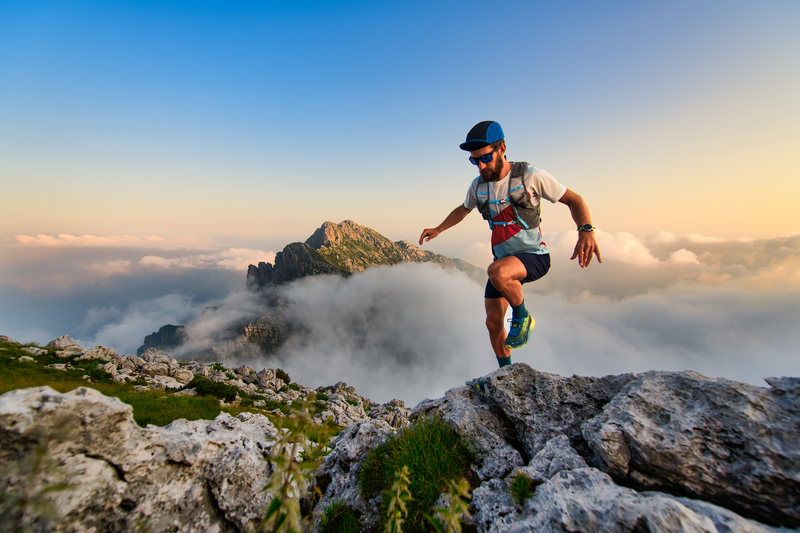
94% of researchers rate our articles as excellent or good
Learn more about the work of our research integrity team to safeguard the quality of each article we publish.
Find out more
ORIGINAL RESEARCH article
Front. Plant Sci. , 16 August 2021
Sec. Plant Breeding
Volume 12 - 2021 | https://doi.org/10.3389/fpls.2021.665122
Wheat (Triticum aestivum L.) is one of the most important crops in the world. Here, four yield-related traits, namely, spike length, spikelets number, tillers number, and thousand-kernel weight, were evaluated in 272 Chinese wheat landraces in multiple environments. Five multi-locus genome-wide association studies (FASTmrEMMA, ISIS EN-BLASSO, mrMLM, pKWmEB, and pLARmEB) were performed using 172,711 single-nucleotide polymorphisms (SNPs) to identify yield-related quantitative trait loci (QTL). A total of 27 robust QTL were identified by more than three models. Nine of these QTL were consistent with those in previous studies. The remaining 18 QTL may be novel. We identified a major QTL, QTkw.sicau-4B, with up to 18.78% of phenotypic variation explained. The developed kompetitive allele-specific polymerase chain reaction marker for QTkw.sicau-4B was validated in two recombinant inbred line populations with an average phenotypic difference of 16.07%. After combined homologous function annotation and expression analysis, TraesCS4B01G272300 was the most likely candidate gene for QTkw.sicau-4B. Our findings provide new insights into the genetic basis of yield-related traits and offer valuable QTL to breed wheat cultivars via marker-assisted selection.
Wheat (Triticum aestivum L.) is one of the most important crops in the world. A 100% increase in crop production by 2050 will be needed to keep pace with projected human population growth (Ray et al., 2013). Thus, it is imperative to increase crop yield. Wheat yield consists of three main components, including spike number per plant, grain number per spike, and thousand-kernel weight (TKW). Spike number per plant is determined by tillers number (TN) per plant. Spikelets number (SN) per spike and spike length (SL) significantly affect grain number per spike. Understanding the genetic basis of these yield-related traits can contribute to improving wheat yield.
Chinese wheat landraces have been widely used for breeding cultivated varieties of wheat (Dai et al., 2009). Wheat landraces show high genetic diversity and extensive phenotypic variation, such as early maturity, high numbers of kernel per spikelet, and good adaption to local environmental conditions (He and Huang, 2001; Hao et al., 2008). Genetic analyses of Chinese wheat landraces have revealed the basis of agronomic traits, such as yield-related traits (spikelets number per spike, tillers number, and thousand-kernel weight), plant morphological traits (flag leaf length, flag leaf width, and plant height; Liu et al., 2017; Ma et al., 2020), stress resistance (pre-harvest sprouting, drought-related traits, and phosphorus-deficiency tolerance; Zhou et al., 2017; Lin et al., 2019, 2020a), and disease resistance (powdery mildew and stripe rust; Xiao et al., 2013; Long et al., 2019). Analysis of gene diversity and polymorphism information content revealed the high diversity of Chinese wheat landraces (Liu et al., 2017; Long et al., 2019). Thus, genetic analysis of yield traits using Chinese wheat landraces can provide important insights into wheat breeding.
With the development of next-generation sequencing (NGS), genome-wide association study (GWAS) has become an effective way of detecting complex quantitative characteristics and is also widely applied in Arabidopsis (Atwell et al., 2010; Bac-Molenaar et al., 2016), rice (Huang et al., 2010; Zhu et al., 2016), maize (Lu et al., 2010, 2012; Yang et al., 2014), Aegilops tauschii (Liu et al., 2015a,b; Qin et al., 2015, 2016), and wheat (Liu et al., 2014, 2017; Maccaferri et al., 2015; Sukumaran et al., 2015; Lin et al., 2017). Moreover, the previous studies have discovered genes via GWAS directly. In rice, Yano identified a gene comprehensively controlling rice architecture using GWAS (Yano et al., 2019). Kim reported a novel resistance gene, Xa43(t), for bacterial blight (Kim and Reinke, 2019). In particular, GWAS has gradually been applied to wheat landraces. Using GWAS, a total of 149 significant markers for 21 agronomic traits were detected in 723 wheat landraces (Liu et al., 2017). A total of 51 loci significantly associated with adult-plant resistance to stripe rust were discovered in wheat landrace through GWAS (Long et al., 2019). Recently, a major locus of coleoptile length on chromosome 6B was revealed by GWAS in 707 Chinese wheat landraces (Ma et al., 2020).
