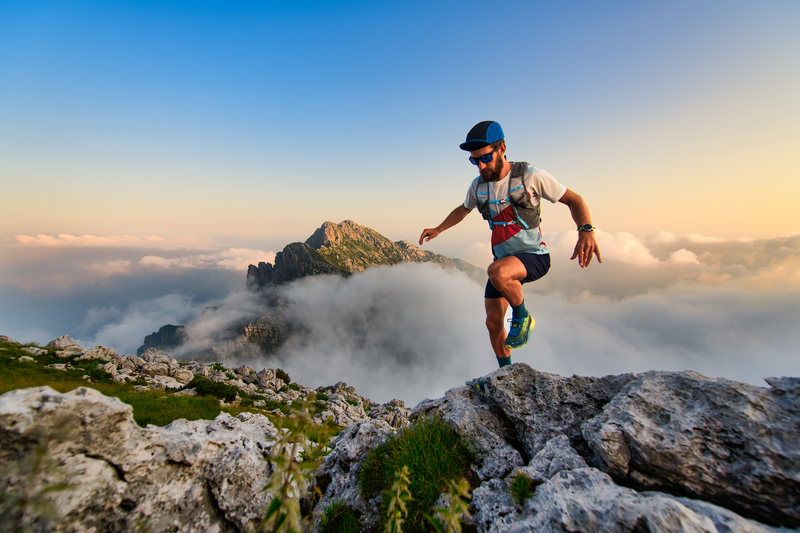
95% of researchers rate our articles as excellent or good
Learn more about the work of our research integrity team to safeguard the quality of each article we publish.
Find out more
ORIGINAL RESEARCH article
Front. Plant Sci. , 26 February 2021
Sec. Plant Breeding
Volume 12 - 2021 | https://doi.org/10.3389/fpls.2021.611106
Spikelet number is an important target trait for wheat yield improvement. Thus, the identification and verification of novel quantitative trait locus (QTL)/genes controlling spikelet number are essential for dissecting the underlying molecular mechanisms and hence for improving grain yield. In the present study, we constructed a high-density genetic map for the Kechengmai1/Chuanmai42 doubled haploid (DH) population using 13,068 single-nucleotide polymorphism (SNP) markers from the Wheat 55K SNP array. A comparison between the genetic and physical maps indicated high consistence of the marker orders. Based on this genetic map, a total of 27 QTLs associated with total spikelet number per spike (TSN) and fertile spikelet number per spike (FSN) were detected on chromosomes 1B, 1D, 2B, 2D, 3D, 4A, 4D, 5A, 5B, 5D, 6A, 6B, and 7D in five environments. Among them, five QTLs on chromosome 2D, 3D, 5A, and 7D were detected in multiple environments and combined QTL analysis, explaining the phenotypic variance ranging from 3.64% to 23.28%. Particularly, QTsn/Fsn.cib-3D for TSN and FSN [phenotypic variation explained (PVE) = 5.97–23.28%, limit of detection (LOD) = 3.73–18.51] is probably a novel locus and located in a 4.5-cM interval on chromosome arm 3DL flanking by the markers AX-110914105 and AX-109429351. This QTL was further validated in other two populations with different genetic backgrounds using the closely linked Kompetitive Allele-Specific PCR (KASP) marker KASP_AX-110914105. The results indicated that QTsn/Fsn.cib-3D significantly increased the TSN (5.56–7.96%) and FSN (5.13–9.35%), which were significantly correlated with grain number per spike (GNS). We also preliminary analyzed the candidate genes within this locus by sequence similarity, spatial expression patterns, and collinearity analysis. These results provide solid foundation for future fine mapping and cloning of QTsn/Fsn.cib-3D. The developed and validated KASP markers could be utilized in molecular breeding aiming to increase the grain yield in wheat.
Bread wheat (Triticum aestivum L.) is one of the most important stable foods in the world, providing more than 20% of the calories and protein in our daily diet (Yao et al., 2019; Liu et al., 2020). It was reported that world population is expected to reach nine billion by 2050, which will require raising overall food production by at least 70% to fulfill future food demand (Yang et al., 2012). Conversely, arable land and water resources for agriculture continue to dwindle, and climate change also causes adverse effects on crop productivity (Evans et al., 2008; Butterworth et al., 2010; Ceccarelli et al., 2010). Thus, increasing wheat yield is an urgent task to fulfill global food and nutritional security.
Grain yield of wheat is a complex trait affected by a multitude of genetic and environmental factors and is usually constituted of three major components, i.e., thousand kernel weight (TKW), grain number per spike (GNS), and spike number per unit area (Wang et al., 2018; Kuzay et al., 2019). GNS potential is largely established by the spike architecture. Similar to other Triticeae species, wheat contains an unbranched inflorescence, and a number of spikelets are directly attached to the inflorescence axis in a distichous phyllotaxis, with a terminal spikelet at its apex (Koppolu and Schnurbusch, 2019). The spikelet is made of an indeterminate number of florets attached to a secondary axis (rachilla) (Hanif and Langer, 1972; Wolde et al., 2019). Therefore, the number of fertile spikelet number per spike (FSN) and fertile floret per spikelet ultimately determined GNS (Guo et al., 2017; Golan et al., 2019). Previous studies have showed that floret fertility is severely affected by environmental and genetic factors as an abortion process of floral structures existing continuously during the whole floral developmental process (Guo and Schnurbusch, 2015; Sakuma et al., 2019). By comparison, spikelet number is determined at an early stage of wheat reproductive development, exhibiting less environmentally sensitive with high heritabilities (Zhang et al., 2018; Gao et al., 2019). Thus, understanding the genetic factors underlying spikelet number would provide the prerequisite information necessary to improve wheat yield.
Similar to other yield-related traits, spikelet number is a complicated quantitative trait [quantitative trait locus (QTL)] controlled by multiple genes in wheat. Thus, determination of the number, chromosomal localization, and genetic effects of these polygenes is desirable for obtaining optimal genotype in breeding practice (Ma et al., 2007; Chen et al., 2020). However, due to the complexity of wheat genome, only a few genes associated with spikelet number or spike morphology have been cloned through homologous cloning approach. For instance, WAPO1, an ortholog of the rice gene APO1, on chromosome 7AL could regulate spikelet number by effect inflorescence development (Kuzay et al., 2019; Voss-fels et al., 2019). The domestication gene Q on chromosome 5A encodes a member of AP2 transcription factor family and can regulate rachis fragility, glume tenacity, head length, and spikelet density (Faris et al., 2003; Simons et al., 2006; Debernardi et al., 2017; Greenwood et al., 2017; Jiang et al., 2019). The photoperiod sensitivity gene Ppd-1 on chromosome 2D is associated with multiple pollinator traits, including spikelet number, spike length (SL), and number of days to heading (Okada et al., 2019); TaMOC1, an ortholog gene of rice MOC1, is significantly associated with spikelet number (Zhang et al., 2015); FZP gene can drive supernumerary spikelet (Dobrovolskaya et al., 2015); TaDEP1, an ortholog of rice DEP1, relates to increase SL and reduces the spikelet number (Huang et al., 2009).
