- 1LEAF – Linking Landscape, Environment, Agriculture and Food – Research Center, Associated Laboratory TERRA, Instituto Superior de Agronomia, Universidade de Lisboa, Lisboa, Portugal
- 2BioISI – Biosystems and Integrative Sciences Institute, Faculty of Sciences, Universidade de Lisboa, Lisboa, Portugal
Plant phenotyping is an emerging science that combines multiple methodologies and protocols to measure plant traits (e.g., growth, morphology, architecture, function, and composition) at multiple scales of organization. Manual phenotyping remains as a major bottleneck to the advance of plant and crop breeding. Such constraint fostered the development of high throughput plant phenotyping (HTPP), which is largely based on imaging approaches and automatized data retrieval and processing. Field phenotyping still poses major challenges and the progress of HTPP for field conditions can be relevant to support selection and breeding of grapevine. The aim of this review is to discuss potential and current methods to improve field phenotyping of grapevine to support characterization of inter- and intravarietal diversity. Vitis vinifera has a large genetic diversity that needs characterization, and the availability of methods to support selection of plant material (polyclonal or clonal) able to withstand abiotic stress is paramount. Besides being time consuming, complex and expensive, field experiments are also affected by heterogeneous and uncontrolled climate and soil conditions, mostly due to the large areas of the trials and to the high number of traits to be observed in a number of individuals ranging from hundreds to thousands. Therefore, adequate field experimental design and data gathering methodologies are crucial to obtain reliable data. Some of the major challenges posed to grapevine selection programs for tolerance to water and heat stress are described herein. Useful traits for selection and related field phenotyping methodologies are described and their adequacy for large scale screening is discussed.
Introduction
The EU is the leading global wine producer, with about 44% of the world’s vine-growing area (circa 3.2 million ha) and sustaining about 57% of wine production by volume (OIV, 2020). European Mediterranean countries lead the cultivated area of grapevine for wine production worldwide (OIV, 2020) but they are also increasingly exposed to more adverse weather conditions, with air temperatures rising from 2 to 5°C in major winemaking regions in parallel with changes in precipitation patterns or/and higher frequency of extreme weather events, such as heat waves (IPCC, 2014; Fraga, 2020; Lorenzo et al., 2021).
These changes have a serious impact on the sustainability of the wine sector in Mediterranean countries (e.g., Spain, France, Italy, Greece, and Portugal). Several agronomic strategies are already being implemented in viticulture to face climate challenges, and adapt to more severe heat and drought. The use of deficit irrigation is one of the most common (see Santesteban et al., 2019 for a review), but several others have been proposed and reviewed (see Gutiérrez-Gamboa et al., 2021 or Naulleau et al., 2021), and their economic consequences for the producers were analyzed (Merloni et al., 2018).
The use of better adapted plant material is another priority, namely in terms of late ripening varieties (Wolkovich et al., 2018), heat/drought tolerant clones (Van Leeuwen et al., 2013; Bota et al., 2016), and rootstocks adapted or modified to forthcoming climate conditions (Ollat et al., 2016; Prinsi et al., 2021). However, field phenotyping and grapevine selection are laborious and expensive, and still pose major challenges. The progress of high throughput plant phenotyping (HTPP) for field conditions can be relevant to support selection and breeding of grapevine. Therefore, the aim of this review is to identify potential strategies and methods to improve field phenotyping of grapevine to support characterization of inter- and intravarietal diversity. In fact, Vitis vinifera has a large genetic diversity that needs characterization to support selection of better adapted plant material (polyclonal or clonal), namely to abiotic stress.
The Impact of Heat and Water Stress on Grapevine Physiology
Stomatal behavior is a crucial functional trait and stomatal responses to the environment are determinant for plant adaptation. Stomata influence CO2 uptake into the leaf along with water loss due to transpiration, actively regulating plant water status and leaf temperature (Jones, 1992; Matthews and Lawson, 2019). Stomata respond to chemical stimuli (biochemical control due to hormonal control) and to leaf water status (hydraulic control; Pantin et al., 2013) that mediate environmental inputs, such as light intensity and quality, air CO2 concentration, and vapor pressure deficit (VPD; Buckley, 2019). Increasing soil water use is associated with hydraulic traits, to enable gas exchange under more negative water potentials, as observed by Dayer et al. (2020) in Semillon. Stomatal conductance to water vapor (gs) on Chardonnay did not respond to air temperature below 30°C, but dropped under a combination of high air temperature and high air VPD (Greer, 2020). Different stomatal behaviors have been described for other varieties, thus the interaction between air temperature and VPD must be considered when addressing stomatal responses (Dayer et al., 2020; Greer, 2020).
Some varieties show a tight stomatal control (isohydric), whereas others show a less efficient stomatal control in response to water stress (anisohydric). Nevertheless, such classification of Vitis varieties as isohydric or anisohydric remains controversial since differences in stomatal behavior among varieties are far more complex and largely depend on growing conditions (Chaves et al., 2010; Lovisolo et al., 2010; Villalobos-Gonzalez et al., 2019; Gambetta et al., 2021). In fact, it was shown that a variety can behave as both iso- and anisohydric, according to the level of water deficit, which defies the standard classification that implies a single behavior (Levin et al., 2019). Gambetta et al. (2020) suggested a more integrative definition of drought tolerance in grapevine, by resorting to four core physiological traits: maximum transpiration rate; stomatal regulation (expressed as the relation between stomatal conductance and leaf water potential); turgor loss point; and root volume. Bringing these parameters together, the authors suggested that it is possible to calculate, at any moment, for a vineyard under defined environmental conditions, the “stress distance,” i.e., the amount of time (e.g., number of days) that it withstands without watering before reaching a critical water potential.
The plasticity of leaf morphology is another factor of adaptation and evolution (Fritz et al., 2018). The role of leaf epidermis characteristics (cuticle, indumentum, pavement cells, and stomata) and mesophyll anatomy can have an impact on responses to abiotic stresses (Tomás et al., 2014; MacMIllan et al., 2021). Leaf morphology and structure may affect stomatal behavior, leaf gas exchange, and mesophyll conductance (Tomás et al., 2014). Stomatal density and stomatal index can influence varietal leaf gas exchange characteristics as well as thermal regulation capacity (Gago et al., 2019). Costa et al. (2012) found no differences in stomatal density between Cabernet Sauvignon, Touriga Nacional, Syrah, Trincadeira, and Aragonez (syn. Tempranillo), but reported differences in gs, leaf temperature, and leaf photosynthesis, suggesting that other factors besides the number of stomata regulate leaf gas exchange in grapevine. Gago et al. (2019) reported that Grenache Noir had significantly smaller leaf surface area than Syrah, but significantly thicker leaf blades. This calls for improved knowledge on morphological, anatomical, and physiological traits influencing the response to heat and drought of the Vitis germplasm.
The role of abscisic acid (ABA) in stomatal closure is well established; this hormone plays a key role particularly in isohydric or near-isohydric plants (Sampaio Filho et al., 2018; Dayer et al., 2020), by inducing faster ABA-related gene modulation (dal Santo et al., 2016). Stomatal sensitivity to ABA is variable among varieties (Rossdeutsch et al., 2016; Simonneau et al., 2017). Rossdeutsch et al. (2016) concluded that Vitis sp. genotypes with contrasting levels of drought adaptation differ in key steps involved in ABA metabolism and signaling, both when well-watered and drought stressed.
Grapevine’s photosynthetic apparatus is defined as resilient, but extreme climate conditions will affect it negatively, through the overreduction of the photosynthetic electron carriers, production of reactive oxygen species (ROS), and photoinhibition (Mittler, 2006). In Mediterranean summer conditions, grapevine plants growing under heat and drought are usually exposed simultaneously to photoinhibitory light conditions, high air temperatures, and moderate to severe soil water deficits (Carvalho and Amâncio, 2019). If stress persists and carbon fixation is reduced, oxidative stress may take place (Carvalho and Amâncio, 2019). When drought co-occurs with high light intensities an increase in ROS production by the photosynthetic apparatus can also arise (Mullineaux et al., 2006), leading to photoinhibition of photosynthesis.
Heat stress physiology in turn, at both leaf and berry levels, should be evaluated to better understand the impacts of drought and high soil and air temperatures on grapevine physiology and morphology of leaves, berries, and bunches (Costa et al., 2019a; Field et al., 2020). This is particularly important because berries tend to ripe earlier in warmer conditions, due to the effect of heat in anticipating phenological events (Van Leeuwen and Destrac-Irvine, 2017).
Plant phenology and growth are largely driven by air temperature and soil water availability (Parker et al., 2011). In fact, Verdugo-Vásquez et al. (2020) developed a climate-based model to estimate grapevine phenology, taking into account meteorological data and microclimate data at the plant level. Concomitantly, berry composition is affected by water availability and heat, with extreme temperatures and severe drought affecting negatively vigor, yield, and berry composition (Chaves et al., 2010), such as a lower content of anthocyanins (van Leeuwen and Darriet, 2016; Zarrouk et al., 2016). In addition, acidity, in particular related to malic acid content, decreases in high air temperature (van Leeuwen and Darriet, 2016). Consequently, the modern wine industry must find adequate varieties to maintain berry quality traits, such as acidity, under extreme and adverse climate conditions. Aspects such as berry sensitiveness to drought and sunburn were recently revised by Gambetta et al. (2021), attesting the relevance of the problem for the academy and the industry.
The Role of Plant Material to Mitigate Stress and Decrease Risks of Combined Heat Waves and Drought
Using optimal adapted plant material (rootstocks and V. vinifera varieties) for a specific region is a long term adaptation strategy crucial for grower’s revenue and sustainability of the sector (less water, pesticides, and fertilizers required; Figure 1). Grapevine has a high level of phenotypic plasticity and genotypes can respond by adapting their growth morphology, leaf gas exchange, and berries’ metabolic characteristics. Such plasticity was recently reported in a three season study of 30 varieties, indicating possible adaptations to climate change, such as the earlier and shorter ripening phase of white varieties to avoid the warmest period of the season (Gashu et al., 2020).
Autochthonous grapevine varieties represent a strong natural and historical mark, add great value to top quality wines, and are an essential raw-material to face future challenges. Therefore, a better characterization of existing variability between and within varieties is necessary, especially if we consider the need to adapt to scenarios of climate change. Usually, varieties original from the Mediterranean basin are perceived as drought tolerant, such as the widely-used Grenache, Cinsault, Carignan, Cabernet Sauvignon, Sangiovese, Zinfandel, and Nebbiolo (Fraga et al., 2012; Van Leeuwen et al., 2019), and the less extensively spread Xinistery from Cyprus (Van Leeuwen et al., 2019). Some Portuguese varieties have also been described as well adapted to abiotic stress, such as Cerceal-Branco, Encruzado, Touriga Franca, and Viosinho (Carvalho et al., 2017). Furthermore, the existence of intravarietal variability in grapevine is the available resource for polyclonal selection (Resolution OIV-VITI 564B-2019; OIV, 2019) and clonal selection (OIV-VITI 564A-2017; OIV, 2017) aiming at climate change adaption.
Despite having a small land area, Portugal is extremely rich in autochthonous varieties. As a result, a coherent strategy has been developed to stop the ongoing erosion of intravarietal genetic diversity of all autochthonous varieties, to improve methods of conservation, to evaluate the intravarietal diversity for selection focused on yield, important must quality traits, and tolerance to abiotic and biotic stresses (Martins and Gonçalves, 2015; Gonçalves et al., 2016; Carvalho et al., 2020). This strategy has been implemented in the field by the National Network for Grapevine selection and by the Portuguese Association for Grapevine Diversity (PORVID).
The resources available in Portugal to perform field phenotyping to select superior clones within Portuguese grapevine varieties comprise a network of more than 185 field trials of 63 varieties, distributed along the country, and established according to efficient experimental designs to carry out selection. Rootstocks influence resistance to abiotic stress, namely to drought (Pavlousek, 2011; Harbertson and Keller, 2012). The combination of tolerant rootstocks with tolerant clones could be the most effective long term strategy to overcome adverse climate limitations that currently affect Portugal and other Southern European countries (Santos et al., 2020). The graft-scion incompatibility remains a major issue as it can limit response to heat and drought (Tedesco et al., 2020).