Because of the mass of data involved in the process of GWAS, several multi-locus models have been designed to increase efficiency (Wang et al., 2016). Compared with the single-locus model, the multi-locus models can help improve the detection power of GWAS (Xu et al., 2018). Therefore, multi-locus models have recently been popularized in plant GWAS, such as in the photosynthetic response to low phosphorus stress in soybean (Lü et al., 2018), fatty acid content in rapeseed (Guan et al., 2019), forage quality-related traits in sorghum (Li et al., 2018), salt tolerance of direct seeding in rice (Cui et al., 2018), callus regenerative traits, starch pasting properties, and stalk lodging resistance-related traits in maize (Ma et al., 2018; Xu et al., 2018; Zhang et al., 2018), agronomic traits in barley (Hu et al., 2018), and free amino acid levels in wheat (Peng et al., 2018). All these studies have successfully discovered novel quantitative trait loci (QTL).
In the present study, a total of 272 Chinese wheat landraces were evaluated in multiple environments to improve our understanding of the genetic basis of four yield-related traits. Five multi-locus GWAS models were performed to identify robust QTL using 172,711 SNPs. Major QTL were validated in two recombinant inbred line (RIL) populations. Furthermore, we presumed candidate genes for the major QTL. This study provides new QTL of yield-related traits that may help wheat breading in the near future.
A total of 272 wheat landraces, obtained from 10 major wheat-growing zones in China, were utilized in this study (Supplementary Table S1). All landraces were planted in six environments: Ya’an (103°370 E, 22°420 N) in 2012 (E1), Wenjiang (103°410 E, 30°360 N) in 2013, 2014, and 2015 (E2, E3, and E4), and Chongzhou (103°390 E, 30°330 N) in 2014 and 2015 (E5 and E6). Each row of material was 30 cm apart and 1.5 m long, and contained 15 seeds. Field management referred to criteria commonly practiced in wheat production. Four yield-related traits were evaluated in at least four environments: SL – the average length of the main spikes from five plants; SN – the average total number of spikelets from five main spikes of the plant; TN – the average number of tillers in five plants; TKW – the average weight of five samples of 1,000 kernels randomly selected from a given genotype. Two bi-parental populations [Huimai × Datianquxiaomai (HD) and Huimai × Heshangmai (HH)] were used to validate the results. The parents of bi-parental populations were selected from 272 wheat landraces. The TKW of these two RIL populations was evaluated in Chongzhou in 2019, and Huimai resulted significantly more productive than Datianquxiaomai and Heshangmai (higher TKW).
To eliminate environmental effects, the best linear unbiased prediction (BLUP) value for each trait was calculated across environments and used for statistical analysis. The BLUP was calculated using the methodology of Piepho et al. (2008) as previously described (Liu et al., 2017). The broad-sense heritability (H2) value was calculated using SAS v8.1 (SAS Institute Inc., Cary, NC, United States) and is defined as:
where Vg, Vge, and Ve are the estimates of genetic variance, the genotype × environment interaction, and environmental variance, respectively (Smith et al., 1998). Correlation analyses were performed using SPSS 20 (IBM SPSS Statistics; IBM Corp., Armonk, NY, United States).
Genomic DNA was extracted from a single plant for each of the accessions using the cetyltrimethylammonium bromide method (Murray and Thompson, 1980). DNA samples with an A260/280 ratio of 1.8–2.0 and a concentration of >80 ng μl−1 were used for genotyping. The 272 accessions had been already genotyped by wheat 660 K array, including 630,517 probes (Axiom® Wheat660 SNP array; Affymetrix, Santa Clara, CA, United States) by Zhou et al. (2017). The software, bowtie2, was employed in this study to obtain physical locations of 172,711 SNPs based on IWGSC RefSeq v1.0.1
The population structure was performed by Structure2.3.4 based on the Bayesian clustering algorithm (Pritchard et al., 2000). Ten runs of STRUCTURE were performed with a K between 1 and 10 using the admixture model with 100,000 replicates for burn-in and 100,000 replicates for MCMC. The R package “mrMLM” in R Project for Statistical Computing was used to examine the association between markers and yield-related traits. Marker-trait associations were performed using five multi-locus models, including FASTmrEMMA (Wen et al., 2017), ISIS EM-BLASSO (Tamba et al., 2017), mrMLM (Wang et al., 2016), pKWmEB (Ren et al., 2018), and pLARmEB (Zhang et al., 2017). All five models were adjusted by both population structure and family relationship. A logarithm of odds (LOD) value ≥3 was used as the screening criterion (Guan et al., 2019). According to previous studies, the linkage disequilibrium decay of wheat ranges from 3.5 to 23 Mb (Kidane et al., 2019; Li et al., 2019; Luján Basile et al., 2019). Significant SNPs were therefore selected with a physical distance ≤10 Mb and referred to as a conservative QTL.
To further validate the significant QTL identified for TKW, the peak SNP with this locus was converted into a kompetitive allele-specific polymerase chain reaction (KASP) marker based on the probe sequence. KASP primers were designed and analyzed as described in previous studies (Lin et al., 2020b, 2021) and produced by Sangon Biotech (Shanghai, China; Supplementary Table S2). The KASP marker detected different alleles at this locus in the two bi-parental populations. Eighty-two lines were selected for each of the alleles from both populations. These lines were used to evaluate differences in TKW between the two allele groups using a Student’s t-test in SPSS 20 (IBM SPSS Statistics; IBM Corp., Armonk, NY, United States).
Based on IWGSC RefSeq v1.0, predicted genes within ~10 Mb of the physical location of the QTL were selected. We undertook two different methods to predict the possible existence of candidate genes. The first method was expression analysis. Based on data from WheatExp,2 genes expressed highly at stages Z71 and Z75 were the most important, due to the key stages in kernel development (Zadoks et al., 1974). Fragments per kilobase of exon model (FPKM) represented gene expression level. As in Lin et al. (2020b), genes expressing less than two per million mapped reads at any stage were removed. FPKM is fragments read per thousand bases per million mappings and represented gene expression level.
