- Key Laboratory of Germplasm Enhancement, Physiology and Ecology of Food Crops in Cold Region, Ministry of Education, Northeast Agricultural University, Harbin, China
Over-application of nitrogen (N) fertilizer in fields has had a negative impact on both environment and human health. Domesticated rice varieties with high N use efficiency (NUE) reduce fertilizer requirements, enabling sustainable agriculture. Genome-wide association study (GWAS) analysis of N absorption and utilization traits under low and high N conditions was performed to obtain 12 quantitative trait loci (QTLs) based on genotypic data including 151,202 single-nucleotide polymorphisms (SNPs) developed by re-sequencing 267 japonica rice varieties. Eighteen candidate genes were obtained by integrating GWAS and transcriptome analyses; among them, the functions of OsNRT2.4, OsAMT1.2, and OsAlaAT genes in N transport and assimilation have been identified, and OsJAZ12 and OsJAZ13 also play important roles in rice adaptation to abiotic stresses. A NUE-related candidate gene, OsNAC68, was identified by quantitative real-time PCR (qRT-PCR) analyses. OsNAC68 encodes a NAC transcription factor and has been shown to be a positive regulator of the drought stress response in rice. Overexpression of OsNAC68 significantly increased rice NUE and grain yield under deficient N conditions, but the difference was not significant under sufficient N conditions. NUE and grain yield significantly decreased under both N supply conditions in the osbnac68 mutant. This study provides crucial insights into the genetic basis of N absorption and utilization in rice, and a NUE-related gene, OsNAC68, was cloned to provide important resources for rice breeding with high NUE and grain yield.
Introduction
Rice is the most important food crop in the world, and sustainable and healthy crop development is important for global food security. Under the severe reality of increasing populations and decreasing land resources, the rice yield per unit area has been steadily enhanced by improving rice varieties, supporting advanced cultivation techniques, and increasing production investment. Nitrogen (N) is an important limiting element in rice production and plays an important role in the growth and yield of rice. However, behind the substantial increase in rice yield, excessive and unreasonable N fertilizer input has also incurred a series of problems, such as the decline in nitrogen use efficiency (NUE), the increase in production costs, and air and water pollution, which seriously affect sustainable rice production. How to reduce N fertilizer input in agricultural production and continuously increase crop yield has become a major issue in sustainable agricultural development.
In recent years, many NUE-related genes in rice have been discovered by quantitative trait locus (QTL) mapping and genome-wide association studies (GWASs) (Xu et al., 2012; Li et al., 2017). Through cloning and functional identification of these genes, the in-depth study of their mode of action and regulatory mechanisms not only has laid a good foundation for improving rice NUE-related research but also has important theoretical significance and practical application value (Gao et al., 2019, 2020; Tang et al., 2019; Guo et al., 2020; Yu et al., 2020).
Glutamate synthase is a key enzyme in rice N metabolism. Overexpression of its coding gene (OsNADH-GOGAT1) can increase spikelet grain weight and improve NUE of rice (Yamaya et al., 2002). Overexpression of OsPTR9 can increase N uptake efficiency and yield in rice under normal growth conditions (Fang et al., 2013). Sun et al. (2014) showed that DEP1, a key gene for the increase of super rice yield in China, also plays a vital role in improving NUE of rice. Rice with dep1-1 allelic variations is N-insensitive in vegetative growth and has increased N uptake and assimilation. The natural variation of a base in the nitrate transporter, NRT1.1b, can increase NUE in indica rice, and the introduction of indica OsNRT1.1b into japonica varieties can improve its NUE and yield (Hu et al., 2015). The functional study of the OsNRT1.1b homologous gene, OsNRT1.1a, showed that the yield and NUE of OsNRT1.1a-overexpression lines were significantly improved under low and high N conditions (Wang et al., 2018a). GRF4 is the key gene of the gibberellin signal transduction pathway, which can promote N absorption and utilization and promote plant photosynthesis, thereby promoting plant growth and development. The high-level accumulation of the GRF4 protein can synergistically improve crop photosynthesis and NUE, so that rice can achieve higher yields with appropriate reduction in N fertilizer application (Li et al., 2018). NGR5 is a target of the gibberellin receptor, GID1, in promoting proteasome damage. Increasing NGR5 activity can improve NUE and yield in rice (Wu et al., 2020).
In summary, it is of great theoretical significance and practical application value to explore NUE-related genes in rice, study their function and expression characteristics, and analyze their molecular mechanisms. In this study, a rice NUE-related gene, OsNAC68, was identified by integrating GWAS and transcriptome analyses. OsNAC68-overexpression, transgenic rice (133-7, 133-11) and CRISPR/Cas9 gene editing knock-down expression mutant, osnac68 (131-13, 131-36), were used as experimental materials to carry out the current study. The function of OsNAC68 in rice NUE and yield formation was analyzed.
Materials and Methods
Plant Materials and Genotyping
The natural population for the GWAS comprised 267 japonica rice varieties, which were provided by the Institute of Crop Science, Chinese Academy of Agricultural Sciences. These varieties come from 45 countries and regions including China, Japan, the United States, etc.; all 267 japonica rice varieties included temperate japonica and tropical japonica rice (Supplementary Table 1). The high-density single-nucleotide polymorphism (SNP) loci were obtained from the “3K RG 4.8mio”-filtered SNP dataset (Wang et al., 2018b). A total of 151,202 SNPs were obtained for GWAS analysis by removing rare alleles [minor allele frequency (MAF < 5%)] and SNP markers with more than 20% missing markers (Supplementary Figure 1). The genetic structure of the population was calculated using STRUCTURE 2.3.4 software; the K (population) was preset to 1–10, and the length of the burn-in period at the beginning of Markov chain Monte Carlo (MCMC) was set to 10,000 times and then to “no counting.” The MCMC after iteration was set to 10,000, and then the appropriate population (K) value was selected according to the principle of maximum likelihood (Pritchard et al., 2000).
Evaluation of N Absorption and Utilization Traits
Rice seeds were air-dried naturally and kept at 55°C for 5 days to break dormancy. They were then surface-sterilized with 1% sodium hypochlorite solution for 10 min, rinsed with sterile deionized water, and soaked in distilled water at 30°C in dark conditions for 2 days. The seeds were grown in a N-sufficient nutrient solution in a growth chamber (28/25°C; 10 h light/14 h dark). At the three-leaf heart stage, seedlings with the same growth were selected and supplied with 0.96 (Low-N) or 2.88 mMol L–1 ppm (High-N) of N using NH4NO3 as the source and grown under natural conditions for 30 days during the growing season. Each group included 30 seedlings. The hydroponic nutrient solution was formulated according to a previous research (Xin et al., 2019a). After 30 days of low and high N treatments, the above-ground part was harvested to determine N accumulation under low N conditions (LNA, mg plant–1), N accumulation under high N conditions (HNA, mg plant–1), NUE under low N conditions (LNUE, g g–1), and NUE under high N conditions (HNUE, g g–1). The samples were dried at 80°C to a constant weight, and shoot biomass was measured with a balance. Samples were then powdered with a micro-pulverizer (FZ102, China), and an element analyzer (Elementar Vario MACRO cube, Germany) was used to determine N contents. The heritability calculation of phenotypic data was conducted using lme4 (R package). NUE (g g–1) was calculated as:
GWAS Analysis
GWAS was conducted via the mixed linear model (MLM) method using Tassel 5.0 software (Bradbury et al., 2007). The population structure (Q) and kinship calculated among individuals were used to adjust the population structure. The threshold was set at P < 3.31 × 10–6 (0.05/151,202) by the Bonferroni correction method (Li et al., 2019). To obtain the loci with the lowest P-value, redundant SNPs were filtered in a least-distance (LD) interval, and the SNP with the lowest P-value was considered the lead SNP. The Manhattan plot and quantile–quantile (Q–Q) plot were produced using the “CMplot” package in R.