Fast, robust, and accurate screening of specific traits to assess tolerance to abiotic stress of rootstocks and V. vinifera varieties is crucial to obtain plant material able to cope with climate change. Phenotyping technologies (for controlled and field conditions) have undergone great progress in the last decade. The latest innovations and respective application to different crops have been intensively described (Araus et al., 2018; Qiu et al., 2018; Das Choudhury et al., 2019; Pieruschka and Schurr, 2019; Roitsch et al., 2019; Jiang and Li, 2020; Li et al., 2020; Moreira et al., 2020; Yang et al., 2020; Jin et al., 2021). A multiple set of methods and technologies are now available to support the evaluation of quantitative traits, including crop yield and tolerance to abiotic stresses. In this review, the available phenotyping methodologies will be analyzed in light of their potential use to evaluate inter- and intravarietal variability and to support selection of grapevine genotypes for tolerance to abiotic stress.
Phenotyping in Grapevine
Definitions, Scales, and Approaches
Phenotyping is the process of systematically determining, analyzing, and predicting all or part of an organism’s phenotype, and the concept was used for the first time in the 1950s. However, it was only in 2013 that Fiorani and Schurr (2013) coined the term “plant phenotyping,” defining it as “the set of methodologies and protocols used to accurately measure plant growth, architecture, and composition at different scales.” Phenotyping aims at providing valuable data to improve management of biodiversity resources, to foster crop/variety adaptability to the environment and resistance against pests and diseases (Costa et al., 2019b,c) as well as to identify superior traits such as yield and quality. Phenotype, as the result of the genotype (G), the environment (E), and the interaction between them (G×E) is dynamic, complex and comprises multiple quantitative traits that make it hard to study, and especially, to quantify.
Phenotyping methodologies and procedures to characterize and select individuals with particular traits and clear advantages at the level of stress resistance, yield performance, and fruit quality traits, require a systematic approach and organized data collection to facilitate further analysis. Plant phenotyping can be carried out at different levels of biological organization with similar aims but yielding different outputs. Molecular phenotyping involves transcriptomics, proteomics, metabolomics, and related areas such as lipidomics, and can be targeted to single-cell phenotyping, in which the effects of a mutation can be studied through changes in a single cell (Schiefelbein, 2015). On the other end, there are field and ecosystem level phenotyping.
Molecular phenotyping focuses on the investigation of gene function and/or biochemical pathways underpinning physiological mechanisms affecting development, productivity, and stress responses. In grapevine, it aims at developing biotechnology programs to scan for tolerance (Ciaffi et al., 2019) or to develop improved varieties that enable the production of specific wines (DeBolt et al., 2006; Harris et al., 2013) or that are tolerant to biotic stresses (Agüero et al., 2005). At this level, phenotyping approaches are often destructive and require extensive sample manipulation and processing. Therefore, the concept of HTPP refers mainly to whole-plant phenotyping and is largely based in automated image capture and analysis [e.g., Red, Green, Blue (RGB), thermal, multispectral, and fluorescence imaging].
Modern HTPP platforms are coupled to controlled environment growth facilities, allowing large scale screening, isolation of the genetic component of the phenotype, and selection of the most promising genotypes. Large scale plant phenotyping has been extensively studied and developed under controlled conditions, especially for screening of model plants such as Arabidopsis (Merlot et al., 2002), but also for cereals (Raskin and Ladyman, 1988), canola (Knoch et al., 2019), or pepper (Toledo-Martín et al., 2016). In the last few years, good progress was made in the use of remote and proximal sensing tools to meet the phenotyping needs of annual crops, namely by using automated multi-sensor phenotyping machines.1 Such platforms are sophisticated and costly. Therefore, low-cost or more cost-effective phenotyping options are being developed (Reynolds et al., 2019), among them, user-built cost-controlled prototypes (e.g., in the project INTERPHENO2).
However, as crops are subjected to multiple stresses, changing in duration and intensity along time, selected genotypes must be tested under conditions that are more realistic, namely in field conditions. Field phenotyping is complex, since environmental conditions cannot be controlled and it is difficult to homogenize sampling conditions. Also, field phenotyping infrastructures are not easily available due to their high costs. Some initiatives have been implemented to use field phenotyping technologies based on ground and aerial platforms. For example, the European project EMPHASIS, which is on its implementation phase, aims to create a permanent European HTPP infrastructure network, has a work package fully dedicated to field phenotyping.3
Under the plant-breeder’s perspective, an efficient phenotyping must take into account two standpoints: (1) the availability of adequate tools to measure the target traits and (2) the planning of phenotyping. Concerning the first aspect, plant breeding needs simple, fast and HTPP methods well adapted to the main agronomic, physiological, and technological traits. The second aspect is related to the actions before and after phenotyping, that is, the rules that must be followed to ensure that the obtained data can be suitable for an efficient use of the acquired measurements, namely, for selection purposes and comparative experiments. This is particularly relevant to feed biodata infrastructures (ex. EU ELIXIR project or BioData.pt. which is the Portuguese distributed infrastructure for biological data).
Current Technologies and Strategies to Screen Grapevine Germplasm
The need to identify grapevine varieties/genotypes with specific characteristics that enable them to deal with challenges posed by climate change is universally recognized. Nevertheless, we are still far from having reliable, fast, and efficient methodologies for grapevine phenotyping at reduced cost, especially in field conditions. The use of phenotyping devices in woody perennial crops with complex canopies and architecture, such as grapevine still poses difficulties. However, the principle of using indirect non-contact measurements to quantify physiological traits is suitable for grapevine field phenotyping. This approach is getting more attention in parallel with the increasing availability of proximal and remote sensing technologies, especially for “stress-tolerance” based on imaging (RGB, thermal, chlorophyll fluorescence, and hyperspectral). There is also an increasing number of available tools for image processing and analysis, and of algorithms that can support a phenotyping decision (Tsaftaris et al., 2016; Barradas et al., 2021), some developed specifically for grapevine (de Castro et al., 2018; di Gennaro et al., 2019). The potential applications of different imaging approaches for selection and stress monitoring are briefly described below and summarized in Table 1.
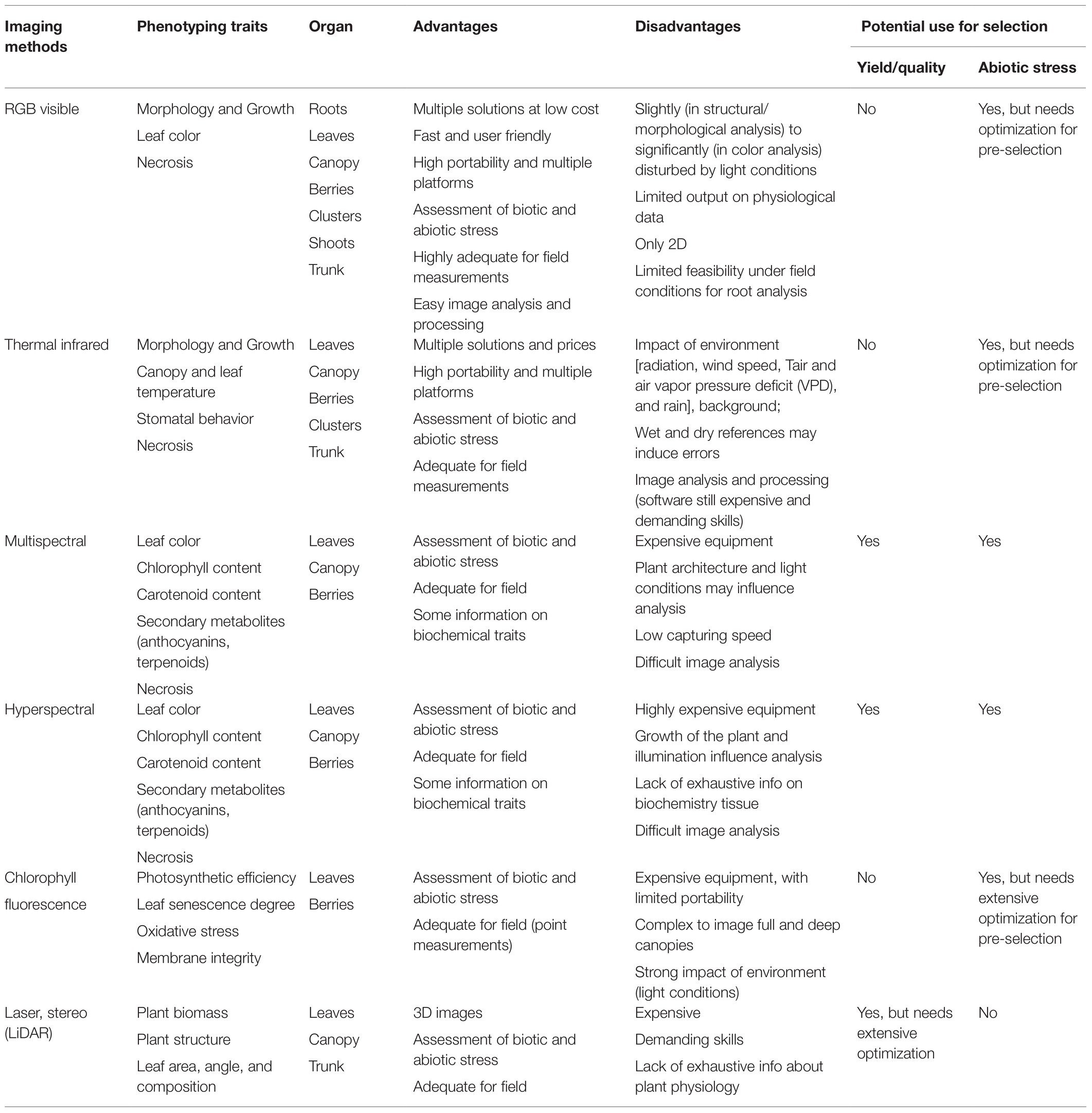
Table 1. List of imaging methodologies, their advantages, disadvantages, and potential application to screen grapevine plants in field conditions (genotype selection for yield, berry quality, and for abiotic stress).
Visible RGB Imaging
Shoot growth, leaf area, and yield are important agronomical and morphological parameters used for different crops. Traditionally they are quantified by weighing or manually measuring shoot elongation and leaf area. Despite the ease of these measurements, they are very time-consuming and inadequate for large scale phenotyping. RGB imaging, currently widespread in consumer-grade digital cameras and mobile phones, but also available in a diversity of industrial devices tailored for artificial vison (Pajares et al., 2016), is an efficient method to assess leaf area and yield in grapevine (Mabrouk and Sinoquet, 1998; Nuske et al., 2011; Diago et al., 2012; Arnó et al., 2013; Dogan et al., 2018).
Pipeline image analysis performed after automatic selection of representative pixels for each category, such as “soil,” “leaves,” “wood,” or “grapes,” showed high correlation, for leaf area and fruit yield, with the values obtained by destructive methods (Diago et al., 2012). RGB images also proved to be a feasible tool to estimate yield. Berry detection was based not only on the color but also on berries geometry, specifically the radial symmetry, to distinguish them from the background even when green (Nuske et al., 2011; Abdelghafour et al., 2019; Briglia et al., 2019). More recently and using a robot as movable ground platform, Victorino et al. (2020) collected image-based indicators to support yield prediction at different phenological stages in grapevine. The authors reported that bunch volume and bunch projected area had significantly high correlation coefficients with yield, regardless of the fruit’s occlusion.
Red, Green, Blue images have also been used to estimate the whole plant leaf area (LA) and fresh biomass in grapevine (Coupel-Ledru et al., 2014), both relevant traits to assess plant vigor. Regarding fruits, cluster compactness is an important trait to select table and wine grapes and the assessing methodology is based on the OIV descriptors, using morphological features of the clusters, and can also be estimated using image analysis, a faster and non-destructive alternative of characterization (Palacios et al., 2019). These authors developed a mobile sensing platform that automatically captures RGB images of grapevine canopies and fruiting zones at night using artificial illumination.
The distinction between the individual plants in the foreground and the vineyard in the background poses a major challenge for sensor-based phenotyping, particularly when RGB images are used, since similar color distributions occur in both. To overcome this difficulty, Klodt et al. (2015) developed a method of background subtraction based on taking two images of each plant for depth reconstruction, which were then successfully used to evaluate 3D leaf surface areas and the ratio fruit-to-leaf in new grapevine breeding lines.