The second method to predict the possible existence of candidate genes was annotation. All genes were also used to perform homologous annotations in rice and Arabidopsis using the KEGG Orthology Based Annotation System 3.0 (KOBAS 3.0; Xie et al., 2011).3 Functional annotations were performed via UniProt4 and EnsemblPlants.5
The four traits among the 272 wheat landraces varied considerably (Table 1). Based on the BLUP values, the SL ranged from 6.33 to 14.63 (cm). SN ranged from 19.43 to 27.36 (count). TN ranged from 8.11 to 18.10 (count), and TKW ranged from 17.90 to 40.47 (g). The heritability of these four traits ranged from 0.64 to 0.93 (Table 1). TN and TKW showed medium heritability, whereas SL and SN showed high heritability. Correlation analysis showed that all correlations were significant (p < 0.05), except the correlation between SL and SN (Table 2), indicating that the changes in these two traits are independent.
Table 1. Phenotype variation and heritability of spike length (SL), spikelets number per spike (SN), tillers number (TN), and thousand-kernel weight (TKW) based on BLUP values for the 272 accessions.
Table 2. Correlation of spike length (SL), spikelets number per spike (SN), tillers number (TN), and TKW based on BLUP values.
A total of 172,711 polymorphic SNPs were obtained [after filtering for missing values ≤10% and minor allele frequency (MAF) ≥0.05] from the Wheat 660 K SNP arrays. This subset was used to perform GWAS. Based on LOD values ≥3, a total of 308 significant SNP markers were identified using the five multi-locus models. Detailed significant SNP markers information and the number of significant SNP markers detected by different models are shown in Supplementary Table S3 and Figure 1, respectively. In FASTmrEMMA, the least number of significant SNP markers was detected, with only 35. A total of 10, 8, 6, and 11 significant SNP markers were detected for SL, SN, TN, and TKW, respectively, with phenotypic variation explained (PVE) up to 6.55%. In the model of ISIS EM-BLASSO, 73 significant SNP markers were revealed. A total of 20, 23, 15, and 15 were detected for SL, SN, TN, and TKW, respectively, with PVE up to 9.27%. The mrMLM model could detect 67 significant markers. A total of 18, 20, 14, and 15 were detected for SL, SN, TN, and TKW, respectively, with PVE up to 13.09%. In the model of pKWmEB, the most number of significant SNP markers was detected (81), and a total of 23, 25, 19, and 14 were detected for SL, SN, TN, and TKW, respectively, with PVE up to 18.78%. The pLARmEB model detected 52 significant SNP markers, and 12, 15, 11, and 14 were detected for SL, SN, TN, and TKW, respectively, with PVE up to 11.09%. Significant markers existed in the above three models, and no more than 10 Mb was considered as a robust QTL.
Figure 1. Comparison of the number of detected quantitative trait loci (QTL) from the five methods. The five methods are mrMLM, FASTmrEMMA, ISIS EM-BLASSO, pLARmEB, and pKWmEB. The traits included spike length (SL), spikelets number per spike (SN), thousand-kernel weight, and tillers number (TN). Total denotes the total QTL number for each trait.
Twenty-seven robust QTL were identified by more than three different multi-locus models and were considered as robust QTL (Table 3).
Seven QTL associated with SL were identified on chromosomes 1A, 1D, 2A, 2B, 3A, 4A, and 7B, with the PVE up to 11.44%, and the LOD values ranging from 3.45 to 14.68. QSl.sicau-3A, located at 650.86–651.84 Mb, was identified in four models, with LOD values up to 14.68. Nine QTL associated with SN were identified on chromosomes 1B, 2A, 2B, 3B, 5A, and 7A, with PVE up to 7.61%, and LOD values up to 15.85. QSn.sicau-7A, located at 671.48 Mb, was detected by four models. The highest LOD (15.85) and PVE (7.61%) values on this QTL were detected by the pKWmEB model. Four QTL for TN were identified on chromosomes 1B, 2B, 5B, and 6D, with a PVE up to 9.58%, and the LOD values up to 8.42. QTn.sicau-6D, located at 469.60–472.74 Mb, had the highest PVE value which was 9.58%. Seven QTL associated with TKW were identified on chromosomes 2B, 3A, 4B, 6B, 7A, and 7B, with PVE values up 18.78%, and the LOD values up to 12.04. QTkw.sicau-4B, identified by the pKWmEB model, explained the highest PVE (18.78%). Interestingly, among all robust QTL detected in the present study, QTkw.sicua-4B resulted that with the highest PVE value. It can be regarded as a major QTL, contributing to breed more productive wheat cultivars, so it was validated in the first instance.
To validate the genetic effect of the peak SNP for QTkw.sicau-4B, a KASP marker (KASP-AX-108886949) was developed and the differences between TKW of landraces carrying alternatively A/G allele were calculated, both in natural population and in two RIL populations. Among the 272 landraces tested, the average TKW of landraces carrying genotype allele A was heavier than those carrying allele G (p < 0.01; Table 4; Figure 2A). The difference was 22.87%. In the HD population, genotypes were divided into two groups: 42 with allele A and 40 with allele G. The TKW of genotypes with allele A ranged from 28.75 to 44.04 g, whereas those with allele G ranged from 22.28 to 39.27 g. The average TKW with allele A was heavier than that with allele G (p < 0.01; Table 4; Figure 2B). In the RIL population HH, there were 39 with allele A and 43 with allele G. The TKW of genotypes with allele A ranged from 22.52 to 44.56 (g), whereas those with allele G ranged from 19.03 to 37.47 g. The average TKW with allele A was heavier than with allele G (p < 0.01; Table 4; Figure 2C). The difference in TKW between genotypes ranged from 15.33 to 16.81%, with an average value of 16.07% among the two RIL populations. Thus, the developed KASP marker may be useful for breeding cultivars carrying a high-TKW allele.
Figure 2. Alleles effects of QTkw.sicau-4B in (A) natural (B) HD, and (C) HH populations. HD: Huimai × Datianquxiaomai; HH: Huimai × Heshangmai; BLUP, best linear unbiased prediction; and 2019CZ: in Chongzhou in 2019. ** significant at p < 0.01.