Candidate Gene Prediction
The region of lead SNPs ± 100 kb was defined as the candidate region for NUE. The gene annotation and RNA sequencing data that were collected (Supplementary Table 2) under low and control N conditions in previous studies (Xin et al., 2019a,b) were combined to predict candidate genes.
RNA Extraction and Quantitative Real-Time PCR Analysis
For analysis of candidate gene expression in leaves, six N-inefficient and N-efficient varieties were selected according to the N absorption and utilization traits in 267 japonica rice varieties under low and high N conditions. The procedure and management of the experiment were the same as those in the above-mentioned experiment. Total RNA was extracted from rice leaves using the TransZol Up RNA Kit (TransGen Biotech, Beijing, China). Complementary DNA was synthesized from total RNA using the HiFiScript cDNA Synthesis Kit (CWBio, Beijing, China). Quantitative real-time PCR (qRT-PCR) analysis was performed according to a previous study (Xin et al., 2019a). All primer information is shown in Supplementary Table 3.
Vector Construction and Plant Transformation
The CRISPR/Cas9 gene editing vector construction was conducted as described by Li et al. (2017). Two target sequences (including PAM) (TACTTGCCGGTGAGGTCGTCGGG, ACCGTGCGGTCCAAGACACCGG) were selected within the target genes, and their targeting specificity was confirmed using a BLAST search against the rice genome (Hsu et al., 2013). Rice transformation was performed as described previously (Nishimura et al., 2006). Genomic DNA was extracted from these transformants, and primer pairs flanking the designed target site were used for PCR amplification. The PCR products (300–500 bp) were sequenced directly and identified using the Degenerate Sequence Decoding method (Ma et al., 2015). Knockout lines were confirmed by PCR sequencing with primers 5′-CCGCCGACTTCGGCTCCC-3′ and 5′-GGGAGGTGGGGCGGCCATG-3′ (Supplementary Figure 2). The cDNA was cloned into the pBWA(V) HS vector between the 35S promoter and terminator, generating a 35S::NAC68 construct. OsNAC68 amplification occurred with primers 5′-CAGTGGTCTCACAACATGTCCCCCTCCCGCCCC-GACG-3′ and 5′-CGATGGTCTCACAAGAACCTGATGAATTTGCCA-3′ (Supplementary Figure 3).
Determination of Yield and NUE of Genetically Modified Materials
The control rice, “Shennong 9816” (wild-type, WT), OsNAC68-overexpression (133-7, 133-11) lines, and osnca68-mutant (131-13, 131-36) rice were planted in pots (inner diameter 30 cm). The experiment was conducted at Northeast Agricultural University, China in 2020. The tests included two N application rate treatments, 60 kg (pure nitrogen) ha–1 (deficient nitrogen, DN) and 180 kg (pure nitrogen) ha–1 (sufficient nitrogen, SN), using urea as the source (46% N content). N fertilizer was applied with basal, tillering, and panicle fertilizer at a ratio of 6:3:1 at the relevant growth stage. Phosphate fertilizer (P2O5) was applied once as a basal fertilizer at a rate of 90 kg ha–1. Potash (K2O) fertilizer was applied as a basal and panicle fertilizer at a ratio of 5:5, at a rate of 90 kg ha–1. With the exception of the different N fertilizer application rates, the other cultivation requirements were identical for all pots. In the mature stage, the yield per plant, the number of effective ears per plant, the number of grains per ear, the seed setting rate, and the thousand-grain weight were calculated. The rice plants were divided into shoots and panicles at the maturity stage, and they were killed at 105°C for 30 min, dried at 80°C to a constant weight, and weighed to determine the accumulation and distribution of dry matter. The dried tissues were ground into powder and passed through a 100-mesh sieve. The N concentration of each tissue was measured with an elemental analyzer to calculate the total N accumulation (TNA). N utilization efficiency for biomass production (NUpE, g g–1) was calculated as:
N utilization efficiency for grain production (grain yield) (NUgE, g g−1) was calculated as:
Results
Phenotypic Variation in the Natural Population
To assess the phenotypic variation in NUE in 267 japonica rice varieties at the seedling stage, four NUE-related traits were evaluated: LNA, HNA, LNUE, and HNUE. The means, standard deviations, and ranges of LNA, HNA, LNUE, and HNUE at the seedling stage of natural populations are presented in Supplementary Table 4. The heritability of the NUE-related traits was higher (68.3–77.2%), indicating that population NUE-related traits are largely affected by genetic factors. The mean LNA was lower than HNA, and the mean LNUE was higher than HNUE, indicating that under low N conditions, the N acquisition of rice from the growth environment was limited, and the N utilization ability of rice to adapt to low N stress was strengthened. Statistical analysis (Figure 1) revealed continuous variation in each trait, which was consistent with the genetic characteristics of quantitative traits controlled by multiple genes. In addition, based on these traits, we identified six N-inefficient varieties and six N-efficient varieties in the natural population (Supplementary Table 5).
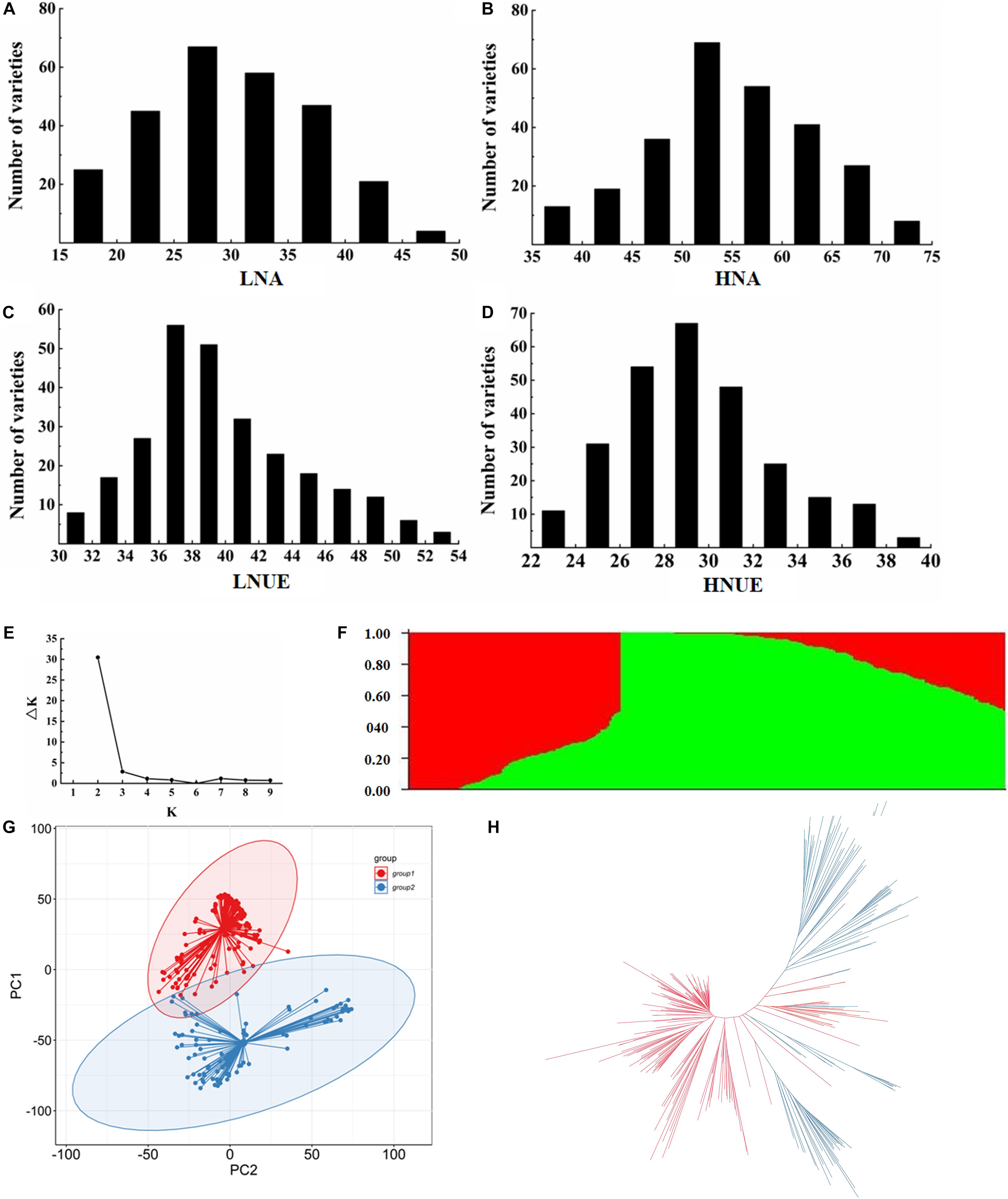
Figure 1. Phenotypic variation and population structure in 267 japonica rice varieties. (A–D) represent the LNA, HNA, LNUE, and HNUE of natural populations, respectively. (E) The variation curve of ΔK with K value. (F) Subgroups (K = 2) inferred using ADMIXTURE software. (G) Principal component analysis of 267 japonica rice varieties. Colors of red and blue represent G1 and G2 in (F), respectively. (H) Neighbor-joining tree of 267 japonica rice varieties. Colors of red and blue represent G1 and G2 in (F), respectively.