Faster image data retrieval will be crucial to gain efficiency in field phenotyping. Imaging acquisition using more or less complex ground-based platforms (robots, tractors, and quads) must still be optimized for the vineyard. This poses major challenges namely related to irregular and rocky soils, different plant spacing, and orientation. Due to the frequency of image acquisition and the storage capacity, driving speed for data acquisition in field conditions has been limited to 0.5–1km/h (Zheng et al., 2021), even though other authors refer the possibility of reaching 5km/h for on-the-go imaging (Kicherer et al., 2015; Gutiérrez et al., 2017).
Infrared Thermography
Infrared thermography also shows potential for phenotyping in both controlled and field conditions, namely to assess drought stress (Jones et al., 2002; Costa et al., 2013; Diago et al., 2016). Stomatal conductance to water vapor correlates with the plant’s water status and regulates evaporative cooling, making plant temperature increase when stomata are closed. According to these principles there have been attempts to use thermography instead of the time-consuming leaf gas exchange measurements to assess plant water status and transpiration (Jones et al., 2002; Möller et al., 2007; Briglia et al., 2019). However, factors of environmental variability, such as wind speed, radiation, and air humidity could affect the robustness of thermal imaging data as compared to the actual plant status (Costa et al., 2013). The use of phenotyping vehicles following the concept of “mobile tunnel,” equipped with artificial broadband light sources, as is the case of the Phenoliner (Kicherer et al., 2017), may minimize those environmental disturbances. Another strategy to minimize environmental disturbances is the use of so-called thermal indexes. One of the most commonly applied, is the crop water stress index (CWSI; Clawson et al., 1989), based on the use of wet and dry reference surface temperatures. A high and stable correlation between CWSI and leaf conductance (gL) is found when CWSI is calculated using the temperature at the center of the canopy or its sunlight fraction (Clawson et al., 1989). A high positive correlation between gL and stem water potential (ψstem) during the season was also found (Irmak et al., 2000). Grapevine water status can be estimated through CWSI by using thermal imaging system and a RGB digital camera (Möller et al., 2007) in which the color image is used to select pixels with specific features, such as sunlit pixels, to create masks of soil and masks of shadowed leaves to enable the analysis of the temperature in the thermal images only in sunlit leaves. In turn, Matese et al. (2018) found a high correlation between CWSI obtained from proximal and remote thermal sensing and the physiological parameters net photosynthesis (Pn) and effective quantum yield of photosystem II (Fv′/Fm′) in the varieties Vermentino, Cabernet Sauvignon, and Cagnulari. Other reports emphasize the fact that canopy size and architecture, together with leaf orientation can result in different temperature readings for identical values of stomatal conductance (Grant et al., 2007; Grant et al., 2016). Furthermore, the use of wet and dry references, required to compute CWSI or other thermal indexes (e.g., stomatal conductance index – IG), may conflict with HTPP in field conditions, namely in air borne phenotyping. Therefore, alternative approaches, such as direct comparison between control irrigated and drought stressed plants, must be further developed.
An important and recent development in thermography, is the use of low cost equipment (e.g., thermal camera connected to a smartphone) to calculate water status indices, including CWSI and the stomatal conductance index (Petrie et al., 2019; Jouzier, 2020). Even though these instruments are less accurate, they are simpler and less expensive in monitoring plant stress responses and could also be used as pre-selection scanning to identify contrasting genotypes in terms of leaf/canopy temperature. However, when the expected temperature differences are small, such as in the case of studying intra-varietal variability, the effectiveness of this method is very limited.
Infrared sensors together with RGB sensors were also used in depth (3D) cameras. Recent technological advances that have been used for field phenotyping of grapevines have enabled the manufacture of consumer-grade depth cameras able to produce RGB information, infrared images, and 3D depth data (Milella et al., 2019). These systems might provide an alternative to the more expensive light detection and ranging (LiDAR) systems, in three-dimensional (3D) canopy reconstruction (Milella et al., 2019).
Chlorophyll Fluorescence (Conventional and Imaging)
The emission of chlorophyll fluorescence (CF) is widely used as a contactless method to assess photochemical use of energy and its non-photochemical dissipation (NPQ). The intensity of CF is variable over time depending on the photosynthetic activity and has been used to estimate plant stress, maximum potential PSII efficiency (Fv/Fm), quantum yield, and electron transport rate (ETR). Chlorophyll fluorescence has been extensively used in the assessment of biotic and abiotic stress evaluation in grapevine (Su et al., 2015; Carvalho et al., 2016; Ju et al., 2018) and it has been introduced in HTPP (Marques da Silva, 2016). At leaf level, it is measured mostly with two classes of instruments: pulse amplitude modulating fluorometers and continuous excitation fluorometers. Chlorophyll fluorescence induction (CFIN) is widely used in stress physiology research related to photosynthesis as it provides several relevant information and it is both non-destructive and cheap (since it uses continuous excitation fluorometers, much cheaper than modulated fluorometers; Humplík et al., 2015). Vitis species present significant interspecific and intervarietal differences in the patterns of rapid fluorescence induction (Marques da Silva et al., 2020). However, conventional fluorometry (modulated or continuous) is a point measurement, where the signal is collected generally by an optical fiber that is in contact to or in close proximity to the leaf. This means that leaves have to be manually selected and processed, making automation impossible and thereby excluding these techniques from HTPP processes. On the contrary, chlorophyll fluorescence imaging (CFI) can collect whole-plant images and might be, therefore, included in HTPP platforms.
The use of CFI allows the study of spatial and temporal heterogeneities in fluorescence emission patterns at the level of cells, leaves, plants, or a whole field, and has potential use to identify stress tolerance and for genotype screening in breeding programs (Gorbe and Calatayud, 2012; Osório et al., 2014; Cen et al., 2017; McAusland et al., 2019; Sánchez-Moreiras et al., 2020). CFI is useful to asses stomatal patchiness and heterogeneity of photosynthetic activity (Omasa and Takayama, 2003), overcoming the problems of point measurements due to the high variability at leaf level (Ehlert and Hincha, 2008). Furthermore, imaging fluorimeters may allow the measurement of several samples (replicates) at the same time. However, assessment of fluorescence parameters that require sample dark adaptation (e.g., Fv/Fm) is not feasible in field phenotyping, but informative parameters not requiring dark adaptation (e.g., the photosynthetic ETR) can be measured, although the requirement of a low intensity modulated measuring pulse poses technical difficulties for remote measurements. Fluorescence imaging is under rapid technical development and new instruments are now available (Herritt et al., 2020). Nevertheless, the high cost and limited operational performance in field can hinder their use in large scale field phenotyping in grapevine in the near future.
Relevant information on stress conditions can also be obtained from the analysis of the spectral signature of chlorophyll fluorescence, which is collected after laser excitation in the laser induced fluorescence technique (Gameiro et al., 2016). This technique does not require sample dark adaptation or close proximity to the sample and therefore might be suitable for field HTPP (Marques da Silva, 2016; Marques da Silva and Utkin, 2018).
Multispectral and Hyperspectral Imaging
Several important photosynthesis-related parameters can be investigated through the spectral composition of the light reflected by the plant, fruits, leaves, and canopy. The principle is that reflectance differences are related to chlorophyll, carotenoids, nitrogen, or water content (Walter et al., 2015), in particular, the reflectance analyzed in the visible, near-infrared, and short wavelength infrared spectrum (SWIR). The latter is used for the estimation of plant’s water status. The reflectance can be measured by spectrometers (Barradas et al., 2021), which provide point measurements (low/absent spatial resolution, very high spectral resolution), and by multispectral or hyperspectral cameras, which provide images (high spatial resolution, low/very low spectral resolution). A multitude of reflectance indexes have been published (for review, see Xue and Su, 2017), but most are suitable only for spectroscopic measurements, since they require the input of reflectance obtained at specific wavelengths, i.e., in a very narrow spectral band. However, as discussed above, point measurements are not suitable for HTPP and, therefore, multispectral or hyperspectral imaging is necessary. These measurements, initially used for remote sensing analysis of natural ecosystems, are also suitable for plant/crop phenotyping (Humplík et al., 2015), the main limitation, as for the canopy temperature analysis, is the spatial variability to which the plants are subjected during the measurement, and also the very high costs of the hyperspectral cameras (Table 1). SWIR measurements are at the basis of indices like the normalized difference vegetation index (NDVI), an estimator of the chlorophyll (chl) content, and the proportion of chl a in relation to chl b, and the photochemical reflectance index (PRI) which allows to estimate the photosynthetic efficiency by measuring the redox status of carotenoids (Humplík et al., 2015) that is part of the non-photochemical de-excitation pathway (Demmig-Adams et al., 2012), and in turn is correlated with photosynthetic light use efficiency (Sims and Gamon, 2002). A portable apparatus for NDVI ground-based measurement was tested in grapevine to estimate plant vigor by vine leaf area index (VLAI; Drissi et al., 2009), and the authors reported that the sensor is adequate to estimate plant vigor as VLAI and canopy gap, but only before the canopy growth saturates the response.
Two indices based on reflectance measurements, R690/R600 and R740/R800, where R690 and R740 are the chlorophyll fluorescence emission peaks and R600 and R800 are bands not affected by chlorophyll fluorescence, have been used to indirectly track changes in steady-state chlorophyll fluorescence due to heat and water stress (Dobrowski et al., 2005). Both indices had a strong positive curvilinear relation with steady state fluorescence (Fs).
More recently, Gutiérrez et al. (2018) showed the feasibility of a novel approach to classify leaves from several grapevine varieties grown in field conditions. The authors used on-the-go hyperspectral imaging at considerable speed (5km/h) and different machine learning algorithms.
Near infrared (NIR) hyperspectral imaging was also used to accurately predict anthocyanin content and evolution during development of Cabernet Sauvignon grapes from veraison to ripening (Chen et al., 2015). Also, NIR hyperspectral imaging was used to predict the quantification of total phenolic anthocyanins and flavanols in grapes of two red varieties, Syrah and Aragonez (syn Tempranillo; Nogales-Bueno et al., 2015). The results identified quantifiable differences between the two varieties regarding these parameters and, interestingly the authors observed a large range of distribution of values in each variety. Another study, performed in red and white varieties of table grapes, successfully used NIR hyperspectral imaging to predict sugar, total flavonoid, and total anthocyanin contents (Gabrielli et al., 2021). Sen et al. (2016) showed that the combination of visible and mid infrared (MIR, 4,000–650cm−1) ranges with methods of multivariate analysis improved the prediction of anthocyanin compounds and total phenols in wine as opposed to using NIR range alone. All these studies emphasize the importance of the robustness of the models adjusted. Furthermore, these traits are subject to high environmental variability, which can significantly change the rates of accumulation and degradation of sugars, flavonoids, and anthocyanins (Rienth et al., 2021). Also, intracluster berry heterogeneity can also be a main bias for individual berry phenotyping (Rienth et al., 2021). However, berry composition parameters have been used in grapevine selection (Table 2) with a high degree of success, and the effects of the environment can be overcome with an appropriate experimental set up and with sampling in several seasons (Gonçalves et al., 2016). Therefore, it may be possible to apply NIR hyperspectral imaging to clonal phenotyping to obtain data on berry composition for selection.