We observed superior allele A frequency in 10 major wheat-growing zones (Figure 3). The frequencies show that the superior allele A showed an 8% frequency in Chinese wheat landraces, and more than half the materials from the Northern Spring Wheat Zone and the Northwestern Spring Wheat Zone carried allele A. The Northern Winter Wheat Zone, Yellow and Huai River Valleys Facultative Wheat Zone, Middle and Low Yangtze Valleys Autumn-Sown Spring Wheat Zone, Southwestern Autumn-Sown Spring Wheat Zone, and Qinghai-Tibetan Plateau Spring–Winter also had some materials with allele A. Materials from the Southern Autumn-Sown Spring Wheat Zone, Northeastern Spring Wheat Zone, and Xinjiang Winter–Spring Wheat Zone did not carry allele A.
Figure 3. Allele frequency of QTkw.sicau-4B in 10 major wheat-growing zones. Blue means allele A proportion in Chinese wheat landrace and red means allele G proportion in Chinese wheat landrace. NW (Northern Winter Wheat Zone), Y&H (Yellow and Huai River Valleys Facultative Wheat Zone), YTS (Middle and Low Yangtze Valleys Autumn-Sown Spring Wheat Zone), SAS (Southern Autumn-Sown Spring Wheat Zone), SWAS (Southwestern Autumn-Sown Spring Wheat Zone), NES (Northeastern Spring Wheat Zone), NS (Northern Spring Wheat Zone), NWS (Northwestern Spring Wheat Zone), Q&T (Qinghai-Tibetan Plateau Spring–Winter Wheat Zone), and XJ (Xinjiang Winter–Spring Wheat Zone).
A total of 46 high-confidence genes which were identified in IWGSC RefSeq v1.0 flanked QTkw.sicau-4B by no more 5 Mb. The search for candidate genes was undertaken following two pipelines. The expression analysis of the candidates was investigated in the two most interesting stages for kernel development: Z71 and Z75. Nine genes were expressed at both stages, namely, TraesCS4B01G272300, TraesCS4B01G272500, TraesCS4B01G273400, TraesCS4B01G273500, TraesCS4B01G274200, TraesCS4B01G274500, TraesCS4B01G275400, TraesCS4B01G275500, and TraesCS4B01G276300. Based on these sequences, we found homologous genes using KOBAS 3.0 (see footnote 3). Three genes were identified as TKW-regulating candidate genes (Table 5). TraesCS4B01G272300, TraesCS4B01G275400, and TraesCS4B01G276200 were homologous to OsMCA1 (Liu et al., 2015c), H2B (Ma et al., 2019a), and EDR1 (Prasad et al., 2009) in rice, respectively.
Yield has always been a focus of global wheat research. With the development of NGS technology, GWAS has been applied extensively to detect complex traits (Liu et al., 2014; Maccaferri et al., 2015; Sukumaran et al., 2015). Previous studies found that the accuracy and efficiency of models, including generalized linear, mixed linear, and multi-locus models, have constantly improved (Zhang et al., 2005; Price et al., 2006; Li et al., 2018). In the present study, we observed not consistent results between different multi-locus models. FASTmrEMMA was more conservative, and pKWmEB was the most effective model of the five models (Supplementary Table S3; Figure 1); this finding is in agreement with the previous studies (Li et al., 2018; Lü et al., 2018). QTL detected by multiple, multi-locus models are considered as robust QTL useful for more precise studies. Among the 308 significant SNP markers, 27 reliable and robust QTL were identified in four yield-related traits in this study.
Using BLAST against the IWGSC RefSeq v1.0, we tried to determine the physical location of QTL flanking markers. Among these 27 robust QTL, nine loci (QSl.sicau-1A, QSl.sicau-2A, QSl.sicau-2B, QSn.sicua-2A.1, QSn.sicau-2A.3, QSn.sicua-5A, QSn.sicau-7A, QTn.sicua-6D, and QTkw.sicau-3A) were overlapped with, or located close to, previously reported QTL.
For the trait of SL, three QTL identified in present study were reported previously (QSl.sicau-1A, QSl.sicau-2A, and QSl.sicau-2B). QTL for SL located at 572.23 to 572.35 Mb, between markers Xmwg632 and Xbarc213 (Yu et al., 2014), were close to QSl.sicau-1A (566.11–568.36 Mb). QSl.sicau-2A (735.26–742.14 Mb) resulted overlapping with QEl.fcu-2A, reported by Faris et al. (2014) and flanked by Xwmc181 (728.6 1 Mb) and fcp651 (738.43 Mb) markers. Moreover, QSl.sicau-2B (29.19–29.71 Mb) overlapped with the previous reported QTL: Qsl2B.2 flanking by Xbarc200 at 26.59 Mb (Xu et al., 2014) and flanking by excalibur_c40567_1893 at 28.37 Mb (Zhai et al., 2016).
For SN, four robust QTL identified in the present study were reported previously. QSns.sau-2A.1 (Ma et al., 2019b) exists between markers AX-111610554 and AX-110495160, located at 35.78 to 36.00 Mb, and is close to our QSn.sicua-2A.1 (31.70–32.45 Mb). QSn.sicau-2A.3 for SN located on chromosome 2A at 741.83–741.98 Mb resulted close to QCmp.fcu-2A identified between Xwmc181 and Xfcp651 at 728.60–738.44 Mb (Faris et al., 2014). Wang et al. (2011) discovered a robust QTL controlling spikelets number per spike in three environments; the QTL detected with marker Xgwm126 (671.4 Mb) and Xgwm291 (698.2 Mb), and overlapped with QSn.sicua-5A (678.62–682.92 Mb) in the present study. QSn.sicau-7A, located on chromosome 7A at 671.48 Mb, is close to TaAPO1, located at 674.08 Mb, and confirmed to play an important role in regulating SN (Kuzay et al., 2019; Ma et al., 2019b; Muqaddasi et al., 2019).
For TN, Bilgrami et al. (2020) reported a QTL MQTL6D-4 in physical position 456.46–469.25 Mb with a mean R2 of 14.08%, which is close to QTn.sicau-6D (469.60–472.74 Mb) in the present study.
