- 1Bean Program, Agrobiodiversity Area, International Center for Tropical Agriculture (CIAT), Cali, Colombia
- 2Bean Program, Agrobiodiversity Area, International Center for Tropical Agriculture (CIAT), Kampala, Uganda
Root rot in common bean is a disease that causes serious damage to grain production, particularly in the upland areas of Eastern and Central Africa where significant losses occur in susceptible bean varieties. Pythium spp. and Fusarium spp. are among the soil pathogens causing the disease. In this study, a panel of 228 lines, named RR for root rot disease, was developed and evaluated in the greenhouse for Pythium myriotylum and in a root rot naturally infected field trial for plant vigor, number of plants germinated, and seed weight. The results showed positive and significant correlations between greenhouse and field evaluations, as well as high heritability (0.71–0.94) of evaluated traits. In GWAS analysis no consistent significant marker trait associations for root rot disease traits were observed, indicating the absence of major resistance genes. However, genomic prediction accuracy was found to be high for Pythium, plant vigor and related traits. In addition, good predictions of field phenotypes were obtained using the greenhouse derived data as a training population and vice versa. Genomic predictions were evaluated across and within further published data sets on root rots in other panels. Pythium and Fusarium evaluations carried out in Uganda on the Andean Diversity Panel showed good predictive ability for the root rot response in the RR panel. Genomic prediction is shown to be a promising method to estimate tolerance to Pythium, Fusarium and root rot related traits, indicating a quantitative resistance mechanism. Quantitative analyses could be applied to other disease-related traits to capture more genetic diversity with genetic models.
Introduction
Common bean (Phaseolus vulgaris L.) is one of the most important grain legumes for direct human consumption (Broughton et al., 2003). It provides protein, complex carbohydrates, and valuable micronutrients for more than 300 million people in the tropics and 100 million people in Africa alone (Pathania et al., 2014). Common bean production is increasingly affected by root rot diseases, caused by a complex of soil-borne pathogens such as Rhizoctonia solani, Fusarium solani f. sp. phaseoli, Sclerotium rolfsii, and Pythium spp. (Paparu et al., 2018). Bean yield losses have been attributed to root rot in Eastern and Central Africa where beans are grown extensively (Otsyula et al., 2002; Nzungize et al., 2012). Yield losses of up to 100% have been reported in Uganda, and up to 70% in Rwanda and Kenya (Otsyula et al., 2003); during 1991 and 1993 many farmers stopped growing bean crops due to a serious outbreak of root rot (Nzungize et al., 2012). Disease severity is influenced by several factors, such as poor soil drainage, low fertility, high temperatures and humidity conditions as well as inadequate crop rotation. These environmental factors affect the composition of the root rot disease complex, e.g., in warmer, dryer environments Sclerotium may be the major pathogen.
The genera Pythium, is considered as an important plant pathogen which attacks multiple crops and is ubiquitous on different continents (Van West et al., 2003). Numerous species of Pythium have been recognized as major pathogens, and the most common are P. aphanidermatum, P. ultimum, and P. myriotylum which are responsible for hypocotyl and root diseases leading to damping off (i.e., plant death during seedling stage) in many crops including dry beans (Abawi and Widmer, 2000; Binagwa et al., 2016). Pieczarka and Abawi (1978) reported that P. ultimum acts synergistically with F. solani f. sp. phaseoli increasing root rot damage to beans, whereas R. solani is apparently antagonistic to P. ultimum, reducing the severity of root rot. Pythium and Fusarium spp. were the most frequently isolated fungi in a study in Rwanda and Uganda (Rusuku et al., 1997; Mukankusi et al., 2010), also finding Macrophomina phaseolina, R. solani, and Fusarium oxysporum. Several studies have reported a higher prevalence of some pathogens inside a complex; for example, Iran, Kenya and Turkey, F. solani predominated over R. solani and M. phaseolina (Erper et al., 2007; Mwang’ombe et al., 2007; Naseri, 2008), while in Michigan (United States), Fusarium presented the highest prevalence, followed by Rhizoctonia. Additionally, they concluded that the composition of the bean root rot pathogens appears not to depend on the year or the country, because the three main groups of pathogens were consistently recovered (Jacobs et al., 2019). Meanwhile, Fusarium and Pythium occurred in higher proportions at the CIAT station in Popayán, Colombia (Rojas, 2019).
Identification of beans with genetic resistance to Pythium spp. and other root rot diseases is key to allowing breeders to generate farmer preferred cultivars. Consequently, a number of genotypes providing some level of resistance have been identified such as MLB49-89A, MLB48-89A SCAM-80CM/15, and AND1055 (Buruchara and Rusuku, 1992; Mukankusi et al., 2011); RWR2075 and RWR1946 (Buruchara and Kimani, 1999; Namayanja et al., 2014); and RWR719 and AND1062 (Nzungize et al., 2011). Evaluating combined stresses of Pythium irregulare with waterlogging in 194 varieties, Navy Veracruz, Negro Argel, and Phavul 77 showed some level of resistance (Li et al., 2016). Moderately resistant varieties to Fusarium (G1459, G4795, G5658, Umumbano – G2333, MLB49-89A, Hoima-Kaki, Umgeni and RWR719) and Rhizoctonia (PI310668, PI533249) were also reported (Mukankusi et al., 2010; Peña et al., 2013); however, few resistant lines were identified, and new and better resistance sources are required to broaden the genetic base of resistant germplasm for breeders.
Interspecific crosses of P. vulgaris with sister species were generated at the CIAT bean breeding program in Cali-Colombia aiming to transfer disease resistance, and abiotic stress tolerance into common bean (Beebe et al., 2011; Butare et al., 2011; Beebe, 2012). A major resistance locus to common bacterial blight was transferred to dry bean from the donor Phaseolus acutifolius, resulting in lines with high levels of disease resistance in field evaluations (Singh and Muñoz, 1999). Germplasm derived from Phaseolus coccineus × P. vulgaris crosses were reported to harbor combined resistance to F. solani and P. ultimum (Mukankusi et al., 2018). Interspecific crosses are considered to be an option to broaden the genetic base of common bean for their adaptation to different environments.
Several studies investigated the inheritance of resistance to bean root rot. P. ultimum was reported to be conditioned by polygenic inheritance or quantitative inheritance (Dickson and Abawi, 1974; Navarro et al., 2008), whereas other studies suggest qualitative single dominant gene in resistance for P. ultimum (Otsyula et al., 2003). Ongom et al. (2012) reported single gene resistance for P. ultimum and quantitative resistance for Fusarium solani in RWR719-derived populations. Fusarium and Pythium resistances were not related. Mahuku et al. (2005) reported a SCAR marker associated with Pythium resistance gene in RWR719 and AND1062 genotypes. Genetics of root rot related traits such as plant emergence and vigor were studied in recombinant inbred line (RIL) populations (Campa et al., 2010), also under flooded and non-flooded conditions (P. ultimum and P. irregulare) (Soltani et al., 2018).
Several studies have identified quantitative trait loci (QTL) for Fusarium root rot resistance in RILs populations. QTL were identified for F. solani (Schneider et al., 2001; Román-Avilés and Kelly, 2005; Hagerty et al., 2015; Kamfwa et al., 2015b), Fusarium cuneirostrum (Nakedde et al., 2016) and Fusarium brasiliense (Schneider et al., 2001). Even though many studies have been carried out, molecular markers are rarely used in routine breeding. Better resistance sources are desirable, as are markers that work in breeding materials outside the studied RIL populations.
The availability of SNPs markers due to high-throughput genotyping technologies has allowed the development of molecular tools such as Genome-wide association studies (GWAS) and genomic prediction (GP) as support for breeding programs. GWAS aims to detect phenotype to genotype associations to identify major effect genes. GPs are based on a genetic model using genome wide markers simultaneously to estimate genomic breeding values based on the sum of all marker effects. GP is better suited to capture quantitative effects based on many minor genes (Wang et al., 2009).
The objective of the study was to evaluate a panel of lines with interspecific introgressions from Phaseolus coccineus for general root rot in the field, and specifically for Pythium root rot in the greenhouse. The genetic basis of root rot response was evaluated by GWAS and GP to improve knowledge on the inheritance of Pythium root rot resistance and to develop molecular breeding tools that can be deployed in the breeding program.
Materials and Methods
Plant Material
A panel of 228 lines (termed RR panel) was evaluated for root rot traits (Table 1). These lines were developed with the pedigrees SAP1-15 × ALB (181×), ABA58 × ALB300-2 (28×) and WAF18 × ALB328 (19×). The ALB lines were obtained from interspecific crosses; P. vulgaris × P. coccineus, [(SAB516 × (SAB258 × G35066) and (SAB514 × (SAB258 × G35464-5Q)], of which the majority were generated using the parent G35066 and only two using the parent G35464-5Q. F3 individuals were evaluated for root rot-related pathogens in 2014 at Popayan (2°25′N latitude, 76°40′W longitude, 1,730 m.a.s.l.) and 12 of the best and 12 of the least resistant families were selected to generate a population for genetic analysis. Ten individual selections from each family were advanced at the CIAT-Palmira station (3°29′N latitude; 76°21′W, 965 m.a.s.l.), finally resulting in 198 F5 lines, coded as Root rot resistance Andean lines (RRR). Furthermore, 30 bulk-derived lines, coded as Root Rot Andean lines (RRA) were selected for their outstanding performance for root rot resistance (Table 1). MLB49-89A was used as a resistance check for Pythium, while GLP2 and CAL96 were used as susceptible checks based on published results (Otsyula et al., 2002). Additional controls of the bean improvement program were used in the field trial (AND1055 and Calima as susceptible; Amadeus as intermediate, and Dicta17 as resistant).
Different data sets previously reported for other common bean root rot-related pathogens in the Andean Diversity Panel ADP were also used in this study. The ADP represents the genetic diversity of cultivated germplasm of the Andean genepool, containing landraces, breeding lines and varieties from public and private breeding programs (Cichy et al., 2015a, b; Kamfwa et al., 2015a; Tock et al., 2017; Oladzad et al., 2019; Zitnick-Anderson et al., 2020). The ADP was evaluated for Pythium ultimum (Pyth_UGA) and Fusarium cuneirostrum (Fus_UGA) under greenhouse conditions at the CIAT-Kawanda research station (Uganda) as described by Amongi et al. (2020) and Onziga Dramadri et al. (2020). This panel was also evaluated under greenhouse conditions for resistance to Rhizoctonia solani (Rhiz_USA) and Fusarium solani (Fus_USA) at North Dakota State University (United States) as described by Oladzad et al. (2019) and Zitnick-Anderson et al. (2020). In addition, we used the Angular Leaf Spot (ALS) datasets reported by Nay et al. (2019). These datasets consisted of 316 genotypes (termed extBALSIT panel) which were evaluated under greenhouse and field conditions at multiple sites in Colombia and Uganda.
Root Rot Phenotyping Under Greenhouse Conditions
A pure colony of a pathogenic isolate of Pythium myriotylum preserved on potato carrot agar was reactivated on potato dextrose agar at 24°C for 7 days and then, slants of the pathogen were increased on sterilized sorghum seeds. The inoculum was prepared following the method described by Castellanos et al. (2016). The sterilized soil:sand mix (3:1 ratio) was infected using 0.15 g of inoculum per kg of soil, and then poured into a fiber glass tray (190 × 90 × 25 cm).