In addition, numerous QTLs for spikelet number have been identified on almost all the 21 chromosomes in wheat using different genetic populations and natural varieties (Cui et al., 2012; Yu et al., 2014; Gao et al., 2015; Luo et al., 2016; Zhai et al., 2016; Deng et al., 2017; Liu et al., 2018b; Wang et al., 2018; Koppolu and Schnurbusch, 2019; Ma et al., 2019; Yao et al., 2019; Kuang et al., 2020). Nevertheless, only a few of QTLs have been genetically verified, offering a foundation for fine mapping and map-based cloning, which greatly restrict the dissection of the molecular mechanisms underlying spikelet number as well as improvement of spikelet number in wheat breeding. Thus, the identification and verification of novel QTL/genes for spikelet number are vital.
In this study, we constructed a high-density genetic map using the Wheat 55K single-nucleotide polymorphism (SNP) array and a doubled haploid (DH) population derived from a cross between two wheat elite varieties K1 and CM42. QTL mapping for two spikelet number-related traits, total spikelet number per spike (TSN), and FSN was performed, and subsequently, a major QTL on chromosome 3DL was further validated in different genetic backgrounds.
Quantitative trait locus mapping was conducted using a population of 187 DH lines derived from a cross of K1 × CM42 (K1/CM42). CM42, kindly provided by Prof. Wuyun Yang (Sichuan Academy of Agricultural Science, China), is a backbone parent with excellent agronomic traits such as high TKW and high yield (Yang et al., 2009). K1, bred by our lab, is characterized by high spikelet number per spike and GNS. Two populations, including a recombinant inbred line (RIL) populations derived from a cross of K1 × Kechengmai4 (K1/K4, 70 F7 RILs) and a F2 populations derived from a cross of K1 × Yangfumai2 (K1/YFM2, 75 F2 lines), were employed to further validate the major QTL.
The K1/CM42 lines along with parents were grown in five replicates following randomized complete-block design at the following two experimental sites: Shuangliu of Sichuan province (103°52′E, 30°34′N) and Shifang of Sichuan province (104°11′E, 31°6’N), China. The field trials were carried out in two crop seasons of 2017–18 and 2018–19 at Shuangliu, and three crop seasons of 2016–17, 2017–18, and 2018–19 at Shifang. Each plot consisted of five 200-cm rows with an inter-row spacing of 20 cm. The sowing density was 50 seeds per row. The K1/K4 lines were generated by single-seed descent and cropped at Shuangliu and Shifang in 2018–19 crop seasons. Each plot consisted of two 200-cm rows with an inter-row spacing of 20 cm. The K1/YFM2 population was cropped at Shuangliu in 2019–20 crop seasons in 200-cm rows with 15-cm space between individuals.
The TSN, FSN, plant height (PH), and SL of the K1/CM42 and K1/K4 populations were manually measured at maturity by using plants from middle row of each plot. For the K1/CM42 population, the primary spike of 10 random plants from each family in 2017–18 and 2018–19 crop seasons and three random plants from each family in 2016–17 crop seasons were selected. For the K1/K4 population, the primary spikes of five random plants from each family were selected. For the K1/YFM2 population, the TSN, FSN, PH, and SL of primary spike of each individual were measured. Subsequently, all selected spikes were harvested and manually threshed for evaluating GNS, TKW, grain width (GW), and grain length (GL) using SC-G software (WSeen, Hangzhou, China).
The frequency distribution of traits in each environment and their correlation were calculated using SPSS version 20.0 for Windows (IBM SPSS, Armonk, NY, United States). The best linear unbiased predictions (BLUPs), which were used for QTL detection, correlation analyses, and effect analyses, were calculated using the R package “lme4.” Analysis of variance (ANOVA) was performed using the data from 2017–18 and 2018–19 crop seasons in the K1/CM42 population by SPSS. The broad-sense heritability (H2) was calculated according to the method described by Smith et al. (1998) and Muqaddasi et al. (2019). Student’s t test used to evaluate the significance of difference was performed by SPSS.
Genomic DNA of each line in the K1/CM42 population and their parents was extracted using the 2 × cetyl trimethylammonium bromide (CTAB) method and hybridized on the Wheat 55K SNP array by China Golden Marker (Beijing, China). DNA integrity was checked and confirmed on agarose gels, and DNA quantity was measured by spectrophotometry.
IciMapping 4.1 (Meng et al., 2015) and JoinMap 4.1 were used for genetic construction and QTL detection in the present study. First, the function of “bin” in IciMapping 4.1 was used to remove redundant markers based on their segregation patterns in the mapping population with the parameters of “Missing Rates” and “Distortion Value” being set as 20% and 0.01, respectively. Then, the function of “Population” in JoinMap 4.1 was used to create groups with limit of detection (LOD) score values ranging from 2 to 10. Finally, the Kosambi mapping function was used to order the bin markers with the parameters being set as LOD ≥ 5 and round = 3 in JoinMap 4.1. To reduce the complexity of QTL mapping analyses, only one marker was selected as a delegate from each bin to construct the linkage map. QTL detection in each environment was performed by IciMapping 4.1 with the inclusive composite interval mapping (ICIM), and a test of 1,000 permutations was used to identify the LOD threshold that corresponded to a genome-wide false discovery rate of 5% (P < 0.05). The missing phenotype was deleted in QTL analysis.
Probe DNA sequences for selected SNP were subjected to design Kompetitive Allele-Specific PCR (KASP) markers using the Triticeae Multi-omics Center1. Sequence probes for the FAM signal and the HEX signal were added to the primers of two parental genotypes, respectively. The KASP assays were performed in Bio-Rad CFX96 real-time PCR system with 10-μl reaction volumes consisting of 5 μl of 2 × master mix, 0.2 μl of primer mix, 3 μl of ddH2O, and 2 μl of DNA sample (50–150 ng/μl). Thermal cycling conditions were 94°C for 15 min hot-start activation, followed by a touchdown phase of 10 cycles (94°C for 20 s, touchdown at 61°C initially and then decreased by 0.6°C per cycle for 60 s) and finally 26 cycles of regular PCR (94°C for 20 s, 55°C for 60 s, and rest plates at 37°C for 1 min). Further cycling and resting were performed if the clustering is not significant: 94°C for 20 s, followed by 57°C for 60 s (3–10 cycles per step). The information of DNA sequences flanking the SNP was kindly provided by Prof. Jizeng Jia’s Lab (Institute of Crop Sciences, Chinese Academy of Agricultural Sciences).
The physical positions of flanking markers were obtained by blasting against (E-value of 1e-10) the genome assembly of Chinese Spring (CS)2. Genes among the mapping interval were extracted from IWGSC RefSeq v1.1 annotation3. The annotations and functions of a given gene were analyzed using UniProt4. Gene collinearity among wheat, barley, rice, and maize was performed using the Triticeae-Gene Tribe5. The temporal and spatial gene expression patterns were extracted from Wheat Expression Browser6. To further explore the potential candidate genes, we designed the corresponding specific primers1,7 to isolate and sequence these candidate genes (Tsingke Biotech Co., Ltd., China). The primer sequences were listed in Supplementary Table 2. Twenty-five-microliter PCR reaction volumes were as follows: 12.5 μl of 2 × EasyTaq PCR SuperMix (TransGen Biotech Co., Ltd., China), 3 μl of DNA sample, 1 μl of primer mix, and 7.5 μl of ddH2O.
