- 1College of Forestry, Henan Agricultural University, Zhengzhou, China
- 2Henan Ecological and Environmental Monitoring Center, Zhengzhou, China
- 3College of Resources and Environment, Henan Agricultural University, Zhengzhou, China
Leaf chlorophyll content is an important indicator of the growth and photosynthesis of maize under water stress. The promotion of maize physiological growth by (AMF) has been studied. However, studies of the effects of AMF on the leaf chlorophyll content of maize under water stress as observed through spectral information are rare. In this study, a pot experiment was carried out to spectrally estimate the leaf chlorophyll content of maize subjected to different durations (20, 35, and 55 days); degrees of water stress (75%, 55% and 35% water supply) and two inoculation treatments (inoculation with Funneliformis mosseae and no inoculation). Three machine learning algorithms, including the back propagation (BP) method, least square support vector machine (LSSVM) and random forest (RF) method, were used to estimate the leaf chlorophyll content of maize. The results showed that AMF increased the leaf chlorophyll content, net photosynthetic rate (A), stomatal conductance (gs), transpiration rate (E), and water use efficiency (WUE) of maize but decreased the intercellular carbon dioxide concentration (Ci) of maize and atmospheric vapor pressure deficit (VPD) regardless of the water stress duration and degree. The first-order differential spectral data can better reflect the correlation between leaf chlorophyll content and spectrum of inoculated maize when compared with original spectral data. The BP model performed bestin modeling the maize leaf chlorophyll content, yielding the largest R2-values and smallest root mean square error (RMSE) values, regardless of stress duration. These results provide a reliable basis for the effective monitoring of the leaf chlorophyll content of maize under water stress.
Introduction
Maize is one of the most important food crops in Asia. However, water stress is a main constraint of crop production at the global scale and is expected to increase in coming years (Lesk et al., 2016), and global crop yields are heavily affected by this constraint (Daryanto et al., 2016). Therefore, increasing the utilization efficiency of existing water resources is an effective way to mitigate agricultural water use limitations (Schewe et al., 2014).
Water stress affects the photosynthesis, transpiration, and water use efficiency (WUE) of plants and their absorption and utilization of water and nutrients and hinders the physiological and biochemical processes of plants (Kahil et al., 2015; Ortega-Farias et al., 2021). Plants can cope with water stress through their adaptive strategies (Pavithra and Yapa, 2018), and the symbiosis between arbuscular mycorrhizal fungi (AMF) and plants plays an important role in plants adaptations to water stress (Pavithra and Yapa, 2018). AMF canengage in mutualism with more than 80% of terrestrial plants in the world (Smith and Read, 2008). Plants provide carbohydrates to AMF, and AMF play a crucial role in the growth and nutrient uptake of host plants through beneficial physiological processes. Moreover, AMF improve plant performance under abiotic stresses such as drought (Pavithra and Yapa, 2018), pollution (Liu et al., 2018), or salinity (Arafat and He, 2011). AMF have been shown to improve plant absorption of water, increase photosynthetic pigments, stomatal conductance (Duc et al., 2018; Meddich et al., 2021), intercellular CO2 concentration, transpiration rate, root volume and diameter, and stimulate H+-ATPase activity and gene expression of plants in response to water stress (Cheng et al., 2021). Bromus species inoculated with AMF enhanced superoxide dismutase, peroxidase, catalase, and ascorbate peroxidase activities (Karimkhani et al., 2021) to help plants to tolerate water stress. In addition, inoculation with F. mosseae through the improvement of ionic and biochemical status of the plant can mitigate the detrimental effects of water-deficit stress on maize plants (Bahraminia et al., 2020). AMF increase water use and reduce oxidation damage by stimulating antioxidant activities (Pedranzani et al., 2016) and regulating water absorption and transport by aquaporin genes and endogenous hormones (Pedranzani et al., 2016; Quiroga et al., 2017; Cheng et al., 2021). Plant physiological phenomena are reflected in the healthy status of leaves. Leaf chlorophyll content is an important index for measuring plant photosynthesis and growth. However, the extraction and detection of leaf pigments by traditional chemical monitoring methods is tedious, destructive, discontinuous and time consuming. A spectro radio meter can be used to obtain plant spectral information through reflective rays at different wavelengths (700-1300 nm) with relatively high reflectivity observed in the near infrared region. The spectral reflectance of leaves in different wavebands represents different leaf characteristics of plants. Spectral reflectance in the visible and near infrared regions differs between plants under water stress and healthy plants. Spectro radio metric methods yield measurements more quickly, continuously and economically than traditional laboratory methods (Basayigit and Ozkul, 2015).
The vegetation indexes have been used to build inversion model of chlorophyll content of wheat, as the red edge parameters used to estimate chlorophyll content of plant under the stripe rust, water or salt stress have been investigated in many studies (Gu et al., 2008; Jiang et al., 2010; El-Hendawy et al., 2021). In the past, most models employed to estimate the leaf chlorophyll content have been based on linear regression method; however, this method cannot describe the complex relationship between modeling factors and dependent variables. Therefore, the modeling accuracy was not high. The use of machine learning algorithms is an effective way to express the complex relationship between the modeling factors and dependent variables (Saitta et al., 2011; Sonobe et al., 2021). The back propagation (BP) approach has been used to determine plant diseases (Saleem et al., 2019), and this methodology has potential in the analysis of hyperspectral reflectance data. The random forest (RF) method is a regression technique that combines numerous decision trees to classify or predict the value of a variable, and it has been used for estimating vegetation properties (Li et al., 2017; Chen et al., 2018) as well as for classification and regression (Biau and Scornet, 2015). Least square support vector machine (LSSVM) is a nonlinear system modeling method that has been proposed in recent years. LSSVM requires a small number of training samples and can approach nonlinear systems with high accuracy, and it has been widely used in many fields (Li et al., 2010; Mall and Suykens, 2015). However, studies involving the hyperspectral estimation of chlorophyll content in maize inoculated with AMF under drought stress are rare. In our research, pot experiments were conducted by controlling the amount of water, and the chlorophyll content of maize inoculated with AMF was monitored spectrally. Comparisons and analyses of the physiological characteristics and spectral response of AMF-inoculated and control maize under water stress were performed in this study. An inversion model of the chlorophyll content and multiple spectral variables was established. The objectives of this study were to (1) compare the chlorophyll content and other physiological characteristics of maize with or without inoculation under water stress, (2) determine the spectral response of AMF-inoculated and non-inoculated maize to water stress, and construct the best spectral estimation model of the chlorophyll content of maize under water stress.
Methods
Materials
Maize seeds were obtained from the Henan Academy of Agricultural Sciences; and Funneliformis mosseae was obtained from the Research Institute of Plant Nutrition and Resources in the Beijing Academy of Agriculture and Forestry Sciences and cultured in a microbial laboratory at Henan Agricultural University. River sand with poor nutrients was collected as a substrate and then air dried, passed through a 1 mm sieve and mixed thoroughly. The basic properties of the soil are listed in Table 1. The soil was sterilized at 121°C for 2 h, air dried and prepared for the pot experiment.
Experimental Design
The experiment was conducted in a greenhouse at the Forestry College at Henan Agricultural University on August 15, 2019. The inoculation treatments included inoculation with F. mosseae and no inoculation; and the water stress treatments included (a) no stress, irrigation to 75% of the maximum water holding capacity of the soil (WS75%; achieved with 150 mL water per plant); (b) moderate stress, wherein irrigation was provided to 55% of the maximum water holding capacity of the soil (WS55%; achieved with 110 mL water per plant), and (c) severe stress, wherein irrigation was provided to 35% of the maximum water holding capacity of the soil (WS35%; achieved with 70 mL water per plant). The maize plants were subjected to the water stress treatments for 20, 35, or 55 days after sprouting (on August 20, 2019), in other words, the water stress starts on September 10, September 25, and October 15, 2019, respectively. With 20, 35, and 55 days after sprouting representing the seedling stage, three leaf stage and jointing stage, respectively, of maize growth. Each treatment was performed with 5 replicates. Therefore, a total of 90 pots of maize were established (2 (inoculation treatments) × 3 (water stress degree treatments) × 3 (water stress duration treatments) × 5(replicates)).
