- 1Queensland Alliance for Agriculture and Food Innovation (QAAFI), The University of Queensland, Brisbane, QLD, Australia
- 2Corteva Agriscience, Johnston, IA, United States
The diverse consequences of genotype-by-environment (GxE) interactions determine trait phenotypes across levels of biological organization for crops, challenging our ambition to predict trait phenotypes from genomic information alone. GxE interactions have many implications for optimizing both genetic gain through plant breeding and crop productivity through on-farm agronomic management. Advances in genomics technologies have provided many suitable predictors for the genotype dimension of GxE interactions. Emerging advances in high-throughput proximal and remote sensor technologies have stimulated the development of “enviromics” as a community of practice, which has the potential to provide suitable predictors for the environment dimension of GxE interactions. Recently, several bespoke examples have emerged demonstrating the nascent potential for enhancing the prediction of yield and other complex trait phenotypes of crop plants through including effects of GxE interactions within prediction models. These encouraging results motivate the development of new prediction methods to accelerate crop improvement. If we can automate methods to identify and harness suitable sets of coordinated genotypic and environmental predictors, this will open new opportunities to upscale and operationalize prediction of the consequences of GxE interactions. This would provide a foundation for accelerating crop improvement through integrating the contributions of both breeding and agronomy. Here we draw on our experience from improvement of maize productivity for the range of water-driven environments across the US corn-belt. We provide perspectives from the maize case study to prioritize promising opportunities to further develop and automate “enviromics” methodologies to accelerate crop improvement through integrated breeding and agronomic approaches for a wider range of crops and environmental targets.
Introduction
Sustainable improvement of on-farm crop yield productivity, through improving yield potential and yield stability, is a complex long-term objective for both breeders and agronomists (Duvick et al., 2004; Hall and Richards, 2013; Fischer et al., 2014; Hatfield and Walthall, 2015; Beres et al., 2020; Cooper et al., 2021; Hunt et al., 2021). Heterogeneity of current environmental conditions that impact crop yield and the influences of climate change continually challenge the definition of the Target Population of Environments (TPE) for both breeders and agronomists (Chapman et al., 2012; Harrison et al., 2014; Lobell et al., 2015; Voss-Fels et al., 2019; Hammer et al., 2020; Cooper et al., 2021; Smith et al., 2021). Many of the important environmental details required for interpretation of experimental results and to enable prediction of genotype reaction-norms are not currently captured routinely for the multi-environment trials (METs) conducted by breeders and agronomists. Further, for most crop breeding programs the relationships between the environments sampled in METs and the dominant environmental conditions of the TPE are neither well understood nor adequately quantified (Cooper and DeLacy, 1994; Cooper et al., 2021). Improved sensor technologies and prediction methodologies are urgently required to characterize and study environments within breeding and agronomy METs and to quantify the relationships between the environments sampled in METs for all stages of crop improvement programs and their importance for the TPE (Messina et al., 2020; Crespo-Herrera et al., 2021; Kusmec et al., 2021; Potgieter et al., 2021; Smith et al., 2021).
Genotype-by-environment interactions (GxE) have been long recognized as important factors impacting successful application of selection in plant breeding and for the yield stability of cultivars released from breeding programs (Comstock and Moll, 1963; Finlay and Wilkinson, 1963; Allard and Bradshaw, 1964; Eberhart and Russell, 1966; Blum, 1988; Nyquist and Baker, 1991; Cooper and DeLacy, 1994; Cooper et al., 2020). Similarly, agronomists have a long history of investigating the environmental responses of cultivars developed by breeding programs under on-farm management systems (French and Schultz, 1984; Passioura, 2002, 2006, 2007; Sadras and Angus, 2006; Kirkegaard and Hunt, 2010; Van Ittersum et al., 2013; Holzworth et al., 2014; Assefa et al., 2018; Archontoulis et al., 2020; Hunt et al., 2021). Farmers seek improved technology combinations based on genotypes and agronomic management that can consistently deliver yield productivity close to the potential of their on-farm environments, while managing the risk of crop failure (Hammer et al., 2014; Hunt et al., 2021). As a constructive step toward improving the predictability of on-farm crop productivity, there has been continual refinement of the definition of environments in METs and the agricultural TPE to recognize the important role of crop management and for investigation of the influences of genotype-by-environment-by-management (GxExM) interactions (Kirkegaard and Hunt, 2010; Hammer et al., 2014, 2020; Hatfield and Walthall, 2015; Beres et al., 2020; Peng et al., 2020; Cooper et al., 2021; Hunt et al., 2021; Potgieter et al., 2021; Smith et al., 2021). Thus, we can study genetic improvements from the perspective of the breeder, crop management improvements from the perspective of the agronomist, and improvement in genotype–management technology combinations from the perspective of the farmer. In all cases, an improved understanding of the environmental context for achievable yield performance can enhance their contributions to further improve on-farm crop productivity. Hence the importance of the nascent technologies and methods of enviromics.
Perspective: Harnessing Enviromics for Crop Improvement
While the use of the terminology “enviromics” is relatively recent, the motivations and concepts for studying agricultural environments in METs and the TPE to accelerate crop improvement have a long history. There have been many calls for enhanced attention to environmental characterization to accelerate crop improvement. Plant breeders have long sought environmental definitions and covariates to assist interpretation of plant responses and the GxE interactions detected in METs and to understand their relevance for the on-farm TPE (Finlay and Wilkinson, 1963; Allard and Bradshaw, 1964; Baker, 1988; Blum, 1988; Cooper and Hammer, 1996; Boer et al., 2007; Heslot et al., 2014; Jarquín et al., 2014; Pauli et al., 2016; Xu, 2016; Ly et al., 2018; Bustos-Korts et al., 2019, 2021; Millet et al., 2019; Costa-Neto et al., 2020, 2021; Porker et al., 2020; Crossa et al., 2021; Li et al., 2021; Resende et al., 2021; Smith et al., 2021). The role of water availability and impact of drought on crop yield and investigations to determine the traits contributing to crop productivity under drought conditions have received significant attention from breeders (e.g., Blum, 1988; Fukai and Cooper, 1995; Campos et al., 2004; Bänziger et al., 2006; Ribaut, 2006; Messina et al., 2011, 2018; Cooper et al., 2014a), agronomists (French and Schultz, 1984; Sadras and Angus, 2006; Kirkegaard and Hunt, 2010; Van Ittersum et al., 2013; Hunt et al., 2021), and physiologists (Richards and Passioura, 1989; Ludlow and Muchow, 1990; Bolaños and Edmeades, 1996; Passioura, 2002, 2006, 2007; Messina et al., 2011, 2015, 2019; Araus and Cairns, 2014; Hammer et al., 2014; Araus et al., 2018; Sinclair, 2018; Simmons et al., 2021). Complexity, cost, and the timeliness of detailed measurements of the water status of environments and genotypic variation for plant responses to water deficits under field conditions have limited adoption and application of many discoveries and methods to the scale of breeding programs. Recently, new proximal and remote sensor technologies and data modelling capabilities have become available to enhance characterization of environments and measure plant responses under field conditions at higher throughput and at greater scales to enhance applications for crop improvement and yield prediction (Pauli et al., 2016; Guan et al., 2017; Araus et al., 2018; Messina et al., 2018; Van Eeuwijk et al., 2019; Cooper et al., 2020; Messina et al., 2020; Peng et al., 2020; Schwalbert et al., 2020; Costa-Neto et al., 2021; Jain et al., 2021; Jin et al., 2021; Potgieter et al., 2021; Smith et al., 2021; Yang et al., 2021).