Significant differences (P < 0.001) on TSN and FSN between K1 and CM42 across five environments and their corresponding BLUP data were detected. K1 showed more TSN and FSN than did parent CM42 (Figure 1 and Table 1). In addition, we observed the development of spikelet, and we found that K1 showed more TSN at a very early stage of spike development than that of CM42, as well as at heading and maturity stages, indicating that spikelet number is determined at an early stage of wheat reproductive development (Figure 1). For the K1/CM42 lines, the TSN and FSN ranged from 16.3 to 26.8 and from 14.2 to 25.8, respectively, showing wide and significant variation and approximately normal distribution (Table 1 and Figure 2). In addition, the TSN and FSN values across all environments and BLUP data were significantly positively correlated (P < 0.001), showing high broad-sense heritabilities (0.85 for TSN and 0.82 for FSN), indicating that they were mainly under genetic control (Table 1).
Figure 1. Spike morphology of the parents and representative lines. The morphology of spikes of K1 and CM42 at young spike stage when terminal spikelet appeared (A), heading stage (awn was cut) (B), and maturity (awn was cut) (C) are shown. (D) The mean of total spikelet number per spike (TSN) and fertile spikelet number per spike (FSN) of K1 and CM42 at maturity in five environments. (E) The morphology of spikes of representative lines from the K1/CM42 population; ∗∗∗ represents significance at P < 0.001.
Table 1. Phenotypic variation and heritability (H2) of TSN and FSN for the parents and the K1/CM42 lines in different environments.
Figure 2. Phenotypic performances, distribution, and correlation coefficients for total spikelet number per spike (TSN) (A) and fertile spikelet number per spike (FSN) (B) of parents and K1/CM42 lines in five environments and their corresponding best linear unbiased prediction (BLUP) values; ∗∗∗ represents significance at P < 0.001.
Phenotypic correlations between TSN, FSN, and other yield related traits are listed in Table 2. Significant and positive correlations (P < 0.001) between TSN and FSN and GNS, and significant and negative correlation (P < 0.01) between TSN and GL, and FSN and GL were observed in the K1/CM42 population. The highest positive correlation was detected between TSN and FSN (r = 0.96). No significant correlation was detected between TSN and TKW, TSN and PH, TSN and SL, FSN and TKW, FSN and PH, and FSN and SL were observed in the K1/CM42 population.
Among 53,063 SNPs from the Wheat 55K SNP array, 14,645 SNP markers (27.6%) were polymorphic between the two parental lines. Of them, 822 SNPs had more than 20% missing data points, 10 SNPs were not anchored on the physical linkage map, and 745 SNPs were not anchored on the genetic linkage map. Finally, the rest 13,068 SNP markers (call rate ≥ 80%) were used for linkage analysis and map construction. These markers were divided into 2,406 bins: 1,384 bins contain one SNP marker only, 1,022 bins contain multiple SNPs, and the largest bin contains 626 SNP markers on 4B. Linkage analysis showed that 2,406 bin markers were mapped on 27 linkage maps for the 21 chromosomes of wheat, in which two linkage groups were constructed for each of chromosomes 1A, 1D, 5A, 5B, 6A, and 6D. Based on the genetic information, all SNP markers including bin markers and redundant markers were integrated onto the genetic map with a total length of 3,091.39 cM and an average interval distance of 1.28 cM per bin and 0.24 cM per marker. All of the mapped markers were located to the A (33.75%), B (45.98%), and D (20.26%) genomes with a total length of 1,009.07, 895.54, and 1,186.79 cM, respectively. In addition, the lengths of constructed linkage maps range from 17.67 (1A-1) to 206.47 cM (5D); the markers on linkage maps range from 20 (1D-2) to 1228 (4B); the average interval distance between adjacent markers ranges from 0.08 (4B) to 4.37 cM (1D-2); and the average interval distance between adjacent bin markers ranges from 0.63 (2B) to 5.14 cM (1D-2) (Table 3 and Supplementary Table 1). Based on the physical locations of these SNPs on the CS genome, the marker order was relatively consistent with that in the wheat genome assembly on most of the chromosomes (1A, 1B, 2A, 3B, 3D, 4A, 4B, 5A, 5B,5D, 6A, 6D, 7A, 7B, and 7D) (Supplementary Figure 1).
Phenotypic data of TSN and FSN evaluated in five environments and their corresponding BLUP values were used for QTL mapping, where the BLUP values were treated as an additional environment. A total of 27 QTLs for TSN and FSN were detected, which were located on chromosomes 1B, 1D, 2B, 2D, 3D, 4A, 4D, 5A, 5B, 5D, 6A, 6B, and 7D, respectively. Among them, five QTLs defined as stable were detected in multiple environments and combined QTL analysis, explaining the phenotypic variance ranging from 3.64% to 23.28%. The rest QTLs were detected in one environment, explaining 2.47–18.4% of the phenotypic variance (Table 4).
For TSN, 14 QTLs were detected on chromosomes 1B, 1D, 2B, 2D, 3D, 5A, 5B, 5D, 6A, 6B, and 7D. Of them, a major QTL QTsn.cib-3D was stably detected in four environments and combined QTL analysis, explaining 6.5% to 23.15% of the phenotypic variance with LOD values ranging from 6.1 to 18.51. Another stable QTL QTsn.cib-2D was detected in two environments and combined QTL analysis, explaining 3.64–10.23% of the phenotypic variance. The positive alleles of the two loci were all contributed by K1. For the remaining 12 QTLs, they were detected in one environment and explained 2.47–15.04% of the phenotypic variance. The positive alleles of eight loci were from K1, and four loci were from CM42 (Table 4).
For FSN, 13 QTLs were identified on chromosomes 1B, 1D, 2D, 3D, 4A, 4D, 5A, 5B, 5D, and 7D. Of them, QFsn.cib-3D was a major QTL and stably detected in four environments and combined QTL analysis, explaining 5.97–23.28% of the phenotypic variance with LOD values ranging from 3.73 to 14.94. QFsn.cib-7D was detected in two environments and combined QTL analysis, explaining 7.51–12.58% of the phenotypic variance. The positive alleles of the two loci were contributed by K1. QFsn.cib-5A was detected in two environments with the phenotypic variance ranging from 5.86% to 18.4%, and the positive allele was contributed by CM42. The rest of the 10 QTLs were detected in single environment, accounting for 4.42–18.26% of the phenotypic variance. The positive alleles of seven and three loci were contributed by K1 and CM42, respectively (Table 4).
Remarkably, a comparison of the intervals of loci for TSN and FSN revealed that QTsn.cib-3D and QFSN.cib-3D were co-located in the interval of AX-110914105–AX-109429351. We thus temporarily designated the locus as QTsn/Fsn.cib-3D (Table 4).
QTsn/Fsn.cib-3D was a major and stable QTL influencing TSN and FSN. We subsequently developed a KASP marker (KASP_AX-110914105) based on SNP of the flanking marker (AX-110914105) (Supplementary Table 2). As expected, on the basis of the genotyping results for KASP_AX-110914105 in the K1/CM42 population, significant differences (P < 0.001) on TSN and FSN were detected between lines with homozygous alleles from K1 and CM42 in each environment and BLUP data (Figure 3). In addition, we further analyzed the effects of QTsn/Fsn.cib-3D on yield-related traits in the K1/CM42 population. The results revealed that QTsn/Fsn.cib-3D significantly affected GNS (7.61%) and GL (1.81%) but did not influence the TKW, PH, SL, and GW (Figure 4).
