- National Engineering Laboratory for Crop Molecular Breeding, National Center of Space Mutagenesis for Crop Improvement, Institute of Crop Sciences, Chinese Academy of Agricultural Sciences, Beijing, China
Agronomic traits such as heading date (HD), plant height (PH), thousand grain weight (TGW), and spike length (SL) are important factors affecting wheat yield. In this study, we constructed a high-density genetic linkage map using the Wheat55K SNP Array to map quantitative trait loci (QTLs) for these traits in 207 recombinant inbred lines (RILs). A total of 37 QTLs were identified, including 9 QTLs for HD, 7 QTLs for PH, 12 QTLs for TGW, and 9 QTLs for SL, which explained 3.0–48.8% of the phenotypic variation. Kompetitive Allele Specific PCR (KASP) markers were developed based on sequencing data and used for validation of the stably detected QTLs on chromosomes 3A, 4B and 6A using 400 RILs. A QTL cluster on chromosome 4B for PH and TGW was delimited to a 0.8 Mb physical interval explaining 12.2–22.8% of the phenotypic variation. Gene annotations and analyses of SNP effects suggested that a gene encoding protein Photosynthesis Affected Mutant 68, which is essential for photosystem II assembly, is a candidate gene affecting PH and TGW. In addition, the QTL for HD on chromosome 3A was narrowed down to a 2.5 Mb interval, and a gene encoding an R3H domain-containing protein was speculated to be the causal gene influencing HD. The linked KASP markers developed in this study will be useful for marker-assisted selection in wheat breeding, and the candidate genes provide new insight into genetic study for those traits in wheat.
Introduction
Wheat (Triticum aestivum L.) is one of the most important cereal crops worldwide, providing a food source for 30% of the human population (Mayer et al., 2014). Improving the yield potential of wheat is of great significance for meeting the food demand from an increasing population (Tshikunde et al., 2019). Agronomic traits such as heading date (HD), plant height (PH), thousand grain weight (TGW), and spike length (SL) are important factors affecting yield and always targeted by wheat breeders (Tshikunde et al., 2019). Recent advances in wheat genomics have accelerated the genetic dissection of important agronomic traits, and a large number of quantitative trait loci (QTLs) for these traits have been identified (Rasheed and Xia, 2019).
Heading date is crucial for adaptation to different environments and yield stability in wheat (Snape et al., 2001). Over a hundred QTLs for HD located across all wheat chromosomes have been detected (Milec et al., 2014; Kiseleva and Salina, 2018). The cloned genes affecting HD or flowering in wheat are mainly classified into three groups: vernalization (VRN), photoperiod (Ppd), and earliness per se (Eps) genes (Snape et al., 2001). Four VRN genes (VRN1, VRN2, VRN3, and VRN4) located on chromosome 5 or 7 of the A/B/D genomes, have been identified by map-based cloning (Yan et al., 2003, 2004, 2006; Kippes et al., 2015; Xie et al., 2019). The Ppd genes for photoperiod responses in wheat are mainly located on chromosomes 2A, 2B, and 2D (Beales et al., 2007). The Eps genes were identified on chromosome 1Am in Triticum monococcum (Alvarez et al., 2016) and on long arm of chromosome 1D in hexaploid wheat (Zikhali et al., 2014).
Plant height is another important factor affecting yield potential in wheat (Flintham et al., 1997). Twenty-five reduced height genes (Rht), Rht1 to Rht25, have been identified in wheat (Mo et al., 2018). According to the distinct responses to exogenous gibberellic acid (GA), these Rht genes were classified into GA-sensitive or GA-insensitive categories (Lou et al., 2016). The “green revolution” genes Rht-B1b (Rht1) and Rht-D1b (Rht2) located on chromosome 4B and 4D, respectively, encode truncated DELLA proteins, which are involved in the gibberellin signaling pathway (Peng et al., 1999). Rht4, Rht5, Rht7, Rht8, Rht9, Rht12, Rht13, Rht22, and Rht23 are located on 2B, 3B, 2A, 2D, 7B, 5A, 7B, 7A, and 5D, respectively (Peng et al., 1999; Ellis et al., 2005; Asplund et al., 2012; Chen et al., 2015; Vikhe et al., 2017). Rht24 is located on 6AL (Tian et al., 2017; Wurschum et al., 2017) while Rht14, Rht16, Rht18, and Rht25 are located on 6AS (Haque et al., 2011; Grant et al., 2018; Mo et al., 2018).
TGW is one of the three essential components of grain yield. Most of the cloned genes associated with TGW in wheat were identified using a homology-based strategy (Chen et al., 2020). The wheat TaGL3-5A gene has been cloned, and a SNP in the 11th exon of TaGL3-5A is associated with variation in grain length and TGW (Yang et al., 2019). In addition, the TaGW2 gene in wheat is well studied for its function in regulating grain weight (Su et al., 2011; Bednarek et al., 2012; Jaiswal et al., 2015; Simmonds et al., 2016; Zhai et al., 2018; Zhang et al., 2018). Through genetic linkage analyses, stable QTLs explaining over 10% of the phenotypic variance for TGW were identified on chromosomes 1A (Varshney et al., 2000), 1B (Mir et al., 2012), 2D (Ma et al., 2019), 3A (Cui et al., 2014a), 3D (Cui et al., 2014a; Kumar et al., 2016), 4A (Araki et al., 1999), 4B (Kumar et al., 2016; Guan et al., 2018; Xu et al., 2019; Chen et al., 2020), 5A (Börner et al., 2002; Cuthbert et al., 2008; Mir et al., 2012; Kumar et al., 2016), 5B (Yang et al., 2020), 5D (Li et al., 2018), 6A (Mir et al., 2012), 6D (Cui et al., 2014a), 7A (Kumar et al., 2006, 2016; Mir et al., 2012), and 7D (Chen et al., 2020).
Spike architecture traits such as spike length (SL) are tightly related to grain production in wheat (Yao et al., 2019). A number of studies have identified stable QTLs for SL on chromosomes 1A, 1B, 2D, 3A, 3B, 4A, 4B, 5A, 5B, 6A, 6B, 6D, 7A, 7B, and 7D (Li et al., 2002, 2018; Marza et al., 2006; Deng et al., 2011; Cui et al., 2012a; Liu et al., 2018a; Cao et al., 2019; Chai et al., 2019; Wolde et al., 2019; Yao et al., 2019; Hu et al., 2020). It has been reported that the Q gene on chromosome 5A, which encodes an AP2 transcription factor, affects SL in wheat (Kawaura et al., 2009).
To obtain the genetic basis for HD, PH, TGW, and SL, we conducted QTL mapping based on a RIL population in the present study. In our previous study we used Bulked Segregant Analysis (BSA) and identified VRN-B1 as the gene responsible for HD variation in the RIL population (Li et al., 2020). In this study, we used the Wheat55K SNP Array to map QTLs for HD, PH, TGW, and SL in this RIL population. Moreover, we validated the major QTLs on chromosomes 3A, 4B, and 6A by developing Kompetitive Allele Specific PCR (KASP) markers based on sequencing data and predicted candidate genes for PH, TGW, and HD according to gene annotation and SNP effects analysis.
Materials and Methods
Plant Materials and Phenotype Evaluation
As previously described (Li et al., 2020), a RIL population (400 lines) derived from a cross between an early heading mutant (eh1) and Lunxuan987 (LX987) was used for genetic mapping; generations F6 to F8 of the RIL population were included in this study. The RIL and parent lines were planted at the Zhongpuchang field station of the Institute of Crop Sciences, Chinese Academy of Agricultural Sciences (Beijing, China) during the 2015–2016, 2016–2017, and 2017–2018 cropping seasons. For each year, the experiment was conducted once and we selected three representative plants for phenotypic collection. A total of 15 plants for each line were planted in a row of 1 m, and the field conditions were managed according to local standard practices.
For HD, when more than half of the spikes had emerged from two thirds of the plants in a line, the date for that line was recorded (Li et al., 2020). At agronomic harvest maturity, three representative plants from the middle of each row showing uniform growth status were used for PH, TGW, and SL evaluation and the mean values from these three plants were used for QTL mapping. PH from each representative plant was measured from the ground to the tip of the spike excluding awns. After drying, the grain weight from each representative plant was measured and the number of grains was counted. TGW was calculated as the plant grain weight divided by the number of grains per plant multiplied by 1,000. SL from main stem of each representative plant was measured from the base of the rachis to the tip of the terminal spikelet excluding awns. The HD, PH, and TGW data were collected in 2016, 2017, and 2018, and the SL data were collected in 2016 and 2018. Analyses of variance, correlation coefficients, and broad sense heritability were performed using the ANOVA analysis tools of the QTL IciMapping v4.1 program1.
Genotyping
Genomic DNA of each RIL and parent line was extracted as previously described (Li et al., 2020). After assessment of DNA integrity and quantity, the DNA from 207 lines that were also used for KASP assay, along with the parent DNA samples were hybridized to the Wheat55K SNP Array containing 53,063 markers. The genotyping was performed by China Golden Marker (Beijing) Biotech Co. Ltd2.
Genetic Map Construction and QTL Analysis
High quality genotyping data were obtained by filtering with a Dish QC threshold of >0.82 and a Call-Rate threshold of >95%. The BIN function of IciMapping 4.1 was used to remove redundant markers from poly-high-resolution (PHR) SNPs, and the SNPs with >25% missing data were filtered out. The genetic map was constructed by randomly selecting only one marker from each bin using the MAP function of IciMapping 4.1. The threshold of the logarithm of odds (LOD) score was set to 2.5, and the Kosambi map function was used to calculate the map distance from recombination frequencies. Composite interval mapping (ICIM) in IciMapping 4.1 was selected to identify QTLs for HD, PH, TGW, and SL. The mean values of phenotypic traits for each line in each cropping season were used for QTL analysis. QTL region was determined by the positions of left and right markers identified by IciMapping 4.1, and physical positions of markers on the wheat reference genome v1.0 are shown in Supplementary Table 1. QTLs for the same traits identified in 2 or 3 years were considered to be stable. Multi-Environment Traits (MET) analysis of QTL IciMapping v4.1 was used for assessment of QTL × environment interactions (Li et al., 2015).
