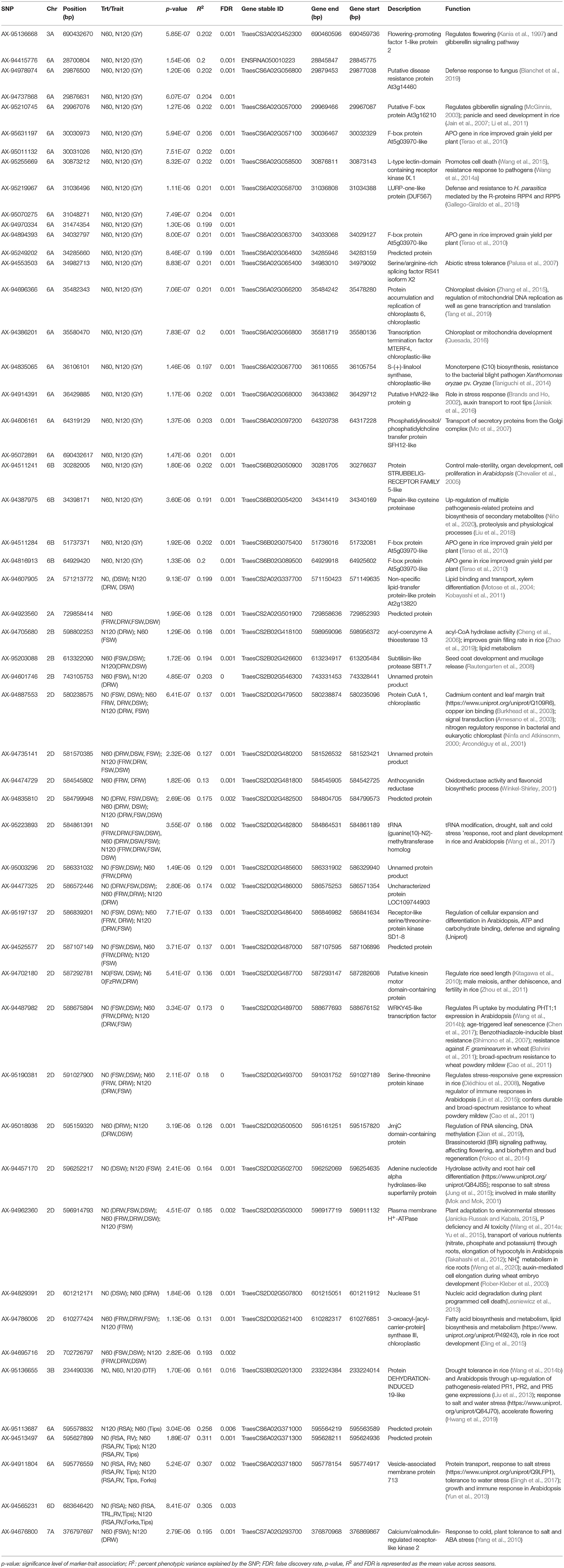
Table 3. The significant marker-trait associations (MTAs) and putative candidate genes identified across different treatments for the NUE-related, root, plant morphological, yield, and yield-related traits in a genome wide association study (GWAS) conducted on nested synthetic wheat introgression libraries.
The Manhattan plots depicting the significant -log (p-values) for the MTAs associated with nitrogen use efficiency (NUE)-related traits, root traits, and yield/yield related traits measured in the present study at different levels of N are presented in Supplementary Figures 4, 5 and Figure 4, respectively. Location of significant MTAs and SNP marker density distributed across 21 wheat chromosomes is presented in Figure 5. The SNPs for positively correlated traits, such as GY, BY, tips, RSA, RV, and forks appeared to be collocated on Chr 6A at the different levels of N (Table 3). A genomic region on 2D (ranging from 576,749,639 to 702,726,797 bp) contained 25 detected MTAs for a range of traits (FRW, DRW, FSW, and DSW) across seasons and treatments (Table 3 and Figure 5). A cluster of 17 SNPs spanning a 7.7 Mb region on the short arm of 6A showed association with GY at N60 and N120 (Figure 5). Across seasons and treatments, significant association in a 198 kb region on the long arm of Chr 6A were detected for root traits (RSA, RV, tips and forks). The SNP, AX-94565231 at 683.64 Mb on the long arm of Chr 6D showed association with different root traits (RSA, RV, tips, and forks) across seasons and treatments. In the N60 treatment, significant associations for FLW were detected in a 5.6 Mb region (549,799,824–544,201,748 bp) on the long arm of 4A. Interestingly, the association of the trait, DTF with SNP AX-95136655 on Chr 3B at 234.49 Mb was common under N0, N60, and N120 treatments (Figure 5). In the N0 treatment, a significant association harboring three strongly associated SNPs (AX-94593608, AX-94786978, and AX-95134564) spanning the genomic region 76 bp on the long arm of 3A was detected for NPT (Table 3). Further, single SNPs were identified in association with different traits at different N levels. For example, the SNP AX-94914391 (36.43 Mb, 6A) was significantly associated with SB at N0 and with GY at both N60 and N120 (Figure 5). The SNP AX-94705680 (598.80 Mb, 2B) showed association with FSW at N60 and with DRW at N120. The SNP network indicating the significant marker-trait interactions is presented in Figure 6.
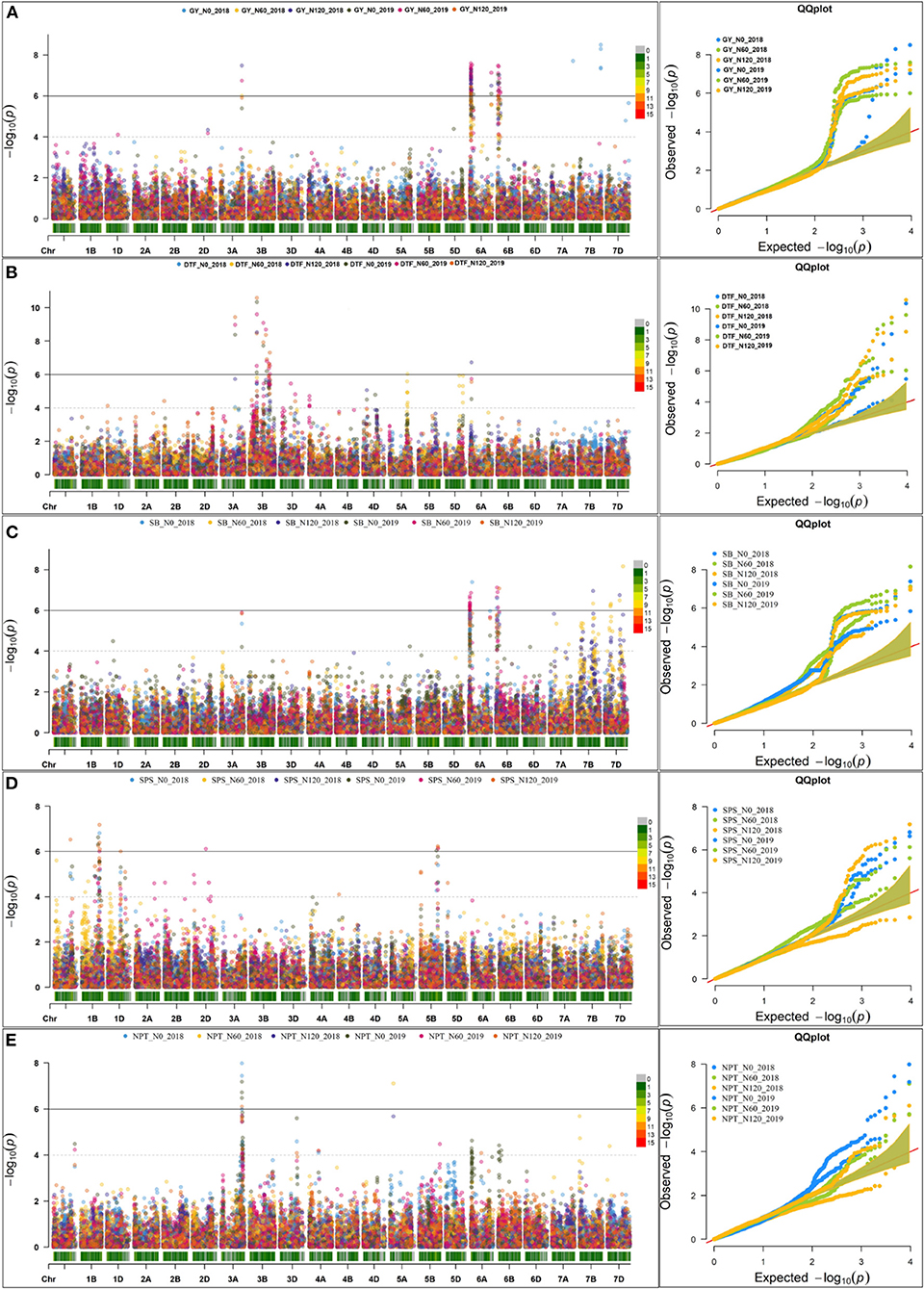
Figure 4. Manhattan plot and qq plot for the yield and yield-related traits across seasons at three different levels of nitrogen (N) (N0, N60, and N120). (A) grain yield (GY), (B) About 50% days to flowering (DTF), (C) shoot biomass (SB), (D) spikelets per spike (SPS), and (E) number of productive tillers.
