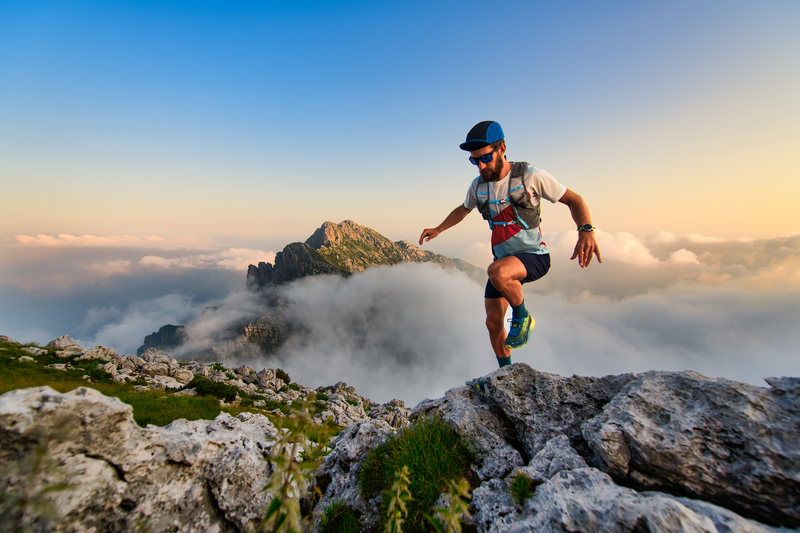
94% of researchers rate our articles as excellent or good
Learn more about the work of our research integrity team to safeguard the quality of each article we publish.
Find out more
REVIEW article
Front. Plant Sci. , 14 April 2021
Sec. Plant Abiotic Stress
Volume 12 - 2021 | https://doi.org/10.3389/fpls.2021.668512
This article is part of the Research Topic Photosynthesis Under Fluctuating Light View all 15 articles
Plants in natural environments receive light through sunflecks, the duration and distribution of these being highly variable across the day. Consequently, plants need to adjust their photosynthetic processes to avoid photoinhibition and maximize yield. Changes in the composition of the photosynthetic apparatus in response to sustained changes in the environment are referred to as photosynthetic acclimation, a process that involves changes in protein content and composition. Considering this definition, acclimation differs from regulation, which involves processes that alter the activity of individual proteins over short-time periods, without changing the abundance of those proteins. The interconnection and overlapping of the short- and long-term photosynthetic responses, which can occur simultaneously or/and sequentially over time, make the study of long-term acclimation to fluctuating light in plants challenging. In this review we identify short-term responses of plants to fluctuating light that could act as sensors and signals for acclimation responses, with the aim of understanding how plants integrate environmental fluctuations over time and tailor their responses accordingly. Mathematical modeling has the potential to integrate physiological processes over different timescales and to help disentangle short-term regulatory responses from long-term acclimation responses. We review existing mathematical modeling techniques for studying photosynthetic responses to fluctuating light and propose new methods for addressing the topic from a holistic point of view.
Plants in natural environments are exposed to light and other environmental conditions that fluctuate on timescales ranging over orders of magnitude. The rate of photosynthesis under any given set of conditions will be a function of the light absorbed, the capacity for charge separation in each photosystem, and of the use of that energy to drive carbon assimilation and other metabolic processes. To maximize light capture efficiency at all times, plants need to ensure that the capacities of electron transport and metabolism exceed the maximum rate of light absorption across the full range of environmental conditions experienced. This, however, is unlikely to be the optimal solution overall, in terms of resource allocation between different processes.
Plants growing under different conditions may be limited by, for example, light and water availability, nitrogen and other nutrients, and other abiotic constraints. Plants exposed to low irradiance will tend to invest less in electron transport proteins and enzymes of carbon assimilation, and more in light capture (antenna proteins), aiming to achieve the best photosynthetic performance given the environmental conditions (Anderson et al., 1988; Stewart et al., 2015). Conversely, a drop in temperature will slow down enzymatic activities and diffusion limited processes but will not affect energy absorption or electron transfer. Thus, plants exposed to prolonged low temperature tend to invest more in enzymes, in order to restore the balance between light capture and carbon assimilation (Stitt and Hurry, 2002).
A response to a sustained change in growth conditions over multiple days which involves a change in gene expression is defined as acclimation. Two different types of acclimation can be distinguished: developmental and dynamic (Walters, 2005; Athanasiou et al., 2010). In both, plants adjust their physiology to suit the prevailing environmental conditions. Developmental acclimation includes morphological changes, occurring when tissues develop under different environmental conditions. Dynamic acclimation occurs in fully developed organs, with fixed morphology, and involves changes in protein content and composition, which in turn affects different metabolic fluxes and metabolite concentration. Such alterations ensure optimum resource use under the new condition, and give plants the necessary plasticity to withstand changes in their environment (such as seasonal temperature and moisture changes, light fluctuation, etc.). Following this definition, we can distinguish photosynthetic acclimation from regulation (Herrmann et al., 2019b), the latter encompassing processes that alter the activity of particular steps in photosynthesis over a time scale of seconds or/and minutes, without changing the abundance of the proteins involved. It is important to note that regulatory processes may be involved in pathways controlling acclimation and will in turn be affected by the acclimation response itself.
In natural environments and in crop fields, plants receive light energy in the canopy through sunflecks (Pearcy, 1990). The duration and distribution of these are highly variable, impacting the overall photosynthetic yield (Rascher and Nedbal, 2006; Foo et al., 2020). Due to the high frequency of high-low light cycles, responses that avoid photoinhibition and maximize photosynthetic yield are required. Short-term responses to fluctuating light involves almost immediate changes in the thylakoid membranes [e.g., induction of Non-Photochemical Quenching (NPQ), including high energy-state quenching (qE) and state transitions], alteration in the activation state of enzymes (e.g., Benson-Calvin Cycle) and changes in stomatal conductance (Tikkanen et al., 2006, 2010). Meanwhile, long-term acclimation responses might include, amongst others, an increase in the pool size of the xanthophyll cycle pigments and in the PSBS protein content (Wei et al., 2020), which in turn enhance its photoprotective capacity. The interconnection and overlapping of these processes, which can occur simultaneously or sequentially over time, challenge the study of the sensing and signaling pathways involved in long-term fluctuating light acclimation in plants. Thus, an holistic approach is required, to which mathematical modeling techniques can make important contributions.
Systems modeling applies various mathematical techniques to describe and conceptualize the structural and dynamic components of a system, such as a set of biochemical pathways. Mathematical modeling can be applied at different levels and over different time-scales, describing processes inside an organelle, across the whole cell or even multiple tissues (Dada and Mendes, 2011; Gomes de Oliveira Dal'Molin et al., 2015; Shaw and Cheung, 2018). This approach has been extensively applied in biology, including studies of photosynthetic acclimation and regulation of sugar metabolism in plants (Nägele and Weckwerth, 2014; Zakhartsev et al., 2016; Herrmann et al., 2019a, 2020). Mathematical modeling is restricted by the available biological knowledge, and by the assumptions under which that knowledge is synthesized in the model. However, if the model assumptions represent an accurate description of the biological system under study, in silico studies can provide insights into the underlying processes that yield experimentally useful information. Often, modeling techniques are employed to generate new hypotheses about a complex system in an efficient, targeted, and cost-effective manner (Kitano, 2002). Thus, mathematical modeling has the potential to disentangle the many observed biochemical changes in a plant's responses to fluctuating environmental conditions to help identify sensors, signals, or acclimation responses.
For the purpose of this review we will consider immediate changes that occur upon changes in light as the inputs of a plant system (i.e., the sensors), and the long-term responses that result from sustained changes in light regimes as the corresponding outputs (i.e., the long-term acclimation responses). Considering the different timescales in which light can effectively fluctuate in natural environments, we will discuss potential signal transduction pathways that could act as links between the inputs and outputs of the system, triggering acclimation. Overall, we aim to gain a deeper understanding on the following questions: How do plants integrate environmental fluctuations over time and how do they tailor their responses accordingly?
Fluctuations in irradiance are immediately reflected in the chloroplast and in the physiology of leaves, triggering different short-term responses aimed at maximizing photosynthesis, while protecting the photosynthetic apparatus from photo-oxidative damage (Standfuss et al., 2005; Yamori, 2016). These short-term responses can also be important in triggering long-term acclimation, which is determined not only by the intensity of the incident light, but also by the frequency of oscillations (Qiao et al., 2020). In this section, we will address the different regulatory processes that act as potential inputs for the long-term acclimation responses to fluctuating light in plants (Figure 1).
Figure 1. Short and long-term responses to fluctuating light. Schematic representation of the physiological processes defined as inputs and outputs of photosynthetic acclimation to fluctuating light in plants (see sections System Inputs: Short-Term Responses to Fluctuating Light and System Outputs: Long-Term Acclimation to Fluctuating Light). Colored gradient triangles depict accumulation of ROS or intensification of a process. LHCII, Light Harvesting Complex II; PSII, Photosystem II; PSI, Photosystem I; NPQ, Non-Photochemical Quenching; CET, Cyclic Electron Transport; Cyt b6f , Cytochrome b6f ; PQ, Plastoquinone; PQH2, Plastoquinol. Created with BioRender.com.
Over-reduction of the electron transport chain, which occurs when light absorption exceeds the immediate capacity for CO2 fixation, can result in electrons “spilling over” to oxygen, leading to the production of Reactive Oxygen Species (ROS). Most directly, this includes singlet excited oxygen (1O2), superoxide (), hydrogen peroxide (H2O2), and hydroxyl radicals (HO). ROS generation can be triggered in the chloroplast by many environmental factors, including high light, salinity, drought, pathogens, etc. Therefore, plants have evolved a plethora of ROS scavenging mechanisms to minimize the harmful effects of increased ROS levels (Pospíšil, 2012; Foyer, 2018). Although ROS are also generated in other cell compartments, such as mitochondria and peroxisomes, the main sites of ROS production in the chloroplast are photosystem I (PSI) and photosystem II (PSII; Tripathy and Oelmüller, 2012; Pospíšil, 2016).
Oxygen can be reduced to by electrons derived from both photosystems (Zulfugarov et al., 2014; Pospíšil, 2016; Takagi et al., 2016). can be converted to H2O2 and O2, a process that is catalyzed by the enzyme superoxide dismutase (SOD) in the chloroplast stroma (Pospíšil, 2012). H2O2 can subsequently be converted to water, in the so called water-water cycle, via a series of redox reactions (Awad et al., 2015). Although the scavenging of H2O2 can act as an alternative electron sink, the electron flux through this pathway is quickly saturated (Driever and Baker, 2011). Thus, excess H2O2 from the chloroplast can pass to the nucleus, where it modulates gene expression and triggers plant acclimation responses (Figure 1) (Exposito-Rodriguez et al., 2017).
1O2 is mainly produced via energy transfer from triplet excited chlorophylls (3Chl*) to oxygen in PSII (Krieger-Liszkay et al., 2008; Pospíšil, 2016). Unlike H2O2, 1O2 is believed not to diffuse to the nucleus, due to its short lifetime (~200 ns; Skovsen et al., 2005). However, 1O2 produced in PSII has been shown to directly react with carotenoids and thylakoid lipids, causing oxidative damage. Oxidative products of carotenoids have been shown to have a signaling role in stress responses (Hideg et al., 1998; Triantaphylidès and Havaux, 2009; Ramel et al., 2012).
Arguably, the most important photo-protective processes in plants are collectively measured by the parameter Non-photochemical quenching (NPQ). This term includes different components that exhibit distinct activation and deactivation kinetics (Standfuss et al., 2005; Johnson and Ruban, 2009; Niyogi, 2009; Ruban et al., 2012; Derks et al., 2015). The major, and fastest, component of NPQ is high energy state quenching (qE). qE is activated by the formation of a pH gradient (ΔpH) between the thylakoid lumen and the chloroplast stroma, and involves protonation of PSII subunit S (PsbS) and the de-epoxidation of zeaxanthin from violaxanthin through the xanthophyll cycle (Pascal et al., 2005; Takizawa et al., 2007; Ruban et al., 2012).
An increase in light intensity results in more protons being transferred from the chloroplast stroma to the thylakoid lumen via photosynthetic electron flow and, as a result, the pH in the thylakoid lumen drops. Protonation of PsbS leads to conformational changes in LHCII, which in turn increase the amount of energy that is quenched as heat, preventing ROS overproduction and protecting PSII from photodamage (Henmi et al., 2004; Vass, 2012; Zavafer et al., 2015). Under fluctuating light conditions, changes in ΔpH are a transient signal for qE, forming and decaying within seconds. However, the interconversion of violaxanthin and zeaxanthin in response to changes in irradiance occurs over a longer timescale (minutes).
Cyclic electron transport (CET) is another regulatory process involved in photoprotection in plants (Finazzi and Johnson, 2016; Yamori et al., 2016; Yamamoto and Shikanai, 2019), which plays an important role in generating the ΔpH required to trigger qE (Suorsa et al., 2016; Nakano et al., 2019). In CET, electrons are transferred from ferredoxin back to the plastoquinone (PQ) pool, and subsequently to PSI through Cyt b6f and plastocyanin (PC). Thus, CET imports protons from the stroma to the lumen for ATP generation, but without net production of NADPH (Breyton et al., 2006; Joliot and Johnson, 2011). CET helps to balance the ATP:NADPH ratio under circumstances where the rate of consumption of reducing equivalents is reduced. In Arabidopsis, two main pathways for cyclic electron flow have been identified (Munekage et al., 2008; Shikanai, 2014). In the antimycin A-sensitive pathway, electrons are transferred from ferredoxin to PQ via a pathway involving the PGR5/PGRL1 complex (Munekage et al., 2002; DalCorso et al., 2008). In contrast, in the antimycin A-insensitive pathway, the electron transfer to the PQ pool is facilitated by NADH:plastoquinone oxidoreductase (NDH; Joliot and Johnson, 2011; Shikanai, 2014). For an extensive review of CET, see Nawrocki et al. (2019).
Of the two pathways of CET, the antimycin A-sensitive pathway has been particularly linked to PSI photoprotection under fluctuating conditions (Suorsa et al., 2012; Yamamoto and Shikanai, 2019). Studies in the pgr5 mutant of Arabidopsis show that PGR5 participates in photosynthetic control of Cyt b6f (Nandha et al., 2007), protecting PSI from photodamage under low/high light cycles (Suorsa et al., 2012, 2013; Yamamoto and Shikanai, 2019). In addition, PGR5 plays a role in the acceptor-side regulation of PSI. Accordingly, it was recently observed that over-expression of PGR5 in the C4 plant Flaveria bidentis, enhances the electron sink downstream of PSI, increasing photoprotection (Tazoe et al., 2020). The water-water cycle has also been suggested as a PSI photoprotective mechanism under fluctuating light conditions, although its activity is strongly species dependent (Huang et al., 2019b; Yang et al., 2020). In this cycle, stromal antioxidants enzymes catalyze ROS conversion into water (Asada, 1999), a process which was recently suggested to be more relevant for PSI photoprotection than CET in angiosperms (Sun et al., 2020).
In addition to qE, another important NPQ component in plants is qT, a form of quenching associated with state transitions (for a review see Minagawa, 2011). This process regulates the distribution of excitation energy between both photosystems, under conditions where the incident light favors the excitation of one over the other. To mitigate such changes, plants can adjust the energy excitation between PSII and PSI within minutes, by altering the distribution of light harvesting proteins between them. The imbalance in the excitation level of the photosystems is sensed through changes in the redox state of the PQ pool (Lemeille and Rochaix, 2010). In particular, reduction of the PQ pool and binding of PQH2 at the Qo site of the Cyt b6f , activates a specific kinase (STN7) that phosphorylates LHCII trimers (Wollman and Lemaire, 1988; Vener et al., 1995; Zito et al., 1999; Depège et al., 2003; Bellafiore et al., 2005). Phosphorylation induces LHCII detachment from PSII and partial (or total) attachment to PSI, triggering transition to State II (Kyle et al., 1983; Larsson et al., 1983). Meanwhile, when the PQ pool is oxidized, dephosphorylation of LHCII triggers the opposite phenomenon and transition to State I (Pribil et al., 2010; Shapiguzov et al., 2010). The signals produced from the redox state of the PQ pool are naturally transient, however, evidence shows a direct and rapid regulation of chloroplast gene expression in response to changes in PQ redox state (Pfannschmidt et al., 1999).