Figure 3. Genetic map of the major quantitative trait locus (QTL) QTsn/Fsn.cib-3D and its effect. Genetic map integrated with the developed Kompetitive Allele-Specific PCR (KASP) marker KASP_AX-110914105 (A). The black area is the interval of the major QTL QTsn/Fsn.cib-3D (A). Effects of the major QTL QTsn/Fsn.cib-3D on total spikelet number per spike (TSN) (B) and fertile spikelet number per spike (FSN) (C) shown as box plots calculated after grouping the K1/CM42 population into two classes based on the KASP marker; K1 and CM42 indicate the lines with and without positive alleles of QTsn/Fsn.cib-3D; ∗∗∗ represents significance at P < 0.001.
Figure 4. Effects of QTsn/Fsn.cib-3D on grain number per spike (GNS), spike length (SL), plant height (PH), thousand kernel weight (TKW), grain width (GW), and grain length (GL) in the K1/CM42 population. K1 and CM42 indicate the lines with and without positive alleles of QTsn/Fsn.cib-3D; ∗∗ and ∗∗∗ represent significance at P < 0.01 and P < 0.001, respectively.
Since the favorable allele of QTsn/Fsn.cib-3D was from K1, the other two populations (K1/K4 and K1/YFM2) containing a common parent K1 were used to validate the effects of QTsn/Fsn.cib-3D in different genetic backgrounds. The KASP marker KASP_AX-110914105 closely linked to QTsn/Fsn.cib-3D was used to assess the alleles from the common parent K1. For the K1/K4 population, 27 lines were found to be homozygous for the K1 allele, and the remaining 41 lines were found to be homozygous for the non-K1 allele (Figure 5). For the K1/YFM2 population, 17 plants were found to be homozygous for the K1 allele, 19 plants were found to be homozygous for the non-K1 allele, and the remaining 39 plants were found to be heterozygous allele (Figure 5). Subsequently, Student’s t-test was performed to analyze significant differences on TSN and FSN (P < 0.05) between classes with different allele. As expected, the lines with the homozygous K1 allele had significantly (P < 0.01) higher TSN (5.56–7.96%) and FSN (5.13–9.35%) than those carrying the non-K1 homozygous allele (Figure 5). Moreover, a significant difference on TSN (6.62%) and FSN (7.71%) was also detected between lines with K1 homozygous allele and heterozygous allele (Figure 5).
Figure 5. Validation of QTsn/Fsn.cib-3D in two populations with different genetic environments. Fluorescence PCR typing results of the Kompetitive Allele-Specifc PCR (KASP) marker KASP_AX-110914105 in K1/K4 (A) and K1/YFM2 (D) populations. Orange circle represents lines carrying the allele of K1; blue box represents lines with the allele of K4 (A) and YFM2 (D), respectively. Effects of QTsn/Fsn.cib-3D on total spikelet number per spike (TSN) (B) and fertile spikelet number per spike (FSN) (C) in the K1/K4 population. Effects of QTsn/Fsn.cib-3D on total spikelet number per spike (TSN) (E) and fertile spikelet number per spike (FSN) (F) in the K1/YFM2 population; ∗∗ and ∗∗∗ represent significance at P < 0.01 and P < 0.001, respectively; AA represents the homozygous allele from K1; aa represents the homozygous allele from non-K1; Aa represents the heterozygous allele.
Alignment of the flanking markers of QTsn/Fsn.cib-3D showed that it was corresponding to a physical interval of 549.49–555.2 Mb on chromosome arm 3DL (Figure 6 and Table 4). According to CS reference genome, there were 76 predicated genes in this interval (Figure 6). An analysis of the spatial expression patterns (Borrill et al., 2016; Ramírez-González et al., 2018) showed that 40 genes were expressed in various tissues, and several of them were abundantly expressed in spikes, indicating that they are supposed to be involved in spike development (Figure 6). Among these genes, TraesCS3D02G439000, TraesCS3D02G439200, TraesCS3D02G442000, TraesCS3D02G443900, and TraesCS3D02G445400 are likely associated with spike growth and development according to the gene annotation (Supplementary Table 3). To further analyze potential polymorphism of these candidate genes between K1 and CM42, and K4 and YFM2, we designed gene-specific primers to isolate and resequence the five genes (Supplementary Table 2). For TraesCS3D02G439000, TraesCS3D02G439200, and TraesCS3D02G442000, no sequence variation in coding sequences (cds) between K1 and the other three parents was detected (Supplementary Figures 4–6). For TraesCS3D02G443900, three SNPs in the cds between K1 and the other three parents were detected, and two of them were non-synonymous SNPs (Figure 7 and Supplementary Figure 7). Interestingly, we isolated and assembled the complete coding sequences of TraesCS3D02G445400 from K1 and YFM2 using these designed specific primers. However, only partial coding sequences could be obtained from CM42 and K4 (Supplementary Figure 8). We speculated that significant differences may exist in the sequences of TraesCS3D02G445400 between K1 and CM42 and K4. Based on these sequences, two synonymous SNPs in the coding sequence between K1 and the other three parents, and extra six synonymous SNPs and three non-synonymous SNPs between K1 and YFM2 were detected, respectively (Figure 7 and Supplementary Figure 8).
Figure 6. Expression patterns of genes in the physical interval of QTsn/Fsn.cib-3D in various tissues. Black areas are the genetic interval (A) and physical interval of QTsn/Fsn.cib-3D on chromosome 3D (B); (C) and (D) represent the genes that were expressed in various tissues and their expression patterns.
Figure 7. Sequence analysis of the TraesCS3D02G443900 (A) and TraesCS3D02G445400 (B) showing the single-nucleotide polymorphisms (SNPs) and corresponding amino acid variations between K1 and CM42, and K4 and YFM2. The nucleotide of K1 and the other parents is shown in red and black, respectively. Blue box and gray line on gene structure schematic diagrams represent the exome and intron or UTR, respectively.
Grain number per spike as one of three major components determining wheat yield is ultimately determined by the number of FSN and fertile floret per spikelet. In the present study, by assessing two spikelet number-related traits TSN and FSN in a DH population among five environments, we found that TSN and FSN were significantly and positively correlated with GNS (Table 2) with a high heritability (0.85 for TSN and 0.82 for FSN) (Table 1). The results were consistent with previous knowledge (Che et al., 2018; Würschum et al., 2018; Zhang et al., 2019) that they are environmentally stable yield components and mainly determined by genetic factors. Therefore, increasing spikelet number could be considered as an effective strategy for increasing GNS and hence the grain yield.
Quantitative trait locus analysis is a well-established and widely used tool for dissecting the genetic basis of complex traits, and a high-density genetic map plays a fundamental role in QTL analysis (Salvi and Tuberosa, 2005; Cooper et al., 2009; Yamamoto et al., 2009). In the present study, by using the wheat 55K SNP array, a high-density genetic map containing 13,068 SNPs with a total length of 3,091.39 cM was constructed. The marker order was relatively consistent with that in the wheat genome assembly on most of the chromosomes (Supplementary Table 1 and Supplementary Figure 1). It is well known that the SNP markers in the Wheat 55K SNP array were well chosen from the 660K SNP array. Compared with previously reported genetic maps, we extracted 2,739 and 4,860 common mapped markers from the Wheat 55K and 660K SNP array, respectively (Supplementary Table 4) (Cui et al., 2017; Liu et al., 2018a). The genetic positions of these markers were relatively consistent in the three genetic maps (Supplementary Figures 2, 3), indicating that the genetic map constructed in present study was accurate and credible and could be effectively used for QTL analysis.