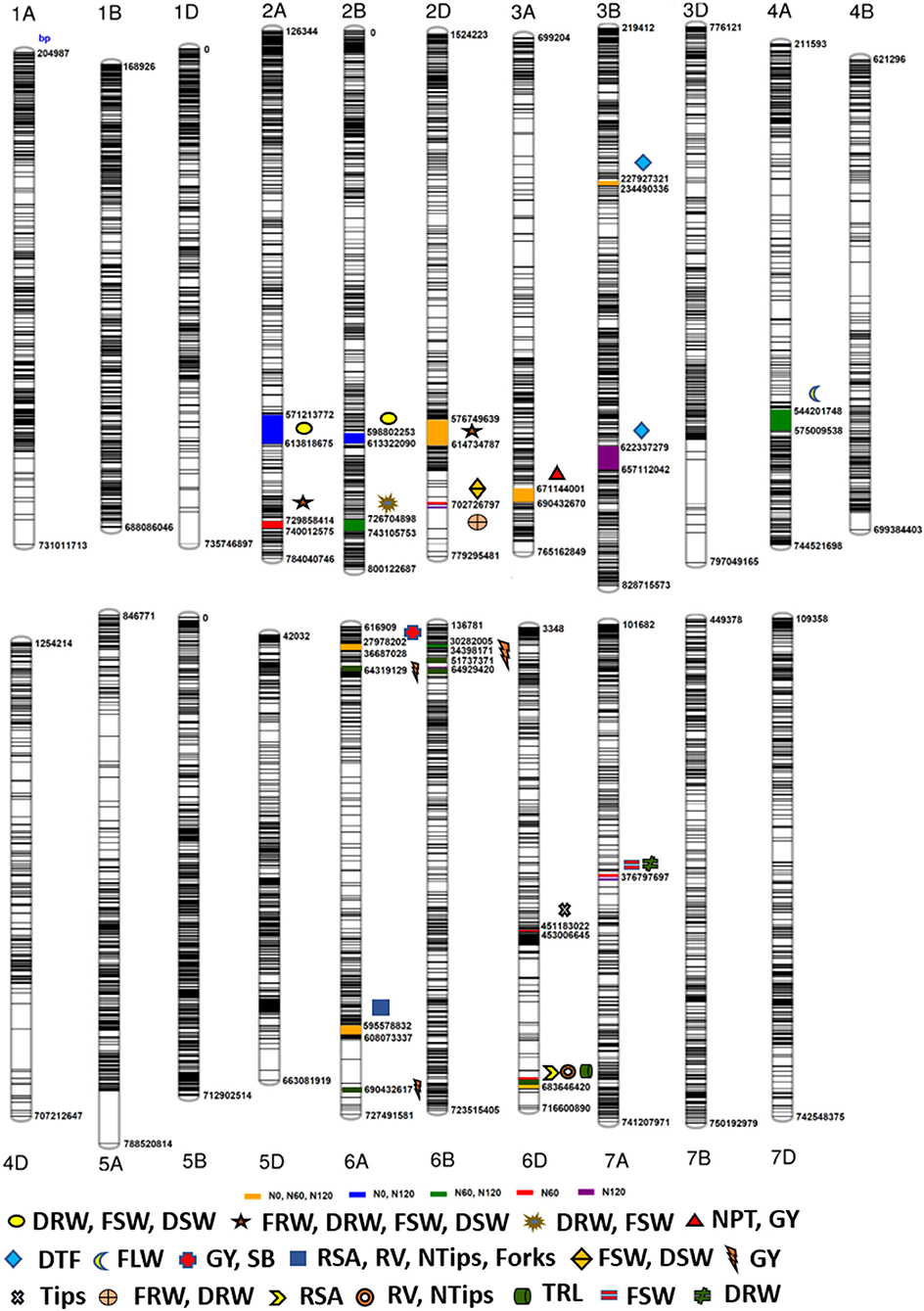
Figure 5. Schematic representation of the single nucleotide polymorphism (SNP) distribution along the 21 chromosomes of wheat. The chromosome map showing genomic regions where marker-trait associations (MTAs) for different nitrogen-use efficiency (NUE)-related trait, root traits, yield, and yield-related traits. The numbers below each chromosome indicate chromosome numbers. The bp represents the physical position of the SNPs on the chromosome in base pair.
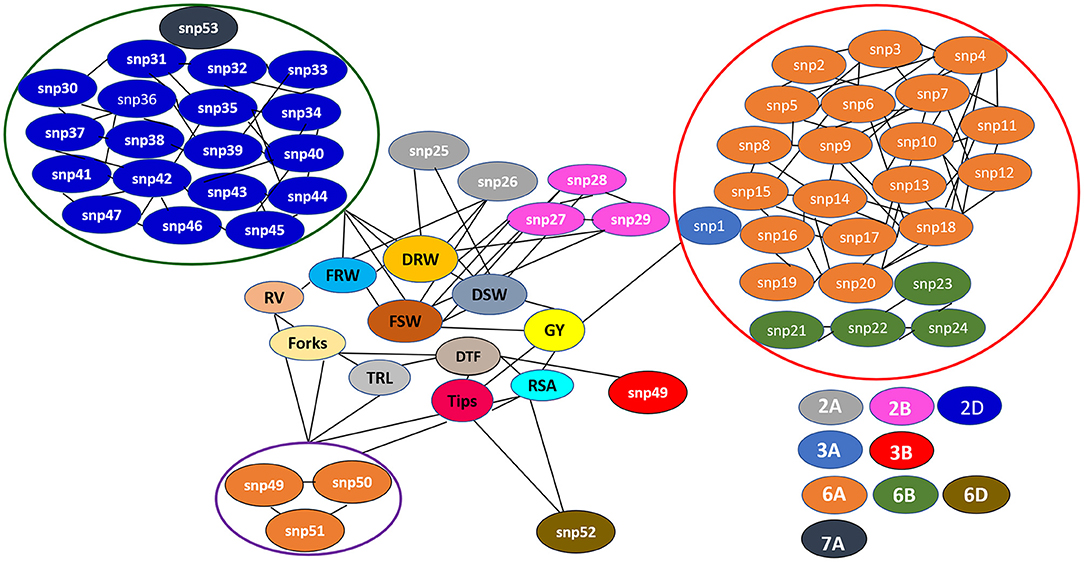
Figure 6. The single nucleotide polymorphism (SNP) network indicating consistent significant marker-trait interactions across seasons and treatments (N0, N60, and N120). snp1: AX-95136668; snp2: AX-94415776; snp3: AX-94978974; snp4: AX-94737868; snp5: AX-95210745; snp6: AX-95631197; snp7: AX-95011132; snp8: AX-95255669; snp9: AX-95219967; snp10: AX-95070275; snp11: AX-94970334; snp12: AX-94894393; snp13: AX-95249202; snp14: AX-94553503; snp15: AX-94696366; snp16: AX-94386201; snp17: AX-94835065; snp18: AX-94914391; snp19: AX-94606161; snp20: AX-95072891; snp21: AX-94511241; snp22: AX-94387975; snp23: AX-94511284; snp24: AX-94816913; snp25: AX-94607905; snp26: AX-94923560; snp27: AX-94705680; snp28: AX-95203088; snp29: AX-94601746; snp30: AX-94887553; snp31: AX-94735141; snp32: AX-94474729; snp33: AX-94835810; snp34: AX-95223893; snp35: AX-95003296; snp36: AX-94477325; snp37: AX-95197137; snp38: AX-94525577; snp39: AX-94702180; snp40: AX-94487982; snp41: AX-95190381; snp42: AX-95018936; snp43: AX-94457170; snp44: AX-94962360; snp45: AX-94829391; snp46: AX-94786006; snp47: AX-94695716; snp48: AX-95136655; snp49: AX-95113687; snp50: AX-94513497; snp51: AX-94911804; snp52: AX-94565231; snp53: AX-94676800.
Candidate Gene Identification and Functional Annotation
In order to identify candidate genes underlying the consistent MTAs, we surveyed putative candidates in a 1 Mb upstream and 1 Mb downstream region the identified significant SNPs using EnsemblPlants (http://plants.ensembl.org/index.html). Detailed information on the identified candidate genes is presented in Table 3.
The GO term of identified putative candidate genes was categorized into four groups according to their trait relatedness; The NUE uptake-related (LCC, SPAD, FSW, DSW), root morphological [maximum root length (MRL), TRL), RSA, root diameter (RD), RV, NF, N tips, FRW, and DRW], plant morphological [flag leaf length (FLL), FLW, plant height (PHT)] and GY/yield-attributing traits (days to flowering (DTF), SPS, NPT, SB, and GY]. Most of the putative candidate genes in NUE-uptake related traits across treatments were associated with protein phosphorylation/proteolysis, recognition of pollen, molybdoprotein cofactor biosynthetic process, and transmembrane transport (Supplementary Table 6). Some were part of the cellular component organization and molecular functions of binding molecules and ions, catalytic activity, peptidase activity, and transmembrane transport activity (Supplementary Table 6 and Supplementary Figure 6). The putative candidate genes for the root morphological traits were associated with N compound metabolic processes, phosphorylation, proteolysis, catabolic processes, response to stresses, and regulation of flower development by delineating the composition, and architecture of gene regulatory network underlying flower development and carbohydrate metabolism (Supplementary Table 6 and Supplementary Figure 7). The cellular components include chloroplast, ribosome, membrane, cytoplasm, nucleus, and mitochondria (Supplementary Tables 6, 7). The primary molecular functions related to these genes were catalytic activity (protease, peptidase, hydrolase, transferase, ligase, and oxidoreductase), and binding activity (small molecule binding, ion binding, lipid binding, and carbohydrate derivative binding) (Supplementary Table 6 and Supplementary Figure 7). The putative candidate genes for the plant morphological traits were mainly associated with phosphorylation, response to light-intensity, stress-related responses, and metabolic processes. They were related to the molecular functions of metal ion binding, catalytic activity, kinase activity, and DNA/RNA/ATP binding (Supplementary Table 6 and Supplementary Figure 8). The yield and yield-attributing traits-related putative candidate genes were associated with phosphorylation, metabolic process, protein folding, catabolic process, response to water-stress and light, flower development, and pollen recognition (Supplementary Table 6 and Supplementary Figure 8). The molecular functions include catalytic activity (peptidase, hydrolase, lyase, oxidoreductase, and transferase), binding activity (ion, metal, ATP/GTP, polysaccharide, protein, and DNA), and metabolic activity (Supplementary Table 6 and Supplementary Figure 9).
