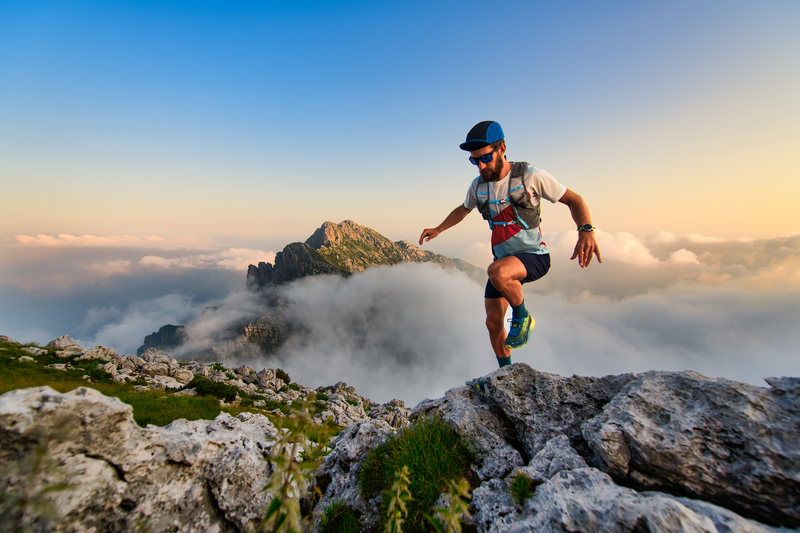
94% of researchers rate our articles as excellent or good
Learn more about the work of our research integrity team to safeguard the quality of each article we publish.
Find out more
ORIGINAL RESEARCH article
Front. Plant Sci. , 17 August 2021
Sec. Plant Breeding
Volume 12 - 2021 | https://doi.org/10.3389/fpls.2021.713890
As an important component, 1,000 kernel weight (TKW) plays a significant role in the formation of yield traits of wheat. Kernel size is significantly positively correlated to TKW. Although numerous loci for kernel size in wheat have been reported, our knowledge on loci for kernel area (KA) and kernel circumference (KC) remains limited. In the present study, a recombinant inbred lines (RIL) population containing 371 lines genotyped using the Wheat55K SNP array was used to map quantitative trait loci (QTLs) controlling the KA and KC in multiple environments. A total of 54 and 44 QTLs were mapped by using the biparental population or multienvironment trial module of the inclusive composite interval mapping method, respectively. Twenty-two QTLs were considered major QTLs. BLAST analysis showed that major and stable QTLs QKc.sau-6A.1 (23.12–31.64 cM on 6A) for KC and QKa.sau-6A.2 (66.00–66.57 cM on 6A) for KA were likely novel QTLs, which explained 22.25 and 20.34% of the phenotypic variation on average in the 3 year experiments, respectively. Two Kompetitive allele-specific PCR (KASP) markers, KASP-AX-109894590 and KASP-AX-109380327, were developed and tightly linked to QKc.sau-6A.1 and QKa.sau-6A.2, respectively, and the genetic effects of the different genotypes in the RIL population were successfully confirmed. Furthermore, in the interval where QKa.sau-6A.2 was located on Chinese Spring and T. Turgidum ssp. dicoccoides reference genomes, only 11 genes were found. In addition, digenic epistatic QTLs also showed a significant influence on KC and KA. Altogether, the results revealed the genetic basis of KA and KC and will be useful for the marker-assisted selection of lines with different kernel sizes, laying the foundation for the fine mapping and cloning of the gene(s) underlying the stable QTLs detected in this study.
Common wheat (Triticum aestivum L.) is one of the most important food crops in the world and provides ~20% of the protein and calories in the human diet (Chaves et al., 2013). In recent years, due to the decrease in arable land area, population growth, and many other factors, it has been difficult for grain production to meet human demand. It is very important to increase the yield of common wheat to relieve the pressure on grain and meet social demand.
The 1,000 kernel weight (TKW) and kernel number per spike (KN) have important effects on the wheat yield, and they are usually considered key factors of yield formation. Previous studies showed that compared with the KN, the TKW might have a higher heritability, with a heritability range of 0.59–0.80 (Xiao and He, 2003). The kernel size is significantly positively correlated with the TKW. Therefore, in the long history of wheat breeding and domestication, the kernel size has been a major selection and breeding objective and has been widely used for selection to improve wheat yield (Gegas et al., 2010). Larger seeds usually showed a better yield and commerciality. The genetic improvement of kernel size is beneficial to increase the TKW and thus increase the yield of common wheat.
The kernel traits of wheat are complex agronomic traits and are controlled by multiple genes (Li et al., 2018). In previous studies, several quantitative trait loci (QTLs) or genes related to kernel traits have been identified and mapped, which were distributed on all of the chromosomes of common wheat (Gegas et al., 2010; Prashant et al., 2012; Tyagi et al., 2015; Kumar et al., 2016; Brinton et al., 2017; Yan et al., 2017; Li et al., 2018; Ma et al., 2019a; Cao et al., 2020; Liu et al., 2020). For instance, Tyagi et al. (2015) mapped QTLs related to kernel traits on 19 wheat chromosomes, except for the 2D and 3D chromosomes. Ma et al. (2019a) mapped a major QTL on a 2D chromosome that controls the kernel length (KL), kernel width (KW), and TKW. Liu et al. (2020) identified QTLs related to the TKW in the DH population and distributed them to wheat 1A, 2D, 4B, 4D, 5A, 5D, 6A, and 6D chromosomes. Several studies also indicated that a QTL across the dwarf gene Rht-B1 on 4BS could affect both the KW and TKW (Ramya et al., 2010; Gao et al., 2015; Li et al., 2018; Ren et al., 2021). Although the cloning of related genes in common wheat is relatively slow due to its large genome (approximately 17.9 Gb) and high repeat sequence content compared with rice and maize (Simmonds et al., 2014; Su et al., 2018), to date, many genes related to the kernel traits of wheat have been cloned and verified. For instance, TaGW2 on 6A was identified as a TKW QTL in a mapping population (Simmonds et al., 2014), and then the analysis of the haplotype structure of the 6A chromosome explains that the QTL region detected for KC and KA is different from the TKW QTL on 6A (Brinton et al., 2020). Moreover, TaGW2 and TaGS5 were also cloned based on their homologous genes OsGW2 and OsGS5 in rice, respectively (Wang et al., 2016; Zhai et al., 2018). TaMOC1-7A was cloned based on the MOC1 gene in rice, and it was found that it could affect both the TKW and number of spikelets (Zhang et al., 2015). Several genes related to the TKW, such as TaSnRK2.3-1A (Miao et al., 2017), TaSnRK2.3-1B (Miao et al., 2017), TaSUS2-2A (Hou et al., 2014), TaCwi-A1 (Ma et al., 2012), TaFlo2-A1 (Sajjad et al., 2017), TaSUS2-2B (Jiang et al., 2011), and Tabas1-B1 (Zhu et al., 2016), were also cloned. More QTL mapping of kernel traits is ongoing, which laid a foundation for future gene cloning and application. Although numerous loci for the kernel traits in wheat have been reported, our knowledge on loci for the kernel area (KA) and kernel circumference (KC) remains limited (Kumari et al., 2018). Moreover, although several QTLs for the TKW, KL, and KW have been reported, most of them showed strong QTL × genotype and epistatic QTL × QTL interactions, which limited their further application in molecular marker-assisted breeding (MAS) (Campbell et al., 2003; Gupta et al., 2007; Prashant et al., 2012; Cabral et al., 2018).
