- 1Key Laboratory for Plant Diversity and Biogeography of East Asia, Kunming Institute of Botany, CAS, Kunming, China
- 2University of Chinese Academy of Sciences, Beijing, China
The genetic architecture within a species in the Himalaya-Hengduan Mountains (HHM) region was considered as the consolidated consequence of historical orogenesis and climatic oscillations. The visualization of dispersal corridors as the function of population genetic connectivity became crucial to elucidate the spatiotemporal dynamics of organisms. However, geodiversity and physical barriers created by paleo geo-climatic events acted vigorously to impact notable alterations in the phylogeographic pattern and dispersal corridors. Therefore, to achieve detailed phylogeography, locate dispersal corridors and estimate genetic connectivity, we integrated phylogeography with species distribution modelling and least cost path of Mirabilis himalaica (Edgew.) Heimerl in the HHM. We amplified four cpDNA regions (petL-psbE, rps16-trnK, rps16 intron, trnS-trnG), and a low copy nuclear gene (G3pdh) from 241 individuals of 29 populations. SAMOVA, genealogical relationships, and phylogenetic analysis revealed four spatially structured phylogroups for M. himalaica with the onset of diversification in late Pliocene (c. 3.64 Ma). No recent demographic growth was supported by results of neutrality tests, mismatch distribution analysis and Bayesian skyline plot. Paleo-distribution modelling revealed the range dynamics of M. himalaica to be highly sensitive to geo-climatic change with limited long-distance dispersal ability and potential evolutionary adaptation. Furthermore, river drainage systems, valleys and mountain gorges were identified as the corridors for population genetic connectivity among the populations. It is concluded that recent intense mountain uplift and subsequent climatic alterations including monsoonal changes since Pliocene or early Pleistocene formulated fragmented habitats and diverse ecology that governed the habitat connectivity, evolutionary and demographic history of M. himalaica. The integrative genetic and geospatial method would bring new implications for the evolutionary process and conservation priority of HHM endemic species.
Introduction
The Himalaya and the Hengduan Mountains (HHM) are one of the main biodiversity hotspots of the Northern Hemisphere formed as a result of the collision between the Indian and Eurasian plate, started c. 55–50 million years ago (Ma) (Yin and Harrison, 2000; Dupont-Nivet et al., 2010). Mulch and Chamberlain (2006) suggests that the orogeny of the Hengduan Mountains occurred as a final propagation of the uplift after late Miocene (c. 10 Ma). In addition, recent violent Qinghai-Tibetan Plateau (QTP) uplifts (c. 3.6–1.5 Ma), and associated intensification of the Asian monsoon (c. 2.6–3.6 Ma) caused dramatic changes in the biodiversity of HHM and QTP regions alongside alterations in regional landforms and environmental heterogeneity (Li et al., 1979; Li and Fang, 1999; An et al., 2001).
The incredible biodiversity and genetic architecture in the HHM region were considered as the aftereffect of historical orogenesis independently or in combination with climatic oscillations (Avise, 2009; Favre et al., 2015). Moreover, the geological and climatic factors enormously influence the spatiotemporal pattern of temperate plant species, including historical dispersal and gene flow among the populations (Yu et al., 2015). Such orogenic uplift of mountains results in the formation of the geographical barriers, leading to fragmented habitat, loss of dispersal corridors, and restricted gene flow among populations; such factors furnish circumstances for divergence owing to genetic drift and natural selection (Liu et al., 2006; Meng et al., 2017). Historical dispersal process is likely to be a key factor to determine the current spatial population structure of species affected by the geological and climatic alterations (Hewitt, 2000). Therefore, it is crucial to locate historical dispersal for a better understanding of the demographic and evolutionary history of species, as an outcome of paleo geo-climatic events (Brown and Yoder, 2015).
Phylogeographic approach integrated with ensemble species distribution modelling (SDM) analysis provides comprehension of how paleo-environmental alterations in landscape and climate have governed species distributions and demographical history (Avise, 2000; Wang and Yan, 2014). In addition, landscape genetics has been widely used for modelling the dispersal corridors of species (Wang et al., 2009; Yu et al., 2017). Least cost path (LCP) uses landscape genetic approach coupled with species distribution models and population genetic data to recognize population genetic connectivity in a spatially explicit framework (Chan et al., 2011; Yu et al., 2015). LCP contributes to the most suitable habitat and fewest movement barriers providing the best theoretical route for dispersal in organisms (Larkin et al., 2004).
Over the last decade, a number of phylogeographic studies have investigated the link between organismic evolution of plant species and the geologic uplift associated with climate changes in the HHM and QTP (Qiu et al., 2011; Jian et al., 2015; Luo et al., 2016). For instance, phylogeographic studies on Primula tibetica related to the effects of Quaternary climatic oscillations in QTP (Ren et al., 2017a), Metrocoris sichuanensis concerned with geological effects in Sichuan Basin (Ye et al., 2018), and Allium section Sikkimensia linked with Hengduan Mountains massif uplift (Xie et al., 2019). They have demonstrated that the dramatic uplift independently or in combination with Pleistocene glaciations influenced their patterns of genetic variation. However, few studies have compared the relative significance of geo-climatic mechanisms influencing the historical dispersal and the population genetic connectivity in this region (Rana et al., 2019).
The present study focuses on the HHM endemic Mirabilis himalaica (Nyctaginaceae) that distributes from the Western Himalaya to the Hengduan Mountains that stretch from N India, WC Nepal, Bhutan, and S Xizang, SW Gansu, N Sichuan, NW Yunnan in the HHM region (Lu et al., 2003; Wang et al., 2019). The genus Mirabilis L. constitutes ~60 spp., predominantly in temperate and tropical North America and South America and single species in Asia (M. himalaica; Lu et al., 2003; Spellenberg, 2003; Wang et al., 2019). Mirabilis himalaica grows in thickets, grasslands, dry and warm river valleys between 1,400–3,800 m (Lu et al., 2003; Wang et al., 2019). For the present work, we hypothesize that the geological events to be the most important driver, if the divergence occurs before mid-Pleistocene with relatively old divergence time. We further presumed that the Pleistocene climate along with geological events greatly influenced the lineage colonization and spatiotemporal distribution of the M. himalaica. Therefore, we set the following primary goals for our research: 1) to identify genetic diversity and phylogeographic structure; 2) to date the divergence time between lineages and locate population genetic connectivity during the late Quaternary in the HHM region; 3) and to elucidate how paleo geological change and climatic oscillations have affected comprehensive evolutionary and demographic history of M. himalaica. This study represents the integrative approach of maternally inherited (cpDNA) and biparentally inherited (G3pdh) population genetic data in combination with the SDM and LCP approach throughout the distribution range of species to outreach the role played by the historical processes on the present-day spatial population structure of organisms in the HHM.