Development of KASP Markers and QTL Validation
According to the SNPs between eh1 and LX987 identified by RNA sequencing (RNA-seq) (Li et al., 2020), KASP markers around or in the region of stable QTLs specific for different subgenomes were designed using the polyploid primer design pipeline PolyMarker3. After evaluation of the polymorphisms between two parent lines, the developed KASP markers were used for genotyping the entire mapping population. The successfully developed KASP markers are listed in Supplementary Table 2. A total of 400 RILs were genotyped with KASP markers on chromosomes 3A, 4B, and 6A. The reaction volume and PCR procedures for the KASP assay were as previously described (Li et al., 2020), and the CFX 96 Real-Time System (Bio Rad, Hercules, CA, United States) was used for PCR and data analysis. QTL analysis was conducted using IciMapping 4.1.
Analysis of SNP Effects and Prediction of Candidate Genes
Based on RNA-seq data, which was collected from young spikes of eh1 and LX987 when eh1 was beginning to head (Li et al., 2020), the SNPs between eh1 and LX987 covering the intervals of flanking markers from QTL validation were obtained for SNP effects analysis. SNP effects were analyzed by Python4 according to the example and scripts from the website5. A score for missense variation is generated that reflects the predicted effect of the SNP on gene function. The more negative a score, the larger the effects on gene function. The SNPs with larger effects on gene function were speculated to be located in the candidate genes. Gene functions were predicted by searching for homologous genes in rice (Oryza sativa) and Arabidopsis thaliana using the Triticeae Multi-omics Center website6.
Results
Phenotypic Variation in the RIL Population
Our previous study showed that there is variation in HD in a RIL population of 400 lines derived from a cross between the early heading mutant eh1 and LX987 (Li et al., 2020). In addition to HD, we also found that PH, TGW, and SL differed between eh1 and LX987; the values of these traits were significantly lower in eh1 than in LX987 from 2016–2018 (Table 1). Therefore, phenotypic investigation of PH, TGW, and SL in the RIL population was also conducted from 2016–2018. In the RIL population, the percent variation in PH, TGW, and SL ranged from 9.1% to 14.4% from 2016–2018, and all three traits showed moderate h2 values ranging from 0.77 to 0.82 (Table 1). In addition, PH, TGW, and SL from 2016–2018 followed a normal distribution and strong transgressive segregation was observed in the RIL population (Figure 1). Analysis of variance of PH, TGW, and SL for the multiple environment trials in the RIL population indicated that these traits were affected by environmental conditions (Supplementary Table 3).
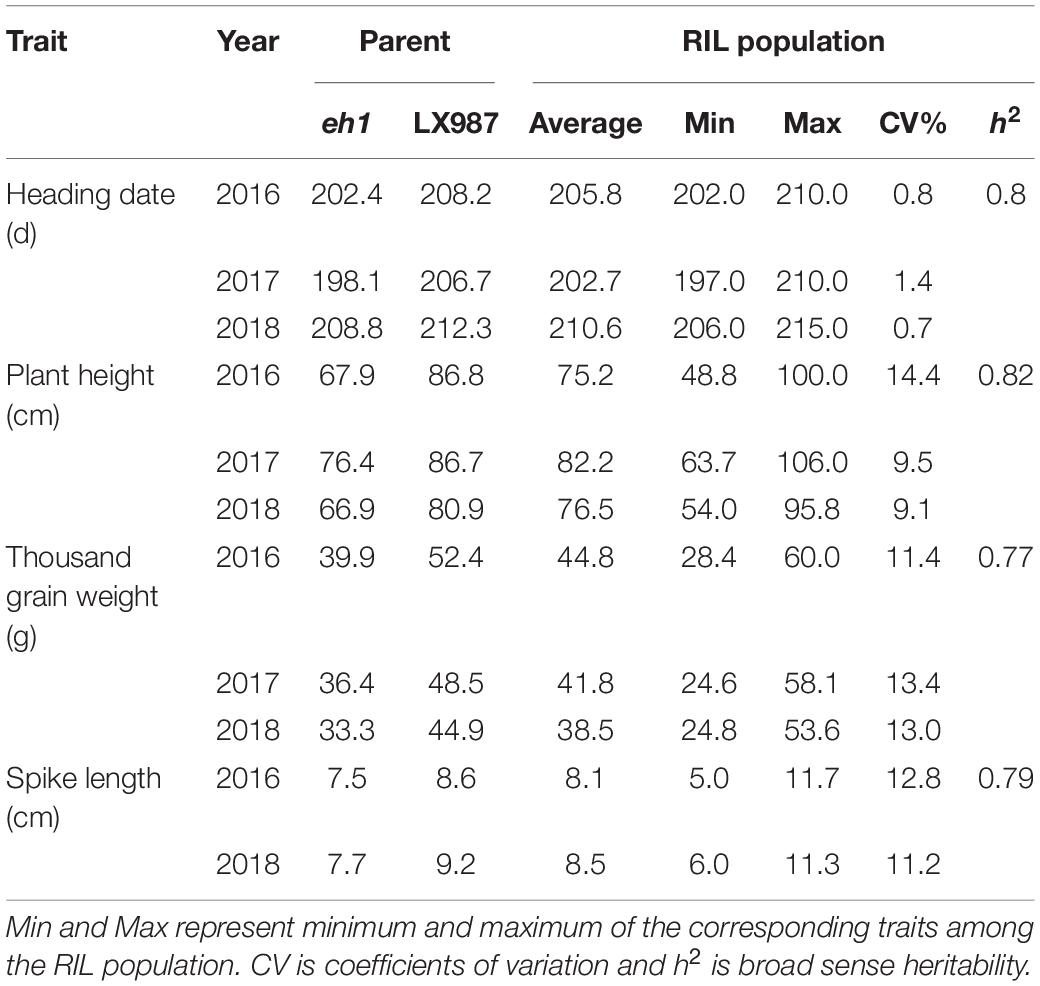
Table 1. Summary statistics for heading date, plant height, thousand grain weight, and spike length for the two parents and the RIL population in 2016–2018.
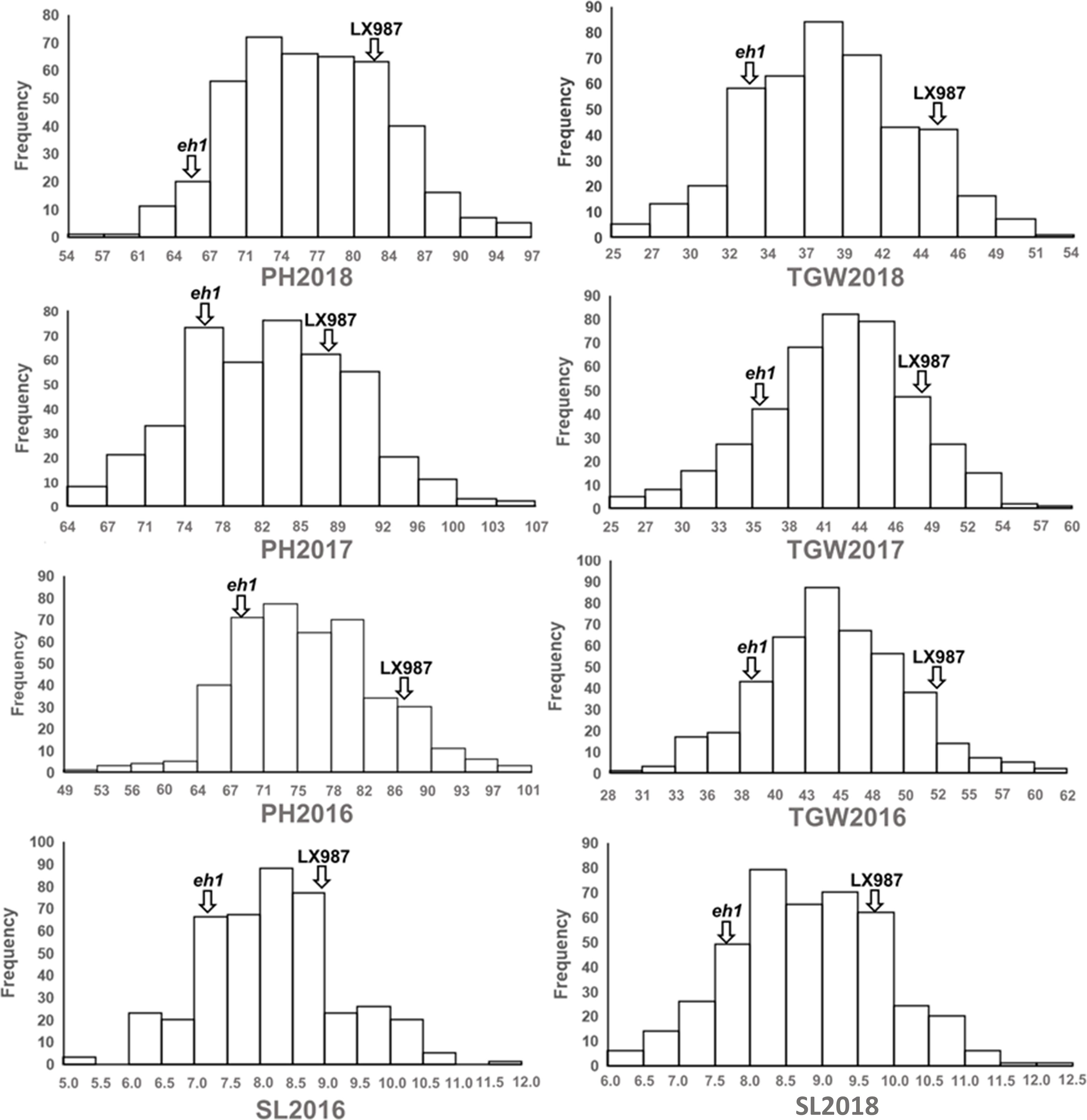
Figure 1. Phenotypic of distribution of plant height (PH), thousand grain weight (TGW), and spike length (SL) in the RIL population from 2016 to 2018. Phenotypic values of the two parents were marked by vertical arrows.