Genetic linkage maps play an important role in the analysis of genetic components of agronomic traits. The large genetic distance between QTLs and flanking molecular markers often limits their application in MAS. With the development of high-throughput sequencing technology, high-density genetic linkage maps have been increasingly widely used in the study of various crops, such as rice (Xie et al., 2010), maize (Chen et al., 2014), eggplants (Barchi et al., 2012), and grapes (Wang et al., 2012). In recent years, high-density genetic linkage maps based on SNP micro-arrays have played an important role and have been widely used for QTL mapping for various agronomic traits in common wheat (Cui et al., 2017; Sun et al., 2020). Due to its economy and practicability, the Wheat55K SNP array has been employed for many gene mapping studies (Li et al., 2018; Ren et al., 2018; Huang et al., 2019; Ma et al., 2019b; Zhang et al., 2019).
In the present study, QTLs for KA and KC were identified using a genetic map constructed with the Wheat55K SNP array and phenotypic observations in multiple environments. Major QTL for KA and KC were further validated in the RIL population by KASP markers. The major and stable QTLs detected in this study will be useful for further MAS and map-based cloning.
The elite wheat cultivar Chuannong18, which was released by the Sichuan Provincial Variety Examination and Committee in 2003, has been planted in southwestern China on nearly one million hectares. T1208 is a high-yield wheat line developed by our laboratory. In the present study, a 371 RIL population developed by the cross Chuannong18 (CN18) and T1208 was used for QTL analyses based on a high-density genetic linkage map constructed by the Wheat55K SNP array (Hu et al., 2017; Ren et al., 2018, 2021).
The 371 lines of the RIL population and the two parents were planted in the Qionglai District, Chengdu Plain, Sichuan Province, China (30°250'N, 103°28'E, altitude 493.3 m) in the 2016, 2018, and 2019 cropping seasons. A randomized complete block design with three replications was used for the field experiment. Each plot was 3 m long and consisted of four rows with a 25 cm spacing between rows. The plant density was 160 seedlings per square meter. Cultivation management was the same as the field standardized management method in the Chengdu Plain. Herbicides and fungicides were applied to ensure that there were no pests or diseases in the field during the growth process and to avoid influencing the corresponding agronomic traits. One square meter from the central part of each plot was harvested to determine the kernel traits. Kernels were based on 12% moisture when measured (seeds were dried and measured by a grain moisture tester, PM-8188, Kett, Tokyo, Japan). The KA and KC were measured by a Wanshen SC-G automatic seed test analyzer (Hangzhou Wanshen Testing Technology Co., Ltd.). During the measurement, more than 100 seeds of each line were scanned for analysis. After scanning and taking pictures, each seed would be circled and the area of each seed and the circumference of each seed would be calculated by software. This method provides new parameters to determine the size of the kernels. And the TKW, KL, KW, kernel diameter ratio (KDR), and kernel weight per spike (KWPS) of each line were also calculated by the seed test analyzer, and the plant height (PH) was measured directly in the field during the filling stage. Those data were retrieved from our previous studies (Ren et al., 2021, Supplementary Table 1).
The average measurement of each trait of three replicates was used in the subsequent analysis. The heritability and ANOVA of each trait were performed as described previously (Hu et al., 2017; Ren et al., 2021). The Pearson's correlation coefficient was calculated using SPSS Ver. 22.0 (IBM SPSS, Armonk, NY, United States). For each trait, the best linear unbiased estimation (BLUE) was calculated across environments using the ANOVA function in IciMapping Ver4.1 assuming fixed effects for the genotype (Lin et al., 2020).
A high-density genetic linkage map constructed previously using the Wheat55K SNP array was used in this study (Ren et al., 2021). In the RIL population, the markers were distributed in 21 linkage groups and covered a total genetic distance of 4192.62 cM with mean, minimum, and maximum marker densities of 0.36, 0.13, and 1.01 cM between adjacent markers, respectively (Ren et al., 2021). We used IciMapping 4.1 based on the biparental populations (BIPs) module with inclusive composite interval mapping (ICIM, http://www.isbreeding.net) (Meng et al., 2015) for QTL detection in the RIL population. The values of the KA and KC in each replicate of different years were assembled to conduct combined QTL analysis to identify the combined QTLs with additive-by-environment (A by E) interactive effects in an MET module using the ICIM method (Meng et al., 2015). The parameters of QTL analysis were set as follows: logarithm of odds (LOD) = 1,000 permutations, step = 1 cM, and PIN = 0.001. The CI of each QTL was determined by LOD > 3. An epistatic analysis was also performed by the IciMappingVer.4.1 EPI (epistatic) module, and the default parameter settings were used (LOD = 5, step = 1 cM, and stepwise regression probability < 0.0001) (Meng et al., 2015; Ren et al., 2021). QTLs were named based on the International Rules of Genetic Nomenclature (http://wheat.pw.usda.gov/ggpag es/wgc/98/Intro.htm). “Kc” represents the kernel circumference, “Ka” represents the kernel area, and “Sau” represents the Sichuan Agricultural University.
The flanking molecular markers of QTLs were compared with the Chinese Spring genome reference sequence [International Wheat Genome Sequencing Consortium (IWGSC) RefSeq version 1.0; https://urgi.versailles.inra.fr/download/iwgsc/] and T. Turgidum ssp. dicoccoides genome reference sequence (The Triticeae Multiomics Center, http://202.194.139.32/). After the physical locations of the QTL region were determined, the information of genes or sequences of the regions was downloaded from Wheatmine (https://urgi.versailles.inra.fr/WheatMine/begin.do). Finally, sequence alignment was performed, and the functions of the genes or sequences involved in these QTL regions were analyzed on the UniProt website (http://www.UniProt.org/).
By finding the sequence of the Wheat55K SNP molecular markers located in the QTL interval, the KASP primers were designed on the PolyMarker online website (http://www.polymarker.info/). A set of one KASP primer contained a total of three primer sequences: forward primer 1, forward primer 2, and a universal primer. The 5′ end of the forward primer 1 needs to add the FAM fluorophore group (5′-GAAGGTGACCAAGTTCATGCT- 3′), and the 5' end of the forward primer 2 needs to add the HEX fluorophore group (5′- GAAGGTCGGAGTCAACGGATT- 3′). All of the sequences of KASP primers designed in this experiment are listed in Table 4. A total of 127 lines for the KC and 128 lines for the KA were randomly selected from the RIL populations and used to perform genotyping using these KASP markers.
The PCRs were performed in a total volume of 10 μl containing 4.5 μl of 1×KASP Master mixture (JasonGen, www.jasongen.com), 2 μl (50 ng/μl) of template DNA, 2 μl of KASP primer mixture (forward primer 1:forward primer 2: universal primer: ddH2O = 6:6:15:23), and 1.5 μl of ddH2O. The whole process was carried out on real-time PCR (BioRad, CFX-96) system. The PCR procedure was as follows: 10 min at 95°C and 30 cycles of 20 s at 95°C and 40 s at 61–55°C (drop 0.6°C, per cycle).
The KC and KA values of CN18 were lower than those of T1208 in the phenotypic data of the 3 year experiments (Table 1, Figure 1, Supplementary Table 2). The KC values of CN18 and T1208 were in the ranges of 16.97–18.13 and 17.46–19.02 mm in the 3 years of data, respectively. The KA values of CN18 and T1208 were in the ranges of 17.55–19.00 mm2 and 17.71–20.64 mm2 in the 3 years of data, respectively. In the RIL population, the KC and KA showed continuous variation, and both had the phenomenon of transgressive inheritance (Table 1, Figure 1). The absolute value of skewness and kurtosis of the phenotypic distribution in the population was <1 (Table 1), which is consistent with a normal distribution and is a typical quantitative trait (Figure 1). Moreover, the KA and KC exhibited a high h2 (broad-sense heritability); the h2 of KA was 0.92, and the h2 of KC was 0.88. The coefficient of variation (CV) of KC was lower than that of KA, which suggested that KA had a higher degree of variation. A significant positive correlation was observed between the KC and KA in all the three environments (Table 2). It was also indicated that these two kernel-size traits are mainly affected by genetic factors, while environmental factors have less influence.