For TKW, using a doubled haploid population Zhang et al. (2014) identified a QTL for TKW on chromosome 3A (Qtkw3A-1) at 625.79–690.79 Mb, which is close to QTkw.sicau-3A, and mapped at 686.12 Mb in this study. In general, nine QTL were identified in the same position as those in previous studies; the remaining 18 are potentially novel QTL.
Among robust and novel QTL, we focused on QTkw.sicau-4B that showed the highest PVE value. To validate this major QTL for TKW, we developed a KASP marker according to the sequence of peak marker, AX-108886949 (Supplementary Table S2). According to high PVE value and low frequency, we believe this QTL could help wheat breeding.
Three genes identified by functional annotation showed relationship with TKW. TraesCS4B01G275400 is orthologous to H2B (Histone H2B monoubiquitination), which regulates abscisic acid signaling, and is a target of UBP26, which acts as a transcriptional repressor involved in kernel development (Ma et al., 2019a). TraesCS4B01G276200 is orthologous to OsCDR1. Weights between OsCDR1 transgenic lines and wild-type plants differed (Prasad et al., 2009). TraesCS4B01G272300 is orthologous to rice OsMCA1, which mutants showed obviously affected TKW (Liu et al., 2015c). TraesCS4B01G272300 gene, highly expressed in the grain development stage (Supplementary Figure S1), appears to be the most promising candidate gene for QTkw.sicau-4B. In our further study, expressions of this candidate gene will be validated by qRT-PCR in different grain development stage. Transgenic tests will also be applied to validate its function.
The original contributions presented in the study are included in the article/Supplementary Material, further inquiries can be directed to the corresponding author.
YL and KYZ drafted and revised the manuscript and contributed to data analysis. HYH, JXJ, SFY, and QW performed the phenotypic evaluation and helped with data analysis. CXL, JM, GDC, and ZSY helped to draft the manuscript. YXL designed and coordinated the study and revised the manuscript. All authors have read and approved the final manuscript for publication.
This study was supported by the National Natural Science Foundation of China (31771794), the National Key Research and Development Program of China (2016YFD0101004 and 2017YFD0100900), and the International Science & Technology Cooperation Program of the Bureau of Science and Technology of Chengdu China (No. 2015DFA306002015-GH03-00008-HZ).
The authors declare that the research was conducted in the absence of any commercial or financial relationships that could be construed as a potential conflict of interest.
All claims expressed in this article are solely those of the authors and do not necessarily represent those of their affiliated organizations, or those of the publisher, the editors and the reviewers. Any product that may be evaluated in this article, or claim that may be made by its manufacturer, is not guaranteed or endorsed by the publisher.
The authors thank Dr. Lihui Li (Chinese Academy of Agricultural Sciences, China) for providing plant materials.
The Supplementary Material for this article can be found online at https://www.frontiersin.org/articles/10.3389/fpls.2021.665122/full#supplementary-material
1. ^http://www.wheatgenome.org/
2. ^https://wheat.pw.usda.gov/WheatExp/
Atwell, S., Huang, Y. S., Vilhjalmsson, B. J., Willems, G., Horton, M., Li, Y., et al. (2010). Genome-wide association study of 107 phenotypes in Arabidopsis thaliana inbred lines. Nature 465, 627–631. doi: 10.1038/nature08800
Bac-Molenaar, J. A., Granier, C., Keurentjes, J. J., and Vreugdenhil, D. (2016). Genome-wide association mapping of time-dependent growth responses to moderate drought stress in Arabidopsis. Plant Cell Environ. 39, 88–102. doi: 10.1111/pce.12595
Bilgrami, S. S., Ramandi, H. D., Shariati, V., Razavi, K., Tavakol, E., Fakheri, B. A., et al. (2020). Detection of genomic regions associated with tiller number in Iranian bread wheat under different water regimes using genome-wide association study. Sci. Rep. 10:14034. doi: 10.1038/s41598-020-69442-9
Cui, Y., Zhang, F., and Zhou, Y. (2018). The application of multi-locus GWAS for the detection of salt-tolerance loci in rice. Front. Plant Sci. 9:1464. doi: 10.3389/fpls.2018.01464
Dai, S., Yan, Z., Liu, D., Zhang, L., Wei, Y., and Zheng, Y. (2009). Evaluation on Chinese bread wheat landraces for low pH and aluminum tolerance using hydroponic screening[J]. Agric. Sci. China 8, 285–292. doi: 10.1016/S1671-2927(08)60211-4
Faris, J. D., Zhang, Z., Garvin, D. F., and Xu, S. S. (2014). Molecular and comparative mapping of genes governing spike compactness from wild emmer wheat. Mol. Gen. Genomics. 289, 641–651. doi: 10.1007/s00438-014-0836-2
Guan, M., Huang, X., Xiao, Z., Jia, L., Wang, S., Zhu, M., et al. (2019). Association mapping analysis of fatty acid content in different ecotypic rapeseed using mrMLM. Front. Plant Sci. 9:1872. doi: 10.3389/fpls.2018.01872
Hao, C., Dong, Y., Wang, L., You, G., Zhang, H., Ge, H., et al. (2008). Genetic diversity and construction of core collection in Chinese wheat genetic resources. Chin. Sci. Bull. 53, 1518–1526. doi: 10.1007/s11434-008-0212-x
Hu, X., Zuo, J., Wang, J., Liu, L., Sun, G., Li, C., et al. (2018). Multi-locus genome-wide association studies for 14 main agronomic traits in barley. Front. Plant Sci. 9:1683. doi: 10.3389/fpls.2018.01683
Huang, X., Wei, X., Sang, T., Zhao, Q., Feng, Q., Zhao, Y., et al. (2010). Genome-wide association studies of 14 agronomic traits in rice landraces. Nat. Genet. 42, 961–967. doi: 10.1038/ng.695
Kidane, Y. G., Gesesse, C. A., Hailemariam, B. N., Desta, E. A., Mengistu, D. K., Fadda, C., et al. (2019). A large nested association mapping population for breeding and quantitative trait locus mapping in Ethiopian durum wheat. Plant Biotechnol. J. 17, 1380–1393. doi: 10.1111/pbi.13062
Kim, S. M., and Reinke, R. F. (2019). A novel resistance gene for bacterial blight in rice, Xa43(t) identified by GWAS, confirmed by QTL mapping using a bi-parental population. PLoS One 14:e211775. doi: 10.1371/journal.pone.0211775
Kuzay, S., Xu, Y., Zhang, J., Katz, A., Pearce, S., Su, Z., et al. (2019). Identification of a candidate gene for a QTL for spikelet number per spike on wheat chromosome arm 7AL by high-resolution genetic mapping. Theor. Appl. Genet. 132, 2689–2705. doi: 10.1007/s00122-019-03382-5
Li, J., Tang, W., Zhang, Y., Chen, K., Wang, C., Liu, Y., et al. (2018). Genome-wide association studies for five forage quality-related traits in sorghum (Sorghum bicolor L.). Front. Plant Sci. 9:1146. doi: 10.3389/fpls.2018.01146
Li, G., Xu, X., Tan, C., Carver, B. F., Bai, G., Wang, X., et al. (2019). Identification of powdery mildew resistance loci in wheat by integrating genome-wide association study (GWAS) and linkage mapping. Crop J. 7, 294–306. doi: 10.1016/j.cj.2019.01.005
Lin, Y., Chen, G., Hu, H., Yang, X., Zhang, Z., Jiang, X., et al. (2020a). Phenotypic and genetic variation in phosphorus-deficiency-tolerance traits in Chinese wheat landraces. BMC Plant Biol. 20:330. doi: 10.1186/s12870-020-02492-3
Lin, Y., Jiang, X., Hu, H., Zhou, K., Wang, Q., Yu, S., et al. (2021). QTL mapping for grain number per spikelet in wheat using a high-density genetic map. Crop J. doi: 10.1016/j.cj.2020.12.006 (in press).