Terminology has emerged in combination with the advances in the technologies for studying the characteristics of environments in METs, for their applications to assist interpretation of GxE interactions, and to quantify reaction-norms for genotypes. To ensure we benefit from the deep history of studying agricultural environments and how they influence plant responses, crop performance, and adaptation for the TPE, we include what has previously been referred to as environmental characterization (Fukai and Cooper, 1995; Chapman et al., 2000; Löffler et al., 2005; Chenu et al., 2011; Mathews et al., 2011; Kholová et al., 2013; Pauli et al., 2016; Smith et al., 2021) and envirotyping (Cooper et al., 2014b; Pauli et al., 2016; Xu, 2016; Porker et al., 2020; Couëdel et al., 2021; Smith et al., 2021) within scope of the applications of enviromics for crop improvement. The convention we adopt is that the terminology of enviromics represents the collective of activities that are undertaken to study, measure, and quantify the characteristics of micro- and macroenvironments and how they influence responses of plants (genotypes) at the field, MET and TPE levels. Within the domain of enviromics, the terminology of environmental characterization is used to refer to the applied activities that use the methodologies and technologies of enviromics to characterize the important environmental variables that are influential on the plant responses observed within field conditions and experimental METs. Further, the concept and terminology of envirotyping are applied to uses of the available environmental characterization information to identify appropriate groupings of the environments sampled in METs and to quantify their relationships to the TPE to assist interpretations of plant responses to the environments, any GxE interactions, and differences in reaction-norms of genotypes at the levels of the MET and the TPE. The level of envirotyping resolution that can be applied extends along a continuum from coarse-grained to fine-grained, depending on the target situation (Cooper et al., 2014a,b, 2020). We encourage the constructive transdisciplinary dialogue that is required to provide an improved understanding of the environmental variables that determine important GxE interactions and ultimately identification of sets of coordinated environmental and genomic predictors of variation for genotypic reaction-norms within the MET and the TPE (e.g., Messina et al., 2011; Ly et al., 2018; Bustos-Korts et al., 2019, 2021; Millet et al., 2019).
Example: Harnessing Enviromics for Maize Yield Improvement in the US Corn-Belt
The genetic improvement in grain yield of temperate maize for the US corn-belt provides a useful case study for considering past and potential roles of enviromic technologies to contribute to strategies focused on accelerating yield improvement for the future TPE. Past contributions to improvements in on-farm yield productivity of maize from both genetics and agronomy have been documented (Russell, 1991; Duvick et al., 2004; Duvick, 2005; Cooper et al., 2014a). The influences of GxE and GxExM interactions on grain yield variation have been investigated (Boer et al., 2007; Messina et al., 2009; Gaffney et al., 2015; Assefa et al., 2018; Cooper et al., 2020; Rogers et al., 2021). Environmental heterogeneity within the TPE and its influence on GxE interactions for yield have been quantified (Löffler et al., 2005; Cooper et al., 2020; Crespo-Herrera et al., 2021; Rogers et al., 2021) and the important influence of drought on grain yield recognized (Boyer et al., 2013; Gaffney et al., 2015; Kimm et al., 2020). The environmental and genetic determinants of GxE interactions for grain yield of maize have been investigated by variance components, stability analysis, and more recently through extensions of these approaches using molecular markers and crop models (Eberhart and Russell, 1966; Boer et al., 2007; Gage et al., 2017; Messina et al., 2018; Cooper et al., 2020; Rogers et al., 2021). Agronomic management strategies that reduce on-farm yield gaps have been developed (Grassini et al., 2011; Assefa et al., 2018). There is ongoing interest in using improved understanding of the environmental determinants of yield performance, adaptation, and reaction-norms of maize hybrids that provide a focus for testing and further development of enviromic methodologies (Cooper et al., 2014a,b, 2020; Gage et al., 2017; Messina et al., 2020; Kusmec et al., 2021; Rogers et al., 2021).
Trait GxE interactions identified from the results of METs can be investigated in terms of models of the reaction-norms of genotypes across an environmental gradient (Figure 1A). When modelling genotype reaction-norms, in the absence of informative descriptors to order the environments, the mean yield of all genotypes that were tested in an environment has been used as an environmental gradient for such investigations, e.g., Finlay and Wilkinson (1963), Allard and Bradshaw (1964), and Eberhart and Russell (1966) are early examples. In such cases, there has always been a recognition of the need for more informative environmental descriptors to enhance the predictive skill of models for new environments outside of the sample obtained in METs.
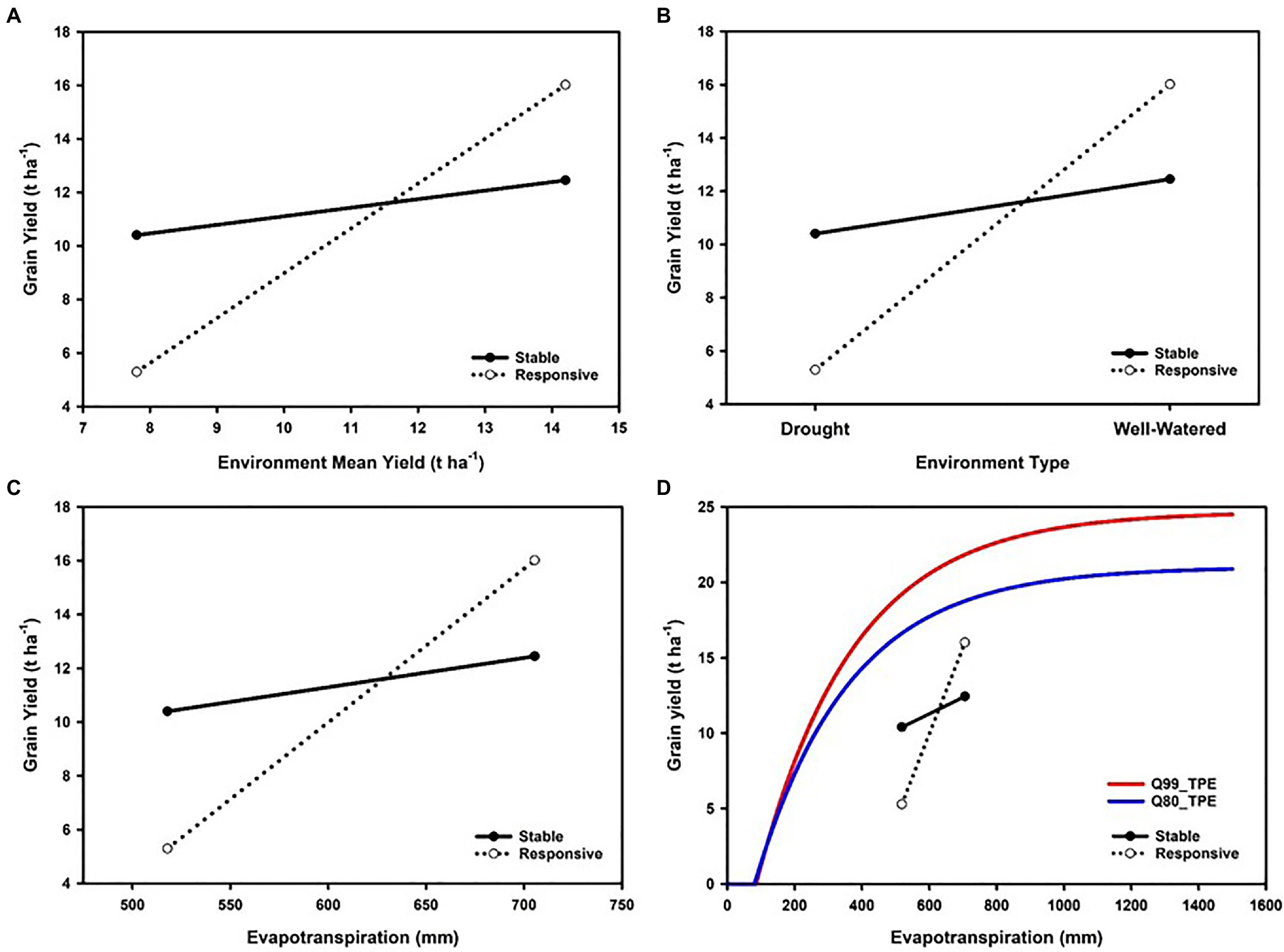
Figure 1. Enviromics progression from (A) coarse-grained to (D) fine-grained characterization of environmental gradients for a maize multi-environment trial (MET) to assist interpretation of grain yield genotype-by-environment (GxE) interactions and genotypic variation for reaction-norms: (A) environments distinguished on mean grain yield of all genotypes tested (e.g., Finlay and Wilkinson, 1963), (B) environments distinguished on levels of water inputs; water-limited (drought) versus water-sufficient (well-watered), (C) environments distinguished on levels of water availability quantified as whole season crop evapotranspiration, (D) environments distinguished on evapotranspiration and considered in relation to the modelled 99th percentile and 80th percentile yield-evapotranspiration fronts for modelled genotype-by-environment-by-management (GxExM) scenarios for the US corn-belt following the methodology of Cooper et al. (2020).