Table 1. Phenotypic variation and heritability of characters in different environments in parents and populations.
Figure 1. Phenotypic frequency distribution and box plot of kernel size in RIL Population. C, CN18; T, T1208.
Using the ICIM–BIP method, 54 additive QTLs for the KC and KA were detected, and these QTLs were mapped to chromosomes 1A, 2A, 2B, 2D, 3D, 4A, 4B, 4D, 5A, 5B, 5D, 6A, 6B, 6D, 7A, 7B, and 7D (Supplementary Table 3). Among them, 22 QTLs that were detected during more than 1 year (or in the mean data) or explained >10% of the phenotypic variation and had an LOD > 3 were considered major QTLs and are listed in Table 3.
Thirty KC QTLs were mapped (Table 3, Supplementary Table 3, and Figure 2). The LOD of each QTL ranged from 4.22 to 42.65, and each QTL explained 1.28–34.68% of the KC variation. The additive effect values of 16 QTLs were negative, indicating that the genetic effects of these QTLs were derived from CN18. The other 14 QTLs were derived from T1208 because their additive effect values were positive (Supplementary Table 3). Among them, 11 QTLs were considered as major QTLs. QKc.sau.6A.1 was mapped in the interval of the 6A chromosome (23.12-31.64 cM) in 3 years and explained 9.77, 29.73, and 34.68% of the phenotypic variation, respectively (Table 3). The additive effect values of QKc.sau.6A.1 were positive, indicating that the genetic effects of QKc.sau.6A.1 were derived from T1208. Moreover, QKc.sau-5A.1 (56.75–80.36 cM on 5A) and QKc.sau-5B.2 (162.79–163.07 cM on 5B) were mapped in 2 years and explained 2.8–8.31% and 4.33–6.47% of the phenotypic variation, respectively (Supplementary Table 3, Table 3).
Figure 2. The additive QTLs for kernel area (KA) and kernel circumference (KC) in the genetic map. Red for KC and green for KA. Thirty KC and 24 KA QTLs were mapped to chromosomes 1A, 2A, 2B, 2D, 3D, 4A, 4B, 4D, 5A, 5B, 5D, 6A, 6B, 6D, 7A, 7B, and 7D, respectively. The major and stable QTLs QKc.sau.6A.1 (23.12–31.64 cM, closet marker: AX-109894590) and QKa.sau.6A.2 (66.00–66.57 cM, closet marker: AX-108852271) were mapped on 6A chromosome. The positions, interval, and closet markers of other QTLs are listed in Table 3. The scale label of the linkage map is 2 cM, which is showed in the left.
Twenty-four KA QTLs were also mapped (Table 3, Supplementary Table 3, and Figure 2). The LOD of each QTL ranged from 3.28 to 47.61, and each QTL explained 1.29–23.40% of the KA variation. Eleven QTLs and 13 QTLs were derived from CN18 or T1208, respectively, due to their additive effect values (Supplementary Table 3). Among them, 11 QTLs were considered major QTLs. QKa.sau-6A.2 was mapped in the interval of the 6A chromosome (66.00–66.57 cM) in all the 3 years and explained 20.99, 21.86, and 15.12% of the phenotypic variation, respectively (Table 3). The additive effect values of QKa.sau.6A.2 were negative, indicating that the genetic effects of QKa.sau.6A.2 were derived from CN18. In addition, QKa.sau-4B (67.53–72.39 cM on 4B), QKa.sau-4D.1 (94.86–123.09 cM on 4B), QKa.sau-5B (150.91–151.20 cM on 5B), QKa.sau-5D.1 (91.93–101.11 cM on 5D), QKa.sau-6A.1 (16.78–34.64 cM on 6A), and QKa.sau-6D.3 (218.76–219.04 cM on 6A) were mapped in 2 years and explained 2.17–4.44%, 1.29–5.24%, 3.44–5.44%, 2.34–5.57%, 11.98–16.22%, and 13.97–15.08% of the phenotypic variation, respectively (Supplementary Table 3, Table 3).
Using the MET method of ICIM, 24 cQTLs of the KC, and 20 cQTLs of the KA were mapped (Supplementary Table 4).
In the MET analysis, 20 cQTLs of the KC and 17 cQTLs of the KA were the same as the QTL analysis by the BIP method. The major QTLs that could be mapped for more than 2 years (three for KC and eight for KA) by the ICIM–BIP method were also mapped by the MET method (Supplementary Table 4, Table 3). Among these cQTLs for the KC and KA, PVE (A) (representing phenotypic variation explained by additive and dominance effects) ranged from 0.26 to 37.33% and 0.24 to 29.20%, respectively. The PVE (A by E) (additive and dominance by environment effects for corresponding QTLs) were in the ranges of 0.26–4.44% and 0.09–5.22%, respectively. The value of PVE (A by E) was significantly lower than the value of PVE (A). This indicates that both the KA and KC are mainly influenced by genetic factors.
The results also showed that the phenotypic variation of KC explained by the interactive effects of the environment and cQKc.sau-6A.1 was small [PVE (A by E) = 1.00%], while the phenotypic variation explained by additive and dominance effects was high [PVE(A) = 37.33%]. This indicates that QKc.sau-6A.1 is a stable and major QTL for KC. On the other hand, the PVE (A by E) of cQKa.sau-6A.2 was only 0.13%, and the PVE (A) was 29.20%. This indicates that QKa.sau-6A.2 is a stable and major QTL for KA.
A total of nine digenic epistatic QTLs of KC and four digenic epistatic QTLs of KA were mapped (Supplementary Table 5, Figure 3) and explained 8.30–21.28% and 5.25–14.36% of the phenotypic variation, respectively (Supplementary Table 5).
Figure 3. Chromosomal locations of digenic epistatic QTL for KC (left) and KA (right). A total of nine digenic epistatic QTLs of KC and four digenic epistatic QTLs of KA were mapped, respectively.
The negative epistatic effect values (add by add) suggest that the epistatic effect of the recombinant genotype was higher than that of the parental genotype. The epistatic effect values (add by add) of four KC QTLs were negative, and five of them were positive (Supplementary Table 5). This result suggested that both the recombinant genotype and the parental genotype have epistatic effects on the KC. In contrast, the epistatic effect values (add by add) of all the four QTLs for KA were positive. This result suggested that the epistatic effect of the recombinant genotype was smaller than that of the parental genotype on the KA (Supplementary Table 5).
Among these epistatic QTLs, eQKc.sau-6A corresponded to QKc.sau-6A.2, which was mapped by the BIP method. Digenetic epistatic additive effects were found between eQKc.sau-6A and two loci (eQKc.sau-1BL and eQKc.sau-4D.2) and explained 8.52 and 8.91% of the phenotypic variation, respectively (Supplementary Table 5). It is suggested that the KC is influenced by both additive and dominant effects and epistatic effects. On the other hand, the KA is mainly affected by the interaction between random loci. These random loci indirectly influence the phenotype through interactions with each other. Moreover, digenetic epistatic additive effects were found between eQKc.sau-4D.1 and eQKc.sau-2B.2 for the KC, which explained 21.28% of the phenotypic variation but were only detected in 1 year. And digenetic epistatic additive effects were found between eQKa.sau-7D.1 and eQKa.sau-4D for the KA, which explained 14.36% of the phenotypic variation and were detected in 2016 and 2018 (Supplementary Table 5). It is suggested that although epistasis plays a large role in phenotypes, it is not stable.