Three separate evaluations were performed in the greenhouse at the CIAT-Palmira station (Table 1). On each evaluation, 228 RR lines and 21 checks (15 ALB lines, 4 Andean lines and 2 Mesoamerican lines) were sown in trays containing soil infected with P. myriotylum, using rows of 10 seeds per genotype (60 genotypes per tray) following an augmented-block design. The lines MLB49-89A and GLP2 were used as resistant and susceptible controls on each tray. To favor the growth of the inoculum, the soil was watered regularly and the temperature inside the greenhouse was maintained between 24° (night) to 32°C (day). The evaluations of the root hypocotyl and stem were carried out at 7, 14, 21, and 28 days after planting. After the last evaluation, the plants were uprooted to score the root damage following the standard 1 to 9 visual scale developed at CIAT. In this scale, 1 = no visible disease symptoms. 3 = light discoloration either without necrotic lesions or with approximately 10% of the hypocotyl and root tissues covered with lesions, 5 = approximately 25% of the hypocotyl and root tissues covered with lesions but tissues remain firm with deterioration of the root system. Heavy discoloration symptoms may be evident, 7 = approximately 50% of the hypocotyl and root tissues covered with lesions combined with considerable softening, rotting and reduction of the root system. 9 = approximately 75% or more of the hypocotyl and root tissues affected with advanced stages of rotting combined with a severe reduction in the root system (van Schoonhoven and Pastor-Corrales, 1987). The sequential Pythium response (Pyth) scores obtained for the RR panel in the greenhouse were used to calculate the progress of the disease using the area under the disease-progress curve (AUDPC).
Root Rot Phenotyping in the Field
A total of 216 genotypes, including 198 RR lines and 18 checks (12 ALB lines, 4 Andean and 2 Mesoamerican lines), were evaluated in a field with natural root rot infection at the CIAT-Popayán station (Table 1). Two months before the planting date, the susceptible genotype CAL96 was planted to increase the inoculum and 20 days after germination it was incorporated in the soil. Vegetative material was allowed to break down for 20 days. The trial was planted following an Alpha-Lattice design with four replicates. The experimental units were one-row plots of 2.21 m in length with a row-to-row distance of 0.8 m and 15 seeds per meter. The evaluated variables were: number of plants emerged per complete plot 12 days after sowing (NEPl); three evaluations were conducted for plant vigor (PVg) (12, 35, and 60 days after sowing), using a visual PVg score from 1 to 9 (7 to 9 poor amount of aerial biomass, 4 to 6 acceptable amount of aerial biomass and 1- to 3 excellent amount of aerial biomass). In addition, the weight of 100 seeds (100SdW, g 100 seeds–1, weighed on an analytical scale) was evaluated in an unreplicated seed multiplication trial. No destructive sampling was conducted in the field.
DNA and GBS, SNP Calling
Genotype-by-sequencing (GBS) data for the RR population was generated as described by Gil et al. (2019). DNA was extracted with the Urea-based protocol, according to the method described by Chen et al. (1992) and followed by quality check on 0.8% agarose gels. Quantification was conducted with Quant-iTTM PicoGreen® dsDNA Assay Kit (Invitrogen, Carlsbad, CA), measured in a Synergy H1m (BioTek Instruments, Inc., Winooski, VT, United States). DNA quantity was determined by regression against a standard sample of known DNA concentration. The library for GBS was prepared at CIAT, following the protocol described by Elshire et al. (2011) and sequenced in an Illumina HiSeq 2500 sequencer at the HudsonAlpha Genome Sequencing Center1. The ADP accessions were previously genotyped with the same GBS protocol, and the data was retrieved from the ARS-Feed the Future Bean Research Team2.
The GBS reads from the RR and ADP populations were mapped to the Phaseolus vulgaris reference genome of the accession G19833 (v2.1) (Schmutz et al., 2014). The SNP calling process and VCF filtering was performed using NGSEP (v4.0.3) (Tello et al., 2019), following recommended parameters for GBS data (Perea et al., 2016). The filtering of the VCF removed markers falling in repetitive regions of the genome, as described by Lobaton et al. (2018), genotype call quality below 40, MAF below 0.02, a per-marker heterozygosity rate above 0.02 and markers with less than 285 (∼57%) genotype calls. The filtered VCF contained approx. 20% of missing data that was imputed using BEAGLE (v5.0) (Browning et al., 2018), setting the effective population size at 100 and providing the genetic map reported by Diaz et al. (2020). The genetic position of the final markers was obtained by fitting spline regressions on that genetic map with the R function “smooth.spline” (v4.0.3) with default parameters.
Statistical Analysis
The AUDPC data from four different evaluations in the greenhouse performed on the RR panel was modeled using the following formula:
Where y is a vector of AUDPCs calculated from the four sequential evaluations of Pyth scores, Gi is the effect of the ith genotype, Ej is the effect of the jth evaluation, (GE)ij is the genotype-evaluation interaction term, (ET)jk is the effect of the kth tray nested within the jth evaluation, and εijk is the error term corresponding to yijk. In this model, the terms (GE)ij, (ET)ik were treated as random effects. The term Gi was treated either as fixed (to calculate best linear unbiased estimators - BLUEs) or random (to obtain an estimate of the genetic variance and to calculate best linear unbiased predictors - BLUPs). Additional models that did not include the evaluation term Ej and its interactions from equation 1 were fitted for each evaluation separately in order to assess their individual variance components, heritabilities, and comparability of BLUEs. We assumed that every random term u and the residual ε adjusts to a normal distribution with mean 0 and independent variances u~ and ε~.
The spatial arrangement of the experiment in the field was used to assign row and column coordinates to each plot. The PVg scores that were obtained from three separate evaluations were modeled using the following formula:
Where y is a vector of PVg scores obtained from the field trial, Gi is the effect of the ith genotype, Ej is the effect of the jth evaluation, (GE)ij is the genotype-evaluation interaction term, (ER)jk is the effect of the kth replication nested within the jth evaluation, (ERc)jkl and (ERr)jkm are the effects of the lth column and mth row nested within the jkth replication, respectively. In this model, the terms (GE)ij, (ER)jk, (ERc)jkl, and (ERr)jkm were treated as random effects. The term Gi was treated either as fixed (to calculate BLUEs) or random (to obtain an estimate of the genetic variance and to calculate BLUPs). We assumed every random term u~ and the residual ε~.
The NEPl and the individual PVg evaluations were modeled fitting a linear mixed model with spatial components using the functions “SpATS” and “PSANOVA” of the R package SpATS (Rodriguez-Alvarez et al., 2020). The phenotypic observations with residuals beyond +/−3 standard deviations from zero in Eqs. 1, 2 and the SpATS model were classified as outliers and removed. The variance explained by the random terms in equations 1 and 2 was tested using the Likelihood Ratio Test (LRT). This value was compared with a chi-square value with one degree of freedom and the significance p-value was adjusted following Self and Liang (1987). Broad-sense heritability estimates were calculated using the method proposed by Cullis et al. (2006), using the formula:
Where υBLUP is the mean variance of a difference of two BLUPs of genotypic effects, is the genetic variance.
New variables were also calculated to obtain greater clarity about the general behavior of Pythium. The root rot damage score RRD_field is the result of joining the BLUEs of NEPl and PVg traits, assuming that both variables are directly related to the resistance or susceptibility response to the disease in each genotype. These variables were standardized to have mean 0 and variance of 1 before combining them. NEPl was added as a negative value to PVg, so as to obtain a new variable that was positively correlated with the general standard Pyth score proposed by van Schoonhoven and Pastor-Corrales (1987). In addition, an overall root rot damage score RRD_all, combined field and greenhouse traits in the same way. Pearson correlations coefficients between the different traits were calculated, and their significance was tested using a two-tailed t-test.
Genome-Wide Association Analyses for Root Rot Disease
The association analyses for RR were performed using the multi-locus random-SNP-effect Mixed Linear Model tools (mrMLM v4.0), which includes six multi-locus methods (Zhang et al., 2020) and FarmCPU (Liu et al., 2016). The six methods that the mrMLM software includes are: (1) mrMLM, (2) FASTmrMLM (Fast multi-locus random-SNP-effect EMMA), (3) ISIS EM-BLASSO (Iterative Sure Independence Screening EM-Bayesian LASSO), (4) pLARmEB (polygenic-background-control based least angle regression plus empirical Bayes), (5) pKWmEB (polygenic background - control-based Kruskal-Wallis test plus empirical Bayes); and (6) fast mrMLM (FASTmrMLM). The association models account for population structure using the top five principal components as covariates to control for population structure. In mrMLM each marker on the genome is scanned. The Bonferroni correction threshold is replaced by a less stringent selection criterion to identify significant associations. Then, all the markers that are potentially associated with the trait are included in a multi-locus genetic model, their effects are estimated by an empirical Bayes model and all the nonzero effects were further identified by likelihood ratio test for true QTL.
Genomic Predictions for Root Rot Disease
Genomic prediction (GP) was assessed on each trait of the RR, ADP and extBALSIT panels separately by cross validation, partitioning each panel 50 times randomly into 70% training and 30% validation population. GPs were performed using single Gaussian kernel Bayesian Reproducing Kernel Hilbert Spaces (RKHS) regressions with a fixed bandwidth parameter h = 0.5. These predictions were executed using the R package BGLR (v1.0.8) (Perez and de los Campos, 2014) with 10,000 iterations of the sample, 1,000 samples discarded (burn-in) and a thinning factor of 5 to compute posterior means. Prediction ability is expressed as a Pearson correlation coefficient between the observed and predicted breeding values in the RR and ADP panels.
To characterize the influence of the number of SNPs on the GP accuracy of the RR traits, the SNP markers were pruned using two different strategies. The first considered the linkage disequilibrium (LD) between SNP markers. For this, we used the option “-indep-pairwise” of PLINK (v1.90b6.9), with 50 kbp windows, a shift parameter of 5 kbp and maximum pairwise r2 thresholds of 0.3, 0.5, 0.7, 0.9, and 0.95 (Chang et al., 2015). The second strategy pruned SNP markers randomly throughout the genome.
Genomic Predictions Between Different Traits and Data Sets
In order to simulate a breeding scenario of employing genomic predictions to select future populations, a pairwise cross-prediction scenario between root rot evaluations on the RR and ADP panels was performed. This scenario was divided in two separate cases. The first consisted in using the data from a given trait (training population) to predict every other trait in the same panel, producing 18 different combinations of training-validation datasets. This case was useful in the RR panel to validate the prediction performance that the greenhouse data can have on the field data, and vice versa. Similarly, this case was useful in the ADP to validate the prediction ability when using data from a root rot pathogen to predict the phenotypic response to another root rot pathogen. The second case used data from a given trait-panel to predict every other trait in a different panel, producing 24 different combinations of training-validation datasets. The second case was useful to explore the prediction ability across populations (ADP-RR), conditions (greenhouse-field) and causal agents of root rots.
Results
Root Rot Evaluations in Greenhouse and Field
In this study we evaluated different symptoms of root rot disease in field experiments, and more specifically Pythium myriotylum root rot in the greenhouse. Symptoms included poor seedling establishment/damping-off (NEPl), poor development of aerial biomass (PVg), and root necrosis (Pyth). The phenotypic responses showed normal distributions for most traits in line with a quantitative nature of the disease response (Figure 1). Trait values for positive and negative controls were found as expected on the extremes of the distribution. No clear transgressive segregation for resistance beyond positive checks was observed.