Although the classical view of state transitions has been associated with spectral changes in the quality of the incident light, thylakoid phosphorylation can also be triggered dynamically by changes in light intensity (Tikkanen et al., 2010; Grieco et al., 2012; Mekala et al., 2015). While under low white light intensity LHCII phosphorylation levels are maximal, under high light conditions LHCII phosphorylation is down-regulated and PSII core phosphorylation increases (Tikkanen et al., 2010). These opposite states do not change the relative excitation of PSII and PSI, but their regulatory function is related with the maintenance of an equal excitation pressure between both photosystems (Tikkanen et al., 2010). The main kinases and phosphatases involved in this phosphorylation pathway are STN7/STN8 and TAP38/PPH1, respectively; being their regulation particularly relevant under low light conditions (Tikkanen et al., 2010; Mekala et al., 2015). When light intensity increases, other regulatory mechanisms (such as NPQ) become more important for photoprotection (Tikkanen et al., 2010; Grieco et al., 2012). Under fluctuating light conditions, a role for STN7-dependent phosphorylation was also found in PSI photoprotection, through the maintenance of the redox stability of the electron transport chain (Grieco et al., 2012).
Both STN7 and STN8 are also capable of phosphorylating a range of proteins in the chloroplast (Schönberg et al., 2017), extending their involvement in the short-term response to fluctuating light onto further processes of acclimation. The regulatory pathways related with thylakoid protein phosphorylation have a key role in the photosynthetic responses to a changing environment, and their participation in the signals transduction pathway for acclimation needs further elucidation (reviewed by Grieco et al., 2016).
Ion homeostasis in the chloroplast is relevant to light sensing, not only due to its effect on enzymatic activity, but also due to its contribution to the regulation of the proton and electric potentials across the thylakoid membrane (Finazzi et al., 2015). Proton motive force (PMF), the driver of ATP synthesis, consists of two components: ΔΨ, the electrical potential gradient that is built due to ions moving in and out of the thylakoid lumen, and ΔpH. When proton concentration significantly increases in the thylakoid lumen, qE is activated, leading to the loss of energy as heat (Henmi et al., 2004; Vass, 2012; Zavafer et al., 2015). To maintain ATP production without promoting acidification of the lumen, which leads to NPQ activation, fluxes of counter ions (Cl− influx, Mg2+ and K+ efflux) regulate the ΔΨ component of the PMF (Carraretto et al., 2013; Armbruster et al., 2014; Herdean et al., 2016).
Finetuning ΔpH and ΔΨ to better suit different environmental conditions can facilitate fast modulation of photosynthetic activity under fluctuating light conditions. For instance, a transporter that has been linked to fast photosynthetic regulation in Arabidopsis is AtVCCN1, which transports Cl− ions into the chloroplast lumen (Herdean et al., 2016). Influx of Cl− ions into the lumen triggers an increase in the ΔpH/ΔΨ ratio, by decreasing H+ efflux from the thylakoid membranes, inducing a faster NPQ response under sudden increases in light intensities. By contrast, potassium influx to the lumen via the K+ antiport (KEA3), has been identified as an important factor in the transition from high to low light (Armbruster et al., 2014, 2016; Galvis et al., 2020). KEA3 transfers K+ into the lumen and H+ out to the chloroplast stroma, decreasing ΔpH but maintaining the ΔΨ necessary for ATP production. KEA3 activity accelerates NPQ relaxation during the transition to low light, leading to a fast recovery of CO2 assimilation (Armbruster et al., 2014).
Another K+ transporter, the two-pore K+ channel (TPK3), has been suggested to play a pivotal role in thylakoid ultrastructure organization and plant growth in Arabidopsis (Carraretto et al., 2013). TPK3 exports K+ and Ca+ ions, and is thought to modulate fast regulation of PMF to optimize photosynthetic activity under different light environments (Carraretto et al., 2013). However, recent results obtained by Höhner et al. (2019) showed that TPK3 is localized in the tonoplast and is not involved in photosynthetic regulation. These authors suggest the involvement of an as yet unknown additional K+ channel in photosynthetic acclimation to fluctuating light.
The light-induced enzymatic activation of the Benson-Calvin cycle was first discovered by Buchanan and colleagues in the 1960s, showing that CO2 fixation was activated by light (reviewed by Buchanan et al., 2002; Michelet et al., 2013). This light activation pathway, called the ferredoxin/thioredoxin (Fd/TRX) system, regulates carbon metabolic pathways through post-translational redox modifications (reviewed by Ruelland and Miginiac-Maslow, 1999; Lemaire et al., 2007; Michelet et al., 2013; Nikkanen et al., 2017). Thioredoxins (TRX) in the chloroplast are reduced mainly by ferredoxin (Fd, the PSI electron acceptor), via an enzyme called Ferredoxin-Thioredoxin reductase (FTR). Once reduced, TRX can reduce disulfide bonds in different stromal target proteins, placing the Fd/TRX at the crossroads between the “light” and “dark” reactions of photosynthesis (Ruelland and Miginiac-Maslow, 1999; Lemaire et al., 2007).
Activation of the Fd/TRX system will directly depend on the redox state of the chloroplast, meaning that changes in the photosynthetic electron flow will activate/deactivate different target enzymes under changing light regimes. This on/off switch acts as a significant regulatory process, leading to the adjustment of the carbon metabolism under different conditions. However, most studies on the regulatory role of Fd/TRX have been conducted under continuous light conditions, and research on their involvement in fluctuating light responses is limited (Collin et al., 2004; Nikkanen and Rintamäki, 2014; Geigenberger et al., 2017).
A study performed on the Arabidopsis knockout mutants trxm1/m2 showed the role of thioredoxins in the short-term responses to fluctuating light (Thormählen et al., 2017). Mutant plants showed alterations in the light activation of the enzyme malate dehydrogenase (MDH) and the malate/oxaloacetate (Mal/OAA) shuttle, a higher NPQ and a lower PSII quantum efficiency. This phenotype was only evident under fluctuating light conditions, with these alterations being more pronounced with increasing numbers of high-low light cycles. By contrast, no phenotypic differences were seen between the mutants and the WT plants under constant light.
Stomatal responses play a critical role in the availability of CO2 for carbon fixation, and it has been shown that stomatal dynamics limit photosynthesis under fluctuating light (Qu et al., 2016; Papanatsiou et al., 2019; De Souza et al., 2020; Kimura et al., 2020). Since stomatal responses are slower than photochemical and biochemical regulatory changes, a sudden change in light intensity could cause chloroplast CO2 concentration to decrease (Huang et al., 2015; Vialet-Chabrand et al., 2017). A decrease in CO2 concentration (and thus carbon fixation) implies that fewer electrons are being directed to the Benson-Calvin cycle, favoring the over-reduction of the electron transport chain and triggering ROS generation. At the same time, a decrease in CO2 availability also increases O2 binding to Rubisco, its oxygenase activity and photorespiration (Huang et al., 2015). Interestingly, it was recently shown that stomatal opening and closure dynamics can acclimate to different growth light regimes, anticipating future variations in light, and adjusting CO2 availability to the prevailing light condition (Matthews et al., 2018).
Photorespiration is a metabolic pathway that recycles 2-phosphoglycolate (2PG), a toxic product of the oxygenase activity of Rubisco, into 3-phospholycerate (3PGA; reviewed by Foyer et al., 2009; Bauwe et al., 2010; Eisenhut et al., 2019). This recycling requires several enzymatic steps that are distributed across three different organelles: the chloroplast, the peroxisome, and the mitochondrion. Although the photorespiratory pathway can represent a substantial loss of CO2 fixation, its involvement in photoprotection, nitrogen assimilation, and abiotic stress responses make it a crucial process for plants (reviewed by Foyer et al., 2009; Bauwe et al., 2010; Timm and Bauwe, 2013; Voss et al., 2013; Eisenhut et al., 2019). Nevertheless, its participation under fluctuating light conditions has not been extensively studied (Huang et al., 2015; Schneider et al., 2019). Huang et al. (2015) showed that, under fluctuating light conditions, a strong activation of the photorespiratory pathway allows the consumption of reducing equivalents, decreasing the reduction pressure of the electron transport chain and avoiding ROS generation. In addition, RuBP regeneration is also accelerated, favoring carbon fixation under these circumstances.
Acclimation to environmental fluctuations involves changes in gene expression and protein abundance, which result in the modification of the structure and composition of tissues. In particular, dynamic acclimation occurs in developed tissues, constrained by the existing structures, and involves processes or responses that take several days to be achieved. These responses might depend on the plant species and on the intensity and duration of the environmental fluctuation (Yin and Johnson, 2000). The processes involved in dynamic acclimation are not necessarily irreversible, and they will persist as long as the prevailing environmental condition is maintained. In the following sections, we will focus on the changes involved in the dynamic acclimation of photosynthesis under fluctuating light conditions (Figure 1).
When plants are grown at higher irradiances, they typically develop leaves with a high capacity for photosynthesis (see Walters, 2005). Fully developed leaves transferred from low to high light can also increase their photosynthetic capacity, typically over a period of a week (Athanasiou et al., 2010; Dyson et al., 2015). This acclimation response involves extensive changes across the whole of the leaf proteome, with marked increases in the concentration of Rubisco and other enzymes involved in the Benson-Calvin cycle, as well as down-stream enzymes involved in carbon assimilation (Miller et al., 2017).
Schneider et al. (2019) observed an upregulation of some Benson-Calvin cycle enzyme genes, such as fructose-1,6-bisphosphate aldolase 1 (FBA1) and sedoheptulose-1,7-bisphosphatase (SBSPASE), in Arabidopsis plants subjected to fluctuating light for 3 days. These enzymes were previously shown to participate in the regulation of the metabolic flux of carbon in plants (Lefebvre et al., 2005; Uematsu et al., 2012; Simkin et al., 2015, 2017). In addition, SBSPASE and FBA1 were also found to be regulated by the Fd/TRX system (Breazeale et al., 1978; Sahrawy et al., 1997; Dunford et al., 1998), suggesting a fine-tuning regulation of this long-term acclimation by a short-term mechanism. However, despite the increased activity and/or concentration of their Benson-Calvin enzymes, when compared to constant light conditions, plants under fluctuating light do not necessarily show an enhancement of their CO2 fixation capacity (Watling et al., 1997; Vialet-Chabrand et al., 2017; Schneider et al., 2019). Studies show that proteomic and transcriptomic changes in response to fluctuating light do not always align, suggesting a role of post-transcriptional regulations in the modulation of long-term acclimation responses (Athanasiou et al., 2010; Dyson et al., 2015; Miller et al., 2017; Schneider et al., 2019; Niedermaier et al., 2020).
Furthermore, as part of the acclimation response of carbon metabolism to fluctuating light, an increase in the expression of photorespiratory genes, and their corresponding protein content, was also observed in Arabidopsis (Schneider et al., 2019; Niedermaier et al., 2020). This metabolic response was shown to be particularly significant under high light fluctuation periods (Huang et al., 2015). Under low light fluctuating regimes, an increase in the photorespiratory pathway was deemed insignificant (Kono et al., 2014), possibly due to a lower accumulation of reducing equivalents.
In addition to metabolic alterations, changes in the thylakoid membrane protein composition play an important role in light acclimation (reviewed by Walters, 2005; Anderson et al., 2012; Kaiser et al., 2018; Johnson and Wientjes, 2020). For instance, plants grown under high light have been observed to have a lower PSII/PSI ratio, but higher concentrations of Cyt b6f and ATPase (reviewed by Evans, 1988; Eskins et al., 1991; Walters and Horton, 1994; Bailey et al., 2001; Walters, 2005). Furthermore, high light may also reduce the amount of LHCII and increase the chlorophyll a/b ratio, which is related with changes in light harvesting complexes concentration and photosystems ratio (Leong and Anderson, 1984; Yang et al., 1998; Bailey et al., 2001). By contrast, LHCII concentration increases when light exposure is limiting for plant growth, although under these conditions, a compensating decrease in PSII levels is also observed (Evans, 1988; Bailey et al., 2001). Some, but not necessarily all, of these responses are seen when plants are exposed to step changes in irradiance. In Arabidopsis, transfer from low to moderately high light resulted in an increase in Cyt b6f and ATPase, without measurable changes in chlorophyll content or the total amount of LHC proteins (Athanasiou et al., 2010; Miller et al., 2017).
In addition to acclimation to overall light intensity, when plants are exposed to different light qualities, the protein composition of the thylakoid membranes may also change (reviewed by Anderson et al., 1988). Long-term acclimation responses include changes in LHCII concentration, and Chl a/b and PSII/PSI ratio (Chow et al., 1990; Kim et al., 1993; Walters and Horton, 1995; Murchie and Horton, 1998). Such changes, occurring within days; help balance the electron transport rate under situations where either photosystem is preferentially excited by light. Importantly, these alterations differ from state transitions, which occur in seconds to minutes and do not include changes in thylakoid membrane composition, only re-distribution of LHCII and photosystem macro-organization.
Overall, when light intensity or quality change, plant acclimation responses tend to balance light absorption and assimilation (Rott et al., 2011; Yamori et al., 2011). Nevertheless, understanding the effect of fluctuating light in natural environments is far more complex. Sunflecks will have a direct impact on light intensity, but natural shade cast by vegetation will also affect the incident light quality. Thus, natural light fluctuations in the ecosystems result in complex inputs, which might induce contradictory output responses. For instance, the PSII/PSI ratio will change in opposite directions with a decrease in light intensity or with exposure to a high far-red/red ratio, conditions that can be imposed by vegetative shading (Murchie and Horton, 1998; Bailey et al., 2001). Consequently, predicting a thylakoid membrane specific response to fluctuating light in natural environments is not an easy task. Furthermore, many of these responses are species-dependent (Murchie and Horton, 1998; Yin and Johnson, 2000).
Similar to what happens following sudden increases in light intensity, frequent exposure to oscillating periods of high light induces over-reduction of the electron transport chain, triggering an increase in ROS production and photoinhibition (Shimakawa and Miyake, 2018; Huang et al., 2019a). Thus, long-term acclimation to fluctuating light can also involve an enhancement of photoprotective mechanisms. For instance, Schneider et al. (2019) observed an up-regulation of H2O2 scavenging enzymes, such as glutathione peroxidase (GPX7) and catalase (CAT2), in Arabidopsis leaves exposed to 3 days of fluctuating light. In agreement, these plants also increased their ascorbate pool size, indicating an improvement in ROS scavenging and antioxidant response (Schneider et al., 2019).
Fluctuating light can increase PSBS content and the concentration of pigments of the xanthophyll cycle, leading to a strengthening of the photoprotective capacity of NPQ (Barker et al., 1997; Niinemets et al., 1998; Alter et al., 2012; Caliandro et al., 2013). This acclimation response was demonstrated to be, at least partially, regulated at the transcriptional level (Schneider et al., 2019). The relevance of NPQ as a long-term acclimation response to fluctuating light was recently shown using tobacco transgenic lines overexpressing PSBS and zeaxanthin epoxidase (ZEP) and violaxanthin de-epoxidase (VDE), the key enzymes in the xanthophyll cycle (Kromdijk et al., 2016). These plants showed a higher CO2 assimilation compared to the WT, leading to a higher dry mass accumulation under field conditions.
In addition to NPQ, genes related to CET were also upregulated in response to fluctuating light (Schneider et al., 2019). Proteomic results obtained under the same conditions, and by the same authors, suggest a specific role of the NDH-like complex in this long-term acclimation mechanism (Niedermaier et al., 2020). Nevertheless, mutants lacking PGR5 were shown to suffer strong PSI photoinhibition under fluctuating light (Suorsa et al., 2012; Kono and Terashima, 2016), meaning that the involvement of the antimycin A-sensitive CET pathway in this acclimation process cannot be ruled out. It is worth mentioning that a higher CET flux will contribute to a higher ΔpH, facilitating NPQ generation under photoinhibitory conditions (Munekage et al., 2002). Thus, the involvement of CET in this long-term acclimation response will not only avoid PSI photoinhibition, but also increase thermal dissipation. Consequently, short-term responses to sudden increases in light intensity are also being improved by this long-term response.