Materials and Methods
Sampling, DNA Extraction, PCR Amplification, and Sequencing
In total, 241 individuals, from 29 populations covering the possible geographical range i.e. from the Western Himalaya to the Hengduan Mountains were sampled for the phylogeographic study of Mirabilis himalaica (Figure 1 and Supplementary Table S1). The fresh sampled leaves of 6–10 individuals from each population (Table 1) were at least 30 m distance apart and dried in silica gel. Voucher specimens were deposited at National Herbarium and Plant Laboratories (KATH), Nepal, and Kunming Institute of Botany (KUN), China. Total genomic DNA was extracted from c. 30 mg silica dried leaves using a DNAsecure Plant Kit (Tiangen Biotechnology Co. Ltd., Beijing, China) following the manufacturer's protocol. After preliminary screening, we amplified four cpDNA regions i.e. petL-psbE, rps16-trnK, rps16 intron, and trnS-trnG, and a low copy nuclear gene i.e. G3pdh for each individual. PCRs were conducted in a 30 µl containing 2 µl DNA template (10–50 ng/µl), 15 µl 2x Taq Plus Master Mix with dye (Tiangen Biotech.), 1 µl 10 µM of each primer (see Supplementary Methods S1 for detail).
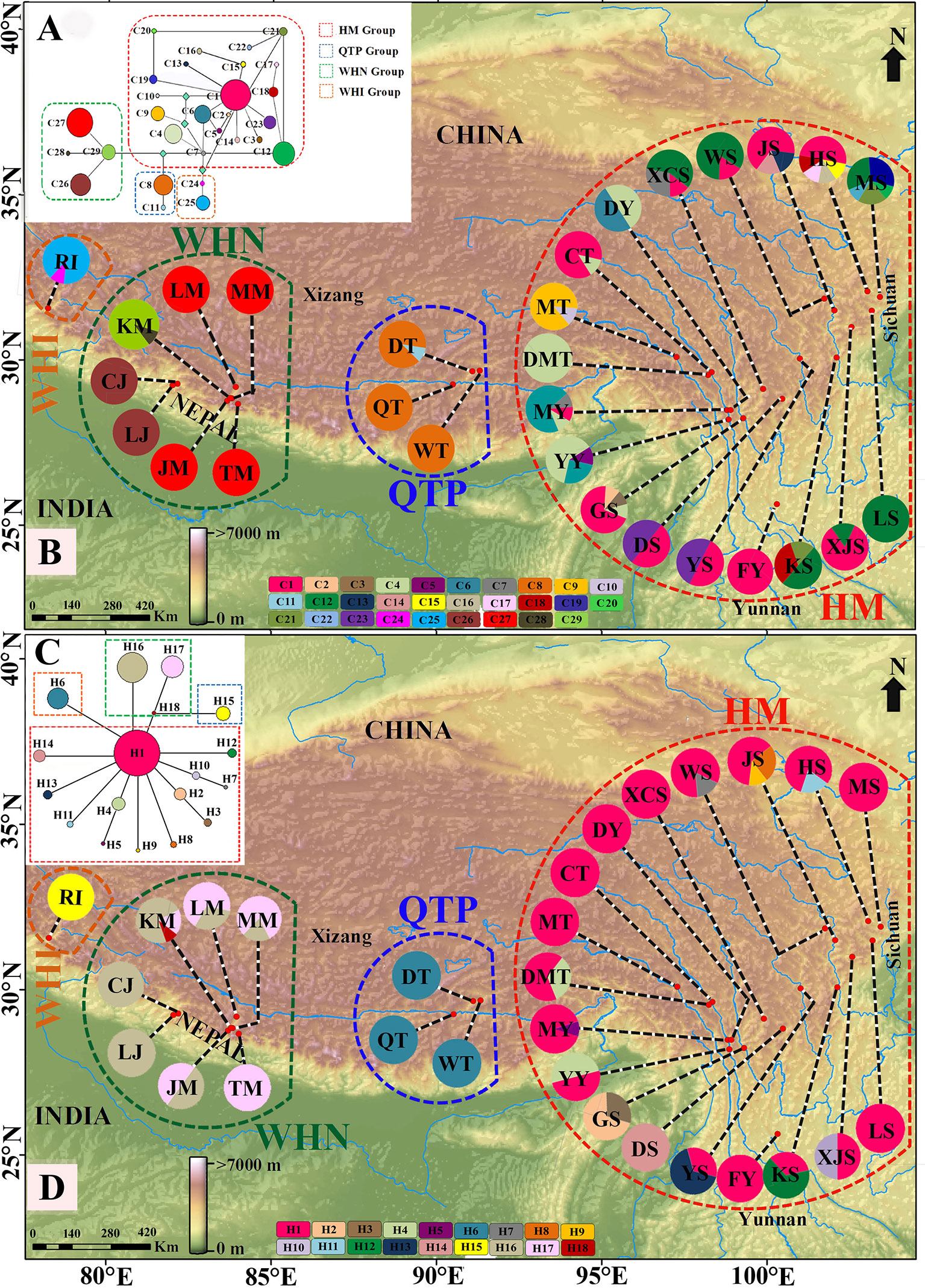
Figure 1 The MP median-joining networks of 29 chlorotypes (C1–C29) of cpDNA marker (A)/18 haplotypes (H1–H18) of G3pdh marker (C) and geographic locations of 29 populations of M. himalaica along with the distribution of the 29 chlorotypes of cpDNA (B)/18 haplotypes of G3pdh (D) detected among them (see Table 1 for population codes). In network figure, the size of circles corresponds to the frequency of each chloro/haplotypes and the light green squared box represents chloro/haplotypes missing from the dataset and each branch represents one mutation. Pie charts indicate the sample of each population and divisions within correspond to chloro/haplotypes with a number of individuals. The dotted line delineates the phylogroups (HM, Hengduan Mountains group; QTP, Qinghai-Tibetan Plateau group; WHN, Western Himalaya Nepal group; and WHI, Western Himalaya India group). Map source: http://www.diva‐gis.org/ and http://www.worldclim.org.