SNP Validation and Population Structure Analysis
The high-density SNP loci were obtained from the “3K RG 4.8mio”-filtered SNP dataset (Wang et al., 2018b). A total of 151,202 SNPs were obtained for GWAS, distributed on 12 chromosomes, with an average of 12,600.17 SNPs per chromosome. The genetic structure of 267 japonica germplasm resources was stratified (Figure 1E). When K = 2, the population was divided into two subgroups, designated G1 (temperate japonica) and G2 (tropical japonica). Principal component analysis and phylogenetic analysis are shown in Figures 1G,H.
GWAS for N Absorption- and Utilization-Related Traits in a Natural Population
GWAS was performed via the MLM method, with principal component analysis and kinship matrix (PCA + K) as covariates to correction of the population structure, in Tassel 5.0 software. The Manhattan and Q–Q plots for the GWAS results are shown in Figure 2. Taking −log10 (P) ≥ 6.25 as the threshold, 12 lead SNPs significantly associated with LNA, HNA, LNUE, and HNUE are listed in Table 2. These SNPs were located on chromosomes 1, 2, 3, 5, 7, 10, and 11 with −log10 (P) values ranging from 6.284 to 8.052. Three significant lead SNPs for the LNA were distributed on chromosomes 1 and 11 and were named qLNA1-1, qLNA1-2, and qLNA11, respectively. Three significant lead SNPs for the HNA were distributed on chromosomes 1, 2, and 10 and named qHNA1, qHNA2, and qHNA10, respectively. Three significant lead SNPs for the LNUE were distributed on chromosomes 1, 5, and 7 and named qLNUE1, qLNUE5, and qLNUE7, respectively. Three significant lead SNPs for the HNUE were distributed on chromosomes 3, 5, and 7 and named qHNUE3, qHNUE5, and qHNUE7, respectively (Figure 2 and Table 1). These lead SNPs are critical for N absorption and utilization at the seedling stage of rice, and there may be candidate genes for N absorption and utilization nearby.
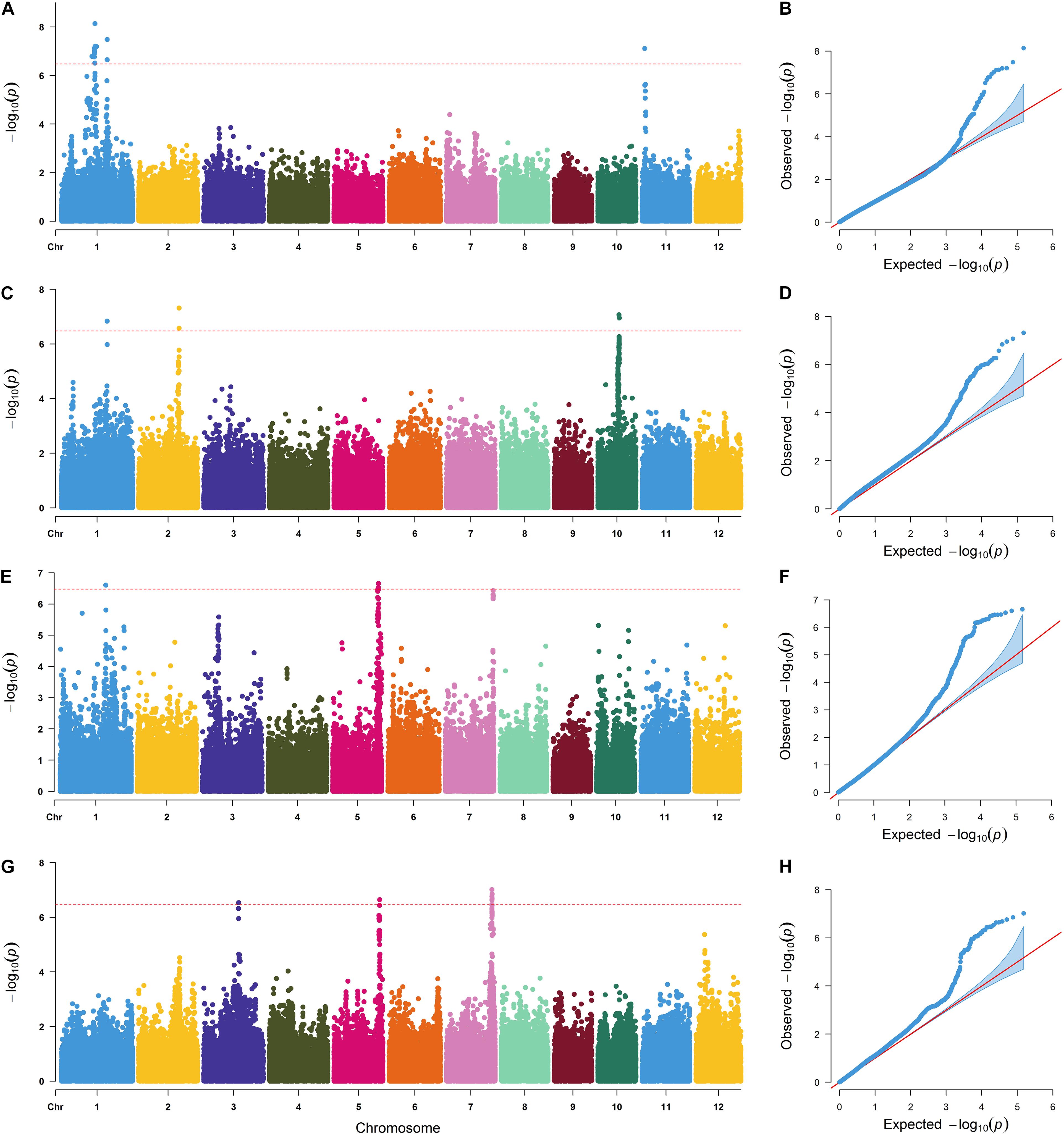
Figure 2. Manhattan plots and quantile–quantile (Q–Q) plots of genome-wide association studies for the LNA, HNA, LNUE, and HNUE. (A) Manhattan plot for the LNA. (B) Q–Q plot for the LNA. (C) Manhattan plot for the HNA. (D) Q–Q plot for the HNA. (E) Manhattan plot for the LNUE. (F) Q–Q plot for the LNUE. (G) Manhattan plot for the HNUE. (H) Q–Q plot for the HNUE.