Selection of Promising Lines With Stable Performance for Use in Breeding
To identify stable breeding lines across treatments and seasons, a GGE biplot method was used. The first two principal components (PCs) explained 77.7% (PC1 = 50.3%, PC2 = 27.4%) of the total GGE variation in the data (Figure 7). The ranking of breeding lines based on their mean GY and stability across seasons and treatments (Supplementary Table 7) was used to identify 20 breeding lines with high and stable yields across seasons and treatments (Supplementary Table 8). The percentage increase in the GY of top 20 breeding lines derived from the nested introgression libraries possessing high and stable GY (kg ha−1) compared to the respective recipient parent averaged across two seasons under three different N treatments is presented in Figure 8. Based on GY data across seasons and treatments, the top 10 N-irresponsive (NIR-top grain yielders) and 10 N-responsive (NR-poor grain yielders) breeding lines were also identified (Table 4 and Figure 9).
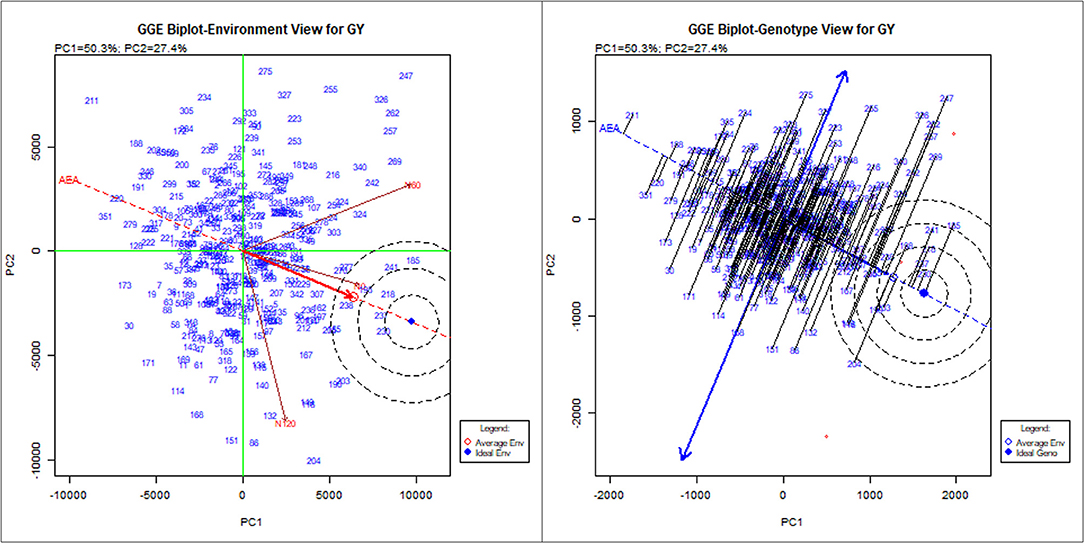
Figure 7. The GGE biplot showing the performance of 352 nested synthetic wheat introgression lines across seasons and treatments (N0, N60, and N120). The environment view refers to the three different levels of nitrogen (N) application: N0, N60, and N120. The genotype view refers to the 352 nested synthetic wheat introgression lines. The numeric number refers to the coding for the introgression lines, which is given in detail in the Supplementary Table 7.
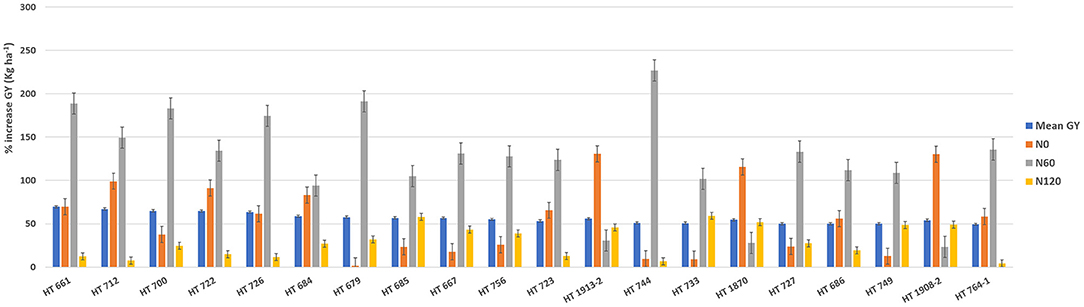
Figure 8. The percentage increase in the grain yield (GY) of top 20 breeding lines derived from the nested introgression libraries possessing high and stable GY (kg ha−1) compared to the respective recipient parent averaged across two seasons under three different nitrogen (N) treatments. The numeric values above the bar graph indicate the mean GY (kg ha−1) performance of breeding lines across seasons.
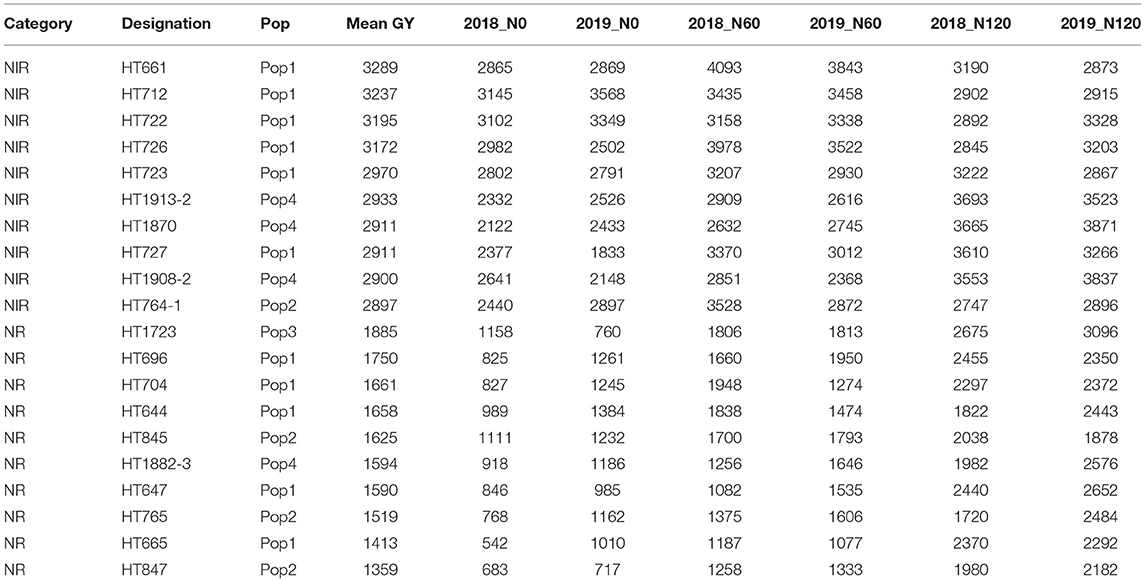
Table 4. The top 10 nitrogen irresponsive (NIR) and 10 nitrogen responsive (NR) breeding lines with contrasting grain yield (GY; kg ha−1) derived from pooled mean over two seasons and three treatments.
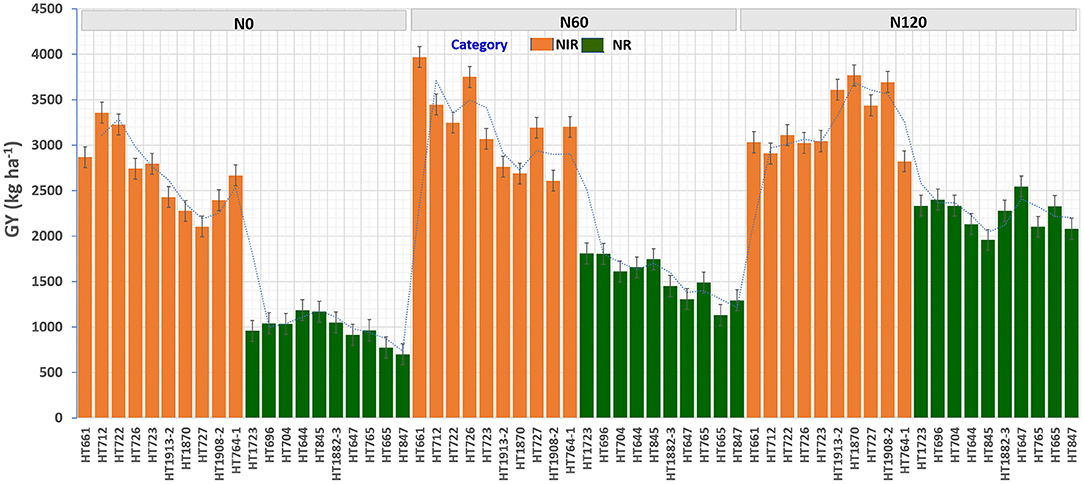
Figure 9. The grain yield (GY) (kg ha−1) performance of top 10 nitrogen irresponsive (NIR) and 10 nitrogen responsive (NR) breeding lines averaged over two seasons under three N treatments.
Further analysis was undertaken to assess the significant differences between the mean values of the allelic classes of MTAs for root growth and GY using the Kruskal–Wallis test. The presence of favorable alleles with significant differences was checked in promising breeding lines. This allowed for the selection of 20 promising breeding lines possessing favorable allele combinations for improving the plant root growth (Figure 10A) and grain GY under N limitation (Figure 10B).