Analysis of the pairwise correlations between HD, PH, TGW, and SL suggested that TGW and SL were significantly positively correlated with PH while SL was significantly negatively correlated with HD (Table 2). However, the correlations between SL and PH, SL and HD were weak (Table 2).
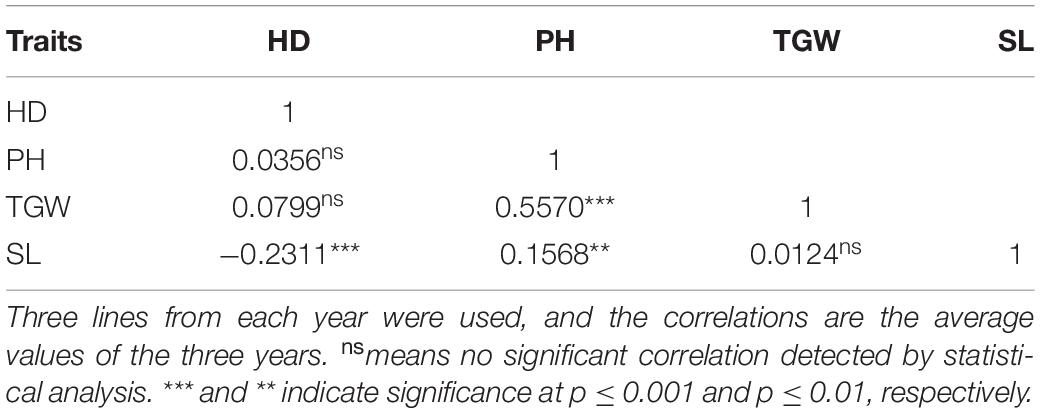
Table 2. Correlation coefficient analyses among heading date (HD), plant height (PH), thousand grain weight (TGW), and spike length (SL) in the RIL population.
Genetic Map Construction
Among the 400 RILs, 207 lines were randomly selected for genotyping using a Wheat55K SNP Array with 53,063 tags selected from the Wheat660K SNP Array (Ren et al., 2018). Since PHR SNPs are recommended for polyploid species and have the highest reliability, only PHR SNP probes were kept. SNPs with the same genotype in both parents were removed. Finally, 6505 SNP markers were obtained for genetic map construction (Table 3). These markers were divided into 1097 unique loci with the number distributed on each chromosome ranging from 10 to 96 (Table 3). The genetic map spanned 3496.1 cM in length with an average density of 5.2 cM/locus (Table 3).
QTL Mapping Analysis
A total of 37 QTLs for HD, PH, and TGW from 2016–2018, and SL from 2016 and 2018, were identified by QTL mapping analysis (Table 4 and Figure 2). These QTLs with LOD values ranging from 2.8 to 38.9 were distributed on 15 chromosomes and explained 3.0–48.8% of the phenotypic variation (Table 4 and Figure 2). There were 9, 7, 12, and 9 QTLs detected for HD, PH, TGW, and SL, respectively (Table 4 and Figure 2).
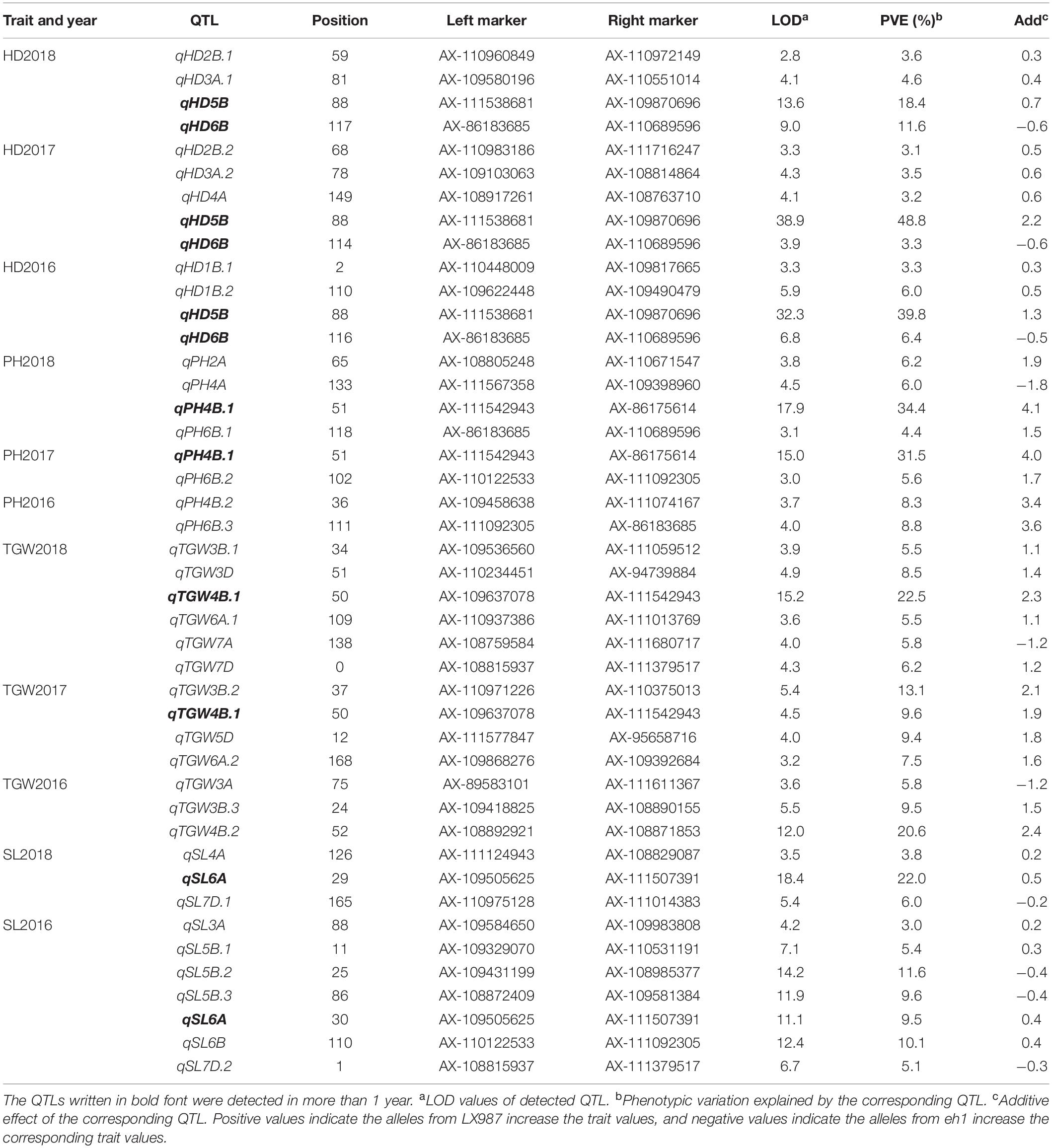
Table 4. QTLs for heading date (HD), plant height (PH), thousand grain weight (TGW), and spike length (SL) in 2016, 2017, and 2018 identified by IciMapping 4.1.
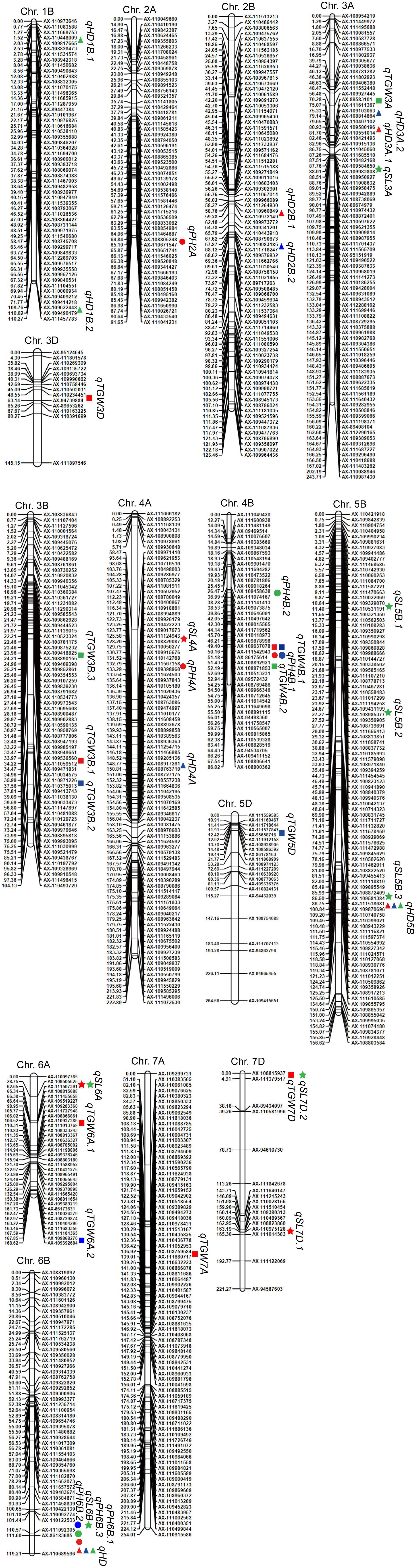
Figure 2. Chromosomal locations of the identified QTLs for HD, PH, TGW, and SL in 2016, 2017, and 2018. Triangles, circles, squares, and stars represent HD, PH, TGW, and SL, respectively. The colors green, blue, and red indicate data from 2016, 2017, and 2018, respectively.
QTLs for HD were detected on chromosomes 1B (2), 2B (2), 3A (2), 4A (1), 5B (1), and 6B (1) (Table 4 and Figure 2). Notably, qHD5B and qHD6B were detected in all 3 years. qHD5B explained 18.4–48.8% of the phenotypic variation while qHD6B accounted for 3.3–11.6% of the phenotypic variation (Table 4). qHD3A.1 was located close to qHD3A.2, and qHD2B.1 was located close to qHD2B.2. qHD2B.1 was detected in 2018 and explained 3.6% of the phenotypic variation, and qHD2B.2 was detected in 2017 and explained 3.1% of the phenotypic variation (Table 4). For all of the QTLs except qHD6B the allele increasing HD was contributed by LX987 (Table 4).