An important distinction is drawn between GxE interactions that are a consequence of differences in magnitude of genetic variance among environments and those that result in changes in the rank of the genotypes across the environmental gradient (Baker, 1988; Cooper and DeLacy, 1994; Van Eeuwijk et al., 2001, 2016). Such analyses can be applied to the empirical results and genomic predictions for any stage of a breeding program (Cooper et al., 2014a,b). When water availability is recognized as an important contributor to the differences in mean yield levels of environments, the environmental gradient can be investigated and characterized in terms of environmental descriptors of water availability, e.g., plant available water content in the soil, and crop evapotranspiration. Many approaches have been attempted, ranging from coarse-grained to fine-grained characterization of environmental differences in water availability. A common coarse-grained approach is to categorize environments as either water-limited (drought) or water-sufficient (irrigated or well-watered), e.g., Gaffney et al. (2015) (Figure 1B). To complement such environment characterization of METs, plant breeders and agronomists have conducted controlled side-by-side experiments imposing treatments based on levels of water inputs through managing irrigation levels to represent water-deficit and water-sufficient environments expected in the TPE. When drought is of sufficient importance in the TPE to become a long-term breeding target, this has in some cases justified the establishment of specialized field-based research facilities to enable more fine-grained consideration of the continuum of environments ranging from water-deficient to water-sufficient (e.g., Fischer et al., 1989; Cooper et al., 1995, 2014a, 2020; Weber et al., 2012; Rebetzke et al., 2013).
Enviromic technologies have been incorporated within the operations of such dedicated field-based drought research facilities to enable the detailed characterization of the environmental conditions within experiments (Cooper et al., 2014a; Reynolds et al., 2020) and to understand and predict important GxExM interactions at the different stages of a breeding program (Cooper et al., 2014a,b). Such integration of enviromic technologies into breeding operations has enabled definition and quantification of key environmental variables, detailed studies of trait contributions to yield variation within breeding program cycles and prediction of trait contributions to yield improvement for the TPE (Messina et al., 2011, 2015, 2018; Cooper et al., 2014a). The upscaling of the environmental characterization of water availability in drought experiments, based on proximal and remote sensor technologies, has been enabled through using the environmental measurements directly, e.g., vapor pressure deficit, evapotranspiration, rainfall, temperature, or as inputs to crop models to quantify daily water balance throughout the crop life cycle, from planting to harvest (French and Schultz, 1984; Chapman et al., 2000; Sadras and Angus, 2006; Gaffney et al., 2015; Messina et al., 2015). The integrated use of the environmental measurements with a suitable crop model (e.g., Messina et al., 2019) enables a continuum of coarse-grained to fine-grained characterization of environments. Recent applications of the integrated sensor and crop modelling approach have investigated characterization of environmental water sufficiency in terms of crop level evapotranspiration and the timing of water deficits in relation to crop growth and development using the concept of crop-level water supply/demand ratio determined on a daily time step (Muchow et al., 1996; Chapman et al., 2000; Chenu et al., 2011; Messina et al., 2015; Cooper et al., 2020). Therefore, using such advances in enviromic capabilities to characterize breeding and agronomy METS, the environmental gradient used to study genotypic reaction-norms can be refined from the coarse-grained view of a contrast between water-limited and water-sufficient (Figure 1B) and quantified in terms of important environmental variables, such as the crop-level evapotranspiration (Figure 1C).
Environmental descriptors such as seasonal crop-level evapotranspiration also have been extensively used by agronomists to study the expected yield potential of crops based on water availability for the range of environments that comprise a TPE and to quantify the yield-gaps between the yield potential and the on-farm water-limited yield levels that are achieved by farmers (French and Schultz, 1984; Sadras and Angus, 2006; Van Ittersum et al., 2013; Fischer et al., 2014; Sadras et al., 2015). A curated global yield-gap atlas is available for a range of crops (Van Bussel et al., 2015).1 Applying the methodology for yield-gap analysis, Cooper et al. (2020) developed a water-limited yield front for the US corn-belt by parameterizing a crop model for a range of maize hybrids. The water-limited yield fronts they obtained represent a yield potential reaction-norm where yield was related to in-season crop evapotranspiration.
Crop evapotranspiration provides a useful environmental descriptor to study GxE interactions in plant breeding METs and to study GxExM interactions and yield-gaps in agronomy METs. Therefore, given suitable enviromic technologies to measure crop evapotranspiration (Guan et al., 2017; He et al., 2019; Cooper et al., 2020) a common view of genotypic reaction-norms for breeding and agronomic applications can be constructed (Figure 1D). Applying yield-evapotranspiration fronts estimated for maize in the US corn-belt, Cooper et al. (2020) investigated the opportunities to close yield-gaps from an integrated breeding and agronomic perspective. With availability of genotypic and environmental predictors, such an integrated view of GxExM interactions can be predicted for all stages of a plant breeding program to inform selection and hybrid advancement by breeders and to assist agronomists to provide decision support services to identify suitable combined genotype and management technologies for farmers to reduce on-farm yield productivity gaps (Cooper et al., 2014b). Figure 2 provides an example of such an integrated view, constructed by superimposing a yield-evapotranspiration front modelled for the TPE of the US corn-belt and the empirical yield results for two contrasting maize hybrids that were obtained from a MET where an enviromic approach was applied to quantify the range of crop evapotranspiration levels sampled in the MET. With this integrated view the GxExM interactions associated with the empirical yield results from the MET can be investigated from a breeding perspective selecting for improved yield potential and yield stability and from an agronomy perspective to identify genotype and management technology combinations to close yield-gaps given the crop available water and the achievable yield for an environment.