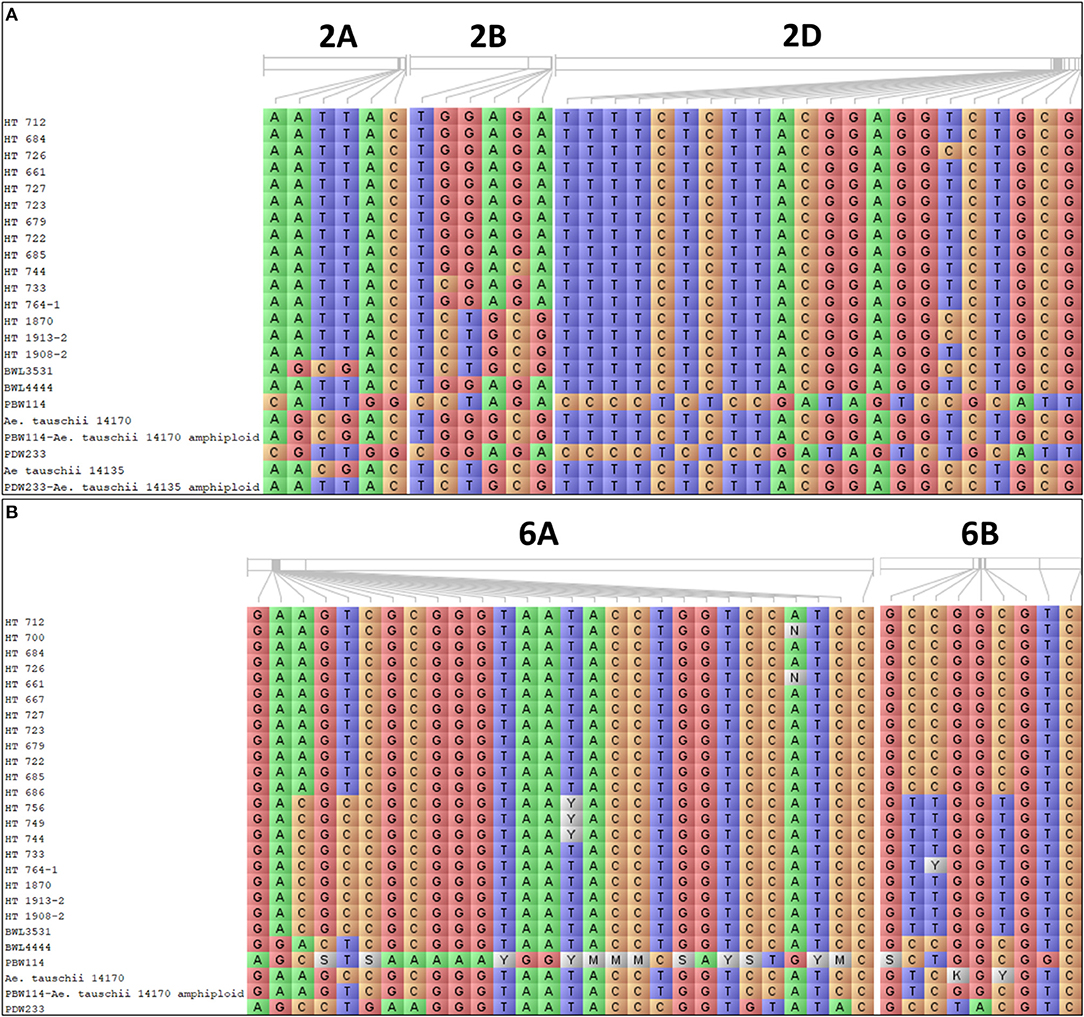
Figure 10. The allelic constitution of the selected promising breeding lines, wild accessions of Ae. tauschii, cultivated and synthetic wheats for the (A) root-related traits and (B) grain yield (GY).
Discussion
An increase in crop production by the development of high-yielding varieties is largely dependent on the supply of N fertilizers. Excessive application of nitrogenous fertilizer is becoming very expensive which accounts for the great loss of economic profit to the farmers in addition to the negative impacts on the environment (Hawkesford and Griffiths, 2019). The reliable phenotyping under low nitrogen input is very challenging and affected by genotype (G), environment (E), and the G x E interactions (Rao et al., 2018). Proper understanding of the genotype behavior, identification, and development of N-efficient genotypes without compromising the GY is a paramount need for improving the NUE. Notably, very few wheat breeding programs are targeting the development of N-efficient genotypes. In crop plants, such as wheat, the efforts are constrained due to a lack of variation in the cultivated germplasm for NUE. The narrow genetic diversity and fewer recombination events in the biparental mapping populations may result in poor quantitative trait loci (QTL) detection power (Gangurde et al., 2019). The next-generation high-resolution mapping populations, such as nested synthetic wheat introgression libraries used in the present study may provide a vast and untapped source of genetic variations for the NUE-related traits due to high numbers of recombination events. The use of synthetic hexaploid wheat in the present study presents an effective genetic resource for transferring the agronomically important genes from wild relatives to the common wheat. The introgression of favorable alleles associated with root traits and GY from Ae. tauschii wild accessions to cultivated wheat (Figure 10) indicated the potential of synthetic wheat providing new sources for improving yield potential and NUE when bred with the modern wheat varieties.
The different traits associated with N uptake and NUE were studied in nested synthetic wheat introgression libraries at three different nitrogen levels. The ANOVA results revealed the native variation across the genotypes toward the N response which had given the possibility to identify the NUE lines under different levels of N. The genotypic variations purely reveal the phenotypic plasticity of the breeding lines toward traits. The diverse responses have been observed among the breeding lines across different levels of N, despite similar growth conditions and an equal amount of nitrogenous fertilizer application in a given N level as indicated by significant differences among the genotypes within and across treatments and non-significant differences among the replications. Significant G x E, G x S, G x E, and G x T x S interactions indicated that the seasons and environments under different levels of N application were a critical factor in explaining the genotypic variance for the traits measured in the present study. The results reported in the present study concurred with other reported studies on rice (Srikanth et al., 2016) and wheat (Sial et al., 2005; Belete et al., 2018).
In general, the increase in GY was correlated with the increase in the rate of N fertilizer application, which might be due to the availability of sufficient N for the proper growth and development of the plants. Šarčević et al. (2014) reported a 10% reduction of GY at low N condition compared to normal condition in wheat. The significant and positive correlation among different root traits and GY and yield-attributing traits indicated complementary functional roles of the root traits in improving the GY by improving the nutrient acquisition from the soil. The collocation of MTAs for the correlated traits strengthens the significance of MTAs. A significant positive correlation between GY and NUE-related traits in wheat, maize, and oilseed rape (Fageria et al., 2010; He et al., 2017; Belete et al., 2018) signified the importance of NUE-related traits in improving the GY under limited N conditions.
Different mapping approaches using nested-association mapping (NAM) populations successfully exploited the genetics of complex traits and facilitated the discovery of candidate genes in rice (Fragoso et al., 2017), wheat (Hu et al., 2018; Jordan et al., 2018), maize (Yu et al., 2008; McMullen et al., 2009) and soybean (Song et al., 2017; Xavier et al., 2018). For NUE-related traits, significant genetic variations in hybrids, open-pollinated populations, large germplasm panels, backcross, and recombinant inbred line populations in different cereal crops, such as rice, wheat, maize, and oilseed rape were observed (Chen et al., 2014; Li et al., 2015; Vijayalakshmi et al., 2015; Ertiro et al., 2017; He et al., 2017; Rao et al., 2018). Mapping for NUE -related traits using different populations and mapping approaches highlight the complex nature of the trait.
In the present study, the nested synthetic wheat introgression libraries were designed for the identification of genomic regions associated with traits related to NUE using genome-wide association study (GWAS) keeping into account, the genetic effects produced in each genetic background. The associated SNPs were used to track the potential candidate genes associated with a particular trait of interest. The presence of high phenotypic variability in the nested synthetic introgression libraries coupled with the high marker density across the whole genome provided a strong base for the association mapping.
Interestingly, the genes responsive to nutrient uptake under water stress (Diédhiou et al., 2008; Janicka-Russak and Kabała, 2015; Wang et al., 2017), shoot growth, root and plant development (Wang et al., 2017), nutrient uptake and transport of various nutrients (Takahashi et al., 2012; Wang et al., 2014b; Weng et al., 2020) reported to be collocated with 126 Mb genomic region on Chr 2D constituting 25 MTAs which stood out as hot-spot for different traits (FRW, DRW, FSW, and DSW) in the present study. This indicates the positive interactions between root traits, nutrient uptake, and plant growth and development. The 7.7 Mb region on the short-arm of Chr 6A constituting 17 SNPs associated with GY showed collocation with the genes that were directly or indirectly involved in improving the GY in different cereal crops. These include the genes controlling flowering (Kania et al., 1997), panicle, and seed development (Jain et al., 2007; Li et al., 2011), GY (Terao et al., 2010), resistance to pathogenesis (Taniguchi et al., 2014; Wang et al., 2015; Niño et al., 2020) and abiotic stress tolerance (Brands and Ho, 2002; Palusa et al., 2007). The MTAs associated with different root traits, such as RSA, RV, tips, and forks in the present study were located near the earlier reported genes involved in regulating abscisic acid sensitivity and root growth development in Arabidopsis (Rodriguez et al., 2014) and adaptation under water stress conditions in wheat (Singh et al., 2017). Interestingly, the gene accelerating flowering in Arabidopsis (Hwang et al., 2019) was observed to be collocated with the SNP AX-95136655 associated with DTF on Chr 3B in the present study. The collocation of identified MTAs with earlier reported genes controlling the photosynthetic traits, root development, plant growth, nutrient uptake and transport, flowering, resistance to pathogenesis, and stress-responsive genes further confirms the contribution of these identified traits/MTAs in improving the N uptake/utilization and GY under N-limited conditions. The identified nitrogen irresponsive (NIR) breeding lines with favorable alleles in combination with the multiple traits might serve as potential donors for the development of N-efficient wheat varieties.
Conclusions
The nested synthetic introgression libraries covering extensive phenotypic variability coupled with huge genome coverage were used to identify the significant MTAs associated with nitrogen-use efficiency (NUE)-related traits in wheat. Significant phenotypic variations for the NUE-related traits, yield, and yield-related traits among genotypes, treatments, seasons, and their interactions (genotype x treatment, genotype x season, treatment x season, and genotype x treatment x season) were observed. Stable marker-trait associations (MTAs) identified for different traits measured in the present study comigrating with various genes associated with nitrogen (N) uptake/utilization and improving grain yield (GY) may help to harness their benefits in genomics-assisted breeding programs. The identification of N-efficient breeding lines may serve as novel donors in genomics-assisted introgression programs. The identification and introgression of superior haplotype improving NUE while maintaining GY using haplotype-based breeding may open new avenues in designing next-generation N-efficient high-yielding wheat varieties.