Short-term input responses, such as redox changes in the photosynthetic apparatus, occur on very rapid timescales (μsec-min), close to those of the natural fluctuations of the light environment. These inputs may trigger cellular changes, such as protein phosphorylation or thiol reductions, which respond more slowly to the changing conditions (minutes). Nevertheless, all these inputs are transient, and so, their putative role in signaling for long-term plant acclimation, which occurs over days, is not obvious. The concentrations of metabolites can also change on rapid timescales, in direct response to changing light conditions; but in some cases their accumulation provides the potential to generate signals which average out the short-term fluctuations in the environment. In the following section, we will address some of the most discussed pathways participating in photosynthetic acclimation to fluctuating light in plants (Figure 2).
Figure 2. Signals transduction pathways of long-term acclimation to fluctuating light. Schematic representation of the different putative signaling pathways involved in photosynthetic acclimation to fluctuating light, as described in section Signals Transduction Pathways for Long-Term Acclimation to Fluctuating Light. Colored gradient triangles depict accumulation of a metabolite or enzyme. LHCII, Light Harvesting Complex II; PSII, Photosystem II; PSI, Photosystem I; Fd, Ferredoxin; PQ, Plastoquinone; PQH2, Plastoquinol; STN7, STN7 kinase; CSK, Chloroplast Sensor Kinase; SnRK1, SNF1-related kinase 1 β-CC, β-cyclocitral; PAP, 3′-phosphoadenosine 5′-phosphate; MEcPP, methylerythritol cyclodiphosphate; TPT, Triose-phosphate transporter; GTP2, glucose 6-phosphate/phosphate translocator; G6P, glucose-6-phosphate; T6P, trehalose-6-phosphate; F6P, fructose-6-phosphate; S6P, sucrose-6-phosphate; F1,6BP, fructose-1,6-biphosphate; TPS, Trehalose phosphate synthase; HXK, Hexokinase; Dea/Act, Deactivation/Activation. Created with BioRender.com.
Retrograde signals originating from photosynthesis have been studied extensively, aiming to describe chloroplast-nucleus communication and regulation of gene expression (Pfannschmidt et al., 1999; Fey et al., 2005b; Wilson et al., 2006; Foyer et al., 2012; Karpiński et al., 2013; Gollan et al., 2015; Matsubara et al., 2016; Leister, 2019). Within these signaling pathways, the participation of ROS originating from photosynthetic electron flow, has been widely discussed (Pfannschmidt et al., 2009; Ramel et al., 2012; Szechyńska-Hebda and Karpiński, 2013; Kim, 2020). For instance, H2O2 can diffuse from the chloroplast, creating a signal cascade ultimately affecting nuclear gene expression (Maruta et al., 2012). Meanwhile, oxidation of β-carotene by 1O2 has been shown to lead to the formation of the volatile compound β-cyclocitral (β-CC), which triggers changes in nuclear gene expression under stress conditions (Ramel et al., 2012; Havaux, 2014; Tian, 2015). Nevertheless, the specific factors participating in this ROS-related signal transduction pathways are still far from being completely elucidated (reviewed by Leister, 2019; Kim, 2020).
In addition, we can think the chloroplast as an environmental sensor for plants, with the redox state of the electron transport chain being a key gear for sensing fluctuations in the environment. Amongst the components of the electron transport chain, the involvement of the PQ pool in retrograde signaling has been suggested, facilitating a rapid physiological response to changing light conditions (El Bissati and Kirilovsky, 2001; Fey et al., 2005a; Bräutigam et al., 2009). Different proteins have been shown to be regulated by the redox state of PQ, and in a ROS-independent manner (Adamska and Kloppstech, 1991; Kimura et al., 2003; Yabuta et al., 2004), and a link between the redox state of the PQ pool and photosystem gene expression has even been observed (Pfannschmidt et al., 1999; El Bissati and Kirilovsky, 2001).
Although it is clear that ROS retrograde signaling and chloroplast redox changes participate in light acclimation, it is still not yet understood how these signals are integrated under fluctuating light. The short lifetime of these chloroplast redox changes imply that other factors might be integrating the redox variations over time. Puthiyaveetil et al. (2008) suggested that a key protein participating in retrograde signaling is the Chloroplast Sensor Kinase (CSK) (Figure 2; Puthiyaveetil et al., 2008). This protein is widely seen in photosynthetic organisms and has been shown to bind an iron-sulfur cluster, which can sense changes in the chloroplast redox state (Ibrahim et al., 2020). The activation/deactivation of the kinase regulates the expression of different photosynthetic genes (Puthiyaveetil et al., 2008; Ibrahim et al., 2020), supporting its participation in the adjustment of the stoichiometry of photosynthetic complexes under different light conditions. Interestingly, CSK gene expression was found to be upregulated under fluctuating light in Arabidopsis (Schneider et al., 2019).
The STN7 kinase phosphorylates LHCII and some PSII subunits upon changes in light conditions, triggering state transitions and regulating PSII turnover (Bellafiore et al., 2005; Tikkanen et al., 2006, 2010; Wagner et al., 2008; Pietrzykowska et al., 2014). Although STN7 involvement in short-term regulatory responses to fluctuating light has been well-described, it was also shown that stn7 mutants are unable to undergo forms of long-term acclimation under changing light regimes (Bonardi et al., 2005; Pesaresi et al., 2009). When stn7 mutants were grown under fluctuating light they exhibited reduced growth and a lower seed yield and were incapable of adjusting their thylakoid composition to the conditions experienced. In agreement, STN7 gene expression was increased after 3 days of fluctuating light in Arabidopsis (Schneider et al., 2019), again highlighting its potential involvement in long-term acclimation. Thus, STN7 seems to play a critical role in both short- and long-term responses to fluctuating light regimes in plants, either directly or indirectly (Bonardi et al., 2005; Wagner et al., 2008; Bräutigam et al., 2009; Leister, 2019).
Although it is still unknown which proteins participate downstream of STN7 in the long-term acclimation response, one of the putative proteins is TSP9. TSP9 is a plant specific nuclear-encoded protein, found in the thylakoid membranes, which is phosphorylated by STN7 upon illumination (Carlberg et al., 2003; Zer and Ohad, 2003). The phosphorylated form dissociates from the thylakoid membrane and has been proposed to act as a signaling molecule regulating gene expression under changing light conditions (Carlberg et al., 2003; Zer and Ohad, 2003; Fristedt et al., 2009). Nevertheless, downregulation of TSP9 in Arabidopsis plants did not affect the long-term response to light changes (Pesaresi et al., 2009), but its mutation was shown to affect state transitions and NPQ (Fristedt et al., 2009). These results suggest the involvement of TSP9 in the short-term responses to fluctuating light, but not in long-term acclimation. The downstream factors involved in the STN7 long-term acclimation signaling pathway, are still elusive (Leister, 2019).
A putative role for signal integration was suggested for the volatile compound β-cyclocitral (β-CC; Ramel et al., 2012; Havaux, 2014; Tian, 2015); the accumulation of 3′-phosphoadenosine 5′-phosphate (PAP) and its regulation by the SAL1 phosphatase (Estavillo et al., 2011; Chan et al., 2016); and the isopropanoid precursor methylerythritol cyclodiphosphate (MEcPP; Xiao et al., 2012). The accumulation of chloroplast tetrapyrrole biosynthesis intermediates was also suggested to be involved in retrograde signaling (reviewed by Nott et al., 2006; Tabrizi et al., 2016), although this model has been questioned (Mochizuki et al., 2008; Moulin et al., 2008).
The accumulation of these metabolites is directly connected to an increase in ROS production and/or redox changes in the chloroplast (Figure 2), and its involvement in the acclimation response to different stress conditions has been described (Estavillo et al., 2011; Ramel et al., 2012; Xiao et al., 2012; Chan et al., 2016). Thus, it is feasible to think that a putative increase in their accumulation over a certain threshold might also trigger an acclimation response to fluctuating light, allowing the integration of different signals over time. This hypothesis is an interesting starting point to close the gap between the short and long-term responses to this environmental condition.
The significance of carbon metabolites as signals for acclimation relies on the fact that photosynthate partitioning varies with changes in irradiance and photoperiod (Mengin et al., 2017). Furthermore, different studies support a role for the accumulation of some of these carbon sinks in the plant's response to several environmental conditions. For instance, sucrose shows a significant role in cold acclimation, triggering changes in gene expression and anthocyanin biosynthetic pathways (Solfanelli et al., 2006; Rekarte-Cowie et al., 2008). In addition, evidence supports a sucrose signaling role in many developmental processes in the plant's life cycle (reviewed by Horacio and Martinez-Noel, 2013).
Sucrose concentrations in plant tissues correlate with light intensity, and their synthesizing enzymes fluctuate over the photoperiod (Cheikh and Brenner, 1992; Horacio and Martinez-Noel, 2013). In addition, sucrose downregulates CO2 fixation through alteration of gene expression (Pamplin and Chapman, 1975; Sheen, 1990; Rook et al., 1998; Wiese et al., 2004), and through a negative feedback regulation due to inorganic phosphate (Pi) availability (Hurry et al., 2000; Ensminger et al., 2006). Sucrose synthesis and degradation participate in Pi cycling between the chloroplast and the cytosol (Hurry et al., 2000). Alterations in sucrose synthesis in the cytosol may decrease Pi availability in the chloroplast, inhibiting ATP synthesis and, as a consequence, RuBP regeneration and carbon fixation (Hurry et al., 2000; Ensminger et al., 2006).
Due to its constitutive presence in the cytosol of plant cells (Figure 2), a role for sucrose as a signaling molecule in photosynthetic acclimation may imply that its accumulation needs to exceed a certain threshold (Horacio and Martinez-Noel, 2013). Alter et al. (2012) analyzed the concentration of soluble sugars (glucose, fructose, and sucrose) in Arabidopsis plants under constant and fluctuating light conditions, without observing any differences in total soluble sugar concentration between treatments. Nevertheless, the individual concentration of each sugar was not independently assessed, and changes in sugar ratios under fluctuating light cannot be ruled out.
Photosynthesis, through the Benson-Calvin cycle, produces glycerate-3-phosphate, which is reduced to triose-phosphate (triose-P) in successive reactions that consume NADPH and ATP. Triose-P can be used to regenerate ribulose-1,5-bisphosphate (RuBP) in the Benson-Calvin cycle, or, when in excess, can be transformed to end products such as sucrose or/and starch (Figure 2; reviewed by Ensminger et al., 2006). Sucrose is synthesized in the cytosol, for which triose-P is exported from the chloroplast through the triose-phosphate translocator (TPT; Figure 2). When the rate of triose-P export is lower than its rate of synthesis, starch is synthesized in the chloroplast (Zeeman et al., 2004). Carbon flux to starch is also an important strategy to avoid carbon sink limitations under photoinhibitory conditions (Ensminger et al., 2006).
Fixed carbon may also be imported back from the cytosol in the form of glucose-6-phosphate (G6P), through the glucose 6-phosphate/phosphate translocator GPT2 (Figure 2; Niewiadomski et al., 2005; Dyson et al., 2015). GTP2 expression is known to be associated with alterations in carbon metabolism and high light responses, leading to photosynthetic acclimation (Athanasiou et al., 2010; Kunz et al., 2010; Dyson et al., 2015). GTP2 might directly affect the relative concentrations of G6P between cell compartments, affecting the metabolic signals triggering photosynthetic responses to different environmental stimulus. For instance, G6P positively regulates sucrose synthesis, by activating SPS and inhibiting sucrose synthase (SUS- an enzyme participating in sucrose catabolism). This inhibition in sucrose degradation is through inhibition of SNF1-related kinase 1 (SnRK1), which participates in the regulation of carbon metabolism, ABA signaling, stress responses and development (Jossier et al., 2009; Zhang et al., 2009; Cho et al., 2012). Thus, regulating the G6P concentration in the cytosol might have a direct impact on metabolism, growth and acclimation under different environmental conditions.
In a similar way to G6P, trehalose-6-phosphate (T6P) has been described as having an important role in the regulation of carbon assimilation and sugar status in plants (reviewed by Ponnu et al., 2011). T6P is an intermediate in trehalose biosynthesis, synthesized from UDP-Glucose and G6P in the cytosol, by the enzyme trehalose phosphate synthase (TPS; Figure 2; Häusler et al., 2014). T6P is a signal of sucrose availability, alters the rate of starch biosynthesis in the chloroplast and participates in the cross-talk of metabolic regulations through inhibition of SnRK1 (Lunn et al., 2006; Zhang et al., 2009; Yadav et al., 2014). In particular, T6P was also shown to down-regulate genes related to the photosynthetic process, which are normally up-regulated by SnRK1 (Zhang et al., 2009).
Other proposed sugar sensing molecules are the hexokinases (HXKs), which catalyze the phosphorylation of glucose and fructose and have been defined as evolutionarily conserved glucose sensors (Figure 2; reviewed by Granot et al., 2014). HXKs are able to down-regulate the expression of photosynthetic genes, reduce chlorophyll levels and photosynthetic rates (Jang et al., 1997; Dai et al., 1999; Xiao et al., 2000). As a consequence, HXKs are capable of modulating photosynthesis in a glucose dependent-manner, integrating short-term changes in the environment with their corresponding photosynthetic responses (Moore et al., 2003). In agreement, within guard cells, HXK also regulates stomatal closure, supporting a negative coordinated regulation of photosynthesis by hexose availability (Kelly et al., 2013; Granot et al., 2014).
Our review of the literature shows that experimental studies that link rapid responses to sustained long-term changes are rare, as they are laborious and often technically infeasible. Mathematical modeling, however, has the potential to overcome some of these limitations, helping to identify mechanisms by which plants integrate short-term responses to the environment over time. A holistic understanding of fluctuating light acclimation is a challenge, involving many timescales. The following section covers a range of mathematical modeling techniques that have previously been applied to study photosynthesis (Table 1), and further proposes new modeling techniques that could be employed to deepen our understanding of photosynthetic acclimation to fluctuating light in plants.
Table 1. A brief overview of the primary types of models applied to study photosynthetic responses to fluctuating light.
Mathematical modeling within biology is ruled by two paradigms: empirical modeling and mechanistic modeling. Empirical modeling, also known as statistical modeling, fits a model to the data without considering the underlying biological processes (Table 1). Through the observation of a repeated pattern, it is assumed that future events of the same type will result in the same pattern. A straightforward example of an empirical model is the non-rectangular hyperbola of net carbon gas exchange fitted to light response curves (Johnson and Murchie, 2011). While the fit of this model has stood the validation test of time, possible underlying biological mechanisms have only recently been discussed (Retkute et al., 2015; Herrmann et al., 2020). Stegemann et al. (1999) constructed an empirical model relating fluctuating diurnal changes in light intensity to net photosynthesis. By fitting their model parameters to data obtained from two different tree species, they successfully estimated carbon uptake without explaining the mechanisms behind their photosynthetic responses to light.
Empirical models are a powerful tool when the underlying processes are not known and form the premise of machine learning algorithms (Kotsiantis et al., 2007; Angelov and Gu, 2019). The reliability of empirical models improves vastly with the amount of input data available (Kotsiantis et al., 2007), a limitation that is becoming less hindering in the current ‘omics era. Nonetheless, extrapolation of empirical models is difficult and, typically, good predictions cannot be made outside of the range of previously measured values. For example, an empirical model of photosynthesis with parameters fitted to a specific light and temperature regime is unlikely to be transferable to another light and temperature regime, and, instead, the model parameters must be estimated anew (Herrmann et al., 2020).
By contrast, mechanistic models, albeit harder to construct, have several advantages over empirical models (Table 1). Mechanistic models break down a system into smaller components, and the processes by which these components interact with one another are then captured by mathematical equations. Mechanistic models require an in-depth understanding of the system components and their interactions in space and time. However, once a mechanistic model is constructed and its parameters are successfully estimated, few input data are required for outcome prediction. Furthermore, if the same mechanisms apply under different conditions, or outside the range of the initial input values, the model can be applied beyond the range of the initial training data (Geritz and Kisdi, 2012; Ratti, 2018).
Kinetic models of metabolic pathways are examples of mechanistic models: a pathway is broken down into its metabolites and the way in which these metabolites interact with one another can, for example, be described by mass action law or Michaelis-Menten kinetics (Schallau and Junker, 2010). Many successful kinetic models of photosynthesis have been built, and their ability to capture fluctuating light conditions is discussed in the next section. Kinetic models, as with all others mechanistic models, are limited by our knowledge of the system under study.