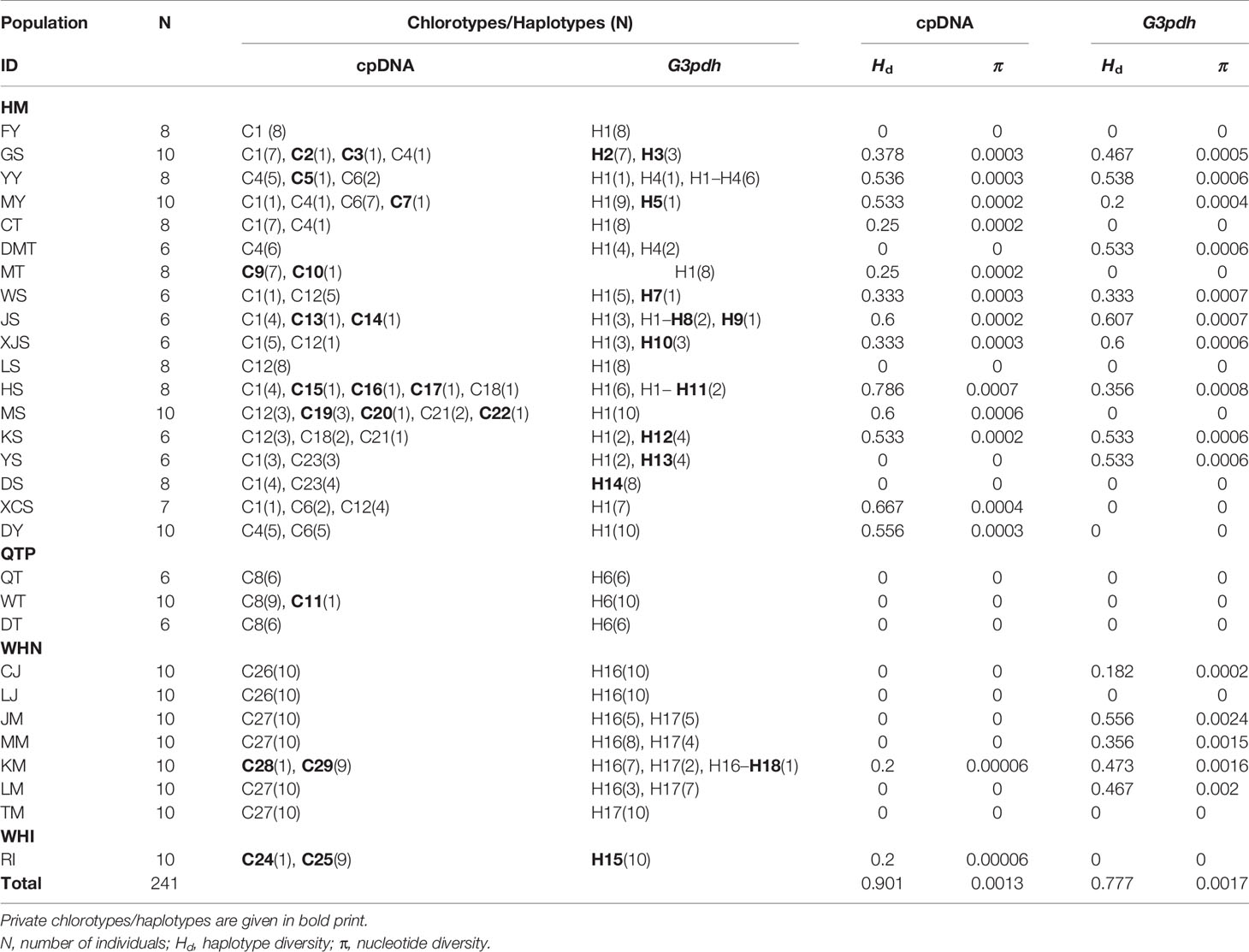
Table 1 Haplotype diversity and nucleotide diversity within populations of M. himalaica based on cpDNA and low copy nuclear gene (G3pdh) sequence.
The sequences of four cpDNA regions were concatenated, revised manually and aligned in GENEIOUS 7.0.2 (Kearse et al., 2012). Chromatograms of the G3pdh with “double peaks” at polymorphic sites were further analyzed by inferring the identity of haplotypes within a heterogeneous individual through haplotype subtraction (Clark, 1990; Christe et al., 2014) using PHASE algorithm in DNASP 5.0 (Librado and Rozas, 2009). The unique cpDNA/G3pdh haplotypes within populations were identified using DNASP 5.0 by considering the polymorphic sites only. Newly generated haplotype sequence data have been deposited in GenBank (petL-psbE: MK792906–MK792916; rps16-trnK: MK792917–MK792925; trnS-trnG: MK792926–MK792935; rps16 intron: MK803108–MK803115; G3pdh: MK803090–MK803107).
Genetic Polymorphism and Structure Analyses
Unique chlorotypes of cpDNA and haplotypes of G3pdh were determined in DNASP 5.0 (Librado and Rozas, 2009). Geographical distributions of chlorotypes/haplotypes were plotted using ArcGIS 10.2.1 (ESRI, Inc., Redlands, CA, USA). To quantify the level of genetic variation, total haplotype diversity (HT) and average within-population diversity (HS) (Nei, 1975) were calculated. GST and NST were used to estimate differentiation between populations (Nei, 1975). NST was compared to GST using U-statistics; an observed value of NST > GST generally indicates the presence of phylogeographical structure (Pons and Petit, 1996). We computed all these parameters employing HAPLONST (Pons and Petit, 1996). DNASP was used to analyze the genetic diversity parameters, including the haplotype diversity (Hd; Nei and Tajima, 1981) and nucleotide diversity (π; Nei and Li, 1979).
Spatial analysis of molecular variance (SAMOVA v2.0; Dupanloup et al., 2002), was implemented to define the number of groups of populations (K). We also performed an analysis of molecular variance (AMOVA) to examine the genetic variation using ARLEQUIN v3.5 (Excoffier and Lischer, 2010). The F-statistic (FST/FSC/FCT) was calculated, and significance was tested for overall as well as regional populations. “Isolation by distance” (IBD) was evaluated by regressing the net nucleotide divergence between populations (DA) in contrary to their geographical distance, applying Mantel's (1967) test with 999 permutations in GENALEX 6.5 (Peakall and Smouse, 2012). Network v5.0.0 (Bandelt et al., 1999; http://www.fluxus-engineering.com) was employed to construct median-joining networks of the cpDNA and G3pdh sequences to assess their genealogical relationships. All variable sites were included and weighted equally.