Data Availability Statement
The original data and related information presented in the study are included in the article/Supplementary Material, further inquiries can be directed to the corresponding author.
Author Contributions
NS and PC designed this study. AK provided the genotypic data of two populations and contributed to the development of nested introgression libraries. NS and MS conducted the field experiments. NS analyzed the data and wrote the manuscript. NS, SK, and PC provided resources. AK, MS, SK, VP-S, AS, AB, TB, and PC revised the manuscript. VP-S provided support with SPAD meter. All authors contributed to the article and approved the submitted version.
Funding
The work was compiled under the projects funded by the Department of Biotechnology, Govt. of India (Grant No. BT/IN/UK-VNC/42/RG/2015-16 and BT/PR30871/BIC/101/1159/2018).
Conflict of Interest
The authors declare that the research was conducted in the absence of any commercial or financial relationships that could be construed as a potential conflict of interest.
Publisher's Note
All claims expressed in this article are solely those of the authors and do not necessarily represent those of their affiliated organizations, or those of the publisher, the editors and the reviewers. Any product that may be evaluated in this article, or claim that may be made by its manufacturer, is not guaranteed or endorsed by the publisher.
Acknowledgments
We are thankful to the Department of Biotechnology, Govt. of India for providing grants.
Supplementary Material
The Supplementary Material for this article can be found online at: https://www.frontiersin.org/articles/10.3389/fpls.2021.738710/full#supplementary-material
References
Allen, A. M., Winfield, M. O., Burridge, A. J., Downie, R. C., Benbow, H. R., Barker, G. L., et al. (2017). Characterization of a Wheat Breeders' Array suitable for high-throughput SNP genotyping of global accessions of hexaploid bread wheat (Triticum aestivum). Plant Biotech. J. 15, 390–401. doi: 10.1111/pbi.12635
An, D., Su, J., Liu, Q., Zhu, Y., Tong, Y., Li, J., et al. (2006). Mapping QTLs for nitrogen uptake in relation to the early growth of wheat (Triticum aestivum L.). Plant Soil 284, 73–84. doi: 10.1007/s11104-006-0030-3
Arcondéguy, T., Jack, R., and Merrick, M. (2001). PII signal transduction proteins, pivotal players in microbial nitrogen control. Microbio. Mol. Bio. Rev. 65:80. doi: 10.1128/MMBR.65.1.80-105.2001
Arnesano, F., Banci, L., Benvenuti, M., Bertini, I., Calderone, V., Mangani, S., et al. (2003). The evolutionarily conserved trimeric structure of CutA1 proteins suggests a role in signal transduction. J. Bio. Chem. 278, 45999–46006. doi: 10.1074/jbc.M304398200
Bahrini, I., Ogawa, T., Kobayashi, F., Kawahigashi, H., and Handa, H. (2011). Overexpression of the pathogen-inducible wheat TaWRKY45 gene confers disease resistance to multiple fungi in transgenic wheat plants. Breed Sci. 61, 319–236. doi: 10.1270/jsbbs.61.319
Belete, F., Dechassa, N., Molla, A., and Tana, T. (2018). Effect of nitrogen fertilizer rates on grain yield and nitrogen uptake and use efficiency of bread wheat (Triticum aestivum L.) varieties on the Vertisols of central highlands of Ethiopia. Agri. Food Sec. 7, 1–2. doi: 10.1186/s40066-018-0231-z
Bianchet, C., Wong, A., Quaglia, M., Alqurashi, M., Gehring, C., Ntoukakis, V., et al. (2019). An Arabidopsis thaliana leucine-rich repeat protein harbors an adenylyl cyclase catalytic center and affects responses to pathogens. J. Plant Physio. 232, 12–22. doi: 10.1016/j.jplph.2018.10.025
Boeven, P. H., Longin, C. F. H., Leiser, W. L., Kollers, S., Ebmeyer, E., and Würschum, T. (2016). Genetic architecture of male floral traits required for hybrid wheat breeding. Theor. App. Genet. 129, 2343–2357. doi: 10.1007/s00122-016-2771-6
Brands, A., and Ho, T. H. (2002). Function of a plant stress-induced gene, HVA22 Synthetic enhancement screen with its yeast homolog reveals its role in vesicular traffic. Plant Physio. 130, 1121–1131. doi: 10.1104/pp.007716
Buchner, P., and Hawkesford, M. J. (2014). Complex phylogeny and gene expression patterns of members of the NITRATE TRANSPORTER 1/PEPTIDE TRANSPORTER family (NPF) in wheat. J. Exp. Bot. 65, 5697–5510. doi: 10.1093/jxb/eru231
Burkhead, J. L., Abdel-Ghany, S. E., Morrill, J. M., Pilon-Smits, E. A., and Pilon, M. (2003). The Arabidopsis thaliana CUTA gene encodes an evolutionarily conserved copper binding chloroplast protein. Plant J. 34, 856–867. doi: 10.1046/j.1365-313X.2003.01769.x
Cao, A., Xing, L., Wang, X., Yang, X., Wang, W., Sun, Y., et al. (2011). Serine/threonine kinase gene Stpk-V, a key member of powdery mildew resistance gene Pm21, confers powdery mildew resistance in wheat. Proc. Nat. Acad. Sci. U.S.A. 108, 7727–7732. doi: 10.1073/pnas.1016981108
Cavanagh, C. R., Chao, S., Wang, S., Huang, B. E., Stephen, S., Kiani, S., et al. (2013). Genome-wide comparative diversity uncovers multiple targets of selection for improvement in hexaploid wheat landraces and cultivars. Proc. Nat. Acad. Sci. U.S.A. 110, 8057–8062. doi: 10.1073/pnas.1217133110
Chen, B., Xu, K., Li, J., Li, F., Qiao, J., Li, H., et al. (2014). Evaluation of yield and agronomic traits and their genetic variation in 488 global collections of Brassica napus L. Genet. Res. Crop Evo. 61, 979–999. doi: 10.1007/s10722-014-0091-8
Chen, L., Xiang, S., Chen, Y., Li, D., and Yu, D. (2017). Arabidopsis WRKY45 interacts with the DELLA protein RGL1 to positively regulate age-triggered leaf senescence. Mol. Plant 10, 1174–1189. doi: 10.1016/j.molp.2017.07.008
Cheng, Z., Song, F., Shan, X., Wei, Z., Wang, Y., Dunaway-Mariano, D., et al. (2006). Crystal structure of human thioesterase superfamily member 2. Biochem. Bio. Res. Com. 349, 172–177. doi: 10.1016/j.bbrc.2006.08.025
Chevalier, D., Batoux, M., Fulton, L., Pfister, K., Yadav, R. K., Schellenberg, M., et al. (2005). STRUBBELIG defines a receptor kinase-mediated signaling pathway regulating organ development in Arabidopsis. Proc. Nat. Acad. Sci. U.S.A. 102, 9074–9079. doi: 10.1073/pnas.0503526102
Coque, M., Martin, A., Veyrieras, J. B., Hirel, B., and Gallais, A. (2008). Genetic variation for N-remobilization and postsilking N-uptake in a set of maize recombinant inbred lines: QTL detection and coincidences. Theor. App. Genet. 117, 729–747. doi: 10.1007/s00122-008-0815-2
Deng, Z., Cui, Y., Han, Q., Fang, W., Li, J., and Tian, J. (2017). Discovery of consistent QTLs of wheat spike-related traits under nitrogen treatment at different development stages. Front. Plant Sci. 8:2120. doi: 10.3389/fpls.2017.02120
Diédhiou, C. J., Popova, O. V., Dietz, K. J., and Golldack, D. (2008). The SNF1-type serine-threonine protein kinase SAPK4 regulates stress-responsive gene expression in rice. BMC Plant Bio. 8, 1–3. doi: 10.1186/1471-2229-8-49
Ding, W., Lin, L., Zhang, B., Xiang, X., Wu, J., Pan, Z., et al. (2015). OsKASI, a β-ketoacyl-[acyl carrier protein] synthase I, is involved in root development in rice (Oryza sativa L.). Planta 242, 203–213. doi: 10.1007/s00425-015-2296-2
Earl, D. A. (2012). STRUCTURE HARVESTER: a website and program for visualizing STRUCTURE output and implementing the Evanno method. Conser. Genet. Res. 4, 359–361. doi: 10.1007/s12686-011-9548-7
Ertiro, B. T., Beyene, Y., Das, B., Mugo, S., Olsen, M., Oikeh, S., et al. (2017). Combining ability and testcross performance of drought-tolerant maize inbred lines under stress and non-stress environments in Kenya. Plant Breed. 136, 197–205. doi: 10.1111/pbr.12464
Fageria, N. K., De Morais, O. P., and Dos Santos, A. B. (2010). Nitrogen use efficiency in upland rice genotypes. J. Plant Nut. 33, 1696–1711. doi: 10.1080/01904167.2010.496892
FAO (2019). FAO World Fertilizer Trends and Outlook to 2020. Available online at: http://www.fao.org/3/a-i6895e.pdf (accessed June 11, 2019).