Lin, Y., Jiang, X., Tao, Y., Yang, X., Wang, Z., Wu, F., et al. (2020b). Identification and validation of stable quantitative trait loci for grain filling rate in common wheat (Triticum aestivum L.). Theor. Appl. Genet. 133, 2377–2385. doi: 10.1007/s00122-020-03605-0
Lin, Y., Liu, S., Liu, Y., Liu, Y., Chen, G., Xu, J., et al. (2017). Genome-wide association study of pre-harvest sprouting resistance in Chinese wheat founder parents. Genet. Mol. Biol. 40, 620–629. doi: 10.1590/1678-4685-gmb-2016-0207
Lin, Y., Yi, X., Tang, S., Chen, W., Wu, F., Yang, X., et al. (2019). Dissection of phenotypic and genetic variation of drought-related traits in diverse Chinese wheat landraces. Pant Genome 12. doi: 10.3835/plantgenome2019.03.0025
Liu, Z., Cheng, Q., Sun, Y., Dai, H., Song, G., Guo, Z., et al. (2015c). A SNP in OsMCA1 responding for a plant architecture defect by deactivation of bioactive GA in rice. Plant Mol. Biol. 87, 17–30. doi: 10.1007/s11103-014-0257-y
Liu, Y., Lin, Y., Gao, S., Li, Z., Ma, J., Deng, M., et al. (2017). A genome-wide association study of 23 agronomic traits in Chinese wheat landraces. Plant J. 91, 861–873. doi: 10.1111/tpj.13614
Liu, Y., Wang, L., Deng, M. L., Li, Z., Lu, Y., Wang, J., et al. (2015a). Genome-wide association study of phosphorus-deficiency-tolerance traits in Aegilops tauschii. Theor. Appl. Genet. 128, 2203–2212. doi: 10.1007/s00122-015-2578-x
Liu, Y., Wang, L., Mao, S., Liu, K., Lu, Y., Wang, J., et al. (2015b). Genome-wide association study of 29 morphological traits in Aegilops tauschii. Sci. Rep. 5:15562. doi: 10.1038/srep15562
Liu, S., Yang, X., Zhang, D., Bai, G., Chao, S., Bockus, W., et al. (2014). Genome-wide association analysis identified SNPs closely linked to a gene resistant to soil-borne wheat mosaic virus. Theor. Appl. Genet. 127, 1039–1047. doi: 10.1007/s00122-014-2277-z
Long, L., Yao, F., Yu, C., Ye, X., Cheng, Y., Wang, Y., et al. (2019). Genome-wide association study for adult-plant resistance to stripe rust in Chinese wheat landraces (Triticum aestivum L.) From the Yellow and Huai River valleys. Front. Plant Sci. 10:596. doi: 10.3389/fpls.2019.00596
Lu, Y., Xu, J., Yuan, Z., Hao, Z., Xie, C., and Li, X. (2012). Comparative LD mapping using single SNPs and haplotypes identifies QTL for plant height and biomass as secondary traits of drought tolerance in maize. Mol. Breed. 30, 407–418. doi: 10.1007/s11032-011-9631-5
Lü, H., Yang, Y., Li, H., Liu, Q., Zhang, J., Yin, J., et al. (2018). Genome-wide association studies of photosynthetic traits related to phosphorus efficiency in soybean. Front. Plant Sci. 9:1226. doi: 10.3389/fpls.2018.01226
Lu, Y., Zhang, S., Shah, T., Xie, C., Hao, Z., and Li, X. (2010). Joint linkage-linkage disequilibrium mapping is a powerful approach to detecting quantitative trait loci underlying drought tolerance in maize. Proc. Natl. Acad. Sci. U. S. A. 107, 19585–19590. doi: 10.1073/pnas.1006105107
Luján Basile, S. M., Ramírez, I. A., Crescente, J. M., Conde, M. B., Demichelis, M., Abbate, P., et al. (2019). Haplotype block analysis of an Argentinean hexaploid wheat collection and GWAS for yield components and adaptation. BMC Plant Biol. 19:553. doi: 10.1186/s12870-019-2015-4
Ma, J., Ding, P., Liu, J., Li, T., Zou, Y., Habib, A., et al. (2019b). Identification and validation of a major and stably expressed QTL for spikelet number per spike in bread wheat. Theor. Appl. Genet. 132, 3155–3167. doi: 10.1007/s00122-019-03415-z
Ma, J., Lin, Y., Tang, S., Duan, S., Wang, Q., Wu, F., et al. (2020). A genome-wide association study of coleoptile length in different Chinese wheat landraces. Front. Plant Sci. 11:677. doi: 10.3389/fpls.2020.00677
Ma, L., Liu, M., Yan, Y., Qing, C., Zhang, X., Zhang, Y., et al. (2018). Genetic dissection of maize embryonic callus regenerative capacity using multi-locus genome-wide association studies. Front. Plant Sci. 9:561. doi: 10.3389/fpls.2018.00561
Ma, S., Tang, N., Li, X., Xie, Y., Xiang, D., Fu, J., et al. (2019a). Reversible histone H2B monoubiquitination fine-tunes abscisic acid signaling and drought response in rice. Mol. Plant 12, 263–277. doi: 10.1016/j.molp.2018.12.005
Maccaferri, M., Zhang, J., Bulli, P., Abate, Z., Chao, S., Cantu, D., et al. (2015). A genome-wide association study of resistance to stripe rust (Puccinia striiformis f. sp. tritici) in a worldwide collection of hexaploid spring wheat (Triticum aestivum L.). G3 5, 449–465. doi: 10.1534/g3.114.014563