Phylogenetic Analyses and Divergence Dating
Phylogenetic relationships among chlorotypes of cpDNA along with sequences of three closest species from Mirabilis L. (M. jalapa, EF079612; M. multiflora, EF079603; M. albida, EF079602/KR014118) as outgroups were constructed by Bayesian inference (BI) in MRBAYES v3.2 (Ronquist et al., 2012) and BEAST v1.8.4 (Drummond and Rambaut, 2007; Drummond et al., 2012) under the GTR+I nucleotide substitution model selected by Akaike information criterion (AIC) in jModelTEST v2.1.6 (Guindon and Gascuel, 2003; Posada and Buckley, 2004) (see Supplementary Methods S1 for detail parameters). BEAST v1.8.4 was employed to estimate the temporal intraspecific divergence times (crown ages) of chlorotypes/haplotypes. We assumed a strict clock (P = 0.85; i.e. P > 0.05), based on a likelihood-ratio test (Felsenstein, 1988) in PAUP* 4.0b10 (Swofford, 2002) and constant population size for the coalescent tree prior to the distribution of divergence times. We used two secondary calibration points (Figure 2) to estimate the lineage divergence times based on Wang et al., 2019 (A: 13.13 ± 4.2 Ma; 95% highest posterior density [HPD] intervals: 6.91–20.62 Ma; i.e. a crown age for Mirabilis, and B: 5.22 ± 1.7 Ma; 95% 2.53–8.18 Ma i.e. divergence time of M. himalaica from its North American counterparts; Figure 2) (see Supplementary Methods S1 for detail parameters). Three independent runs of 20 million generations were carried out, with number of chains = 4, and sampling every 1,000 generations, where first 20% of the trees were discarded as burn-in. TREEANNOTATOR v1.8.4 (Drummond and Rambaut, 2007; Drummond et al., 2012) was used to obtain a maximum-credibility tree and FIGTREE v1.4.0 (Rambaut, 2012) was employed to view the resulting tree.
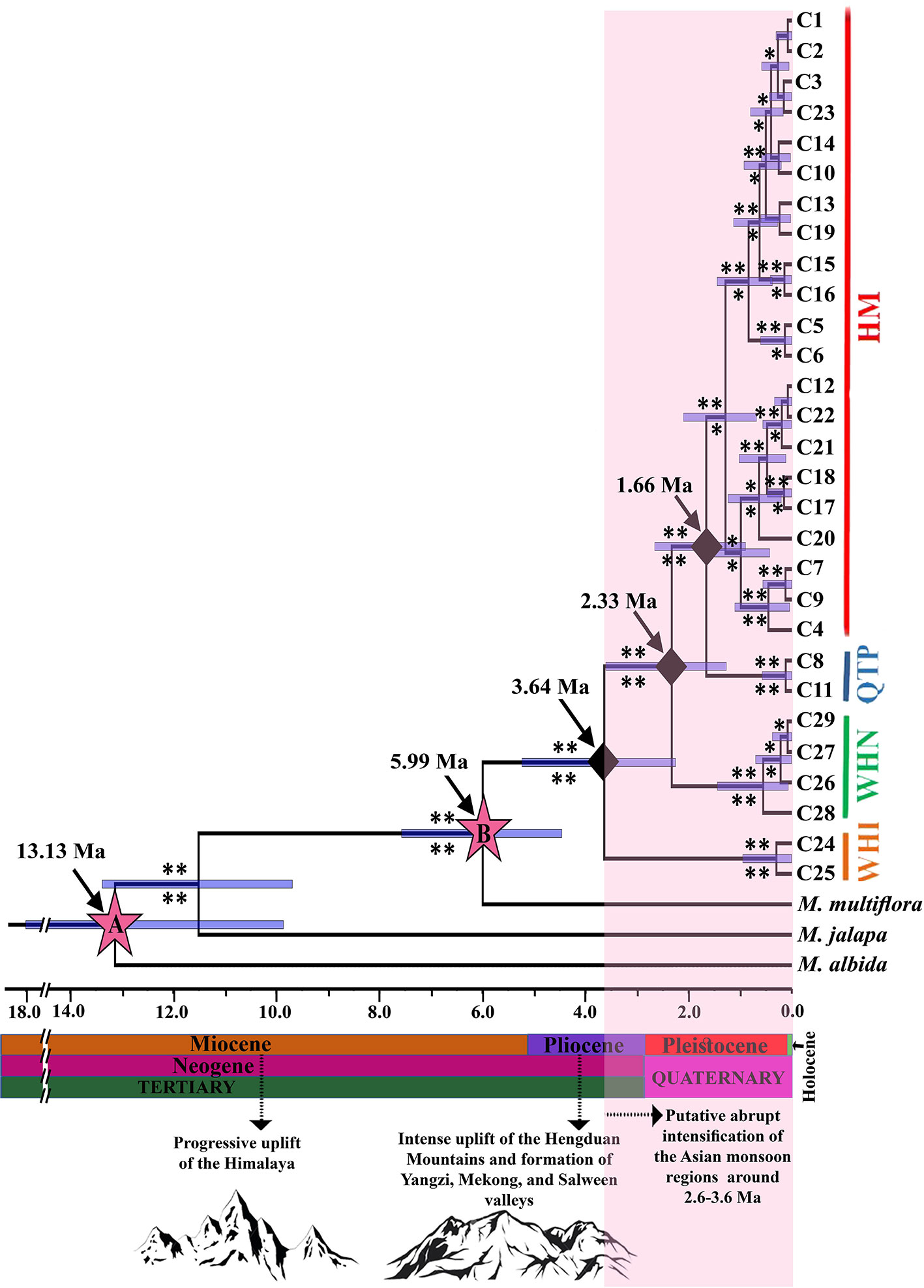
Figure 2 Maximum credibility clade (MCC) tree with estimated divergence times (Ma; Million Years ago) and ancestral area reconstruction of M. himalaica estimated using BEAST analysis. Asterisks indicate posterior probabilities based on BEAST (above the branches) and Bayesian analysis (below the branches). A single asterisk indicates weak or moderate support (0.5 < posterior probabilities < 0.95); two asterisks indicate strong support (posterior probabilities > 0.95). Node A and B represent the secondary calibration points based on Wang et al. (2019). The geological time scale with historical events is shown at the bottom of the phylogenetic tree.
Historical Demographic Analyses
Tajima's (1989) D and Fu's (1997) FS of neutrality tests were performed using ARLEQUIN v3.5 (Excoffier and Lischer, 2010), with 1,000 simulated samples. Pairwise mismatch distribution analysis (MDA; Rogers and Harpending, 1992) was performed in ARLEQUIN v3.5 to detect demographic expansion events of M. himalaica. The MDA compared the observed frequency distribution of pairwise differences among haplotypes with their theoretical distribution expected under the ‘pure population growth’ and ‘spatial expansion’ models, respectively. With the sum of squared deviations (SSD) and Harpending's (1994) raggedness index (HRag) ‘goodness of fit’ was tested, using 1,000 parametric bootstrap replicates.
In addition, Bayesian skyline plot (BSP: Drummond et al., 2005) generated in BEAST v1.8.4 was used to reconstruct the effective population size fluctuations since the time of the most recent common ancestor for each marker and the combined markers (cpDNA and G3pdh). The MCMC chains (nchains = 4) were run for 20 million generations sampling every 100 generations, with effective sample size (ESS) greater than 200. We used the same settings for three independent runs to ensure the consistency of the results. The demographic history through time was reconstructed using TRACER v1.7.1 (Rambaut et al., 2018).