Fontaine, J. X., Ravel, C., Pageau, K., Heumez, E., Dubois, F. B., Hirel, L., et al. (2009). A quantitative genetic study for elucidating the contribution of glutamine synthetase, glutamate dehydrogenase and other nitrogen-related physiological traits to the agronomic performance of common wheat. Theor. App. Genet. 119, 645–662. doi: 10.1007/s00122-009-1076-4
Fragoso, C. A., Moreno, M., Wang, Z., Heffelfinger, C., Arbelaez, L. J., Aguirre, J. A., et al. (2017). Genetic architecture of a rice nested association mapping population. G3 Genes Genomes Genetics 7, 1913–1926. doi: 10.1534/g3.117.041608
Gaju, O., Allard, V., Martre, P., Snape, J., Heumez, E., LeGouis, J., et al. (2011). Identification of traits to improve the nitrogen-use efficiency of wheat genotypes. Field Crop Res. 123, 139–152. doi: 10.1016/j.fcr.2011.05.010
Gallego-Giraldo, L., Posé, S., Pattathil, S., Peralta, A. G., Hahn, M. G., Ayre, B. G., et al. (2018). Elicitors and defense gene induction in plants with altered lignin compositions. New Phytol. 219, 1235–1251. doi: 10.1111/nph.15258
Gangurde, S. S., Kumar, R., Pandey, A. K., Burow, M., Laza, H. E., Nayak, S. N., et al. (2019). “Climate-smart groundnuts for achieving high productivity and improved quality: current status, challenges, and opportunities,” in: Genomic Designing of Climate-Smart Oilseed Crops, ed C. Kole (Cham: Springer Nature Switzerland AG). doi: 10.1007/978-3-319-93536-2_3
Habash, D. Z., Bernard, S., Schondelmaier, J., Weyen, J., and Quarrie, S. A. (2007). The genetics of nitrogen use in hexaploid wheat: N utilization, development and yield. Theor. App. Genet. 14, 403–419. doi: 10.1007/s00122-006-0429-5
Hawkesford, M. J., and Griffiths, S. (2019). Exploiting genetic variation in nitrogen use efficiency for cereal crop improvement. Current. Opin. Plant. Bio. 49, 35–42. doi: 10.1016/j.pbi.2019.05.003
He, H., Yang, R., Li, Y., Ma, A., Cao, L., Wu, X., et al. (2017). Genotypic variation in nitrogen utilization efficiency of oilseed rape (Brassica napus) under contrasting N supply in pot and field experiments. Front. Plant Sci. 8:1825. doi: 10.3389/fpls.2017.01825
Hickman, J. E., Palm, C. A., Mutuo, P., Melillo, J. M., and Tang, J. (2014). Nitrous oxide (N2O) emissions in response to increasing fertilizer addition in maize (Zea mays L.) agriculture in western Kenya. Nut. Cycl. Agro. 100, 177–187. doi: 10.1007/s10705-014-9636-7
Hu, J., Guo, C., Wang, B., Ye, J., Liu, M., Wu, Z., et al. (2018). Genetic properties of a nested association mapping population constructed with K semi-winter and spring oilseed rapes. Front. Plant Sci. 9:1740. doi: 10.3389/fpls.2018.01740
Hwang, K., Susila, H., Nasim, Z., Jung, J. Y., and Ahn, J. H. (2019). Arabidopsis ABF3 and ABF4 transcription factors act with the NF-YC complex to regulate SOC1 expression and mediate drought-accelerated flowering. Mol. Plant 12, 489–505. doi: 10.1016/j.molp.2019.01.002
Jain, M., Nijhawan, A., Arora, R., Agarwal, P., Ray, S., Sharma, P., et al. (2007). Genome-wide analysis, classification, temporal and spatial gene expression during panicle and seed development, and regulation by light and abiotic stress. Plant Physio. 143, 1467–1483. doi: 10.1104/pp.106.091900
Janiak, A., Kwaśniewski, M., and Szarejko, I. (2016). Gene expression regulation in roots under drought. J. Exp. Bot. 67, 1003–1014. doi: 10.1093/jxb/erv512
Janicka-Russak, M., and Kabała, K. (2015). The Role of Plasma Membrane H+-ATPase in Salinity Stress of Plants. Cham: Springer. doi: 10.1007/978-3-319-08807-5_3
Jordan, K. W., Wang, S., He, F., Chao, S., Lun, Y., Paux, E., et al. (2018). The genetic architecture of genome-wide recombination rate variation in allopolyploid wheat revealed by nested association mapping. Plant J. 95, 1039–1054. doi: 10.1111/tpj.14009
Jung, Y. J., Melencion, S. M. B., Lee, E. S., Park, J. H., Alinapon, C. V., Oh, H. T., et al. (2015). Universal stress protein exhibits a redox-dependent chaperone function in Arabidopsis and enhances plant tolerance to heat shock and oxidative stress. Front. Plant Sci. 6:1141. doi: 10.3389/fpls.2015.01141
Kania, T., Russenberger, D., Peng, S., Apel, K., and Melzer, S. (1997). FPF1 promotes flowering in Arabidopsis. Plant Cell 9, 1327–1338. doi: 10.1105/tpc.9.8.1327
Kitagawa, K., Kurinami, S., Oki, K., Abe, Y., Ando, T., Kono, I., et al. (2010). A novel kinesin 13 protein regulating rice seed length. Plant Cell Physio. 51, 1315–1329. doi: 10.1093/pcp/pcq092
Kobayashi, Y., Motose, H., Iwamoto, K., and Fukuda, H. (2011). Expression and genome-wide analysis of the xylogen-type gene family. Plant Cell Physio. 52, 1095–1106. doi: 10.1093/pcp/pcr060
Laperche, A., Le Gouis, J., Hanocq, E., and Brancourt-Hulmel, M. (2008). Modelling nitrogen stress with probe genotypes to assess genetic parameters and genetic determinism of winter wheat tolerance to nitrogen constraint. Euphytica 161, 259–271. doi: 10.1007/s10681-007-9433-3
Lesniewicz, K., Karlowski, W. M., Pienkowska, J. R., Krzywkowski, P., and Poreba, E. (2013). The plant S1-like nuclease family has evolved a highly diverse range of catalytic capabilities. Plant Cell Physio. 54, 1064–1078. doi: 10.1093/pcp/pct061
Li, M., Tang, D., Wang, K., Wu, X., Lu, L., Yu, H., et al. (2011). Mutations in the F-box gene LARGER PANICLE improve the panicle architecture and enhance the grain yield in rice. Plant Biotech J. 9, 1002–1013. doi: 10.1111/j.1467-7652.2011.00610.x
Li, P., Chen, F., Cai, H., Liu, J., Pan, Q., Liu, Z., et al. (2015). A genetic relationship between nitrogen use efficiency and seedling root traits in maize as revealed by QTL analysis. J. Exp. Bot. 66, 3175–3188. doi: 10.1093/jxb/erv127
Li, Y. D., Wang, Y. J., Tong, Y. P., Gao, J. G., Zhang, J. S., and Chen, S. Y. (2005). QTL mapping of phosphorus deficiency tolerance in soybean (Glycine max L. Merr.). Euphytica 142, 137–142. doi: 10.1007/s10681-005-1192-4
Liang, Q., Cheng, X., Mei, M., Yan, X., and Liao, H. (2010). QTL analysis of root traits as related to phosphorus efficiency in soybean. Ann. Bot. 106, 223–234. doi: 10.1093/aob/mcq097
Liao, H., Yan, X., Rubio, G., Beebe, S. E., Blair, M. W., and Lynch, J. P. (2004). Genetic mapping of basal root gravitropism and phosphorus acquisition efficiency in common bean. Funct. Plant Bio. 31, 959–970. doi: 10.1071/FP03255
Lin, Z. J. D., Liebrand, T. W., Yadeta, K. A., and Coaker, G. (2015). PBL13 is a serine/threonine protein kinase that negatively regulates Arabidopsis immune responses. Plant Physio. 169, 2950–2962. doi: 10.1104/pp.15.01391
Liu, H., Hu, M., Wang, Q., Cheng, L., and Zhang, Z. (2018). Role of papain-like cysteine proteases in plant development. Front. Plant Sci. 9:1717. doi: 10.3389/fpls.2018.01717
Liu, W. X., Zhang, F. C., Zhang, W. Z., Song, L. F., Wu, W. H., and Chen, Y. F. (2013). Arabidopsis Di19 functions as a transcription factor and modulates PR1, PR2, and PR5 expression in response to drought stress. Mol. Plant 6, 1487–1502. doi: 10.1093/mp/sst031
Mackay, I. J., Bansept-Basler, P., Barber, T., Bentley, A. R., Cockram, J., Gosman, N., et al. (2014). An eight-parent multiparent advanced generation inter-cross population for winter-sown wheat: creation, properties, and validation. G3: Genes Genom. Genet. 4, 1603–1610. doi: 10.1534/g3.114.012963
Mahjourimajd, S., Kuchel, H., Langridge, P., and Okamoto, M. (2016). Evaluation of Australian wheat genotypes for response to variable nitrogen application. Plant Soil 399, 247–255. doi: 10.1007/s11104-015-2694-z
McGinnis, K. M. (2003). The Arabidopsis SLEEPY1 Gene Encodes a putative F-Box subunit of an SCF E3 Ubiquitin Ligase. Plant Cell 15, 1120–1130. doi: 10.1105/tpc.010827
McMullen, M. D., Kresovich, S., Villeda, H. S., Bradbury, P., Li, H., Sun, Q., et al. (2009). Genetic properties of the maize nested association mapping population. Science 325, 737–740. doi: 10.1126/science.1174320
Ming, F., Zheng, X., Mi, G., He, P., Zhu, L., and Zhang, F. (2000). Identification of quantitative trait loci affecting tolerance to low phosphorus in rice (Oryza Sativa L.). Chinese Sci. Bull. 45, 520–525. doi: 10.1007/BF02887097
Mo, P., Zhu, Y., Liu, X., Zhang, A., Yan, C., and Wang, D. (2007). Identification of two phosphatidylinositol/phosphatidylcholine transfer protein genes that are predominately transcribed in the flowers of Arabidopsis thaliana. J. Plant Physio. 164, 478–486. doi: 10.1016/j.jplph.2006.03.014
Mok, D. W., and Mok, M. C. (2001). Cytokinin metabolism and action. Ann. Rev. Plant Bio. 52, 89–118. doi: 10.1146/annurev.arplant.52.1.89
Morita, S., Suga, T., and Yamazaki, K. (1988). The relationship between root length density and yield in rice plants. Jpn. J. Crop Sci. 57, 438–443. doi: 10.1626/jcs.57.438
Motose, H., Sugiyama, M., and Fukuda, H. (2004). A proteoglycan mediates inductive interaction during plant vascular development. Nature 429, 873–878. doi: 10.1038/nature02613
Ninfa, A. J., and Atkinsonm, M. R. (2000). PII signal transduction proteins. Trends Micro. 8, 172–179. doi: 10.1016/S0966-842X(00)01709-1
Niño, M. C., Kang, K. K., and Cho, Y. G. (2020). Genome-wide transcriptional response of papain-like cysteine protease-mediated resistance against Xanthomonas oryzae pv. oryzae in rice. Plant Cell Rep. 39, 457–472. doi: 10.1007/s00299-019-02502-1
Obara, M., Sato, T., Sasaki, S., Kashiba, K., Nagano, A., Nakamura, I., et al. (2004). Identification and characterization of a QTL on chromosome 2 for cytosolic glutamine synthetase content and panicle number in rice. Theor. App. Genet. 110, 1–10. doi: 10.1007/s00122-004-1828-0
Orsel, M., Chopin, F., Leleu, O., Smith, S. J., Krapp, A., Daniel-Vedele, F., et al. (2006). Characterization of a two-component high-affinity nitrate uptake system in Arabidopsis. Physiology and protein-protein interaction. Plant Physio. 142, 1304–1317. doi: 10.1104/pp.106.085209
Palusa, S. G., Ali, G. S., and Reddy, A. S. (2007). Alternative splicing of pre-mRNAs of Arabidopsis serine/arginine-rich proteins: regulation by hormones and stresses. Plant J. 49, 1091–1107. doi: 10.1111/j.1365-313X.2006.03020.x
Pandey, M. K., Monyo, E., Ozias-Akins, P., Liang, X., Guimarães, P., Nigam, S. N., et al. (2012). Advances in Arachis genomics for peanut improvement. Biotechnol. Adv. 30, 639–651. doi: 10.1016/j.biotechadv.2011.11.001
Pandey, M. K., Roorkiwal, M., Singh, V. K., Ramalingam, A., Kudapa, H., Thudi, M., et al. (2016). Emerging genomic tools for legume breeding: current status and future prospects. Front. Plant Sci. 7:455. doi: 10.3389/fpls.2016.00455
Prasad, M., Varshney, R. K., Kumar, A. H. S., Balyan, P. C., Sharma, K. J., et al. (1999). A microsatellite marker associated with a QTL for grain protein content on chromosome arm 2DL of bread wheat. Theor. App. Genet. 99, 341–345. doi: 10.1007/s001220051242
Pritchard, J. K., and Wen, W. (2004). Documentation for the STRUCTURE Software Version 2. Chicago, IL: Department of Human Genetics, University of Chicago.