Dynamic models generally encompass time-dependent-models that capture changes over time (Table 1). These models employ a set of ordinary differential equations, or partial differential equations, considering one or more independent variables. Dynamic models can be empirical or mechanistic; however, in biochemistry, dynamic models appear most commonly in the form of kinetic models. Kinetic models of biochemical pathways are mechanistic, dynamic models, as they consider changes in metabolite concentrations over time. Due to a combinatorial explosion of the parameter estimation, dynamic models are generally limited to a handful of equations.
Multiple dynamic models of photosynthesis and the Benson-Calvin cycle reactions exist (Farquhar et al., 1980, 2001; Harley and Tenhunen, 1991; Poolman et al., 2000); however, most of them have not been applied to study fluctuating light acclimation. Kirschbaum et al. (1997) and Pearcy et al. (1997) are among the few to have extended the original Farquhar et al. (1980) model to study fluctuating light regimes over a time frame of seconds to hours. Mott and Woodrow (2000), however, addressed the question of nitrogen resource allocation under fluctuating light regimes by using a much simpler model of rubisco and rubisco activase. In addition, Porcar-Castell et al. (2006) incorporated both regulatory and feedback mechanisms in their dynamic model of PSII, and were able to validate experimentally obtained photochemical and non-photochemical quantum yields under fluctuating light. However, none of these models consider a long-term acclimation of plants to changing light regimes, as they have been parametrized for a much shorter timescale.
Retkute et al. (2015) employed a semi-empirical dynamic model to describe carbon uptake over time as a function of light availability and a constant maximum photosynthetic capacity (Pmax). Pmax is calculated to give the maximum possible carbon uptake over the time-weighted average of a light pattern, representing the acclimation state of the plant. How plants shift from one acclimation state to another, and alter their Pmax accordingly, was discussed by Herrmann et al. (2020) using a time- and temperature-dependent model. However, the sensors and signals that trigger a new photosynthetic acclimated state remain elusive.
As discussed in section Signals Transduction Pathways for Long-Term Acclimation to Fluctuating Light, and highlighted in our previous studies (Dyson et al., 2015; Herrmann et al., 2020), carbon fluxes between the cytosol and the chloroplast seem to be important factors in the photosynthetic acclimation responses of plants. In particular, the resulting changes in sugar vs. starch production have been shown to be crucial for acclimation to different light regimes (Dyson et al., 2015). Modeling the changes in carbon metabolism under fluctuating light could be useful to identify key signals leading to acclimation responses in plants. For instance, using a simple kinetic model and a sensitivity analysis of the model parameters, Nägele and Weckwerth (2014) analyzed the control of sugar homeostasis in plants, and suggested that allosteric effectors alone can account for a considerable readjustment of metabolic homeostasis.
Metabolic control analysis (MCA) quantifies the extent to which fluxes, or concentrations, depend on the model parameters. Thus, when applied to kinetic models, MCA provides a valuable tool for identifying parameters, and thus enzymes, that exert the greatest metabolic control over the fluxes (or species concentrations) in a defined model (ap Rees and Hill, 1994; Poolman et al., 2000). The fact that detailed regulatory information can be included in these dynamic kinetic models represents one of their greater advantages; although at the same time limits the size and complexity over which they can be feasibly solved. Often, a trade-off between the level of mechanistic detail and the feasibility to solve the model is required (Harley and Tenhunen, 1991).
Photosynthetic acclimation to a sustained change in light regime typically occurs over multiple days. As Athanasiou et al. (2010) observed, a new photosynthetic state is reached only 1 week after a change in exposure from low to high light. Acclimation responses to changes in light regimes are typically not represented by kinetic models, which tend to be parametrized over a timescale of seconds to hours. Instead, genome-scale steady-state models which tend to operate over multiple days and weeks can be used to study photosynthetic acclimation (Table 1; Herrmann et al., 2019a).
Genome-scale, steady-state, metabolic models, employ what are known as constraint-based modeling (CBM) techniques (Lewis et al., 2012), and operate under the assumption that internal metabolite concentrations are constant over time. This approximation is generally valid over longer time-frames, because the changes in metabolic flux leading to new equilibrium states are usually faster when compared to acclimation responses. Whilst these types of models are able to capture the final acclimated steady-state of plant metabolism, they fail to incorporate the mechanisms that initiate and lead to that new steady-state.
More recent variations of CBM techniques aim to overcome these limitations by employing dynamic CBM techniques (Mahadevan et al., 2002; Grafahrend-Belau et al., 2013). Shaw and Cheung (2018), for example, built a dynamic multi-tissue model by using the output of one steady-state model as the input of another steady-state model. By defining a steady-state model at each time point, they were able to effectively analyse resource allocation in plants over days and weeks. The model by Shaw and Cheung (2018) is based on the diel model first published by Cheung et al. (2014), which combined both a day-time (light-dependent) steady-state model and a night-time (light-independent) steady-state model. Dynamic models constructed of steady-state models are thus able to incorporate time-dependent changes, but typically consider changes over a timescale of multiple days.
Different ‘omics datasets can be used to incorporate enzyme regulatory mechanisms into genome-scale stoichiometric models, making it, for instance, possible to study redox changes at a resolution of second to hours with longer process steady-state models (Jamshidi and Palsson, 2010). This kind of analysis, however, has yet to be applied to plants exposed to fluctuating light.
Incorporating data measured over different timescales, and both discrete (e.g., state switching) and continuous scales (e.g., sink metabolite accumulation), poses an immense challenge for the study of photosynthetic acclimation under fluctuating light conditions. In order to incorporate models describing different processes over different periods of times, dimensionality reduction techniques will be necessary. These techniques are designed to reduce model complexity by discarding components that have little effect on the overall outcome of interest (Hummer and Szabo, 2015; Snowden et al., 2017). Successful dimensionality reduction should lead to the identification of essential model components required for predictive power; each fine-tuned according to the timescale over which it must operate, and the magnitude for which can shows significant effects on the system itself.
Purvis et al. (2009) use dimensionality reduction to combine multiple small-scale kinetic models in the human platelet P2Y1 signaling system, and convert it into a single holistic model. Employing known dimensionality reduction techniques (Hummer and Szabo, 2015; Ali Eshtewy and Scholz, 2020) on fast existing kinetic models of photosynthesis, may pave the way for their incorporation into slower process models (such as the genetic changes involved in light acclimation). Unfortunately, neither dimensionality reduction nor model validation techniques are frequently employed in plant sciences. Model validation, both at the experimental and theoretical level (Hasdemir et al., 2014), will need to be done before a model is deemed suitable to be integrated holistically.
Hybrid models, which incorporate both discrete and continuous information, are starting to gain attention in other disciplines (Henzinger, 2000; Bortolussi and Policriti, 2008). A simple analogy for such a hybrid automaton is a thermostat, whereby the law of thermodynamics are described by ordinary differential equations (continuous) but the state of the heater is either on or off (discrete). Thus, we can imagine multiple metabolite concentrations changing on a continuous scale in response to environmental fluctuations, which could emerge in an on/off output response (such as the ones described in sections Signals Transduction Pathways for Long-Term Acclimation to Fluctuating Light and System Outputs: Long-Term Acclimation to Fluctuating Light of the present review, respectively). However, such a system has yet to be identified in plant acclimation to fluctuating light.
The alternative to the mechanistic approaches described above, would be to take an empirical modeling approach. With an ever-increasing and overwhelming amount of multi-omic data available, there are numerous supervised learning algorithms that could be applied to identify “biomarkers” of a given acclimation stage (Mjolsness and DeCoste, 2001; Saeys et al., 2007). While the identification of molecular predictors is promising, these approaches typically do not reveal any information about the mechanisms by which the identified molecules trigger the final acclimated state of the plant. This empirical approach, however, does hold the potential for validating existing hypotheses or generating new hypotheses for experimental validation. For example, if both STN7 and TSP9 were identified as predictors for light acclimation, this would support the idea that TSP9 acts downstream of STN7 in promoting a long-term acclimation response.
Finally, stochastic models can account for random variations in inputs and result in a probability distribution of potential outcomes (Table 1; Guerriero et al., 2014; Retkute et al., 2018). The application of stochastic models to photosynthesis remains limited as of today but holds a great potential for identifying potential thresholds for acclimation. For instance, one could imagine a metabolite concentration that fluctuates in response to environmental changes triggering an acclimation process only once a given threshold concentration is passed. This could be the case of the proposed ROS and carbon-related metabolites discussed above (see section Signals Transduction Pathways for Long-Term Acclimation to Fluctuating Light).
To facilitate the integration of different modeling techniques, rigorously standardized tools are required. For instance, the open platform www.e-photosynthesis.org hosts a collection of dynamic plant models, translated to the Systems Biology Mark-up Language (SBML), and provides a good starting point for any modeler interested in photosynthetic acclimation. Currently, the project consists largely of model parametrized over short timescale, but hopefully it will be extended to longer-time physiological processes in the future, such that an effective integration of the two will be possible.
As this review has shown, there is a gap between studies that consider short-term responses to changes in light conditions, and those that consider long-term acclimation processes. This gap is evident from both an experimental and a theoretical viewpoint, and is likely the result of the difficulties associated with studying interconnected processes that occur over different timescales. The photosynthetic apparatus is highly complex; thus, understanding the regulatory networks of fluctuating light responses over time will require a deeper understanding of the system itself. This situation is even more complex if we consider that many experimental studies focus on well-defined, non-random changes in irradiance that do not necessarily reflect realistic field conditions (Annunziata et al., 2017).
It is also worth mentioning that, in the present review, we mainly describe how changes in light regimes affects processes at a single-cell level. Under field conditions, as captured in canopy level models, fluctuating light often result in heterogeneous light absorbance across leaves and cells. How these differences at the cellular level are integrated within and across tissues remains an important topic for further consideration in the future, given that this heterogeneity may result in emergent properties that cannot be captured by single-cell models. Emergent properties are those which arise from an interaction of model components, and which cannot be described by either of the components on their own (Bhalla and Iyengar, 1999; Peak et al., 2004; Aderem, 2005). Emergent properties are the reason why it is often difficult to explain the mechanistic basis of empirical models, and are why both approaches are needed to enhance our understanding of photosynthetic acclimation to fluctuating light conditions.
It is well-established that realistic models of complex biological signals will require regulation, feedback signals and non-linear dynamic components (Csete and Doyle, 2002). Identifying potential emergent properties of such systems will require the integration and careful dimensionality reduction of multiple processes (Rascher and Nedbal, 2006). Models will need to be specific enough to capture the individual processes that together lead to emergent system properties but, at the same time, need to be general enough to differentiate noise from signal (Gillespie, 2000; Mélykúti et al., 2010). As a conclusion, a combination of existing and emerging modeling techniques will be required to capture the emergent properties and signaling pathways related to photosynthetic acclimation to fluctuating light in plants.
PC, AG, and GJ contributed mainly to the sections System Inputs: Short-Term Responses to Fluctuating Light, System Outputs: Long-Term Acclimation to Fluctuating Light, and Signals Transduction Pathways for Long-Term Acclimation to Fluctuating Light of the present review. HH and J-MS contributed mainly to section Metabolic Modeling in Unraveling the Photosynthetic Acclimation to Fluctuating Light in Plants. All authors co-wrote and approved the manuscript.
This work was support by BBSRC studentships to AG and HH (BB/M011208/1) and BBSRC research grants to GJ and J-MS (BB/J04103/1 and BB/S009078/1).
The authors declare that the research was conducted in the absence of any commercial or financial relationships that could be construed as a potential conflict of interest.
The authors would like to thank Dr. Beata Czajkowska, Mr. Josef Oliver, and Ms. Norazreen Binti Abd Rahman (University of Manchester) for useful discussions.