Based on the QTL mapping results and sequences of the molecular markers of SNP array, two KASP markers were developed and used to validate two stable QTLs. The KASP marker KASP-AX-109894590 was closely linked to QKc.sau-6A.1, and KASP-AX-109380327 was closely linked to QKa.sau-6A.2 (Table 4). These KASP markers were used to identify the genotype. For QKc.sau-6A.1, KASP-AX-109894590 was used to identify the alleles in the RIL population and were classified into two groups. A total of 127 lines were randomly selected from the RIL population for genotyping using KASP-AX-109894590 molecular markers. Among the 127 lines, 33 lines had the same genotype as CN18, while 94 lines had the same genotype as T1208 (Figure 5, Figures 4A–C). The genotype “AA” shows that QKc.sau-6A.1 carried the homozygous alleles from T1208. And the genotype “aa” shows that QKc.sau-6A.1 carried the homozygous alleles from non-T1208. The results of the t-test showed that the “AA” genotypes had higher phenotypic values of KC in all environments and in the mean values than the “aa” genotypes (P < 0.001) (Figure 5A). For QKa.sau-6A.2, KASP-AX-109380327 was used to identify the alleles in the RIL population and were also classified into two groups. A total of 128 lines were randomly selected from the RIL population for genotyping using KASP-AX-109380327 molecular markers. Among the 128 lines, 49 lines had the same genotype as CN18, while 79 lines had the same genotype as T1208 (Figure 5, Figures 4D–F). The genotype “BB” shows that QKa.sau-6A.2 carried the homozygous alleles from CN18. And the genotype “bb,” shows that QKa.sau-6A.2 carried the homozygous alleles from non-CN18. The results of the t-test showed that the “BB” genotypes had lower phenotypic values of KA in all environments and in the mean values than the “bb” genotypes (P < 0.001) (Figure 5B).
Figure 4. Genotyping results of KASP markers related to KC in RIL population (A–C) and KA in RIL population (D–F). KASP-AX-109894590 (For QKc.sau-6A.1) and KASP-AX-109894590 (QKa.sau-6A.2) were used to identify the alleles in the RIL population and were classified into two groups.
Figure 5. Effects of QKc.sau-6A.1(A) and QKa.sau-6A.2 (B) in RIL population. “+” Indicates the lines with the genotypes “AA” or “BB,” “–” indicates the lines without the genotypes “aa” or “bb.” *, **, and ***, significant at P < 0.05, 0.01, and 0.001, respectively.
Based on the genotyping results of KASP-AX-109894590 and KASP-AX-109380327 molecular markers and the BLUE value of each yield trait, the effects of QKc.sau-6A.1 and QKa.sau-6A.2 on the other six yield traits were analyzed. The results showed that “AA” genotypes had significant positive effects on the PH, TKW, KL, KDR, and KWPS (P < 0.05) and had no significant effects on the KW (Table 5). On the other hand, “BB” genotypes had no significant effects on the PH and KW and had significant negative effects on the TKW, KL, KDR, and KWPS (P < 0.05) (Table 5). To further analyze the relationship between the TKW and these two QTLs, a total of 86 lines were randomly selected from the RIL population for analysis. Among these 86 lines, 40 lines were “AAbb” genotypes, 30 lines were “AABB” or “aabb” genotypes, and 16 lines were “aaBB” genotypes. The TKW of the “AABB” or “aabb” genotype lines was significantly higher than that of the “aaBB” genotype lines (7.98%). The TKW of the “AAbb” genotype lines were significantly higher than those of the “aaBB” genotype lines (9.79%). However, there were no significant differences between the “AAbb” genotype lines and the “AABB” or “aabb” genotype lines (Figure 6). The QTL QKc.sau-6A.1 showed better effects on the TKW than the QTL QKa.sau-6A.2.
Figure 6. Number of favorable alleles for two kernel-related QTLs on thousand grain weight in the Chuannong18/T1208 RIL population based on the across-environment BLUEs. ***Significant at P < 0.001. Genotype of 40 lines were AAbb, 30 lines were AABB or aabb, and 16 lines were aaBB. The TKW of the AAbb, AABB, and aabb genotype lines was significantly higher than that of the aaBB genotype lines.
The major and stable QTL QKa.sau-6A.2, which was related to the KA, was mapped on the 6A chromosome from 66.00 to 66.57 cM. The flanking molecular markers of QKa.sau-6A.2 were compared with the Chinese Spring and T. Turgidum ssp. dicoccoides genome reference sequences. The interval region of this QTL was only 0.57 cM. The two flanking molecular markers of QKa.sau-6A.2 (AX-108852271 and AX111808526) were located at 73.09–73.80 Mb of the physical map of the Chinese Spring genome reference sequence (73095235, 73803389) and 72.06–72.74 Mb of the physical map of the T. Turgidum ssp. dicoccoides genome reference sequence (72058928, 72735090) (Figure 7). A total of 15 or 19 genes were included in this region in Chinese Spring and T. Turgidum ssp. dicoccoides, respectively, and 11 of them were the same (Supplementary Table 6, Figure 7).
Figure 7. The physical position for QKa.sau-6A.2 and its comparison with previous reported QTLs for kernel size. The two flanking molecular markers of QKa.sau-6A.2 (AX-108852271 and AX111808526) were located at 73.09–73.80 Mb of the physical map of the Chinese Spring genome reference sequence (73095235, 73803389) and 72.06–72.74 Mb of the physical map of the T. Turgidum ssp. dicoccoides genome reference sequence (72058928, 72735090). A total of 15 or 19 genes were included in this region in Chinese Spring and T. Turgidum ssp. dicoccoides, respectively.
The yield of wheat is a very complex agronomic trait that is a quantitative trait and controlled by multiple genes (Heidari et al., 2011). Among the yield-related traits of wheat, such as the flowering time, spike numbers per unit area, and number of kernels per spike, these traits tend to have lower heritability than the TKW and are more affected by environmental factors than the TKW (Xiao and He, 2003; Liu et al., 2020). The TKW is positively correlated with the wheat yield and is significantly affected by the kernel size. Kernel size-related traits are also controlled by multiple genes (Li et al., 2018; Yan et al., 2019; Yu et al., 2019). However, many studies on kernel size–related traits usually focused on the KL or KW (Bednarek et al., 2012; Mohler et al., 2016; Zhang et al., 2017; Ma et al., 2019a; Yang et al., 2019; Ren et al., 2021). In this study, to identify more QTLs or genes related to kernel size, we used scanning instruments combined with software analysis to carry out QTL mapping for KA and KC. The traditional methods for measuring the kernel size focused more on the KL and KW, and they revealed that KL and KW had a significant correlation with the TKW. However, in this study, compared with the KL and KW, the KC and KA showed a better coefficient of correlation with the TKW (Table 6). It is suggested that the KA or KC might be better parameters to reflect kernel size. However, KC/KA and TKW were controlled by different QTLs/genes in the wheat genome. For instance, Brinton et al. (2020) indicated that the QTLs for KC and KA were different from the TKW-controlling gene TaGW2. In this study, we mapped the two most stable and major QTLs for the KC and KA, namely, QKc.sau-6A.1 and QKa.sau-6A.2, which were detected in multiple environments. The average LOD values of these two QTLs were as high as 30.11 and 39.56, while they could explain 22.25 and 20.34% of the phenotypic variation, respectively (Table 3). These two QTLs for KA and KC were also located on the different regions of the QTLs for TKW (Ren et al., 2021). It is indicated that, QKc.sau-6A.1 and QKa.sau-6A.2 were different from the QTLs for TKW.