Maize was inoculated with F. mosseaeat 50 g/pot (20 spores/g), and the non-inoculated maize was inoculated with the same amount of sterilized microbial inoculum. NH4NO3, KH2PO4, and K2SO4 were added to achieve 100 mg N/kg, 30 mg P/kg, and 150 K mg/kg, respectively, for fertilization. Before water stress, the plants were irrigated with 75% of the maximum water holding capacity of soil during the growth process, and the soil moisture meter (LB9007, QingDao, in China) was used for real-time monitoring. Maize seedling was irrigated in time every day to ensure that the soil moisture was maintained between70% and 75%. Maize seeds were disinfected with 10% H2O2 for 10 min, rinsed with water 2-3 times and then washed with deionized water4-5 times. Seeds were cultured in an incubator at 25-28°C for 24 h. Two seeds were sown in each pot (caliber × height × bottom diameter = 12 × 13 × 9.5 cm) before germination, and the best growing seedling was kept. The light intensity was controlled by 10 high-pressure sodium lamps, and the air temperature and humidity were controlled at 25°C and 20%, respectively, by an air-conditioning unit.
Measurements
Leaf spectral collection and physiological measurement were carried out after each of the three water stress durations, and five pots were randomly selected from each water stress treatment.
Spectrometric Determination
Leaf spectra were acquired with a Field Spec 3 ground spectrometer (ASD Company, United States) under dark conditions. The wave band range of the ground spectrometer was 350-1025 nm, and the spectral resolution was 3 nm. To accurately reflect the whole plant growth status, each pot was placed on a black workbench, and two leaves collected from each of the upper, middle and lower layers of the plant were measured. The halogen lamp used for the indoor test matched the spectrometer and was placed above the sample at an angle of 15° (the angle between the halogen lamp and ground normal) and 20 cm from the target. The field angle of the probe was 25°when observed from10 cm above the target (with the imaged object formed an angle with two edges of the maximum probe range). Each leaf was measured to obtain spectral information, and a whiteboard correction was conducted every 30 min before and during the measurements. Each leaf was lit from four directions during measurement, and five spectra were collected for each lighting direction to avoid errors of leaf curling. Reflectance data were obtained from the average spectral values from all directions.
Physiological and Biochemical Determination
The chlorophyll contents of the leaves were determined using a SPAD-502 instrument (Konica Minolta, Japan). This device determines the relative chlorophyll content using dual wavelength optical absorbance measurements (at wavelengths of 620 and 940 nm) of leaf samples. The chlorophyll content was obtained from 5 selected points on each leaf during measurement, and the average value was taken to reflect the chlorophyll content of the whole leaf.
Photosynthetic parameters such as intercellular CO2 concentration (Ci), net photosynthetic rate (A), stomatal conductance (gs), transpiration rate (E), and water use efficiency (WUE) of maize leaves and water vapor pressure (VPD) were measured by a LI-6400 photosynthesis instrument (LI-COR, United States).
The drying method was adopted to measure the aboveground and belowground biomass of maize: the roots and shoots of maize were heated at 105°C for 30 min, dried at 75°C for 3 days, and weighed to determine the dry weight of maize. Mycorrhizal colonization was determined by the Phillips and Hayman methods (Phillips and Hayman, 1970).
Determination of Soil Properties
The soil property analysis shown in Table 1 was performed using standard soil test procedures. The soil pH values were measured at a 1:2.5 soil/water ratio (w/v) via a pHmeter (Gao et al., 2015). The soil total carbon content (TC) and total nitrogen content (TN) were measured by Dumas combustion using an ElementarVario MAX CN analyzer with the combustion chamber set at 900°C and an oxygen flow rate of 125 mL min–1. The soil available phosphorus content (AP) was measured via the molybdenum antimony colorimetric method, and the soil available nitrogen content (AN) was determined by the alkali hydrolysis diffusion method (Bao, 1999).
Data Analysis
Data Smoothing
During spectral data acquisition, the spectral curve may contain noise because the energy response varies according to the waveband and environment. Therefore, the spectral data must be smoothed. In this study, the Savitzky-Golay (SG) method was used to smooth the spectral curve. This method not only removes the high-frequency components but also retains the characteristic trends of the original curve, and the denoising effect of the SG method is better than that of other methods. The SG algorithm is a least-squares convolution smoothing method that is synthetically applied according to polynomial fitting order and smoothing degree; however, the error of the polynomial fitting curve is calculated by the first derivative. In this study, the SG smoothing method was realized by MATLAB 2014.
First-Order Differential Processing Method
Derivative spectroscopy can reduce the influences of light, atmospheric scattering, absorption and background on the spectral characteristics of targets and there by obviously enhance the correlations between the first-order differential processing of the original spectral reflectance and biochemical indexes. In this study, the smoothed original spectral curves were processed with the first-order differential method.
Modeling Methods
The LSSVM, BP, and RF models were used to estimate the chlorophyll content in the leaves through reflectance spectra, and they were implemented in MATLAB 2014.
The LSSVM method is widely used for complex nonlinear modeling. If the training sample set is (xi,yi), with i = 1, 2, …, n, then x∈Rd and y∈R. The main idea of the support vector machine (SVM) is as follows: first, a nonlinear mapping φ (⋅) is used to map the sample input space Rd to the feature space: φ (x) = (φ (x1), φ (x2),…, φ (xn)); next, the optimal decision function y = wT⋅φ (x)+b is constructed in this high-dimensional feature space, and then the model parameters w and b are determined based on the principle of structural risk minimization. The calculation formula of structural risk is as follows:
Where c is the normalization parameter and Remp is the loss function, which is also called the empirical risk. The common loss functions include the first loss function, the quadratic loss function and the Hubber loss function. Different loss functions represent different SVM models; LSSVM is the SVM with the quadratic loss function. That is, Remp = , where is the prediction error of the model to the training samples.
The training of sample data is performed via the BP method, and the weights and thresholds of the network are constantly modified, so that the error function decreases along the negative gradient direction and approaches the expected output. BP is a widely used neural network model that is primarily applied for function approximation, model recognition and classification, data compression and time series prediction. The BP network is composed of an input layer, a hidden layer and an output layer. The hidden layer can have one or more layers. The network adopts the S-type transfer function: f(x) = 1/(1 + ex). The error function E = ∑i(Ti + Oi)2/2 (where Ti is the expected output and Oi is the calculated output of the network) can be minimized by adjusting the weights and thresholds of the network.
The RF method builds multiple decision trees and fuses them to obtain a more accurate and stable model, which is represented by the combination of the bagging idea and random selection features. The RF method constructs multiple decision trees. When a certain sample needs to be predicted, the prediction results of each tree in the forest for the sample are counted, and the final result is selected from these prediction results by the voting method. Randomness is reflected in two aspects: the selection features and selection of samples. Thus, each tree in the forest has both similarities and differences from other trees.
Statistical Methods
Analysis of variance (ANOVA) was used to compare the effects of AMF among treatments on the mycorrhizal colonization, the growth and photosynthetic physiological progress of maize using the IBM SPSS 23.0 software program (SPSS Inc., Chicago, IL, United States). The least significant difference (LSD) test at the 0.05 probability level (p) was used to compare the means of the measured traits. Correlations between spectral and leaf chlorophyll content and two tailed test (significant level α was 0.05) were determined by the IBM SPSS 23.0 (SPSS Inc, Chicago, IL, United States). The figures were plotted using Origin 8.0 (OriginLab Corporation, Northampton, MA, United States).
Results
Mycorrhizal Colonization
Mycorrhizal colonization represents an intimate association between fungi and host plants. With increasing stress duration and degree, mycorrhizal colonization decreased gradually (Figure 1). Mycorrhizal colonization of inoculated maize was highest under the75% water supply regardless of stress duration and lowest under the35% water supply. Under the stress duration of 20 days, the mycorrhizal colonization of inoculated maize under a 75% and 55% water supply was increased significantly (p < 0.05) by 111.9% and 69% respectively, compared with that of inoculated maize under the 35% water supply. Under the stress duration of 35 days, the mycorrhizal colonization of inoculated maize under a 75% and 55% water supply was significantly increased (p < 0.05), by 100% and 29.2%, respectively, compared with that of maize under the 35% water supply; however, the mycorrhizal colonization of non-inoculated maize was zero, it may be because the maize grew on the sterile soil in an aseptic environment.