Over the past decades, QTLs that control spikelet number have been extensively studied and identified on almost every wheat chromosome. In this study, five stable QTLs for TSN and FSN were detected on chromosomes 2D, 3D, 5A, and 7D. Of them, QTsn/Fsn.cib-3D associated with TSN and FSN was detected as a major QTL for spikelet number. This QTL was mapped in a 4.455-cM interval and physically corresponding to 549.49 and 555.2 Mb on chromosome arm 3DL according to the physical location of flanking markers (Table 4 and Figure 3). As far as we know, several QTLs for spikelet number on chromosome 3D including QSns.sau-3D, QSsn.czm-3D, QSspn.cau-3D, and QSpn.ipk-3D had been reported in previous studies. QSsn.czm-3D and QSspn.cau-3D were located on the short arm of chromosome 3D flanked by 3DS_2577014_1698-IWA4559 and Xcau.3D-5-Xcau.3D-6, respectively (Cui et al., 2012; Chen et al., 2020). QSns.sau-3D detected by Luo et al. (2016) was located between gpw3109 (306.5 Mb) and gdm8 (357.1 Mb). Given that the physical intervals of these QTLs were different from those of the QTsn/Fsn.cib-3D, the QTsn/Fsn.cib-3D may be different from these loci. The remaining QTL, QSpn.ipk-3D, detected by Pestsova et al. (2006) was located between Xgwm383 (532.19 Mb) and Xgwm3 (579.89 Mb). Although the QTL seems overlapped with QTsn/Fsn.cib-3D, it was a minor QTL detected in one environment, explaining only 3.9% of the phenotypic variance. These results indicated that QTsn/Fsn.cib-3D is probably a novel and major QTL for spikelet number. Additionally, the rest three stable QTLs QFsn.cib-7D, QTsn.cib-2D, and QFsn.cib-5A in present study were overlapped or close to QTLs for spikelet number reported previously, indicating they are likely alleles. For example, QFsn.cib-7D was located between 66.54 and 90.62 Mb on chromosome arm 7DS. This interval was overlapped with QTspn.cau-7D and QFspn.cau-7D reported by Chen et al. (2020). QTsn.cib-2D mapped in the physical interval of 534.32–648.11 Mb on chromosome arm 2DL was overlapped with QTssn.WJ.2D.2 and QFsn.WY.2D (Cui et al., 2012). QFsn.cib-5A mapped between 455.42 and 459.97 Mb on chromosome arm 5AL was close to QFsn.WY.5A.2 detected by Cui et al. (2012).
Similar to previous studies (Chen et al., 2020; Cui et al., 2012), TSN is significantly and positively correlated with FSN. Thus, QTLs, especially the major QTLs, for TSN and FSN were usually identified in same locus. For instance, seven stable QTLs for spikelet number were identified by Chen et al. (2020), and five of them were associated with TSN and FSN simultaneously. The loci of QTsn/Fsn.czm-1A and QTsn/Fsn.czm-1D were identified by Zhou et al. (2017), showing significant effects on TSN and FSN. A QTL cluster for FSN and TSN identified on chromosome 5D was located in the same region as that detected by Li et al. (2007) and Cui et al. (2012). Moreover, as one of the subcomponent of GNS, an increase of spikelet number could directly increase GNS (Hai et al., 2008). Therefore, it is not surprising that the major QTL QTsn/Fsn.cib-3D identified in present study showed significant and positive effects on TSN, FSN, and GNS (Figures 4, 5). Additionally, we further analyzed the effects of QTsn/Fsn.cib-3D on other yield-related traits including TKW, SL, PH, GW, and GL in the K1/CM42 populations. QTsn/Fsn.cib-3D showed moderate negative influence on GL, but no influence on TKW, PH, SL, and GW (Figure 4). These results suggested that QTsn/Fsn.cib-3D combined with developed KASP markers has potential application values in wheat breeding.
In the interval of QTsn/Fsn.cib-3D, there are 76 confidence genes in CS (Supplementary Table 3). Based on the expression analysis and gene annotation, five genes TraesCS3D02G439000, TraesCS3D02G439200, TraesCS3D02G442000, TraesCS3D02G443900, and TraesCS3D02G445400 are abundantly expressed in spikes, indicating they are likely associated with spike growth and development (Figure 6). Of them, TraesCS3D02G445400 encodes a glutathione S-transferase, which has been reported to be related to panicle and spikelet numbers, and plays an important role during the growth and development processes in rice (Kim et al., 2013; Hu et al., 2014). TraesCS3D02G442000 encodes an auxin response factor. Previous studies have revealed that auxin signal transduction acts critical roles in modulating various biological processes including growth, development, and stress resistance (Qiao et al., 2018; Brauer et al., 2019). TraesCS3D02G439200 is an ortholog of OsHAK5 of the rice and encodes a potassium transporter (Supplementary Figure 9). OsHAK5 plays a crucial role in maintaining rice architecture including PH, root, and tiller by altering cellular chemiosmotic gradients and regulating ATP-dependent polar auxin transport (Yang et al., 2020). TraesCS3D02G439000 encodes an F-box family protein, which is known to be involved in the vegetative and reproductive growth and development of many plants (Van Den Burg et al., 2008; Ma et al., 2019). TraesCS3D02G443900 encodes a BTB domain-containing protein, which was reported to be involved in multiple functions including floral organ development and disease resistance in Arabidopsis, rice, maize, and tomato (Gingerich et al., 2005; Chen et al., 2014; Chern et al., 2014; Xu et al., 2016). To further explore the candidate gene, we isolated and assembled the coding sequences of the five genes from four parents. Sequence alignment showed that two non-synonymous SNPs and one synonymous SNP in coding sequence of TraesCS3D02G443900 were detected between K1 and CM42, and K4 and YFM2; two synonymous SNPs in the coding sequence of TraesCS3D02G445400 were found between K1 and CM42, and K4 and YFM2; and the extra six synonymous SNPs and three non-synonymous SNPs were found between K1 and YFM2, respectively (Figure 7). For the rest three genes TraesCS3D02G439000, TraesCS3D02G439200, and TraesCS3D02G442000, no sequence variation in coding sequences was simultaneously detected between K1 and the other three parents (Supplementary Figures 4, 5, 6). Thus, TraesCS3D02G443900 and TraesCS3D02G445400 could be considered as a focus for further work on fine mapping and gene cloning.
The original contributions presented in the study are included in the article/Supplementary Material, further inquiries can be directed to the corresponding author/s.
TL undertook the field trials and subsequent analyses of all available data, including the phenotyping and population genotyping, and drafted this manuscript. GD assisted in the field trials. YT, YS, JW, JC, ZY, XQ, XP, HZ, and JL participated in phenotype measurement. MY discussed results and revised the manuscript. HL and YW designed the experiments, guided the entire study, participated in the data analysis, discussed the results, and revised the manuscript. All authors read and reviewed the manuscript.