Previous studies have mapped several QTLs also related to the KA and KC (Xiao et al., 2011; Tyagi et al., 2015; Zhao et al., 2015; Yan et al., 2017; Kumari et al., 2018). However, we did not find the same QTL loci as QKc.sau-6A.1 and QKa.sau-6A.2 by comparing the physical locations of the molecular markers flanking both of them on the Chinese Spring reference genome. In addition, we compared the genes controlling kernel size on the 6A chromosome, such as TaGW2-6A (Bednarek et al., 2012) and TaTPP-6AL1 (Zhang et al., 2017), but their physical locations were different from the two QTLs mapped in this study. It is suggested that QKc.sau-6A.1 and QKa.sau-6A.2 were two new stable and major QTLs. In addition, QKc.sau-2A, QKc.sau-2B, and QKa.sau-2A.1 were consistent with the QTLs reported by Xin et al. (2020), which explained 5.01, 3.38, and 2.26% of the variation in this study, respectively. QKc.sau-7B.2 and QKa.sau-6D.3 were consistent with the QTLs reported by Xiao et al. (2011), which explained 4.22 and 14.45% of the variation in this study, respectively.
Previous studies showed that epistasis plays an important role in the inheritance of complex quantitative traits (Cao et al., 2001; Liao et al., 2001; Luo et al., 2001). In this study, the epistatic effects of the KC and KA were analyzed by using the ICIM method, and nine pairs and four pairs of digenic epistatic QTLs were detected, respectively. The results of epistatic analysis showed that there were interactions between one locus and several other loci in the detected epistatic QTLs. For instance, eQKc.sau-2B.1 had epistatic effects with eQKc.sau-2B.3 and eQKc.sau-2D, and eQKa.sau-4A had epistatic effects with eQKa.sau-7B and eQKa.sau-7D.2 (Figure 3, Supplementary Table 5). However, their explained phenotypic variation values were different, indicating that the same locus had different epistatic effects on other loci. In addition, there were interactions between additive QTLs and random loci (Supplementary Table 5). It is also suggested that additive QTL not only directly affects phenotypic traits but also interacts with other loci to indirectly affect phenotypic traits. Several epistasis QTLs, such as eQKc.sau-4D.1 and eQKa.sau-7D.1, showed high PVE for the phenotypic variation (Supplementary Table 5). The PVE of six pairs of digenic epistasis QTLs for KC was higher than 10%, and the other three were also close to 10%. It is suggested that KC is more susceptible to epistasis. However, most epistatic QTLs were only detected in 1 year, only few epistatic QTLs, such as eQKa.sau-4D and eQKa.sau-7D.1 were detected more than 1 year (Supplementary Table 5). It is suggested that in addition to single additive QTLs, some epistatic QTLs also played important roles for KC, although they were not stable in every year.
Compared with traditional techniques, MAS is more accurate and more efficient, and it can usually greatly shorten the breeding period and reduce costs (Kuchel et al., 2007; Gupta et al., 2010). Therefore, in recent years, more attention has been given to the combination of MAS and traditional breeding technology, which may become a new breakthrough in crop breeding programs (Kuchel et al., 2007; Gupta et al., 2010). The rapid development of genome sequence techniques and QTL mapping methods has provided a powerful tool for the study of complex quantitative traits (Sun et al., 2020). A large number of QTLs and genes that affect yield, agronomy, quality, and biological and abiotic resistance have been identified by means of genome-wide linkage maps of molecular markers (Ramya et al., 2010; Simmonds et al., 2014; Gao et al., 2015; Yan et al., 2017; Li et al., 2018; Su et al., 2018; Ma et al., 2019a; Cao et al., 2020; Liu et al., 2020; Ren et al., 2021). KASP technology plays an important role in QTL mapping in wheat (Tan et al., 2017). The principle KASP is based on the reading of terminal fluorescence signals for judgment. Each reaction uses dual-color fluorescence to detect two genotypes of SNP sites, and different SNPs correspond to different fluorescent signals. KASP technology does not require the synthesis of specific fluorescent primers for each SNP site. Based on the unique ARM PCR principle, all SNP sites can be detected by universal fluorescent primer amplification (Semagn et al., 2014). KASP technology has the advantage of low cost and high accuracy, and thus, it has good application potential in wheat research (Allen et al., 2013; Hu et al., 2019).
In this study, two KASP markers, KASP-AX-109894590 and KASP-AX-109380327, were developed and closely linked to QKc.sau-6A.1 and QKa.sau-6A.2, respectively (Table 4). These two KASP markers were used to genotype more than 100 lines randomly selected from the RIL population. These two markers can genotype different alleles in the population (Figure 4). All of the lines having the same fluorescent signal as T1208 when tested by KASP-AX-109894590 indicated that these lines carried the homozygous alleles “AA” from T1208, and these lines exhibited significantly higher KC and TKW (Figures 5, 6, Table 5). On the other hand, all of the lines having the same fluorescent signal as CN18 when tested by KASP-AX-109380327 indicated that these lines carried the homozygous alleles “BB” from CN18, and these lines exhibited significantly lower KA and TKW (Figures 5, 6, Table 5). These results indicated that these two QTLs located on the 6A chromosome were validated. The major QTLs QKc.sau-6A.1 and QKa.sau-6A.2 that were mapped in this study might have an important influence on the heredity of kernel-related traits, and the KASP markers closely linked to them could be used for MAS in future breeding programs.
Fifteen candidate genes were mapped in the region from 73.09 to 73.80 Mb on chromosome 6AS of wheat, and 19 genes were mapped in the region from 72.06 to 72.74 Mb on chromosome 6AS of wild emmer wheat (Figure 7, Supplementary Table 6). Among them, 11 genes were the same. Some of those genes may be related to kernel traits. For instance, TraesCS6A01G104300, which encodes GDSL-like lipase, plays an important role in seed development and plant growth (Tan et al., 2014). The protein encoded by TraesCS6A01G104200 has the domain of an F-box protein, which is involved in the nutrition as well as reproductive growth and development in many plants, and it can be used as the site of protein interaction to provide the basis for grain filling (Van den Burg et al., 2008; Ma et al., 2019a). However, the target genes need to be verified and confirmed by further studies.
The original contributions presented in the study are included in the article/Supplementary Material, further inquiries can be directed to the corresponding author/s.
TR and ZL designed the experiments. TR and ZR created the RIL population. TR, TF, SC, XO, YC, QJ, YD, ZS, WP, and ZR participated in phenotype measurement. TR, ZL, TF, and ZR did the field experiments. TR, ZL, and TF participated in data analysis and processing. TF performed QTL analysis. TR wrote the manuscript. All authors participated in the research and approved the final manuscript.
The authors declare that the research was conducted in the absence of any commercial or financial relationships that could be construed as a potential conflict of interest.
All claims expressed in this article are solely those of the authors and do not necessarily represent those of their affiliated organizations, or those of the publisher, the editors and the reviewers. Any product that may be evaluated in this article, or claim that may be made by its manufacturer, is not guaranteed or endorsed by the publisher.