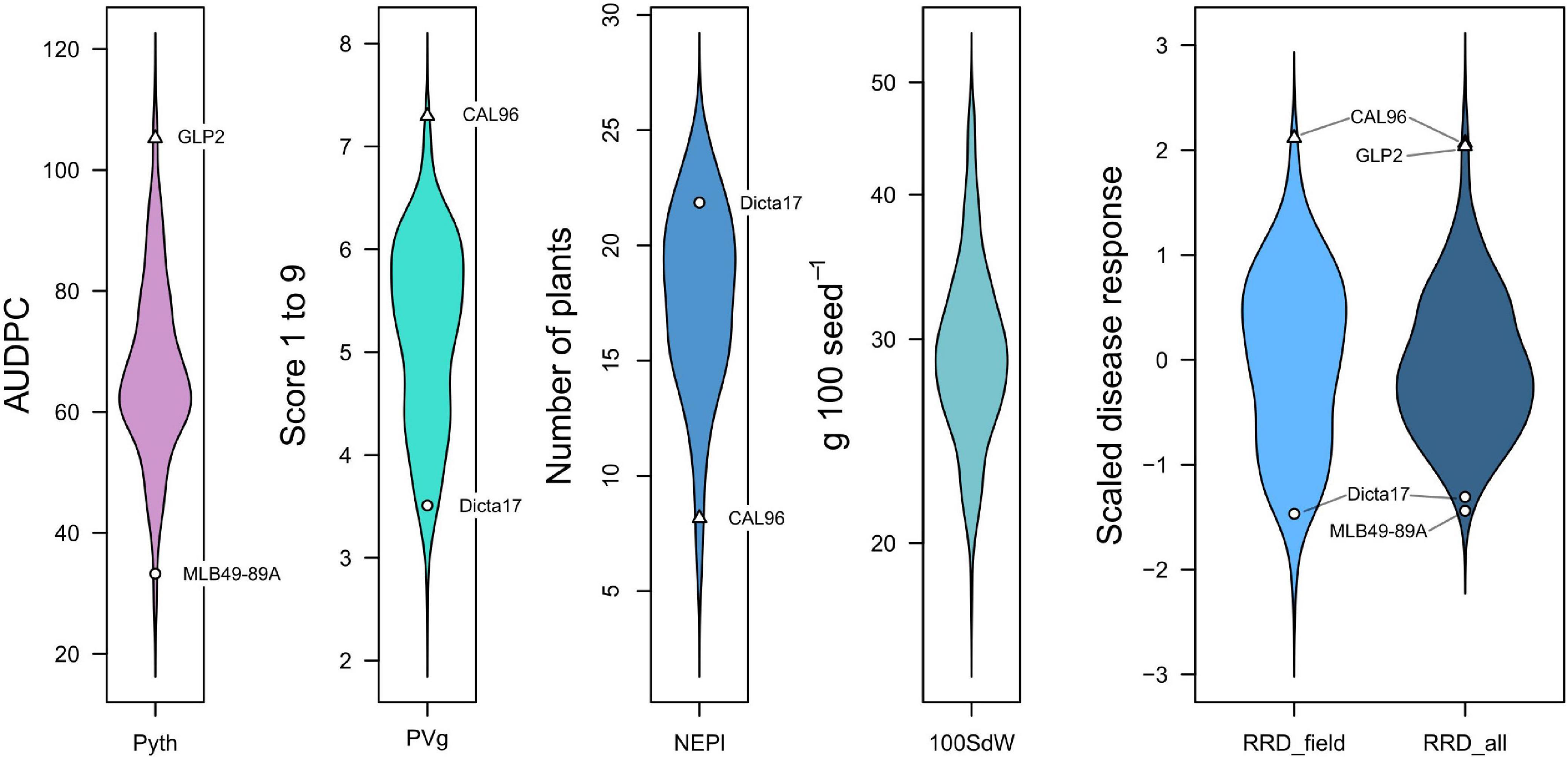
Figure 1. Density distribution of root rot and other traits evaluated in greenhouse and field trials. Resistant checks are marked with circles (MLB49-89A, Dicta17) and triangles indicate susceptible checks (GLP2, CAL96). Traits: Pyth (P. myriotylum disease response in the greenhouse as AUDPC), NEPl (number of emerged plants 12 days after sowing in the field), PVg (Plant vigor in the field), RRD_field (root rot damage in the field), RRD_all (root rot damage combining all data sets), and 100SdW (100 seed weight).
Significant phenotypic variation was detected among lines for Pyth, PVg, and NEPl (Table 2). The genotypic variation was the main source of variability in the trials studied. The estimated broad-sense heritability values were high for all traits (Figure 2 and Supplementary Figure 1); the highest was noted for PVg and NEPl (0.94 and 0.85, respectively). The correlations for Pyth between the three replications over time were significant (0.47–0.56) demonstrating an acceptable level repeatability for these trait evaluations (Supplementary Figure 1) under the presence of significant genotype × evaluation interactions (Table 2). Similarly, Pyth evaluated in the greenhouse showed significant correlations with other disease-related traits (PVg, NEPl, RRD_field, and RRD_all), as well as 100SdW (ranging 0.43–0.77) (Figure 2 and Supplementary Figure 1). This indicates acceptable levels of comparability between the evaluations in the greenhouse and the evaluations in the field. NEPl-PVg correlations were expectedly negative as the traits have inverse scales: resistance to the disease is represented by high NEPl numbers and small PVg scores. The correlation of 100SdW and the disease response may be due to the large seeded Andean parents which are susceptible to root rots. Alternatively, seed size may affect germination speed and susceptibility during the germination phase, which may explain the disease correlation of NEPl but not PVg. Taken together, these results indicate that the Pyth evaluations in the greenhouse and the emergence and vigor evaluations in the field have the same genetic base, and that the same attributes of the lines were evaluated in these quite different trials.
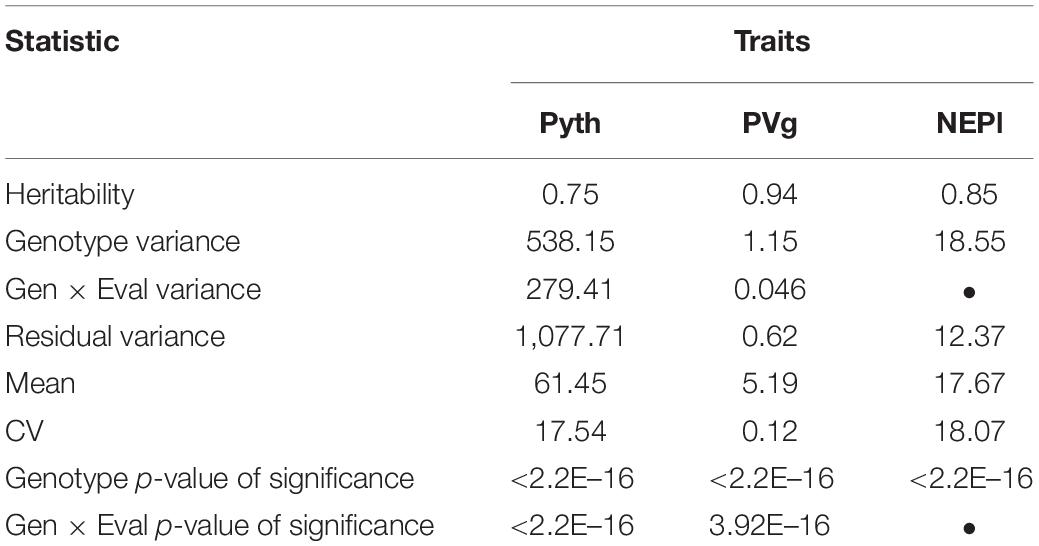
Table 2. Broad-sense heritability and statistics of the mixed models used to analyze the RR panel data from the field and the greenhouse.
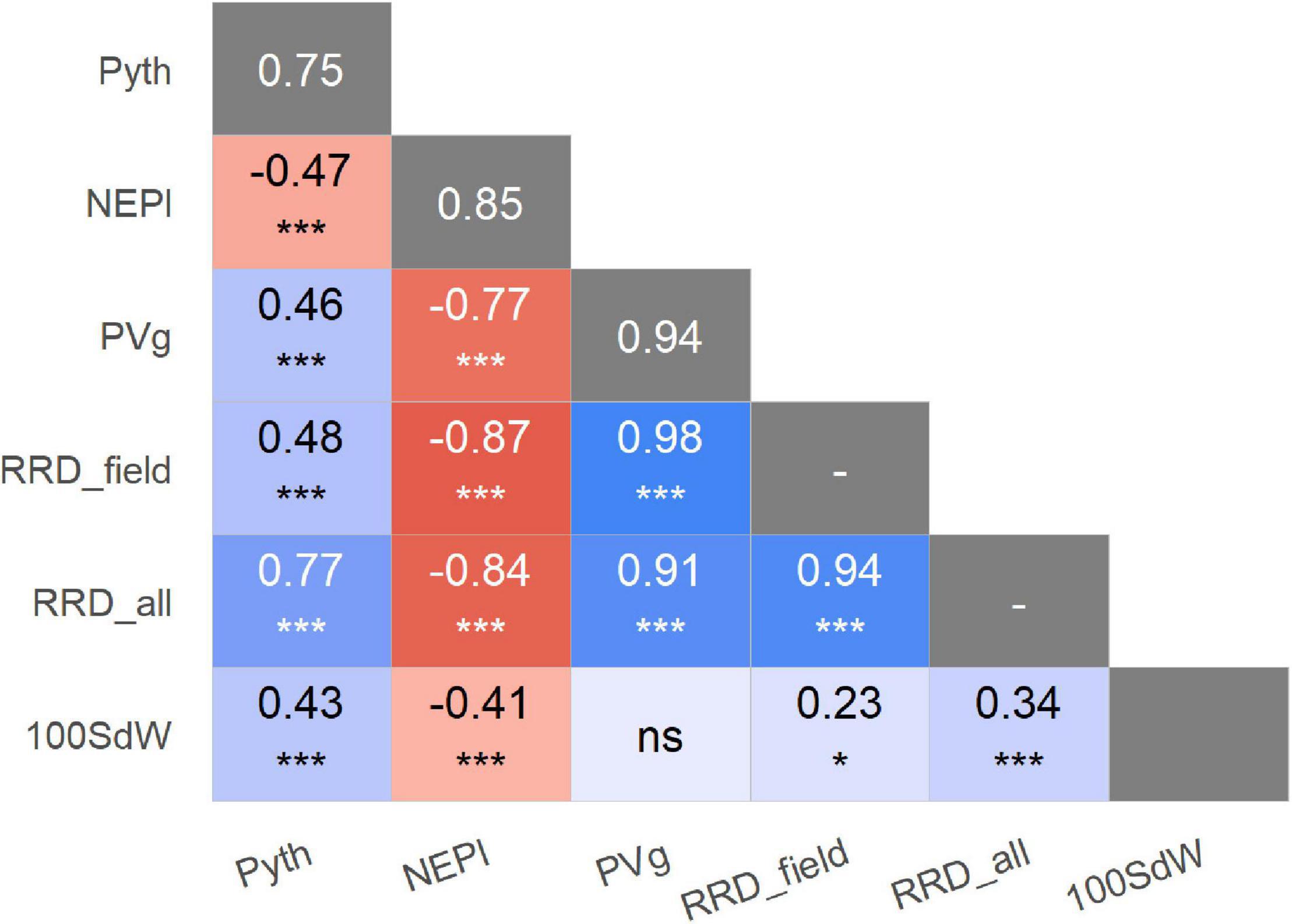
Figure 2. Phenotypic correlations of traits evaluated in greenhouse and field trials, expressed as Pearson coefficients. Significance of correlations indicated as: *p < 0.05; ***p < 0.001; ns not significant. The broad-sense heritabilities are presented in the main diagonal with gray background. Pyth (P. myriotylum in greenhouse), NEPl (number of emerged plants 12 days after sowing), PVg (Plant vigor in field), RRD_field (root rot damage), RRD_all (root rot damage combining all data set), and 100SdW (seed weight).