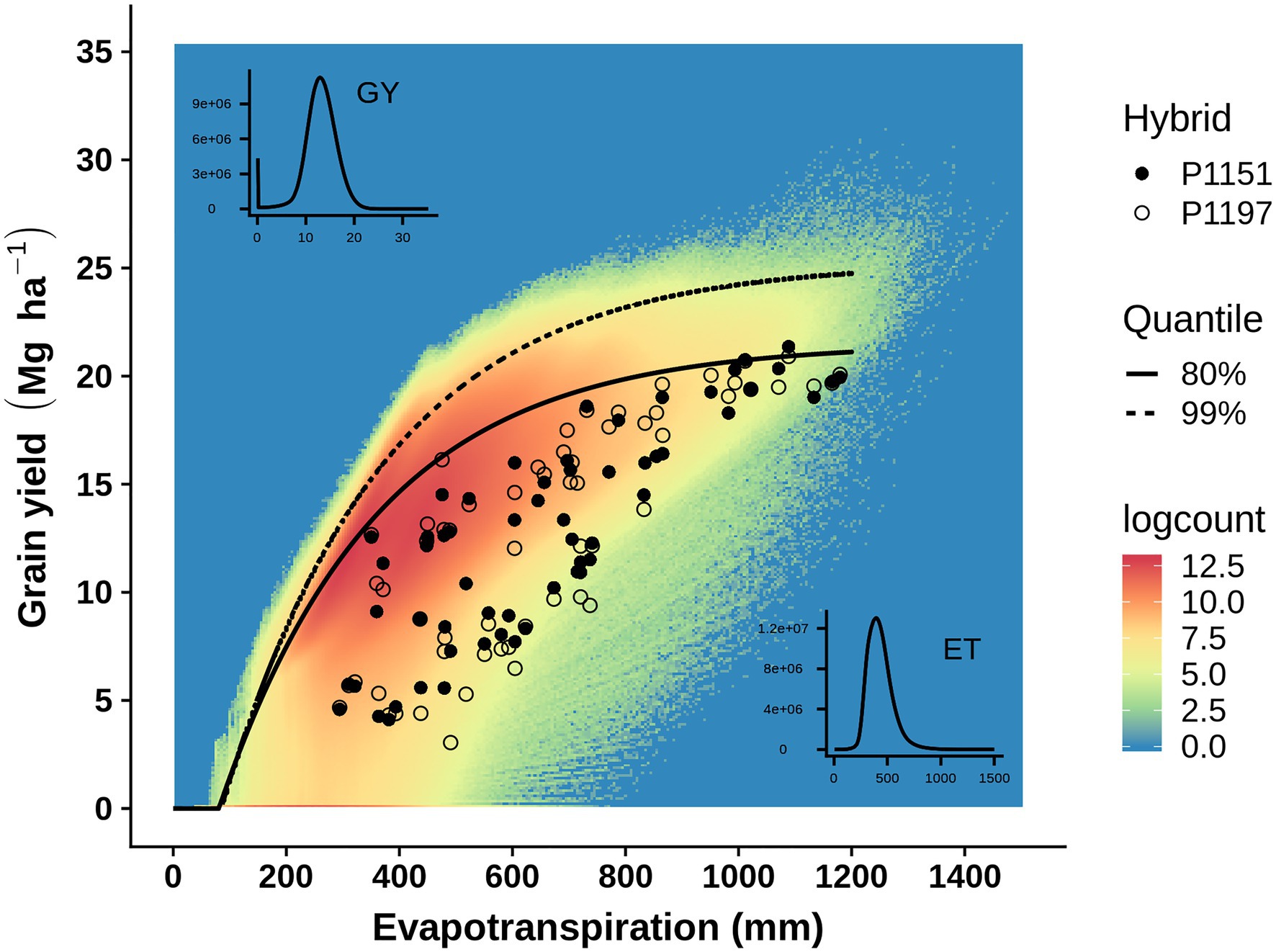
Figure 2. Enviromics applied to assess how well a MET represents a target population of environments (TPE). Example of empirical grain yield results from a maize MET compared to GxExM expectations for the US corn-belt TPE. To compare the empirical MET results and modelled TPE expectations, environments were characterized in terms of crop evapotranspiration to quantify the gradient from water-limited (low evapotranspiration) to water-sufficient environments (high evapotranspiration). Enviromics approaches were applied to the environments sampled in the MET to obtain the inputs for a crop model, which was used to estimate crop evapotranspiration following Cooper et al. (2020). The MET example focuses on the yield comparison between two hybrids, P1197 (Responsive; Figure 1) and P1151 (Stable; Figure 1). The empirical yield-evapotranspiration results for the MET are superimposed on the simulated cloud of yield-evapotranspiration outcomes for the TPE. The estimates of the 99th and 80th percentile yield-evapotranspiration fronts for the TPE provide a reference for interpreting the empirical yield-evapotranspiration results hybrid reaction-norms obtained for the MET. Whenever the empirical yield for a GxExM combination falls below the 80th percentile yield-evapotranspiration front a yield-gap is associated with the on-farm yield. The empirical results from the MET can then be analyzed to identify on-farm situations where the yield-gap can be reduced by choice of genotype (e.g., stable or responsive), agronomic management (e.g., plant density, irrigation strategy), or genotype–management technology combinations.
Improvements in proximal and remote sensor technologies to quantify and upscale measurement of important environmental variables determining GxExM interactions, e.g., evapotranspiration (Figure 2; Guan et al., 2017; He et al., 2019; Kimm et al., 2020), open a wide range of opportunities for applications of enviromic technologies to accelerate crop improvement by integrating breeding and agronomy (Cooper et al., 2020; Peng et al., 2020; Kusmec et al., 2021) and enabling environment-specific predictions (Rogers et al., 2021). For maize breeding in the US corn-belt, early applications of these enviromic technologies have been integrated into the operations of crop improvement programs and are in operational use today (Cooper et al., 2014a,b, 2020; Gaffney et al., 2015; Messina et al., 2018). Such applications of enviromics to analyze GxExM interactions for yield are not restricted to water and drought. Alternative environmental descriptors, such as nitrogen availability (Bänziger et al., 1999; DeBruin et al., 2017; Mueller et al., 2019; Udvardi et al., 2021), can also be applied as appropriate for the crop breeding target, cropping system, and TPE.
Discussion
Given the ubiquity of GxExM interactions for crop grain yield within an agricultural TPE, it is expected that further developments in the domain of enviromics will continue and their applications will expand as plant breeders incorporate these technologies within their breeding operations. With the continuing advances in crop genomics (Morrell et al., 2012; Yuan et al., 2017; Tao et al., 2021; Varshney et al., 2021) and phenotyping (Araus and Cairns, 2014; Araus et al., 2018; Van Eeuwijk et al., 2019; Messina et al., 2021; Smith et al., 2021), a wide array of suitable genomic predictors are available and becoming cost-effective options for many crop breeding applications. Agronomists and physiologists have invested in the development of methods for measuring important environmental variables (Chenu et al., 2011; Guan et al., 2017; Smith et al., 2021) and suitable crop models to integrate the multiple influences of environmental conditions on yield outcomes for different genotypes (Chapman et al., 2003; Messina et al., 2006, 2018, 2019; Chenu et al., 2009; Holzworth et al., 2014; Muller and Martre, 2019; Wang et al., 2019). These same methods can be developed to provide suitable environmental predictors for envirotyping and to enhance genomic prediction (Cooper et al., 2014a,b; Jarquín et al., 2014; Messina et al., 2018; Voss-Fels et al., 2019; Costa-Neto et al., 2021; Resende et al., 2021). An integrated breeding–agronomy approach to accelerate crop improvement is within reach through operationalizing the genomic, enviromic, phenomics, and quantitative modelling processes required to obtain suitable genotypic and environmental predictors for appropriate stages of crop improvement programs. Successful applications have been demonstrated for commercial maize breeding in the US corn-belt (Cooper et al., 2014a; Gaffney et al., 2015). Opportunities are emerging for development of integrated breeding-agronomy approaches for other crops and target regions to tackle current GxExM challenges and the anticipated impacts of climate change (Hatfield and Walthall, 2015; Hammer et al., 2019; Beres et al., 2020; de los Campos et al., 2020; Messina et al., 2020; Ramirez-Villegas et al., 2020; Crossa et al., 2021; Hunt et al., 2021; Kusmec et al., 2021; Smith et al., 2021; Udvardi et al., 2021).
Data Availability Statement
The original contributions presented in the study are included in the article/supplementary material, further inquiries can be directed to the corresponding author.
Author Contributions
MC and CM conceived and wrote the perspective. All authors contributed to the article and approved the submitted version.
Conflict of Interest
The authors declare that the research was conducted in the absence of any commercial or financial relationships that could be construed as a potential conflict of interest.
Funding
The contribution was supported by the University of Queensland and the Queensland Alliance for Agriculture and Food Innovation through funding for the Chair of Prediction-based Crop Improvement held by MC.
Publisher’s Note
All claims expressed in this article are solely those of the authors and do not necessarily represent those of their affiliated organizations, or those of the publisher, the editors and the reviewers. Any product that may be evaluated in this article, or claim that may be made by its manufacturer, is not guaranteed or endorsed by the publisher.
Acknowledgments
The authors thank Tom Tang for assistance with the creation of Figure 2.