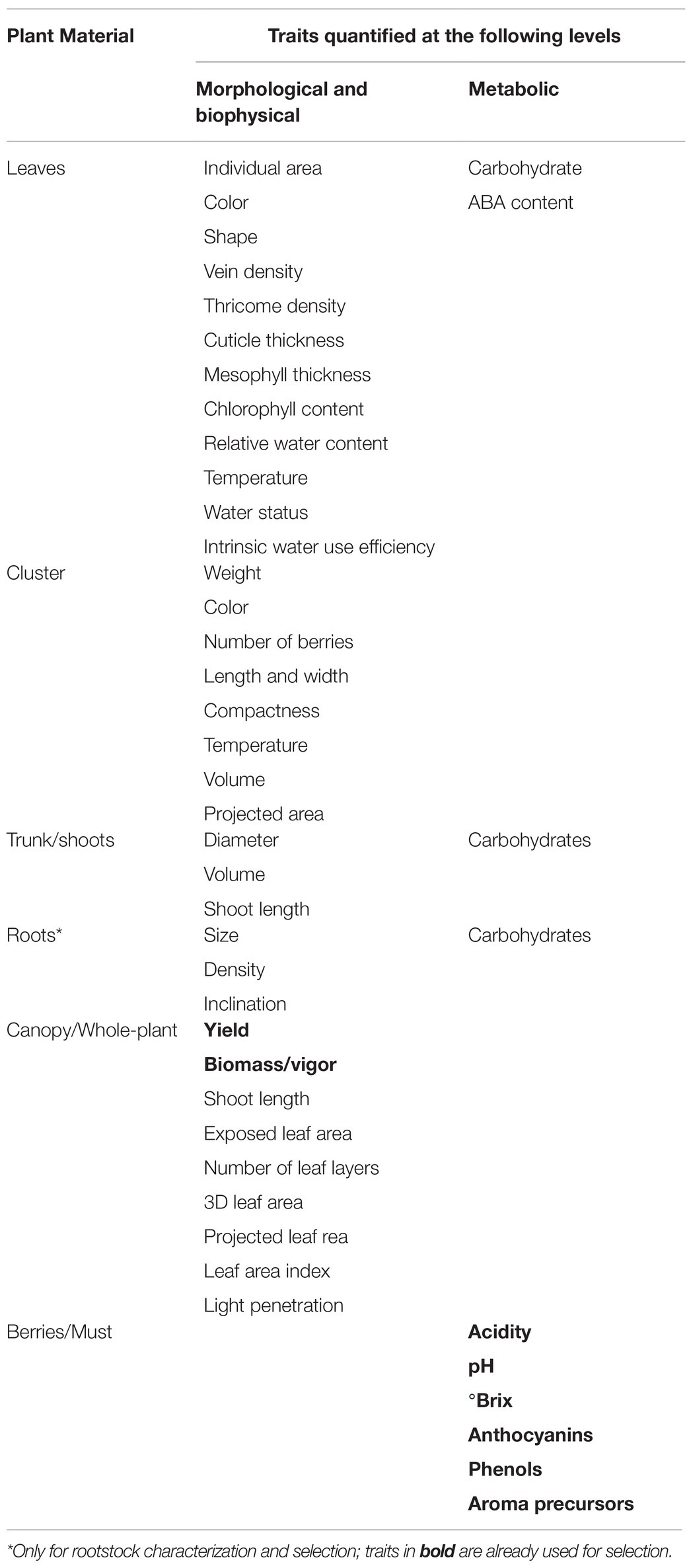
Table 2. Non-exhaustive list of phenotypic traits used in studies focused on agronomic, morphological, and eco-physiological characterization of grapevine genotypes.
Light Detection and Ranging
Light detection and ranging (LiDAR) is an active sensing technology that emits short-wavelength lasers, that can be visible, ultraviolet, or near- infrared light, to measure the distance from the sensor to the target according to laser speed and flight time recorded by a timer (Lin, 2015). These measurements are then translated into a 3D structure, built on the angle of the emitting laser collected by an angle encoder. LiDAR has some advantages, such as high throughput, high spatial resolution, high reproducibility, and the characteristics that make it suitable for field measurements, independency from light conditions, and the ability of the short-wavelength laser to penetrate the vegetation canopy (Jin et al., 2021). LiDAR sensors were first used in viticulture in 1998 to estimate several viticultural indices characterizing foliage distribution as well as attributes of the light microclimate in the canopy (Mabrouk and Sinoquet, 1998). The values obtained correlated well with those obtained by traditional methods and the authors were able to calculate bunch exposure and relate it with grape composition, namely sugar content, anthocyanins, and phenolics (Mabrouk and Sinoquet, 1998). This represented a major breakthrough in the estimation of key viticultural traits in an indirect, fast, reproducible, and non-destructive approach. The geometry of plant canopies can also be calculated using LiDAR, during the winter dormancy period, to calculate pruning weight, a previously laborious but extremely informative parameter to calculate plant vigor (Tagarakis et al., 2013, 2018). LAI was also successfully estimated with a laser sensor (Arnó et al., 2013), the authors obtained good correlations between LAI and canopy volume, as well as between LAI and tree area index. Nowadays, automated mobile platforms that move along rows scanning the vines are available. They are able to identify different managing systems and to calculate pruning weight, trunk, and cordon volume (Siebers et al., 2018). Water deficit can also be indirectly calculated through the measurement of plant leaf area, as it correlates well with the apparent soil electrical conductivity (ECa), giving an indication of the plant’s water needs (Tsoulias et al., 2019).
Evaluating Success of Phenotyping for Plant Breeding and Selection
A well planned phenotyping procedure is a critical task in plant breeding because it is the starting point for any efficient selection of plants, as illustrated for grapevine in Figure 1. When working with quantitative traits (the most frequent and economically important ones, such as yield, tolerance, or quality), it is necessary to understand the meaning of the obtained phenotypic value. This requires the quantification of the part of the measured trait that is due to the genotypic causes.
In classical models of quantitative genetics (i.e., balanced data with no random effects other than those associated with genotypes and error, and diagonal variance-covariance matrices), the proportion of total variance (phenotypic variance) that is genetic is called broad sense heritability (Falconer and Mackay, 1996). At the level of the mean of the genotypes, the classical concept of broad-sense heritability () is defined as
where , , and are the genotypic variance, error variance, and number of replicates, respectively. The broad sense heritability is an important indicator of the quality of the experimental design of the trials for the evaluation of a target quantitative trait and, consequently, of the success of genetic selection. Due to its importance in the context of plant breeding of quantitative traits, several studies addressed the problem of defining the establishment of a generalized measure applicable to more complex models (Cullis et al., 2006; Oakey et al., 2006; Piepho and Möhring, 2007; Welham et al., 2010), including in the context of grapevine selection (Gonçalves et al., 2013). To summarize all these approaches, an approximate generalized measure of broad-sense heritability can be presented as
where is the average of the predicted error variance of genotypic effects and is the genotypic variance.
Another key concept is the prediction of genetic gain (R) for the several traits evaluated. In the context of ancient grapevine varieties and under the classical models, it is defined as
where is the differential of selection, that is, the difference between the selected group of genotypes and the mean of the population and is the broad-sense heritability (Falconer and Mackay, 1996). Similarly, the genetic gain of selection is the mean of the Empirical Best Linear Unbiased Predictors (EBLUPs) of the genotypic effects of the top-ranked selected genotypes. This last definition is also applicable for more complex models. A selection based on EBLUPs of the genotypic effects of the best model would be more efficient and lead to higher genetic gains.
To quantify and obtain high values of heritability and high predicted genetic gains, that is, to achieve precision and accuracy in the evaluation of quantitative traits, agronomic experiments demand a well-planned phenotyping, which involves the establishment of field trials with efficient experimental designs (with repetition, randomization, and efficient control of spatial variation) and correspondent appropriate models for data analysis (mixed models).
Agronomic experiments are usually large, expensive, and take many years to accomplish. Additionally, they are typically subject to high background variability due to soil fertility and availability of water trends in the field (spatial variation) and cultural techniques, and other environmental deviations. This variability must be controlled through the type of experimental design. Typically the effective control of background variability is made by blocking or by using covariates together with sufficient replication of genotypes (Piepho and Edmondson, 2018). The experimental designs used in agriculture to reach these objectives have a long history and are routinely used in agronomic experiments, such as randomized complete block designs, latin squares, split-plot designs, and the family of incomplete block designs (Giesbrecht and Gumpertz, 2004). Nowadays, precision agriculture tools (e.g., soil water sensors, EC, and NDVI maps) can also be used to optimize the establishment of the experimental design in the field. These tools can help find homogenous patterns of soil composition and water availability that enable the definition of incomplete and complete blocks.
In grapevine, field trials for polyclonal selection comprise a representative sample of the intravarietal variability of the variety under study (Martins and Gonçalves, 2015; OIV, 2019). The experimental designs useful for screening a large number of genotypes and to provide reliable guidance to select the best genotypes are described in Gonçalves et al. (2010), and the most efficient are alpha designs and resolvable row-column designs.
The application and testing of HTPP methodologies in field trials with adequate experimental designs and the need to quantify the quality of the measurements obtained are constant concerns in the plant breeding context. For example, Tattaris et al. (2016) used spring wheat lines trials, established under an alpha-lattice design, with either two or three replications, to test HTPP monitoring of plant physiological traits (canopy temperature and a vegetation index). Tattaris et al. (2016) compared three remote sensing approaches using a low flying unmanned aerial vehicle, with that of proximal sensing, and satellite-based imagery to determine the most viable approaches for large scale crop genetic improvement. The results obtained supported the use of those techniques for HTPP for both precision and efficiency. In turn, Singh et al. (2019) demonstrated the considerable power of unmanned aerial systems or drone-based phenotyping as a HTPP alternative to visual assessments for the complex phenological trait of lodging, which significantly impacts yield and quality in many crops including wheat. They tested and validated quantitative assessment of lodging on 2,640 wheat breeding plots over the course of 2years using differential digital elevation models. A total of 590 and 595 unique wheat entries along with the check varieties were planted in alpha-lattice field design during seasons 2016 and 2017, respectively. The broad-sense heritability of visual and digital lodging measures ranged between 0.50 and 0.59. Andrade-Sanchez et al. (2014), proved that a tractor-based phenotyping system was capable of reliably acquiring and recording data for canopy temperature, height and reflectance on experimental plots of cotton plants throughout the growing season in the field. To prove that, they evaluated field trials with 25 Pima cotton cultivars arranged as a lattice design with four replications in a total of 200 plots. Measurements of canopy height, NDVI, and temperature all showed large differences among cultivars and expected interactions of cultivars with water regime and time of day. Broad-sense heritability ranged from 0.86 to 0.96 for canopy height, from 0.28 to 0.90 for the NDVI, and from 0.01 to 0.90 for temperature. Also in the context of high throughput phenotyping, Junker et al. (2015) highlighted some experimental procedures to optimize the quantitative evaluation of crop plant performance. In grapevine, Carvalho et al. (2020) evaluated abiotic stress tolerance, measured by the surface leaf temperature (SLT) of clones under environmental conditions of drought and extreme heat for 3years. SLT sets the boundary condition for the latent and sensible heat transport through vegetation, soil, and atmosphere, depending on the availability of moisture at the interface soil- atmosphere (Fuchs, 1990), giving an estimate of the response of a leaf to the environmental parameters affecting it at any time (air temperature, relative humidity, solar radiation, leaf resistance, and boundary layer resistance; Udompetaikul et al., 2011). By utilizing simple measurement devices and an experimental set up that enables the separation between environmental influence and the physiological response, it is possible to study the relationship between these parameters. A plant is able to keep a SLT lower than ambient temperature by controlling stomatal aperture and thus leaf gas exchange through stomata. The capacity to control stomata opening and thus CO2 intake for photosynthesis regardless of high air temperature gives the clones that hold it an advantage to face heat stress without loss of yield and quality of the grapes produced. The application of the methodology was done in a field trial with 255 different clones established according to a resolvable incomplete block experimental design with five complete blocks: each complete block comprised the effect of the complete block and the effect of the day; each column within each complete block, with approximately 13 plots, constituted an incomplete block, which comprised the effect of the time of day. With this type of experimental design, it was possible to prove the existence of significant genetic variability within the variety for the trait SLT and the values of generalized broad sense heritability ranged between 0.44 and 0.54, corresponding to a quantifiable genetic component difference of 3°C between the coolest and warmest of the 255 genotypes measured in three consecutive seasons.
In short, in the context of plant breeding, to perform fast, massive, or HTPP, the establishment of field trials with adequate experimental designs and the estimation of several genetic and statistical parameters, that provide information about the meaning and the quality of the data obtained, is mandatory.
Traits to Use in Phenotyping for Selection
A more sustainable viticulture must involve the use of locally adapted varieties and selected material of those varieties. Phenotyping must enable a reliable identification of genotypes with the desired traits, whether yield, specific berry composition, or tolerance to stress and should contribute to estimate their genotypic diversity.
So far, grapevine selection within ancient varieties relies on the exhaustive gathering of specific data from all the genotypes in an experimental field (with biological replicates it generally reaches more than 3,000 plants; Martins and Gonçalves, 2015). Any possibility of automation without loss of reproducibility or precise quantification should be very welcome. Moreover, the data gathered are so dependent of the effects of the environment that only an efficient experimental design allows to control those effects, and most importantly, to quantify the contribution of the genetic component, the so called broad sense heritability.