This work is supported by the Strategic Priority Research Program of the Chinese Academy of Sciences (Grant No. XDA08020205), National Key R&D Program of China (2016YFD0100102), Science and Technology Support Project of Sichuan Province, China (2016NZ0103), and Key Project of Crop Breeding of Sichuan Province (2016NYZ0030).
The authors declare that the research was conducted in the absence of any commercial or financial relationships that could be construed as a potential conflict of interest.
We thank Prof. Lifeng Gao of Institute of Crop Sciences, Chinese Academy of Agricultural Sciences, for providing us the sequence information of wheat 55K arrays for KASP marker development. The Triticeae Multi-omics Center (http://202.194.139.32/) is also acknowledged for providing an integrated platform of tools and genomic data, bringing great convenience to our work.
The Supplementary Material for this article can be found online at: https://www.frontiersin.org/articles/10.3389/fpls.2021.611106/full#supplementary-material
Brauer, E. K., Rocheleau, H., Balcerzak, M., Pan, Y. L., Fauteux, F., Liu, Z. Y., et al. (2019). Transcriptional and hormonal profiling of Fusarium graminearum-infected wheat reveals an association between auxin and susceptibility. Physiol. Mol. Plant Pathol. 107, 33–39. doi: 10.1016/j.pmpp.2019.04.006
Borrill, P., Ramirez-Gonzalez, R., and Uauy, C. (2016). expVIP: a customizable RNA-seq data analysis and visualization platform. Plant Physiol. 170, 2172–2186. doi: 10.1104/pp.15.01667
Butterworth, M. H., Semenov, M. A., Barnes, A., Moran, D., West, J. S., and Fitt, B. D. L. (2010). North-South divide: contrasting impacts of climate change on crop yields in Scotland and England. J. R. Soc. Interf. 7, 123–130. doi: 10.1098/rsif.2009.0111
Ceccarelli, S., Grando, S., Maatougui, M., Michael, M., Slash, M., Haghparast, R., et al. (2010). Plant breeding and climate changes. J. Agric. Sci. 148, 627–637. doi: 10.1017/S0021859610000651
Che, Y. H., Song, N., Yang, Y. P., Yang, X. M., Duan, Q. Q., Zhang, Y., et al. (2018). QTL Mapping of six spike and stem traits in hybrid population of agropyron gaertn. in multiple environments. Front. Plant Sci. 9:11. doi: 10.3389/fpls.2018.01422
Chen, Z., Cheng, X., Chai, L., Wang, Z., Du, D., Wang, Z., et al. (2020). Pleiotropic QTL influencing spikelet number and heading date in common wheat (Triticum aestivum L.). Theor. Appl. Genet. 133, 1825–1838. doi: 10.1007/s00122-020-03556-6
Chen, Z., Wang, B., Dong, X., Liu, H., Ren, L., Chen, J., et al. (2014). An ultra-high density bin-map for rapid QTL mapping for tassel and ear architecture in a large F2 maize population. BMC Genom. 15:433. doi: 10.1186/1471-2164-15-433
Chern, M., Bai, W., Ruan, D., Oh, T., Chen, X., and Ronald, P. C. (2014). Interaction specificity and coexpression of rice NPR1 homologs 1 and 3 (NH1 and NH3), TGA transcription factors and Negative Regulator of Resistance (NRR) proteins. BMC Genom. 15:461. doi: 10.1186/1471-2164-15-461
Cooper, M. E., Van Eeuwijk, F. A., Hammer, G. L., Podlich, D. W., and Messina, C. (2009). Modeling QTL for complex traits: detection and context for plant breeding. Curr. Opin. Plant Biol. 12, 231–240. doi: 10.1016/j.pbi.2009.01.006
Cui, F., Ding, A., Li, J., Zhao, C., Wang, L., Wang, X., et al. (2012). QTL detection of seven spike-related traits and their genetic correlations in wheat using two related RIL populations. Euphytica 186, 177–192.
Cui, F., Zhang, N., Fan, X., Zhang, W., Zhao, C. H., Yang, L. J., et al. (2017). Utilization of a Wheat660K SNP array-derived high-density genetic map for high-resolution mapping of a major QTL for kernel number. Sci. Rep. 7:3788.
Debernardi, J. M., Lin, H., Chuck, G. S., Faris, J. D., and Dubcovsky, J. (2017). microRNA172 plays a crucial role in wheat spike morphogenesis and grain threshability. Development 144, 1966–1975.
Deng, Z. Y., Cui, Y., Han, Q. D., Fang, W. Q., Li, J. F., and Tian, J. C. (2017). Discovery of consistent QTLs of wheat spike-related traits under nitrogen treatment at different development stages. Front. Plant Sci. 8:16. doi: 10.3389/fpls.2017.02120
Dobrovolskaya, O., Pont, C., Sibout, R., Martinek, P., Badaeva, E. D., Murat, F., et al. (2015). Frizzy panicle drives supernumerary spikelets in bread wheat. Plant Physiol. 167, 189–199. doi: 10.1104/pp.114.250043
Evans, N., Baierl, A., Semenov, M. A., Gladders, P., and Fitt, B. D. L. (2008). Range and severity of a plant disease increased by global warming. J. R. Soc. Interf. 5, 525–531. doi: 10.1098/rsif.2007.1136
Faris, J. D., Fellers, J. P., Brooks, S. A., and Gill, B. S. (2003). A bacterial artificial chromosome contig spanning the major domestication locus Q in wheat and identification of a candidate gene. Genetics 164, 311–321.
Gao, F. M., Wen, W., Liu, J. D., Rasheed, A., Yin, G. H., Xia, X. C., et al. (2015). Genome-wide linkage mapping of QTL for yield components, plant height and yield-related physiological traits in the Chinese wheat cross zhou 8425B/Chinese Spring. Front. Plant Sci. 6:1099. doi: 10.3389/fpls.2015.01099
Gao, X., Wang, N., Wang, X., and Zhang, X. S. (2019). Architecture of wheat inflorescence: insights from rice. Trends Plant Sci. 24, 802–809. doi: 10.1016/j.tplants.2019.06.002
Gingerich, D. J., Gagne, J. M., Salter, D. W., Hellmann, H., Estelle, M., Ma, L., et al. (2005). Cullins 3a and 3b assemble with members of the broad complex/tramtrack/ bric-a-brac (BTB) protein family to form essential ubiquitin-protein ligases (E3s) in arabidopsis. J. Biol. Chem. 280, 18810–18821. doi: 10.1074/jbc.M413247200
Golan, G., Ayalon, I., Perry, A., Zimran, G., Ade-Ajayi, T., Mosquna, A., et al. (2019). GNI-A1 mediates trade-off between grain number and grain weight in tetraploid wheat. Theor. Appl. Genet. 132, 2353–2365. doi: 10.1007/s00122-019-03358-5
Greenwood, J. R., Finnegan, E. J., Watanabe, N., Trevaskis, B., and Swain, S. M. (2017). New alleles of the wheat domestication gene Q reveal multiple roles in growth and reproductive development. Development 144, 1959–1965.