Muqaddasi, Q. H., Brassac, J., Koppolu, R., Plieske, J., Ganal, M. W., Röder, M. S., et al. (2019). TaAPO-A1, an ortholog of rice ABERRANT PANICLE ORGANIZATION 1, is associated with total spikelet number per spike in elite European hexaploid winter wheat (Triticum aestivum L.) varieties. Sci. Rep. 9:13853. doi: 10.1038/s41598-019-50331-9
Murray, M. G., and Thompson, W. F. (1980). Rapid isolation of high molecular weight plant. DNA Nucleic Acids Res 8, 4321–4325. doi: 10.1093/nar/8.19.4321
Peng, Y., Liu, H., Chen, J., Shi, T., Zhang, C., Sun, D., et al. (2018). Genome-wide association studies of free amino acid levels by six multi-locus models in bread wheat. Front. Plant Sci. 9:1196. doi: 10.3389/fpls.2018.01196
Piepho, H. P., Möhring, J., Melchinger, A. E., and Büchse, A. (2008). BLUP for phenotypic selection in plant breeding and variety testing. Euphytica 161, 209–228. doi: 10.1007/s10681-007-9449-8
Prasad, B. D., Creissen, G., Lamb, C., and Chattoo, B. B. (2009). Overexpression of rice (Oryza sativa L.) OsCDR1 leads to constitutive activation of defense responses in rice and Arabidopsis. Mol. Plant-Microbe Interact. 22:1635. doi: 10.1094/MPMI-22-12-1635
Price, A. L., Patterson, N. J., Plenge, R. M., Weinblatt, M. E., Shadick, N. A., Reich, D., et al. (2006). Principal components analysis corrects for stratification in genome-wide association studies. Nat. Genet. 38, 904–909. doi: 10.1038/ng1847
Pritchard, J. K., Stephens, M., and Donnelly, P. (2000). Inference of population structure using multilocus genotype data. Genetics 155, 945–959. doi: 10.1093/genetics/155.2.945
Qin, P., Lin, Y., Hu, Y., Liu, K., Mao, S., Li, Z., et al. (2016). Genome-wide association study of drought-related resistance traits in Aegilops tauschii. Genet. Mol. Biol. 39, 398–407. doi: 10.1590/1678-4685-GMB-2015-0232
Qin, P., Wang, L., Liu, K., Mao, S., Li, Z., Gao, S., et al. (2015). Genome-wide association study of Aegilops tauschii traits under seedling-stage cadmium stress. Crop J. 3, 405–415. doi: 10.1016/j.cj.2015.04.005
Ray, D. K., Mueller, N. D., West, P. C., and Foley, J. A. (2013). Yield trends are insufficient to double global crop production by 2050. PLoS One 8:e66428. doi: 10.1371/journal.pone.0066428
Ren, W. L., Wen, Y. J., Dunwell, J. M., and Zhang, Y. M. (2018). pKWmEB: integration of Kruskal-Wallis test with empirical Bayes under polygenic background control for multi-locus genome-wide association study. Heredity 120, 208–218. doi: 10.1038/s41437-017-0007-4
Smith, S. E., Kuehl, R. O., Ray, I., Hui, R., and Soleri, D. (1998). Evaluation of simple methods for estimating broad-sense heritability in stands of randomly planted genotypes. Crop Sci. 38, 1125–1129. doi: 10.2135/cropsci1998.0011183X003800050003x
Sukumaran, S., Dreisigacker, S., Lopes, M., Chavez, P., and Reynolds, M. P. (2015). Genome-wide association study for grain yield and related traits in an elite spring wheat population grown in temperate irrigated environments. Theor. Appl. Genet. 128, 353–363. doi: 10.1007/s00122-014-2435-3
Tamba, C. L., Ni, Y. L., and Zhang, Y. M. (2017). Iterative sure independence screening EM-Bayesian LASSO algorithm for multi-locus genome-wide association studies. PLoS Comput. Biol. 13:e1005357. doi: 10.1371/journal.pcbi.1005357
Wang, S. B., Feng, J. Y., Ren, W. L., Huang, B., Zhou, L., Wen, Y. J., et al. (2016). Improving power and accuracy of genome-wide association studies via a multi-locus mixed linear model methodology. Sci. Rep. 6:19444. doi: 10.1038/srep19444
Wang, J., Liu, W., Wang, H., Li, L., Wu, J., Yang, X., et al. (2011). QTL mapping of yield-related traits in the wheat germplasm 3228. Euphytica 177, 277–292. doi: 10.1007/s10681-010-0267-z
Wen, Y. J., Zhang, H., Ni, Y. L., Huang, B., Zhang, J., Feng, J. Y., et al. (2017). Methodological implementation of mixed linear models in multi-locus genome-wide association studies. Brief. Bioinform. 18:906. doi: 10.1093/bib/bbx028
Xiao, M., Song, F., Jiao, J., Wang, X., Xu, H., and Li, H. (2013). Identification of the gene Pm47 on chromosome 7BS conferring resistance to powdery mildew in the Chinese wheat landrace Hongyanglazi. Theor. Appl. Genet. 126, 1397–1403. doi: 10.1007/s00122-013-2060-6
Xie, C., Mao, X., Huang, J., Ding, Y., Wu, J., Dong, S., et al. (2011). KOBAS 2.0: a web server for annotation and identification of enriched pathways and diseases. Nucleic Acids Res. 39(suppl.2), W316–W322. doi: 10.1093/nar/gkr483
Xu, Y., Wang, R., Tong, Y., Zhao, H., Xie, Q., Liu, D., et al. (2014). Mapping QTLs for yield and nitrogen-related traits in wheat: influence of nitrogen and phosphorus fertilization on QTL expression. Theor. Appl. Genet. 127, 59–72. doi: 10.1007/s00122-013-2201-y