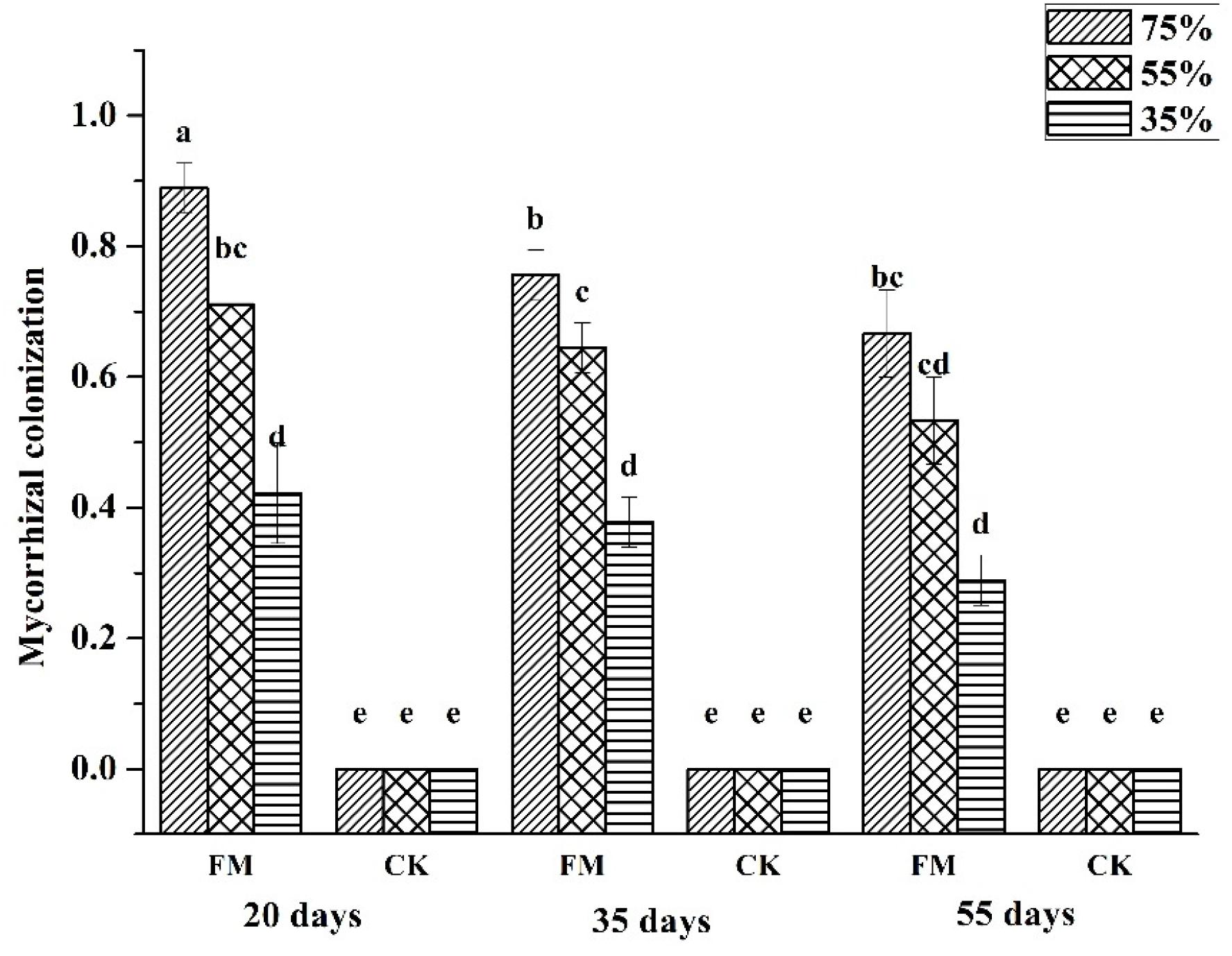
Figure 1. Mycorrhizal colonization of maize under different treatments. FM, inoculated with F. mosseae; CK, without inoculation.
Maize Growth
With increasing water stress, the chlorophyll content of the leaves in both the inoculated and non-inoculated maize decreased gradually; however, when the water stress duration was 20 days, the chlorophyll content of AMF-inoculated maize was4.33, 3.28 and 4.66 more than that of non-inoculated maize under a 75%, 55%, and 35% water supply, respectively. When the water stress duration was 35 or 55 days, the differences in chlorophyll content between AMF-inoculated and non-inoculated maize were not significant when a normal water supply was used; however, when the water stress duration was 35 days, the chlorophyll content of inoculated maize was significantly higher than that of non-inoculated maize when the water supply was 55% or 35%. Under the stress duration of 20 days, the aboveground biomass of AMF-inoculated maize was increased significantly, by 25.7%, compared with that of non-inoculated maize when the water supply was 55%. Moreover, the aboveground biomass of inoculated maize was greater than that of maize without inoculation under the other stress durations, although the differences were not significant (Table 2). The independent effects of stress duration, water stress degree, and inoculation were significant; however, the interaction effect of any two or three factors was not significant (Table 3).
Photosynthetic Physiological Parameters of Maize
As shown in Table 2, water stress inhibited the photosynthesis and transpiration of maize, where as AMF inoculation alleviated the physiological damage of water stress to maize. Regardless of the water stress duration or watering conditions, Ci in the leaves of maize was significantly higher in non-inoculated maize than in AMF-inoculated maize (p < 0.05). As the degree of water stress increased, the Ci of the leaves of both inoculated and non-inoculated maize increased gradually. Under the stress duration of 20 days, the A of the inoculated maize was higher than that of the non-inoculated maize (p > 0.05). However, under a stress duration of 35 days, and a 75% and 55% water supply, the A of the inoculated maize was 1.6 and 1.28 times higher, respectively, than that of non-inoculated maize (p < 0.05). Under a stress duration of 55 days and a 55% water supply, the A of the inoculated maize was increased significantly, by 30%, compared with that of the non-inoculated maize (p < 0.05).
When the stress duration was 20 or 35 days, AMF significantly improved the gs of maize leaves regardless of the degree of water stress. The stomatal conductance of AMF-inoculated maize was significantly higher than that of non-inoculated maize regardless of water stress duration or degree (p < 0.05). With increasing stress duration, the transpiration effect of AMF-inoculated maize became greater than that of non-inoculated maize. When the stress duration was 55 days, under a 75% and 55% water supply, the E of AMF-inoculated maize was significantly higher than that of maize without inoculation, by 0.22 and 0.09 times, respectively (p < 0.05). Under the stress durations of 20 days, the atmospheric VPD was significantly lower (p < 0.05)in the inoculated maize than in the non-inoculated maize. Under the stress durations of 20 days, the WUE of leaves did not significantly differ between the inoculated and non-inoculated maize with a normal water supply, however, under a 75% and 55% water supply, the leaf WUE of the inoculated maize was significantly higher than that of the non-inoculated maize (p < 0.05).
Each type of treatment (stress duration, water stress degree, and inoculation) had a significant effect (p < 0.05)on the photosynthetic parameters of maize leaves. However, the interaction effect of any two or three treatment parameters was not significant for any parameter except for Ci, VPD, and E (Table 3).
Spectral Characteristics of Maize Leaves
At the three water stress durations, the general trend of the spectral curve of maize with different treatments was similar. When the stress duration was 20 or 35 days, at the green peak (550 nm), and red edge (700 nm), the leaf reflectance of non-inoculated maize was significantly higher (except under the 35% water supply) than that of inoculated maize (Figure 2). In non-inoculated maize under a 75% water supply, leaf reflectance was highest at wavelengths of 550 nm and 700 nm at a stress duration of 20 days whereas in non-inoculated maize with a 35% water supply, leaf reflectance was highest at wavelengths of 550 nm and 700 nm at a stress duration of 35 days. When the stress duration was 55 days, the leaf reflectance of inoculated maize with a 35% water supply was highest at a wavelength of 550 nm; and that of non-inoculated maize with a 75% water supply was highest at a wavelength of 700 nm. The highest reflectance of maize leaves under a stress duration of 20 days was higher than that of leaves under 35 and 55 days of stress at the wavelength of 550 and 700 nm (Figure 2).