We gratefully acknowledge the financial support from the National Natural Science Foundation of China (#31801357), and the Foundation of Sichuan Province Science and Technology Support Program (#2019YJ0510, #2021YJ0509, #2021JDRC0127). We also thank Professor Shigui Li for providing the automatic grain analyzer equipment.
The Supplementary Material for this article can be found online at: https://www.frontiersin.org/articles/10.3389/fpls.2021.713890/full#supplementary-material
Allen, A. M., Barker, G., Wilkinson, P., Burridge, A., Winfield, M., Coghill, J., et al. (2013). Discovery and development of exome-based, co-dominant single nucleotide polymorphism markers in hexaploid wheat (Triticum aestivum L.). Plant Biotechnol. J. 11, 279–295. doi: 10.1111/pbi.12009
Barchi, L., Lanteri, S., Portis, E., et al. (2012). A RAD tag derived marker based eggplant linkage map and the location of QTLs determining anthocyanin pigmentation. PLoS ONE 7:e43740. doi: 10.1371/journal.pone.0043740
Bednarek, J., Boulaflous-Stevens, A., Girousse, C., Ravel, C., Tassy, C., Barret, P., et al. (2012). Down-regulation of the TaGW2 gene by RNA interference results in decreased grain size and weight in wheat. J. Exp. Bot. 63, 5945–5955. doi: 10.1093/jxb/ers249
Brinton, J., Ramirez-Gonzalez, R. H., Simmonds, J., Wingen, L., Orford, S., and Griffiths, S. (2020). A haplotype-led approach to increase the precision of wheat breeding. Commun. Biol. 3:712. doi: 10.1038/s42003-020-01413-2
Brinton, J., Simmonds, J., Minter, F., Leverington-Waite, M., Snape, J., and Uauy, C. (2017). Increased pericarp cell length underlies a major quantitative trait locus for grain weight in hexaploid wheat. New Phytol. 215, 1026–1038. doi: 10.1111/nph.14624
Cabral, A. L., Jordan, M. C., Larson, G., Somers, D. J., Humphreys, D. G., and McCartney, C. A. (2018). Relationship between QTL for grain shape, grain weight, test weight, milling yield, and plant height in the spring wheat cross RL4452/‘AC Domain'. PLoS ONE 13:e0190681. doi: 10.1371/journal.pone.0190681
Campbell, B. T., Baenziger, P. S., Gill, K. S., Eskridge, K. M., Budak, H., Erayman, M., et al. (2003). Identification of QTLs and environmental interactions associated with agronomic traits on chromosome 3A of wheat. Crop Sci. 43, 1493–1505. doi: 10.2135/cropsci2003.1493
Cao, G., Zhu, J., He, C., Gao, Y., Yan, J., and Wu, P. (2001). Impact of epistasis and QTL × environment interaction on the developmental behavior of plant height in rice (Oryza sativa L.). Theor. Appl. Genet. 103, 153–160. doi: 10.1007/s001220100536
Cao, S. H., Xu, D. A., Hanif, M., Xia, X. C., and He, Z. H. (2020). Genetic architecture underpinning yield component traits in wheat. Theor. Appl. Genet. 133, 1811–1823. doi: 10.1007/s00122-020-03562-8
Chaves, M. S., Martinelli, J. A., Wesp-Guterres, C., Graichen, F. A., Brammer, S. P., Scagliusi, S. M., et al. (2013). The importance for food security of maintaining rust resistance in wheat. Food Secur. 5, 157–176. doi: 10.1007/s12571-013-0248-x
Chen, Z. L., Wang, B. B., Dong, X. M., Liu, H., Ren, L. H., Chen, J., et al. (2014). An ultra-high density bin-map for rapid QTL mapping for tassel and ear architecture in a large F2 maize population. BMC Genomics 15:433. doi: 10.1186/1471-2164-15-433
Cui, F., Zhang, N., Fan, X., Zhang, W., Zhao, C., Yang, L., et al. (2017). Utilization of a Wheat660K SNP array-derived high-density genetic map for high-resolution mapping of a major QTL for kernel number. Sci. Rep. 7:3788. doi: 10.1038/s41598-017-04028-6
Gao, F. M., Wen, W. E., Liu, J. D., Rasheed, A., Yin, G. H., Xia, X. C., et al. (2015). Genome-wide linkage mapping of QTL for yield components, plant height and yield-related physiological traits in the Chinese wheat cross Zhou 8425B/Chinese Spring. Front. Plant Sci. 6:1099. doi: 10.3389/fpls.2015.01099
Gegas, V. C., Nazari, A., Griffths, S., Simmonds, J., Fish, L., Orford, S., et al. (2010). A genetic framework for grain size and shape variation in wheat. Plant Cell. 22, 1046–1056. doi: 10.1105/tpc.110.074153
Gupta, P. K., Balyan, H. S., Kulwal, P. L., Kumar, N., Kumar, A., Mir, R. R., et al. (2007). QTL analysis for some quantitative traits in bread wheat. J. Zhejiang Univ. Sci. 8, 807–814. doi: 10.1631/jzus.2007.B0807
Gupta, P. K., Langridge, P., and Mir, R. R. (2010). Marker-assisted wheat breeding: present status and future possibilities. Mol Breed. 26, 145–161. doi: 10.1007/s11032-009-9359-7
Heidari, B., Sayed-Tabatabaei, B. E., Saeidi, G., Kearsey, M., and Suenaga, K. (2011). Mapping QTL for grain yield, yield components, and spike features in a doubled haploid population of bread wheat. Genome 54, 517–527. doi: 10.1139/g11-017
Hou, J., Jiang, Q. Y., Hao, C. Y., Wang, Y. Q., Zhang, H. N., and Zhang, X. Y. (2014). Global selection on sucrose synthase haplotypes during a century of wheat breeding. Plant Physiol. 164, 1918–1929. doi: 10.1104/pp.113.232454
Hu, J., Li, J., Wu, P., Li, Y., Qiu, D., Qu, Y., et al. (2019). Development of SNP, KASP, and SSR Markers by BSR-Seq technology for saturation of genetic linkage map and efficient detection of wheat powdery mildew resistance gene Pm61. Int. J. Mol. Sci. 20:E750. doi: 10.3390/ijms20030750
Hu, Y., Ren, T., Li, Z., Tang, Y., Ren, Z., and Yan, B. (2017). Molecular mapping and genetic analysis of a QTL controlling spike formation rate and tiller number in wheat. Gene 634, 15–21. doi: 10.1016/j.gene.2017.08.039
Huang, S., Wu, J. H., Wang, X. T., Mu, J. M., Xu, Z., Zeng, Q. D., et al. (2019). Utilization of the genomewide wheat 55K SNP array for genetic analysis of stripe rust resistance in common wheat line P9936. Phytopathology 109, 819–827. doi: 10.1094/PHYTO-10-18-0388-R
Jiang, Q. Y., Hou, J., Hao, C. Y., Wang, L. F., Ge, H. M., Dong, Y. S., et al. (2011). The wheat (T. aestivum) sucrose synthase 2 gene (TaSus2) active in endosperm development is associated with yield traits. Funct. Integr. Genomics. 11, 49–61. doi: 10.1007/s10142-010-0188-x
Kuchel, H., Fox, R., Reinheimer, J., Mosionek, L., Willey, N., Bariana, H., et al. (2007). The successful application of a marker-assisted wheat breeding strategy. Mol. Breed. 20, 295–308. doi: 10.1007/s11032-007-9092-z
Kumar, A., Mantovani, E. E., Seetan, R., Soltani, A., Echeverry-Solarte, M., Jain, S., et al. (2016). Dissection of genetic factors underlying wheat kernel shape and size in an elite × nonadapted cross using a high density SNP linkage map. Plant Genome 9, 1–22. doi: 10.3835/plantgenome2015.09.0081
Kumari, S., Jaiswal, V., Mishra, V. K., Paliwal, R., Balyan, H. S., and Gupta, P. K. (2018). QTL mapping for some grain traits in bread wheat (Triticum aestivum L.). Physiol. Mol. Biol. Plants 24, 909–920. doi: 10.1007/s12298-018-0552-1