Xu, Y., Yang, T., Zhou, Y., Yin, S., Li, P., Liu, J., et al. (2018). Genome-wide association mapping of starch pasting properties in maize using single-locus and multi-locus models. Front. Plant Sci. 9:1311. doi: 10.3389/fpls.2018.01311
Yang, N., Lu, Y., Yang, X., Huang, J., Zhou, Y., and Ali, F. (2014). Genome wide association studies using a new nonparametric model reveal the genetic architecture of 17 agronomic traits in an enlarged maize association panel. PLoS Genet. 10:e1004573. doi: 10.1371/journal.pgen.1004573
Yano, K., Morinaka, Y., Wang, F., Huang, P., Takehara, S., Hirai, T., et al. (2019). GWAS with principal component analysis identifies a gene comprehensively controlling rice architecture. Proc. Natl. Acad. Sci. 116, 21262–21267. doi: 10.1073/pnas.1904964116
Yu, M., Mao, S., Chen, G., Pu, Z., Wei, Y., Zheng, Y., et al. (2014). QTLs for uppermost internode and spike length in two wheat RIL populations and their affect upon plant height at an individual QTL level. Euphytica 200, 95–108. doi: 10.1007/s10681-014-1156-7
Zadoks, J. C., Chang, T. T., and Konzak, C. F. (1974). A decimal code for the growth stages of cereals. Weed Res. 14, 415–421. doi: 10.1111/j.1365-3180.1974.tb01084.x
Zhai, H., Feng, Z., Li, J., Liu, X., Xiao, S., Ni, Z., et al. (2016). QTL analysis of spike morphological traits and plant height in winter wheat (Triticum aestivum L.) using a high-density SNP and SSR-based linkage map. Front. Plant Sci. 7:01617. doi: 10.3389/fpls.2016.01617
Zhang, X., Deng, Z., Wang, Y., Li, J., and Tian, J. (2014). Unconditional and conditional QTL analysis of kernel weight related traits in wheat (Triticum aestivum L.) in multiple genetic backgrounds. Genetica 142, 371–379. doi: 10.1007/s10709-014-9781-6
Zhang, J., Feng, J. Y., Ni, Y. L., Wen, Y. J., Niu, Y., Tamba, C. L., et al. (2017). pLARmEB: integration of least angle regression with empirical Bayes for multilocus genome-wide association studies. Heredity 118, 517–524. doi: 10.1038/hdy.2017.8
Zhang, Y., Liu, P., Zhang, X., Zheng, Q., Chen, M., Ge, F., et al. (2018). Multi-locus genome-wide association study reveals the genetic architecture of stalk lodging resistance-related traits in maize. Front. Plant Sci. 9:611. doi: 10.3389/fpls.2018.00611
Zhang, Y. M., Mao, Y., Xie, C., Smith, H., Luo, L., et al. (2005). Mapping quantitative trait loci using naturally occurring genetic variance among commercial inbred lines of maize (Zea mays L.). Genetics 169, 2267–2275. doi: 10.1534/genetics.104.033217
Zhou, Y., Tang, H., Cheng, M. P., Dankwa, K. O., Chen, Z. X., Li, Z. Y., et al. (2017). Genome-wide association study for pre-harvest sprouting resistance in a large germplasm collection of Chinese wheat landraces. Front. Plant Sci. 8:401. doi: 10.3389/fpls.2017.00401
Keywords: validation, wheat landraces, candidate genes, quantitative trait loci, association study, yield-related traits
Citation: Lin Y, Zhou K, Hu H, Jiang X, Yu S, Wang Q, Li C, Ma J, Chen G, Yang Z and Liu Y (2021) Multi-Locus Genome-Wide Association Study of Four Yield-Related Traits in Chinese Wheat Landraces. Front. Plant Sci. 12:665122. doi: 10.3389/fpls.2021.665122
Received: 07 February 2021; Accepted: 20 July 2021;
Published: 16 August 2021.
Edited by:
Laurent Gentzbittel, Skolkovo Institute of Science and Technology, RussiaReviewed by:
Delfina Barabaschi, Council for Agricultural and Economics Research, ItalyCopyright © 2021 Lin, Zhou, Hu, Jiang, Yu, Wang, Li, Ma, Chen, Yang and Liu. This is an open-access article distributed under the terms of the Creative Commons Attribution License (CC BY). The use, distribution or reproduction in other forums is permitted, provided the original author(s) and the copyright owner(s) are credited and that the original publication in this journal is cited, in accordance with accepted academic practice. No use, distribution or reproduction is permitted which does not comply with these terms.
*Correspondence: Yaxi Liu, bGl1eWF4aUBzaWNhdS5lZHUuY24=, orcid.org/0000-0001-6814-7218
†These authors have contributed equally to this work
Disclaimer: All claims expressed in this article are solely those of the authors and do not necessarily represent those of their affiliated organizations, or those of the publisher, the editors and the reviewers. Any product that may be evaluated in this article or claim that may be made by its manufacturer is not guaranteed or endorsed by the publisher.
Research integrity at Frontiers
Learn more about the work of our research integrity team to safeguard the quality of each article we publish.