For PH, 7 QTLs were identified on chromosomes 2A (1), 4A (1), 4B (2), and 6B (3) (Table 4 and Figure 2). qPH4B.1 was identified in 2017 and 2018 (Table 4 and Figure 2), with LOD scores of 15.0 and 17.9 and explaining 31.5% and 34.4% of the phenotypic variation, respectively (Table 4). Three co-located QTLs were identified on chromosome 6B from 2016–2018, which explained 4.4%–8.8% of the variation in PH (Table 4 and Figure 2). For all of the QTLs except qPH4A the allele increasing PH was contributed by LX987 (Table 4).
For TGW, 12 QTLs were detected on chromosomes 3A (1), 3B (3), 3D (1), 4B (2), 5D (1), 6A (2), 7A (1), and 7D (1) (Table 4 and Figure 2). qTGW4B.1 was detected in 2017 and 2018, explaining 9.6% and 22.5% of the variation in TGW, respectively (Table 4). qTGW4B.2, which was located close to qTGW4B.1, was detected in 2016 and explained 20.6% of the phenotypic variation (Table 4). In addition, the QTLs qTGW3B.1 and qTGW3B.2 were located close to each other and explained 5.5% and 13.1% of phenotypic variation in 2018 and 2017, respectively (Table 4). For all of the QTLs except qTGW7A and qTGW3A, the allele increasing TGW was contributed by LX987 (Table 4).
For SL, 9 QTLs were identified on chromosomes 3A (1), 4A (1), 5B (3), 6A (1), 6B (1), and 7D (2). qSL6A was detected in 2016 and 2018, explaining 9.5% and 22.0% of phenotypic variation, respectively (Table 4). qSL5B.2 and qSL5B.3 showed high contributions to phenotypic variation, 11.6% and 9.6%, respectively (Table 4). For qSL7D.1, qSL5B.2, qSL5B.3, and qSL7D.2, the negative alleles were contributed by LX987 while for the other QTLs the allele increasing SL was contributed by LX987 (Table 4).
Four QTL clusters were identified on chromosomes 3A, 4B, 5B, and 6B (Table 5). For the QTL cluster on chromosome 3A, qHD3A.1 and qHD3A.2 were co-localized with qTGW3A and qSL3A in a region ranging from 70.28 cM to 88.01 cM. On chromosome 4B, qPH4B.1 for PH was clustered with two QTLs for TGW, with the alleles from LX987 increasing PH and TGW. For the QTL cluster on chromosome 5B, qHD5B, which was detected in all 3 years, was clustered with qSL5B.3 (Tables 4, 5); however, the positive alleles for these QTLs were derived from opposite parents (Table 4). Three QTLs for PH on chromosome 6B were clustered with qHD6B and qSL6B, with the alleles from LX987 increasing PH and SL (Tables 4, 5).

Table 5. QTL clusters affecting two or more traits. QTLs from each year located within 10 cM and affected two or more traits were identified as a QTL cluster.
To evaluate the QTL × environment interactions, Multi-Environment Traits (MET) analysis was employed by using QTL IciMapping v4.1 (Li et al., 2015). Similarly, 33 QTLs were identified by MET analysis (Supplementary Table 4). Among them, 10 QTLs showed significant interactions with environment, including the major QTLs qHD5B, qPH4B.1, and qTGW4B.1.
QTL Validation by Mapping With Molecular Markers
The QTLs on chromosomes 3A (qHD3A), 4B (qPH4B.1 and qTGW4B.1), 5B (qHD5B), and 6A (qSL6A) were stably detected in different years. We selected these QTLs for validation using KASP markers developed based on RNA-seq data (Li et al., 2020). In a recent study we reported that the VRN-B1 gene located on chromosome 5B around the qHD5B region was responsible for HD variation in the RIL population (Li et al., 2020). For the validation of qHD3A, we successfully developed seven KASP markers around or in the 55K SNP array-mapped region, and delimited the QTL to a genetic interval of 1.29 cM between markers 3A128b and 3A16, spanning approximately 2.5 Mb (Figure 3A). The LOD scores of this QTL were 5.7 and 7.5, explaining 6.0% and 8.0% of the variation of HD, in 2017 and 2018, respectively (Table 6). For the QTLs on chromosome 4B, nine KASP markers were successfully developed, and the QTLs for PH were narrowed down to a genetic interval of 1.11 cM flanked by markers 4B271b and 4B288b, corresponding to an approximately 0.8 Mb physical region (Figure 3B). This QTL was detected in 2017 and 2018, with LOD scores of 22.1 and 19.8, and explaining 22.8% and 20.5% of the variation in PH, respectively (Table 6). Consistent with this, the QTL for TGW identified in 2016 and 2018 was mapped between markers 4B271b and 4B288b with LOD scores of 11.1 and 16.8 and explaining 12.2% and 17.8% of the variation in TGW, respectively (Figure 3B and Table 6). For the validation of qSL6A, we successfully developed 10 KASP markers for genetic mapping. In 2016 and 2018, a major QTL for SL with LOD scores of 12.6 and 23.5 and explaining 13.1% and 22.5% of phenotypic variation, respectively, was detected between markers 6A51 and 6A419 within a genetic interval of 31.8 cM (Figure 3C and Table 6). Due to the large region for this QTL, we did not conduct further analysis of the candidate genes.
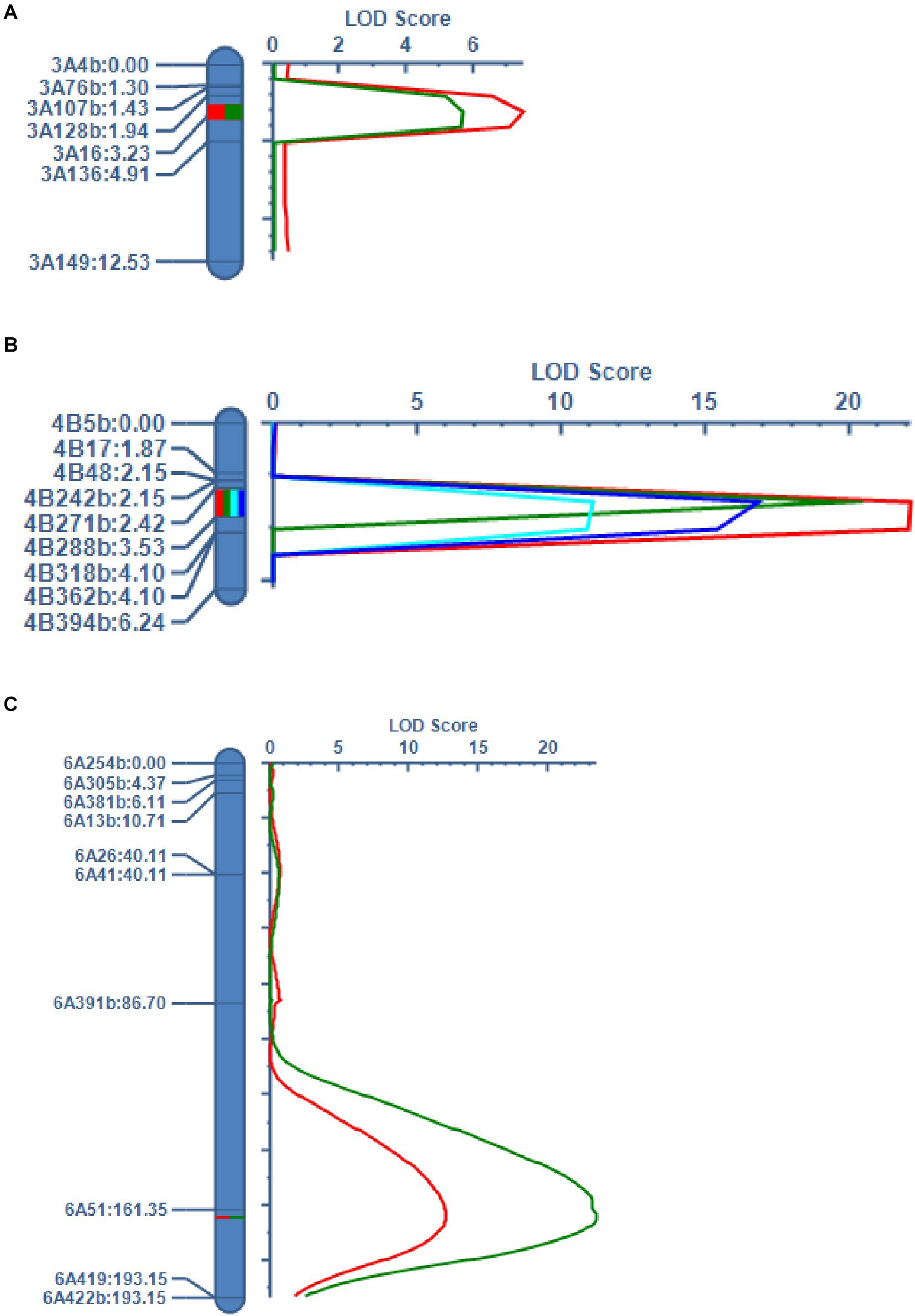
Figure 3. QTL validation using KASP markers developed based on RNA-seq data. (A) QTLs for HD on chromosome 3A. The green and red curves represent LOD scores from 2017 and 2018, respectively. (B) QTLs for PH and TGW on chromosome 4B. The red and green curves represent LOD scores for PH from 2017 and 2018, respectively. The light blue and blue curves represent LOD scores for TGW from 2016 and 2018, respectively. (C) QTLs for SL on chromosome 6A. The red and green curves represent LOD scores from 2016 and 2018, respectively. The marker name and genetic position of each marker are indicated on the left side of each chromosome.