Population Structure and Identification of Best Crosses and Lines
The population structure of the RR panel was evaluated by principal component analysis (PCA) using 15,004 SNP markers. These markers were located mainly on the euchromatic regions of the genome due to the (i) use of the methylation-sensitive enzyme ApeKI for the GBS library preparation and (ii) the matrix filter that removed markers from repetitive regions of the genome (Supplementary Figure 2A). However, the genetic scale shows a more uniform distribution of markers along the genome that enriches the regions with higher gene density and recombination frequencies (Supplementary Figure 2B). The first and second PCs explained 24.71% of the variability, revealing moderate but significant population structure (Figure 3). These two PCs mostly separate lines based on their pedigree, with some overlaps. The most separated cluster on the lower left is formed by lines with the pedigree ABA58 × ALB300-2. They originate from a different F2:3 family than the other lines of that same pedigree. During population development, F2:3 families were selected based on their good or bad performance under root rot pressure. This performance is largely mirrored by the advanced RIL lines (Supplementary Figure 3). The best crosses that combine resistance to Pyth in the greenhouse and root rots in the field were SAP1-15 × ALB300-1 and ABA58 × ALB300-2 (Figure 4). The best performing lines were RRR160, RRR161, RRR165, RRR82, RRR84, RRR86, and RRR90, which subsequently have the best score for RRD_all. The susceptible check CAL96 is on the poorest end of the spectrum for both Pyth and RRD_field. The ALB parental lines, however, appear intermediate and not among the best performing lines. Evaluations of parental lines in a previous experiment (Supplementary Table 1) showed Phaseolus coccineus ancestors and ALB lines superior to Andean parental lines, however, ALB lines probably underwent unintended selection for root rot tolerance during population advance, interfering with a quantitative comparison of resistance levels or the segregation of potential resistance genes.
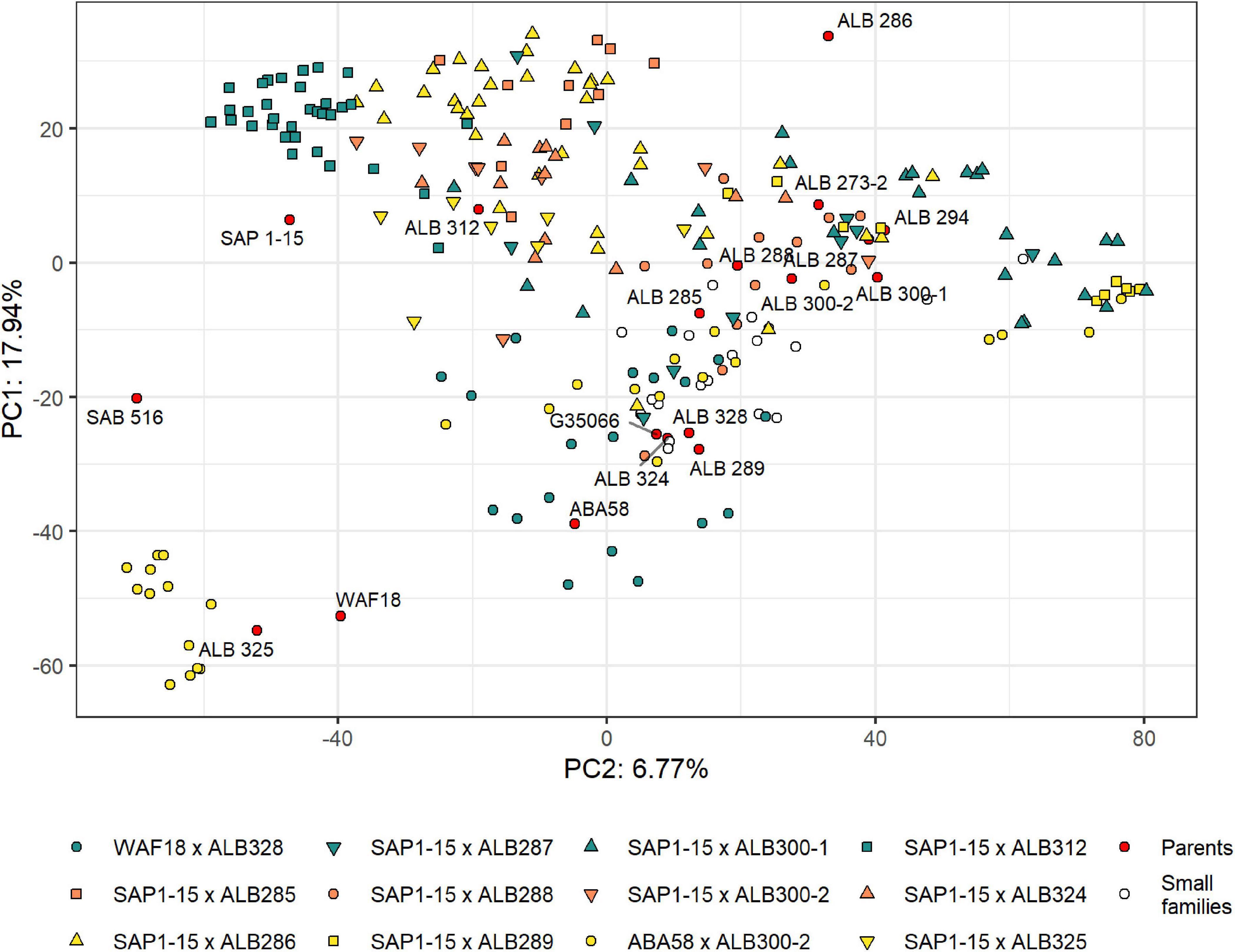
Figure 3. Principal Component Analysis (PCA) displaying the population structure of the RR panel and parental lines. The pedigrees of each larger family (n > 5) are represented with colored symbols, smaller families (n < 5) are represented with an empty circle. The parents used for the different crosses are located within the graph as red tagged circles.
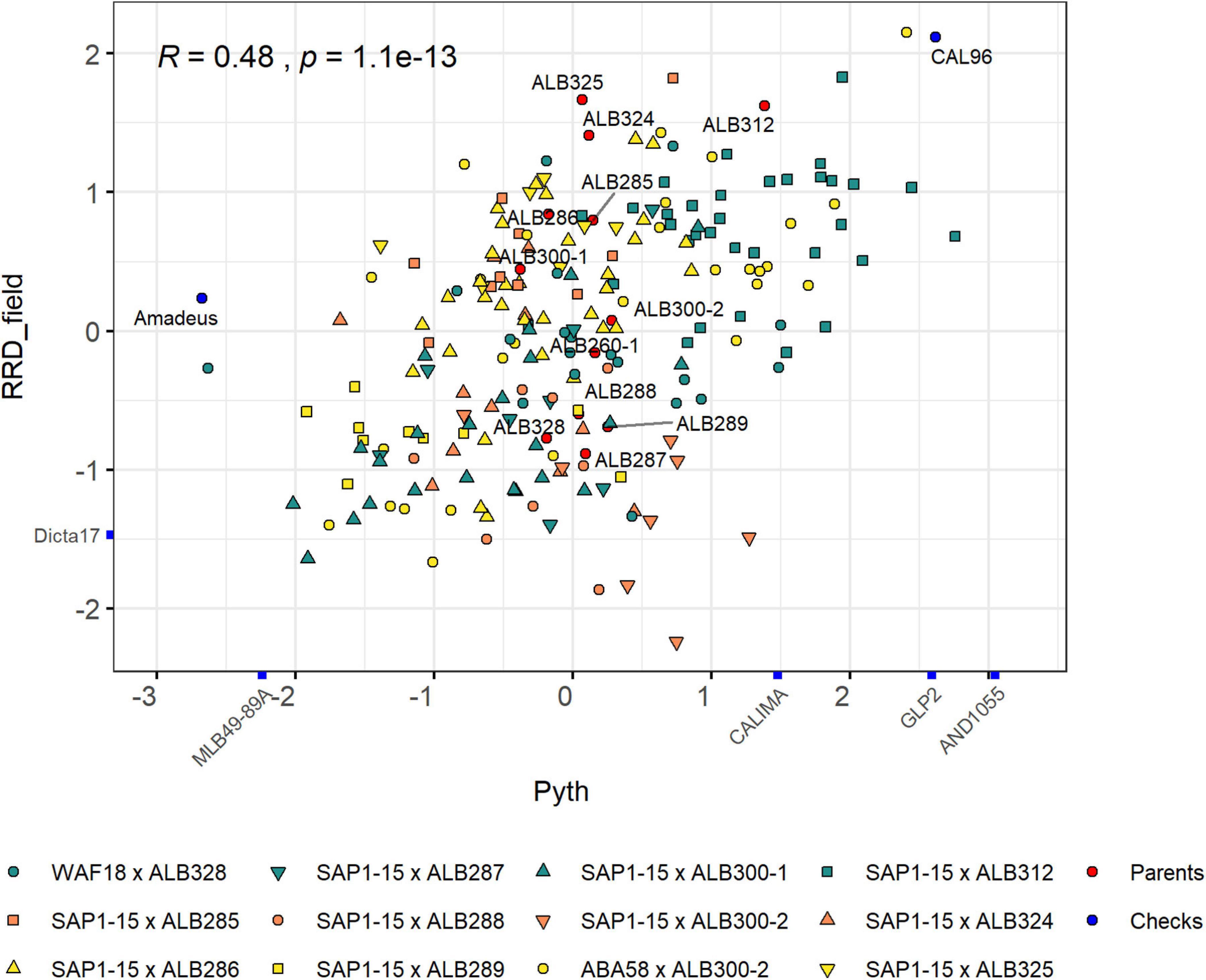
Figure 4. Relationship between Pythium damage in the greenhouse and root rot damage in the field for the RR panel. These measurements were scaled to facilitate the comparison. The pedigree of each family is represented with colored symbols. The control checks and the parents used for the different crosses are depicted in blue and red symbols, respectively.
GWAS for Root Rot Damage
GWAS was performed testing 7 different algorithms to identify QTL for root rot disease traits and their associated markers (Supplementary Table 2). These analyses identified possible genomic regions underlying root rot responses; however, numerous inconsistencies between the different models were revealed. We considered SNP markers with significant associations having p < 1 × 10–5 in two or more models. A QTL located on chromosome 5 at 80,772 bp for PVg, RRD_field and RRD_all stood out explaining 6.86–30.61% of the phenotypic variation. Also, on the start of chromosome 8 four models identified QTL for field root rot traits. However, only one model reported a single significant marker associated with Pyth, showing no overlap with field traits, which would be expected for a major resistance gene. Data for 100 seed weight (100SdW) from the unreplicated seed multiplication trial was evaluated as a control trait for which QTL are usually identified. Five out of seven models identified QTL for this trait at the start of chromosome 7 (100SdW7.1 and 100SdW7.2), presenting the highest LOD identified in 4 out of 7 models. In addition, six models identified other QTL in chromosomes 6 (100SdW6.1) and 8 (100SdW8.1) with lower significance. Identification of strong QTL signals for 100SdW confirmed that this data set is suitable for GWAS; interestingly, not all GWAS models identified these QTL. Taken together, no major resistance genes were found, hence, the results suggest a quantitative inheritance for the observed heritable root rot disease tolerance.