Adamska, I., and Kloppstech, K. (1991). Evidence for an association of the early light-inducible protein (ELIP) of pea with photosystem II. Plant Mol. Biol. 16, 209–223. doi: 10.1007/BF00020553
Aderem, A. (2005). Systems biology: its practice and challenges. Cell 121, 511–513. doi: 10.1016/j.cell.2005.04.020
Ali Eshtewy, N., and Scholz, L. (2020). Model reduction for kinetic models of biological systems. Symmetry 12:863. doi: 10.3390/sym12050863
Alter, P., Dreissen, A., Luo, F.-L., and Matsubara, S. (2012). Acclimatory responses of Arabidopsis to fluctuating light environment: comparison of different sunfleck regimes and accessions. Photosynth. Res. 113, 221–237. doi: 10.1007/s11120-012-9757-2
Anderson, J. M., Chow, W. S., and Goodchild, D. J. (1988). Thylakoid membrane organisation in sun/shade acclimation. Funct. Plant Biol. 15, 11–26. doi: 10.1071/PP9880011
Anderson, J. M., Horton, P., Kim, E. H., and Chow, W. S. (2012). Towards elucidation of dynamic structural changes of plant thylakoid architecture. Philos. Trans. R. Soc. B Biol. Sci. 367, 3515–3524. doi: 10.1098/rstb.2012.0373
Angelov, P. P., and Gu, X. (2019). Empirical Approach to Machine Learning. Cham: Springer. doi: 10.1007/978-3-030-02384-3
Annunziata, M. G., Apelt, F., Carillo, P., Krause, U., Feil, R., Mengin, V., et al. (2017). Getting back to nature: a reality check for experiments in controlled environments. J. Exp. Bot. 68, 4463–4477. doi: 10.1093/jxb/erx220
ap Rees, T., and Hill, S. A. (1994). Metabolic control analysis of plant metabolism. Plant Cell Environ. 17, 587–599. doi: 10.1111/j.1365-3040.1994.tb00151.x
Armbruster, U., Carrillo, L. R., Venema, K., Pavlovic, L., Schmidtmann, E., Kornfeld, A., et al. (2014). Ion antiport accelerates photosynthetic acclimation in fluctuating light environments. Nat. Commun. 5, 1–8. doi: 10.1038/ncomms6439
Armbruster, U., Leonelli, L., Correa Galvis, V., Strand, D., Quinn, E. H., Jonikas, M. C., et al. (2016). Regulation and levels of the thylakoid K+/H+ antiporter KEA3 shape the dynamic response of photosynthesis in fluctuating light. Plant Cell Physiol. 57, 1557–1567. doi: 10.1093/pcp/pcw085
Asada, K. (1999). The water-water cycle in chloroplasts: scavenging of active oxygens and dissipation of excess photons. Annu. Rev. Plant Biol. 50, 601–639. doi: 10.1146/annurev.arplant.50.1.601
Athanasiou, K., Dyson, B. C., Webster, R. E., and Johnson, G. N. (2010). Dynamic acclimation of photosynthesis increases plant fitness in changing environments. Plant Physiol. 152, 366–373. doi: 10.1104/pp.109.149351
Awad, J., Stotz, H. U., Fekete, A., Krischke, M., Engert, C., Havaux, M., et al. (2015). 2-cysteine peroxiredoxins and thylakoid ascorbate peroxidase create a water-water cycle that is essential to protect the photosynthetic apparatus under high light stress conditions. Plant Physiol. 167, 1592–1603. doi: 10.1104/pp.114.255356
Bailey, S., Walters, R. G., Jansson, S., and Horton, P. (2001). Acclimation of Arabidopsis thaliana to the light environment: the existence of separate low light and high light responses. Planta 213, 794–801. doi: 10.1007/s004250100556
Barker, D. H., Logan, B. A., Adams, W. W. III., and Demmig-Adams, B. (1997). The response of xanthophyll cycle-dependent energy dissipation in Alocasia brisbanensis to sunflecks in a subtropical rainforest. Funct. Plant Biol. 24, 27–33. doi: 10.1071/PP96059
Bauwe, H., Hagemann, M., and Fernie, A. R. (2010). Photorespiration: players, partners and origin. Trends Plant Sci. 15, 330–336. doi: 10.1016/j.tplants.2010.03.006
Bellafiore, S., Barneche, F., Peltier, G., and Rochaix, J.-D. (2005). State transitions and light adaptation require chloroplast thylakoid protein kinase STN7. Nature 433, 892. doi: 10.1038/nature03286
Bhalla, U. S., and Iyengar, R. (1999). Emergent properties of networks of biological signaling pathways. Science 283, 381–387. doi: 10.1126/science.283.5400.381
Bonardi, V., Pesaresi, P., Becker, T., Schleiff, E., Wagner, R., Pfannschmidt, T., et al. (2005). Photosystem II core phosphorylation and photosynthetic acclimation require two different protein kinases. Nature 437, 1179–1182. doi: 10.1038/nature04016
Bortolussi, L., and Policriti, A. (2008). “Hybrid systems and biology,” in International School on Formal Methods for the Design of Computer, Communication and Software Systems (Heildelberg: Springer), 424–448. doi: 10.1007/978-3-540-68894-5_12
Bräutigam, K., Dietzel, L., Kleine, T., Ströher, E., Wormuth, D., Dietz, K.-J., et al. (2009). Dynamic plastid redox signals integrate gene expression and metabolism to induce distinct metabolic states in photosynthetic acclimation in Arabidopsis. Plant Cell 21, 2715–2732. doi: 10.1105/tpc.108.062018
Breazeale, V. D., Buchanan, B. B., and Wolosiuk, R. A. (1978). Chloroplast sedoheptulose 1, 7-bisphosphatase: evidence for regulation by the ferredoxin/thioredoxin system. Z. Naturforsch. C 33, 521–528. doi: 10.1515/znc-1978-7-812
Breyton, C., Nandha, B., Johnson, G. N., Joliot, P., and Finazzi, G. (2006). Redox modulation of cyclic electron flow around photosystem I in C3 plants. Biochemistry 45, 13465–13475. doi: 10.1021/bi061439s
Buchanan, B. B., Schürmann, P., Wolosiuk, R. A., and Jacquot, J.-P. (2002). The ferredoxin/thioredoxin system: from discovery to molecular structures and beyond. Photosynth. Res. 73, 215–222. doi: 10.1023/A:1020407432008
Caliandro, R., Nagel, K. A., Kastenholz, B., Bassi, R., Li, Z., Niyogi, K. K., et al. (2013). Effects of altered α-and β-branch carotenoid biosynthesis on photoprotection and whole-plant acclimation of Arabidopsis to photo-oxidative stress. Plant Cell Environ. 36, 438–453. doi: 10.1111/j.1365-3040.2012.02586.x
Carlberg, I., Hansson, M., Kieselbach, T., Schröder, W. P., Andersson, B., and Vener, A. V. (2003). A novel plant protein undergoing light-induced phosphorylation and release from the photosynthetic thylakoid membranes. Proc. Natl. Acad. Sci. U.S.A. 100, 757–762. doi: 10.1073/pnas.0235452100
Carraretto, L., Formentin, E., Teardo, E., Checchetto, V., Tomizioli, M., Morosinotto, T., et al. (2013). A thylakoid-located two-pore K+ channel controls photosynthetic light utilization in plants. Science 342, 114–118. doi: 10.1126/science.1242113
Chan, K. X., Mabbitt, P. D., Phua, S. Y., Mueller, J. W., Nisar, N., Gigolashvili, T., et al. (2016). Sensing and signaling of oxidative stress in chloroplasts by inactivation of the SAL1 phosphoadenosine phosphatase. Proc. Natl. Acad. Sci. U.S.A. 113, E4567–E4576. doi: 10.1073/pnas.1604936113
Cheikh, N., and Brenner, M. L. (1992). Regulation of key enzymes of sucrose biosynthesis in soybean leaves: effect of dark and light conditions and role of gibberellins and abscisic acid. Plant Physiol. 100, 1230–1237. doi: 10.1104/pp.100.3.1230
Cheung, C. Y. M., Poolman, M. G., Fell, D. A., Ratcliffe, R. G., and Sweetlove, L. J. (2014). A diel flux balance model captures interactions between light and dark metabolism during day-night cycles in C3 and crassulacean acid metabolism leaves. Plant Physiol. 165, 917–929. doi: 10.1104/pp.113.234468
Cho, Y.-H., Hong, J.-W., Kim, E.-C., and Yoo, S.-D. (2012). Regulatory functions of SnRK1 in stress-responsive gene expression and in plant growth and development. Plant Physiol. 158, 1955–1964. doi: 10.1104/pp.111.189829
Chow, W. S., Melis, A., and Anderson, J. M. (1990). Adjustments of photosystem stoichiometry in chloroplasts improve the quantum efficiency of photosynthesis. Proc. Natl. Acad. Sci. U.S.A. 87, 7502–7506. doi: 10.1073/pnas.87.19.7502
Collin, V., Lamkemeyer, P., Miginiac-Maslow, M., Hirasawa, M., Knaff, D. B., Dietz, K.-J., et al. (2004). Characterization of plastidial thioredoxins from Arabidopsis belonging to the new y-type. Plant Physiol. 136, 4088–4095. doi: 10.1104/pp.104.052233
Csete, M. E., and Doyle, J. C. (2002). Reverse engineering of biological complexity. Science 295, 1664–1669. doi: 10.1126/science.1069981
Dada, J. O., and Mendes, P. (2011). Multi-scale modelling and simulation in systems biology. Integr. Biol. 3, 86–96. doi: 10.1039/c0ib00075b
Dai, N., Schaffer, A., Petreikov, M., Shahak, Y., Giller, Y., Ratner, K., et al. (1999). Overexpression of Arabidopsis hexokinase in tomato plants inhibits growth, reduces photosynthesis, and induces rapid senescence. Plant Cell 11, 1253–1266. doi: 10.1105/tpc.11.7.1253
DalCorso, G., Pesaresi, P., Masiero, S., Aseeva, E., Schünemann, D., Finazzi, G., et al. (2008). A complex containing PGRL1 and PGR5 is involved in the switch between linear and cyclic electron flow in Arabidopsis. Cell 132, 273–285. doi: 10.1016/j.cell.2007.12.028
De Souza, A. P., Wang, Y., Orr, D. J., Carmo-Silva, E., and Long, S. P. (2020). Photosynthesis across African cassava germplasm is limited by Rubisco and mesophyll conductance at steady state, but by stomatal conductance in fluctuating light. New Phytol. 225, 2498–2512. doi: 10.1111/nph.16142
Depège, N., Bellafiore, S., and Rochaix, J.-D. (2003). Role of chloroplast protein kinase Stt7 in LHCII phosphorylation and state transition in Chlamydomonas. Science 299, 1572–1575. doi: 10.1126/science.1081397
Derks, A., Schaven, K., and Bruce, D. (2015). Diverse mechanisms for photoprotection in photosynthesis. Dynamic regulation of photosystem II excitation in response to rapid environmental change. Biochim. Biophys. Acta 1847, 468–485. doi: 10.1016/j.bbabio.2015.02.008
Driever, S. M., and Baker, N. R. (2011). The water–water cycle in leaves is not a major alternative electron sink for dissipation of excess excitation energy when CO2 assimilation is restricted. Plant Cell Environ. 34, 837–846. doi: 10.1111/j.1365-3040.2011.02288.x
Dunford, R. P., Durrant, M. C., Catley, M. A., and Dyer, T. A. (1998). Location of the redox-active cysteines in chloroplast sedoheptulose-1, 7-bisphosphatase indicates that its allosteric regulation is similar but not identical to that of fructose-1, 6-bisphosphatase. Photosynth. Res. 58, 221–230. doi: 10.1023/A:1006178826976
Dyson, B. C., Allwood, J. W., Feil, R., Xu, Y., Miller, M., Bowsher, C. G., et al. (2015). Acclimation of metabolism to light in Arabidopsis thaliana: the glucose 6-phosphate/phosphate translocator GPT2 directs metabolic acclimation. Plant Cell Environ. 38, 1404–1417. doi: 10.1111/pce.12495
Eisenhut, M., Roell, M. S., and Weber, A. P. M. (2019). Mechanistic understanding of photorespiration paves the way to a new green revolution. New Phytol. 223, 1762–1769. doi: 10.1111/nph.15872
El Bissati, K., and Kirilovsky, D. (2001). Regulation of psbA and psaE expression by light quality in Synechocystis species PCC 6803. A redox control mechanism. Plant Physiol. 125, 1988–2000. doi: 10.1104/pp.125.4.1988
Ensminger, I., Busch, F., and Huner, N. P. A. (2006). Photostasis and cold acclimation: sensing low temperature through photosynthesis. Physiol. Plant. 126, 28–44. doi: 10.1111/j.1399-3054.2006.00627.x
Eskins, K., Jiang, C. Z., and Shibles, R. (1991). Light-quality and irradiance effects on pigments, light-harvesting proteins and Rubisco activity in a chlorophyll- and light- harvesting-deficient soybean mutant. Physiol. Plant. 83, 47–53. doi: 10.1111/j.1399-3054.1991.tb01280.x
Estavillo, G. M., Crisp, P. A., Pornsiriwong, W., Wirtz, M., Collinge, D., Carrie, C., et al. (2011). Evidence for a SAL1-PAP chloroplast retrograde pathway that functions in drought and high light signaling in Arabidopsis. Plant Cell 23, 3992–4012. doi: 10.1105/tpc.111.091033
Evans, J. (1988). Acclimation by the thylakoid membranes to growth irradiance and the partitioning of nitrogen between soluble and thylakoid proteins. Funct. Plant Biol. 15:93. doi: 10.1071/PP9880093
Exposito-Rodriguez, M., Laissue, P. P., Yvon-Durocher, G., Smirnoff, N., and Mullineaux, P. M. (2017). Photosynthesis-dependent H2O2 transfer from chloroplasts to nuclei provides a high-light signalling mechanism. Nat. Commun. 8, 1–11. doi: 10.1038/s41467-017-00074-w
Farquhar, G. D., Von Caemmerer, S., and Berry, J. A. (2001). Models of photosynthesis. Plant Physiol. 125, 42–45. doi: 10.1104/pp.125.1.42
Farquhar, G. D., von Caemmerer, S., and von Berry, J. A. (1980). A biochemical model of photosynthetic CO2 assimilation in leaves of C3 species. Planta 149, 78–90. doi: 10.1007/BF00386231
Fey, V., Wagner, R., Bräutigam, K., and Pfannschmidt, T. (2005a). Photosynthetic redox control of nuclear gene expression. J. Exp. Bot. 56, 1491–1498. doi: 10.1093/jxb/eri180
Fey, V., Wagner, R., Braütigam, K., Wirtz, M., Hell, R., Dietzmann, A., et al. (2005b). Retrograde plastid redox signals in the expression of nuclear genes for chloroplast proteins of Arabidopsis thaliana. J. Biol. Chem. 280, 5318–5328. doi: 10.1074/jbc.M406358200
Finazzi, G., and Johnson, G. N. (2016). Cyclic electron flow: facts and hypotheses. Photosynth. Res. 129, 227–230. doi: 10.1007/s11120-016-0306-2
Finazzi, G., Petroutsos, D., Tomizioli, M., Flori, S., Sautron, E., Villanova, V., et al. (2015). Ions channels/transporters and chloroplast regulation. Cell Calcium 58, 86–97. doi: 10.1016/j.ceca.2014.10.002
Foo, C. C., Burgess, A. J., Retkute, R., Tree-Intong, P., Ruban, A. V., and Murchie, E. H. (2020). Photoprotective energy dissipation is greater in the lower, not the upper, regions of a rice canopy: a 3D analysis. J. Exp. Bot. 71, 7382–7392. doi: 10.1093/jxb/eraa411
Foyer, C. H. (2018). Reactive oxygen species, oxidative signaling and the regulation of photosynthesis. Environ. Exp. Bot. 154, 134–142. doi: 10.1016/j.envexpbot.2018.05.003
Foyer, C. H., Bloom, A. J., Queval, G., and Noctor, G. (2009). Photorespiratory metabolism: genes, mutants, energetics, and redox signaling. Annu. Rev. Plant Biol. 60, 455–484. doi: 10.1146/annurev.arplant.043008.091948
Foyer, C. H., Neukermans, J., Queval, G., Noctor, G., and Harbinson, J. (2012). Photosynthetic control of electron transport and the regulation of gene expression. J. Exp. Bot. 63, 1637–1661. doi: 10.1093/jxb/ers013
Fristedt, R., Carlberg, I., Zygadlo, A., Piippo, M., Nurmi, M., Aro, E.-M., et al. (2009). Intrinsically unstructured phosphoprotein TSP9 regulates light harvesting in Arabidopsis thaliana. Biochemistry 48, 499–509. doi: 10.1021/bi8016334
Galvis, V. C., Strand, D. D., Messer, M., Thiele, W., Bethmann, S., Hübner, D., et al. (2020). H+ transport by K+ EXCHANGE ANTIPORTER3 promotes photosynthesis and growth in chloroplast ATP synthase mutants. Plant Physiol. 182, 2126–2142. doi: 10.1104/pp.19.01561
Geigenberger, P., Thormählen, I., Daloso, D. M., and Fernie, A. R. (2017). The unprecedented versatility of the plant thioredoxin system. Trends Plant Sci. 22, 249–262. doi: 10.1016/j.tplants.2016.12.008
Geritz, S. A., and Kisdi, É. (2012). Mathematical ecology: why mechanistic models? J. Math. Biol. 65, 1411. doi: 10.1007/s00285-011-0496-3