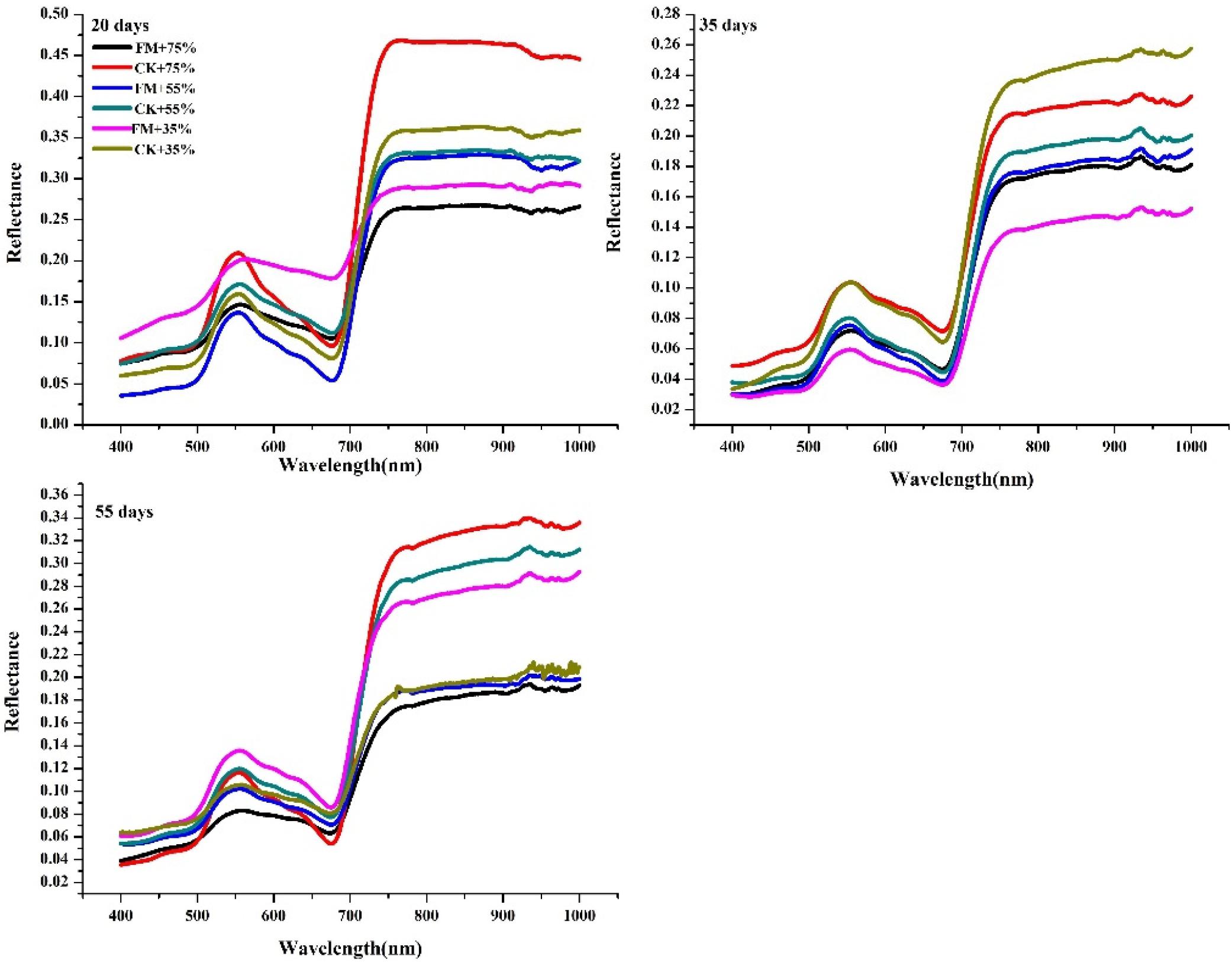
Figure 2. Spectra of maize leaves at each stress level and different inoculation durations. FM, inoculated with F. mosseae; CK, without inoculation.
Correlation Analysis of Chlorophyll Content and First-Order Spectral Differential Variables in Maize
For the first-order derivative of spectral reflection, the spectral reflectance of inoculated maize leaves had larger correlation coefficients with the leaf chlorophyll content than that without inoculation under three water stress durations (Figure 3). When the stress duration was 20 or 55 days, the correlation coefficients between the first-order differential reflectance and the chlorophyll content of inoculated maize showed unstable performance at the range of 800-1000 nm especially, but during 400-800 nm, the maximum correlation coefficients values are in the inoculated maize at 550 nm and 700 nm, respectively (α < 0.05). When the stress duration was 35 days, the correlation coefficients between the first-order differential reflectance and the chlorophyll content of inoculated maize showed larger than that without inoculation at two peak areas (the wavelengths of 550-700 nm and 850-950 nm; Figure 3) (α < 0.05).
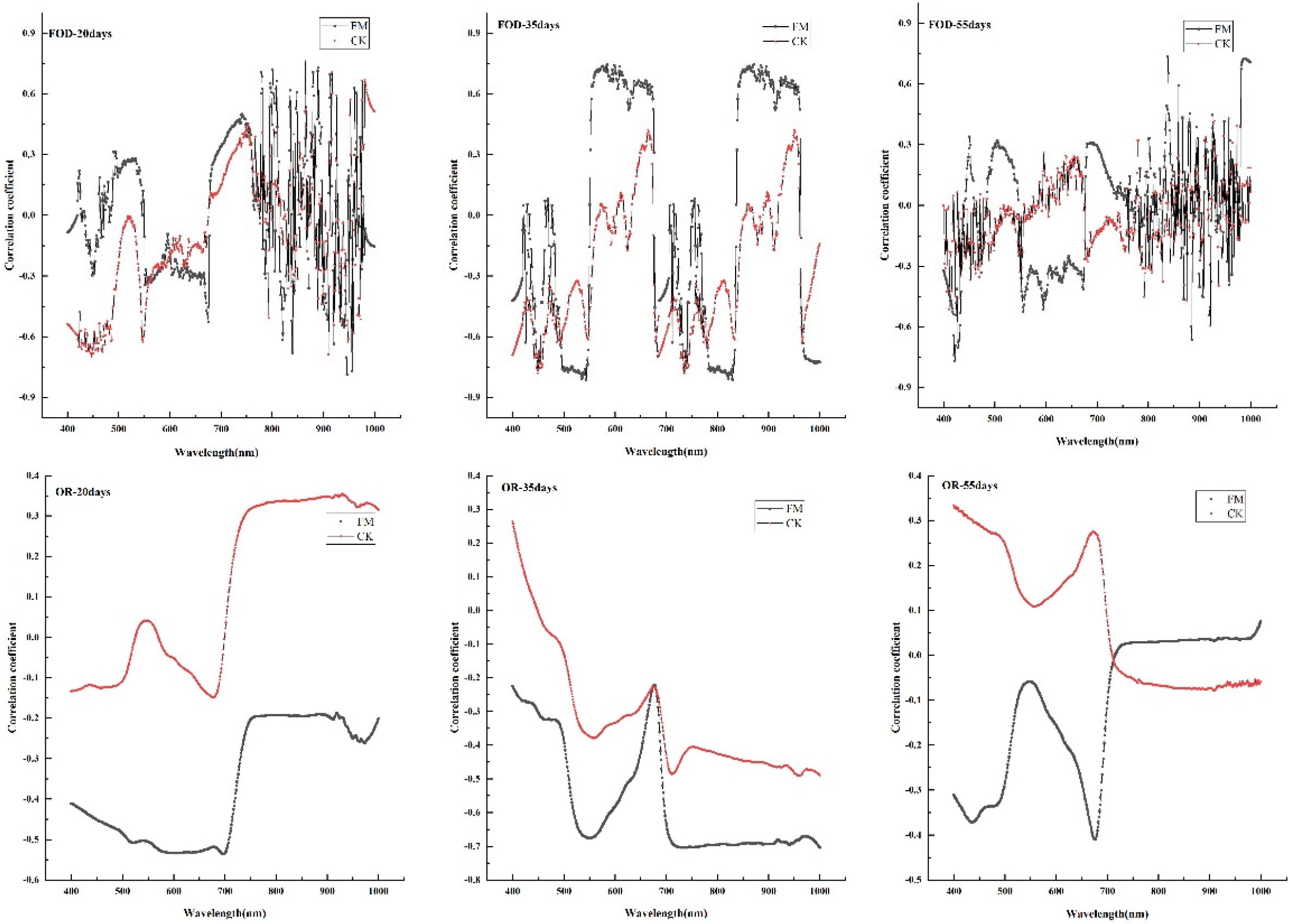
Figure 3. Correlation between spectral reflectance and leaf color value under different treatments. FM, inoculated with F. mosseae; CK, without inoculation. OR-20 days:water stress duration was 20 days with the original spectral data; OR-35 days: water stress duration was 35 days with the original spectral data; OR-55 days: water stress duration was 55 days with the original spectral data; FOD-20 days:water stress duration was 20 days with the first-order derivative of spectral data; FOD-35 days: water stress duration was 35 days with the first-order derivative of spectral data; FOD-55 days: water stress duration was 55 days with the first-order derivative of spectral data.