Footnotes
References
Allard, R. W., and Bradshaw, A. D. (1964). Implications of genotype-environmental interactions in applied breeding. Crop Sci. 4, 503–508. doi: 10.2135/cropsci1964.0011183X000400050021x
Araus, J. L., and Cairns, J. E. (2014). Field high-throughput phenotyping, the new frontier in crop breeding. Trends Plant Sci. 19, 52–61. doi: 10.1016/j.tplants.2013.09.008
Araus, J. L., Kefauver, S. C., Zaman-Allah, M., Olsen, M. S., and Cairns, J. E. (2018). Translating high-throughput phenotyping into genetic gain. Trends Plant Sci. 23, 451–466. doi: 10.1016/j.tplants.2018.02.001
Archontoulis, S. V., Castellano, M. J., Licht, M. A., Nichols, V., Baum, M., Huber, I., et al. (2020). Predicting crop yields and soil-plant nitrogen dynamics in the US corn belt. Crop Sci. 60, 721–738. doi: 10.1002/csc2.20039
Assefa, Y., Carter, P., Hinds, M., Bhalla, G., Schon, R., Jeschke, M., et al. (2018). Analysis of long term study indicates both agronomic optimal plant density and increase maize yield per plant contributed to yield gain. Sci. Rep. 8:4937. doi: 10.1038/s41598-018-23362-x
Baker, R. J. (1988). “Differential response to environmental stress.” in Proceedings of the 2nd International Conference on Quantitative Genetics. Sinauer Associates, Inc., Sunderland, Massachusetts. eds B. S. Weir, E. J. Eisen, M. M. Goodman, and G. Namkoong. May 30–June 5, 1987, 492–504.
Bänziger, M., Edmeades, G. O., and Lafitte, H. R. (1999). Selection for drought tolerance increases maize yields across a range of nitrogen levels. Crop Sci. 39, 1035–1040. doi: 10.2135/cropsci1999.0011183X003900040012x
Bänziger, M., Setimela, P. S., Hodson, D., and Vivek, B. (2006). Breeding for improved abiotic stress tolerance in maize adapted to southern Africa. Agric. Water Manag. 80, 212–224. doi: 10.1016/j.agwat.2005.07.014
Beres, B. L., Hatfield, J. L., Kirkegaard, J. A., Elgenbrode, S. D., Pan, W. L., Lollato, R. P., et al. (2020). Toward a better understanding of genotype x environment x management interactions: a global wheat initiative agronomic research strategy. Front. Plant Sci. 11:828. doi: 10.3389/fpls.2020.00828
Boer, M. P., Wright, D., Feng, L., Podlich, D. W., Luo, L., Cooper, M., et al. (2007). A mixed-model quantitative trait loci (QTL) analysis for multiple-environment trial data using environmental covariables for QTL-by-environment interactions, with an example in maize. Genetics 177, 1801–1813. doi: 10.1534/genetics.107.071068
Bolaños, J., and Edmeades, G. O. (1996). The importance of anthesis-silking interval in breeding for drought tolerance in tropical maize. Field Crop Res. 48, 65–80. doi: 10.1016/0378-4290(96)00036-6
Boyer, J. S., Byrne, P., Cassman, K. G., Cooper, M., Delmer, D., Greene, T., et al. (2013). The U.S. drought of 2012 in perspective: a call to action. Glob. Food Sec. 2, 139–143. doi: 10.1016/j.gfs.2013.08.002
Bustos-Korts, D., Boer, M. P., Chenu, K., Zheng, B., Chapman, S., and van Eeuwijk, F. (2021). Genotype specific P-spline response surfaces assist interpretation of regional wheat adaptation to climate change. in silico Plants diab018. doi: 10.1093/insilicoplants/diab018
Bustos-Korts, D., Malosetti, M., Chenu, K., Chapman, S., Boer, M. P., Zheng, B., et al. (2019). From QTLs to adaptation landscapes: using genotype-to-phenotype models to characterize G×E over time. Front. Plant Sci. 10:1540. doi: 10.3389/fpls.2019.01540
Campos, H., Cooper, M., Habben, J. E., Edmeades, G. O., and Schussler, J. R. (2004). Improving drought tolerance in maize: a view from industry. Field Crop Res. 90, 19–34. doi: 10.1016/j.fcr.2004.07.003
Chapman, S. C., Chakraborty, S., Dreccer, M. F., and Howden, S. M. (2012). Plant adaptation to climate change – opportunities and priorities in breeding. Crop Pasture Sci. 63, 251–268. doi: 10.1071/CP11303
Chapman, S., Cooper, M., Podlich, D., and Hammer, G. (2003). Evaluating plant breeding strategies by simulating gene action and dryland environment effects. Agron. J. 95, 99–113. doi: 10.2134/agronj2003.0099
Chapman, S. C., Hammer, G. L., Butler, D. G., and Cooper, M. (2000). Genotype by environment interactions affecting grain sorghum. III. Temporal sequences and spatial patterns in the target population of environments. Aust. J. Agric. Res. 51, 223–233. doi: 10.1071/AR99022
Chenu, K., Chapman, S. C., Tardieu, F., McLean, G., Welcker, C., and Hammer, G. L. (2009). Simulating the yield impacts of organ-level quantitative trait loci associated with drought response in maize: a “gene-to-phenotype” modeling approach. Genetics 183, 1507–1523. doi: 10.1534/genetics.109.105429
Chenu, K., Cooper, M., Hammer, G. L., Mathews, K. L., Dreccer, M. F., and Chapman, S. C. (2011). Environment characterization as an aid to wheat improvement: interpreting genotype-environment interactions by modelling water-deficit patterns in north-eastern Australia. J. Exp. Bot. 62, 1743–1755. doi: 10.1093/jxb/erq459
Comstock, R. E., and Moll, R. H. (1963). “Genotype-environment interactions,” in Statistical Genetics and Plant Breeding. eds. W. D. Hanson and H. F. Robinson (Washington, DC, USA: National Academy of Sciences – National Research Council), 164–196.
Cooper, M., and DeLacy, I. H. (1994). Relationships among analytical methods used to study genotypic variation and genotype-by-environment interaction in plant breeding multi-environment experiments. Theor. Appl. Genet. 88, 561–572. doi: 10.1007/BF01240919
Cooper, M., Gho, C., Leafgren, R., Tang, T., and Messina, C. (2014a). Breeding drought-tolerant maize hybrids for the US corn-belt: discovery to product. J. Exp. Bot. 65, 6191–6204. doi: 10.1093/jxb/eru064
Cooper, M., and Hammer, G. L. (eds.) (1996). Plant Adaptation and Crop Improvement. Wallingford, UK: CAB International.
Cooper, M., Messina, C. D., Podlich, D., Totir, L. R., Baumgarten, A., Hausmann, N. J., et al. (2014b). Predicting the future of plant breeding: complementing empirical evaluation with genetic prediction. Crop Pasture Sci. 65, 311–336. doi: 10.1071/cp14007
Cooper, M., Tang, T., Gho, C., Hart, T., Hammer, G., and Messina, C. (2020). Integrating genetic gain and gap analysis to predict improvements in crop productivity. Crop Sci. 60, 582–604. doi: 10.1002/csc2.20109
Cooper, M., Voss-Fels, K. P., Messina, C. D., Tang, T., and Hammer, G. L. (2021). Tackling GxExM interactions to close on-farm yield-gaps: creating novel pathways for crop improvement by predicting contributions of genetics and management to crop productivity. Theor. Appl. Genet. 134, 1625–1644. doi: 10.1007/s00122-021-03812-3
Cooper, M., Woodruff, D. R., Eisemann, R. L., Brennan, P. S., and DeLacy, I. H. (1995). A selection strategy to accommodate genotype-by-environment interaction for grain yield of wheat: managed-environments for selection among genotypes. Theor. Appl. Genet. 90, 492–502.