Candidate Gene Analysis Using GWAS and RNA-seq
A total of 271 genes were retrieved from the above 11 SNPs ± 100 kb region (Supplementary Table 6), and 18 candidate genes (Table 2) were obtained by combining the previously reported RNA sequencing data of rice leaves and roots under low N conditions (Supplementary Table 2). The functions of the OsNRT2.4, OsAMT1-2, and OsAlaAT genes in N transport and assimilation have been identified (Kikuchi et al., 1999; Sonoda et al., 2003; Feng et al., 2011). OsJAZ12 and OsJAZ13 also play important roles in rice adaptation to abiotic stresses (Ye et al., 2009). Through further integrated analysis, we selected 13 candidate genes to compare expression levels between N-inefficient and N-efficient varieties using qRT-PCR analysis under low and high N conditions (Figure 3). The qRT-PCR results showed that the expression levels of Os01g0675800 in different rice varieties were significantly different, and that Os01g0675800 showed higher expression levels in N-efficient varieties than in N-inefficient varieties under both N conditions (Supplementary Table 7). Os01g0675800 was near qLNA1-2, qLNA1-2, and qLNUE1. These results suggest that Os01g0675800 is likely to be a candidate gene for qLNA1-2, qHNA1, and qLNUE1.
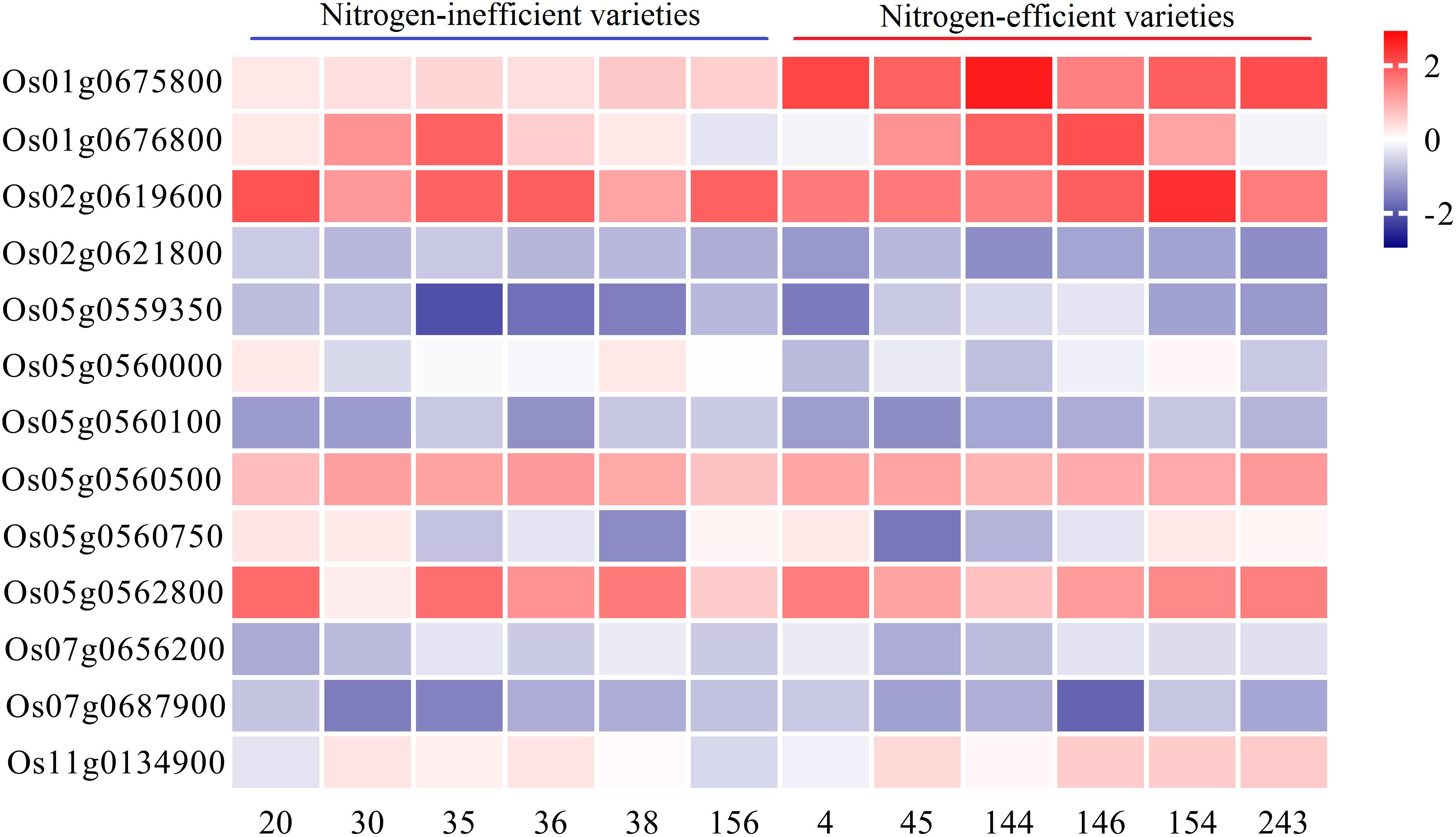
Figure 3. Relative expression of candidate genes. qRT-PCR verification of the transcript levels of candidate genes under low and high N conditions.
Effects of OsNAC68 on Grain Yield Under Different N Levels
Os01g0675800 encodes a NAC transcription factor (OsNAC68), which has been shown to be a positive regulator of drought response in rice (Shim et al., 2018). To confirm the function of OsNAC68 on grain yield and NUE of rice, we generated osnac68-mutant (131-13, 131-36) lines using the CRISPR/Cas9 method and generated OsNAC68-overexpression (133-7, 133-11) lines in which the OsNAC68 gene was driven by the 35S promoter. Compared with the WT, the yield per plant of OsNAC68-overexpression transgenic rice lines increased by 17.88 and 4.72% under DN and SN conditions, respectively, whereas the yield per plant of osnac68 mutant decreased by 21.15 and 17.97% under DN and SN conditions, respectively (Figure 4 and Supplementary Table 8). The yield-related traits analysis showed that the effective panicle number per plant of OsNAC68-overexpression transgenic rice was significantly higher than that of the WT under the two N supply levels, but the seed setting rate was significantly decreased. The grain number per panicle and 1,000 grain weight were not significantly different compared with the WT. Under both N supply levels, the grain number per panicle and the effective panicle number per plant of the osnac68 mutant were significantly lower than those of the WT, and the 1,000 grain weight and seed setting rate decreased, but the difference was not significant compared with the WT (Figure 4 and Supplementary Table 8).