For the original spectral data, there was a negative correlation between leaf chlorophyll content and spectral reflectance of inoculated maize under three water stress durations, but a positive correlation between that of non-inoculated maize before 500 nm under the stress duration of 35 days and before 700 nm under the stress duration of 55 days. There are two peaks of correlation coefficient between leaf color value and spectral reflectance of inoculated maize at 550 and 700 nm were larger than that of non-inoculated maize under the stress duration of 35 or 55 days (Figure 3) (α < 0.05).
Compared with the original spectral data, the first-order differential processing can better reflect the correlation between leaf color value and spectrum of inoculated maize.
Hyperspectral Estimation Model of the Chlorophyll Content of Maize and Its Validation
To better reflect the chlorophyll content of inoculated maize under drought stress, the results of correlation analysis of the first-order differential spectral data were used to establish, an estimation model of the first-order differential values from sensitive bands corresponding to chlorophyll content. The LSSVM, RF, and BP nonlinear models based on machine learning were used. A regression analysis was carried out between the predicted and measured values, and the estimation accuracy of the models was evaluated by the coefficient of determination (R2) values, root mean square error (RMSE)values and p-Value of the LSSVM, RF and BP models established with different spectral variables that were significant at the 0.001 level (Figures 4A–C). The results indicated that all of these models could be used to estimate the chlorophyll content of inoculated maize under drought stress. The BP model was better than the others achieving the largest R2-values (R2 = 0.9796 for the 20 days duration, R2 = 0.9951 for the 35 days duration, and R2 = 0.9479 for the 55 days duration) and smallest RMSEs (RMSE = 0.3875 for the20-day duration, RMSE = 0.2473 for the35-day duration, and RMSE = 0.6431 for the 55-day duration) regardless of the stress duration.
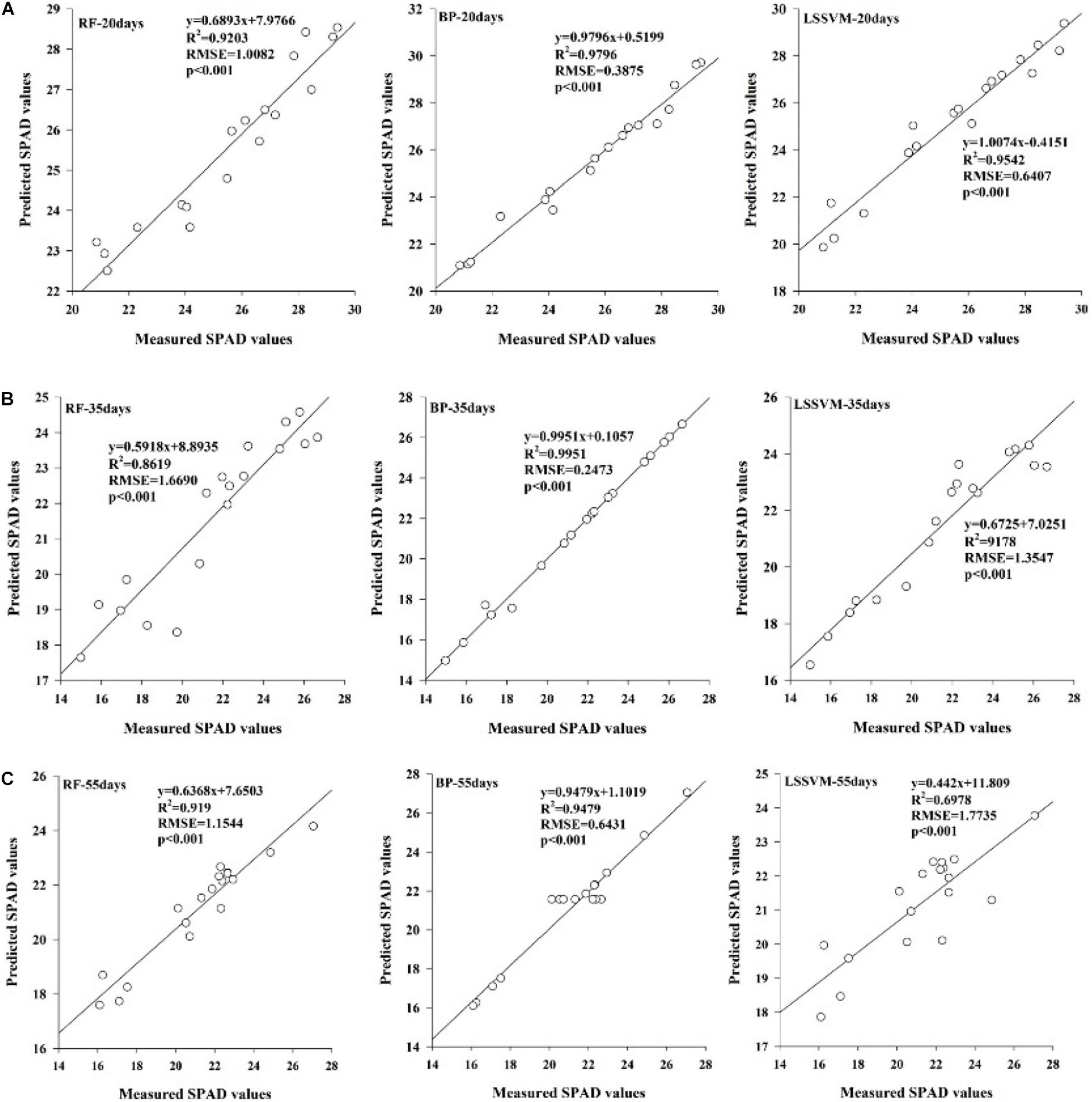
Figure 4. Relationships of the measured SPAD values and predicted values based on the three machine learning algorithms under water stress durations of 20 days (A), 35 days (B), and 55 days (C).
Discussion
Water stress is a worldwide problem that affects the growth, yield and physiological processes of crops (Begum et al., 2019). AMF symbiosis has been reported to enhance plant tolerance to water stress (Duc et al., 2018; Zhang et al., 2018). Inoculation with F. mosseaehas been shown to enhance plant dry weights compared to those of non-mycorrhizal plants under control and water-deficit stress conditions (Bahraminia et al., 2020). In this study, AMF colonization was negatively affected by water stress, possibly because of declines in spore germination and growth, reductions in the number of AMF structures, the inhibition of hyphal growth and expansion in soil (Salloum et al., 2018), or reductions in the supply of carbohydrate by host plants (Tyagi et al., 2017). However, AMF inoculation improved the growth of maize regardless of the stress degree and duration, possibly because the AMF facilitated maize absorption of available phosphorus and nitrogen under water stress (Ghorchiani et al., 2018). Moreover, with increasing degree of water stress, the stress-mitigation function of the AMF became more obvious (Pavithra and Yapa, 2018). The AMF may have reduced the damage of water stress to plant growth by changing plant physiological processes (Pavithra and Yapa, 2018) and radial root water transport (Quiroga et al., 2019).