Gillespie, D. T. (2000). The chemical Langevin equation. J. Chem. Phys. 113, 297–306. doi: 10.1063/1.481811
Gollan, P. J., Tikkanen, M., and Aro, E.-M. (2015). Photosynthetic light reactions: integral to chloroplast retrograde signalling. Curr. Opin. Plant Biol. 27, 180–191. doi: 10.1016/j.pbi.2015.07.006
Gomes de Oliveira Dal'Molin, C., Quek, L.-E., Saa, P. A., and Nielsen, L. K. (2015). A multi-tissue genome-scale metabolic modeling framework for the analysis of whole plant systems. Front. Plant Sci. 6:4. doi: 10.3389/fpls.2015.00004
Grafahrend-Belau, E., Junker, A., Eschenröder, A., Müller, J., Schreiber, F., and Junker, B. H. (2013). Multiscale metabolic modeling: dynamic flux balance analysis on a whole-plant scale. Plant Physiol. 163, 637–647. doi: 10.1104/pp.113.224006
Granot, D., Kelly, G., Stein, O., and David-Schwartz, R. (2014). Substantial roles of hexokinase and fructokinase in the effects of sugars on plant physiology and development. J. Exp. Bot. 65, 809–819. doi: 10.1093/jxb/ert400
Grieco, M., Jain, A., Ebersberger, I., and Teige, M. (2016). An evolutionary view on thylakoid protein phosphorylation uncovers novel phosphorylation hotspots with potential functional implications. J. Exp. Bot. 67, 3883–3896. doi: 10.1093/jxb/erw164
Grieco, M., Tikkanen, M., Paakkarinen, V., Kangasjärvi, S., and Aro, E.-M. (2012). Steady-state phosphorylation of light-harvesting complex II proteins preserves photosystem I under fluctuating white light. Plant Physiol. 160, 1896–1910. doi: 10.1104/pp.112.206466
Guerriero, M. L., Akman, O. E., and van Ooijen, G. (2014). Stochastic models of cellular circadian rhythms in plants help to understand the impact of noise on robustness and clock structure. Front. Plant Sci. 5:564. doi: 10.3389/fpls.2014.00564
Harley, P. C., and Tenhunen, J. D. (1991). Modeling the photosynthetic response of C3 leaves to environmental factors. Model. Crop Photosynth. Biochem. 19, 17–39. doi: 10.2135/cssaspecpub19.c2
Hasdemir, D., Hoefsloot, H. C. J., Westerhuis, J. A., and Smilde, A. K. (2014). How informative is your kinetic model?: using resampling methods for model invalidation. BMC Syst. Biol. 8:61. doi: 10.1186/1752-0509-8-61
Häusler, R. E., Heinrichs, L., Schmitz, J., and Flügge, U.-I. (2014). How sugars might coordinate chloroplast and nuclear gene expression during acclimation to high light intensities. Mol. Plant 7, 1121–1137. doi: 10.1093/mp/ssu064
Havaux, M. (2014). Carotenoid oxidation products as stress signals in plants. Plant J. 79, 597–606. doi: 10.1111/tpj.12386
Henmi, T., Miyao, M., and Yamamoto, Y. (2004). Release and reactive-oxygen-mediated damage of the oxygen-evolving complex subunits of PSII during photoinhibition. Plant Cell Physiol. 45, 243–250. doi: 10.1093/pcp/pch027
Henzinger, T. A. (2000). “The theory of hybrid automata,” in Verification of Digital and Hybrid Systems, eds M. Kemal Inan and R. P. Kurshan (Cham: Springer), 265–292. doi: 10.1007/978-3-642-59615-5_13
Herdean, A., Teardo, E., Nilsson, A. K., Pfeil, B. E., Johansson, O. N., Ünnep, R., et al. (2016). A voltage-dependent chloride channel fine-tunes photosynthesis in plants. Nat. Commun. 7, 1–11. doi: 10.1038/ncomms11654
Herrmann, H. A., Dyson, B. C., Vass, L., Johnson, G. N., and Schwartz, J.-M. (2019a). Flux sampling is a powerful tool to study metabolism under changing environmental conditions. NPJ Syst. Biol. Appl. 5, 1–8. doi: 10.1038/s41540-019-0109-0
Herrmann, H. A., Schwartz, J.-M., and Johnson, G. N. (2019b). Metabolic acclimation—a key to enhancing photosynthesis in changing environments? J. Exp. Bot. 70, 3043–3056. doi: 10.1093/jxb/erz157
Herrmann, H. A., Schwartz, J.-M., and Johnson, G. N. (2020). From empirical to theoretical models of light response curves-linking photosynthetic and metabolic acclimation. Photosynth. Res. 145, 5–14. doi: 10.1007/s11120-019-00681-2
Hideg, É., Kálai, T., Hideg, K., and Vass, I. (1998). Photoinhibition of photosynthesis in vivo results in singlet oxygen production detection via nitroxide-induced fluorescence quenching in broad bean leaves. Biochemistry 37, 11405–11411. doi: 10.1021/bi972890+
Höhner, R., Galvis, V. C., Strand, D. D., Völkner, C., Krämer, M., Messer, M., et al. (2019). Photosynthesis in Arabidopsis is unaffected by the function of the vacuolar K+ channel TPK3. Plant Physiol. 180, 1322–1335. doi: 10.1104/pp.19.00255
Horacio, P., and Martinez-Noel, G. (2013). Sucrose signaling in plants: a world yet to be explored. Plant Signal. Behav. 8:e23316. doi: 10.4161/psb.23316
Huang, W., Hu, H., and Zhang, S. B. (2015). Photorespiration plays an important role in the regulation of photosynthetic electron flow under fluctuating light in tobacco plants grown under full sunlight. Front. Plant Sci. 6:621. doi: 10.3389/fpls.2015.00621
Huang, W., Yang, Y.-J., and Zhang, S.-B. (2019a). Photoinhibition of photosystem I under fluctuating light is linked to the insufficient ΔpH upon a sudden transition from low to high light. Environ. Exp. Bot. 160, 112–119. doi: 10.1016/j.envexpbot.2019.01.012
Huang, W., Yang, Y.-J., and Zhang, S.-B. (2019b). The role of water-water cycle in regulating the redox state of photosystem I under fluctuating light. Biochim. Biophys. Acta 1860, 383–390. doi: 10.1016/j.bbabio.2019.03.007
Hummer, G., and Szabo, A. (2015). Optimal dimensionality reduction of multistate kinetic and Markov-state models. J. Phys. Chem. B 119, 9029–9037. doi: 10.1021/jp508375q
Hurry, V., Strand, Å., Furbank, R., and Stitt, M. (2000). The role of inorganic phosphate in the development of freezing tolerance and the acclimatization of photosynthesis to low temperature is revealed by the pho mutants of Arabidopsis thaliana. Plant J. 24, 383–396. doi: 10.1046/j.1365-313x.2000.00888.x
Ibrahim, I. M., Wu, H., Ezhov, R., Kayanja, G. E., Zakharov, S. D., Du, Y., et al. (2020). An evolutionarily conserved iron-sulfur cluster underlies redox sensory function of the Chloroplast Sensor Kinase. Commun. Biol. 3, 1–11. doi: 10.1038/s42003-019-0728-4
Jamshidi, N., and Palsson, B. Ø. (2010). Mass action stoichiometric simulation models: incorporating kinetics and regulation into stoichiometric models. Biophys. J. 98, 175–185. doi: 10.1016/j.bpj.2009.09.064
Jang, J.-C., León, P., Zhou, L., and Sheen, J. (1997). Hexokinase as a sugar sensor in higher plants. Plant Cell 9, 5–19. doi: 10.1105/tpc.9.1.5
Johnson, G., and Murchie, E. (2011). “Gas exchange measurements for the determination of photosynthetic efficiency in Arabidopsis leaves,” in Chloroplast Research in Arabidopsis, ed R. Paul Jarvis (Totowa, NJ: Humana Press), 311–326. doi: 10.1007/978-1-61779-237-3_17
Johnson, M. P., and Ruban, A. V. (2009). Photoprotective energy dissipation in higher plants involves alteration of the excited state energy of the emitting chlorophyll(s) in the light harvesting antenna II (LHCII). J. Biol. Chem. 284, 23592–23601. doi: 10.1074/jbc.M109.013557
Johnson, M. P., and Wientjes, E. (2020). BBA - Bioenergetics The relevance of dynamic thylakoid organisation to photosynthetic. Biochim. Biophys. Acta 1861:148039. doi: 10.1016/j.bbabio.2019.06.011
Joliot, P., and Johnson, G. N. (2011). Regulation of cyclic and linear electron flow in higher plants. Proc. Natl. Acad. Sci. U.S.A. 108, 13317–13322. doi: 10.1073/pnas.1110189108
Jossier, M., Bouly, J., Meimoun, P., Arjmand, A., Lessard, P., Hawley, S., et al. (2009). SnRK1 (SNF1-related kinase 1) has a central role in sugar and ABA signalling in Arabidopsis thaliana. Plant J. 59, 316–328. doi: 10.1111/j.1365-313X.2009.03871.x
Kaiser, E., Matsubara, S., Harbinson, J., Heuvelink, E., and Marcelis, L. F. M. (2018). Acclimation of photosynthesis to lightflecks in tomato leaves: interaction with progressive shading in a growing canopy. Physiol. Plant. 162, 506–517. doi: 10.1111/ppl.12668
Karpiński, S., Szechyńska-Hebda, M., Wituszyńska, W., and Burdiak, P. (2013). Light acclimation, retrograde signalling, cell death and immune defences in plants. Plant Cell Environ. 36, 736–744. doi: 10.1111/pce.12018
Kelly, G., Moshelion, M., David-Schwartz, R., Halperin, O., Wallach, R., Attia, Z., et al. (2013). Hexokinase mediates stomatal closure. Plant J. 75, 977–988. doi: 10.1111/tpj.12258
Kim, C. (2020). ROS-driven oxidative modification: its impact on chloroplasts-nucleus communication. Front. Plant Sci. 10:1729. doi: 10.3389/fpls.2019.01729
Kim, J. H., Glick, R. E., and Melis, A. (1993). Dynamics of photosystem stoichiometry adjustment by light quality in chloroplasts. Plant Physiol. 102, 181–190. doi: 10.1104/pp.102.1.181
Kimura, H., Hashimoto-Sugimoto, M., Iba, K., Terashima, I., and Yamori, W. (2020). Improved stomatal opening enhances photosynthetic rate and biomass production in fluctuating light. J. Exp. Bot. 71, 2339–2350. doi: 10.1093/jxb/eraa090
Kimura, M., Yamamoto, Y. Y., Seki, M., Sakurai, T., Sato, M., Abe, T., et al. (2003). Identification of arabidopsis genes regulated by high light–stress using cDNA microarray. Photochem. Photobiol. 77, 226–233. doi: 10.1562/0031-8655(2003)0770226IOAGRB2.0.CO2
Kirschbaum, M. U. F., Küppers, M., Schneider, H., Giersch, C., and Noe, S. (1997). Modelling photosynthesis in fluctuating light with inclusion of stomatal conductance, biochemical activation and pools of key photosynthetic intermediates. Planta 204, 16–26. doi: 10.1007/s004250050225
Kitano, H. (2002). Systems biology: a brief overview. Science 295, 1662–1664. doi: 10.1126/science.1069492
Kono, M., Noguchi, K., and Terashima, I. (2014). Roles of the cyclic electron flow around PSI (CEF-PSI) and O 2-dependent alternative pathways in regulation of the photosynthetic electron flow in short-term fluctuating light in Arabidopsis thaliana. Plant Cell Physiol. 55, 990–1004. doi: 10.1093/pcp/pcu033
Kono, M., and Terashima, I. (2016). Elucidation of photoprotective mechanisms of PSI against fluctuating light photoinhibition. Plant Cell Physiol. 57, 1405–1414. doi: 10.1093/pcp/pcw103
Kotsiantis, S. B., Zaharakis, I., and Pintelas, P. (2007). Supervised machine learning: A review of classification techniques. Emerg. Artif. Intell. Appl. Comput. Eng. 160, 3–24. doi: 10.1007/s10462-007-9052-3
Krieger-Liszkay, A., Fufezan, C., and Trebst, A. (2008). Singlet oxygen production in photosystem II and related protection mechanism. Photosynth. Res. 98, 551–564. doi: 10.1007/s11120-008-9349-3
Kromdijk, J., Głowacka, K., Leonelli, L., Gabilly, S. T., Iwai, M., Niyogi, K. K., et al. (2016). Improving photosynthesis and crop productivity by accelerating recovery from photoprotection. Science 354, 857–861. doi: 10.1126/science.aai8878
Kunz, H. H., Häusler, R. E., Fettke, J., Herbst, K., Niewiadomski, P., Gierth, M., et al. (2010). The role of plastidial glucose-6-phosphate/phosphate translocators in vegetative tissues of Arabidopsis thaliana mutants impaired in starch biosynthesis. Plant Biol. 12, 115–128. doi: 10.1111/j.1438-8677.2010.00349.x
Kyle, D. J., Staehelin, L. A., and Arntzen, C. J. (1983). Lateral mobility of the light-harvesting complex in chloroplast membranes controls excitation energy distribution in higher plants. Arch. Biochem. Biophys. 222, 527–541. doi: 10.1016/0003-9861(83)90551-9
Larsson, U. K., Jergil, B., and Andersson, B. (1983). Changes in the lateral distribution of the light-harvesting chlorophyll-a/b—protein complex induced by its phosphorylation. Eur. J. Biochem. 136, 25–29. doi: 10.1111/j.1432-1033.1983.tb07700.x
Lefebvre, S., Lawson, T., Fryer, M., Zakhleniuk, O. V., Lloyd, J. C., and Raines, C. A. (2005). Increased sedoheptulose-1, 7-bisphosphatase activity in transgenic tobacco plants stimulates photosynthesis and growth from an early stage in development. Plant Physiol. 138, 451–460. doi: 10.1104/pp.104.055046
Leister, D. (2019). Piecing the puzzle together: the central role of reactive oxygen species and redox hubs in chloroplast retrograde signaling. Antioxid. Redox Signal. 30, 1206–1219. doi: 10.1089/ars.2017.7392
Lemaire, S. D., Michelet, L., Zaffagnini, M., Massot, V., and Issakidis-Bourguet, E. (2007). Thioredoxins in chloroplasts. Curr. Genet. 51, 343–365. doi: 10.1007/s00294-007-0128-z
Lemeille, S., and Rochaix, J.-D. (2010). State transitions at the crossroad of thylakoid signalling pathways. Photosynth. Res. 106, 33–46. doi: 10.1007/s11120-010-9538-8
Leong, T.-Y., and Anderson, J. M. (1984). Adaptation of the thylakoid membranes of pea chloroplasts to light intensities. I. Study on the distribution of chlorophyll-protein complexes. Photosynth. Res. 5, 105–115. doi: 10.1007/BF00028524
Lewis, N. E., Nagarajan, H., and Palsson, B. O. (2012). Constraining the metabolic genotype–phenotype relationship using a phylogeny of in silico methods. Nat. Rev. Microbiol. 10, 291–305. doi: 10.1038/nrmicro2737
Louarn, G., Frak, E., Zaka, S., Prieto, J., and Lebon, E. (2015). An empirical model that uses light attenuation and plant nitrogen status to predict within-canopy nitrogen distribution and upscale photosynthesis from leaf to whole canopy. AoB Plants 7:plv116. doi: 10.1093/aobpla/plv116
Lunn, J. E., Feil, R., Hendriks, J. H. M., Gibon, Y., Morcuende, R., Osuna, D., et al. (2006). Sugar-induced increases in trehalose 6-phosphate are correlated with redox activation of ADPglucose pyrophosphorylase and higher rates of starch synthesis in Arabidopsis thaliana. Biochem. J. 397, 139–148. doi: 10.1042/BJ20060083
Mahadevan, R., Edwards, J. S., and Doyle, F. J III. (2002). Dynamic flux balance analysis of diauxic growth in Escherichia coli. Biophys. J. 83, 1331–1340. doi: 10.1016/S0006-3495(02)73903-9
Maruta, T., Noshi, M., Tanouchi, A., Tamoi, M., Yabuta, Y., Yoshimura, K., et al. (2012). H2O2-triggered retrograde signaling from chloroplasts to nucleus plays specific role in response to stress. J. Biol. Chem. 287, 11717–11729. doi: 10.1074/jbc.M111.292847
Matsubara, S., Schneider, T., and Maurino, V. G. (2016). Dissecting long-term adjustments of photoprotective and photo-oxidative stress acclimation occurring in dynamic light environments. Front. Plant Sci. 7:1690. doi: 10.3389/fpls.2016.01690
Matthews, J. S. A., Vialet-Chabrand, S., and Lawson, T. (2018). Acclimation to fluctuating light impacts the rapidity of response and diurnal rhythm of stomatal conductance. Plant Physiol. 176, 1939–1951. doi: 10.1104/pp.17.01809
Mekala, N. R., Suorsa, M., Rantala, M., Aro, E.-M., and Tikkanen, M. (2015). Plants actively avoid state transitions upon changes in light intensity: role of light-harvesting complex II protein dephosphorylation in high light. Plant Physiol. 168, 721–734. doi: 10.1104/pp.15.00488
Mélykúti, B., Burrage, K., and Zygalakis, K. C. (2010). Fast stochastic simulation of biochemical reaction systems by alternative formulations of the chemical Langevin equation. J. Chem. Phys. 132:164109. doi: 10.1063/1.3380661