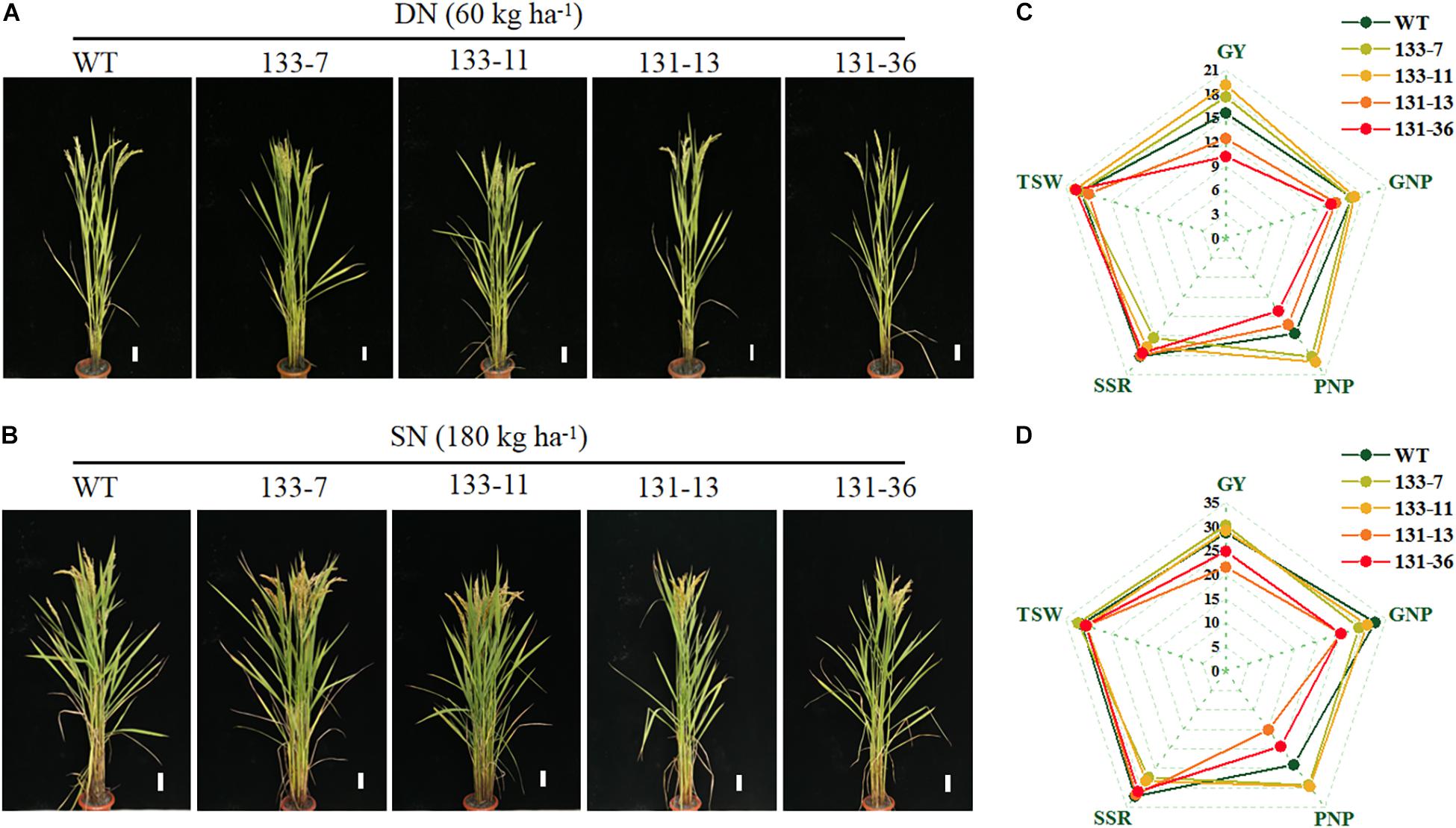
Figure 4. Effects of OsNAC68 on phenotypes and grain yield of rice under different nitrogen fertilizer levels. (A,B) Phenotypes of the whole rice plants WT and transgenic lines under DN and SN conditions at the ripening stage. Scale bar, 5 cm. (C,D) Grain yield of the WT and transgenic lines under DN and SN conditions. GY, grain yield; GNP, grain number per panicle; PNP, panicle number per plant; SSR, seed setting rate; TSW, 1,000 grain weight.
Effects of OsNAC68 on N Uptake and Utilization in Rice Under Different N Levels
Compared with the WT, overexpression of OsNAC68 significantly increased TNA (except 133-7), NUpE, and NUgE, whereas the osnac68 mutant significantly decreased TNA, NUpE, and NUgE under DN conditions (Figure 5). Compared with the WT, overexpression of OsNAC68 increased TNA, NUpE, and NUgE (except 133-7), but the difference was not significant, whereas the osnac68 mutant significantly decreased TNA, NUpE, and NUgE under SN conditions.
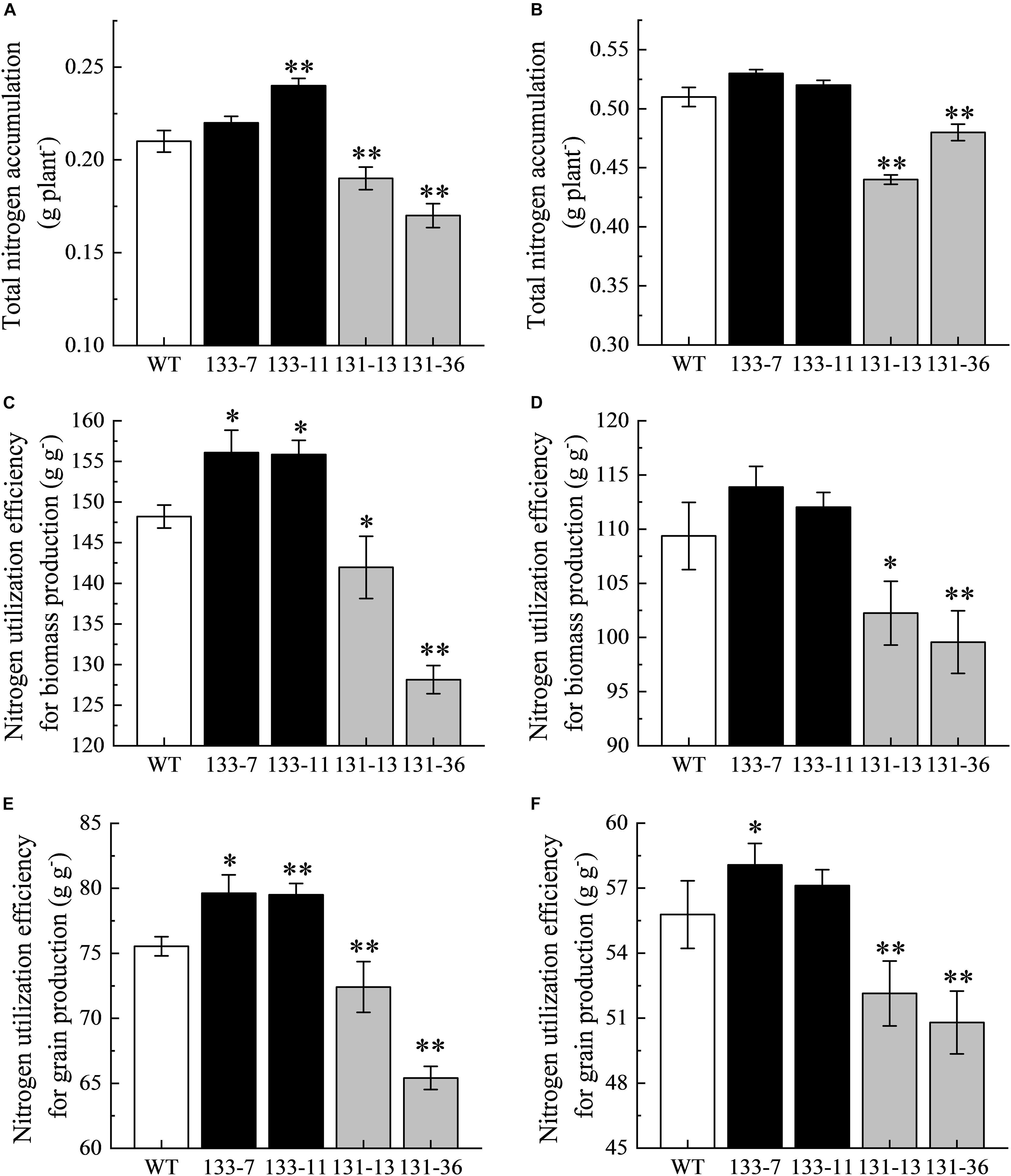
Figure 5. Evaluation of total nitrogen accumulation (TNA), nitrogen utilization efficiency for biomass production (NUpE), and nitrogen utilization efficiency for grain production (NUgE) in transgenic rice. (A,B) TNA under DN and SN conditions. (C,D) NUpE under DN and SN conditions. (E,F) NUgE under DN and SN conditions. Values are mean ± SD (n = 5), ∗P < 0.05, ∗∗P < 0.01 (t-test).
Discussion
N is present in the substances necessary for plant growth and development, such as proteins, amino acids, and phytohormones. These substances participate in physiological and metabolic regulation in plants and control various life activities and yield formation of plants. Too much or too little use of N fertilizer will cause economic losses. Improving the NUE of plants can reduce the amount of N fertilizer applied, which is essential for reducing agricultural costs, controlling environmental pollution, and ensuring food security. NUE is a measure of the difference between obtaining N from the environment and using it for growth and development (Garnett et al., 2009; Nunes-Nesi et al., 2010). An ideal, N-efficient variety needs to absorb more N from the environment and use the absorbed N to produce more biomass and grain yield.