Photosynthesis is an important indicator of physiological sensitivity to water stress (Chaves et al., 2009). In our study, water stress inhibited photosynthesis in maize. Similar results have been reported by others (Hazrati et al., 2016; Ahanger et al., 2017). Many studies have shown that water stress reduces A, gs and E (Yan et al., 2016; Hura et al., 2007). The reduced photosynthesis under water stress is due to reductions in the coupling factor and production of ATP (Tezara et al., 1999). AMF improved the photosynthesis of maize under the different treatments in this study as well as previous studies (Sánchez-Blanco et al., 2004; Khalvati et al., 2005). In this study, in maize under water stress, Ci and VPD decreased and the A, gs, E and WUE increased in AMF-inoculated maize relative to non-inoculated maize. Increased stomatal functioning enhances CO2 entry into the leaf tissues and its successful assimilation was observed in AMF-inoculated plants, such results have also been reported in AMF-inoculated wheat (Zhou et al., 2015). The high photosynthetic rates of A MF-inoculated plants under water stress could be explained by the increased production of photosynthetic pigments and a higher carboxylation efficiency relative to that of non-inoculated plants (Sánchez-Blanco et al., 2004; Zhu et al., 2014).
Though the leaf chlorophyll content of maize was measured by SPAD instrument in a real time, fast and non-destructive way in this study, it was a relative value with the limitation that not taking into consideration of the biomass (Lei et al., 2019). In our study, AMF inoculation increased the leaf chlorophyll content of maize under water stress, consistent with there port of other researchers (Begum et al., 2019). It may be due to the key role of Mg in chlorophyll synthesis, and Mg uptake increased in mycorrhizal plants (Mathur et al., 2018). The spectral reflectance of leaves is closely related to the leaf surface characteristics, leaf thickness, water content, and the contents of chlorophyll and other pigments (Feng et al., 2004). The wavelength region (500 to 900 nm) contains wavelengths with pigment absorption features (Merzlyak et al., 2003) as well as the red-edge (700 to 750 nm) (Mutanga and Skidmore, 2007). In our study, at the green peak (550 nm) and red edge (700 nm), The spectral reflectance of maize without inoculation under water stress was much higher than that of AMF-inoculated maize, it may be due to the more water utilization rate and chlorophyll content of inoculated maize compared to that without inoculation (Begum et al., 2019). The spectra lreflectance of maize leaves decreased with water stress duration, potentially due to greater damage of water stress to the leaves of maize at the seedling stage than at the later growth stages. The results of this study indicated that hyperspectral technology can be used to monitor changes in chlorophyll content in maize inoculated with mycorrhizae and exposed to water stress.
In the present study, compared with the original spectra, the first-order differential reflectance spectra better reflected the chlorophyll content of leaves at 400-800 nm. In other study, the correlation curve between the original spectrum and the chlorophyll relative content value was the best between the wavelengths 509-650 nm. The correlation between the first derivative spectrum and chlorophyll relative content value was the best and most stable at 450∼500 nm (Zhu et al., 2020). It can be explained by that the original spectrum can eliminate the influence of background noise on the spectrum, and reduce the scattering and absorption of light by atmosphere in the process of spectrum acquisition, after first-order differential processing (Mahlein et al., 2012; Manzo et al., 2013). The correlation coefficient between leaf color value and first-order differential spectral reflectance of inoculated maize at 550 and 700 nm were larger than that of non-inoculated maize under the stress duration of 35 or 55 days. The results varied in different conditions and environments. Hunt et al. (2013) found that the correlation between chlorophyll content and spectral reflectance at the canopy scale was strong in the visible region (400-760 nm). Some study showed that the red edge slope is the maximum reflectance in the red band, which can better reflect the chlorophyll content of plants and is often closely related to the photosynthetic rate of plants (Verrelst et al., 2010).
Our results demonstrated that all the models, constructed using the LSSVM, RF, and BP methods, could be used to estimate the chlorophyll content of inoculated maize under drought stress. The BP model showed the highest R2 and slope and the lowest RMSE regardless of the water stress duration. BP model was more stable and accurate than others at estimation of leaf chlorophyll content (Shao et al., 2018). The best estimating model for the relationship between the leaf relative chlorophyll content and the reflectance spectra was the partial least squares (PLS) in the field experiment about winter wheat (Zhang et al., 2016). It may due to BP model was suitable for estimating the chlorophyll content in greenhouse experiment.
Conclusion
Arbuscular mycorrhizal fungi treatment increased the leaf chlorophyll content, A, gs, E, and WUE of maize but decreased the Ciofmaize and VPD regardless of the water stress duration or degree. The first-order differential reflectance of inoculated maize leaves was more significantly correlated with the chlorophyll content of maize than was the original spectral reflectance. Of the three machine learning models, the BP model achieved the largest coefficient of determination and smallest RMSE regardless of the stress duration. Thus, the BP model represented the optimal method of estimating the leaf chlorophyll content of inoculated maize.
Data Availability Statement
The original contributions presented in the study are included in the article/supplementary material, further inquiries can be directed to the corresponding author/s.
Author Contributions
LY supervised the work, contributed to data interpretation, and writing and revision of the manuscript. JS designed and performed the investigation, analyzed the data, contributed to data interpretation, and drafted the manuscript. XY provided the test site and revised the manuscript. JW and LL provided experimental instruments and modification of manuscript. EG and YK contributed to modification of manuscript. All authors contributed to the article and approved the submitted version.
Funding
This work was supported by the School-level Innovation Fund (Nos. KJCX2020A06 and KJCX2020A05) and the Key R&D and Promotion Special Projects in Henan Province (Nos. 192102310184 and 202102310352).
Conflict of Interest
The authors declare that the research was conducted in the absence of any commercial or financial relationships that could be construed as a potential conflict of interest.
Acknowledgments
We thank the College of Resources and Environment of Henan Agricultural University for providing equipment.
References
Ahanger, M. A., Tittal, M., Mir, R. A., and Agarwal, R. (2017). Alleviation of water and osmotic stress-induced changes in nitrogen metabolizing enzymes in Triticum aestivum L. cultivars by potassium. Protoplasma 254, 1953–1963. doi: 10.1007/s00709-017-1086-z
Arafat, A. H. A. L., and He, C. X. (2011). Effect of arbuscular mycorrhizal fungi on growth, mineral nutrition, antioxidant enzymes activity and fruit yield of tomato grown under salinity stress. Sci. Hortic. 127, 228–233. doi: 10.1016/j.scienta.2010.09.020
Bahraminia, M., Zarei, M., Ronaghi, A., Sepehri, M., and Etesami, H. (2020). Ionomic and biochemical responses of maize plant (Zea mays L.) inoculated with Funneliformis mosseae to water-deficit stress. Rhizosphere 16, 1–8. doi: 10.1016/j.rhisph.2020.100269
Bao, S. D. (1999). “Methods of soil phosphorus and potassium,” in Analysis of Soil Agriculture Chemistry, 3rd Edn, ed. S. D. Bao (Beijing: China Agriculture Press). (in Chinese).
Basayigit, L., and Ozkul, M. (2015). Estimating Zn content of pepper under different fertilizer applications using a handheld spectrometer. Int. J. Agric. Biol. 17, 1–8.