Purcell, S., Neale, B., Todd-Brown, K., Thomas, L., Ferreira, M. A., Bender, D., et al. (2007). PLINK: a tool set for whole-genome association and population-based linkage analyses. The Amer. J. Hum. Genet. 81, 559–575. doi: 10.1086/519795
Qian, Y., Chen, C., Jiang, L., Zhang, J., and Ren, Q. (2019). Genome-wide identification, classification and expression analysis of the JmjC domain-containing histone demethylase gene family in maize. BMC Genom. 20:256. doi: 10.1186/s12864-019-5633-1
Quesada, V. (2016). The roles of mitochondrial transcription termination factors (MTERFs) in plants. Physio. Plant 157, 389–399. doi: 10.1111/ppl.12416
Rao, I. S., Neeraja, C. N., Srikanth, B., Subrahmanyam, D., Swamy, K. N., Rajesh, K., et al. (2018). Identification of rice landraces with promising yield and the associated genomic regions under low nitrogen. Sci. Rep. 8:9200. doi: 10.1038/s41598-018-27484-0
Rasheed, A., and Xia, X. (2019). From markers to genome-based breeding in wheat. Theor. App. Genet. 132, 767–784. doi: 10.1007/s00122-019-03286-4
Rautengarten, C., Usadel, B., Neumetzler, L., Hartmann, J., Büssis, D., and Altmann, T. (2008). A subtilisin-like serine protease essential for mucilage release from Arabidopsis seed coats. Plant J. 54, 466–480. doi: 10.1111/j.1365-313X.2008.03437.x
Reddy, P. P. (2017). Fertilizer Management. Agro-ecological Approaches to Pest Management for Sustainable Agriculture. Singapore: Springer. doi: 10.1007/978-981-10-4325-3
Rober-Kleber, N., Albrechtová, J. T., Fleig, S., Huck, N., Michalke, W., Wagner, E., et al. (2003). Plasma membrane H+-ATPase is involved in auxin-mediated cell elongation during wheat embryo development. Plant Physio. 131, 1302–1312. doi: 10.1104/pp.013466
Rodriguez, L., Gonzalez-Guzman, M., Diaz, M., Rodrigues, A., Izquierdo-Garcia, A. C., Peirats-Llobet, M., et al. (2014). C2-domain abscisic acid-related proteins mediate the interaction of PYR/PYL/RCAR abscisic acid receptors with the plasma membrane and regulate abscisic acid sensitivity in Arabidopsis. Plant Cell 26, 4802–4820. doi: 10.1105/tpc.114.129973
Russo, T. A., Tully, K., Palm, C., and Neill, C. (2017). Leaching losses from Kenyan maize cropland receiving different rates of nitrogen fertilizer. Nut. Cyc. Agro. 108, 195–209. doi: 10.1007/s10705-017-9852-z
Sandhu, N., Subedi, S. R., Singh, V. K., Sinha, P., Kumar, S., Singh, S. P., et al. (2019). Deciphering the genetic basis of root morphology, nutrient uptake, yield, and yield-related traits in rice under dry direct- seeded cultivation systems. Sci. Rep. 9:9334. doi: 10.1038/s41598-019-45770-3
Sandhu, N., Torres, R. O., Sta Cruz, M. T., Maturan, P. C., Jain, R., Kumar, A., et al. (2015). Traits and QTLs for development of dry direct-seeded rainfed rice varieties. J. Exp. Bot. 66, 225–244. doi: 10.1093/jxb/eru413
Šarčević, H., Jukić, K., Ikić, I., and Lovrić, A. (2014). Estimation of quantitative genetic parameters for grain yield and quality in winter wheat under high and low nitrogen fertilization. Euphytica 199, 57–67. doi: 10.1007/s10681-014-1154-9
Scott, M. F., Ladejobi, O., Amer, S., Bentley, A. R., Biernaskie, J., Boden, S. A., et al. (2020). Multi-parent populations in crops: A toolbox integrating genomics and genetic mapping with breeding. Heredity 125, 396–416. doi: 10.1038/s41437-020-0336-6
Shimono, M., Sugano, S., Nakayama, A., Jiang, C. J., Ono, K., Toki, S., et al. (2007). Rice WRKY45 plays a crucial role in benzothiadiazole-inducible blast resistance. Plant Cell 19, 2064–2076. doi: 10.1105/tpc.106.046250
Sial, M. A., Arain, M. A., Khanzada, S. H. A. M. A. D. A.D., Naqvi, M. H., Dahot, M. U., and Nizamani, N. A. (2005). Yield and quality parameters of wheat genotypes as affected by sowing dates and high temperature stress. Pak. J. Bot. 37, 575.