Therefore, an effective selection of grapevine genotypes takes several years, requiring much labor and costs. The need to evaluate hundreds of genotypes in several repetitions occupies between 1.0 and 2.0ha and an efficient control of the field installation cannot allow the use of ready-made grafted plants. With all these constraints, such trials are only viable for economically prized varieties.
With respect to the data gathering itself, currently, only yield and berry composition have been exhaustively tested and quantified in selection trials (Gonçalves et al., 2016; Table 2). Yield is quantified by handpicking and weighing the production of each plant in the trial. However, the most time- and labor-consuming task of selection is berry composition analysis, which requires collection of individual berry samples from all grapevines and making the quantifications of pH, total acidity, and soluble solids and, in red varieties, anthocyanins, and phenols, in the lab, following standardized and well-established protocols.
The use of traits such as leaf temperature or the simpler RGB offer still limitations in assessing properly yield but mostly berry quality traits. More testing to find robust correlations between Tleaf or leaf color or canopy size and yield and quality are needed therefore, to make them used in selection for berry composition and final yield per plant.
Conclusion and Prospects
Screening and characterization of inter- and intravarietal variability of autochthonous grapevine varieties has a crucial importance not only for the Portuguese but also for the global wine industry. The future competitiveness and higher sustainability of the sector should be largely based on the use of well adapted plant material (rootstocks and varieties). Drought and heat stress are major driving forces for grapevine selection and breeding as means to identify the most resistant and better adapted grapevine varieties/genotypes. In fact, one of the medium/long term strategies to respond to climate change adversities and the problems of increased stress is based on the selection and use of superior genotypes.
A major future challenge for grapevine field phenotyping is to exhaustively evaluate relevant traits for selection purposes, such as tolerance to abiotic stress. Moreover, the available imaging technologies (e.g., RGB, thermal, and multispectral) need to be adapted and optimized to large field trials (Table 1) to provide reliable quantitative data for a robust, reproducible, and comparable analysis at different levels (leaf, canopy, berry, and cluster). This task can be particularly challenging when dealing with intra-varietal characterization and clonal selection, attending to the potentially smaller differences between genotypes for some traits, namely those related to tolerance to abiotic stress. Also, the possibility for automation of data gathering for traits already under analysis, such as berry composition, would expedite measurements of large experiments.
Proximal and remote sensing technologies have undergone great developments in recent years and have become more accurate, cheaper and, in some cases, more user-friendly. The attention of the scientific and industry communities toward these technologies is very high due to their potential for field analysis and subsequent management of variability in field conditions. In viticulture, they are chiefly applied in the agronomic management of the vineyard as part of the so called “precision viticulture,” but some proximal and remote sensing technologies have potential for phenotyping and selection. For this purpose, it will be necessary to deepen and clarify the link between the indirect digital measurements obtained by sensors and the morphological, eco-physiological, and metabolic parameters under examination, which sometimes is still doubtful. The following step would be to develop specific and standardized protocols to apply these sensing technologies to grapevine phenotyping in field conditions, mainly focused on leaf, berry, or canopy/plant traits that are closely related to physiologically complex phenomena, such as that of tolerance to abiotic stress.
The advance in imaging technologies, robotics and computing will enable to establish and perform new assays for genotype characterization and selection that can be carried out under field conditions. This can also provide more tools to study grapevine development and behavior under climate change conditions.
Author Contributions
JC: idea, writing, editing, reviewing and funding. LC and EG: writing, editing, and reviewing. JMS: writing, reviewing, and funding. All authors contributed to the article and approved the submitted version.
Funding
This work was supported by the Fundação para a Ciência e Tecnologia (FCT) research unit BioISI (UID/MULTI/04046/2019) and the FCT funded R&D project INTERPHENO (PTDC/ASP-PLA/28726/2017). LC acknowledges the funding by FCT through DL57/2016/CP1382/CT0024. Linking Landscape, Environment, Agriculture, and Food (LEAF) research center has been funded by national funds through FCT – Fundação para a Ciência e a Tecnologia, I.P., in the scope of the projects UID/AGR/04129/2013; UID/AGR/04129/2019, and presently UIDB/ and UIDP/04129/2020.
Conflict of Interest
The authors declare that the research was conducted in the absence of any commercial or financial relationships that could be construed as a potential conflict of interest.
Publisher’s Note
All claims expressed in this article are solely those of the authors and do not necessarily represent those of their affiliated organizations, or those of the publisher, the editors and the reviewers. Any product that may be evaluated in this article, or claim that may be made by its manufacturer, is not guaranteed or endorsed by the publisher.
Footnotes
References
Abdelghafour, F., Rosu, R., Keresztes, B., Germain, C. P., and Da Costa, J. P. (2019). A Bayesian framework for joint structure and colour based pixel-wise classification of grapevine proximal images. Comput. Electron. Agric. 158, 345–357. doi: 10.1016/j.compag.2019.02.017
Agüero, C. B., Uratsu, S. L., Greve, C., Powell, A. L. T., Labavitch, J. M., Meredith, C. P., et al. (2005). Evaluation of tolerance to Pierce’s disease and Botrytis in transgenic plants of Vitis vinifera L. expressing the pear PGIP gene. Mol. Plant Pathol. 6, 43–51. doi: 10.1111/J.1364-3703.2004.00262.X
Andrade-Sanchez, P., Gore, M. A., Heun, J. T., Thorp, K. R., Carmo-Silva, A. E., French, A. N., et al. (2014). Development and evaluation of a field-based high-throughput phenotyping platform. Funct. Plant Biol. 41, 68–79. doi: 10.1071/FP13126
Araus, J. L., Kefauver, S. C., Zaman-Allah, M., Olsen, M. S., and Cairns, J. E. (2018). Translating high-throughput phenotyping into genetic gain. Trends Plant Sci. 23, 451–466. doi: 10.1016/j.tplants.2018.02.001
Arnó, J., Escolà, A., Vallès, J. M., Llorens, J., Sanz, R., Masip, J., et al. (2013). Leaf area index estimation in vineyards using a ground-based LiDAR scanner. Precis. Agric. 14, 290–306. doi: 10.1007/s11119-012-9295-0
Barradas, A., Correia, P. M. P., Silva, S., Mariano, P., Pires, M. C., Matos, A. R., et al. (2021). Comparing machine learning methods for classifying plant drought stress from leaf reflectance spectra in Arabidopsis thaliana. Appl. Sci. 11:6392. doi: 10.3390/app11146392
Bota, J., Tomás, M., Flexas, J., Medrano, H., and Escalona, J. M. (2016). Differences among grapevine cultivars in their stomatal behavior and water use efficiency under progressive water stress. Agric. Water Manag. 164, 91–99. doi: 10.1016/j.agwat.2015.07.016
Briglia, N., Montanaro, G., Petrozza, A., Summerer, S., Cellini, F., and Nuzzo, V. (2019). Drought phenotyping in Vitis vinifera using RGB and NIR imaging. Sci. Hortic. 256:108555. doi: 10.1016/j.scienta.2019.108555
Buckley, T. N. (2019). How do stomata respond to water status? New Phytol. 224, 21–36. doi: 10.1111/nph.15899
Carvalho, L. C., and Amâncio, S. (2019). Cutting the Gordian knot of abiotic stress in grapevine: From the test tube to climate change adaptation. Physiol. Plant. 165, 330–342. doi: 10.1111/ppl.12857
Carvalho, L. C., Coito, J. L., Gonçalves, E. F., Chaves, M. M., and Amâncio, S. (2016). Differential physiological response of the grapevine varieties Touriga Nacional and Trincadeira to combined heat, drought and light stresses. Plant Biol. 18, 101–111. doi: 10.1111/plb.12410
Carvalho, L., Gonçalves, E., Amâncio, S., and Martins, A. (2020). Selecting Aragonez genotypes able to outplay climate change–driven abiotic stress. Front. Plant Sci. 11:599230. doi: 10.3389/fpls.2020.599230
Carvalho, L. C., Silva, M., Coito, J. L., Rocheta, M. P., and Amancio, S. (2017). Design of a custom RT-qPCR array for assignment of abiotic stress tolerance in traditional Portuguese grapevine varieties. Front. Plant Sci. 8:1835. doi: 10.3389/fpls.2017.01835
Cen, H., Weng, H., Yao, J., He, M., Lv, J., Hua, S., et al. (2017). Chlorophyll fluorescence imaging uncovers photosynthetic fingerprint of citrus Huanglongbing. Front. Plant Sci. 8:1509. doi: 10.3389/fpls.2017.01509
Chaves, M. M., Zarrouk, O., Francisco, R., Costa, J. M., Santos, T., Regalado, A. P., et al. (2010). Grapevine under deficit irrigation: hints from physiological and molecular data. Ann. Bot. 105, 661–676. doi: 10.1093/aob/mcq030
Chen, S., Zhang, F., Ning, J., Liu, X., Zhang, Z., and Yang, S. (2015). Predicting the anthocyanin content of wine grapes by NIR hyperspectral imaging. Food Chem. 172, 788–793. doi: 10.1016/j.foodchem.2014.09.119
Ciaffi, M., Paolacci, A. R., Paolocci, M., Alicandri, E., Bigini, V., Badiani, M., et al. (2019). Transcriptional regulation of stilbene synthases in grapevine germplasm differentially susceptible to downy mildew. BMC Plant Biol. 19:404. doi: 10.1186/s12870-019-2014-5
Clawson, K. L., Jackson, R. D., and Pinter, P. J. (1989). Evaluating plant water stress with canopy temperature differences. J. Agron. 81, 858–863. doi: 10.2134/agronj1989.00021962008100060004x
Costa, J. M., Egipto, R., Sánchez-Virosta, A., Lopes, C. M., and Chaves, M. M. (2019a). Canopy and soil thermal patterns to support water and heat stress management in vineyards. Agric. Water Manag. 216, 484–496. doi: 10.1016/j.agwat.2018.06.001
Costa, J. M., Grant, O. M., and Chaves, M. M. (2013). Thermography to explore plant-environment interactions. J. Exp. Bot. 64, 3937–3949. doi: 10.1093/jxb/ert029
Costa, J. M., Marques da Silva, J., Pinheiro, C., Barón, M., Mylona, P., Centritto, M., et al. (2019b). Opportunities and limitations of crop phenotyping in southern European countries. Front. Plant Sci. 10:1125. doi: 10.3389/fpls.2019.01125
Costa, J. M., Ortuño, M. F., Lopes, C. M., and Chaves, M. M. (2012). Grapevine varieties exhibiting differences in stomatal response to water deficit. Funct. Plant Biol. 39, 179–189. doi: 10.1071/FP11156
Costa, C., Schurr, U., Loreto, F., Menesatti, P., and Carpentier, S. (2019c). Plant phenotyping research trends, a science mapping approach. Front. Plant Sci. 9:1933. doi: 10.3389/fpls.2018.01933
Coupel-Ledru, A., Lebon, E., Christophe, A., Doligez, A., Cabrera-Bosquet, L., Péchier, P., et al. (2014). Genetic variation in a grapevine progeny (Vitis vinifera L. cvs Grenache x Syrah) reveals inconsistencies between maintenance of daytime leaf water potential and response of transpiration rate under drought. J. Exp. Bot. 65, 6205–6218. doi: 10.1093/jxb/eru228
Cullis, B. R., Smith, A. B., and Coombes, N. E. (2006). On the design of early generation variety trials with correlated data. J. Agric. Biol. Environ. Stat. 11, 381–393. doi: 10.1198/108571106X154443
dal Santo, S., Palliotti, A., Zenoni, S., Tornielli, G. B., Fasoli, M., Paci, P., et al. (2016). Distinct transcriptome responses to water limitation in isohydric and anisohydric grapevine cultivars. BMC Genomics 17:815. doi: 10.1186/s12864-016-3136-x
Das Choudhury, S., Samal, A., and Awada, T. (2019). Leveraging image analysis for high-throughput plant phenotyping. Front. Plant Sci. 10:508. doi: 10.3389/fpls.2019.00508
Dayer, S., Herrera, J. C., Dai, Z., Burlett, R., Lamarque, L. J., Delzon, S., et al. (2020). The sequence and thresholds of leaf hydraulic traits underlying grapevine varietal differences in drought tolerance. J. Exp. Bot. 71, 4333–4344. doi: 10.1093/jxb/eraa186
de Castro, A. I., Jiménez-Brenes, F. M., Torres-Sánchez, J., Peña, J. M., Borra-Serrano, I., and López-Granados, F. (2018). 3-D characterization of vineyards using a novel UAV imagery-based OBIA procedure for precision viticulture applications. Remote Sens. 10:584. doi: 10.3390/rs10040584
DeBolt, S., Cook, D. R., and Ford, C. M. (2006). L-tartaric acid synthesis from vitamin C in higher plants. PNAS 103, 5608–5613. doi: 10.1073/pnas.0510864103
Demmig-Adams, B., Cohu, C. M., Muller, O., and Adams, W. W. (2012). Modulation of photosynthetic energy conversion efficiency in nature: from seconds to seasons. Photosynth. Res. 113, 75–88. doi: 10.1007/s11120-012-9761-6
di Gennaro, S. F., Toscano, P., Cinat, P., Berton, A., and Matese, A. (2019). A low-cost and unsupervised image recognition methodology for yield estimation in a vineyard. Front. Plant Sci. 10:559. doi: 10.3389/fpls.2019.00559
Diago, M. P., Correa, C., Millán, B., Barreiro, P., Valero, C., and Tardaguila, J. (2012). Grapevine yield and leaf area estimation using supervised classification methodology on RGB images taken under field conditions. Sensors 12, 16988–17006. doi: 10.3390/s121216988
Diago, M. P., Fernández-Novales, J., Fernandes, A. M., Melo-Pinto, P., and Tardaguila, J. (2016). Use of visible and short-wave near-infrared hyperspectral imaging to fingerprint anthocyanins in intact grape berries. J. Agric. Food Chem. 64, 7658–7666. doi: 10.1021/acs.jafc.6b01999
Dobrowski, S. Z., Pushnik, J. C., Zarco-Tejada, P. J., and Ustin, S. L. (2005). Simple reflectance indices track heat and water stress-induced changes in steady-state chlorophyll fluorescence at the canopy scale. Remote Sens. Environ. 97, 403–414. doi: 10.1016/j.rse.2005.05.006
Dogan, A., Uyak, C., Keskin, N., Akcay, A., Sensoy, R. I. G., and Ercisli, S. (2018). Grapevine leaf area measurements by using pixel values. C. R. Acad. Bulg. Sci. 71, 772–779. doi: 10.7546/CRABS.2018.06.07
Drissi, R., Goutouly, J. P., Forget, D., and Gaudillere, J. P. (2009). Nondestructive measurement of grapevine leaf area by ground normalized difference vegetation index. Agron. J. 101, 226–231. doi: 10.2134/agronj2007.0167
Ehlert, B., and Hincha, D. K. (2008). Chlorophyll fluorescence imaging accurately quantifies freezing damage and cold acclimation responses in Arabidopsis leaves. Plant Methods 4:12. doi: 10.1186/1746-4811-4-12
Falconer, D. S., and Mackay, T. F. C. (1996). An Introduction to Quantitative Genetics. 4th Edn. London: Prentice Hall.