Begum, H., Ahanger, M. A., Su, Y., Lei, Y., Mustafa, N. S. A., Ahmad, P., et al. (2019). Improved drought tolerance by AMF inoculation in maize (Zea mays) involves physiological and biochemical implications. Plants 8:579. doi: 10.3390/plants8120579
Biau, G., and Scornet, E. (2015). A random forest guided tour. Test 25, 197–227. doi: 10.1007/s11749-016-0481-7
Chaves, M. M., Flexas, J., and Pinheiro, C. (2009). Photosynthesis under drought and salt stress: regulation mechanisms from whole plant to cell. Ann. Bot. 103, 551–560. doi: 10.1093/aob/mcn125
Chen, D. M., Shi, Y. Y., Huang, W. J., Zhang, J. C., and Wu, K. H. (2018). Mapping wheat rust based on high spatial resolution satellite imagery. Comput. Electron Agric. 152, 109–116. doi: 10.1016/j.compag.2018.07.002
Cheng, H. Q., Zou, Y. N., Wu, Q. S., and Kuca, K. (2021). Arbuscular mycorrhizal fungi alleviate drought stress in Trifoliate Orange by regulating H+-ATPase activity and gene expression. Front. Plant Sci. 12:659694. doi: 10.3389/fpls.2021.659694
Daryanto, S., Wang, L., and Jacinthe, P. A. (2016). Global synthesis of drought effects on maize and wheat production. PLoS One 11:e0156362. doi: 10.1371/journal.pone.0156362
Duc, N. H., Csintalan, Z., and Posta, K. (2018). Arbuscular mycorrhizal fungi mitigate negative effects of combined drought and heat stress on tomato plants. Plant Phys. Biochem. 132, 297–307. doi: 10.1016/j.plaphy.2018.09.011
El-Hendawy, S., Elsayed, S., Al-Suhaibani, N., Alotaibi, M., Tahir, M. U., Mubushar, M., et al. (2021). Use of Hyperspectral reflectance sensing for assessing growth and chlorophyll content of spring wheat grown under simulated saline field conditions. Plants Basel 10, 1–26. doi: 10.3390/plants10010101
Feng, X. W., Chen, W. X., and Bao, A. M. (2004). Analysis on the cotton physiological change and its hyperspectral response under the water stress conditions. Arid Land Geogr. 2, 121–126. (in Chinese), doi: 10.4324/9780203221099-25
Gao, Y. C., Wang, J. N., Guo, S. H., Hu, Y. L., Li, T. T., Mao, R., et al. (2015). Effects of salinization and crude oil contamination on soil bacterial community structure in the Yellow River Delta region, China. Appl. Soil Ecol. 86, 165–173. doi: 10.1016/j.apsoil.2014.10.011
Ghorchiani, M., Etesami, H., and Alikhani, H. (2018). Improvement of growth and yield of maize under water stress by co-inoculating an arbuscular mycorrhizal fungus and a plant growth promoting rhizobacterium together with phosphate fertilizers. Agric. Ecosyst. Environ. 258, 59–70. doi: 10.1016/j.agee.2018.02.016
Gu, Y. F., Ding, S. Y., Chen, H. S., Gao, Z. Y., and Xing, Q. (2008). Ecophysiological responses and hyperspectral characteristics of winter wheat (Triticum aestivum) under drought stress. Acta Ecol. Sin. 28, 2690–2697. (in Chinese),Google Scholar
Hazrati, S., Tahmasebi-Sarvestani, Z., Modarres-Sanavy, S. A. M., Mokhtassi-Bidgoli, A., and Nicola, S. (2016). Effects of water stress and light intensity on chlorophyll fluorescence parameters and pigments of Aloe vera L. Plant Physiol. Biochem. 106, 141–148. doi: 10.1016/j.plaphy.2016.04.046
Hunt, E. R., Doraiswamy, P. C., McMurtrey, J. E., Daughtry, C. S. T., Perry, E. M., and Akhmedov, B. (2013). A visible band index for remote sensing leaf chlorophyll content at the canopy scale. Geoinformation 21, 103–112. doi: 10.1016/j.jag.2012.07.020
Hura, T., Hura, K., Grzesiak, M., and Rzepka, A. (2007). Effect of long-term drought stress on leaf gas exchange and fluorescence parameters in C3 and C4 plants. Acta Physiol. Plant 29, 103–113.
Jiang, J. B., Chen, Y. H., and Huang, W. J. (2010). Using hyperspectral remote sensing to estimate canopy chlorophyll density of wheat under yellow rust stress. Spectrosc. Spectral Anal. 30, 2243–2247. (in Chinese)
Kahil, M. T., Dinar, A., and Albiac, J. (2015). Modelling water scarcity and droughts for policy adaptation to climate change in arid and semiarid regions. J. Hydrol. 522, 95–109. doi: 10.1016/j.jhydrol.2014.12.042
Karimkhani, S., Ardakani, M. R., Paknejad, F., and Manavi, P. N. (2021). Alleviation drought stress of Bromus species using mycorrhizal fungi contributed with drought-responsive biomarker. Iran. J. Sci. Technol. Trans. Sci. 45, 481–491. doi: 10.1007/s40995-021-01073-9
Khalvati, M. A., Hu, Y., Mozafar, A., and Schmidhalter, U. (2005). Quantification of water uptake by arbuscular mycorrhizal hyphae and its significance for leaf growth, water relations and gas exchange of barley subjected to drought stress. Plant Biol. 7, 706–712. doi: 10.1055/s-2005-872893
Lei, X. X., Zhao, J., Liu, H. C., Zhang, J. Y., Liang, W. Y., Tian, J. L., et al. (2019). Inversion of chlorophyll content and SPAD value of vegetable leaves based on PROSPECT model. Spectrosc. Spectral Anal. 39, 3256–3260.
Lesk, C., Rowhani, P., and Ramankutty, N. (2016). Influence of extreme weather disasters on global crop production. Nature 529, 84–87. doi: 10.1038/nature16467
Li, Y. J., Tang, X. J., and Liu, J. H. (2010). Application of least square support vector machine based on particle swarm optimization in quantitative analysis of gas mixture. Spectrosc. Spectral Anal. 30, 774–778. (in Chinese),Google Scholar
Li, Z. W., Xin, X. P., Tang, H., Yang, F., Chen, B. R., and Zhang, B. H. (2017). Estimating grassland LAI using the random forests approach and landsat imagery in the meadow steppe of Hulunber, China. J. Integr. Agric. 16, 286–297. doi: 10.1016/S2095-3119(15)61303-X
Liu, L., Li, J. W., Yue, F. X., Yan, X. W., Wang, F. Y., Bloszies, S. A., et al. (2018). Effects of arbuscular mycorrhizal inoculation and biochar amendment on maize growth, cadmium uptake and soil cadmium speciation in Cd-contaminated soil. Chemosphere 194, 495–503. doi: 10.1016/j.chemosphere.2017.12.025
Mahlein, A. K., Steiner, U., Christian, H., Dehne, H. W., and Oerke, E. C. (2012). Hyperspectral imaging for small-scale analysis of symptoms caused by di_erent sugar beet diseases. Plant Methods 8:3. doi: 10.1186/1746-4811-8-3
Mall, R., and Suykens, J. A. K. (2015). Very sparse lssvm reductions for large-scale data. IEEE Trans. Neural Netw. Learn. Syst. 26, 1086–1097. doi: 10.1109/TNNLS.2014.2333879
Manzo, C., Salvini, R., Guastaldi, E., Nicolardi, V., and Protano, G. (2013). Reflectance spectral analyses for the assessment of environmental pollution in the geothermal site of Mt, Amiata (Italy). Atmospher. Environ. 79, 650–665. doi: 10.1016/j.atmosenv.2013.06.038
Mathur, S., Sharma, M. P., and Jajoo, A. (2018). Improved photosynthetic efficacy of maize (Zea mays) plants with arbuscular mycorrhizal fungi (AMF) under high temperature stress. J. Photochem. Photobiol. B 180, 149–154. doi: 10.1016/j.jphotobiol.2018.02.002
Meddich, A., Rahou, Y. A., Boutasknit, A., Ait-El-Mokhtar, M., Fakhech, A., Lahbouki, S., et al. (2021). Role of mycorrhizal fungi in improving the tolerance of melon (Cucumus melo) under two water deficit partial root drying and regulated deficit irrigation. Plant Biosyst. 155, 1–12. doi: 10.1080/11263504.2021.1881644