Ensemble Species Distribution Modelling and Visualizing Dispersal Corridors
An ensemble of 'species distribution modelling (SDM; Guisan and Zimmermann, 2000) was carried out using “Biomod 2” (Thuiller et al., 2014) package implemented in R-programming language (R v3.4.1; R Development Core Team, 2016) for past (Last Interglacial, LIG; 120–140 Ka and Last Glacial Maximum, LGM; c. 22 Ka) (Otto-Bliesner et al., 2006), current (1990–2000) and future (2070; RCP 4.5). The model used 72 spatially filtered occurrence points to prevent from spatial autocorrelation that were checked in the 4-min grid (Rana et al., 2019). The eight predictive bioclimatic variables were selected based on iterative calculations of Variance Inflation Factors (VIF < 10; Fox and Weisberg, 2011) (Table 2), Pearson correlation (r < 0.8; Supplementary Table S2), followed by confirmatory test Principal component analysis (PCA) analysis implemented in R-Programming (Supplementary Table S3). The predictive performances of the 10 simulated models were calibrated and evaluated using 25% of the data that uses AUC (Area under ROC curve) > 0.8, TSS (True Skill Statistics); Cohen's Kappa > 0.7 (Supplementary Table S4). These models were then projected onto plaeo and future (2070, RCP4.5) climatic scenarios based on different General Circulation Models (one LIG, two each for LGM and future) to determine the distribution range shifts and suitable habitats of M. himalaica. The ensemble consensus model was converted into binary presence (1)/absence (0) applying thresholds that allow a maximum of 50% probability of the suitable habitat (Forester et al., 2013). We also reclassified changes in LIG, LGM, and future conditions compared to current suitability into retracted, stable, and expanded areas. (see Supplementary Methods S1 for details).
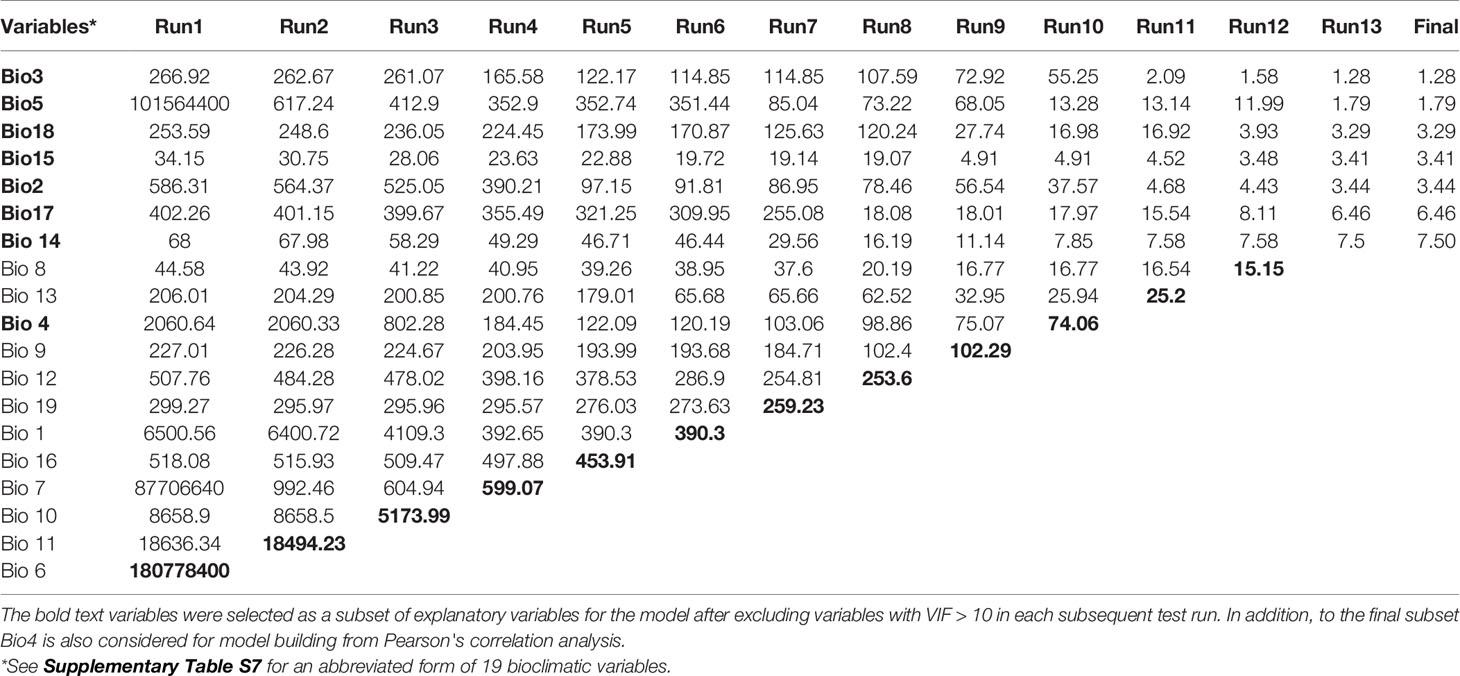
Table 2 Variance Inflation Factor (VIF) in different test runs for the selection of explanatory variables and the correlation between predictor variables.
The dispersal corridors were identified following the least cost path (LCP) methods using SDMtoolbox v2.0 (Brown et al., 2017) under the four climatic scenarios LIG, LGM, current, and future. For this analysis, we adopted the haplotype information of 29 localities, and populations that share haplotypes from two molecular markers (cpDNA and G3pdh) (Figures 1B, D). Firstly, we obtained a dispersal cost layer (resistance layer) by inverting the SDMs, and subsequently, we created a cost distance raster for each sampling locality using the resistance layer. Corridor layers were established based on the cost distance raster between two localities that shared haplotypes. To avoid oversimplifying landscape processes, we classified the value of each corridor layer into four intervals (three cutoff values: 1%, 2%, 5%) and reclassified as new values (5, 2, 1, 0, respectively). Finally, we summed up and standardized all of the pairwise reclassified corridor layers and identified dispersal maps of M. himalaica in an explicit landscape.
Results
Haplotype Diversity and Distributions
The total aligned sequences of the four combined cpDNA regions are 3290 bp (petL-psbE/838 bp, rps16-trnK/602 bp, rps16 intron/883 bp, trnS-trnG/967 bp) with 28 polymorphic sites and 71 indels (Supplementary Table S5). In total, 29 chlorotypes (C1–C29) were identified among 241 individuals (Table 1 and Figure 1B). The most common chlorotype was C1 (shared by 11 populations) followed by C12 (shared by six populations) (Table 1 and Figure 1B). Out of total chlorotypes, WHI and QTP groups (grouping by SAMOVA) harboured two chlorotypes each (6.9%), WHN group contained four chlorotypes (13.8%), and the remaining 21 were occupied by HM group (72.4%); indicating a very high level of molecular diversity in HM region. Nineteen chlorotypes (65.5% of the total) were relatively rare, each of them restricted to a single population, whereas 15 of them were distributed in the HM region.