Gene Annotations and Effects of SNPS in the Validated QTL Regions on Chromosome 4B and 3A
Since qPH4B.1 and qTGW4B.1 were delimited to a physical interval of 0.8 Mb between markers 4B271b and 4B288b by genetic mapping with KASP markers, we analyzed gene models and annotations in this region according to the Chinese Spring (CS) reference genome v1.0 (International Wheat Genome Sequencing Consortium, 2018). In this region, seven high-confidence genes were annotated. Based on BLASTP searches for rice and Arabidopsis homologous genes7, these genes are predicted to encode 40S ribosomal protein S27 (TraesCS4B01G280800), Beta-galactosidase (TraesCS4B01G280900), a Histidine-containing phosphotransfer protein (TraesCS4B01G281000), 60S ribosomal protein L5 (TraesCS4B01G281100), Protein PAM68 (TraesCS4B01G281200), Tribbles homolog 3 (TraesCS4B01G281300), and a Ubiquitin carboxyl-terminal hydrolase family protein (TraesCS4B01G281400) (Supplementary Table 5). We also analyzed sequence variation between LX987 and eh1 in this region based on RNA-seq data. A total of 18 SNPs with each parent homozygous for different alleles were identified (Supplementary Table 6). Analysis of SNP effects suggested that three SNPs were missense mutations. One SNP in TraesCS4B02G281200 located at the 189th position caused a change in the amino acid Leu in LX987 to Trp in eh1 and was predicted to have the largest effect on gene function. Multiple alignment of amino acid sequences of protein PAM68 from grasses indicated that this region is conserved among Brachypodium distachyon, Sorghum bicolor, Zea mays and rice (Supplementary Figure 2).
For the HD QTL on chromosome 3A between markers 3A128b and 3A16, we found that 38 high-confidence genes were annotated in the mapped interval (Supplementary Table 7). In this region, nine homozygous SNPs with genotypes differing between the two parent lines were found based on RNA-seq data. One SNP in TraesCS3A01G086400, which encodes an R3H domain-containing protein, that caused a change from Ser to Pro at the 267th position had the largest effect on gene function (Supplementary Table 8).
Discussion
QTL Mapping Using the WHEAT55K SNP Array
SNP arrays are a powerful and effective approach for QTL mapping (Rasheed et al., 2017). The tags of the Wheat55K Array (Affymetrix® Axiom® Wheat55) were carefully selected from the Wheat660K Array, and all tags were uniformly distributed on 21 chromosomes. Therefore, the 55K Array is suitable for genotyping in QTL studies (Ren et al., 2018). The Wheat55K SNP Array has been utilized for QTL mapping of productive tiller number (Liu et al., 2018b), temporal expression of tiller number (Ren et al., 2018), and leaf rust and stripe rust resistance (Huang et al., 2019; Zhang et al., 2019) in wheat. In this study, we used the Wheat55K SNP Array to genotype 207 RILs and constructed a genetic map containing 6,505 PHR SNP markers (Table 3). PHR SNPs are of high quality and possess better cluster resolution than other SNPs (Marrano et al., 2019), which improves the accuracy of genotyping. The genetic map spanned 3496.1 cM across the 21 chromosomes, which is similar to the total length of genetic maps for 199 wheat RILs constructed by Liu et al. (2018b) and 186 RILs constructed by Huang et al. (2019). We detected a total of 37 QTLs for HD, PH, TGW, and SL by mapping using the 55K SNP array (Table 4 and Figure 2). Among these QTLs, those on chromosomes 3A (qHD3A), 4B (qPH4B.1 and qTGW4B.1), 5B (qHD5B) (Li et al., 2020), and 6A (qSL6A) that were stably detected in different years, were validated using KASP markers (Figure 3). High LOD values ranging from 5.7 to 23.5 were observed for the QTLs that were validated with KASP markers (Table 6), indicating that the QTLs detected using the 55K SNP array data are reliable.
Comparison of the Mapped QTLS With Those Identified in Previous Studies
A total of nine QTLs for HD were mapped on chromosomes 1B, 2B, 3A, 4A, 5B, and 6B (Table 4 and Figure 2). Consistent with these findings, in our previous study we also identified QTLs for HD on chromosomes 2B, 3A, and 5B using BSA of the same RIL population (Li et al., 2020). In addition, qHD1B.1 and qHD4A were mapped to genetic regions similar to those reported by Zhao et al. (2019). qHD2B.2 was mapped to a genetic position similar to that of HD QTLs reported by Hu et al. (2020) and Li et al. (2018). The analysis of the physical positions of the flanking markers in the wheat reference genome indicated that qHD2B.2 is probably the Ppd-B1 gene. The two adjacent QTLs qHD3A.1 and qHD3A.2 are located at a genetic position similar to that of an HD QTL reported by Li et al. (2018). The QTL qHD5B, which was stably detected in different years (Table 4 and Figure 2), is located around gene VRN-B1, and our previous results suggested that the VRN-B1 gene is responsible for HD variation in this RIL population (Li et al., 2020). In addition, the stably detected QTL qHD6B was found at a position similar to that of HD QTLs reported by Perez-Lara et al. (2016) and Li et al. (2018).
Regarding PH, we identified two and three QTLs on chromosomes 4B and 6B, respectively (Table 4 and Figure 2). Consistent with these results, previous studies also reported several PH QTLs on chromosomes 4B and 6B (Gao et al., 2016; Li et al., 2018; Jahani et al., 2019). qPH4B.2, qPH6B.1, qPH6B.2, and qPH6B.3 were mapped to similar genetic positions on chromosomes 4B and 6B as PH QTLs reported by Gao et al. (2015); Li et al. (2018), and Hu et al. (2020). BLAST searches of flanking markers for qPH4B.2 against the wheat reference genome indicated that this region harbors the reported RhtB1b gene (Peng et al., 1999). However, using KASP markers for Rht1 (Rasheed et al., 2016) we found that both of the parent lines, eh1 and LX987, had the same RhtB1b genotype (Supplementary Figure 1), indicating that the detection of qPH4B.2 is not due to RhtB1b. It is possible that there is other variation in the Rht1 gene that causes differences in PH between the parent lines. qPH2A is located at a similar genetic position as a QTL reported by Li et al. (2018), and the qPH4A region overlaps with the physical region reported by Chen et al. (2020). It has been reported that the semi-dominant dwarfing gene Rht-NM9 is located in a region from 178.9 Mb to 187.2 Mb on 2AS (Lu et al., 2015). We performed a BLAST search using the flanking markers for qPH2A and found that this QTL is located in a 143–148 Mb interval according to the CS reference genome. Therefore, we speculate that qPH2A does not harbor the Rht-NM9 gene.
The stably detected QTL qTGW4B.1 co-localized with qTGW4B.2 (Table 4 and Figure 2). These QTLs are located within 49.4–52.19 cM. Guan et al. (2018) reported stable QTLs for TGW located within 22.3–95.8 cM on chromosome 4B. qTGW3A is located at a genetic position similar to that reported in Cui et al. (2014a). qTGW3D is located at positions similar to those reported in Cui et al. (2014b) and Gao et al. (2015). The QTLs qTGW3B.1, qTGW3B.2, qTGW3B.3, qTGW5D, and qTGW7A are located at positions similar to those reported by Li et al. (2018), and qTGW6A.1 is located close to a stable yield and TGW QTL reported by Simmonds et al. (2014). In addition, the QTLs qTGW7A and qTGW7D are located at similar genetic positions as those reported by Cui et al. (2014a) and Guan et al. (2018), respectively.
qSL4A is located at a genetic position similar to that reported by Cui et al. (2012b) and Gao et al. (2015). qSL5B.2 is located at a position similar to that in Cui et al. (2012b). In addition, qSL6B is located at a genetic position similar to that reported by Li et al. (2018) and Hu et al. (2020). To the best of our knowledge, the stably detected QTL qSL6A with a LOD value ranging from 11.1 to 18.4 is likely to be a new QTL (Table 4 and Figure 2).
Pleiotropic QTLS for HD, PH, TGW, and SL
Among the QTLs for HD, PH, TGW, and SL detected in this study, four regions controlled two or more of these traits (Table 5). In addition to Rht1, a previous study identified a “QTL-hotspot” region for yield-related traits on chromosome 4B (Guan et al., 2018). This is consistent with the QTL cluster detected in our study (Table 5). Consistent with the positive correlation between PH and TGW (Table 2 and Supplementary Table 9), the superior alleles of the co-localized QTLs qPH4B.1, qTGW4B.1, and qTGW4B.2 were derived from the same parent line (Table 4). A QTL cluster for HD, PH, and SL that mapped to the interval 101.44–119.21 cM on chromosome 6B (Table 5) is likely the same or similar to a QTL cluster for yield-related traits reported by Li et al. (2018). qHD6B co-localized with qSL6B, with favorable alleles derived from opposite parents (Tables 4, 5). This is consistent with the negative correlation between HD and SL (Table 2).
Candidate Genes Affecting PH, TGW, and HD
Using KASP markers, we delimited the QTL regions for PH and TGW on chromosome 4B to a 0.8 Mb physical region (Figure 3B and Table 6). A recent study identified a QTL cluster for TGW linked to Rht-B1 on chromosome 4B using near-isogenic lines (Guan et al., 2020). This region includes the physical interval identified in our study. According to gene annotation and analysis of the effects of SNPs in the mapped region (Supplementary Table 6), a mutation in TraesCS4B02G281200 encoding a PAM68 protein showed the largest effect on gene function. The PAM68 protein is essential for efficient D1 biogenesis and photosystem II assembly in Arabidopsis (Armbruster et al., 2010, 2013). Split-ubiquitin assays suggested that the C terminus of Arabidopsis PAM68 is required for interaction with the PSII core proteins D1 and CP43 (Armbruster et al., 2010). The variation in the PAM68 protein between LX987 and eh1 is located at the C terminus, and this region is conserved in grasses (Supplementary Figure 2). This indicates that the mutation in PAM68 may affect gene function. Photosynthesis plays an important role in yield improvement (Zhu et al., 2010). It has been reported that mutation of the photosystem 1-F subunit (OsPS1-F) results in reduction of PH and grain yield in rice (Ramamoorthy et al., 2018). Taken together, our results and previous findings suggest that PAM68 is a candidate gene for the PH and TGW QTLs. We found genes TraesCS4B01G281000 and TraesCS4B01G281300 in the QTL region were not expressed in the RNA-seq data, which may due to that the RNA-seq data was collected from spikes in the HD (Li et al., 2020). Therefore, we could not exclude these two genes as candidate genes from the sequences of RNA and the predicted effects of SNP. However, TraesCS4B01G281000 and TraesCS4B01G281300 encode Histidine-containing phosphotransfer protein and Tribbles homolog 3, respectively, which have not been reported for involving in PH and TGW. In this respect, the possibility for these two candidate genes is low.