Field, S. K., Smith, J. P., Morrison, E. N., Emery, R. J. N., and Holzapfel, B. P. (2020). Soil temperature prior to veraison alters grapevine carbon partitioning, xylem sap hormones, and fruit set. Am. J. Enol. Vitic. 71, 52–61. doi: 10.5344/ajev.2019.19038
Fiorani, F., and Schurr, U. (2013). Future scenarios for plant phenotyping. Annu. Rev. Plant Biol. 64, 267–291. doi: 10.1146/annurev-arplant-050312-120137
Fraga, H. (2020). Climate change: a new challenge for the winemaking sector. Agronomy 10:1465. doi: 10.3390/agronomy10101465
Fraga, H., Malheiro, A. C., Moutinho-Pereira, J., and Santos, J. A. (2012). An overview of climate change impacts on European viticulture. Food Energy Secur. 1, 94–110. doi: 10.1002/fes3.14
Fritz, M. A., Rosa, S., and Sicard, A. (2018). Mechanisms underlying the environmentally induced plasticity of leaf morphology. Front. Genet. 9:478. doi: 10.3389/fgene.2018.00478
Fuchs, M. (1990). Infrared measurement of canopy temperature and detection of plant water stress. Theor. Appl. Climatol. 42, 253–261. doi: 10.1007/BF00865986
Gabrielli, M., Lançon-Verdier, V., Picouet, P., and Maury, C. (2021). Hyperspectral imaging to characterize table grapes. Chem. Aust. 9:71. doi: 10.3390/chemosensors9040071
Gago, P., Conejero, G., Martínez, M. C., This, P., and Verdeil, J. L. (2019). Comparative anatomy and morphology of the leaves of grenache noir and Syrah grapevine cultivars. SAJEV 40, 1–9. doi: 10.21548/40-2-3031
Gambetta, G. A., Herrera, J. C., Dayer, S., Feng, Q., Hochberg, U., and Castellarin, S. D. (2020). The physiology of drought stress in grapevine: towards an integrative definition of drought tolerance. J. Exp. Bot. 71, 4658–4676. doi: 10.1093/jxb/eraa245
Gambetta, J. M., Holzapfel, B. P., Stoll, M., and Friedel, M. (2021). Sunburn in grapes: a review. Front. Plant Sci. 11:604691. doi: 10.3389/fpls.2020.604691
Gameiro, C., Utkin, A. B., Cartaxana, P., da Silva, J. M., and Matos, A. R. (2016). The use of laser induced chlorophyll fluorescence (LIF) as a fast and non-destructive method to investigate water deficit in Arabidopsis. Agric. Water Manag. 164, 127–136. doi: 10.1016/j.agwat.2015.09.008
Gashu, K., Sikron Persi, N., Drori, E., Harcavi, E., Agam, N., Bustan, A., et al. (2020). Temperature shift between vineyards modulates berry phenology and primary metabolism in a varietal collection of wine grapevine. Front. Plant Sci. 11:588739. doi: 10.3389/fpls.2020.588739
Giesbrecht, F. G., and Gumpertz, M. L. (2004). Planning, Construction and Analysis of Comparative Experiments. New York: Wiley.
Gonçalves, E., Carrasquinho, I., Almeida, R., Pedroso, V., and Martins, A. (2016). Genetic correlations in grapevine and their effects on selection. Aust. J. Grape Wine Res. 22, 52–63. doi: 10.1111/ajgw.12164
Gonçalves, E., Carrasquinho, I., St. Aubyn, A., and Martins, A. (2013). Broad-sense heritability in mixed models for grapevine initial selection trials. Euphytica 189, 379–391. doi: 10.1007/s10681-012-0787-9
Gonçalves, E., St Aubyn, A., and Martins, A. (2010). Experimental designs for evaluation of genetic variability and selection of ancient grapevine varieties: A simulation study. Heredity 104, 552–562. doi: 10.1038/hdy.2009.153
Gorbe, E., and Calatayud, A. (2012). Applications of chlorophyll fluorescence imaging technique in horticultural research: a review. Sci. Hortic. 138, 24–35. doi: 10.1016/j.scienta.2012.02.002
Grant, O. M., Ochagavía, H., Baluja, J., Diago, M. P., and Tardáguila, J. (2016). Thermal imaging to detect spatial and temporal variation in the water status of grapevine (Vitis vinifera L.). J. Hortic. Sci. Biotechnol. 91, 43–54. doi: 10.1080/14620316.2015.1110991
Grant, O. M., Tronina, Ł., Jones, H. G., and Chaves, M. M. (2007). Exploring thermal imaging variables for the detection of stress responses in grapevine under different irrigation regimes. J. Exp. Bot. 58, 815–825. doi: 10.1093/jxb/erl153
Greer, D. H. (2020). Stomatal and non-stomatal limitations at different leaf temperatures to the photosynthetic process during the post-harvest period for Vitis vinifera cv. Chardonnay vines. N.Z.J. Crop Hortic. Sci. 48, 1–21. doi: 10.1080/01140671.2019.1632213
Gutiérrez, S., Diago, M. P., Fernández-Novales, J., and Tardaguila, J. (2017). On-the-go thermal imaging for water status assessment in commercial vineyards. Adv. Anim. Sci. 8, 520–524. doi: 10.1017/S204047001700108
Gutiérrez, S., Fernández-Novales, J., Diago, M. P., and Tardaguila, J. (2018). On-the-go hyperspectral imaging under field conditions and machine learning for the classification of grapevine varieties. Front. Plant Sci. 9:1102. doi: 10.3389/fpls.2018.01102
Gutiérrez-Gamboa, G., Zheng, W., and Martínez de Toda, F. (2021). Current viticultural techniques to mitigate the effects of global warming on grape and wine quality: a comprehensive review. Food Res. Int. 139:109946. doi: 10.1016/j.foodres.2020.109946
Harbertson, J. F., and Keller, M. (2012). Rootstock effects on deficit-irrigated winegrapes in a dry climate: grape and wine composition. Am. J. Enol. Vitic. 63, 40–48. doi: 10.5344/ajev.2011.11079
Harris, N. N., Luczo, J. M., Robinson, S. P., and Walker, A. R. (2013). Transcriptional regulation of the three grapevine chalcone synthase genes and their role in flavonoid synthesis in shiraz. Aust. J. Grape Wine Res. 19, 221–229. doi: 10.1111/ajgw.12026
Herritt, M. T., Pauli, D., Mockler, T. C., and Thompson, A. L. (2020). Chlorophyll fluorescence imaging captures photochemical efficiency of grain sorghum (Sorghum bicolor) in a field setting. Plant Methods 16:109. doi: 10.1186/s13007-020-00650-0
Humplík, J. F., Lazár, D., Husičková, A., and Spíchal, L. (2015). Automated phenotyping of plant shoots using imaging methods for analysis of plant stress responses—a review. Plant Methods 11:29. doi: 10.1186/s13007-015-0072-8
IPCC (2014). Climate Change 2014: Impacts, Adaptation, and Vulnerability. Part A: Global and Sectoral Aspects. Contribution of Working Group II to the Fifth Assessment Report of the Intergovernmental Panel on Climate Change. eds. C. B. Field, V. R. Barros, D. J. Dokken, K. J. Mach, M. D. Mastrandrea, T. E. Bilir et al. (Cambridge, UK and New York, USA: Cambridge University Press), 1132.