Singh, B., Khurana, P., Khurana, J. P., and Singh, P. (2017). Gene encoding vesicle-associated membrane protein-associated protein from Triticum aestivum (TaVAP) confers tolerance to drought stress. Cell Stress Chaperones 23, 411–428. doi: 10.1007/s12192-017-0854-1
Song, Q., Yan, L., Quigley, C., Jordan, B. D., Fickus, E., Schroeder, S., et al. (2017). Genetic characterization of the soybean nested association mapping population. Plant Genome 10, 1–14. doi: 10.3835/plantgenome2016.10.0109
Srikanth, B., Rao, I. S., Surekha, K., Subrahmanyam, D., Voleti, S. R., and Neeraja, C. N. (2016). Enhanced expression of OsSPL14 gene and its association with yield components in rice (Oryza sativa) under low nitrogen conditions. Gene 576, 441–450. doi: 10.1016/j.gene.2015.10.062
Steffens, B., and Rasmussen, A. (2016). The physiology of adventitious roots. Plant Physio. 170, 603–617. doi: 10.1104/pp.15.01360
Su, J., Xiao, Y., Li, M., Liu, Q., Li, B., Tong, Y., et al. (2006). Mapping QTLs for phosphorus-deficiency tolerance at wheat seedling stage. Plant Soil 281, 25–36. doi: 10.1007/s11104-005-3771-5
Su, J. Y., Zheng, Q., Li, H. W., Li, B., Jing, R. L., Tong, Y. P., et al. (2009). Detection of QTLs for phosphorus use efficiency in relation to agronomic performance of wheat grown under phosphorus sufficient and limited conditions. Plant Sci. 176, 824–836. doi: 10.1016/j.plantsci.2009.03.006
Subedi, S. R., Sandhu, N., Singh, V. K., Sinha, P., Kumar, S., Singh, S. P., et al. (2019). Genome-wide association study reveals significant genomic regions for improving yield, adaptability of rice under dry direct seeded cultivation condition. BMC Genom. 20:471. doi: 10.1186/s12864-019-5840-9
Sun, H., Qian, Q., Wu, K., Luo, J., Wang, S., Zhang, C., et al. (2014). Heterotrimeric G proteins regulate nitrogen-use efficiency in rice. Nat. Genet. 46, 652–656. doi: 10.1038/ng.2958
Takahashi, K., Hayashi, K. I., and Kinoshita, T. (2012). Auxin activates the plasma membrane H+-ATPase by phosphorylation during hypocotyl elongation in Arabidopsis. Plant Physio. 159, 632–641. doi: 10.1104/pp.112.196428
Tang, B., Xie, L., Yi, T., Lv, J., Yang, H., Cheng, X., et al. (2019). Genome-wide identification and characterization of the mitochondrial transcription termination factors (mTERFs) in Capsicum annuum L. Inter. J. Mol. Sci. 21:269. doi: 10.3390/ijms21010269
Taniguchi, S., Hosokawa-Shinonaga, Y. U. M. I., Tamaoki, D., Yamada, S., Akimitsu, K., and Gomi, K. (2014). Jasmonate induction of the monoterpene linalool confers resistance to rice bacterial blight and its biosynthesis is regulated by JAZ protein in rice. Plant Cell Environ. 37, 451–461. doi: 10.1111/pce.12169
Terao, T., Nagata, K., Morino, K., and Hirose, T. (2010). A gene controlling the number of primary rachis branches also controls the vascular bundle formation and hence is responsible to increase the harvest index and grain yield in rice. Theor. App. Genet. 120, 875–893. doi: 10.1007/s00122-009-1218-8
Varshney, R. K., Mohan, S. M., Gaur, P. M., Gangarao, N. V. P. R., Pandey, M. K., Bohra, A., et al. (2013). Achievements and prospects of genomics-assisted breeding in three legume crops of the semi-arid tropics. Biotechnol. Adv. 31, 1120–1134. doi: 10.1016/j.biotechadv.2013.01.001
Vijayalakshmi, P., Vishnukiran, T., Kumari, B. R., Srikanth, B., Rao, I. S., Swamy, K. N., et al. (2015). Biochemical and physiological characterization for nitrogen use efficiency in aromatic rice genotypes. Field Crops Res. 179, 132–143. doi: 10.1016/j.fcr.2015.04.012
Wang, H., Xu, Q., Kong, Y. H., Chen, Y., Duan, J. Y., Wu, W. H., et al. (2014a). Arabidopsis WRKY45 transcription factor activates PHOSPHATE TRANSPORTER1; 1 expression in response to phosphate starvation. Plant Physio. 164, 2020–2029. doi: 10.1104/pp.113.235077
Wang, S., Wong, D., Forrest, K., Allen, A., Chao, S., Huang, B. E., et al. (2014b). Characterization of polyploid wheat genomic diversity using a high-density 90 000 single nucleotide polymorphism array. Plant Biotech. J. 12, 787–796. doi: 10.1111/pbi.12183
Wang, Y., Cordewener, J. H., America, A. H., Shan, W., Bouwmeester, K., and Govers, F. (2015). Arabidopsis lectin receptor kinases LecRK-IX. 1 and LecRK-IX. 2 are functional analogs in regulating Phytophthora resistance and plant cell death. Mol. Plant-Microbe Inter. 28, 1032–1048. doi: 10.1094/MPMI-02-15-0025-R
Wang, Y., Pang, C., Li, X., Hu, Z., Lv, Z., Zheng, B., et al. (2017). Identification of tRNA nucleoside modification genes critical for stress response and development in rice and Arabidopsis. BMC Plant Bio. 17, 1–15. doi: 10.1186/s12870-017-1206-0
Weng, L., Zhang, M., Wang, K., Chen, G., Ding, M., Yuan, W., et al. (2020). Potassium alleviates ammonium toxicity in rice by reducing its uptake through activation of plasma membrane H+-ATPase to enhance proton extrusion. Plant Physio. Biochem. 151 429–437. doi: 10.1016/j.plaphy.2020.03.040
Winfield, M. O., Allen, A. M., Burridge, A. J., Barker, G. L., Benbow, H. R., Wilkinson, P. A., et al. (2016). High-density SNP genotyping array for hexaploid wheat and its secondary and tertiary gene pool. Plant Biotech. J. 14, 1195–1206. doi: 10.1111/pbi.12485
Winkel-Shirley, B. (2001). Flavonoid biosynthesis. A colorful model for genetics, biochemistry, cell biology, and biotechnology. Plant Physio. 126, 485–493. doi: 10.1104/pp.126.2.485
Wissuwa, M., Yano, M., and Ae, N. (1998). Mapping of QTLs for phosphorus-deficiency tolerance in rice (Oryza sativa L.). Theor. App. Genet. 97, 777–783. doi: 10.1007/s001220050955
Xavier, A., Jarquin, D., Howard, R., Ramasubramanian, V., Specht, J. E., Graef, G. L., et al. (2018). Genome-Wide analysis of grain yield stability and environmental interactions in a multiparental soybean population. G3: Genes Genomes Genetics 8, 519–529. doi: 10.1534/g3.117.300300
Xavier, A., Xu, S., Muir, W. M., and Rainey, K. M. (2015). NAM: association studies in multiple populations. Bioinformatics 31, 3862–3864. doi: 10.1093/bioinformatics/btv448
Xu, Y., Wang, R., Tong, Y., Zhao, H., Xie, Q., Liu, D., et al. (2014). Mapping QTLs for yield and nitrogen-related traits in wheat: influence of nitrogen and phosphorus fertilization on QTL expression. Theor. App. Genet. 127, 59–72. doi: 10.1007/s00122-013-2201-y
Yamaya, T., Obara, M., Nakajima, H., Sasaki, S., Hayakawa, T., and Sato, T. (2002). Genetic manipulation and quantitative-trait loci mapping for nitrogen recycling in rice. J. Exp. Bot. 53, 917–925. doi: 10.1093/jexbot/53.370.917
Yan, X., Liao, H., Beebe, S. E., Blair, M. W., and Lynch, J. P. (2004). QTL mapping of root hair and acid exudation traits and their relationship to phosphorus uptake in common bean. Plant Soil 265, 17–29. doi: 10.1007/s11104-005-0693-1
Yang, J. C., Zhang, H., and Zhang, J. H. (2012). Root morphology and physiology in relation to the yield formation of rice. J. Integr. Agric. 11, 920–926. doi: 10.1016/S2095-3119(12)60082-3
Yang, L., Ji, W., Zhu, Y., Gao, P., Li, Y., Cai, H., et al. (2010). GsCBRLK, a calcium/calmodulin-binding receptor-like kinase, is a positive regulator of plant tolerance to salt and ABA stress. J. Exp. Bot. 61, 2519–2533. doi: 10.1093/jxb/erq084
Yokoo, T., Saito, H., Yoshitake, Y., Xu, Q., Asami, T., Tsukiyama, T., et al. (2014). Se14, encoding a JmjC domain-containing protein, plays key roles in long-day suppression of rice flowering through the demethylation of H3K4me3 of RFT1. PLoS ONE 9:e96064. doi: 10.1371/journal.pone.0096064
Yu, J., Holland, J. B., McMullen, M. D., and Buckler, E. S. (2008). Genetic design and statistical power of nested association mapping in maize, Genetics 178, 539–551. doi: 10.1534/genetics.107.074245
Yu, W., Kan, Q., Zhang, J., Zeng, B., and Chen, Q. (2015). Role of the plasma membrane [H+-ATPase in the regulation of organic acid exudation under aluminium toxicity and phosphorus deficiency. Plant Signal Behav. 1:e1106660. doi: 10.1080/15592324.2015.1106660
Yun, H. S., Kwaaitaal, M., Kato, N., Yi, C., Park, S., Sato, M. H., et al. (2013). Requirement of vesicle-associated membrane protein 721 and 722 for sustained growth during immune responses in Arabidopsis. Mol. Cells 35, 481–488. doi: 10.1007/s10059-013-2130-2
Zhang, M., Chen, C., Froehlich, J. E., TerBush, A. D., and Osteryoung, K. W. (2015). Roles of Arabidopsis PARC6 in coordination of the chloroplast division complex and negative regulation of FtsZ assembly. Plant Physio. 170, 250–262. doi: 10.1104/pp.15.01460
Zhao, K., Tung, C. W., Eizenga, G. C., Wright, M. H., Ali, M. L., Price, A. H., et al. (2011). Genome-wide association mapping reveals a rich genetic architecture of complex traits in Oryza sativa. Nat. Commun. 2:467. doi: 10.1038/ncomms1467
Zhao, Y. F., Peng, T., Sun, H. Z., Teotia, S., Wen, H. L., Du, Y. X., et al. (2019). miR1432-Os ACOT (Acyl-CoA thioesterase) module determines grain yield via enhancing grain filling rate in rice. Plant Biotech. J. 17, 712–723. doi: 10.1111/pbi.13009
Zhou, S., Wang, Y., Li, W., Zhao, Z., Ren, Y., Wang, Y., et al. (2011). Pollen semi-sterility1 encodes a kinesin-1-like protein important for male meiosis, anther dehiscence, and fertility in rice. Plant Cell 23, 111–129. doi: 10.1105/tpc.109.073692
Zhu, J., Kaeppler, S. M., and Lynch, J. P. (2005). Mapping of QTL controlling root hair length in maize (Zea mays L.) under phosphorus deficiency. Plant Soil 270, 299–310. doi: 10.1007/s11104-004-1697-y
Keywords: GWAS, MTAs, Nitrogen, SNP markers, Wheat, Yield, synthetic wheat introgression lines
Citation: Sandhu N, Kaur A, Sethi M, Kaur S, Varinderpal-Singh, Sharma A, Bentley AR, Barsby T and Chhuneja P (2021) Genetic Dissection Uncovers Genome-Wide Marker-Trait Associations for Plant Growth, Yield, and Yield-Related Traits Under Varying Nitrogen Levels in Nested Synthetic Wheat Introgression Libraries. Front. Plant Sci. 12:738710. doi: 10.3389/fpls.2021.738710
Received: 09 July 2021; Accepted: 30 August 2021;
Published: 04 October 2021.
Edited by:
Wusirika Ramakrishna, Central University of Punjab, IndiaReviewed by:
Jitendra Kumar, Indian Institute of Pulses Research (ICAR), IndiaAmira M. I. Mourad, Assiut University, Egypt
Copyright © 2021 Sandhu, Kaur, Sethi, Kaur, Varinderpal-Singh, Sharma, Bentley, Barsby and Chhuneja. This is an open-access article distributed under the terms of the Creative Commons Attribution License (CC BY). The use, distribution or reproduction in other forums is permitted, provided the original author(s) and the copyright owner(s) are credited and that the original publication in this journal is cited, in accordance with accepted academic practice. No use, distribution or reproduction is permitted which does not comply with these terms.
*Correspondence: Parveen Chhuneja, pchhuneja@pau.edu; orcid.org/0000-0002-8599-9479