Li, F., Wen, W., He, Z., Liu, J., Jin, H., Cao, S., et al. (2018). Genome wide linkage mapping of yield related traits in three Chinese bread wheat populations using high density SNP markers. Theor. Appl. Genet. 131, 1903–1942. doi: 10.1007/s00122-018-3122-6
Liao, C. Y., Wu, P., Hu, B., and Yi, K. K. (2001). Effects of genetic background and environment on QTLs and epistasis for rice (Oryza sativa L.) panicle number. Theor. Appl. Genet. 103, 104–111. doi: 10.1007/s001220000528
Lin, Y., Jiang, X. J., Tao, Y., Yang, X. L., Wang, Z. Q., Wu, F. K., et al. (2020). Identification and validation of stable quantitative trait loci for grain filling rate in common wheat (Triticum aestivum L.). Theor. Appl. Genet. 133, 2377–2385. doi: 10.1007/s00122-020-03605-0
Liu, T., Wu, L. J., Gan, X. L., Chen, W. J., Liu, B. L., Fedak, G., et al. (2020). Mapping quantitative trait loci for 1000-grain weight in a double haploid population of common wheat. Int. J. Mol. Sci. 21:3960. doi: 10.3390/ijms21113960
Luo, L. J., Li, Z. K., Mei, H. W., Shu, Q. Y., Tabien, R., Zhong, D. B., et al. (2001). Overdominant epistatic loci are the primary genetic basis of inbreeding depression and heterosis in rice. II. Grain yield components. Genetics 158, 1755–1771. doi: 10.1093/genetics/158.4.1755
Ma, D. Y., Yan, J., He, Z. H., Wu, L., and Xia, X. C. (2012). Characterization of a cell wall invertase gene TaCwi-A1 on common wheat chromosome 2A and development of functional markers. Mol. Breed. 29, 43–52. doi: 10.1007/s11032-010-9524-z
Ma, J., Ding, P. Y., Liu, J. J., Li, T., Zou, Y. Y., Habib, A., et al. (2019b). Identification and validation of a major and stably expressed QTL for spikelet number per spike in bread wheat. Theor. Appl. Genet. 132, 3155–3167. doi: 10.1007/s00122-019-03415-z
Ma, J., Zhang, H., Li, S., Zou, Y., Li, T., Liu, J., et al. (2019a). Identification of quantitative trait loci for kernel traits in a wheat cultivar Chuannong16. BMC Genet. 20:77. doi: 10.1186/s12863-019-0782-4
Meng, L., Li, H. H., Zhang, L. Y., and Wang, J. K. (2015). QTL IciMapping: integrated software for genetic linkage map construction and quantitative trait locus mapping in biparental populations. Crop J. 3, 269–283. doi: 10.1016/j.cj.2015.01.001
Miao, L. L., Mao, X. G., Wang, J. Y., Liu, Z. C., Zhang, B., Li, W. Y., et al. (2017). Elite haplotypes of a protein kinase gene TaSnRK2.3 associated with important agronomic traits in common wheat. Front. Plant Sci. 8:368. doi: 10.3389/fpls.2017.00368
Mohler, V., Albrecht, T., Castell, A., Diethelm, M., Schweizer, G., and Hartl, L. (2016). Considering causal genes in the genetic dissection of kernel traits in common wheat. J. Appl. Genet. 57, 467–476. doi: 10.1007/s13353-016-0349-2
Prashant, R., Kadoo, N., Desale, C., Kore, P., Dhaliwal, H. S., Chhuneja, P., et al. (2012). Kernel morphometric traits in hexaploid wheat (Triticum aestivum L.) are modulated by intricate QTL × QTL and genotype × environment interactions. J. Cereal Sci. 56, 432–439. doi: 10.1016/j.jcs.2012.05.010
Ramya, P., Chaubal, A., Kulkarni, K., Gupta, L., Kadoo, N., Dhaliwal, H. S., et al. (2010). QTL mapping of 1000-kernel weight, kernel length, and kernel width in bread wheat (Triticum aestivum L.). J. Appl. Genet. 51, 421–429. doi: 10.1007/BF03208872
Ren, T., Hu, Y., Tang, Y., Li, C., Yan, B., Ren, Z., et al. (2018). Utilization of a Wheat55K SNP array for mapping of major QTL for temporal expression of the tiller number. Front. Plant Sci. 9:333. doi: 10.3389/fpls.2018.00333
Ren, T. H., Fan, T., Chen, S. L., Li, C. S., Chen, Y. Y., Ou, X., et al. (2021). Utilization of a Wheat55K SNP array-derived high-density genetic map for high-resolution mapping of quantitative trait loci for important kernel-related traits in common wheat. Theor. Appl. Genet. 134, 807–821. doi: 10.1007/s00122-020-03732-8
Sajjad, M., Ma, X., Khan, S. H., Shoaib, M., Song, Y. H., Yang, W. L., et al. (2017). TaFlo2-A1, an ortholog of rice Flo2, is associated with thousand grain weight in bread wheat (Triticum aestivum L.). BMC Plant Biol. 17:164. doi: 10.1186/s12870-017-1114-3
Semagn, K., Babu, R., Hearne, S., and Olsen, M. (2014). Single nucleotide polymorphism genotyping using Kompetitive Allele Specific PCR (KASP): overview of the technology and its application in crop improvement. Mol. Breed. 33, 1–14. doi: 10.1007/s11032-013-9917-x
Simmonds, J., Scott, P., Leverington-Waite, M., Turner, A. S., Brinton, J., Korzun, V., et al. (2014). Identifcation and independent validation of a stable yield and thousand grain weight QTL on chromosome 6A of hexaploid wheat (Triticum aestivum L.). BMC Plant Biol. 14, 1–13. doi: 10.1186/s12870-014-0191-9
Su, Q. N., Zhang, X. L., Zhang, W., Zhang, N., Song, L. Q., Liu, L., et al. (2018). QTL detection for kernel size and weight in bread wheat (Triticum aestivum L.) using a high-density SNP and SSR-based linkage map. Front. Plant Sci. 9:1484. doi: 10.3389/fpls.2018.01484
Sun, C. W., Dong, Z. D., Zhao, L., Ren, Y., Zhang, N., and Chen, F. (2020). The Wheat 660K SNP array demonstrates great potential for marker-assisted selection in polyploid wheat. Plant Biotechnol. J. 18, 1354–1360. doi: 10.1111/pbi.13361
Tan, C. T., Yu, H., Yan, Y., Xu, X., Chen, M., Rudd, J. C., et al. (2017). Development and validation of KASP markers for the greenbug resistance gene Gb7 and the Hessian fy resistance gene H32 in wheat. Theor. Appl. Genet. 130, 1867–1884. doi: 10.1007/s00122-017-2930-4
Tan, X. L., Yan, S. Z., Tan, R. K., Zhang, Z. Y., Wang, Z., and Chen, J. (2014). Characterization and expression of a GDSL-like lipase gene from Brassica napus in Nicotiana benthamiana. Protein J. 33, 18–23. doi: 10.1007/s10930-013-9532-z
Tyagi, S., Mir, R. R., Balyan, H. S., and Gupta, P. K. (2015). Interval mapping and meta-QTL analysis of grain traits in common wheat (Triticum aestivum L.). Euphytica 201, 367–380. doi: 10.1007/s10681-014-1217-y
Van den Burg, H. A., Tsitsigiannis, D. I., Rowland, O., Lo, J., Rallapalli, G., MacLean, D., et al. (2008). The F-box protein ACRE189/ACIF1 regulates cell death and defense responses activated during pathogen recognition in tobacco and tomato. Plant Cell. 20, 697–719. doi: 10.1105/tpc.107.056978
Wang, N., Fang, L., Xin, H., Wang, L., and Li, S. (2012). Construction of a high-density genetic map for grape using next generation restriction-site associated DNA sequencing. BMC Plant Biol. 12:148. doi: 10.1186/1471-2229-12-148
Wang, S. S., Yan, X. F., Wang, Y. Y., Liu, H. M., Cui, D. Q., and Chen, F. (2016). Haplotypes of the TaGS5-A1 gene are associated with thousand-kernel weight in Chinese bread wheat. Front. Plant Sci. 7:783. doi: 10.3389/fpls.2016.00783
Xiao, S. H., and He, Z. H. (2003). “Wheat yield and end use quality improvement in China (Chapter 13),” in Chinese Wheat Improvement and Pedigree Analysis, eds Q. S. Zhuang (Beijing: China Agricultural Publish Press), 497–549.