Irmak, S., Haman, D. Z., and Bastug, R. (2000). Determination of crop water stress index for irrigation timing and yield estimation of corn. Agron. J. 92, 1221–1227. doi: 10.2134/agronj2000.9261221x
Jiang, Y., and Li, C. (2020). Convolutional neural networks for image-based high-throughput plant Phenotyping: a review. Plant Phenomics 2020:4152816. doi: 10.34133/2020/4152816
Jin, S., Sun, X., Wu, F., Su, Y., Li, Y., Song, S., et al. (2021). Lidar sheds new light on plant phenomics for plant breeding and management: recent advances and future prospects. ISPRS J. Photogramm. Remote Sens. 171, 202–223. doi: 10.1016/j.isprsjprs.2020.11.006
Jones, H. G., Stoll, M., Santos, T., Sousa, C. D., Chaves, M. M., and Grant, O. M. (2002). Use of infrared thermography for monitoring stomatal closure in the field: application to grapevine. J. Exp. Bot. 53, 2249–2260. doi: 10.1093/jxb/erf083
Jouzier, P. (2020). Smartphone: The winegrowers’ Swiss army knife. IVES Technical Rev. 20. doi: 10.20870/ives-tr.2020.3622
Ju, Y. L., Yue, X. F., Zhao, X. F., Zhao, H., and Fang, Y. L. (2018). Physiological, micro-morphological and metabolomic analysis of grapevine (Vitis vinifera L.) leaf of plants under water stress. Plant Physiol. Biochem. 130, 501–510. doi: 10.1016/j.plaphy.2018.07.036
Junker, A., Muraya, M. M., Weigelt-Fischer, K., Arana-Ceballos, F., Klukas, C., et al. (2015). Optimizing experimental procedures for quantitative evaluation of crop plant performance in high throughput phenotyping systems. Front. Plant Sci. 5:770. doi: 10.3389/fpls.2014.00770
Kicherer, A., Herzog, K., Bendel, N., Klück, H.-C., Backhaus, A., Wieland, M., et al. (2017). Phenoliner: a new field phenotyping platform for grapevine research. Sensors 17:1625. doi: 10.3390/s1707162
Kicherer, A., Herzog, K., Pflanz, M., Wieland, M., Rüger, P., Kecke, S., et al. (2015). An automated field phenotyping pipeline for application in grapevine research. Sensors 15, 4823–4836. doi: 10.3390/s150304823
Klodt, M., Herzog, K., Töpfer, R., and Kremers, D. (2015). Field phenotyping of grapevine growth using dense stereo reconstruction. BMC Bioinformatics 16:143. doi: 10.1186/s12859-015-0560-x
Knoch, D., Abbadi, A., Grandke, F., Meyer, R. C., Samans, B., Werner, C. R., et al. (2019). Strong temporal dynamics of QTL action on plant growth progression revealed through high-throughput phenotyping in canola. Plant Biotechnol. J. 18, 68–82. doi: 10.1111/pbi.13171
Levin, A. D., Williams, L. E., and Matthews, M. A. (2019). A continuum of stomatal responses to water deficits among 17 wine grape cultivars (Vitis vinifera). Funct. Plant Biol. 47, 11–25. doi: 10.1071/FP19073
Li, Z., Guo, R., Li, M., Chen, Y., and Li, G. (2020). A review of computer vision technologies for plant phenotyping. Comput. Electron. Agric. 176:105672. doi: 10.1016/j.compag.2020.105672
Lin, Y. (2015). LiDAR: An important tool for next-generation phenotyping technology of high potential for plant phenomics? Comput. Electron. Agric. 119, 61–73. doi: 10.1016/j.compag.2015.10.011
Lorenzo, N., Diaz-Poso, A., and Roy, D. (2021). Heatwave intensity on the Iberian Peninsula: future climate projections. Atmos. Res. 258:105655. doi: 10.1016/j.atmosres.2021.105655
Lovisolo, C., Perrone, I., Carra, A., Ferrandino, A., Flexas, J., Medrano, H., et al. (2010). Drought-induced changes in development and function of grapevine (Vitis spp.) organs and in their hydraulic and non hydraulic interactions at the whole plant level: a physiological and molecular update. Funct. Plant Biol. 37, 98–116. doi: 10.1071/FP09191
Mabrouk, H., and Sinoquet, H. (1998). Indices of light microclimate and canopy structure of grapevines determined by 3D digitising and image analysis, and their relationship to grape quality. Aust. J. Grape Wine Res. 4, 2–13. doi: 10.1111/j.1755-0238.1998.tb00129.x
MacMIllan, P., Teixeira, G., Lopes, C. M., and Monteiro, A. (2021). The role of grapevine leaf morphoanatomical traits in determining capacity for coping with abiotic stresses: a review. Ciência Téc. Vitiv. 36, 75–88. doi: 10.1051/ctv/ctv2021360175
Marques da Silva, J. (2016). “Monitoring photosynthesis by in vivo chlorophyll fluorescence: application to high-throughput plant phenotyping,” in Applied Photosynthesis—New Progress. ed. M. Najafpour (Rijeka, Croatia: InTech Open), 3–22.
Marques da Silva, J., Figueiredo, A., Cunha, J., Eiras-Dias, J. E., Silva, S., Vanneschi, L., et al. (2020). Using rapid chlorophyll fluorescence transients to classify Vitis genotypes. Plan. Theory 9:174. doi: 10.3390/plants9020174
Marques da Silva, J., and Utkin, A. B. (2018). Application of laser-induced fluorescence in functional studies of photosynthetic biofilms. PRO 6:227. doi: 10.3390/pr6110227
Martins, A., and Gonçalves, E. (2015). “Grapevine breeding programmes in Portugal,” in Grapevine breeding programs for the wine industry. ed. A. G. Reynolds (Amsterdam, Netherlands: Elsevier Ltd.)
Matese, A., Baraldi, R., Berton, A., Cesaraccio, C., Di Gennaro, S. F., Duce, P., et al. (2018). Estimation of water stress in grapevines using proximal and remote sensing methods. Remote Sens. 10, 1–16. doi: 10.3390/rs10010114
Matthews, J. S. A., and Lawson, T. (2019). Climate change and stomatal physiology. Annu. Plant Rev. 2, 713–752. doi: 10.1002/9781119312994.apr0667
McAusland, L., Atkinson, J. A., Lawson, T., and Murchie, E. H. (2019). High throughput procedure utilising chlorophyll fluorescence imaging to phenotype dynamic photosynthesis and photoprotection in leaves under controlled gaseous conditions. Plant Methods 15:109. doi: 10.1186/s13007-019-0485-x
Merloni, E., Camanzi, L., Mulazzani, L., and Malorgio, G. (2018). Adaptive capacity to climate change in the wine industry: A Bayesian network approach. Wine Econ. Policy 7, 165–177. doi: 10.1016/j.wep.2018.11.002
Merlot, S., Mustilli, A. C., Genty, B., North, H., Lefebvre, V., Sotta, B., et al. (2002). Use of infrared thermal imaging to isolate Arabidopsis mutants defective in stomatal regulation. Plant J. 30, 601–609. doi: 10.1046/j.1365-313X.2002.01322.x
Milella, A., Marani, R., Petitti, A., and Reina, G. (2019). In-field high throughput grapevine phenotyping with a consumer-grade depth camera. Comput. Electron. Agric. 156, 293–306. doi: 10.1016/j.compag.2018.11.026
Mittler, R. (2006). Abiotic stress, the field environment and stress combination. Trends Plant Sci. 11, 15–19. doi: 10.1016/j.tplants.2005.11.002
Möller, M., Alchanatis, V., Cohen, Y., Meron, M., Tsipris, J., Naor, A., et al. (2007). Use of thermal and visible imagery for estimating crop water status of irrigated grapevine. J. Exp. Bot. 58, 827–838. doi: 10.1093/jxb/erl115
Moreira, F. F., Oliveira, H. R., Volenec, J. J., Rainey, K. M., and Brito, L. F. (2020). Integrating high-throughput Phenotyping and statistical genomic methods to genetically improve longitudinal traits in crops. Front. Plant Sci. 11:681. doi: 10.3389/fpls.2020.00681
Mullineaux, P. M., Karpinski, S., and Baker, N. R. (2006). Spatial dependence for hydrogen peroxide-directed signaling in light-stressed plants. Plant Physiol. 141, 346–350. doi: 10.1104/pp.106.078162
Naulleau, A., Gary, C., Prévot, L., and Hossard, L. (2021). Evaluating strategies for adaptation to climate change in grapevine production—a systematic review. Front. Plant Sci. 11:607859. doi: 10.3389/fpls.2020.607859
Nogales-Bueno, J., Baca-Bocanegra, B., Rodríguez-Pulido, F. J., Heredia, F. J., and Hernández-Hierro, J. M. (2015). Use of near infrared hyperspectral tools for the screening of extractable polyphenols in red grape skins. Food Chem. 172, 559–564. doi: 10.1016/j.foodchem.2014.09.112
Nuske, S., Achar, S., Gupta, K., Narasimhan, S., and Singh, S. (2011). Visual yield estimation in vineyards: experiments with different varietals and calibration procedures. Carnegie Mellon University. 11-39.
Oakey, H., Verbyla, A., Pitchford, W., Cullis, B., and Kuchel, H. (2006). Joint modeling of additive and non-additive genetic line effects in single field trials. Theor. Appl. Genet. 113, 809–819. doi: 10.1007/s00122-006-0333-z
OIV (2017). Resolution OIV-VITI 564A-2019. International Organisation of Vine and Wine; Process for the clonal selection of vines. Available at: http://www.oiv.int/public/medias/5382/oiv-viti-564a-2017-en.pdf (Accessed August, 2021).
OIV (2019). Resolution OIV-VITI 564B-2019. International Organisation of Vine and Wine; Process for the recovery and conservation of the intravarietal diversity and the polyclonal selection of the vine in grape varieties with wide genetic variability. Available at: http://www.oiv.int/public/medias/6939/oiv-viti-564b-2019-en.pdf (Accessed August, 2021).
OIV (2020). State of the World Vitivinicultural Sector in 2019. International Organisation of Vine and Wine. Available at: http://www.oiv.int/public/medias/7298/oiv-state-of-the-vitivinicultural-sector-in-2019.pdf (Accessed August, 2021).
Ollat, N., Peccoux, A., Papura, D., Esmenjaud, D., Marguerit, E., Tandonnet, J. P., et al. (2016). “Rootstocks as a component of adaptation to environment,” in Grapevine in a changing environment: A molecular and Ecophysiological perspective. 1st Edn. eds. H. Gerós, M. M. Chaves, H. Medrano, and S. Delrot (West Sussex, UK: John Wiley & Sons), 68–108.
Omasa, K., and Takayama, K. (2003). Simultaneous measurement of Stomatal conductance, non-photochemical quenching, and photochemical yield of photosystem II in intact leaves by thermal and chlorophyll fluorescence imaging. Plant Cell Physiol. 44, 1290–1300. doi: 10.1093/pcp/pcg165
Osório, J., Osório, M. L., Correia, P. J., de Varennes, A., and Pestana, M. (2014). Chlorophyll fluorescence imaging as a tool to understand the impact of iron deficiency and resupply on photosynthetic performance of strawberry plants. Sci. Hortic. 165, 148–155. doi: 10.1016/j.scienta.2013.10.042
Pajares, G., García-Santillán, I., Campos, Y., Montalvo, M., Guerrero, J. M., Emmi, L., et al. (2016). Machine-vision systems selection for agricultural vehicles: A guide. J. Imaging 2:34. doi: 10.3390/jimaging2040034
Palacios, F., Diago, M. P., and Tardaguila, J. A. (2019). Non-invasive method based on computer vision for grapevine cluster compactness assessment using a mobile sensing platform under field conditions. Sensors 19:3799. doi: 10.3390/s19173799
Pantin, F., Monnet, F., Jannaud, D., Costa, J. M., Renaud, J., Muller, B., et al. (2013). The dual effect of abscisic acid on stomata. New Phytol. 197, 65–72. doi: 10.1111/nph.12013
Parker, A. K., De Cortázar-Atauri, I. G., Van Leeuwen, C., and Chuine, I. (2011). General phenological model to characterise the timing of flowering and veraison of Vitis vinifera L. Aust. J. Grape Wine Res. 17, 206–216. doi: 10.1111/j.1755-0238.2011.00140.x
Pavlousek, P. (2011). Evaluation of drought tolerance of new grapevine rootstock hybrids. J. Environ. Biol. 32, 543–549.