Mengin, V., Pyl, E., Alexandre Moraes, T., Sulpice, R., Krohn, N., Encke, B., et al. (2017). Photosynthate partitioning to starch in Arabidopsis thaliana is insensitive to light intensity but sensitive to photoperiod due to a restriction on growth in the light in short photoperiods. Plant Cell Environ. 40, 2608–2627. doi: 10.1111/pce.13000
Michelet, L., Zaffagnini, M., Morisse, S., Sparla, F., Pérez-Pérez, M. E., Francia, F., et al. (2013). Redox regulation of the Calvin-Benson cycle: something old, something new. Front. Plant Sci. 4:470. doi: 10.3389/fpls.2013.00470
Miller, M. A. E., O'Cualain, R., Selley, J., Knight, D., Karim, M. F., Hubbard, S. J., et al. (2017). Dynamic acclimation to high light in Arabidopsis thaliana involves widespread reengineering of the leaf proteome. Front. Plant Sci. 8:1239. doi: 10.3389/fpls.2017.01239
Minagawa, J. (2011). State transitions—the molecular remodeling of photosynthetic supercomplexes that controls energy flow in the chloroplast. Biochim. Biophys. Acta 1807, 897–905. doi: 10.1016/j.bbabio.2010.11.005
Mjolsness, E., and DeCoste, D. (2001). Machine learning for science: state of the art and future prospects. Science 293, 2051–2055. doi: 10.1126/science.293.5537.2051
Mochizuki, N., Tanaka, R., Tanaka, A., Masuda, T., and Nagatani, A. (2008). The steady-state level of Mg-protoporphyrin IX is not a determinant of plastid-to-nucleus signaling in Arabidopsis. Proc. Natl. Acad. Sci. U.S.A. 105, 15184–15189. doi: 10.1073/pnas.0803245105
Moore, B., Zhou, L., Rolland, F., Hall, Q., Cheng, W.-H., Liu, Y.-X., et al. (2003). Role of the Arabidopsis glucose sensor HXK1 in nutrient, light, and hormonal signaling. Science 300, 332–336. doi: 10.1126/science.1080585
Mott, K. A., and Woodrow, I. E. (2000). Modelling the role of Rubisco activase in limiting non-steady-state photosynthesis. J. Exp. Bot. 51, 399–406. doi: 10.1093/jexbot/51.suppl_1.399
Moulin, M., McCormac, A. C., Terry, M. J., and Smith, A. G. (2008). Tetrapyrrole profiling in Arabidopsis seedlings reveals that retrograde plastid nuclear signaling is not due to Mg-protoporphyrin IX accumulation. Proc. Natl. Acad. Sci. U.S.A. 105, 15178–15183. doi: 10.1073/pnas.0803054105
Munekage, Y., Hojo, M., Meurer, J., Endo, T., Tasaka, M., and Shikanai, T. (2002). PGR5 is involved in cyclic electron flow around photosystem I and is essential for photoprotection in Arabidopsis. Cell 110, 361–371. doi: 10.1016/S0092-8674(02)00867-X
Munekage, Y. N., Genty, B., and Peltier, G. (2008). Effect of PGR5 impairment on photosynthesis and growth in Arabidopsis thaliana. Plant Cell Physiol. 49, 1688–1698. doi: 10.1093/pcp/pcn140
Murchie, E. H., and Horton, P. (1998). Contrasting patterns of photosynthetic acclimation to the light environment are dependent on the differential expression of the responses to altered irradiance and spectral quality. Plant Cell Environ. 21, 139–148. doi: 10.1046/j.1365-3040.1998.00262.x
Nägele, T., and Weckwerth, W. (2014). Mathematical modeling reveals that metabolic feedback regulation of SnRK1 and hexokinase is sufficient to control sugar homeostasis from energy depletion to full recovery. Front. Plant Sci. 5:365. doi: 10.3389/fpls.2014.00365
Nakano, H., Yamamoto, H., and Shikanai, T. (2019). Contribution of NDH-dependent cyclic electron transport around photosystem I to the generation of proton motive force in the weak mutant allele of pgr5. Biochim. Biophys. Acta 1860, 369–374. doi: 10.1016/j.bbabio.2019.03.003
Nandha, B., Finazzi, G., Joliot, P., Hald, S., and Johnson, G. N. (2007). The role of PGR5 in the redox poising of photosynthetic electron transport. Biochim. Biophys. Acta 1767, 1252–1259. doi: 10.1016/j.bbabio.2007.07.007
Nawrocki, W. J., Bailleul, B., Picot, D., Cardol, P., Rappaport, F., Wollman, F.-A., et al. (2019). The mechanism of cyclic electron flow. Biochim. Biophys. Acta 1860, 433–438. doi: 10.1016/j.bbabio.2018.12.005
Niedermaier, S., Schneider, T., Bahl, M.-O., Matsubara, S., and Huesgen, P. F. (2020). Photoprotective acclimation of the Arabidopsis thaliana leaf proteome to fluctuating light. Front. Genet. 11:154. doi: 10.3389/fgene.2020.00154
Niewiadomski, P., Knappe, S., Geimer, S., Fischer, K., Schulz, B., Unte, U. S., et al. (2005). The Arabidopsis plastidic glucose 6-phosphate/phosphate translocator GPT1 is essential for pollen maturation and embryo sac development. Plant Cell 17, 760–775. doi: 10.1105/tpc.104.029124
Niinemets, Ü., Kull, O., and Tenhunen, J. D. (1998). An analysis of light effects on foliar morphology, physiology, and light interception in temperate deciduous woody species of contrasting shade tolerance. Tree Physiol. 18, 681–696. doi: 10.1093/treephys/18.10.681
Nikkanen, L., and Rintamäki, E. (2014). Thioredoxin-dependent regulatory networks in chloroplasts under fluctuating light conditions. Philos. Trans. R. Soc. Lond. B Biol. Sci. 369:20130224. doi: 10.1098/rstb.2013.0224
Nikkanen, L., Toivola, J., Diaz, M. G., and Rintamäki, E. (2017). Chloroplast thioredoxin systems: prospects for improving photosynthesis. Philos. Trans. R. Soc. B Biol. Sci. 372:20160474. doi: 10.1098/rstb.2016.0474
Niyogi, K. K. (2009). Photoprotection and High Light Responses. Chlamydomonas Sourceb. 3-Vol set 2, 847–870. doi: 10.1016/B978-0-12-370873-1.00031-9
Nott, A., Jung, H.-S., Koussevitzky, S., and Chory, J. (2006). Plastid-to-nucleus retrograde signaling. Annu. Rev. Plant Biol. 57, 739–759. doi: 10.1146/annurev.arplant.57.032905.105310
Pamplin, E. J., and Chapman, J. M. (1975). Sucrose suppression of chlorophyll synthesis in tissue culture: changes in the activity of the enzymes of the chlorophyll biosynthetic pathway. J. Exp. Bot. 26, 212–220. doi: 10.1093/jxb/26.2.212
Papanatsiou, M., Petersen, J., Henderson, L., Wang, Y., Christie, J. M., and Blatt, M. R. (2019). Optogenetic manipulation of stomatal kinetics improves carbon assimilation,water use, and growth. Science 363, 1456–1459. doi: 10.1126/science.aaw0046
Pascal, A. a, Liu, Z., Broess, K., van Oort, B., van Amerongen, H., Wang, C., et al. (2005). Molecular basis of photoprotection and control of photosynthetic light-harvesting. Nature 436, 134–137. doi: 10.1038/nature03795
Peak, D., West, J. D., Messinger, S. M., and Mott, K. A. (2004). Evidence for complex, collective dynamics and emergent, distributed computation in plants. Proc. Natl. Acad. Sci. U.S.A. 101, 918–922. doi: 10.1073/pnas.0307811100
Pearcy, R. W. (1990). Sunflecks and photosynthesis in plant canopies. Annu. Rev. Plant Biol. 41, 421–453. doi: 10.1146/annurev.pp.41.060190.002225
Pearcy, R. W., Gross, L. J., and He, D. (1997). An improved dynamic model of photosynthesis for estimation of carbon gain in sunfleck light regimes. Plant Cell Environ. 20, 411–424. doi: 10.1046/j.1365-3040.1997.d01-88.x
Pesaresi, P., Hertle, A., Pribil, M., Kleine, T., Wagner, R., Strissel, H., et al. (2009). Arabidopsis STN7 kinase provides a link between short- and long-term photosynthetic acclimation. Plant Cell 21, 2402–2423. doi: 10.1105/tpc.108.064964
Pfannschmidt, T., Bräutigam, K., Wagner, R., Dietzel, L., Schröter, Y., Steiner, S., et al. (2009). Potential regulation of gene expression in photosynthetic cells by redox and energy state: approaches towards better understanding. Ann. Bot. 103, 599–607. doi: 10.1093/aob/mcn081
Pfannschmidt, T., Nilsson, A., and Allen, J. F. (1999). Photosynthetic control of chloroplast gene expression. Nature 397, 625–628. doi: 10.1038/17624
Pietrzykowska, M., Suorsa, M., Semchonok, D. A., Tikkanen, M., Boekema, E. J., Aro, E.-M., et al. (2014). The light-harvesting chlorophyll a/b binding proteins Lhcb1 and Lhcb2 play complementary roles during state transitions in Arabidopsis. Plant Cell 26, 3646–3660. doi: 10.1105/tpc.114.127373
Ponnu, J., Wahl, V., and Schmid, M. (2011). Trehalose-6-phosphate: connecting plant metabolism and development. Front. Plant Sci. 2:70. doi: 10.3389/fpls.2011.00070
Poolman, M. G., Fell, D. A., and Thomas, S. (2000). Modelling photosynthesis and its control. J. Exp. Bot. 51, 319–328. doi: 10.1093/jexbot/51.suppl_1.319
Porcar-Castell, A., Bäck, J., Juurola, E., and Hari, P. (2006). Dynamics of the energy flow through photosystem II under changing light conditions: a model approach. Funct. Plant Biol. 33, 229–239. doi: 10.1071/FP05133
Pospíšil, P. (2012). Molecular mechanisms of production and scavenging of reactive oxygen species by photosystem II. Biochim. Biophys. Acta 1817, 218–231. doi: 10.1016/j.bbabio.2011.05.017
Pospíšil, P. (2016). Production of reactive oxygen species by photosystem II as a response to light and temperature stress. Front. Plant Sci. 7:1950. doi: 10.3389/fpls.2016.01950
Pribil, M., Pesaresi, P., Hertle, A., Barbato, R., and Leister, D. (2010). Role of plastid protein phosphatase TAP38 in LHCII dephosphorylation and thylakoid electron flow. PLoS Biol. 8:e1000288. doi: 10.1371/journal.pbio.1000288
Purvis, J. E., Radhakrishnan, R., and Diamond, S. L. (2009). Steady-state kinetic modeling constrains cellular resting states and dynamic behavior. PLoS Comput Biol 5:e1000298. doi: 10.1371/journal.pcbi.1000298
Puthiyaveetil, S., Kavanagh, T. A., Cain, P., Sullivan, J. A., Newell, C. A., Gray, J. C., et al. (2008). The ancestral symbiont sensor kinase CSK links photosynthesis with gene expression in chloroplasts. Proc. Natl. Acad. Sci. U.S.A. 105, 10061–10066. doi: 10.1073/pnas.0803928105
Qiao, M.-Y., Zhang, Y.-J., Liu, L.-A., Shi, L., Ma, Q.-H., Chow, W. S., et al. (2020). Do rapid photosynthetic responses protect maize leaves against photoinhibition under fluctuating light? Photosynth. Res. doi: 10.1007/s11120-020-00780-5. [Epub ahead of print].
Qu, M., Hamdani, S., Li, W., Wang, S., Tang, J., Chen, Z., et al. (2016). Rapid stomatal response to fluctuating light: an under-explored mechanism to improve drought tolerance in rice. Funct. Plant Biol. 43, 727–738. doi: 10.1071/FP15348
Ramel, F., Birtic, S., Ginies, C., Soubigou-Taconnat, L., Triantaphylidès, C., and Havaux, M. (2012). Carotenoid oxidation products are stress signals that mediate gene responses to singlet oxygen in plants. Proc. Natl. Acad. Sci. U.S.A. 109, 5535–5540. doi: 10.1073/pnas.1115982109
Rascher, U., and Nedbal, L. (2006). Dynamics of photosynthesis in fluctuating light. Curr. Opin. Plant Biol. 9, 671–678. doi: 10.1016/j.pbi.2006.09.012
Ratti, E. (2018). ‘Models of' and ‘Models for': on the relation between mechanistic models and experimental strategies in molecular biology. Br. J. Philos. Sci. 71, 773–797. doi: 10.1093/bjps/axy018
Rekarte-Cowie, I., Ebshish, O. S., Mohamed, K. S., and Pearce, R. S. (2008). Sucrose helps regulate cold acclimation of Arabidopsis thaliana. J. Exp. Bot. 59, 4205–4217. doi: 10.1093/jxb/ern262
Retkute, R., Smith-Unna, S. E., Smith, R. W., Burgess, A. J., Jensen, O. E., Johnson, G. N., et al. (2015). Exploiting heterogeneous environments: does photosynthetic acclimation optimize carbon gain in fluctuating light? J. Exp. Bot. 66, 2437–2447. doi: 10.1093/jxb/erv055
Retkute, R., Townsend, A. J., Murchie, E. H., Jensen, O. E., and Preston, S. P. (2018). Three-dimensional plant architecture and sunlit–shaded patterns: a stochastic model of light dynamics in canopies. Ann. Bot. 122, 291–302. doi: 10.1093/aob/mcy067
Rook, F., Gerrits, N., Kortstee, A., Van Kampen, M., Borrias, M., Weisbeek, P., et al. (1998). Sucrose-specific signalling represses translation of the Arabidopsis ATB2 bZIP transcription factor gene. Plant J. 15, 253–263. doi: 10.1046/j.1365-313X.1998.00205.x
Rott, M., Martins, N. F., Thiele, W., Lein, W., Bock, R., Kramer, D. M., et al. (2011). ATP synthase repression in tobacco restricts photosynthetic electron transport, CO2 assimilation, and plant growth by overacidification of the thylakoid lumen. Plant Cell 23, 304–321. doi: 10.1105/tpc.110.079111
Ruban, A. V., Johnson, M. P., and Duffy, C. D. P. (2012). The photoprotective molecular switch in the photosystem II antenna. Biochim. Biophys. Acta 1817, 167–181. doi: 10.1016/j.bbabio.2011.04.007
Ruelland, E., and Miginiac-Maslow, M. (1999). Regulation of chloroplast enzyme activities by thioredoxins: activation or relief from inhibition? Trends Plant Sci. 4, 136–141. doi: 10.1016/S1360-1385(99)01391-6
Saeys, Y., Inza, I., and Larranaga, P. (2007). A review of feature selection techniques in bioinformatics. Bioinformatics 23, 2507–2517. doi: 10.1093/bioinformatics/btm344
Sahrawy, M., Chueca, A., Hermoso, R., Lázaro, J. J., and Gorgé, J. L. (1997). Directed mutagenesis shows that the preceding region of the chloroplast fructose-1, 6-bisphosphatase regulatory sequence is the thioredoxin docking site. J. Mol. Biol. 269, 623–630. doi: 10.1006/jmbi.1997.1054
Schallau, K., and Junker, B. H. (2010). Simulating plant metabolic pathways with enzyme-kinetic models. Plant Physiol. 152, 1763–1771. doi: 10.1104/pp.109.149237
Schneider, T., Bolger, A., Zeier, J., Preiskowski, S., Benes, V., Trenkamp, S., et al. (2019). Fluctuating light interacts with time of day and leaf development stage to reprogram gene expression. Plant Physiol. 179, 1632–1657. doi: 10.1104/pp.18.01443
Schönberg, A., Rödiger, A., Mehwald, W., Galonska, J., Christ, G., Helm, S., et al. (2017). Identification of STN7/STN8 kinase targets reveals connections between electron transport, metabolism and gene expression. Plant J. 90, 1176–1186. doi: 10.1111/tpj.13536
Shapiguzov, A., Ingelsson, B., Samol, I., Andres, C., Kessler, F., Rochaix, J.-D., et al. (2010). The PPH1 phosphatase is specifically involved in LHCII dephosphorylation and state transitions in Arabidopsis. Proc. Natl. Acad. Sci. U.S.A. 107, 4782–4787. doi: 10.1073/pnas.0913810107
Shaw, R., and Cheung, C. Y. (2018). A dynamic multi-tissue flux balance model captures carbon and nitrogen metabolism and optimal resource partitioning during Arabidopsis growth. Front. Plant Sci. 9:884. doi: 10.3389/fpls.2018.00884
Sheen, J. (1990). Metabolic repression of transcription in higher plants. Plant Cell 2, 1027–1038. doi: 10.1105/tpc.2.10.1027
Shikanai, T. (2014). Central role of cyclic electron transport around photosystem I in the regulation of photosynthesis. Curr. Opin. Biotechnol. 26, 25–30. doi: 10.1016/j.copbio.2013.08.012
Shimakawa, G., and Miyake, C. (2018). Changing frequency of fluctuating light reveals the molecular mechanism for P700 oxidation in plant leaves. Plant Direct 2:e00073. doi: 10.1002/pld3.73
Simkin, A. J., Lopez-Calcagno, P. E., Davey, P. A., Headland, L. R., Lawson, T., Timm, S., et al. (2017). Simultaneous stimulation of sedoheptulose 1, 7-bisphosphatase, fructose 1, 6-bisphophate aldolase and the photorespiratory glycine decarboxylase-H protein increases CO2 assimilation, vegetative biomass and seed yield in Arabidopsis. Plant Biotechnol. J. 15, 805–816. doi: 10.1111/pbi.12676