Costa-Neto, G., Galli, G., Carvalho, H. F., Crossa, J., and Fritsche-Neto, R. (2021). EnvRtype: a software to interplay enviromics and quantitative genomics in agriculture. G3 11:jkab040. doi: 10.1093/g3journal/jkab040
Costa-Neto, G. M. F., Morais Júnior, O. P., Heinemann, A. B., de Castro, A. P., and Duarte, J. B. (2020). A novel GIS-based tool to reveal spatial trends in reaction norm: upland rice case study. Euphytica 216, 1–16. doi: 10.1007/s10681-020-2573-4
Couëdel, A., Edreira, J. I. R., Lollato, R. P., Archontoulis, S., Sadras, V., and Grassini, P. (2021). Assessing environment types for maize, soybean and wheat in the United States as determined by spatio-temporal variation in drought and heat stress. Agric. For. Meteorol. 307:108513. doi: 10.1016/j.agrformet.2021.108513
Crespo-Herrera, L. A., Crossa, J., Huerta-Espino, J., Mondal, S., Velu, G., Juliana, P., et al. (2021). Target population of environments for wheat breeding in India: definition, prediction and genetic gains. Front. Plant Sci. 12:638520. doi: 10.3389/fpls.2021.638520
Crossa, J., Fritsche-Neto, R., Montesinos-Lopez, O. A., Costa-Neto, G., Dreisigacker, S., Montesinos-Lopez, A., et al. (2021). The modern plant breeding triangle: optimizing the use of genomics, phenomics, and enviromics data. Front. Plant Sci. 12:651480. doi: 10.3389/fpls.2021.651480
DeBruin, J. L., Schussler, J. R., Mo, H., and Cooper, M. (2017). Grain yield and nitrogen accumulation in maize hybrids released during 1934 to 2013 in the US Midwest. Crop Sci. 57, 1431–1446. doi: 10.2135/cropsci2016.08.0704
de los Campos, G., Pérez-Rodríguez, P., Bogard, M., Gouache, D., and Crossa, J. (2020). A data-driven simulation platform to predict cultivars’ performances under uncertain weather conditions. Nat. Commun. 11:4876. doi: 10.1038/s41467-020-18480-y
Duvick, D. N. (2005). “The contribution of breeding to yield advances in maize (Zea mays L.),” in Advances in Agronomy. Vol. 86. 83–145.
Duvick, D. N., Smith, J. S. C., and Cooper, M. (2004). “Long-term selection in a commercial hybrid maize breeding program,” in Plant Breeding Reviews. Vol. 24. 109–151.
Eberhart, S. A., and Russell, W. A. (1966). Stability parameters for comparing varieties. Crop Sci. 6, 36–40. doi: 10.2135/cropsci1966.0011183X000600010011x
Finlay, K. W., and Wilkinson, G. N. (1963). The analysis of adaptation in a plant-breeding programme. Aust. J. Agric. Res. 14, 742–754. doi: 10.1071/AR9630742
Fischer, T., Byerlee, D., and Edmeades, G. (2014). Crop Yields and Global Food Security: Will Yield Increase Continue to Feed the World? ACIAR Monograph No. 158. Canberra: Australian Centre for International Agricultural Research.
Fischer, K. S., Edmeades, G. O., and Johnson, E. C. (1989). Selection for the improvement of maize yield under moisture-deficits. Field Crop Res. 22, 227–243.
French, R. J., and Schultz, J. E. (1984). Water use efficiency of wheat in a Mediterranean-type environment 1. The relation between yield, water use and climate. Aust. J. Agric. Res. 35, 743–764. doi: 10.1071/AR9840743
Fukai, S., and Cooper, M. (1995). Development of drought-resistant cultivars using physio-morphological traits in rice. Field Crop Res. 40, 67–86. doi: 10.1016/0378-4290(94)00096-U
Gaffney, J., Schussler, J., Löffler, C., Cai, W., Paszkiewicz, S., Messina, C., et al. (2015). Industry-scale evaluation of maize hybrids selected for increased yield in drought-stress conditions of the US corn belt. Crop Sci. 55, 1608–1618. doi: 10.2135/cropsci2014.09.0654
Gage, J. L., Jarquin, D., Romay, C., Lorenz, A., Buckler, E. S., Kaeppler, S., et al. (2017). The effect of artificial selection on phenotypic plasticity in maize. Nat. Commun. 8:1348. doi: 10.1038/s41467-017-01450-2
Grassini, P., Thorburn, J., Burr, C., and Cassman, K. G. (2011). High-yield irrigated maize in the western U.S. corn-belt: I. on-farm yield, yield potential, and impact of agronomic practices. Field Crop Res. 120, 142–150. doi: 10.1016/j.fcr.2010.09.012
Guan, K., Wu, J., Kimball, J. S., Anderson, M. C., Frolking, S., Li, B., et al. (2017). The shared and unique values of optical, fluorescence, thermal and microwave satellite data for estimating large-scale crop yields. Remote Sens. Environ. 199, 333–349. doi: 10.1016/j.rse.2017.06.043
Hall, A. J., and Richards, R. A. (2013). Prognosis for genetic improvement of yield potential and water-limited yield of major grain crops. Field Crop Res. 143, 18–33. doi: 10.1016/j.fcr.2012.05.014
Hammer, G. L., McLean, G., Chapman, S., Zheng, B., Doherty, A., Harrison, M. T., et al. (2014). Crop design for specific adaptation in variable dryland production environments. Crop Pasture Sci. 65, 614–626. doi: 10.1071/CP14088
Hammer, G. L., McLean, G., van Oosterom, E., Chapman, S., Zheng, B., Wu, A., et al. (2020). Designing crops for adaptation to the drought and high-temperature risks anticipated in future climates. Crop Sci. 60, 605–621. doi: 10.1002/csc2.20110
Hammer, G. L., Messina, C., Wu, A., and Cooper, M. (2019). Biological reality and parsimony in crop models – why we need both in crop improvement. in silico Plants 1:diz010. doi: 10.1093/insilicoplants/diz010
Harrison, M. T., Tardieu, F., Dong, Z., Messina, C. D., and Hammer, G. L. (2014). Characterizing drought stress and trait influence on maize yield under current and future conditions. Glob. Chang. Biol. 20, 867–878. doi: 10.1111/gcb.12381
Hatfield, J. L., and Walthall, C. L. (2015). Meeting global food needs: realizing the potential via genetics x environment x management interactions. Agron. J. 107, 1215–1226. doi: 10.2134/agronj15.0076
He, M., Kimball, J. S., Yi, Y., Running, S. W., Guan, K., Moreno, A., et al. (2019). Satellite data-driven modeling of field scale evapotranspiration in croplands using the MOD16 algorithm framework. Remote Sens. Environ. 230:111201. doi: 10.1016/j.rse.2019.05.020
Heslot, N., Akdemir, D., Sorrells, M. E., and Jannink, J.-L. (2014). Integrating environmental covariates and crop modeling into the genomic selection framework to predict genotype by environment interactions. Theor. Appl. Genet. 127, 463–480. doi: 10.1007/s00122-013-2231-5
Holzworth, D., Huth, N. I., deVoil, P. G., Zurcher, E. J., Herrmann, N. I., McLean, G., et al. (2014). APSIM – evolution towards a new generation of agricultural systems simulation. Environ. Model. Softw. 62, 327–350. doi: 10.1016/j.envsoft.2014.07.009
Hunt, J. R., Kirkegaard, J. A., Harris, F. A., Porker, K. D., Rattey, A. R., Collins, M. J., et al. (2021). Exploiting genotype x management interactions to increase rainfed crop production: a case study from South-Eastern Australia. J. Exp. Bot. 72, 5189–5207. doi: 10.1093/jxb/erab250
Jain, P., Liu, W., Zhu, S., Chang, C. Y.-Y., Melkonian, J., Rockwell, F. E., et al. (2021). A minimally disruptive method for measuring water potential in planta using hydrogel nanoreporters. PNAS 118:e2008276118. doi: 10.1073/pnas.2008276118
Jarquín, D., Crossa, J., Lacaze, X., Du Cheyron, P., Daucourt, J., Lorgeou, J., et al. (2014). A reaction norm model for genomic selection using high-dimensional genomic and environmental data. Theor. Appl. Genet. 127, 595–607. doi: 10.1007/s00122-013-2243-1
Jin, X., Zarco-Tejada, P., Schmidhalter, U., Reynolds, M. P., Hawkesford, M. J., Varshney, R. K., et al. (2021). High-throughput estimation of crop traits: a review of ground and aerial phenotyping platforms. IEEE Geosci. Remote Sens. Mag. 9, 200–231. doi: 10.1109/MGRS.2020.2998816
Kholová, J., McLean, G., Vadez, V., Craufurd, P., and Hammer, G. L. (2013). Drought stress characterization of post-rainy season (rabi) sorghum in India. Field Crop Res. 141, 38–46. doi: 10.1016/j.fcr.2012.10.020
Kimm, H., Guan, K., Gentine, P., Wu, J., Bernacchi, C. J., Sulman, B. N., et al. (2020). Redefining droughts for the U.S. corn belt: the dominant role of atmospheric vapor pressure deficit over soil moisture in regulating stomatal behavior of maize and soybean. Agric. For. Meteorol. 287:107930. doi: 10.1016/j.agrformet.2020.107930
Kirkegaard, J. A., and Hunt, J. R. (2010). Increasing productivity by matching farming system management and genotype in water-limited environments. J. Exp. Bot. 61, 4129–4143. doi: 10.1093/jxb/erq245
Kusmec, A., Zheng, Z., Archontoulis, S., Ganapathysubramanian, B., Hu, G., Wang, L., et al. (2021). Interdisciplinary strategies to enable data-driven plant breeding in a changing climate. One Earth 4, 372–383. doi: 10.1016/j.oneear.2021.02.005
Li, X., Guo, T., Wang, J., Bekele, W. A., Sukumaran, S., Vanous, A. E., et al. (2021). An integrated framework reinstating the environmental dimension for GWAS and genomic selection in crops. Mol. Plant 14, 874–887. doi: 10.1016/j.molp.2021.03.010
Lobell, D. B., Hammer, G. L., Chenu, K., Zeng, B., McLean, G., and Chapman, S. C. (2015). The shifting influence of drought and heat stress for crops in Northeast Australia. Glob. Chang. Biol. 21, 4115–4127. doi: 10.1111/gcb.13022
Löffler, C. M., Wei, J., Fast, T., Gogerty, J., Langton, S., Bergman, M., et al. (2005). Classification of maize environments using crop simulation and geographic information systems. Crop Sci. 45, 1708–1716. doi: 10.2135/cropsci2004.0370
Ludlow, M. M., and Muchow, R. C. (1990). A critical evaluation of traits for improving crop yields in water-limited environments. Adv. Agron. 43, 107–153.