We also confirmed and narrowed down the QTL region for HD on chromosome 3A to a 2.5 Mb interval (Figure 3A and Table 6). Analysis of SNP effects suggested that a mutation in TraesCS3A01G086400 has a large effect on gene function (Supplementary Table 8), suggesting that this gene may affect HD in the RIL population. TraesCS3A01G086400 encodes an R3H domain-containing protein, which functions in binding polynucleotides, including DNA, RNA, and single-stranded DNA (Grishin, 1998). Studies on R3H-containing proteins in maize (Saleh et al., 2006) and Arabidopsis (Wang et al., 2019) have suggested that these proteins are involved in stress responses. Whether the R3H domain-containing protein contributes to HD variation need to be further studied.
Conclusion
We identified 37 QTLs for HD, PH, TGW, and SL in a RIL population using the Wheat55K SNP Array, and validated the stably detected QTLs on chromosome 3A, 4B, and 6A using KASP markers. The QTLs on chromosomes 4B and 3A were delimited to a physical interval of 0.8 Mb and 2.5 Mb, respectively. Moreover, the candidate genes affecting PH, TGW, and HD were predicted based on gene annotation and analysis of SNP effects. The linked KASP markers developed in this study will facilitate breeding for yield improvement in wheat.
Data Availability Statement
The original contributions presented in the study are included in the article/Supplementary Material, further inquiries can be directed to the corresponding author/s.
Author Contributions
LL conceived the project and revised the manuscript. HX and YL conducted most of the experiments. HG constructed the RIL population and assisted in collection of the phenotypic data. YX and JG performed genotype analyses. LZ, SZ, and YD participated in field trials. HX wrote the first draft of the manuscript. All authors have read and approved the final manuscript.
Funding
This work was financially supported by the National Natural Science Foundation of China (grant numbers 31801346 and 11775304), the National Key Research and Development Program of China (grant numbers 2020YFE0202300), the China Agriculture Research System (grant number CARS-03), and Fundamental Research Funds for Central Non-Profit of Institute of Crop Sciences, Chinese Academy of Agricultural Sciences (grant number Y2020YJ09).
Conflict of Interest
The authors declare that the research was conducted in the absence of any commercial or financial relationships that could be construed as a potential conflict of interest.
Acknowledgments
We thank Dr. Junli Zhang (Department of Plant Sciences, University of California, Davis, United States) for assisting in SNP effects analysis.
Supplementary Material
The Supplementary Material for this article can be found online at: https://www.frontiersin.org/articles/10.3389/fpls.2021.628478/full#supplementary-material
Supplementary Figure 1 | Genotypes of eh1 and LX987 obtained using the Rht1 KASP markers.
Supplementary Figure 2 | Multiple sequence alignment of PAM68 in Brachypodium distachyon, barley, rice, sorghum, maize, and wheat. The labeling with red asterisk indicates the position of variation between the two parent lines.
Supplementary Table 1 | Physical positions of left and right markers of identified QTLs.
Supplementary Table 2 | The primers used for KASP assays.
Supplementary Table 3 | Variance analysis of PH, TGW, and SL in the 400 RIL. ∗∗∗ indicates significance at p ≤ 0.001.
Supplementary Table 4 | MET analysis of QTLs by environment interactions. QTL with a LOD (AbyE) >2.5 was significant interaction with environment.
Supplementary Table 5 | High-confidence genes located between markers 4B271b and 4B288b.
Supplementary Table 6 | SNPs identified using RNA-seq data for LX987 and eh1 in the mapped region of chromosome 4B.
Supplementary Table 7 | High-confidence genes located between markers 3A128b and 3A16.
Supplementary Table 8 | SNPs identified using RNA-seq data for LX987 and eh1 in the mapped region of chromosome 3A.
Supplementary Table 9 | Correlation coefficient analyses of HD, PH, TGW, and SL among different years.
Footnotes
- ^ http://www.isbreeding.net/
- ^ http://www.cgmb.com.cn/
- ^ http://polymarker.tgac.ac.uk/
- ^ https://www.python.org/
- ^ https://github.com/pinbo/gene_manual_annotation
- ^ http://202.194.139.32/searchtools/
- ^ http://202.194.139.32/searchtools/
References
Alvarez, M. A., Tranquilli, G., Lewis, S., Kippes, N., and Dubcovsky, J. (2016). Genetic and physical mapping of the earliness per se locus Eps-A (m) 1 in Triticum monococcum identifies EARLY FLOWERING 3 (ELF3) as a candidate gene. Funct. Integr. Genom. 16, 365–382. doi: 10.1007/s10142-016-0490-3
Araki, E., Miura, H., and Sawada, S. (1999). Identification of genetic loci affecting amylose content and agronomic traits on chromosome 4A of wheat. Theor. Appl. Genet. 98, 977–984. doi: 10.1007/s001220051158
Armbruster, U., Rühle, T., Kreller, R., Strotbek, C., Zühlke, J., Tadini, L., et al. (2013). The photosynthesis affected mutant68–like protein evolved from a PSII assembly factor to mediate assembly of the chloroplast NAD(P)H dehydrogenase complex in Arabidopsis. Plant Cell 25, 3926–3943. doi: 10.1105/tpc.113.114785
Armbruster, U., Zühlke, J., Rengstl, B., Kreller, R., Makarenko, E., Rühle, T., et al. (2010). The Arabidopsis thylakoid protein PAM68 is required for efficient D1 biogenesis and photosystem II assembly. Plant Cell 22, 3439–3460. doi: 10.1105/tpc.110.077453
Asplund, L., Leino, M., and Hagenblad, J. (2012). Allelic variation at the Rht8 locus in a 19th century wheat collection. Sci. World J. 2012:385610. doi: 10.1100/2012/385610
Beales, J., Turner, A., GriYths, S., Snape, J. W., and Laurie, D. A. (2007). A Pseudo-Response Regulator is misexpressed in the photoperiod insensitive Ppd-D1a mutant of wheat (Triticum aestivum L.). Theor. Appl. Genet. 115, 721–733.
Bednarek, J., Boulaflous, A., Girousse, C., Ravel, C., Tassy, C., Barret, P., et al. (2012). Down-regulation of the TaGW2 gene by RNA interference results in decreased grain size and weight in wheat. J. Exp. Bot. 63, 5945–5955.
Börner, A., Schumann, E., Fürste, A., Cöster, H., Leithold, B., Röder, M., et al. (2002). Mapping of quantitative trait loci determining agronomic important characters in hexaploid wheat (Triticum aestivum L.). Theor. Appl. Genet. 105, 921–936. doi: 10.1007/s00122-002-0994-1
Cao, P., Liang, X., Zhao, H., Feng, B., Xu, E., Wang, L., et al. (2019). Identification of the quantitative trait loci controlling spike-related traits in hexaploid wheat (Triticum aestivum L.). Planta 250, 1967–1981. doi: 10.1007/s00425-019-03278-0
Chai, L., Chen, Z., Bian, R., Zhai, H., Cheng, X., Peng, H., et al. (2019). Dissection of two quantitative trait loci with pleiotropic effects on plant height and spike length linked in coupling phase on the short arm of chromosome 2D of common wheat (Triticum aestivum L.). Theor. Appl. Genet. 132, 1815–1831. doi: 10.1007/s00122-019-03318-z
Chen, S., Gao, R., Wang, H., Wen, M., Xiao, J., Bian, N., et al. (2015). Characterization of a novel reduced height gene (Rht23) regulating panicle morphology and plant architecture in bread wheat. Euphytica 203, 583–594. doi: 10.1007/s10681-014-1275-1
Chen, Z., Cheng, X., Chai, L., Wang, Z., Bian, R., Li, J., et al. (2020). Dissection of genetic factors underlying grain size and fine mapping of QTgw.cau-7D in common wheat (Triticum aestivum L.). Theor. Appl. Genet. 133, 149–162. doi: 10.1007/s00122-019-03447-5
Cui, F., Ding, A., Li, J., Zhao, C., Wang, L., Wang, X., et al. (2012a). QTL detection of seven spike-related traits and their genetic correlations in wheat using two related RIL populations. Euphytica 186, 177–192. doi: 10.1007/s10681-011-0550-7
Cui, F., Ding, A. M., Li, J., Zhao, C. H., Wang, L., Wang, X. Q., et al. (2012b). QTL detection of seven spike-related traits and their genetic correlations in wheat using two related RIL populations. Euphytica 186, 177–192.
Cui, F., Zhao, C., Ding, A., Li, J., Wang, L., Li, X., et al. (2014a). Construction of an integrative linkage map and QTL mapping of grain yield-related traits using three related wheat RIL populations. Theor. Appl. Genet. 127, 659–675. doi: 10.1007/s00122-013-2249-8
Cui, F., Zhao, C. H., Ding, A. M., Li, J., Wang, L., Li, X. F., et al. (2014b). Construction of an integrative linkage map and QTL mapping of grain yield-related traits using three related wheat RIL populations. Theor. Appl. Genet. 127, 659–675.
Cuthbert, J. L., Somers, D. J., Brûlé-Babel, A. L., Brown, P. D., and Crow, G. H. (2008). Molecular mapping of quantitative trait loci for yield and yield components in spring wheat (Triticum aestivum L.). Theor. Appl. Genet. 117, 595–608. doi: 10.1007/s00122-008-0804-5
Deng, S., Wu, X., Wu, Y., Zhou, R., Wang, H., Jia, J., et al. (2011). Characterization and precise mapping of a QTL increasing spike number with pleiotropic effects in wheat. Theor. Appl. Genet. 122, 281–289. doi: 10.1007/s00122-010-1443-1
Ellis, M. H., Rebetzke, G. J., Azanza, F., Richards, R. A., and Spielmeyer, W. (2005). Molecular mapping of gibberellin-responsive dwarfing genes in bread wheat. Theor. Appl. Genet. 111, 423–430. doi: 10.1007/s00122-005-2008-6
Flintham, J. E., Borner, A., Worland, A. J., and Gale, M. D. (1997). Optimizing wheat grain yield: effects of Rht (gibberellin-insensitive) dwarfing genes. J. Agr. Sci. 128, 11–25.