Simkin, A. J., McAusland, L., Headland, L. R., Lawson, T., and Raines, C. A. (2015). Multigene manipulation of photosynthetic carbon assimilation increases CO2 fixation and biomass yield in tobacco. J. Exp. Bot. 66, 4075–4090. doi: 10.1093/jxb/erv204
Skovsen, E., Snyder, J. W., Lambert, J. D. C., and Ogilby, P. R. (2005). Lifetime and diffusion of singlet oxygen in a cell. J. Phys. Chem. B 109, 8570–8573. doi: 10.1021/jp051163i
Snowden, T. J., van der Graaf, P. H., and Tindall, M. J. (2017). Methods of model reduction for large-scale biological systems: a survey of current methods and trends. Bull. Math. Biol. 79, 1449–1486. doi: 10.1007/s11538-017-0277-2
Solfanelli, C., Poggi, A., Loreti, E., Alpi, A., and Perata, P. (2006). Sucrose-specific induction of the anthocyanin biosynthetic pathway in Arabidopsis. Plant Physiol. 140, 637–646. doi: 10.1104/pp.105.072579
Standfuss, J., Terwisscha van Scheltinga, A. C., Lamborghini, M., and Kühlbrandt, W. (2005). Mechanisms of photoprotection and nonphotochemical quenching in pea light-harvesting complex at 2.5 A resolution. EMBO J. 24, 919–928. doi: 10.1038/sj.emboj.7600585
Stegemann, J., Timm, H.-C., and Küppers, M. (1999). Simulation of photosynthetic plasticity in response to highly fluctuating light: an empirical model integrating dynamic photosynthetic induction and capacity. Trees 14, 145–160. doi: 10.1007/s004680050219
Stewart, J. J., Adams, W. W., Cohu, C. M., Polutchko, S. K., Lombardi, E. M., and Demmig-Adams, B. (2015). Differences in light-harvesting, acclimation to growth-light environment, and leaf structural development between Swedish and Italian ecotypes of Arabidopsis thaliana. Planta 242, 1277–1290. doi: 10.1007/s00425-015-2368-3
Stitt, M., and Hurry, V. (2002). A plant for all seasons: alterations in photosynthetic carbon metabolism during cold acclimation in Arabidopsis. Curr. Opin. Plant Biol. 5, 199–206. doi: 10.1016/S1369-5266(02)00258-3
Sun, H., Yang, Y.-J., and Huang, W. (2020). The water-water cycle is more effective in regulating redox state of photosystem I under fluctuating light than cyclic electron transport. Biochim. Biophys. 1861, 148235. doi: 10.1016/j.bbabio.2020.148235
Suorsa, M., Grieco, M., Järvi, S., Gollan, P. J., Kangasjärvi, S., Tikkanen, M., et al. (2013). PGR5 ensures photosynthetic control to safeguard photosystem I under fluctuating light conditions. Plant Signal. Behav. 8:e22741. doi: 10.4161/psb.22741
Suorsa, M., Järvi, S., Grieco, M., Nurmi, M., Pietrzykowska, M., Rantala, M., et al. (2012). PROTON GRADIENT REGULATION5 is essential for proper acclimation of Arabidopsis photosystem I to naturally and artificially fluctuating light conditions. Plant Cell 24, 2934–2948. doi: 10.1105/tpc.112.097162
Suorsa, M., Rossi, F., Tadini, L., Labs, M., Colombo, M., Jahns, P., et al. (2016). PGR5-PGRL1-dependent cyclic electron transport modulates linear electron transport rate in Arabidopsis thaliana. Mol. Plant 9, 271–288. doi: 10.1016/j.molp.2015.12.001
Szechyńska-Hebda, M., and Karpiński, S. (2013). Light intensity-dependent retrograde signalling in higher plants. J. Plant Physiol. 170, 1501–1516. doi: 10.1016/j.jplph.2013.06.005
Tabrizi, S. T., Sawicki, A., Zhou, S., Luo, M., and Willows, R. D. (2016). GUN4-protoporphyrin IX is a singlet oxygen generator with consequences for plastid retrograde signaling. J. Biol. Chem. 291, 8978–8984. doi: 10.1074/jbc.C116.719989
Takagi, D., Takumi, S., Hashiguchi, M., Sejima, T., and Miyake, C. (2016). Superoxide and singlet oxygen produced within the thylakoid membranes both cause photosystem I photoinhibition. Plant Physiol. 171, 1626–1634. doi: 10.1104/pp.16.00246
Takizawa, K., Cruz, J. A., Kanazawa, A., and Kramer, D. M. (2007). The thylakoid proton motive force in vivo. Quantitative, non-invasive probes, energetics, and regulatory consequences of light-induced pmf. Biochim. Biophys. Acta 1767, 1233–1244. doi: 10.1016/j.bbabio.2007.07.006
Tazoe, Y., Ishikawa, N., Shikanai, T., Ishiyama, K., Takagi, D., Makino, A., et al. (2020). Overproduction of PGR5 enhances the electron sink downstream of photosystem I in a C4 plant, Flaveria bidentis. Plant J. 103, 814–823. doi: 10.1111/tpj.14774
Thormählen, I., Zupok, A., Rescher, J., Leger, J., Weissenberger, S., Groysman, J., et al. (2017). Thioredoxins play a crucial role in dynamic acclimation of photosynthesis in fluctuating light. Mol. Plant 10, 168–182. doi: 10.1016/j.molp.2016.11.012
Tian, L. (2015). Recent advances in understanding carotenoid-derived signaling molecules in regulating plant growth and development. Front. Plant Sci. 6:790. doi: 10.3389/fpls.2015.00790
Tikkanen, M., Grieco, M., Kangasjärvi, S., and Aro, E.-M. (2010). Thylakoid protein phosphorylation in higher plant chloroplasts optimizes electron transfer under fluctuating light. Plant Physiol. 152, 723–735. doi: 10.1104/pp.109.150250
Tikkanen, M., Mirva, P., Marjaana, S., Sari, S., Paula, M., Julia, V., et al. (2006). State transitions revisited - A buffering system for dynamic low light acclimation of Arabidopsis. Plant Mol. Biol. 62, 779–793. doi: 10.1007/s11103-006-9088-9
Timm, S., and Bauwe, H. (2013). The variety of photorespiratory phenotypes–employing the current status for future research directions on photorespiration. Plant Biol. 15, 737–747. doi: 10.1111/j.1438-8677.2012.00691.x
Triantaphylidès, C., and Havaux, M. (2009). Singlet oxygen in plants: production, detoxification and signaling. Trends Plant Sci. 14, 219–228. doi: 10.1016/j.tplants.2009.01.008
Tripathy, B. C., and Oelmüller, R. (2012). Reactive oxygen species generation and signaling in plants. Plant Signal. Behav. 7, 1621–1633. doi: 10.4161/psb.22455
Uematsu, K., Suzuki, N., Iwamae, T., Inui, M., and Yukawa, H. (2012). Increased fructose 1, 6-bisphosphate aldolase in plastids enhances growth and photosynthesis of tobacco plants. J. Exp. Bot. 63, 3001–3009. doi: 10.1093/jxb/ers004
Vass, I. (2012). Molecular mechanisms of photodamage in the Photosystem II complex. Biochim. Biophys. Acta 1817, 209–217. doi: 10.1016/j.bbabio.2011.04.014
Vener, A. V., van Kan, P. J. M., Gal, A., Andersson, B., and Ohad, I. (1995). Activation/Deactivation Cycle of Redox-controlled Thylakoid Protein Phosphorylation ROLE OF PLASTOQUINOL BOUND TO THE REDUCED CYTOCHROME bf COMPLEX. J. Biol. Chem. 270, 25225–25232. doi: 10.1074/jbc.270.42.25225
Vialet-Chabrand, S., Matthews, J. S. A., Simkin, A. J., Raines, C. A., and Lawson, T. (2017). Importance of fluctuations in light on plant photosynthetic acclimation. Plant Physiol. 173, 2163–2179. doi: 10.1104/pp.16.01767
Voss, I., Sunil, B., Scheibe, R., and Raghavendra, A. S. (2013). Emerging concept for the role of photorespiration as an important part of abiotic stress response. Plant Biol. 15, 713–722. doi: 10.1111/j.1438-8677.2012.00710.x
Wagner, R., Dietzel, L., Bräutigam, K., Fischer, W., and Pfannschmidt, T. (2008). The long-term response to fluctuating light quality is an important and distinct light acclimation mechanism that supports survival of Arabidopsis thaliana under low light conditions. Planta 228, 573–587. doi: 10.1007/s00425-008-0760-y
Walters, R. G. (2005). Towards an understanding of photosynthetic acclimation. J. Exp. Bot. 56, 435–447. doi: 10.1093/jxb/eri060
Walters, R. G., and Horton, P. (1994). Acclimation of Arabidopsis thaliana to the light environment: changes in composition of the photosynthetic apparatus. Planta 195, 248–256. doi: 10.1007/BF00199685
Walters, R. G., and Horton, P. (1995). Acclimation of Arabidopsis thaliana to the light environment: regulation of chloroplast composition. Planta 197, 475–481. doi: 10.1007/BF00196669
Watling, J. R., Robinson, S. A., Woodrow, I. E., and Osmond, C. B. (1997). Responses of rainforest understorey plants to excess light during sunflecks. Funct. Plant Biol. 24, 17–25. doi: 10.1071/PP96074
Wei, Z., Duan, F., Sun, X., Song, X., and Zhou, W. (2020). Leaf photosynthetic and anatomical insights into mechanisms of acclimation in rice in response to long-term fluctuating light. Plant Cell Environ. 44, 747–761. doi: 10.1111/pce.13954
Wiese, A., Elzinga, N., Wobbes, B., and Smeekens, S. (2004). A conserved upstream open reading frame mediates sucrose-induced repression of translation. Plant Cell 16, 1717–1729. doi: 10.1105/tpc.019349
Wilson, K. E., Ivanov, A. G., Öquist, G., Grodzinski, B., Sarhan, F., and Huner, N. P. A. (2006). Energy balance, organellar redox status, and acclimation to environmental stress. Can. J. Bot. 84, 1355–1370. doi: 10.1139/B06-098
Wollman, F.-A., and Lemaire, C. (1988). Studies on kinase-controlled state transitions in photosystem II and b6f mutants from Chlamydomonas reinhardtii which lack quinone-binding proteins. Biochim. Biophys. Acta 933, 85–94. doi: 10.1016/0005-2728(88)90058-8
Xiao, W., Sheen, J., and Jang, J.-C. (2000). The role of hexokinase in plant sugar signal transduction and growth and development. Plant Mol. Biol. 44, 451–461. doi: 10.1023/A:1026501430422
Xiao, Y., Savchenko, T., Baidoo, E. E. K., Chehab, W. E., Hayden, D. M., Tolstikov, V., et al. (2012). Retrograde signaling by the plastidial metabolite MEcPP regulates expression of nuclear stress-response genes. Cell 149, 1525–1535. doi: 10.1016/j.cell.2012.04.038
Yabuta, Y., Maruta, T., Yoshimura, K., Ishikawa, T., and Shigeoka, S. (2004). Two distinct redox signaling pathways for cytosolic APX induction under photooxidative stress. Plant Cell Physiol. 45, 1586–1594. doi: 10.1093/pcp/pch181
Yadav, U. P., Ivakov, A., Feil, R., Duan, G. Y., Walther, D., Giavalisco, P., et al. (2014). The sucrose–trehalose 6-phosphate (Tre6P) nexus: specificity and mechanisms of sucrose signalling by Tre6P. J. Exp. Bot. 65, 1051–1068. doi: 10.1093/jxb/ert457
Yamamoto, H., and Shikanai, T. (2019). PGR5-dependent cyclic electron flow protects photosystem I under fluctuating light at donor and acceptor sides. Plant Physiol. 179, 588–600. doi: 10.1104/pp.18.01343
Yamori, W. (2016). Photosynthetic response to fluctuating environments and photoprotective strategies under abiotic stress. J. Plant Res. 129, 379–395. doi: 10.1007/s10265-016-0816-1
Yamori, W., Makino, A., and Shikanai, T. (2016). A physiological role of cyclic electron transport around photosystem I in sustaining photosynthesis under fluctuating light in rice. Sci. Rep. 6:20147. doi: 10.1038/srep20147
Yamori, W., Takahashi, S., Makino, A., Price, G. D., Badger, M. R., and von Caemmerer, S. (2011). The roles of ATP synthase and the cytochrome b6/f complexes in limiting chloroplast electron transport and determining photosynthetic capacity. Plant Physiol. 155, 956–962. doi: 10.1104/pp.110.168435
Yang, D. H., Webster, J., Adam, Z., Lindahl, M., and Andersson, B. (1998). Induction of acclimative proteolysis of the light-harvesting chlorophyll a/b protein of photosystem II in response to elevated light intensities. Plant Physiol. 118, 827–834. doi: 10.1104/pp.118.3.827
Yang, Y.-J., Tan, S.-L., Huang, J.-L., Zhang, S.-B., and Huang, W. (2020). The water-water cycle facilitates photosynthetic regulation under fluctuating light in the epiphytic orchid Dendrobium officinale. Environ. Exp. Bot. 180:104238. doi: 10.1016/j.envexpbot.2020.104238
Yin, Z.-H., and Johnson, G. N. (2000). Photosynthetic acclimation of higher plants to growth in fluctuating light environments. Photosynth. Res. 63, 97–107. doi: 10.1023/A:1006303611365
Zakhartsev, M., Medvedeva, I., Orlov, Y., Akberdin, I., Krebs, O., and Schulze, W. X. (2016). Metabolic model of central carbon and energy metabolisms of growing Arabidopsis thaliana in relation to sucrose translocation. BMC Plant Biol. 16:262. doi: 10.1186/s12870-016-0868-3
Zavafer, A., Cheah, M. H., Hillier, W., Chow, W. S., and Takahashi, S. (2015). Photodamage to the oxygen evolving complex of photosystem II by visible light. Sci. Rep. 5:16363. doi: 10.1038/srep16363
Zeeman, S. C., Smith, S. M., and Smith, A. M. (2004). The breakdown of starch in leaves. New Phytol. 163, 247–261. doi: 10.1111/j.1469-8137.2004.01101.x
Zer, H., and Ohad, I. (2003). Light, redox state, thylakoid-protein phosphorylation and signaling gene expression. Trends Biochem. Sci. 28, 467–470. doi: 10.1016/S0968-0004(03)00173-7
Zhang, Y., Primavesi, L. F., Jhurreea, D., Andralojc, P. J., Mitchell, R. A. C., Powers, S. J., et al. (2009). Inhibition of SNF1-related protein kinase1 activity and regulation of metabolic pathways by trehalose-6-phosphate. Plant Physiol. 149, 1860–1871. doi: 10.1104/pp.108.133934
Zito, F., Finazzi, G., Delosme, R., Nitschke, W., Picot, D., and Wollman, F. (1999). The Qo site of cytochrome b6f complexes controls the activation of the LHCII kinase. EMBO J. 18, 2961–2969. doi: 10.1093/emboj/18.11.2961
Keywords: photosynthesis, fluctuating light, mathematical modeling, acclimation, metabolism
Citation: Gjindali A, Herrmann HA, Schwartz J-M, Johnson GN and Calzadilla PI (2021) A Holistic Approach to Study Photosynthetic Acclimation Responses of Plants to Fluctuating Light. Front. Plant Sci. 12:668512. doi: 10.3389/fpls.2021.668512
Received: 16 February 2021; Accepted: 23 March 2021;
Published: 14 April 2021.
Edited by:
Michele Grieco, Martin Luther University of Halle-Wittenberg, GermanyReviewed by:
Wei Huang, Chinese Academy of Sciences, ChinaCopyright © 2021 Gjindali, Herrmann, Schwartz, Johnson and Calzadilla. This is an open-access article distributed under the terms of the Creative Commons Attribution License (CC BY). The use, distribution or reproduction in other forums is permitted, provided the original author(s) and the copyright owner(s) are credited and that the original publication in this journal is cited, in accordance with accepted academic practice. No use, distribution or reproduction is permitted which does not comply with these terms.
*Correspondence: Pablo I. Calzadilla, cGFibG8uY2FsemFkaWxsYUBtYW5jaGVzdGVyLmFjLnVr
†These authors have contributed equally to this work
‡Present address: Helena A. Herrmann, Faculty of Chemistry, Institute of Analytical Chemistry, University of Vienna, Vienna, Austria
Disclaimer: All claims expressed in this article are solely those of the authors and do not necessarily represent those of their affiliated organizations, or those of the publisher, the editors and the reviewers. Any product that may be evaluated in this article or claim that may be made by its manufacturer is not guaranteed or endorsed by the publisher.
Research integrity at Frontiers
Learn more about the work of our research integrity team to safeguard the quality of each article we publish.