Xiao, Y. G., He, S. M., Yan, J., Zhang, Y., Zhang, Y. L., Wu, Y. P., et al. (2011). Molecular mapping of quantitative trait loci for kernel morphology traits in a non-1BL.1RS ×1BL.1RS wheat cross. Crop Pasture Sci. 62, 625–638. doi: 10.1071/CP11037
Xie, W. B., Feng, Q., Yu, H. H., Huang, X. H., Zhao, Q., Xing, Y. Z., et al. (2010). Parent-independent genotyping for constructing an ultrahigh-density linkage map based on population sequencing. Proc. Natl. Acad. Sci. U.S.A. 107, 10578–10583. doi: 10.1073/pnas.1005931107
Xin, F., Zhu, T., Wei, S. W., Han, Y. C., Zhao, Y., Zhang, D. Z., et al. (2020). QTL Mapping of kernel traits and validation of a major QTL for kernel length-width ratio using SNP and bulked segregant analysis in wheat. Sci. Rep. 10:25. doi: 10.1038/s41598-019-56979-7
Yan, L., Liang, F., Xu, H. W., Zhang, X. P., Zhai, H. J., Sun, Q. X., et al. (2017). Identification of QTL for grain size and shape on the D genome of natural and synthetic allohexaploid wheats with near-identical AABB genomes. Front. Plant Sci. 8:1705. doi: 10.3389/fpls.2017.01705
Yan, X. F., Zhao, L., Ren, Y., Dong, Z. D., Cui, D. Q., and Chen, F. (2019). Genome-wide association study revealed that the TaGW8 gene was associated with kernel size in Chinese bread wheat. Sci. Rep. 9:2702. doi: 10.1038/s41598-019-38570-2
Yang, J., Zhou, Y., Wu, Q., Chen, Y., Zhang, P., Zhang, Y., et al. (2019). Molecular characterization of a novel TaGL3-5A allele and its association with grain length in wheat (Triticum aestivum L.). Theor. Appl. Genet. 132, 1799–1814. doi: 10.1007/s00122-019-03316-1
Yu, K., Liu, D. C., Chen, Y., Wang, D. Z., Yang, W. L., Yang, W., et al. (2019). Unraveling the genetic architecture of grain size in einkorn wheat through linkage and homology mapping and transcriptomic profiling. J. Exp. Bot. 70, 4671–4688. doi: 10.1093/jxb/erz247
Zhai, H., Feng, Z., Du, X., Song, Y., Liu, X., Qi, Z., et al. (2018). A novel allele of TaGW2-A1 is located in a finely mapped QTL that increases grain weight but decreases grain number in wheat (Triticum aestivum L.). Theor. Appl. Genet. 131, 539–553. doi: 10.1007/s00122-017-3017-y
Zhang, B., Liu, X., Xu, W. N., Chang, J. Z., Li, A., Mao, X. G., et al. (2015). Novel function of a putative MOC1 ortholog associated with spikelet number per spike in common wheat. Sci. Rep. 5:12211. doi: 10.1038/srep12211
Zhang, P. F., He, Z. H., Tian, X. L., Gao, F. M., Xu, D. A, Liu, J. D., et al. (2017). Cloning of TaTPP-6AL1 associated with grain weight in bread wheat and development of functional marker. Mol. Breed. 37:78. doi: 10.1007/s11032-017-0676-y
Zhang, P. P., Li, X., Gebrewahid, T. W., Liu, H. X., Xia, X. C., He, Z. H., et al. (2019). QTL mapping of adult-plant resistance to leaf and stripe rust in wheat cross SW 8588/Thatcher using the wheat 55K SNP array. Plant Dis. 103, 3041–3049. doi: 10.1094/PDIS-02-19-0380-RE
Zhao, J. L., Wang, H. W., Zhang, X. C., Du, X. Y., Li, A. F., and Kong, L. R. (2015). Association analysis of grain traits with SSR markers between Aegilops tauschii and hexaploid wheat (Triticum aestivum L.). J. Integr. Agric. 14, 1936–1948. doi: 10.1016/S2095-3119(15)61070-X
Zhu, X. F., Zhang, H. P., Hu, M. J., Wu, Z. Y., Jiang, H., Cao, J. J., et al. (2016). Cloning and characterization of Tabas1-B1 gene associated with flag leaf chlorophyll content and thousand-grain weight and development of a gene-specific marker in wheat. Mol. Breed. 36:142. doi: 10.1007/s11032-016-0563-y
Keywords: wheat, QTL mapping, kernel size, wheat55K, KASP
Citation: Ren T, Fan T, Chen S, Ou X, Chen Y, Jiang Q, Diao Y, Sun Z, Peng W, Ren Z, Tan F and Li Z (2021) QTL Mapping and Validation for Kernel Area and Circumference in Common Wheat via High-Density SNP-Based Genotyping. Front. Plant Sci. 12:713890. doi: 10.3389/fpls.2021.713890
Received: 24 May 2021; Accepted: 20 July 2021;
Published: 17 August 2021.
Edited by:
Kun Lu, Southwest University, ChinaReviewed by:
Wilco Ligterink, KeyGene, NetherlandsCopyright © 2021 Ren, Fan, Chen, Ou, Chen, Jiang, Diao, Sun, Peng, Ren, Tan and Li. This is an open-access article distributed under the terms of the Creative Commons Attribution License (CC BY). The use, distribution or reproduction in other forums is permitted, provided the original author(s) and the copyright owner(s) are credited and that the original publication in this journal is cited, in accordance with accepted academic practice. No use, distribution or reproduction is permitted which does not comply with these terms.
*Correspondence: Tianheng Ren, cmVudGhAc2ljYXUuZWR1LmNu; Zhi Li, bGl6aGlAc2ljYXUuZWR1LmNu
†These authors have contributed equally to this work
Disclaimer: All claims expressed in this article are solely those of the authors and do not necessarily represent those of their affiliated organizations, or those of the publisher, the editors and the reviewers. Any product that may be evaluated in this article or claim that may be made by its manufacturer is not guaranteed or endorsed by the publisher.
Research integrity at Frontiers
Learn more about the work of our research integrity team to safeguard the quality of each article we publish.