Gao, F., Wen, W., Liu, J., Rasheed, A., Yin, G., Xia, X., et al. (2015). Genome-wide linkage mapping of QTL for yield components, plant height and yield-related physiological traits in the Chinese wheat cross Zhou 8425B/Chinese Spring. Front. Plant Sci. 6:1099. doi: 10.3389/fpls.2015.01099
Gao, F. M., Liu, J. D., Yang, L., Wu, X. X., Xiao, Y. G., Xia, X. C., et al. (2016). Genome-wide linkage mapping of QTL for physiological traits in a chinese wheat population using the 90K SNP array. Euphytica 209, 789–804.
Grant, N. P., Mohan, A., Sandhu, D., and Gill, K. S. (2018). Inheritance and genetic mapping of the reduced height (Rht18) gene in wheat. Plants 7:58. doi: 10.3390/plants7030058
Grishin, N. V. (1998). The R3H motif: a domain that binds single-stranded nucleic acids. Trends Biochem. Sci. 23, 329–330.
Guan, P., Lu, L., Jia, L., Kabir, M. R., Zhang, J., Lan, T., et al. (2018). Global QTL analysis identifies genomic regions on chromosomes 4A and 4B harboring stable loci for yield-related traits across different environments in wheat (Triticum aestivum L.). Front. Plant Sci. 9:529. doi: 10.3389/fpls.2018.00529
Guan, P., Shen, X., Mu, Q., Wang, Y., Wang, X., Chen, Y., et al. (2020). Dissection and validation of a QTL cluster linked to Rht-B1 locus controlling grain weight in common wheat (Triticum aestivum L.) using near-isogenic lines. Theor. Appl. Genet. 133, 2639–2653. doi: 10.1007/s00122-020-03622-z
Haque, M. A., Martinek, P., Watanabe, N., and Kuboyama, T. (2011). Genetic mapping of gibberellic acid-sensitive genes for semi-dwarfism in durum wheat. Cereal Res. Commun. 39, 171–178.
Hu, J., Wang, X., Zhang, G., Jiang, P., Chen, W., Hao, Y., et al. (2020). QTL mapping for yield-related traits in wheat based on four RIL populations. Theor. Appl. Genet. 133, 917–933. doi: 10.1007/s00122-019-03515-w
Huang, S., Wu, J., Wang, X., Mu, J., Xu, Z., Zeng, Q., et al. (2019). Utilization of the genomewide wheat 55K SNP array for genetic analysis of stripe rust resistance in common wheat Line P9936. Phytopathology 109, 819–827. doi: 10.1094/PHYTO-10-18-0388-R
International Wheat Genome Sequencing Consortium. (2018). Shifting the limits in wheat research and breeding using a fully annotated reference genome. Science 361:eaar7191. doi: 10.1126/science.aar7191
Jahani, M., Mohammadi-Nejad, G., Nakhoda, B., and Rieseberg, L. H. (2019). Genetic dissection of epistatic and QTL by environment interaction effects in three bread wheat genetic backgrounds for yield-related traits under saline conditions. Euphytica 215:103. doi: 10.1007/s10681-019-2426-1
Jaiswal, V., Gahlaut, V., Mathur, S., Agarwal, P., Khandelwal, M. K., Khurana, J. P., et al. (2015). Identification of novel SNP in promoter sequence of TaGW2-6A associated with grain weight and other agronomic traits in wheat (Triticum aestivum L.). PLoS One 10:e0129400. doi: 10.1371/journal.pone.0129400
Kawaura, K., Takaku, M., Imai, T., and Ogihara, Y. (2009). Molecular analysis of the Q gene controlling spike morphology with TILLING lines of common wheat. Genes Genet. Syst. 84, 476–476.
Kippes, N., Debernardi, J. M., Vasquez-Gross, H. A., Akpinar, B. A., Budak, H., Kato, K., et al. (2015). Identification of the VERNALIZATION 4 gene reveals the origin of spring growth habit in ancient wheats from south asia. Proc. Natl. Acad. Sci. U.S.A. 112, E5401–E5410.
Kiseleva, A. A., and Salina, E. A. (2018). Genetic regulation of common wheat heading time. Russ. J. Genet. 54, 375–388. doi: 10.1134/S1022795418030067
Kumar, A., Mantovani, E. E., Seetan, R., Soltani, A., Echeverry-Solarte, M., Jain, S., et al. (2016). Dissection of genetic factors underlying wheat kernel shape and size in an elite x non-adapted cross using a high density SNP linkage map. Plant Genome 9:81. doi: 10.3835/plantgenome2015.09.0081
Kumar, N., Kulwal, P. L., Gaur, A., Tyagi, A. K., Khurana, J. P., Khurana, P., et al. (2006). QTL analysis for grain weight in common wheat. Euphytica 151, 135–144.
Li, F., Wen, W., He, Z., Liu, J., Jin, H., Cao, S., et al. (2018). Genome-wide linkage mapping of yield-related traits in three Chinese bread wheat populations using high-density SNP markers. Theor. Appl. Genet. 131, 1903–1924. doi: 10.1007/s00122-018-3122-6
Li, S., Wang, J., and Zhang, L. (2015). Inclusive composite interval mapping of QTL by environment interactions in biparental populations. PLoS One 10:e0132414.
Li, W. L., Nelson, J. C., Chu, C. Y., Shi, L. H., Huang, S. H., Liu, D. J. et al. (2002). Chromosomal locations and genetic relationships of tiller and spike characters in wheat. Euphytica 125, 357–366.
Li, Y., Xiong, H., Guo, H., Zhou, C., Xie, Y., Zhao, L., et al. (2020). Identification of the vernalization gene VRN-B1 responsible for heading date variation by QTL mapping using a RIL population in wheat. BMC Plant Biol. 20:331. doi: 10.1186/s12870-020-02539-5
Liu, J., Xu, Z. B., Fan, X. L., Zhou, Q., Cao, J., Wang, F., et al. (2018a). A genome-wide association study of wheat spike related traits in china. Front. Plant Sci. 9:1584. doi: 10.3389/fpls.2018.01584
Liu, J. J., Luo, W., Qin, N. N., Ding, P. Y., Zhang, H., Yang, C. C., et al. (2018b). A 55K SNP array-based genetic map and its utilization in QTL mapping for productive tiller number in common wheat. Theor. Appl. Genet. 131, 2439–2450.
Lou, X., Li, X., Li, A., Pu, M., Shoaib, M., Liu, D., et al. (2016). The 160 bp insertion in the promoter of Rht-B1i plays a vital role in increasing wheat height. Front. Plant Sci. 7:307. doi: 10.3389/fpls.2016.00307
Lu, Y., Xing, L., Xing, S., Hu, P., Cui, C., Zhang, M., et al. (2015). Characterization of a putative new semi-dominant reduced height gene, Rht_NM9, in wheat (Triticum aestivum L.). J. Genet. Genom. 42, 685–698. doi: 10.1016/j.jgg.2015.08.007
Ma, J., Zhang, H., Li, S., Zou, Y., Li, T., Liu, J., et al. (2019). Identification of quantitative trait loci for kernel traits in a wheat cultivar Chuannong16. BMC Genet. 20:77. doi: 10.1186/s12863-019-0782-4
Marrano, A., Martínez-García, P. J., Bianco, L., Sideli, G. M., Di Pierro, E. A., Leslie, C. A., et al. (2019). A new genomic tool for walnut (Juglans regia L.): development and validation of the high-density AxiomTM J. regia 700K SNP genotyping array. Plant Biotechnol. J. 17, 1027–1036. doi: 10.1111/pbi.13034
Marza, F., Bai, G. H., Carver, B. F., and Zhou, W. C. (2006). Quantitative trait loci for yield and related traits in the wheat population Ning7840 x Clark. Theor. Appl. Genet. 112, 688–698. doi: 10.1007/s00122-005-0172-3
Mayer, K. F. X., Rogers, J., Doležel, J., Pozniak, C., Eversole, K., Feuillet, C., et al. (2014). A chromosome-based draft sequence of the hexaploid bread wheat (Triticum aestivum) genome. Science 345:6194. doi: 10.1126/science.1251788
Milec, Z., Valarik, M., Bartos, J., and Safar, J. (2014). Can a late bloomer become an early bird? Tools for flowering time adjustment. Biotechnol. Adv. 32, 200–214. doi: 10.1016/j.biotechadv.2013.09.008
Mir, R. R., Kumar, N., Jaiswal, V., Girdharwal, N., Prasad, M., Balyan, H. S., et al. (2012). Genetic dissection of grain weight in bread wheat through quantitative trait locus interval and association mapping. Mol. Breed. 29, 963–972.
Mo, Y., Vanzetti, L. S., Hale, I., Spagnolo, E. J., Guidobaldi, F., Al-Oboudi, J., et al. (2018). Identification and characterization of Rht25, a locus on chromosome arm 6AS affecting wheat plant height, heading time, and spike development. Theor. Appl. Genet. 131, 2021–2035.