Out of 241 individuals, only about 5% (11 individuals) are heterozygous for partial G3pdh sequence under haplotypes H1, H4, H8, H11, H16, and H18. Population YY is the most diverse with six heterozygous individuals. The length of the aligned sequence of G3pdh was 941 bp, containing 18 nuclear haplotypes with 20 polymorphic sites (Supplementary Table S6). Thirteen haplotypes (72.2%) were unique, each endemic to a single population; 11 of them (84.6%) were restricted to the HM region. H1 was the most common haplotype within 16 populations of HM regions (Figure 1C). The chlorotype/haplotype frequencies and distributions in populations are listed and shown in Table 1 and Figure 1.
Genetic Polymorphism and Population Structure
Total haplotype diversity (Hd)/nucleotide diversity (π) for the cpDNA and G3pdh sequences were 0.901/0.0013 and 0.777/0.00173, respectively. For cpDNA, the highest value of Hd and π falls in population HS (Hd = 0.786; π = 0.0006); whereas for G3pdh, population JS exhibited particularly a high value (Hd = 0.607; π = 0.0007) (Table 1). Total genetic diversity (HT) in the overall population of M. himalaica were 0.917/0.803 (cpDNA/G3pdh) (Table 3). The average within-population diversity for both cpDNA/G3pdh (HS = 0.303/0.340) was also relatively high. Both, HT and HS are highest in the HM group (for WHI group not calculated, because of only one population). The inter-population differentiation (GST) of the two datasets i.e. cpDNA and G3pdh was 0.669 and 0.577, respectively. For both datasets, NST was significantly greater than GST (U > 1.96, p < 0.05), indicating the existing phylogeographical structure in M. himalaica (Table 3).

Table 3 Estimates of Genetic diversity and genetic differentiation of M. himalaica using cpDNA and G3pdh sequences.
SAMOVA of cpDNA reached a platform when K = 5 (FCT = 0.872) and revealed a substantial spatial population genetic structure with five groups, i.e. HM (Hengduan Mountain) group with 18 populations, QTP (Qinghai-Tibetan Plateau) group with three populations, WHN (Western Himalaya Nepal: WC Nepal) I group with three populations (CJ, LJ, KM), WHN II group with four populations (MM, JM, LM, TM) and WHI (Western Himalaya India: Himachal Pradesh, India) group with one population (Supplementary Figure S1). SAMOVA of G3pdh data reached a platform when K = 6 (FCT = 0.6996, Supplementary Figure S1) and divided two same groups (QTP and WHI) like that of the cpDNA dataset. While the WHN was separated into two different subgroups (WHN1: CJ, LJ, MM, KM; WHN2: JM, LM, TM), and the HM was also divided into two subgroups (GS vs. other 17 populations). Considering the geographical unit of the HHM and the unstable population MM, we merged the divided subgroups and regarded four structured phylogroups (HM, QTP, WHN, and WHI; Figure 1) for M. himalaica. This grouping is also consistent with the SAMOVA grouping (K = 4) of cpDNA. Thus, based on four phylogroups, there is greater genetic partition among groups (78.58%/52.92%), than among populations within groups (17.30%/25.05%) or within populations (4.11%/22.03%) (Table 4). Examining the genealogical relationships/MJ network for chlorotypes and haplotypes, four clusters (HM, QTP, WHN, and WHI) were formed corresponding to the defined phylogroups (Figures 1A, C). The significant F-statistics from AMOVA suggested structured population within groups (Table 4). Additionally, the Mantel test revealed a statistically significant pattern of (IBD) for both cpDNA and G3pdh (rM = 0.62/0.43; P = 0.001) (Table 4).
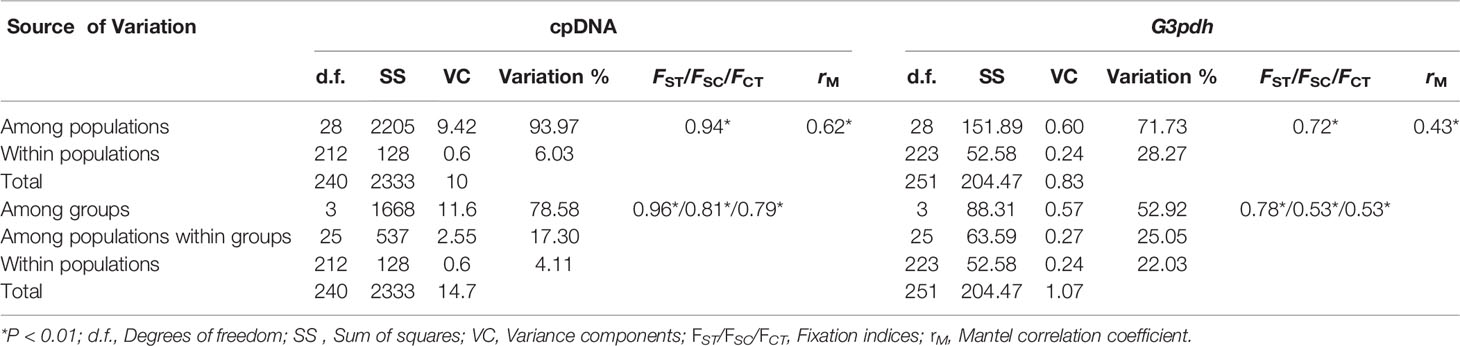
Table 4 Results of Analysis of molecular variance (AMOVA) of M. himalaica for cpDNA and low copy nuclear gene (G3pdh) sequence data, along with the results of the ‘isolation by distance’ analysis using Mantel tests in GenAlEx.
Phylogenetic Relationships and Divergence Time
The cpDNA topologies generated in MRBAYES and BEAST indicated M. himalaica was monophyletic with high posterior probabilities values which clustered the haplotypes into four lineages (Figure 2), corresponding to the four spatial population genetic groupings and genealogical relationships. Based on molecular dating, the onset of diversification for M. himalaica appeared in late Pliocene (crown age: 3.64 Ma; 95% HPD: 2.42–5.62 Ma; Figure 2) i.e. the first splitting event between the WHI lineage and the ancestor of the WHN, QTP, and HM lineages. Subsequently, the second splitting event between WHN lineage and the ancestor of QTP and HM lineage is 2.33 Ma (95% HPD: 1.37–3.87 Ma). Ultimately, the third splitting event between QTP and HM lineages occur during 1.66 Ma (95% HPD: 0.96–2.86 Ma).