Petrie, P. R., Wang, Y., Liu, S., Lam, S., Whitty, M. A., and Skewes, M. A. (2019). The accuracy and utility of a low cost thermal camera and smartphone-based system to assess grapevine water status. Biosyst. Eng. 179, 126–139. doi: 10.1016/j.biosystemseng.2019.01.002
Piepho, H. P., and Edmondson, R. N. (2018). A tutorial on the statistical analysis of factorial experiments with qualitative and quantitative treatment factor levels. J. Agron. Crop Sci. 204, 429–455. doi: 10.1111/jac.12267
Piepho, H. P., and Möhring, J. (2007). Computing heritability and selection response from unbalanced plant breeding trials. Genetics 177, 1881–1888. doi: 10.1534/genetics.107.074229
Pieruschka, R., and Schurr, U. (2019). Plant phenotyping: past, present, and future. Plant Phenomics 2019:7507131. doi: 10.34133/2019/7507131
Prinsi, B., Simeoni, F., Galbiati, M., Meggio, F., Tonelli, C., Scienza, A., et al. (2021). Grapevine rootstocks differently affect physiological and molecular responses of the scion under water deficit condition. Agronomy 11:289. doi: 10.3390/agronomy11020289
Qiu, R., Wei, S., Zhang, M., Li, H., Sun, H., Liu, G., et al. (2018). Sensors for measuring plant phenotyping: a review. Int. J. Agric. Biol. Eng. 11, 1–17. doi: 10.25165/j.ijabe.20181102.2696
Raskin, I., and Ladyman, J. A. R. (1988). Isolation and characterization of a barley mutant with abscisic-acid-insensitive stomata. Planta 173, 73–78. doi: 10.1007/BF00394490
Reynolds, D., Baret, F., Welcker, C., Bostrom, A., Ball, J., Cellini, F., et al. (2019). What is cost-efficient phenotyping? Optimizing costs for different scenarios. Plant Sci. 282, 14–22. doi: 10.1016/j.plantsci.2018.06.015
Rienth, M., Vigneron, N., Darriet, P., Sweetman, C., Burbidge, C., Bonghi, C., et al. (2021). Grape berry secondary metabolites and their modulation by abiotic factors in a climate change scenario–A review. Front. Plant Sci. 12:643258. doi: 10.3389/fpls.2021.643258
Roitsch, T., Cabrera-Bosquet, L., Fournier, A., Ghamkhar, K., Jiménez-Berni, J., Pinto, F., et al. (2019). Review: new sensors and data-driven approaches—A path to next generation phenomics. Plant Sci. 282, 2–10. doi: 10.1016/j.plantsci.2019.01.011
Rossdeutsch, L., Edwards, E., Cookson, S. J., Barrieu, F., Gambetta, G. A., Delrot, S., et al. (2016). ABA-mediated responses to water deficit separate grapevine genotypes by their genetic background. BMC Plant Biol. 16:91. doi: 10.1186/s12870-016-0778-4
Sampaio Filho, I. D. J., Jardine, K. J., de Oliveira, R. C. A., Gimenez, B. O., Cobello, L. O., Piva, L. R. D. O., et al. (2018). Below versus above ground plant sources of abscisic acid (ABA) at the heart of tropical forest response to warming. Int. J. Mol. Sci. 19:2023. doi: 10.3390/ijms19072023
Sánchez-Moreiras, A. M., Graña, E., Reigosa, M. J., and Araniti, F. (2020). Imaging of chlorophyll a fluorescence in natural compound-induced stress detection. Front. Plant Sci. 11:583590. doi: 10.3389/fpls.2020.583590
Santesteban, L. G., Miranda, C., Marín, D., Sesma, B., Intrigliolo, D. S., Mirás-Avalos, J. M., et al. (2019). Discrimination ability of leaf and stem water potential at different times of the day through a meta-analysis in grapevine (Vitis vinifera L.). Agric. Water Manag. 221, 202–210. doi: 10.1016/j.agwat.2019.04.020
Santos, J. A., Fraga, H., Malheiro, A. C., Moutinho-Pereira, J., Dinis, L. T., Correia, C., et al. (2020). A review of the potential climate change impacts and adaptation options for European viticulture. Appl. Sci. 10:3092. doi: 10.3390/app10093092
Schiefelbein, J. (2015). Molecular phenotyping of plant single cell-types enhances forward genetic analyses. Front. Plant Sci. 6:509. doi: 10.3389/fpls.2015.00509
Sen, I., Ozturk, B., Tokatli, F., and Ozen, B. (2016). Combination of visible and mid-infrared spectra for the prediction of chemical parameters of wines. Talanta 161, 130–137. doi: 10.1016/j.talanta.2016.08.057
Siebers, M. H., Edwards, E. J., Jimenez-Berni, J. A., Thomas, M. R., Salim, M., et al. (2018). Fast phenomics in vineyards: development of Grover, the grapevine rover, and LiDAR for assessing grapevine traits in the field. Sensors 18:2924. doi: 10.3390/s18092924
Simonneau, T., Lebon, E., Coupel-Ledru, A., Marguerit, E., Rossdeutsch, L., and Ollat, N. (2017). Adapting plant material to face water stress in vineyards: which physiological targets for an optimal control of plant water status? OENO One 51, 167–179. doi: 10.20870/oeno-one.2017.51.2.1870
Sims, D., and Gamon, J. (2002). Relationships between leaf pigment content and spectral reflectance across a wide range of species, leaf structures and developmental stages. Remote Sens. Environ. 81, 337–354. doi: 10.1016/S0034-4257(02)00010-X
Singh, D., Wang, X., Kumar, U., Gao, L., Noor, M., Imtiaz, M., et al. (2019). High-throughput phenotyping enabled genetic dissection of crop lodging in wheat. Front. Plant Sci. 10:394. doi: 10.3389/fpls.2019.00394
Su, L., Dai, Z., Li, S., and Xin, H. (2015). A novel system for evaluating drought—cold tolerance of grapevines using chlorophyll fluorescence. BMC Plant Biol. 15:82. doi: 10.1186/s12870-015-0459-8
Tagarakis, A. C., Koundouras, S., Fountas, S., and Gemtos, T. (2018). Evaluation of the use of LIDAR laser scanner to map pruning wood in vineyards and its potential for management zones delineation. Precis. Agric. 19, 334–347. doi: 10.1007/s11119-017-9519-4
Tagarakis, A. C., Liakos, V., Chatzinikos, T., Koundouras, S., Fountas, S., and Gemtos, T. (2013). Using laser scanner to map pruning wood in vineyards. Precis. Agric. 13, 633–669. doi: 10.3920/978-90-8686-778-3_78
Tattaris, M., Reynolds, M. P., and Chapman, S. C. (2016). A direct comparison of remote sensing approaches for high-throughput phenotyping in plant breeding. Front. Plant Sci. 7:1131. doi: 10.3389/fpls.2016.01131
Tedesco, S., Pina, A., Fevereiro, P., and Kragler, F. (2020). A phenotypic search on graft compatibility in grapevine. Agronomy 10:706. doi: 10.3390/Agron.10050706
Toledo-Martín, E. M., García-García, M. C., Font, R., Moreno-Rojas, J. M., Gómez, P., Salinas-Navarro, M., et al. (2016). Application of visible/near-infrared reflectance spectroscopy for predicting internal and external quality in pepper: estimation of quality in pepper by NIR spectroscopy. J. Sci. Food Agric. 96, 3114–3125. doi: 10.1002/jsfa.7488
Tomás, M., Medrano, H., Brugnoli, E., Escalona, J. M., Martorell, S., Pou, A., et al. (2014). Variability of mesophyll conductance in grapevine cultivars under water stress conditions in relation to leaf anatomy and water use efficiency. Aust. J. Grape Wine Res. 20, 272–280. doi: 10.1111/ajgw.12069
Tsaftaris, S. A., Minervini, M., and Scharr, H. (2016). Machine learning for plant Phenotyping needs image processing. Trends Plant Sci. 21, 989–991. doi: 10.1016/j.tplants.2016.10.002
Tsoulias, N., Paraforos, D. S., Fountas, S., and Zude-Sasse, M. (2019). Calculating the water deficit spatially using LiDAR laser scanner in an apple orchard. Precis. Agric. 19, 115–121. doi: 10.3920/978-90-8686-888-9_13
Udompetaikul, V., Upadhyaya, S. K., Slaughter, D., Lampinen, B., and Shackel, K. (2011). “Plant water stress detection using leaf temperature and microclimatic information,” in American Society of Agricultural and Biological Engineers Annual International Meeting; August 7-10, 2011; Louisville, Kentucky, USA, 198–208.
van Leeuwen, C., and Darriet, P. (2016). The impact of climate change on viticulture and wine quality. J. Wine Econ. 11, 150–167. doi: 10.1017/jwe.2015.21
Van Leeuwen, C., and Destrac-Irvine, A. (2017). Modified grape composition under climate change conditions requires adaptations in the vineyard. OENO One 51, 147–154. doi: 10.20870/oeno-one.2016.0.0.1647
Van Leeuwen, C., Destrac-Irvine, A., Dubernet, M., Duchêne, E., Gowdy, M., Marguerit, E., et al. (2019). An update on the impact of climate change in viticulture and potential adaptations. Agronomy 9:514. doi: 10.3390/Agron.9090514
Van Leeuwen, C., Roby, J. P., Alonso-Villaverde, V., and Gindro, K. (2013). Impact of clonal variability in Vitis vinifera cabernet franc on grape composition, wine quality, leaf blade stilbene content, and downy mildew resistance. J. Agric. Food Chem. 61, 19–24. doi: 10.1021/jf304687c
Verdugo-Vásquez, N., Acevedo-Opazo, C., Valdés-Gómez, H., Ingram, B., García de Cortázar-Atauri, I., and Tisseyre, B. (2020). Towards an empirical model to estimate the spatial variability of grapevine phenology at the within field scale. Precis. Agric. 21, 107–130. doi: 10.1007/s11119-019-09657-7
Victorino, G. F., Braga, R., Santos-Victor, J., and Lopes, C. M. (2020). Yield components detection and image-based indicators for non-invasive grapevine yield prediction at different phenological phases. OENO One 54, 833–848. doi: 10.20870/oeno-one.2020.54.4.3616
Villalobos-Gonzalez, L., Muñoz-Araya, M., Franck, N., and Pastenes, C. (2019). Controversies in midday water potential regulation and stomatal behavior might result from the environment, genotype, and/or rootstock: evidence from Carménère and syrah grapevine varieties. Front. Plant Sci. 10:1522. doi: 10.3389/fpls.2019.01522
Walter, A., Liebisch, F., and Hund, A. (2015). Plant phenotyping: from bean weighing to image analysis. Plant Methods 11:14. doi: 10.1186/s13007-015-0056-8
Welham, S. J., Gogel, B. J., Smith, A. B., Thompson, R., and Cullis, B. R. (2010). A comparison of analysis methods for late-stage variety evaluation trials. Aust. N. Z. J. Stat. 52, 125–149. doi: 10.1111/j.1467-842X.2010.00570.x
Wolkovich, E. M., García De Cortázar-Atauri, I., Morales-Castilla, I., Nicholas, K. A., and Lacombe, T. (2018). From pinot to Xinomavro in the world’s future wine-growing regions. Nat. Clim. Chang. 8, 29–37. doi: 10.1038/s41558-017-0016-6
Xue, J., and Su, B. (2017). Significant remote sensing vegetation indices: a review of developments and applications. J. Sens. 2017, 1–17. doi: 10.1155/2017/1353691
Yang, W., Feng, H., Zhang, X., Zhang, J., Doonan, J. H., Batchelor, W. D., et al. (2020). Crop phenomics and high-throughput phenotyping: past decades, current challenges, and future perspectives. Mol. Plant 13, 187–214. doi: 10.1016/j.molp.2020.01.008
Zarrouk, O., Brunetti, C., Egipto, R., Pinheiro, C., Genebra, T., Gori, A., et al. (2016). Grape ripening is regulated by deficit irrigation/elevated temperatures according to cluster position in the canopy. Front. Plant Sci. 7:1640. doi: 10.3389/fpls.2016.01640
Zheng, X., Krause, J., Fischer, B., Gruna, R., Topfer, R., and Kicherer, A. (2021). “Phenoliner2.0: RGB and near-infrared (NIR) image acquisition for an efficient phenotyping in grapevine research,” in Proceedings of the 5th International Conference On Optical Characterization of Materials. eds. J. Beyerer and T. Längle; March 16, 2021 (Scientific Publishing: Karlsruhe, Germany), 57–67.
Keywords: heat and water stress, imaging, phenotyping planning, planting material, selection traits, Vitis vinifera
Citation: Carvalho LC, Gonçalves EF, Marques da Silva J and Costa JM (2021) Potential Phenotyping Methodologies to Assess Inter- and Intravarietal Variability and to Select Grapevine Genotypes Tolerant to Abiotic Stress. Front. Plant Sci. 12:718202. doi: 10.3389/fpls.2021.718202
Edited by:
Tommaso Frioni, Catholic University of the Sacred Heart, ItalyReviewed by:
Markus Rienth, University of Applied Sciences and Arts of Western Switzerland, SwitzerlandGastón Gutiérrez Gamboa, Universidad Mayor, Chile
Copyright © 2021 Carvalho, Gonçalves, Marques da Silva and Costa. This is an open-access article distributed under the terms of the Creative Commons Attribution License (CC BY). The use, distribution or reproduction in other forums is permitted, provided the original author(s) and the copyright owner(s) are credited and that the original publication in this journal is cited, in accordance with accepted academic practice. No use, distribution or reproduction is permitted which does not comply with these terms.
*Correspondence: Luísa C. Carvalho, bGNhcnZhbGhvQGlzYS51bGlzYm9hLnB0; J. Miguel Costa, bWlndWVsY29zdGFAaXNhLnVsaXNib2EucHQ=