Genomic Prediction of Root Rot Related Traits and ALS
The accuracy of genomic prediction (GP) models was evaluated using the RKHS model for all traits within each RR trial. In addition, we tested how GP performs in a qualitatively inherited biotic stress trait. GP accuracies of root rot disease traits ranged from 0.7 to 0.8 (Figure 5). The overall root rot disease damage score RRD_all, which incorporates most data, had the highest prediction accuracy (PA). PAs within the RR panel were not significantly affected by the number of SNPs markers, and the data suggests that 1,000–1,500 SNPs is an adequate number to perform predictions in root rot related traits and 100SdW of this study (Supplementary Figure 4). The PAs for the ADP trials were lower, with mean accuracies of 0.42 and 0.53 for the Pythium and Fusarium trials performed in Uganda (Amongi et al., 2020; Onziga Dramadri et al., 2020), and 0.26 and 0.28 for the Fusarium and Rhizoctonia trials performed in North Dakota (Oladzad et al., 2019; Zitnick-Anderson et al., 2020; Figure 5). We also evaluated Angular leaf spot (ALS) response data previously published by Nay et al. (2019), who reported a major disease resistance gene by GWAS in a panel of 316 common bean lines. GP accuracies for ALS response in the three locations (Darien, Quilichao and Kawanda) were high, ranging from 0.60 to 0.75 (Figure 5). The GP models explained 0.68, 0.70, and 0.65% of the phenotypic variation for ALS (broad-sense heritability), which is superior to reported GWAS QTL (between 8.6 and 31.4% explained variance) in capturing the genetic variation of this qualitative disease trait.
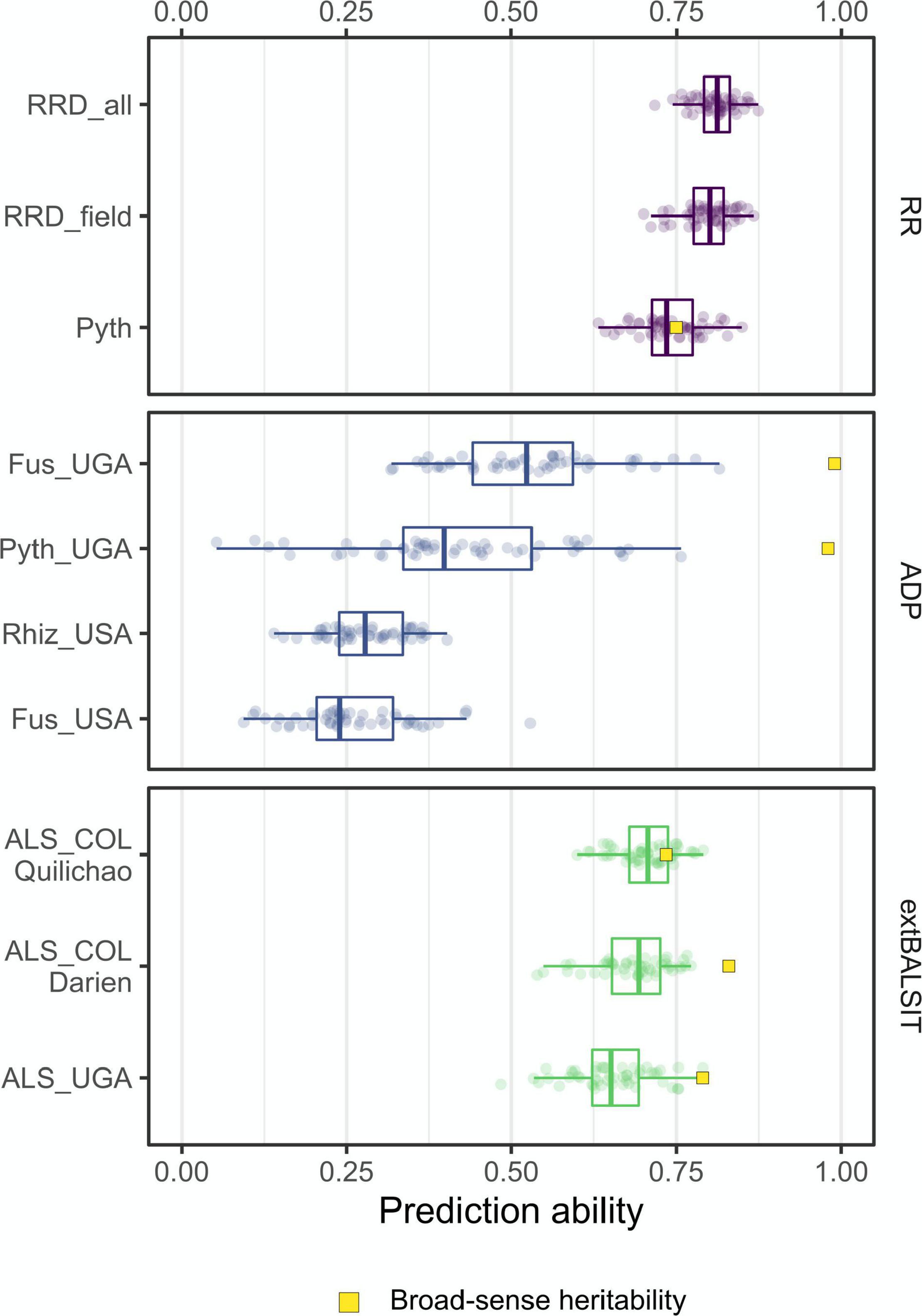
Figure 5. Genomic prediction accuracies for root rot-related traits and Angular Leaf Spot (ALS). Each boxplot shows the distribution of correlation coefficients obtained from the 50-fold cross-validation process. The traits Pyth_COL (P. myriotylum response in the greenhouse) RRD_field (root rot damage in the field) and RRD_all (root rot damage combining both datasets) were evaluated in the RR panel in Colombia (COL). The traits Fus_UGA (F. cuneirostrum), Pyth_UGA (P. ultimum), Rhiz_USA (R. solani) and Fus_USA (F. solani) were evaluated in the ADP panel in Uganda (UGA) (Amongi et al., 2020; Onziga Dramadri et al., 2020) and the USA (Oladzad et al., 2019; Zitnick-Anderson et al., 2020). The ALS response was evaluated in the extBALSIT panel in Colombia (COL) and Uganda (UGA) (Nay et al., 2019). The broad-sense heritability was calculated using the BLUPs of genotypic effects where they were available.
To evaluate how GP can predict performance across different evaluations and traits in the RR population, we used RRD_field data to train a GP model and the greenhouse Pyth data as validation set, and vice versa. The average prediction accuracies were similar, field to greenhouse 0.56 and greenhouse to field 0.54, providing a subtle improvement over the phenotypic correlations of 0.48 (Figure 6). Accuracies are expectedly lower than those obtained from internal cross validation procedures. However, they were very close to their observed phenotypic correlations. In summary, prediction accuracies for these data sets of high heritability are strong and the observed highly significant phenotypic correlations between trials are also represented by the genetic modeling across trials.
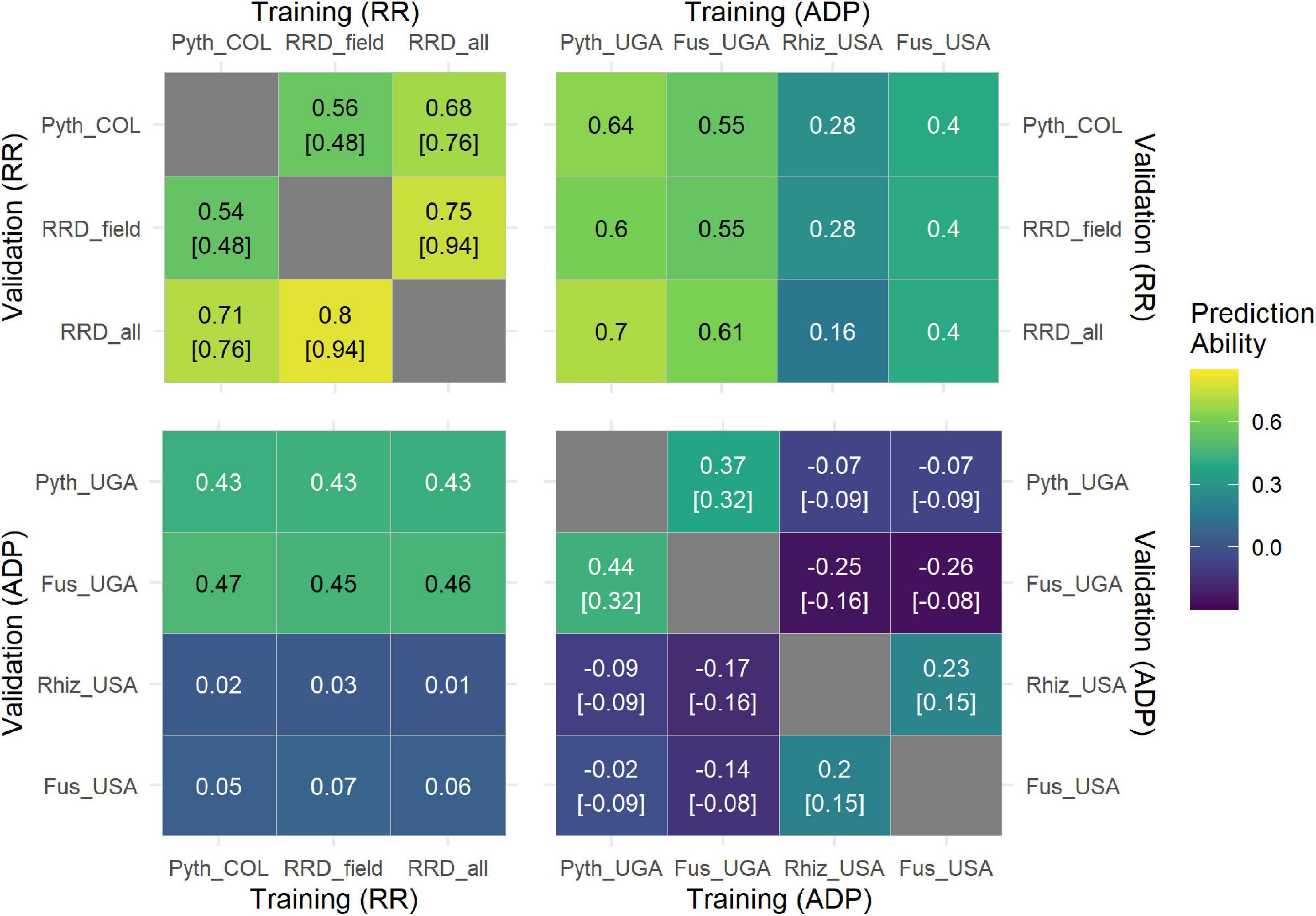
Figure 6. Correlogram with the results of the cross-prediction scenario using different training (X axis) and validation (Y axis) datasets. Predictions within populations are presented in the top-left and bottom-right correlograms. Each value corresponds to the mean correlation coefficient obtained from the 50-fold cross-validation process, while the values in square brackets indicate the phenotypic correlation between each pair of traits. Predictions across populations are presented in the bottom-left and top-right correlograms. Each value corresponds to the correlation coefficient between the observed and predicted breeding values. The cross-prediction scenario was performed for the traits Pyth_COL (P. myriotylum response in the greenhouse) RRD_field (root rot damage in the field) and RRD_all (root rot damage combining both datasets) that were evaluated in the RR panel in Colombia (COL). The traits Fus_UGA (F. cuneirostrum), Pyth_UGA (P. ultimum), Rhiz_USA (R. solani), and Fus_USA (F. solani) were evaluated in the ADP panel in Uganda (UGA) (Amongi et al., 2020; Onziga Dramadri et al., 2020) and the USA (Oladzad et al., 2019; Zitnick-Anderson et al., 2020).