Ly, D., Huet, S., Gauffreteau, A., Rincent, R., Touzy, G., Mini, A., et al. (2018). Whole-genome prediction of reaction norms to environmental stress in bread wheat (Triticum aestivum L.) by genomic random regression. Field Crop Res. 216, 32–41. doi: 10.1016/j.fcr.2017.08.020
Mathews, K. L., Trethowan, R., Milgate, A. W., Payne, T., van Ginkel, M., Crossa, J., et al. (2011). Indirect selection using reference and probe genotype performance in multi-environment trials. Crop Pasture Sci. 62, 313–327. doi: 10.1071/CP10318
Messina, C. D., Cooper, M., Reynolds, M., and Hammer, G. L. (2020). Crop science: a foundation for advancing predictive agriculture. Crop Sci. 60, 544–546. doi: 10.1002/csc2.20116
Messina, C., Hammer, G., Dong, Z., Podlich, D., and Cooper, M. (2009). “Modelling crop improvement in a GxExM framework via gene-trait-phenotype relationships,” in Crop Physiology: Interfacing With Genetic Improvement and Agronomy. eds. V. Sadras and D. Calderini (Netherlands: Elsevier), 235–265.
Messina, C. D., Hammer, G. L., McLean, G., Cooper, M., van Oosterom, E. J., Tardieu, F., et al. (2019). On the dynamic determinants of reproductive failure under drought in maize. in silico Plants 1:diz003. doi: 10.1093/insilicoplants/diz003
Messina, C. D., Jones, J. W., Boote, K. J., and Vallejos, C. E. (2006). A gene-based model to simulate soybean development and yield responses to environment. Crop Sci. 46, 456–466. doi: 10.2135/cropsci2005.04-0372
Messina, C., McDonald, D., Poffenbarger, H., Clark, R., Salinas, A., Fang, Y., et al. (2021). Reproductive resilience but not root architecture underpins yield improvement under drought in maize (Zea mays L.). J. Exp. Bot. doi: 10.1093/jxb/erab231 (in press).
Messina, C. D., Podlich, D., Dong, Z., Samples, M., and Cooper, M. (2011). Yield–trait performance landscapes: from theory to application in breeding maize for drought tolerance. J. Exp. Bot. 62, 855–868. doi: 10.1093/jxb/erq329
Messina, C. D., Sinclair, T. R., Hammer, G. L., Curan, D., Thompson, J., Oler, Z., et al. (2015). Limited-transpiration trait may increase maize drought tolerance in the US corn belt. Agron. J. 107, 1978–1986. doi: 10.2134/agronj15.0016
Messina, C. D., Technow, F., Tang, T., Totir, R., Gho, C., and Cooper, M. (2018). Leveraging biological insight and environmental variation to improve phenotypic prediction: integrating crop growth models (CGM) with whole genome prediction (WGP). Eur. J. Agron. 100, 151–162. doi: 10.1016/j.eja.2018.01.007
Millet, E. J., Kruijer, W., Couple-Ledru, A., Prado, S. A., Cabrera-Bosquet, L., Lacube, S., et al. (2019). Genomic prediction of maize yield across European environmental conditions. Nat. Genet. 51, 952–956. doi: 10.1038/s41588-019-0414-y
Morrell, P. L., Buckler, E. S., and Ross-Ibarra, J. (2012). Crop genomics: advances and applications. Nat. Rev. Genet. 13, 85–96. doi: 10.1038/nrg3097
Muchow, R. C., Cooper, M., and Hammer, G. L. (1996). “Characterizing environmental challenges using models,” in Plant Adaptation and Crop Improvement. eds. M. Cooper and G. L. Hammer (Wallingford, UK: CAB International), 349–364.
Mueller, S. M., Messina, C. D., and Vyn, T. J. (2019). Simultaneous gains in grain yield and nitrogen efficiency over 70 years of maize genetic improvement. Sci. Rep. 9:9095. doi: 10.1038/s41598-019-45485-5
Muller, B., and Martre, P. (2019). Plant and crop simulation models: powerful tools to link physiology, genetics, and phenomics. J. Exp. Bot. 70, 2339–2344. doi: 10.1093/jxb/erz175
Nyquist, W. E., and Baker, R. J. (1991). Estimation of heritability and prediction of selection response in plant populations. Crit. Rev. Plant Sci. 10, 235–322. doi: 10.1080/07352689109382313
Passioura, J. B. (2002). Environmental biology and crop improvement. Funct. Plant Biol. 29, 537–546. doi: 10.1071/FP02020
Passioura, J. (2006). Increasing crop productivity when water is scarce – from breeding to field management. Agric. Water Manag. 80, 176–196. doi: 10.1016/j.agwat.2005.07.012
Passioura, J. (2007). The drought environment: physical, biological and agricultural perspectives. J. Exp. Bot. 58, 113–117. doi: 10.1093/jxb/erl212
Pauli, D., Chapman, S. C., Bart, R., Topp, C. N., Lawrence-Dill, C. J., Poland, J., et al. (2016). The quest for understanding phenotypic variation via integrated approaches in the field environment. Plant Physiol. 172, 622–634. doi: 10.1104/pp.16.00592
Peng, B., Guan, K., Tang, J., Ainsworth, E. A., Asseng, S., Bernacchi, C. J., et al. (2020). Towards a multiscale crop modelling framework for climate change adaptation assessment. Nat. Plants 6, 338–348. doi: 10.1038/s41477-020-0625-3
Porker, K., Coventry, S., Fettell, N. A., Cozzolino, D., and Eglinton, J. (2020). Using a novel PLS approach for envirotyping of barley phenology and adaptation. Field Crop Res. 246:107697. doi: 10.1016/j.fcr.2019.107697
Potgieter, A. B., Zhao, Y., Zarco-Tejada, P. J., Chenu, K., Zhang, Y., Porker, K., et al. (2021). Evolution and application of digital technologies to predict crop type and crop phenology in agriculture. in silico Plants 3:diab017. doi: 10.1093/insilicoplants/diab017
Ramirez-Villegas, J., Milan, A. M., Alexandrov, N., Asseng, S., Challinor, A. J., Crossa, J., et al. (2020). CGIAR modelling approaches for resource-constrained scenarios: I. accelerating crop breeding for a changing climate. Crop Sci. 60, 547–567. doi: 10.1002/csc2.20048
Rebetzke, G. J., Chenu, K., Biddulph, B., Moeller, C., Deery, D. M., Rattey, A. R., et al. (2013). A multisite managed environment facility for targeted trait and germplasm phenotyping. Funct. Plant Biol. 40, 1–13. doi: 10.1071/FP12180
Resende, R. T., Piepho, H. P., Rosa, G. J. M., Silva-Junior, O. B., Silva, F. F., de Resende, M. D. V., et al. (2021). Enviromics in breeding: applications and perspectives on envirotypic-assisted selection. Theor. Appl. Genet. 134, 95–112. doi: 10.1007/s00122-020-03684-z
Reynolds, M., Chapman, S., Crespo-Herrera, L., Molero, G., Mondal, S., Pequeno, D. N. L., et al. (2020). Breeder friendly phenotyping. Plant Sci. 295:110396. doi: 10.1016/j.plantsci.2019.110396
Ribaut, J.-M. (ed.) (2006). Drought Adaptation in Cereals. New York: Food Products Press, The Haworth Press, Inc.