Historical Demography
Tajima’s D and Fu’s Fs values were not significant, suggesting that M. himalaica conforms to the neutral hypothesis and these populations did not experience recent demographic expansion events (Table 5). The mismatch distribution for the overall chlorotypes/haplotypes were multimodal or bimodal (Supplementary Figure S2). While, the SSD and HRag statistics indicated a good statistical fit to the pure population growth and/or the spatial expansion model, excepting pure population growth in cpDNA data (p > 0.05; Table 5). This result suggested M. himalaica has experienced demographic events with structured populations that are shrinking in size or demographic equilibrium (Rogers and Harpending, 1992; Harpending, 1994).

Table 5 Results showing mismatch distribution analysis under models of (A) pure population growth and (B) spatial expansion, and neutrality test for the cpDNA and G3pdh sequence of M. himalaica; tests were conducted in ARLEQUIN with the sum of squared deviations (SSD) and Harpending's (1994) raggedness index (HRag).
BSP reconstructions based on combined markers (cpDNA and G3pdh) showed that the population of M. himalaica was quite stable after an ever-increasing phase during 0.6–0.075 Ma (Supplementary Figure S3), indicating no recent demographic expansion events. This case is similar for cpDNA marker when performed BSP reconstruction, while BSP of G3pdh showed a prolonged phase of demographic stability or no distinct population expansion (Supplementary Figure S3).
Ensemble Species Distribution Modelling and Visualization of Dispersal Corridors
The projected current distributions were generally the representations of the actual distributions and suitable habitat, which is consistent with present occurrence records (Figure 3A) except certain parts of the Eastern Himalaya. The paleodistribution reconstruction showed that during LIG, M. himalaica presented fragmented distribution patterns in the HM, and parts of the Western Himalaya (Figure 3B). Later during LGM, the species became more prominent towards the South of the HM (North Yunnan), WHI, and few in the southeastern QTP region (Figures 3C, D). The LGM distribution range of M. himalaica predicted using two models (CCSM4, MIROC-ESM) varies in some parts of the Western Himalaya and the HM (Figures 3C, D). In terms of the stability, we found that no matter which model was used, the distribution of M. himalaica during the LGM was farther south than the present one, suggesting northward expansion of populations after the LGM (Figures 4C, D). In addition, the predicted future distributions based on the MIROC-ESM model showed more migration to the north-west in the HM and west of the Himalaya compared to the present (Figures 3F and 4F). The result varies from the CCSM4 model, which was showing relatively more expansion towards the west in all regions (Figures 3E and 4E). It was predicted that species range expanded to southwards from LIG to LGM, but the range expansion is towards north-westwards after LGM up to current and the future (Figures 3 and 4).
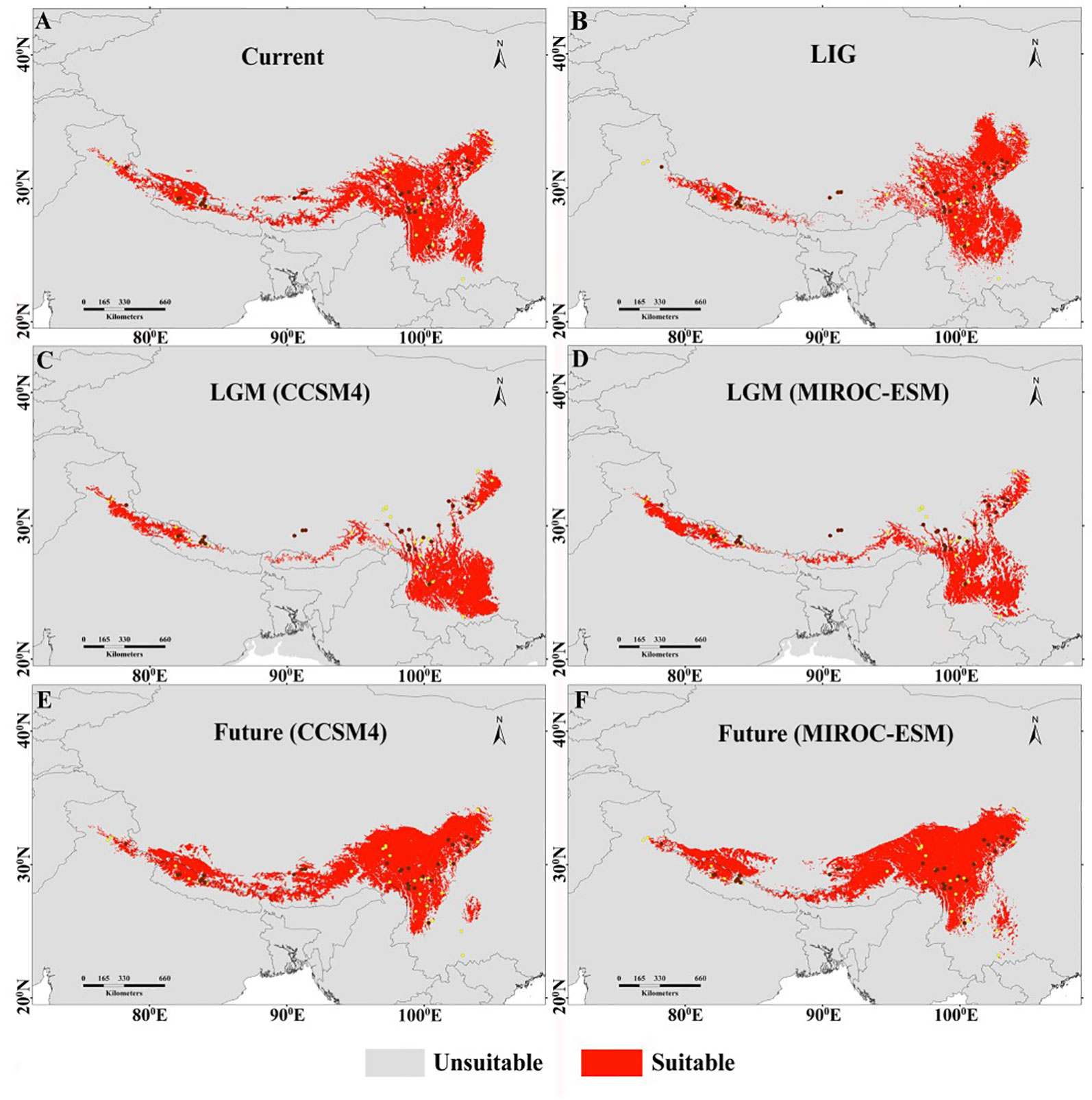
Figure 3 Habitat suitability models of M. himalaica predicted by ensemble Species Distribution Models under the assumption of the four climate scenarios: (A) Current (1990–2000); (B) LIG (c. 120–140 Ka); (C, D), LGM (c. 22Ka; based on the output of two GCMs; and (E, F) future (2070; based on the output of two GCMs under RCP4.5). Brown dots represent the location of populations in this study, and yellow dots correspond to all available occurrence locations used in species distribution modelling. The light grey regions in the map represent the area below the omission error regarded as not suitable, whereas the red regions indicate the area suitable for distribution of the species.