Genomic Prediction for Root Rot Traits Across Populations
To investigate a potential general quantitative tolerance to root diseases, we evaluated GP accuracies across previously published data sets on related traits. PAs across trials were investigated with data sets of Pythium, Fusarium (Fus), and Rhizoctonia (Rhiz) root rot evaluated in the Andean Diversity Panel (ADP) (Oladzad et al., 2019; Amongi et al., 2020; Onziga Dramadri et al., 2020; Zitnick-Anderson et al., 2020). Cross validation within this panel showed highest prediction accuracies for evaluations in Uganda (Pyth_Uga and Fus_Uga) (Figure 6). Evaluating prediction ability across trials, the highest PAs of up to 0.7 were reached using Pyth_UGA data from the ADP evaluated in Uganda to predict RRD_all data in the RR panel in Colombia. Vice versa, the PA was lower (0.43), in line with the much lower genetic variation present in the RR panel, which is an insufficient training set to create a good prediction model for the more diverse ADP. These panels share no common lines. Interestingly, high PAs were also identified across Pyth_UGA and Fus_UGA vs. Pyth_COL data sets, suggesting a common quantitative genetic tolerance for both traits. The genetic correlation evaluated as PA using Fus_UGA and Pyth_UGA as training and validation set, respectively, slightly exceeded the phenotypic correlation between those traits. No high cross prediction ability was found for Rhiz_USA and Fus_USA responses, which also showed low heritabilities and subsequently low PAs within trials.
Discussion
Soil borne diseases are an increasing constraint for common bean production. Efforts to characterize suitable resistance sources, their genetic inheritance, and development of genetic tools are required to protect bean productivity. Root rot evaluations were often reported to suffer from a moderate heritability, e.g., high sample variation for F. solani root rot screening (Schneider et al., 2001). Progress in breeding for field resistance to the root rot complex was hampered by experimental difficulties due to field heterogeneity and large genotype × environment interactions (Beebe et al., 1981; Paez-Garcia et al., 2015). The data set generated in this work in field and greenhouse evaluations looked unimpressive at first, without clear separation of resistant and susceptible genotypes or transgressive segregation. However, the heritability of these trials was high, ranging between 0.71 and 0.94 for root rot related traits, indicating a strong genetic component in the disease response. These results are supported by the suitability of high number of replications, with four replications in the field and three replications over time in the greenhouse.
The strong comparability between field and greenhouse experiments defined by the similarity of their correlations (Figure 2 and Supplementary Figure 2) indicates that the same genetic effects are observed on each trial that was performed for this study. Small scale experiments in the greenhouse should reflect the same disease-related responses in the field (Schneider and Kelly, 2000), as assessments in the greenhouse are often cheaper and allow the evaluation of a large number of samples in a shorter time. The field trial at the Popayan station looked to be affected by root rot to the experienced eye, but it was not clear which pathogen exactly was causing the disease in the trial. A previous collection at this site for fungi and oomycetes identified a large variety of microorganisms, including several Pythium species (Rojas, 2019). In spite of that, we observed strong and comparable correlations between agronomic traits assessed in the field and Pythium damage evaluated in the greenhouse (Figure 2 and Supplementary Figure 1). In addition, their high heritability indicated a weak influence from environmental factors, contributing to their comparability. The relationship between NEPl and reaction to root rot caused by P. myriotylum has been reported by Nzungize et al. (2012). Similarly, plant vigor was reported to be related to Pythium (Campa et al., 2010; Soltani et al., 2018), as the plant’s development is affected even if it survives the seedling stage. These results show the consistency between the data from the field and the greenhouse, and the reliability that a combined analysis using both sources of information can provide.
Diverging reports on the genetics of Pythium-related resistance exist, stating qualitative responses to Pythium root rot controlled by a single dominant gene or the involvement of two or more genes (Otsyula et al., 2003; Mahuku et al., 2005; Nzungize et al., 2012). All the parents of the ALB lines originate from interspecific crosses (P. vulgaris × P. coccineus), so the initial goal of this study was to identify major resistance genes, based on introgressions from P. coccineus (Lobaton et al., 2018). However, ALB lines only showed intermediate resistance. Introgressions from P. coccineus in the ALB lines are few due to selection for P. vulgaris phenotypes, so P. coccineus-derived resistance genes would be expected to follow a more qualitative mode of inheritance. Neither were major resistance genes found in GWAS nor highly resistant lines beyond resistant checks. In spite of this, the dataset used in this study was suitable for association mapping, as GWAS identified strong signals for the control trait 100SdW in chromosomes 6, 7, and 8. The QTL 100SdW6.1 was located at 18,447,838 bp, close to the previously reported QTL 100SdW6.2, identified in the population DOR364 × BAT477 at 20.25 Mbp (Diaz et al., 2017). Several QTL were considered significant by different GWAS models, but no common QTL were identified here for the three evaluated root rot related traits.
Effective genomic cross–predictions of Pythium breeding values between the RR panel and the ADP do not support that the observed tolerance is exotic, but rather indicate a general quantitative tolerance to root rots available in Andean germplasm. Predictions across populations have been reported to be poor in lentil (Haile et al., 2020) and other crops (Charmet et al., 2014; Crossa et al., 2014), hence, the effective allelic diversity between ADP and RR panel must be similar. Taken together, our data suggests a quantitative mode of resistance of the traits evaluated here combining favorable different alleles from several precursors, in line with reports on Fusarium resistance (Ongom et al., 2012; Wang et al., 2018).
Genomic Prediction for Root Rot Disease
This study provided GPs for Pythium and Fusarium root rot disease resistance in common bean in the RR and ADP panels. GP accuracies were high (0.72–0.79 for Pyth and 0.52 for Fus_UGA), in line with the high heritabilities of these traits. GP accuracies of 0.52 and 0.41 were reported in common bean for two types of soybean cyst nematode (Wen et al., 2019). High prediction accuracies have also been reported for the soybean root disease sudden death syndrome (SDS), caused by Fusarium virguliforme where GPs accuracies reached 0.64 for root lesions score and lower precision for other root disease related traits (Bao et al., 2015). Prediction accuracies in common bean have been reported to correlate with heritabilities for other agronomic traits (Keller et al., 2020). We observed a similar trend in the RR and extBALSIT populations, but it was not the case for the ADP evaluations in Uganda (Figure 5), where the PA fell by around 40 points below the estimated heritability. This result can be attributable to the binary distribution with presence/absence scores that the original data display (Amongi et al., 2020; Onziga Dramadri et al., 2020), which confers high heritability estimates, and the RKHS model evaluated in this study, which performed the prediction of a trait with quantitative nature. Wen et al. (2019) confirmed that traits with high heritability would have higher PAs. Accuracies generally fall 10–20 points below the heritability of the respective trait, which is similar to most of the observations in this work.
The major molecular breeding application is often considered to be marker assisted selection (MAS), tagging genes with major effects. Genomic selection, on the other hand, has recently been promoted as a genetic tool to predict quantitative traits, yield and yield-related traits being the most investigated. In this work we have seen good GP accuracies for a quantitatively inherited disease trait, hence we extended this analysis to the well characterized qualitative ALS resistance in common bean. We saw that the GP model captures more genetic variation than GWAS in the clear presence of a major resistance gene, which was reported to explain 8.6–31.4% of variation (Nay et al., 2019). In maize, GPs for disease traits were also reported to be superior than GWAS in explaining the phenotypic variance for the quantitative resistance to maize lethal necrosis disease (Gowda et al., 2015). Also in maize, GPs were reported to be of high potential to help improve resistance to Fusarium ear rot in the absence of significant SNP-trait associations from GWAS (Holland et al., 2020). Using major loci as co-factors in the linear genomic prediction model has been suggested to improve the model in the presence of major genes. However, we did not observe any advantage to this approach, in line with reports that more often than not these cofactors do not improve model performance (Rice and Lipka, 2019). This suggests that most disease traits may be representable with whole genome models. GP, or its application as genomic selection, requires higher investments in genotyping and analytics compared to MAS, while providing superior information about phenotypic predictions. This apparently also holds true for most disease-related traits, which have often been considered the dominion of MAS. The implementation of genomic selection holds great promise to increase selection precision, allow early generation crosses and to generally select for conditions that the breeder does not have direct access to. This is particularly important for a breeding program such as CIAT’s which is targeting impact regions on other continents. Furthermore, phenotypic results from other panels can be used to train models that can be employed in selection, as we have shown in this study, with good predictability from ADP to RR panel. Especially if genomic models can be generated to allow predictions for several traits at a time, the obtained information may justify the higher investment.
Genetic Correlation of Resistance Traits
The correlation between field and greenhouse data is not based on a major resistance gene, as one might have expected for a disease resistance. This leaves the question what type of genetically controlled mechanism is conferring the trait correlations. Evaluations of agronomic plant performance in the field and visual scoring disease damage in the greenhouse are very different traits, and environmental conditions are contrasting between the hot greenhouses at Palmira on the one hand, and the Popayan field station at ∼700 m higher altitude on the other. Hence, the similarity in traits cannot just be attributed to adaptation. Also, we observe a strong genetic correlation with Fusarium response data from Uganda. Thus, the observed genetic tolerance must be a feature regulated by several genes leading to disease tolerance without being based on a specific resistance gene. Also for ALS, where resistance depends on a major gene, a quantitative model explained more variance, suggesting that other genes have an additional effect on the disease response. GP may capture better the unexplained variance not accounted for by major genes. Cross predictability to other germplasms like the ADP panel and other traits such as Fusarium response, indicate that we are looking at a general quantitative tolerance mechanism available in tropical Andean germplasm. This should be further characterized and explored to evaluate if breeding can generate acceptable tolerance levels for farmers following this method.
Conclusion
Soil borne diseases are a growing threat for bean production, a situation likely to be aggravated by climate change. Hence this trait is of high importance for breeding. Some level of genetic resistance or tolerance was found in this panel, showing good correlations of emergence and vigor observed in field trials with greenhouse data, which should aid selection. Genetic studies revealed no major resistance genes. Instead, genomic predictions performed well, with more potential than GWAS/MAS for these disease traits. We found significant predictive ability across the ADP and the RR panel and also across root rot response data for Pythium and Fusarium response. Data suggests a general quantitative resistance toward root rot disease present in tropical germplasm. The interactions between various root rot pathogens in bean disease development need further investigation. Applying GS looks promising for semi-quantitative or even qualitative traits, such as disease traits and more. This method needs to be evaluated with other quantitative and qualitative traits, and employed in breeding.
Data Availability Statement
The SNP marker matrix, the raw and modeled phenotypic data of the RR panel used in this study are available for download at Harvard Dataverse: https://doi.org/10.7910/DVN/SVA5CJ.
Author Contributions
BR, GM, and SB conceived the study. HFB, VA, and LMD conducted the experiments in the field and greenhouse. LMD, JA, and DA-S performed phenotypic and genotypic data analysis, GWAS, genomic predictions, and interpretation of results. CM provided the ADP data from Uganda. BR assisted in the experimental setup and data analysis. LMD, DA-S, and BR drafted the manuscript. All authors contributed to the article and approved the submitted version.
Funding
We acknowledge the U.S. Department of Agriculture National Institute of Food and Agriculture (USDA NIFA, Grant No. 2012-39571-20296) through Michigan State University for funding the project “Developing and Delivering Common Bean (P. vulgaris) Germplasm with Resistance to the Major Soil Borne Pathogens in East Africa.” AVISA - Accelerated varietal improvement and seed delivery of legumes and cereals in Africa (OPP1198373) projects funded by the Bill and Melinda Gates Foundation. We would like to thank USAID for their contributions through the CGIAR Research Program on Grain Legumes and Dryland Cereals.
Conflict of Interest
The authors declare that the research was conducted in the absence of any commercial or financial relationships that could be construed as a potential conflict of interest.
Acknowledgments
We thank Carlos Jara and Alvaro Soler for their support and helpful suggestions. Special thanks to the entire bean team at CIAT for their great support to our research. We thank Olga Spellman (the Alliance of Bioversity International and CIAT) for English editing of this manuscript.
Supplementary Material
The Supplementary Material for this article can be found online at: https://www.frontiersin.org/articles/10.3389/fpls.2021.629221/full#supplementary-material
Footnotes
- ^ hudsonalpha.org/, (accessed July 13, 2020).
- ^ http://arsftfbean.uprm.edu/bean/?p=472, (accessed January 2021).