Merzlyak, M. N., Gitelson, A. A., Chivkunova, O. B., Solovchenko, A. E., and Pogosyan, S. I. (2003). Application of reflectance spectroscopy for analysis of higher plant pigments. Russ. J. Plant Physiol. 50, 704–710. doi: 10.1023/A:1025608728405
Mutanga, O., and Skidmore, A. K. (2007). Red edge shift and biochemical content in grass canopies. ISPRS J. Photogramm. Rem. Sens. 62, 34–42. doi: 10.1016/j.isprsjprs.2007.02.001
Ortega-Farias, S., Espinoza-Meza, S., Lopez-Olivari, R., Araya-Alman, M., and Carrasco-Benavides, M. (2021). Effects of different irrigation levels on plant water status, yield, fruit quality, and water productivity in a drip-irrigated blueberry orchard under Mediterranean conditions. Agric. Water Manag. 249, 1–10. doi: 10.1016/j.agwat.2021.106805
Pavithra, D., and Yapa, N. (2018). Arbuscular mycorrhizal fungi inoculation enhances drought stress tolerance of plants. Groundw. Sustain. Dev. 7, 490–494. doi: 10.1016/j.gsd.2018.03.005
Pedranzani, H., Rodríguez-Rivera, M., Gutiérrez, M., Porcel, R., Hause, B., and Ruiz-Lozano, J. M. (2016). Arbuscular mycorrhizal symbiosis regulates physiology and performance of digitaria eriantha plants subjected to abiotic stresses by modulating antioxidant and jasmonate levels. Mycorrhiza 26, 141–152. doi: 10.1007/s00572-015-0653-4
Phillips, J. M., and Hayman, D. S. (1970). Improved procedures for clearing roots and staining parasitic and vesicular-arbuscular mycorrhizal fungi for rapid assessment of infection. Trans. Br. Mycol. Soc. 55, 158–161. doi: 10.1016/S0007-1536(70)80110-3
Quiroga, G., Erice, G., Aroca, R., Chaumont, F., and Ruiz-Lozano, J. M. (2019). Contribution of the arbuscular mycorrhizal symbiosis to the regulation of radial root water transport in maize plants under water deficit. Environ. Exp. Bot. 167, 1–10. doi: 10.1016/j.envexpbot.2019.103821
Quiroga, G., Erice, G., Aroca, R., Chaumont, F., and Ruiz-Lozano, J. M. (2017). Enhanced drought stress tolerance by the arbuscular mycorrhizal symbiosis in a drought-sensitive maize cultivar is related to a broader and differential regulation of host plant aquaporins than in a drought-tolerant cultivar. Front. Plant Sci. 8: 1056. doi: 10.3389/fpls.2017.01056
Saitta, L., Giordana, A., and Cornuejols, A. (2011). Phase Transitions in Machine Learning. Cambridge: Cambridge University Press.
Saleem, M. H., Potgieter, J., and Arif, K. M. (2019). Plant disease detection and classification by deep learning. Plants 8:468. doi: 10.3390/plants8110468
Salloum, M. S., Menduni, M. F., and Luna, C. M. (2018). A differential capacity of arbuscular mycorrhizal fungal colonization under well-watered conditions and its relationship with drought stress mitigation in unimproved vs. Improved soybean genotypes. Botany 96, 135–144. doi: 10.1139/cjb-2017-0137
Sánchez-Blanco, M. J., Ferrández, T., Morales, M. A., and Alarcón, J. J. (2004). Variations in water status, gas exchange, and growth in Rosmarinus officinalis plants infected with Glomus deserticola under drought conditions. J. Plant Phisiol. 161, 675–682. doi: 10.1078/0176-1617-01191
Schewe, J., Heinke, J., and Gerten, D. (2014). Multi-model assessment of water scarcity under climate change. Proc. Natl. Acad. Sci. U.S.A. 111, 3245–3250. doi: 10.1073/pnas.1222460110
Shao, D. G., Nong, X. Z., Tan, X. Z., Chen, S., Xu, B. L., and Hu, N. J. (2018). Daily water quality forecast of the South-To-North water diversion project of China based on the cuckoo search-back propagation neural network. Water 10:1471. doi: 10.3390/w10101471
Sonobe, R., Yamashita, H., Mihara, H., Morita, A., and Ikka, T. (2021). Hyperspectral reflectance sensing for quantifying leaf chlorophyll content in wasabi leaves using spectral pre-processing techniques and machine learning algorithms. Int. J. Rem. Sens. 42, 1311–1329. doi: 10.1080/01431161.2020.1826065
Tezara, W., Mitchell, V. J., Driscoll, S. D., and Lawlor, D. W. (1999). Water stress inhibits plant photosynthesis by decreasing coupling factor and ATP. Nature 401, 914–917. doi: 10.1038/44842
Tyagi, J., Varma, A., and Pudake, R. N. (2017). Evaluation of comparative effects of arbuscular mycorrhiza (Rhizophagus intraradices) and endophyte (Piriformospora indica) association with finger millet (Eleusine coracana) under drought stress. Eur. J. Soil Biol. 81, 1–10. doi: 10.1016/j.ejsobi.2017.05.007
Verrelst, J., Schaepman, M. E., Malenovs’y, Z., and Clevers, J. G. P. W. (2010). _Effects of woody elements on simulated canopy reflectance: implications for forest chlorophyll content retrieval. Rem. Sens. Environ. 2010, 647–656. doi: 10.1016/j.rse.2009.11.004
Yan, W. M., Zhong, Y. Q., and Shangguan, Z. P. A. (2016). Meta-analysis of leaf gas exchange and water status responses to drought. Sci. Rep. 6:20917. doi: 10.1038/srep20917
Zhang, J. F., Han, W. T., Huang, L. W., Zhang, Z. Y., Ma, Y. M., and Hu, Y. M. (2016). Leaf chlorophyll content estimation of winter wheat based on visible and near-infrared sensors. Sensors 16, 1–11.
Zhang, T., Hu, Y. J., Zhang, K., Tian, C. Y., and Guo, J. X. (2018). Arbuscular mycorrhizal fungi improve plant growth of Ricinus communis by altering photosynthetic properties and increasing pigments under drought and salt stress. Ind. Crops Prod. 117, 13–19. doi: 10.1016/j.indcrop.2018.02.087
Zhou, Q., Ravnskov, S., Jiang, D., and Wollenweber, B. (2015). Changes in carbon and nitrogen allocation, growth and grain yield induced by arbuscular mycorrhizal fungi in wheat (Triticum aestivum L.) subjected to a period of water deficit. Plant Growth Regul. 75, 751–760. doi: 10.1007/s10725-014-9977-x
Zhu, J. Y., He, W. J., Yao, J. M., Yu, Q., Xu, C. Y., Huang, H. G., et al. (2020). Spectral reflectance characteristics and chlorophyll content estimation model of Quercus aquifolioides leaves at different altitudes in Sejila Mountain. Appl. Sci. 10:3636. doi: 10.3390/app10103636
Keywords: arbuscular mycorrhizal fungi, leaf chlorophyll content, spectral reflectance, machine learning algorithms, water stress
Citation: Sun J, Yang L, Yang X, Wei J, Li L, Guo E and Kong Y (2021) Using Spectral Reflectance to Estimate the Leaf Chlorophyll Content of Maize Inoculated With Arbuscular Mycorrhizal Fungi Under Water Stress. Front. Plant Sci. 12:646173. doi: 10.3389/fpls.2021.646173
Received: 14 January 2021; Accepted: 10 May 2021;
Published: 28 May 2021.
Edited by:
Penghao Wang, Murdoch University, AustraliaReviewed by:
Ana María Mendez-Espinoza, Instituto de Investigaciones Agropecuarias (Chile), ChileXiancan Zhu, Anhui Normal University, China
Copyright © 2021 Sun, Yang, Yang, Wei, Li, Guo and Kong. This is an open-access article distributed under the terms of the Creative Commons Attribution License (CC BY). The use, distribution or reproduction in other forums is permitted, provided the original author(s) and the copyright owner(s) are credited and that the original publication in this journal is cited, in accordance with accepted academic practice. No use, distribution or reproduction is permitted which does not comply with these terms.
*Correspondence: Liu Yang, yangliutj@163.com