Guo, Z., and Schnurbusch, T. (2015). Variation of floret fertility in hexaploid wheat revealed by tiller removal. J. Exp. Bot. 66, 5945–5958. doi: 10.1093/jxb/erv303
Guo, Z. F., Chen, D. J., Alqudah, A. M., Roder, M. S., Ganal, M. W., and Schnurbusch, T. (2017). Genome-wide association analyses of 54 traits identified multiple loci for the determination of floret fertility in wheat. N. Phytol. 214, 257–270. doi: 10.1111/nph.14342
Hai, L., Guo, H., Wagner, C., Xiao, S., and Friedt, W. (2008). Genomic regions for yield and yield parameters in Chinese winter wheat (Triticum aestivum L.) genotypes tested under varying environments correspond to QTL in widely different wheat materials. Plant Sci. 175, 226–232. doi: 10.1016/j.plantsci.2008.03.006
Hanif, M., and Langer, R. H. M. (1972). The vascular system of the spikelet in wheat (Triticum aestivum). Ann. Bot. 36, 721–727. doi: 10.1093/oxfordjournals.aob.a084628
Hu, T., Yang, Y., Tan, L., Yang, J., and Wu, Y. (2014). Molecular characteristics, recombinant expression and activity detection of OsGSTL1 from rice. Braz. Arch. Biol. Technol. 57, 70–76. doi: 10.1590/S1516-89132014000100011
Huang, X., Qian, Q., Liu, Z., Sun, H., He, S., Luo, D., et al. (2009). Natural variation at the DEP1 locus enhances grain yield in rice. Nat. Genet. 41, 494–497.
Jiang, Y. F., Chen, Q., Wang, Y., Guo, Z. R., Xu, B. J., Zhu, J., et al. (2019). Re-acquisition of the brittle rachis trait via a transposon insertion in domestication gene Q during wheat de-domestication. N. Phytol. 224, 550–551. doi: 10.1111/nph.15977
Kim, Y. S., Kim, I. S., Bae, M. J., Choe, Y. H., Kim, Y. H., Park, H. M., et al. (2013). Homologous expression of cytosolic dehydroascorbate reductase increases grain yield and biomass under paddy field conditions in transgenic rice (Oryza sativa L. japonica). Planta 237, 1613–1625. doi: 10.1007/s00425-013-1862-8
Koppolu, R., and Schnurbusch, T. (2019). Developmental pathways for shaping spike inflorescence architecture in barley and wheat. J. Integr. Plant Biol. 61, 278–295. doi: 10.1111/jipb.12771
Kuang, C. H., Zhao, X. F., Yang, K., Zhang, Z. P., Ding, L., Pu, Z. E., et al. (2020). Mapping and characterization of major QTL for spike traits in common wheat. Physiol. Mol. Biol. Plants 26, 1295–1307. doi: 10.1007/s12298-020-00823-0
Kuzay, S., Xu, Y., Zhang, J., Katz, A., Pearce, S., Su, Z., et al. (2019). Identification of a candidate gene for a QTL for spikelet number per spike on wheat chromosome arm 7AL by high-resolution genetic mapping. Theor. Appl. Genetics 132, 2689–2705. doi: 10.1007/s00122-019-03382-5
Li, S., Jia, J., Wei, X., Zhang, X., Li, L., Chen, H., et al. (2007). A intervarietal genetic map and QTL analysis for yield traits in wheat. Mol. Breed. 20, 167–178. doi: 10.1007/s11032-007-9080-3
Liu, J. J., Luo, W., Qin, N. N., Ding, P. Y., Zhang, H., Yang, C. C., et al. (2018a). A 55 K SNP array-based genetic map and its utilization in QTL mapping for productive tiller number in common wheat. Theor. Appl. Genet. 131, 2439–2450. doi: 10.1007/s00122-018-3164-9
Liu, K., Sun, X. X., Ning, T. Y., Duan, X. X., Wang, Q. L., Liu, T. T., et al. (2018b). Genetic dissection of wheat panicle traits using linkage analysis and a genome-wide association study. Theor. Appl. Genet. 131, 1073–1090. doi: 10.1007/s00122-018-3059-9
Liu, T., Wu, L., Gan, X., Chen, W., Liu, B., Fedak, G., et al. (2020). Mapping quantitative trait loci for 1000-grain weight in a double haploid population of common wheat. Int. J. Mol. Sci. 21:3960. doi: 10.3390/ijms21113960
Luo, W., Ma, J., Zhou, X. H., Sun, M., Kong, X. C., Wei, Y. M., et al. (2016). Identification of quantitative trait loci controlling agronomic traits indicates breeding potential of tibetan semiwild wheat (Triticum aestivum ssp. tibetanum). Crop Sci. 56, 2410–2420. doi: 10.2135/cropsci2015.11.0700
Ma, J., Ding, P. Y., Liu, J. J., Li, T., Zou, Y. Y., Habib, A., et al. (2019). Identification and validation of a major and stably expressed QTL for spikelet number per spike in bread wheat. Theor. Appl. Genet. 132, 3155–3167. doi: 10.1007/s00122-019-03415-z
Ma, Z. Q., Zhao, D. M., Zhang, C. Q., Zhang, Z. Z., Xue, S. L., Lin, F., et al. (2007). Molecular genetic analysis of five spike-related traits in wheat using RIL and immortalized F2 populations. Mol. Genet. Genom. 277, 31–42. doi: 10.1007/s00438-006-0166-0
Meng, L., Li, H. H., Zhang, L. Y., and Wang, J. K. (2015). QTL IciMapping: integrated software for genetic linkage map construction and quantitative trait locus mapping in biparental populations. Crop. J. 3, 269–283. doi: 10.1016/j.cj.2015.01.001
Muqaddasi, Q. H., Jayakodi, M., Borner, A., and Roder, M. S. (2019). Identification of consistent QTL with large effect on anther extrusion in doubled haploid populations developed from spring wheat accessions in German Federal ex situ Genebank. Theor. Appl. Genet. 132, 3035–3045. doi: 10.1007/s00122-019-03404-2
Okada, T., Jayasinghe, J. E. A. R. M., Eckermann, P., Watson-Haigh, N. S., Warner, P., Hendrikse, Y., et al. (2019). Effects of Rht-B1 and Ppd-D1 loci on pollinator traits in wheat. Theor. Appl. Genet. 132, 1965–1979. doi: 10.1007/s00122-019-03329-w
Pestsova, E. G., Börner, A., and Röder, M. S. (2006). Development and QTL assessment of Triticum aestivum-Aegilops tauschii introgression lines. Theor. Appl. Genet. 112, 634–647. doi: 10.1007/s00122-005-0166-1
Qiao, L. Y., Zhang, W. P., Li, X. Y., Zhang, L., Zhang, X. J., Li, X., et al. (2018). Characterization and expression patterns of auxin response factors in wheat. Front. Plant Sci. 9:13. doi: 10.3389/fpls.2018.01395
Ramírez-González, R. H., Borrill, P., Lang, D., Harrington, S. A., Brinton, J., Venturini, L., et al. (2018). The transcriptional landscape of polyploid wheat. Science 80:361. doi: 10.1126/science.aar6089
Sakuma, S., Golan, G., Guo, Z., Ogawa, T., Tagiri, A., Sugimoto, K., et al. (2019). Unleashing floret fertility in wheat through the mutation of a homeobox gene. Proc. Natl. Acad. Sci. U.S.A. 116, 5182–5187. doi: 10.1073/pnas.1815465116
Salvi, S., and Tuberosa, R. (2005). To clone or not to clone plant QTLs: present and future challenges. Trends Plant Sci. 10, 297–304. doi: 10.1016/j.tplants.2005.04.008
Simons, K., Fellers, J. P., Trick, H. N., Zhang, Z., Tai, Y., Gill, B. S., et al. (2006). Molecular characterization of the major wheat domestication gene Q. Genetics 172, 547–555. doi: 10.1534/genetics.105.044727
Smith, S. E., Kuehl, R. O., Ray, I. M., Hui, R., and Soleri, D. (1998). Evaluation of simple methods for estimating broad-sense heritability in stands of randomly planted genotypes. Crop. Sci. 38, 1125–1129.