References
Abawi, G. S., and Widmer, T. L. (2000). Impact of soil health management practices on soilborne pathogens, nematodes and root diseases of vegetable crops. Appl. Soil Ecol. 15, 37–47. doi: 10.1016/S0929-1393(00)00070-6
Amongi, W., Kato, F., Male, A., Musoke, S., Sebuliba, S., Nakyanzi, B., et al. (2020). Resistance of Andean beans and advanced breeding lines to root rots in Uganda. Afr. Crop Sci. J. 28, 377–397.
Bao, Y., Kurle, J. E., Anderson, G., and Young, N. D. (2015). Association mapping and genomic prediction for resistance to sudden death syndrome in early maturing soybean germplasm. Mol. Breed. 35, 1–14. doi: 10.1007/s11032-015-0324-3
Beebe, S. (2012). “Common bean breeding in the tropics,” in Plant Breeding Reviews, ed. J. Janick (Hoboken, NJ: John Wiley & Sons, Inc), 357–427.
Beebe, S., Ramirez, J., Jarvis, A., Rao, I. M., Mosquera, G., Bueno, J. M., et al. (2011). “Genetic improvement of common beans and the challenges of climate change,” in Crop Adaptation to Climate Change, eds S. S. Yadav, R. J. Redden, J. L. Hatfield, H. Lotze-Campen, and A. E. Hall (Hoboken, NJ: Blackwell Publishing Ltd), 356–369. doi: 10.1002/9780470960929.ch25
Beebe, S. E., Bliss, F. A., and Schwartz, H. F. (1981). Root rot resistance in common bean germplasm of Latin American origin. Plant Dis. 65, 485–489. doi: 10.1094/pd-65-485
Binagwa, P. H., Bonsi, C., Msolla, S. N., and Ritte, I. I. (2016). Morphological and molecular identification of Pythium spp. isolated from common beans (Phaseolus vulgaris) infected with root rot disease. Afr. J. Plant Sci. 10, 1–9. doi: 10.5897/ajps2015.1359
Broughton, W. J., Hern, G., Blair, M., Beebe, S., Gepts, P., and Vanderleyden, J. (2003). Beans (Phaseolus spp.) – model food legumes. Plant Soil 55, 55–128.
Browning, B. L., Zhou, Y., and Browning, S. R. (2018). A one-penny imputed genome from next-generation reference panels. Am. J. Hum. Genet. 103, 338–348. doi: 10.1016/j.ajhg.2018.07.015
Buruchara, R., and Kimani, P. (1999). “Identification of Pythium root rot within market classes of beans,” in Proceeding of the Meeting Demand for Beans in sub-Saharan Africa in Sustainable Ways. Annual report 1999 Project IP2 (Cali, Co: CIAT).
Buruchara, R., and Rusuku, G. (1992). “Root rots research in the Great Lakes region,” in Proceedings of the PAN-Africa bean Pathology Working Group Meeting, eds R. Buruchara and U. Scheidegger (Thika: CIAT).
Butare, L., Rao, I., Lepoivre, P., Polania, J., Cajiao, C., Cuasquer, J., et al. (2011). New genetic sources of resistance in the genus Phaseolus to individual and combined aluminium toxicity and progressive soil drying stresses. Euphytica 181, 385–404.
Campa, A., Pérez-Vega, E., Pascual, A., and Ferreira, J. J. (2010). Genetic analysis and molecular mapping of quantitative trait loci in common bean against Pythium ultimum. Phytopathology 100, 1315–1320. doi: 10.1094/PHYTO-06-10-0161
Castellanos, G., Jara, C., and Mosquera, G. (2016). Bean Pathogens: Practical Guide for Lab and Greenhouse Work. (Publicación CIAT No. 394), 2nd Edn. Cali, CO: CIAT.
Chang, C. C., Chow, C. C., Tellier, L. C., Vattikuti, S., Purcell, S. M., and Lee, J. J. (2015). Second-generation PLINK: rising to the challenge of larger and richer datasets. GigaScience 4:7. doi: 10.1186/s13742-015-0047-8
Charmet, G., Storlie, E., Oury, F. X., Laurent, V., Beghin, D., Chevarin, L., et al. (2014). Genome-wide prediction of three important traits in bread wheat. Mol. Breed. 34, 1843–1852. doi: 10.1007/s11032-014-0143-y
Chen, J., Greenblatt, I. M., and Dellaporta, S. L. (1992). Molecular analysis of Ac transposition and DNA replication. Genetics 130, 665–676.
Cichy, K. A., Porch, T. G., Beaver, J. S., Cregan, P., Fourie, D., Glahn, R. P., et al. (2015a). A diversity panel for Andean bean improvement. Crop Sci. 55, 2149–2160. doi: 10.2135/cropsci2014.09.0653
Cichy, K. A., Wiesinger, J. A., and Mendoza, F. A. (2015b). Genetic diversity and genome-wide association analysis of cooking time in dry bean (Phaseolus vulgaris L.). Theor. Appl. Genet. 128, 1555–1567. doi: 10.1007/s00122-015-2531-z
Crossa, J., Pérez, P., Hickey, J., Burgueño, J., Ornella, L., Cerón-Rojas, J., et al. (2014). Genomic prediction in CIMMYT maize and wheat breeding programs. Heredit 112, 48–60. doi: 10.1038/hdy.2013.16
Cullis, B. R., Smith, A. B., and Coombes, N. E. (2006). On the design of early generation variety trials with correlated data. J. Agric. Biol. Environ. Statist. 11, 381–393. doi: 10.1198/108571106X154443
Diaz, L. M., Ricaurte, J., Cajiao, C., Galeano, G., Rao, I., Beebe, S., et al. (2017). Phenotypic evaluation and QTL analysis of yield and symbiotic nitrogen fixation in a common bean population grown with two levels of phosphorus supply. Mol. Breed. 37, 1–16. doi: 10.1007/s11032-017-0673-1
Diaz, S., Ariza-Suarez, D., Izquierdo, P., Lobaton, J. D., de la Hoz, J. F., Acevedo, F., et al. (2020). Genetic mapping for agronomic traits in a MAGIC population of common bean (Phaseolus vulgaris L.). BMC Genomics. 21:799. doi: 10.7910/DVN/JR4X4C
Dickson, M. H., and Abawi, G. (1974). Resistance to Pythium ultimum in white seeded snap beans (P. vulgaris). Plant Dis. Rep. 58, 774–776.
Elshire, R. J., Glaubitz, J. C., Sun, Q., Poland, J. A., Kawamoto, K., Buckler, E. S., et al. (2011). A robust, simple genotyping-by-sequencing (GBS) approach for high diversity species. PLoS One 6:e19379. doi: 10.1371/journal.pone.0019379
Erper, I., Karaca, G., and Ozkoc, I. (2007). Root rot disease incidence and severity on some legume species grown in Samsun and the fungi isolated from roots and soils. Arch. Phytopathol. Plant Protect. 41, 501–506. doi: 10.1080/03235400600833779
Gil, J., Solarte, D., Lobaton, J. D., Mayor, V., Barrera, S., Jara, C., et al. (2019). Fine-mapping of angular leaf spot resistance gene Phg-2 in common bean and development of molecular breeding tools. Theor. Appl. Genet. 132, 2003–2016. doi: 10.1007/s00122-019-03334-z
Gowda, M., Das, B., Makumbi, D., Babu, R., Semagn, K., Mahuku, G., et al. (2015). Genome-wide association and genomic prediction of resistance to maize lethal necrosis disease in tropical maize germplasm. Theor. Appl. Genet. 128, 1957–1968. doi: 10.1007/s00122-015-2559-0
Hagerty, C. H., Cuesta-Marcos, A., Cregan, P. B., Song, Q., McClean, P., Noffsinger, S., et al. (2015). Mapping Fusarium solani and aphanomyces euteiches root rot resistance and root architecture quantitative trait loci in common bean. Crop Sci. 55, 1969–1977. doi: 10.2135/cropsci2014.11.0805
Haile, T. A., Heidecker, T., Wright, D., Neupane, S., Ramsay, L., Vandenberg, A., et al. (2020). Genomic selection for lentil breeding: empirical evidence. Plant Genome 13, 1–15. doi: 10.1002/tpg2.20002
Holland, J. B., Marino, T. P., Manching, H. C., and Wisser, R. J. (2020). Genomic prediction for resistance to Fusarium ear rot and fumonisin contamination in maize. Crop Sci. 60, 1863–1875. doi: 10.1002/csc2.20163
Jacobs, J. L., Kelly, J. D., Wright, E. M., Varner, G., and Chilvers, M. I. (2019). Determining the soilborne pathogens associated with root rot disease complex of dry bean in Michigan. Plant Health Prog. 20, 122–127. doi: 10.1094/php-11-18-0076-s
Kamfwa, K., Cichy, K. A., and Kelly, J. D. (2015a). Genome-wide association analysis of symbiotic nitrogen fixation in common bean. Theor. Appl. Genet. 128, 1999–2017. doi: 10.1007/s00122-015-2562-5
Kamfwa, K., Cichy, K. A., and Kelly, J. D. (2015b). Genome-wide association study of agronomic traits in common bean. Plant Genome 8, 1–12. doi: 10.3835/plantgenome2014.09.0059
Keller, B., Ariza-Suarez, D., de la Hoz, J., Aparicio, J. S., Portilla, A. E., Buendia, H. F., et al. (2020). Genomic prediction of agronomic traits in common bean under environmental stress. Front. Plant Sci. 11:1001. doi: 10.3389/fpls.2020.01001
Li, Y. P., You, M. P., Norton, S., and Barbetti, M. J. (2016). Resistance to Pythium irregulare root and hypocotyl disease in diverse common bean (Phaseolus vulgaris) varieties from 37 countries and relationships to waterlogging tolerance and other plant and seed traits. Eur. J. Plant Pathol. 146, 147–176. doi: 10.1007/s10658-016-0901-2
Liu, X., Huang, M., Fan, B., Buckler, E., and Zhang, Z. (2016). Iterative usage of fixed and random effect models for powerful and efficient Genome-Wide Association Studies. PLoS Genet. 12:e1005767. doi: 10.1371/journal.pgen.1005767
Lobaton, J. D., Miller, T., Gil, J., Ariza, D., de la Hoz, J. F., Soler, A., et al. (2018). Resequencing of common bean identifies regions of inter-gene pool introgression and provides comprehensive resources for molecular breeding. Plant Genome 11:170068. doi: 10.3835/plantgenome2017.08.0068
Mahuku, G., Buruchara, R., and Navia, M. (2005). “A gene that confers resistance to Pythium root rot in common bean: Genetic characterization and development of molecular markers [poster],” in Centro Internacional de Agricultura Tropical (CIAT). Cali, 1. Available online at: https://hdl.handle.net/10568/57772 (accessed August 2020).
Mukankusi, C., Derera, J., Melis, R., Gibson, P. T., and Buruchara, R. (2011). Genetic analysis of resistance to Fusarium root rot in common bean. Euphytica 182, 11–23. doi: 10.1007/s10681-011-0413-2
Mukankusi, C. M., Amongi, W., Sebuliba, S., Musoke, S., and Acam, C. (2018). Characterisation of Phaseolus coccineus interspecific germplasm accessions for disease resistance, grain market class and yield attributes. Afr. Crop Sci. J. 26:117. doi: 10.4314/acsj.v26i1.9
Mukankusi, M. C., Melis, R., Derera, J., Laing, M., and Buruchara, R. A. (2010). Identification of sources of resistance to Fusarium root rot among selected common bean lines in Uganda. J. Anim. Plant Sci. 7, 876–891.