This study shows that under low and high N conditions, different rice varieties have significant genotypic differences in N absorption and utilization efficiency, which is consistent with previous studies (Zhang et al., 2009; Ju et al., 2015). Previous studies have shown that N absorption and utilization are controlled by different genes (Broadbent et al., 1987; Datta and Broadbent, 1990). Under different levels of N supply, the genetic basis of N-efficiency of rice is related to N absorption and utilization (Senthilvel et al., 2008). Therefore, in this study, the N absorption and utilization indicators (LNA, LNUE, HNA, and HNUE) under low and high N supply were used as the NUE-related traits for GWAS analysis.
NUE is a complex quantitative trait, controlled by major genes and multiple, minor QTLs. In recent years, with the advances in molecular biology, many NUE-related genes have been identified, such as OsDEP1, OsNRT1.1B, OsNAC42, OsNPF6.1, OsNLP4, OsGRF4, OsNGR5, OsDNR1, and OsARE1 (Sun et al., 2014; Hu et al., 2015; Li et al., 2018; Wang et al., 2018; Tang et al., 2019; Wu et al., 2020; Yu et al., 2020). These studies provide a new breeding strategy for the sustainable agricultural development of rice and other crops with “less input, more output, and environmental protection,” which is of great significance both in theory and in application. In this study, 12 QTLs were identified under low and high N conditions. Only two QTLs (SNP_27750585, SNP_27777202) were co-located in NA and NUE traits, indicating that N absorption and utilization have a specific genetic basis. The genes qLNA1-2, qHNA1, and qLNUE1 are at the same or near these sites, as are qLNUE5 and qHNUE5. The results of the QTL interval gene annotation showed that there were no known genes related to N absorption, utilization, and regulation in the interval. This suggested that unknown NUE-related genes are highly likely to exist within the QTL interval, and that these genes are necessary to maintain normal plant growth, so should be detectable under different N supply conditions.
Transcriptome analysis can be used to characterize the response of various plant species to environmental stresses (Wang et al., 2009). Many studies have shown that, compared with normal N conditions, many genes show differential expression, including N metabolism-related, stress resistance-related, and hormone-related genes. Under low N stress conditions, these differentially expressed genes (DEGs) play a crucial role in rice adaptation to low N stress (Yang S. et al., 2015; Yang W. et al., 2015; Xin et al., 2019a,b; Subudhi et al., 2020). In recent years, with the development of high-throughput sequencing technology and the improvement of the transcriptome database, the combination of transcriptome and traditional localization methods has been widely used in candidate gene prediction. Yu et al. (2020) identified a N-efficient gene, OsNLP4, by integrating NUE-related traits, GWAS analysis, and transcriptome data under N-sufficient and N-starved conditions. Yao et al. (2020) used integrated GWAS and transcriptome analysis methods to obtain a series of candidate genes (including ZmSnRk2, ZmPYL, ZmNPR1) for resistance to Fusarium ear rot in maize. Our previous research also identified many saline-alkaline-tolerant candidate genes (OsIRO3, OsSAP16) by integrating GWAS/BSA and transcriptome data analysis (Li et al., 2019; Lei et al., 2020). This study identified 18 potential NUE-related candidate genes by combining the previously reported RNA sequencing data of rice leaves and roots under low N conditions. The functions of OsNRT2.4, OsAMT1-2, and OsAlaAT genes in N transport and assimilation have been identified (Kikuchi et al., 1999; Sonoda et al., 2003; Feng et al., 2011). OsJAZ12 and OsJAZ13 also play important roles in rice adaptation to abiotic stresses (Ye et al., 2009). From further qRT-PCR analysis of varieties with different N use efficiencies, Os01g0675800 was identified as a likely candidate gene for N absorption and utilization.
The gene, Os01g0675800, is a transcription factor of the NAC family. Shim et al. (2018) found that overexpression of OsNAC68 significantly increased drought resistance in rice. The NAC transcription factor family is an important transcription regulatory factor, which is ubiquitous in plants. In the life course of rice, the NAC family is involved in cell growth, tissue development, organ aging, and other processes and plays an important role in the response to external environmental change (Jeong et al., 2010, 2013; Redillas et al., 2012; Lee et al., 2017; Chung et al., 2018). In addition, nitrate signaling regulates root growth through AtNAC4 (Alvarez et al., 2020). In this study, the GY, NA, NUpE, and NUgE of the OsNAC68-overexpression lines were higher than those of the WT, especially under DN conditions. The GY, NA, NUpE, and NUgE of the osnac68-mutant lines were significantly lower than those of the WT under both N conditions. These results indicate that OsNAC68 can mediate the process of N absorption and utilization in rice, thereby affecting rice yield formation. Previous studies found that OsNAC42 can regulate OsNPF6.1 and increase the N acquisition capacity of rice, thereby increasing rice yield (Tang et al., 2019). Ueda et al. (2020) found that OsONAC1 and OsNAC2 can regulate N metabolism-related modules under low N stress. Two transcription factors, AtHAP2C and AtNAC096, and an integrase-type DNA (At4g39780) were found to regulate 53% of N responsive genes throughout the root system as downstream of AtNLP7 (Alvarez et al., 2020). Investigating whether OsNAC68 interacts with the N absorption- and utilization-related genes cascade will help to reveal the molecular mechanism of N absorption and utilization in rice. It is important for the breeding of rice with a high NUE and yield.
Conclusion
In the present study, natural populations were collected to evaluate the N absorption and utilization at the seedling stage. GWAS analysis of N absorption and utilization traits under low and high N conditions was performed to obtain 12 QTLs based on genotypic data including 151,202 SNPs developed by re-sequencing 267 japonica rice varieties. A NUE-related candidate gene, OsNAC68, was identified by GWAS, RNA-seq, and qRT-PCR analyses. The functions of OsNAC68 in rice N absorption and utilization and yield were further verified through overexpression and gene editing technology. However, the regulation mechanism of OsNAC68-mediated N absorption and utilization in rice still needs further study. This study provides resources for breeding aimed at improving rice N absorption and utilization.
Data Availability Statement
The original contributions presented in the study are included in the article/Supplementary Material, further inquiries can be directed to the corresponding author/s.
Author Contributions
DZ and WX designed the study and provided experimental materials. WX analyzed the results, prepared the figures and tables, and wrote the manuscript. All authors discussed the results and commented on the manuscript, read and approved the final manuscript.
Funding
This work was supported by grants from the Major Science and Technology Project of Heilongjiang Province, China (Grant No. 2020ZX16B01), the National Natural Science Foundation of China (U20A2025 and 31872884), and the Natural Science Foundation Joint Guide Project of Heilongjiang (LH2019C035).
Conflict of Interest
The authors declare that the research was conducted in the absence of any commercial or financial relationships that could be construed as a potential conflict of interest.
Supplementary Material
The Supplementary Material for this article can be found online at: https://www.frontiersin.org/articles/10.3389/fpls.2021.670861/full#supplementary-material
Supplementary Figure 1 | SNP density of 267 japonica rice population.
Supplementary Figure 2 | Knockout of OsNAC68 using CRISPR/Cas9 system. Sequence of wild-type transgenic line (WT) and the two mutational lines of OsNAC68 in the target region. Red letters indicated 1-bp insertions (131-13) and 4-bp deletion (131-36), Blue letters indicate the PAM region.
Supplementary Figure 3 | The overexpression of NAC68. (A) Overexpression construct for rice transformation. (B) The expression levels of 133-7, 133-11, and wild-type (WT).
Supplementary Table 1 | Geographical distribution of 267 japonica rice varieties.
Supplementary Table 2 | Differentially expressed genes (DEGs) in leaves and roots under deficient nitrogen conditions compared with sufficient nitrogen conditions.
Supplementary Table 3 | Primers for qRT-PCR in this study.
Supplementary Table 4 | Phenotypic variation in 267 japonica rice varieties under alkalinity condition. SD, standard deviation, CV, coefficient of variation.