Peng, J., Richards, D. E., Hartley, N. M., Murphy, G. P., Devos, K. M., Flintham, J. E., et al. (1999). ‘Green revolution’ genes encode mutant gibberellin response modulators. Nature 400, 256–261. doi: 10.1038/22307
Perez-Lara, E., Semagn, K., Chen, H., Iqbal, M., N’Diaye, A., Kamran, A., et al. (2016). QTLs associated with agronomic traits in the Cutler x AC barrie spring wheat mapping population using single nucleotide polymorphic markers. PLoS One 11:e0160623. doi: 10.1371/journal.pone.0160623
Ramamoorthy, R., Vishal, B., Ramachandran, S., and Kumar, P. P. (2018). The OsPS1-F gene regulates growth and development in rice by modulating photosynthetic electron transport rate. Plant Cell Rep. 37, 377–385. doi: 10.1007/s00299-017-2235-8
Rasheed, A., Hao, Y., Xia, X., Khan, A., Xu, Y., Varshney, R. K., et al. (2017). Crop breeding chips and genotyping platforms: progress, challenges, and perspectives. Mol. Plant 10, 1047–1064. doi: 10.1016/j.molp.2017.06.008
Rasheed, A., Wen, W., Gao, F., Zhai, S., Jin, H., Liu, J., et al. (2016). Development and validation of KASP assays for genes underpinning key economic traits in bread wheat. Theor. Appl. Genet. 129, 1843–1860. doi: 10.1007/s00122-016-2743-x
Rasheed, A., and Xia, X. (2019). From markers to genome-based breeding in wheat. Theor. Appl. Genet. 132, 767–784. doi: 10.1007/s00122-019-03286-4
Ren, T., Hu, Y., Tang, Y., Li, C., Yan, B., Ren, Z., et al. (2018). Utilization of a Wheat55K SNP array for mapping of major QTL for temporal expression of the tiller number. Front. Plant Sci. 9:333. doi: 10.3389/fpls.2018.00333
Saleh, A., Lumbreras, V., Lopez, C., Dominguez-Puigjaner, E., Kizis, D., Pages, M. et al. (2006). Maize DBF1-interactor protein 1 containing an R3H domain is a potential regulator of DBF1 activity in stress responses. Plant J. 46, 747–757. doi: 10.1111/j.1365-313X.2006.02742.x
Simmonds, J., Scott, P., Brinton, J., Mestre, T. C., Bush, M., del Blanco, A., et al. (2016). A splice acceptor site mutation in TaGW2-A1 increases thousand grain weight in tetraploid and hexaploid wheat through wider and longer grains. Theor. Appl. Genet. 129, 1099–1112.
Simmonds, J., Scott, P., Leverington-Waite, M., Turner, A. S., Brinton, J., Korzun, V., et al. (2014). Identification and independent validation of a stable yield and thousand grain weight QTL on chromosome 6A of hexaploid wheat (Triticum aestivum L.). BMC Plant Biol. 14:191. doi: 10.1186/s12870-014-0191-9
Snape, J. W., Butterworth, K., Whitechurch, E., and Worland, A. J. (2001). Waiting for fine times: genetics of flowering time in wheat. Euphytica 119, 185–190. doi: 10.1023/A:1017594422176
Su, Z. Q., Hao, C. Y., Wang, L. F., Dong, Y. C., and Zhang, X. Y. (2011). Identification and development of a functional marker of TaGW2 associated with grain weight in bread wheat (Triticum aestivum L.). Theor. Appl. Genet. 122, 211–223.
Tian, X. L., Wen, W. E., Xie, L., Fu, L. P., Xu, D. G., Fu, C., et al. (2017). Molecular mapping of reduced plant height gene Rht24 in bread wheat. Front. Plant Sci. 8:1379. doi: 10.3389/fpls.2017.01379
Tshikunde, N. M., Mashilo, J., Shimelis, H., and Odindo, A. (2019). Agronomic and physiological traits, and associated quantitative trait loci (QTL) affecting yield response in wheat (Triticum aestivum L.): a review. Front. Plant Sci. 10:1428. doi: 10.3389/fpls.2019.01428
Varshney, R. K., Prasad, M., Roy, J. K., Kumar, N., Harjit, S., Dhaliwal, H. S., et al. (2000). Identification of eight chromosomes and a microsatellite marker on 1AS associated with QTL for grain weight in bread wheat. Theor. Appl. Genet. 100, 1290–1294. doi: 10.1007/s001220051437
Vikhe, P., Patil, R., Chavan, A., Oak, M., and Tamhankar, S. (2017). Mapping gibberellin-sensitive dwarfing locus Rht18 in durum wheat and development of SSR and SNP markers for selection in breeding. Mol. Breed. 37, 1–10.
Wang, H. Y., Liu, C., Ren, Y. C., Wu, M. H., Wu, Z. W., Chen, Y., et al. (2019). An RNA-binding protein MUG13.4 interacts with AtAGO2 to modulate salinity tolerance in Arabidopsis. Plant Sci. 288:110218. doi: 10.1016/j.plantsci.2019.110218
Wolde, G. M., Trautewig, C., Mascher, M., and Schnurbusch, T. (2019). Genetic insights into morphometric inflorescence traits of wheat. Theor. Appl. Genet. 132, 1661–1676. doi: 10.1007/s00122-019-03305-4
Wurschum, T., Langer, S. M., Longin, C. F. H., Tucker, M. R., and Leiser, W. L. (2017). A modern green revolution gene for reduced height in wheat. Plant J. 92, 892–903. doi: 10.1111/tpj.13726
Xie, L., Zhang, Y., Wang, K., Luo, X., Xu, D., Tian, X., et al. (2019). TaVrt2, an SVP-like gene, cooperates with TaVrn1 to regulate vernalization-induced flowering in wheat. New Phytol. 2019:16339. doi: 10.1111/nph.16339
Xu, D., Wen, W., Fu, L., Li, F., Li, J., Xie, L., et al. (2019). Genetic dissection of a major QTL for kernel weight spanning the Rht-B1 locus in bread wheat. Theor. Appl. Genet. 132, 3191–3200. doi: 10.1007/s00122-019-03418-w
Yan, L., Fu, D., Li, C., Blechl, A., Tranquilli, G., Bonafede, M., et al. (2006). The wheat and barley vernalization gene VRN3 is an orthologue of FT. Proc. Natl. Acad. Sci. U.S.A. 103, 19581–19586.
Yan, L., Loukoianov, A., Tranquilli, G., Helguera, M., Fahima, T., and Dubcovsky, J. (2003). Positional cloning of the wheat vernalization gene VRN1. Proc. Natl. Acad. Sci. U.S.A. 100, 6263–6268.
Yan, L. L., Loukoianov, A., Blechl, A., Tranquilli, G., Ramakrishna, W., SanMiguel, P., et al. (2004). The wheat VRN2 gene is a flowering repressor down-regulated by vernalization. Science 303, 1640–1644.
Yang, J., Zhou, Y., Wu, Q., Chen, Y., Zhang, P., Zhang, Y., et al. (2019). Molecular characterization of a novel TaGL3-5A allele and its association with grain length in wheat (Triticum aestivum L.). Theor. Appl. Genet. 132, 1799–1814. doi: 10.1007/s00122-019-03316-1
Yang, L., Zhao, D., Meng, Z., Xu, K., Yan, J., Xia, X., et al. (2020). QTL mapping for grain yield-related traits in bread wheat via SNP-based selective genotyping. Theor. Appl. Genet. 133, 857–872. doi: 10.1007/s00122-019-03511-0
Yao, H., Xie, Q., Xue, S., Luo, J., Lu, J., Kong, Z., et al. (2019). HL2 on chromosome 7D of wheat (Triticum aestivum L.) regulates both head length and spikelet number. Theor. Appl. Genet. 132, 1789–1797. doi: 10.1007/s00122-019-03315-2
Zhai, H. J., Feng, Z. Y., Du, X. F., Song, Y. E., Liu, X. Y., Qi, Z. Q., et al. (2018). A novel allele of TaGW2-A1 is located in a finely mapped QTL that increases grain weight but decreases grain number in wheat (Triticum aestivum L.). Theor. Appl. Genet. 131, 539–553.
Zhang, P., Li, X., Gebrewahid, T. W., Liu, H., Xia, X., He, Z., et al. (2019). QTL mapping of adult-plant resistance to leaf and stripe rust in wheat cross SW 8588/Thatcher using the wheat 55K SNP array. Plant Dis. 103, 3041–3049. doi: 10.1094/pdis-02-19-0380-re
Zhang, Y., Li, D., Zhang, D. B., Zhao, X. G., Cao, X. M., Dong, L. L., et al. (2018). Analysis of the functions of TaGW2 homoeologs in wheat grain weight and protein content traits. Plant J. 94, 857–866.
Zhao, C. H., Sun, H., Liu, C., Yang, G. M., Liu, X. J., Wang, Y. P., et al. (2019). Detection of quantitative trait loci for wheat (Triticum aestivum L.) heading and flowering date. J. Agr. Sci. 157, 20–30. doi: 10.1017/S0021859619000200
Zhu, X. G., Long, S. P., and Ort, D. R. (2010). Improving photosynthetic efficiency for greater yield. Annu. Rev. Plant Biol. 61, 235–261. doi: 10.1146/annurev-arplant-042809-112206
Keywords: QTL, heading date, plant height, thousand grain weight, spike length, wheat
Citation: Xiong H, Li Y, Guo H, Xie Y, Zhao L, Gu J, Zhao S, Ding Y and Liu L (2021) Genetic Mapping by Integration of 55K SNP Array and KASP Markers Reveals Candidate Genes for Important Agronomic Traits in Hexaploid Wheat. Front. Plant Sci. 12:628478. doi: 10.3389/fpls.2021.628478
Received: 12 November 2020; Accepted: 29 January 2021;
Published: 23 February 2021.
Edited by:
Peter Stephen Baenziger, University of Nebraska System, United StatesReviewed by:
Waseem Hussain, International Rice Research Institute (IRRI), PhilippinesShantel Amealia Martinez, Washington State University, United States
Copyright © 2021 Xiong, Li, Guo, Xie, Zhao, Gu, Zhao, Ding and Liu. This is an open-access article distributed under the terms of the Creative Commons Attribution License (CC BY). The use, distribution or reproduction in other forums is permitted, provided the original author(s) and the copyright owner(s) are credited and that the original publication in this journal is cited, in accordance with accepted academic practice. No use, distribution or reproduction is permitted which does not comply with these terms.
*Correspondence: Luxiang Liu, bGl1bHV4aWFuZ0BjYWFzLmNu
†These authors have contributed equally to this work