Mwang’ombe, A., Thiong’o, G., Olubayo, F., and Kiprop, E. (2007). Occurrence of bean root rot on common bean (Phaseolus vulgaris L.) in association with bean stem maggot (Ophiomyia sp.) in EMBU district, Kenya. Plant Pathol. J. 6, 141–146.
Nakedde, T., Ibarra-Perez, F. J., Mukankusi, C., Waines, J. G., and Kelly, J. D. (2016). Mapping of QTL associated with Fusarium root rot resistance and root architecture traits in black beans. Euphytica 212, 51–63. doi: 10.1007/s10681-016-1755-6
Namayanja, A., Nchimbi Msolla, S., Buruchara, R., and Namusoke, A. (2014). Genetic analysis of resistance to Pythium root rot disease in common bean (Phaseolus vulgaris L.) Genotypes. J. Crop Improv. 28, 184–202. doi: 10.1080/15427528.2013.863815
Naseri, B. (2008). Root rot of common bean in Zanjan, Iran: major pathogens and yield loss estimates. Aust. Plant Pathol. 37, 546–551. doi: 10.1071/AP08053
Navarro, F., Sass, M. E., and Nienhuis, J. (2008). Identification and confirmation of quantitative trait loci for root rot resistance in snap bean. Crop Sci. 48, 962–972.
Nay, M. M., Mukankusi, C. M., Studer, B., and Raatz, B. (2019). Haplotypes at the Phg-2 Locus are determining pathotype-specificity of Angular Leaf Spot resistance in common bean. Front. Plant Sci. 10:1126. doi: 10.3389/fpls.2019.01126
Nzungize, J., Gepts, P., Buruchara, R., Male, A., Ragama, P., and Busogoro, J. P. (2011). Introgression of Pythium root rot resistance gene into Rwandan susceptible common bean cultivars. Plant Sci. 5, 193–200.
Nzungize, J. R., Lyumugabe, F., Busogoro, J.-P., and Baudioin, J.-P. (2012). Pythium root rot of common bean: biology and control methods. A review. Biotechnol. Agron. Soc. Environ. 16, 405–413.
Oladzad, A., Zitnick-anderson, K., Jain, S., Simons, K., and Kelly, J. (2019). Genotypes and genomic regions associated with Rhizoctonia solani resistance in common bean. Front. Plant Sci. 10:956. doi: 10.3389/fpls.2019.00956
Ongom, P. O., Nkalubo, S. T., Gibson, P. T., Mukankusi, C. M., and Rubaihayo, P. R. (2012). Evaluating genetic association between Fusarium and Pythium root rots resistances in the bean genotype RWR 719. Afr. Crop Sci. J. 20, 31–39.
Onziga Dramadri, I., Amongi, W., Kelly, J. D., and Mugisha Mukankusi, C. (2020). Genome-wide association analysis of resistance to Pythium ultimum in common bean (Phaseolus vulgaris). Plant Breed. 139, 1168–1180. doi: 10.1111/pbr.12855
Otsyula, R., Buruchara, R., Mahuku, G., and Rubaihayo, P. (2002). Inheritance and Transfer of Root Rot (Pythium) Resistance to Bean Varieties, Vol. 1. Kampala, UG: Centro Internacional de Agricultura Tropical (CIAT), Pan Africa Bean Research Alliance Kawanda Research Station, 169.
Otsyula, R., Rubaihayo, P., and Buruchara, R. (2003). Inheritance of resistance to Pythium root rot in beans (Phaseolus vulgaris) genotypes. Afr. Crop Sci. Conf. Proc. 6, 295–298.
Paez-Garcia, A., Motes, C., Scheible, W.-R., Chen, R., Blancaflor, E., and Monteros, M. (2015). Root traits and phenotyping strategies for plant improvement. Plants 4, 334–355. doi: 10.3390/plants4020334
Paparu, P., Acur, A., Kato, F., Acam, C., Nakibuule, J., Musoke, S., et al. (2018). Prevalence and incidence of four common bean root rots in Uganda. Exp. Agric. 54, 888–900. doi: 10.1017/S0014479717000461
Pathania, A., Sharma, S. K., and Sharma, P. N. (2014). “Common Bean,” in Broadening the Genetic Base of Grain Legumes, eds M. Singh, B. Singh, and M. Dutta (New Delhi: Springer), 11–50. doi: 10.1007/978-81-322-2023-7
Peña, P. A., Steadman, J. R., Eskridge, K. M., and Urrea, C. A. (2013). Identification of sources of resistance to damping-off and early root/hypocotyl damage from Rhizoctonia solani in common bean (Phaseolus vulgaris L.). Crop Protect. 54, 92–99. doi: 10.1016/j.cropro.2013.04.014
Perea, C., De La Hoz, J. F., Cruz, D. F., Lobaton, J. D., Izquierdo, P., Quintero, J. C., et al. (2016). Bioinformatic analysis of genotype by sequencing (GBS) data with NGSEP. BMC Genom. 17:498. doi: 10.1186/s12864-016-2827-7
Perez, P., and de los Campos, G. (2014). Genome-Wide regression and prediction with the BGLR statistical package. Genetics 198, 483–495.
Pieczarka, D., and Abawi, G. (1978). Effect of interactions between Fusarium, Pythium, and Rhizoctonia on severity of bean root rot. Phytopathology 68, 403–408.
Rice, B., and Lipka, A. E. (2019). Evaluation of RR-BLUP genomic selection models that incorporate peak Genome-Wide Association study signals in maize and sorghum. Plant Genome 12, 1–14. doi: 10.3835/plantgenome2018.07.0052
Rodriguez-Alvarez, M. X., Boer, M., Eilers, P., and van Eeuwijk, F. (2020). SpATS-Package: Spatial Analysis of Field Trials With Splines. R Package Version 1.0–4.
Rojas, A. (2019). Caracterización de las Poblaciones de Hongos y Oomicetos Causantes de Pudriciones Radicales en Fríjol, Phaseolus Vulgaris L. Available online at: https://repositorio.unal.edu.co/handle/unal/69825 (accessed August 2020).
Román-Avilés, B., and Kelly, J. D. (2005). Identification of quantitative trait loci conditioning resistance to Fusarium root rot in common bean. Crop Sci. 45, 1881–1890. doi: 10.2135/cropsci2005.0028
Rusuku, G., Buruchara, R. A., Gatabazi, M., and Pastor-Corrales, M. A. (1997). Occurrence and distribution in Rwanda of soilborne fungi pathogenic to the common bean. Plant Dis. 81, 445–449. doi: 10.1094/PDIS.1997.81.5.445
Schmutz, J., McClean, P. E., Mamidi, S., Wu, G. A., Cannon, S. B., Grimwood, J., et al. (2014). A reference genome for common bean and genome-wide analysis of dual domestications. Nat. Genet. 46, 707–713. doi: 10.1038/ng.3008
Schneider, K. A., Grafton, K. F., and Kelly, J. D. (2001). QTL analysis of resistance to Fusarium root rot in bean. Crop Sci. 41, 535–542. doi: 10.2135/cropsci2001.412535x
Schneider, K. A., and Kelly, J. D. (2000). A greenhouse screening protocol for Fusarium root rot in bean. HortScience 35, 1095–1098. doi: 10.21273/hortsci.35.6.1095
Self, S. G., and Liang, K.-Y. (1987). Asymptotic properties of maximum likelihood estimators and likelihood ratio tests under nonstandard conditions. J. Am. Stat. Assoc. 82, 605–610. doi: 10.2307/2289471
Singh, S. P., and Muñoz, C. G. (1999). Resistance to common bacterial blight among Phaseolus species and common bean improvement. Crop Sci. 39, 80–89. doi: 10.2135/cropsci1999.0011183X003900010013x
Soltani, A., Mafimoghaddam, S., Oladzad-Abbasabadi, A., Walter, K., Kearns, P. J., Vasquez-Guzman, J., et al. (2018). Genetic analysis of flooding tolerance in an andean diversity panel of dry bean (Phaseolus vulgaris L.). Front. Plant Sci. 9:767. doi: 10.3389/fpls.2018.00767
Tello, D., Gil, J., Loaiza, C. D., Riascos, J. J., Cardozo, N., Duitama, J., et al. (2019). NGSEP3: Accurate variant calling across species and sequencing protocols. Bioinformatics 35, 4716–4723. doi: 10.1093/bioinformatics/btz275
Tock, A. J., Fourie, D., Walley, P. G., Holub, E. B., Soler, A., Cichy, K. A., et al. (2017). Genome-wide linkage and association mapping of halo blight resistance in common bean to race 6 of the globally important bacterial pathogen. Front. Plant Sci. 8:1170. doi: 10.3389/fpls.2017.01170
van Schoonhoven, A., and Pastor-Corrales, M. A. (1987). Standard System for the Evaluation of Bean Germplasm. Cali, CO: CIAT.
Van West, P., Appiah, A. A., and Gow, N. A. R. (2003). Advances in research on oomycete root pathogens. Physiol. Mol. Plant Pathol. 62, 99–113. doi: 10.1016/S0885-5765(03)00044-4
Wang, J., Chapman, S. C., Bonnett, D. G., and Rebetzke, G. J. (2009). Simultaneous selection of major and minor genes: use of QTL to increase selection efficiency of coleoptile length of wheat (Triticum aestivum L.). Theor. Appl. Genet. 119, 65–74. doi: 10.1007/s00122-009-1017-2
Wang, W., Jacobs, J. L., Chilvers, M. I., Mukankusi, C. M., Kelly, J. D., and Cichy, K. A. (2018). QTL analysis of fusarium root rot resistance in an andean × middle American common bean RIL population. Crop Sci. 58, 1166–1180. doi: 10.2135/cropsci2017.10.0608
Wen, L., Chang, H. X., Brown, P. J., Domier, L. L., and Hartman, G. L. (2019). Genome-wide association and genomic prediction identifies soybean cyst nematode resistance in common bean including a syntenic region to soybean Rhg1 locus. Hortic. Res. 6, 1–12. doi: 10.1038/s41438-018-0085-3
Zhang, Y. W., Tamba, C. L., Wen, Y. J., Li, P., and Ren, W. (2020). mrMLM v4.0: An R platform for multi-locus genome-wide association studies. Genomics, Proteom. Bioinform. (in press). doi: 10.1016/j.gpb.2020.06.006
Keywords: genotyping-by-sequencing (GBS), genome-wide association study (GWAS), genomic prediction (GP), root rot disease complex, Phaseolus vulgaris L., Pythium spp.
Citation: Diaz LM, Arredondo V, Ariza-Suarez D, Aparicio J, Buendia HF, Cajiao C, Mosquera G, Beebe SE, Mukankusi CM and Raatz B (2021) Genetic Analyses and Genomic Predictions of Root Rot Resistance in Common Bean Across Trials and Populations. Front. Plant Sci. 12:629221. doi: 10.3389/fpls.2021.629221
Received: 13 November 2020; Accepted: 16 February 2021;
Published: 12 March 2021.
Edited by:
Roberto Papa, Marche Polytechnic University, ItalyReviewed by:
Yong Suk Chung, Jeju National University, South KoreaHongyan Liu, Shanghai Agrobiological Gene Center, China
Copyright © 2021 Diaz, Arredondo, Ariza-Suarez, Aparicio, Buendia, Cajiao, Mosquera, Beebe, Mukankusi and Raatz. This is an open-access article distributed under the terms of the Creative Commons Attribution License (CC BY). The use, distribution or reproduction in other forums is permitted, provided the original author(s) and the copyright owner(s) are credited and that the original publication in this journal is cited, in accordance with accepted academic practice. No use, distribution or reproduction is permitted which does not comply with these terms.
*Correspondence: Lucy Milena Diaz, l.m.diaz@cgiar.org; Bodo Raatz, bodo81@hotmail.com