Supplementary Table 5 | LNA, HNA, LNUE, and HNUE of nitrogen-inefficient and nitrogen-efficient varieties.
Supplementary Table 6 | Annotated genes in the QTLs region.
Supplementary Table 7 | qRT-PCR verification of the transcript levels of candidate genes under low and high N conditions.
Supplementary Table 8 | Yield-related characteristics of WT and transgenic rice under DN and SN conditions. Values are means ± SD (n = 5), ∗P < 0.05, ∗∗P < 0.01 (t-test).
References
Alvarez, J. M., Schinke, A. L., Brooks, M. D., Pasquino, A., and Leonelli, L. (2020). Transient genome-wide interactions of the master transcription factor NLP7 initiate a rapid nitrogen response cascade. Nat. Commun. 11:1157. doi: 10.1038/s41467-020-14979-6
Bradbury, P. J., Zhang, Z., Kroon, D. E., Casstevens, T. M., Ramdoss, Y., and Buckler, E. S. (2007). TASSEL: software for association mapping of complex traits in diverse samples. Bioinformatics 23, 2633–2635. doi: 10.1093/bioinformatics/btm308
Broadbent, F. E., Datta, S. K. D., and Laureles, E. V. (1987). Measurement of nitrogen utilization efficiency in rice genotypes1. Agron. J. 79, 786–791. doi: 10.2134/agronj1987.00021962007900050006x
Chung, P. J., Jung, H., Choi, Y. D., and Kim, J. K. (2018). Genome-wide analyses of direct target genes of four rice NAC-domain transcription factors involved in drought tolerance. BMC Genom. 19:40. doi: 10.1186/s12864-017-4367-1
Datta, S. K. D., and Broadbent, F. E. (1990). Nitrogen-use efficiency of 24 rice genotypes on an N-deficient soil. Field Crops Res. 23, 81–92. doi: 10.1016/0378-4290(90)90104-J
Fang, Z. M., Xia, K. F., Yang, X., Grotemeyer, M. S., Meier, S., Rentsch, D., et al. (2013). Altered expression of the PTR/NRT1 homologue OsPTR9 affects nitrogen utilization efficiency, growth and grain yield in rice. Plant Biotechnol. J. 11, 446–458. doi: 10.1111/pbi.12031
Feng, H., Yan, M., Fan, X., Li, B., Shen, Q., Miller, A. J., et al. (2011). Spatial expression and regulation of rice high-affinity nitrate transporters by nitrogen and carbon status. J. Exp. Bot. 62, 2319–2332. doi: 10.1093/jxb/erq403
Gao, Y., Xu, Z., Zhang, L., Li, S., Wang, S., and Yang, H. (2020). MYB61 is regulated by GRF4 and promotes nitrogen utilization and biomass production in rice. Nat. Commun. 11:5219. doi: 10.1038/s41467-020-19019-x
Gao, Z. Y., Wang, Y. F., Chen, G., Zhang, A. P., Yang, S. L., Shang, L., et al. (2019). The indica nitrate reductase gene OsNR2 allele enhances rice yield potential and nitrogen use efficiency. Nat. Commun. 10:5207. doi: 10.1038/s41467-019-13110-8
Garnett, T., Conn, V., and Kaiser, B. N. (2009). Root based approaches to improving nitrogen use efficiency in plants. Plant Cell. Environ. 32, 1272–1283. doi: 10.1111/j.1365-3040.2009.02011.x
Guo, N., Hu, J., Yan, M., Qu, H., Luo, L., Tegeder, M., et al. (2020). Oryza sativa lysine-histidine-type transporter 1 functions in root uptake and root-to-shoot allocation of amino acids in rice. Plant J. 103, 395–411. doi: 10.1111/tpj.14742
Hsu, P. D., Scott David, A., Weinstein Joshua, A., Ann, R. F., Silvana, K., Vineeta, A., et al. (2013). DNA targeting specificity of RNAguided Cas9 nucleases. Nat. Biotechnol. 31, 827–832. doi: 10.1038/nbt.2647
Hu, B., Wang, W., Ou, S. J., Tang, J. Y., Li, H., Che, R. H., et al. (2015). Variation in NRT1.1B contributes to nitrate-use divergence between rice subspecies. Nat. Genet. 47, 834–838. doi: 10.1038/ng.3337
Jeong, J. S., Kim, Y. S., Baek, K. H., Jung, H., Ha, S. H., Choi, Y., et al. (2010). Root-specific expression of OsNAC10 improves drought tolerance and grain yield in rice under field drought conditions. Plant Physiol. 153, 185–197. doi: 10.1104/pp.110.154773
Jeong, J. S., Kim, Y. S., Redillas, M. C., Jang, G., Jung, H., Bang, S. W., et al. (2013). OsNAC5 overexpression enlarges root diameter in rice plants leading to enhanced drought tolerance and increased grain yield in the field. Plant Biotechnol. J. 11, 101–114. doi: 10.1111/pbi.12011
Ju, C., Buresh, R. J., Wang, Z., Zhang, H., Liu, L., Yang, J., et al. (2015). Root and shoot traits for rice varieties with higher grain yield and higher nitrogen use efficiency at lower nitrogen rates application. Field Crops Res. 175, 47–55. doi: 10.1016/j.fcr.2015.02.007
Kikuchi, H., Hirose, S., Toki, S., Akama, K., and Takaiwa, F. (1999). Molecular characterization of a gene for alanine aminotransferase from rice (Oryza sativa). Plant Mol. Biol. 39, 149–159. doi: 10.1023/a:1006156214716
Lee, D. K., Chung, P. J., Jeong, J. S., Jang, G., Bang, S. W., Jung, H., et al. (2017). The rice OsNAC6 transcription factor orchestrates multiple molecular mechanisms involving root structural adaptions and nicotianamine biosynthesis for drought tolerance. Plant. Biotechnol. J. 15, 754–764. doi: 10.1111/pbi.12673
Lei, L., Zheng, H., Bi, Y., Yang, L., Liu, H., Wang, J., et al. (2020). Identification of a Major QTL and Candidate Gene Analysis of Salt Tolerance at the Bud Burst Stage in Rice (Oryza sativa L.) Using QTL-Seq and RNA-Seq. Rice 13:55. doi: 10.1186/s12284-020-00416-1
Li, H., Hu, B., and Chu, C. (2017). Nitrogen use efficiency in crops: lessons from Arabidopsis and rice. J. Exp. Bot. 68, 2477–2488. doi: 10.1093/jxb/erx101
Li, N., Zheng, H., Cui, J., Wang, J., Liu, H., Sun, J., et al. (2019). Genome-wide association study and candidate gene analysis of alkalinity tolerance in japonica rice germplasm at the seedling stage. Rice 12:24. doi: 10.1186/s12284-019-0285-y
Li, S., Tian, Y., Wu, K., Ye, Y., Yu, J., Zhang, J., et al. (2018). Modulating plant growth-metabolism coordination for sustainable agriculture. Nature 560, 595–600. doi: 10.1038/s41586-018-0415-5
Ma, X., Zhang, Q., Zhu, Q., Liu, W., Chen, Y., Qiu, R., et al. (2015). A robust CRISPR/Cas9 system for convenient, high-efficiency multiplex genome editing in monocot and dicot plants. Mol. Plant. 8, 1274–1284. doi: 10.1016/j.molp.2015.04.007
Nishimura, A., Ikuko, A., and Makoto, M. (2006). A protocol for agrobacterium-mediated transformation in rice. Nat. Protoc. 1:2796. doi: 10.1038/nprot.2006.469
Nunes-Nesi, A., Fernie, A. R., and Stitt, M. (2010). Metabolic and signaling aspects underpinning the regulation of plant carbon nitrogen interactions. Mol. Plant. 3, 973–996. doi: 10.1093/mp/ssq049
Pritchard, J. K., Stephens, M., and Donnelly, P. (2000). Inference of population structure using multilocus genotype data. Genetics 155, 945–959.