Van Den Burg, H. A., Tsitsigiannis, D. I., Rowland, O., Lo, J., Rallapalli, G., MacLean, D., et al. (2008). The F-box protein ACRE189/ACIF1 regulates cell death and defense responses activated during pathogen recognition in tobacco and tomato. Plant Cell 20, 697–719. doi: 10.1105/tpc.107.056978
Vossfels, K. P., Keeblegagnere, G., Hickey, L. T., Tibbits, J., Nagornyy, S., Hayden, M. J., et al. (2019). High-resolution mapping of rachis nodes per rachis, a critical determinant of grain yield components in wheat. Theor. Appl. Genet. 132, 2707–2719. doi: 10.1007/s00122-019-03383-4
Wang, R., Liu, Y. X., Isham, K., Zhao, W. D., Wheeler, J., Klassen, N., et al. (2018). QTL identification and KASP marker development for productive tiller and fertile spikelet numbers in two high-yielding hard white spring wheat cultivars. Mol. Breed. 38:12. doi: 10.1007/s11032-018-0894-y
Wolde, G. M., Trautewig, C., Mascher, M., and Schnurbusch, T. (2019). Genetic insights into morphometric inflorescence traits of wheat. Theor. Appl. Genet. 132, 1661–1676. doi: 10.1007/s00122-019-03305-4
Würschum, T., Leiser, W. L., Langer, S. M., Tucker, M. R., and Longin, C. F. H. (2018). Phenotypic and genetic analysis of spike and kernel characteristics in wheat reveals long-term genetic trends of grain yield components. Theor. Appl. Genet. 131, 2071–2084. doi: 10.1007/s00122-018-3133-3
Xu, C., Park, S. J., Van Eck, J., and Lippman, Z. B. (2016). Control of inflorescence architecture in tomato by BTB/POZ transcriptional regulators. Genes Dev. 30, 2048–2061. doi: 10.1101/gad.288415.116
Yamamoto, T., Yonemaru, J., and Yano, M. (2009). Towards the understanding of complex traits in rice: substantially or superficially? DNA Res. 16, 141–154. doi: 10.1093/dnares/dsp006
Yang, Q., Zhang, D. F., and Xu, M. L. (2012). A sequential quantitative trait locus fine-mapping strategy using recombinant-derived progeny. J. Integr. Plant Biol. 54, 228–237. doi: 10.1111/j.1744-7909.2012.01108.x
Yang, T., Feng, H., Zhang, S., Xiao, H., Hu, Q., Chen, G., et al. (2020). The potassium transporter OsHAK5 alters rice architecture via ATP-dependent transmembrane auxin fluxes. Plant Commun. 1:100052. doi: 10.1016/j.xplc.2020.100052
Yang, W., Liu, D., Li, J., Zhang, L., Wei, H., Hu, X., et al. (2009). Synthetic hexaploid wheat and its utilization for wheat genetic improvement in China. J. Genet. Genomics 36, 539–546. doi: 10.1016/S1673-8527(08)60145-9
Yao, H. N., Xie, Q., Xue, S. L., Luo, J., Lu, J. K., Kong, Z. X., et al. (2019). HL2 on chromosome 7D of wheat (Triticum aestivum L.) regulates both head length and spikelet number. Theor. Appl. Genet. 132, 1789–1797. doi: 10.1007/s00122-019-03315-2
Yu, M., Chen, G. Y., Zhang, L. Q., Liu, Y. X., Liu, D. C., Wang, J. R., et al. (2014). QTL Mapping for important agronomic traits in synthetic hexaploid wheat derived from Aegiliops tauschii ssp tauschii. J. Integr. Agric. 13, 1835–1844. doi: 10.1016/S2095-3119(13)60655-3
Zhai, H. J., Feng, Z. Y., Li, J., Liu, X. Y., Xiao, S. H., Ni, Z. F., et al. (2016). QTL analysis of spike morphological traits and plant height in winter wheat (Triticum aestivum L.) using a high-density SNP and SSR-based linkage map. Front. Plant Sci. 7:1617. doi: 10.3389/fpls.2016.01617
Zhang, B., Liu, X., Xu, W. N., Chang, J. Z., Li, A., Mao, X. G., et al. (2015). Novel function of a putative MOC1 ortholog associated with spikelet number per spike in common wheat. Sci. Rep. 5, 12211–12211.
Zhang, J. L., Gizaw, S. A., Bossolini, E., Hegarty, J., Howell, T., Carter, A. H., et al. (2018). Identification and validation of QTL for grain yield and plant water status under contrasting water treatments in fall-sown spring wheats. Theor. Appl. Genet. 131, 1741–1759. doi: 10.1007/s00122-018-3111-9
Zhang, Z., Han, H. M., Liu, W. H., Song, L. Q., Zhang, J. P., Zhou, S. H., et al. (2019). Deletion mapping and verification of an enhanced-grain number per spike locus from the 6PL chromosome arm of Agropyron cristatum in common wheat. Theor. Appl. Genet. 132, 2815–2827. doi: 10.1007/s00122-019-03390-5
Keywords: wheat (Triticum aestivum L.), QTL, linkage analysis, spikelet number, KASP, yield
Citation: Li T, Deng G, Tang Y, Su Y, Wang J, Cheng J, Yang Z, Qiu X, Pu X, Zhang H, Liang J, Yu M, Wei Y and Long H (2021) Identification and Validation of a Novel Locus Controlling Spikelet Number in Bread Wheat (Triticum aestivum L.). Front. Plant Sci. 12:611106. doi: 10.3389/fpls.2021.611106
Received: 28 September 2020; Accepted: 29 January 2021;
Published: 26 February 2021.
Edited by:
Sean Mayes, University of Nottingham, United KingdomReviewed by:
Antonio Blanco, University of Bari Aldo Moro, ItalyCopyright © 2021 Li, Deng, Tang, Su, Wang, Cheng, Yang, Qiu, Pu, Zhang, Liang, Yu, Wei and Long. This is an open-access article distributed under the terms of the Creative Commons Attribution License (CC BY). The use, distribution or reproduction in other forums is permitted, provided the original author(s) and the copyright owner(s) are credited and that the original publication in this journal is cited, in accordance with accepted academic practice. No use, distribution or reproduction is permitted which does not comply with these terms.
*Correspondence: Hai Long, aGFpbG9uZ0BjaWIuYWMuY24=; Yuming Wei, eW13ZWlAc2ljYXUuZWR1LmNu
Disclaimer: All claims expressed in this article are solely those of the authors and do not necessarily represent those of their affiliated organizations, or those of the publisher, the editors and the reviewers. Any product that may be evaluated in this article or claim that may be made by its manufacturer is not guaranteed or endorsed by the publisher.
Research integrity at Frontiers
Learn more about the work of our research integrity team to safeguard the quality of each article we publish.