Richards, R. A., and Passioura, J. B. (1989). A breeding program to reduce the diameter of the major xylem vessel in the seminal roots of wheat and its effect on grain yield in rain-fed environments. Aust. J. Agric. Res. 40, 943–950. doi: 10.1071/AR9890943
Rogers, A. R., Dunne, J. C., Romay, C., Bohn, M., Buckler, E. S., Ciampitti, I. A., et al. (2021). The importance of dominance and genotype-by-environment interactions on grain yield variation in a large-scale public cooperative maize experiment. G3 11:jkaa050. doi: 10.1093/g3journal/jkaa050
Sadras, V. O., and Angus, J. F. (2006). Benchmarking water-use efficiency of rainfed wheat in dry environments. Aust. J. Agric. Res. 57, 847–856. doi: 10.1071/AR05359
Sadras, V. O., Cassman, K. G., Grassini, P., Hall, A. J., Bastiaanssen, W. G. M., Laborte, A. G., et al. (2015). FAO Water Reports No. 41. Yield Gap Analysis of Field Crops – Methods and Case Studies. Rome, Italy.
Schwalbert, R., Amado, T., Nieto, L., Corassa, G., Rice, C., Peralta, N., et al. (2020). Mid-season county-level corn yield forecast for US corn belt integrating satellite imagery and weather variables. Crop Sci. 60, 739–750. doi: 10.1002/csc2.20053
Simmons, C. R., Lafitte, H. R., Reimann, K. S., Brugière, N., Roesler, K., Albertsen, M. C., et al. (2021). Success and insights of an industry biotech program to enhance maize agronomic traits. Plant Sci. 307:110899. doi: 10.1016/j.plantsci.2021.110899
Sinclair, T. R. (2018). Effective water use required for improving crop growth rather than transpiration efficiency. Front. Plant Sci. 9:1442. doi: 10.3389/fpls.2018.01442
Smith, D. T., Potgieter, A. B., and Chapman, S. C. (2021). Scaling up high-throughput phenotyping for abiotic stress selection in the field. Theor. Appl. Genet. 134, 1845–1866. doi: 10.1007/s00122-021-03864-5
Tao, Y., Luo, H., Xu, J., Cruickshank, A., Zhao, X., Teng, F., et al. (2021). Extensive variation within the pan-genome of cultivated and wild sorghum. Nat. Plants 7, 766–773. doi: 10.1038/s41477-021-00925-x
Udvardi, M., Below, F. E., Castellano, M. J., Eagle, A. J., Giller, K. E., Ladha, J. K., et al. (2021). A research road map for responsible use of agricultural nitrogen. Front. Sustain. Food Syst. 5:660155. doi: 10.3389/fsufs.2021.660155
Van Bussel, L. G. J., Grassini, P., van Wart, J., Wolf, J., Claessens, L., Yang, H., et al. (2015). From field to atlas: upscaling of location-specific yield gap estimates. Field Crop Res. 177, 98–108. doi: 10.1016/j.fcr.2015.03.005
Van Eeuwijk, F. A., Bustos-Korts, D. V., and Malosetti, M. (2016). What should students in plant breeding know about the statistical aspects of genotype x environment interactions? Crop Sci. 56, 2119–2140. doi: 10.2135/cropsci2015.06.0375
Van Eeuwijk, F., Bustos-Korts, D., Millet, E. J., Boer, M., Kruijer, W., Thompson, A., et al. (2019). Modelling strategies for assessing and increasing the effectiveness of new phenotyping techniques in plant breeding. Plant Sci. 282, 23–39. doi: 10.1016/j.plantsci.2018.06.018
Van Eeuwijk, F. A., Cooper, M., DeLacy, I. H., Ceccarelli, S., and Grando, S. (2001). Some vocabulary and grammar for the analysis of multi-environment trials, as applied to the analysis of FPB and PPB trials. Euphytica 122, 477–490. doi: 10.1023/A:1017591407285
Van Ittersum, M. K., Cassman, K. G., Grassini, P., Wolf, J., Tittonell, P., and Hochman, Z. (2013). Yield gap analysis with local to global relevance: a review. Field Crop Res. 143, 4–17. doi: 10.1016/j.fcr.2012.09.009
Varshney, R. K., Bohra, A., Yu, J., Graner, A., Zhang, Q., and Sorrells, M. E. (2021). Designing future crops: genomics-assisted breeding comes of age. Trends Plant Sci. 26, 631–649. doi: 10.1016/j.tplants.2021.03.010
Voss-Fels, K. P., Cooper, M., and Hayes, B. J. (2019). Accelerating crop genetic gains with genomic selection. Theor. Appl. Genet. 132, 669–686. doi: 10.1007/s00122-018-3270-8
Wang, E., Brown, H. E., Rebetzke, G. J., Zhao, Z., Zheng, B., and Chapman, S. C. (2019). Improving process-based crop models to better capture genotype x environment x management interactions. J. Exp. Bot. 70, 2389–2401. doi: 10.1093/jxb/erz092
Weber, V. S., Melchinger, A. E., Magorokosho, C., Makumbi, D., Bänzinger, M., and Atlin, G. N. (2012). Efficiency of managed-stress screening of elite maize hybrids under drought and low nitrogen for yield under rainfed conditions in southern Africa. Crop Sci. 52, 1011–1020. doi: 10.2135/cropsci2011.09.0486
Xu, Y. (2016). Envirotyping for deciphering environmental impacts on crop plants. Theor. Appl. Genet. 129, 653–673. doi: 10.1007/s00122-016-2691-5
Yang, Y., Guan, K., Peng, B., Pan, M., Jiang, C., and Franz, T. E. (2021). High-resolution spatially explicit land surface model calibration using field-scale satellite-based daily evapotranspiration product. J. Hydrol. 596:125730. doi: 10.1016/j.jhydrol.2020.125730
Keywords: environmental characterisation, envirotyping, yield prediction, drought, crop modelling, crossover genotype by environment interactions, target population of environments, multi-environment trial
Citation: Cooper M and Messina CD (2021) Can We Harness “Enviromics” to Accelerate Crop Improvement by Integrating Breeding and Agronomy? Front. Plant Sci. 12:735143. doi: 10.3389/fpls.2021.735143
Edited by:
Roberto Fritsche-Neto, International Rice Research Institute (IRRI), PhilippinesReviewed by:
Germano Costa-Neto, Cornell University, United StatesGota Morota, Virginia Tech, United States
Copyright © 2021 Cooper and Messina. This is an open-access article distributed under the terms of the Creative Commons Attribution License (CC BY). The use, distribution or reproduction in other forums is permitted, provided the original author(s) and the copyright owner(s) are credited and that the original publication in this journal is cited, in accordance with accepted academic practice. No use, distribution or reproduction is permitted which does not comply with these terms.
*Correspondence: Mark Cooper, bWFyay5jb29wZXJAdXEuZWR1LmF1