Redillas, M. C., Jeong, J. S., Kim, Y. S., Jung, H., Bang, S. W., Choi, Y. D., et al. (2012). The overexpression of OsNAC9 alters the root architecture of rice plants enhancing drought resistance and grain yield under field conditions. Plant. Biotechnol. J. 10, 792–805. doi: 10.1111/j.1467-7652.2012.00697.x
Senthilvel, S., Vinod, K. K., Malarvizhi, P., and Maheswaran, M. (2008). Qtl and qtl environment effects on agronomic and nitrogen acquisition traits in rice. J. Integr. Plant. Biol. 50, 1108–1117. doi: 10.1111/j.1744-7909.2008.00713.x
Shim, J. S., Oh, N., Chung, P. J., Kim, J., Choi, Y. D., and Kim, J. K. (2018). Overexpression of OsNAC14 improves drought tolerance in rice. Front. Plant. Sci. 9:310. doi: 10.3389/fpls.2018.00310
Sonoda, Y., Ikeda, A., Saiki, S., Yamaya, T., and Yamaguchi, J. (2003). Feedback Regulation of the Ammonium Transporter Gene Family AMT1 by Glutamine in Rice. Plant. Cell. Physiol. 44, 1396–1402. doi: 10.1093/pcp/pcg169
Subudhi, P. K., Richard, S., Garcia, R. S., Coronejo, S., and Tapia, R. (2020). Comparative Transcriptomics of Rice Genotypes with Contrasting Responses to Nitrogen Stress Reveals Genes Influencing Nitrogen Uptake through the Regulation of Root Architecture. Int. J. Mol. Sci. 21:5759. doi: 10.3390/ijms21165759
Sun, H. Y., Qian, Q., Wu, K., Luo, J. J., Wang, S. S., Zhang, C. W., et al. (2014). Heterotrimeric G proteins regulate nitrogen-use efficiency in rice. Nat. Genet. 46, 652–656. doi: 10.1038/ng.2958
Tang, W., Ye, J., Yao, X., Zhao, P., Xuan, W., Tian, Y., et al. (2019). Genome-wide associated study identifies NAC42-activated nitrate transporter conferring high nitrogen use efficiency in rice. Nat. Commun. 10:5279. doi: 10.1038/s41467-019-13187-1
Ueda, Y., Ohtsuki, N., Kadota, K., Tezuka, A., Nagano, A. J., Kadowaki, T., et al. (2020). Gene regulatory network and its constituent transcription factors that control nitrogen deficiency responses in rice. N. Phytol. 227, 1434-1452. doi: 10.1111/nph.16627
Wang, Q., Nian, J., Xie, X., Yu, H., Zhang, J., Bai, J., et al. (2018). Genetic variations in ARE1 mediate grain yield by modulating nitrogen utilization in rice. Nat. Commun. 9:735. doi: 10.1038/s41467-017-02781-w
Wang, W., Hu, B., Yuan, D. Y., Liu, Y. Q., Che, R. H., Hu, Y. C., et al. (2018a). Expression of the nitrate transporter gene OsNRT1.1A/OsNPF6.3 confers high yield and early maturation in rice. Plant Cell 30, 638–651. doi: 10.1105/tpc.17.00809
Wang, W., Mauleon, R., Hu, Z., Chebotarov, D., Tai, S., Wu, Z., et al. (2018b). Genomic variation in 3,010 diverse accessions of Asian cultivated rice. Nature 557, 43–49. doi: 10.1038/s41586-018-0063-9
Wang, Z., Gerstein, M., and Snyder, M. (2009). RNA-Seq: a revolutionary tool for transcriptomics. Nat. Rev. Genet. 10, 57–63. doi: 10.1038/nrg2484
Wu, K., Wang, S., Song, W., Zhang, J., Wang, Y., Liu, Q., et al. (2020). Enhanced sustainable green revolution yield via nitrogen-responsive chromatin modulation in rice. Science 367:eaaz2046. doi: 10.1126/science.aaz2046
Xin, W., Zhang, L., Zhang, W., Gao, J., Yi, J., Zhen, X., et al. (2019a). An integrated analysis of the Rice Transcriptome and Metabolome reveals differential regulation of carbon and nitrogen metabolism in response to nitrogen availability. Int. J. Mol. Sci. 20:2349. doi: 10.3390/ijms20092349
Xin, W., Zhang, L., Zhang, W., Gao, J., Yi, J., Zhen, X., et al. (2019b). An integrated analysis of the Rice Transcriptome and Metabolome reveals root growth regulation mechanisms in response to nitrogen availability. Int. J. Mol. Sci. 20:5893. doi: 10.3390/ijms20235893
Xu, G., Fan, X., and Miller, A. J. (2012). Plant Nitrogen Assimilation and Use Efficiency. Ann. Rev. Plant Biol. 63, 153–182. doi: 10.1146/annurev-arplant-042811-105532
Yamaya, T., Obara, M., Nakajima, H., Sasaki, S., Hayakawa, T., Sato, T., et al. (2002). Genetic manipulation and quantitative-trait loci mapping for nitrogen recycling in rice. J. Exp. Bot. 53, 917–925. doi: 10.1093/jexbot/53.370.917
Yang, S., Hao, D., Song, Z., Yang, G., Wang, L., Su, Y., et al. (2015). RNA-Seq analysis of differentially expressed genes in rice under varied nitrogen supplies. Gene 555, 305–317.
Yang, W., Yoon, J., Choi, H., Fan, Y., Chen, R., An, G., et al. (2015). Transcriptome analysis of nitrogen-starvation-responsive genes in rice. BMC Plant Biol. 15:31. doi: 10.1016/j.gene.2014.11.021
Yao, L., Li, Y., Ma, C., Tong, L., Du, F., Xu, M., et al. (2020). Combined genome-wide association study and transcriptome analysis reveal candidate genes for resistance to Fusarium ear rot in maize. J. Integr. Plant Biol. 62, 1535–1551. doi: 10.1111/jipb.12911
Ye, H., Du, H., Tang, N., Li, X., and Xiong, L. (2009). Identification and expression profiling analysis of TIFY family genes involved in stress and phytohormone responses in rice. Plant Mol. Biol. 71, 291–305. doi: 10.1007/s11103-009-9524-8
Yu, J., Xuan, W., Tian, Y., Fan, L., Sun, J., Tang, W., et al. (2020). Enhanced OsNLP4-OsNiR cascade confers nitrogen use efficiency by promoting tiller number in rice. Plant Biotechnol. J. 19, 167–176. doi: 10.1111/pbi.13450
Keywords: nitrogen use efficiency, japonica rice, OsNAC68, RNA-seq, genome-wide association study
Citation: Xin W, Wang J, Li J, Zhao H, Liu H, Zheng H, Yang L, Wang C, Yang F, Chen J and Zou D (2021) Candidate Gene Analysis for Nitrogen Absorption and Utilization in Japonica Rice at the Seedling Stage Based on a Genome-Wide Association Study. Front. Plant Sci. 12:670861. doi: 10.3389/fpls.2021.670861
Received: 22 February 2021; Accepted: 09 April 2021;
Published: 04 June 2021.
Edited by:
Hatem Rouached, Institut National de la Recherche Agronomique (INRA), FranceReviewed by:
Benoit Lacombe, Centre National de la Recherche Scientifique, Center for the National Scientific Research (CNRS), FranceNadia Bouain, Montpellier SupAgro, France
Copyright © 2021 Xin, Wang, Li, Zhao, Liu, Zheng, Yang, Wang, Yang, Chen and Zou. This is an open-access article distributed under the terms of the Creative Commons Attribution License (CC BY). The use, distribution or reproduction in other forums is permitted, provided the original author(s) and the copyright owner(s) are credited and that the original publication in this journal is cited, in accordance with accepted academic practice. No use, distribution or reproduction is permitted which does not comply with these terms.
*Correspondence: Detang Zou, zoudtneau@126.com
†These authors have contributed equally to this work