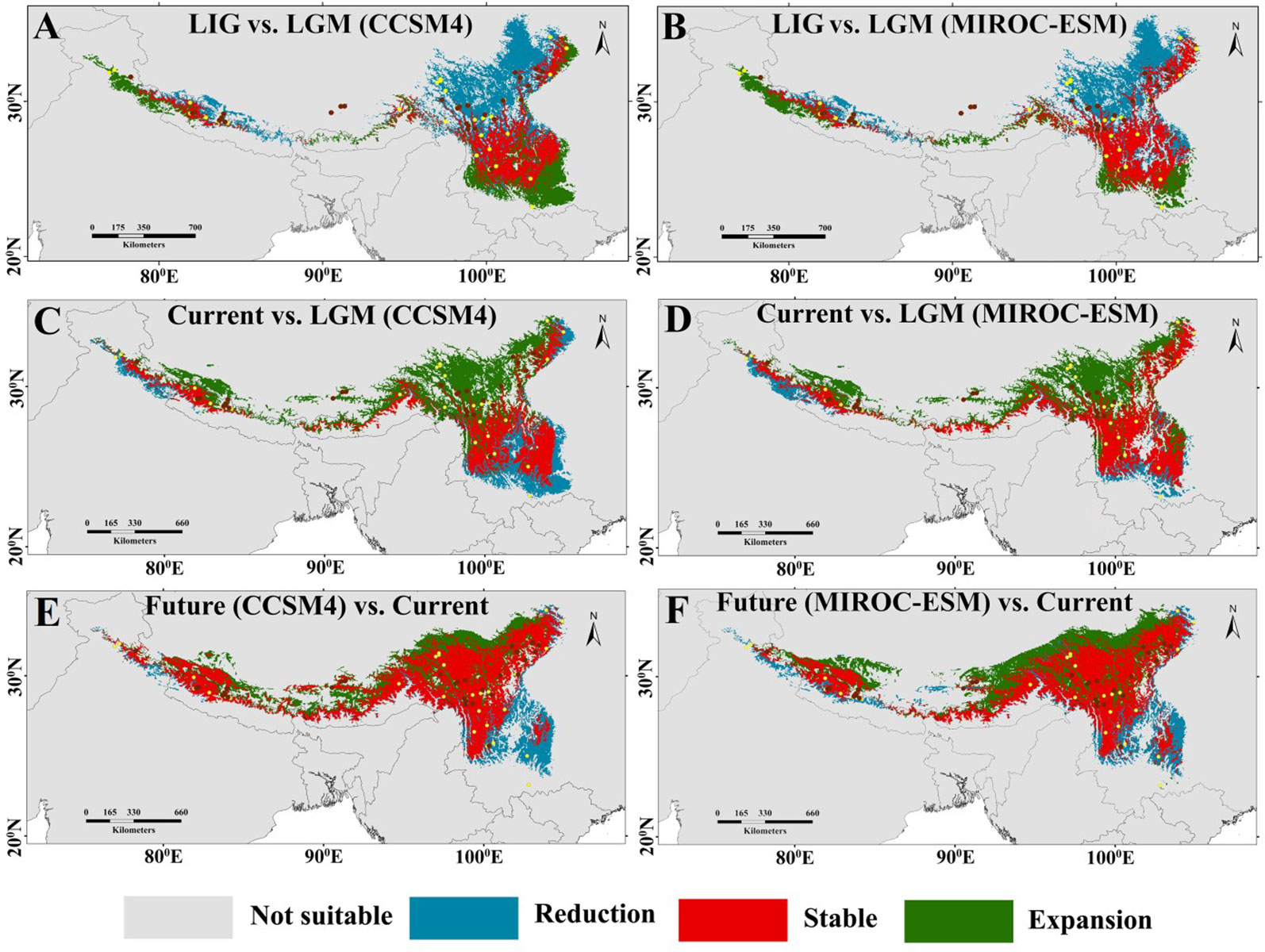
Figure 4 Maps of the potential Reduction, stable, and expansion areas compared between three climate scenarios: (A) LIG versus LGM (based on the output of CCSM4); (B) LIG versus LGM (based on the output of MIROC-ESM); (C) current versus LGM (based on the output of CCSM4); (D) current versus LGM (based on the output of MIROC-ESM); (E) future (based on the output of CCSM4, RCP4.5) versus current; versus current and (F) future (based on the output of MIROC-ESM, RCP4.5) versus current. See legend for representations of coloured regions.
Based on the least cost path analysis, putative dispersal corridors for the four periods (i.e. LIG, LGM, current, and future) were visualized using cpDNA and G3pdh markers (Figure 5). When comparing the dispersal routes across different time periods and genetic markers, dispersal generally followed isolated corridors between regional populations. There were no traces of connectivity between the populations of HM with the QTP and the Western Himalayas, and vice versa. This might be due to significant ridges and peaks of the local landscape in the Himalaya which formed a spatial barrier that intensified and fixed genetic isolation. The result exhibited continuous patterns of landscape connectivity among the populations present in the HM, indicating the existence of a dispersal corridor along mountains in the Hengduan regions. Besides the HM region, partial dispersal routes were also identified in the QTP and the WHN with average to low connectivity level (Figure 5). The cpDNA data did not show connectivity between two population groups from Jumla and Mustang–Manang of WHN; but G3pdh data revealed an additional area of dispersal in WHN beyond the dispersal corridor evidenced from the cpDNA data for the region (Figure 5). However, in this geologically dynamic region, the corridor shifts its route in different periods based on the distribution pattern, indicating that the dispersal corridor is not static for M. himalaica in the HHM. The distinct dispersal corridor in the middle of the HM during LIG through gorges of mountains to Yalongjiang and Daduhe rivers, shift to the south in the LGM showing strong connectivity through the middle Jinshajiang following Yalonjiang and paleo-route of Daduhe river. Similarly, in the current condition the high-value corridor passes through upper Jinshajiang, Yalongjiang, Daduhe rivers and rivulets or streams; whereas, in the future, the species is likely to lose its corridor in the south HM and shift to northwards following the spatial distribution range. In addition, a relatively larger area of dispersal was identified during the LGM period for both the makers (Figures 5B, C, H, I) compared to others. The strongest population genetic connectivity for M. himalaica occurred along with the river systems in the HM region.
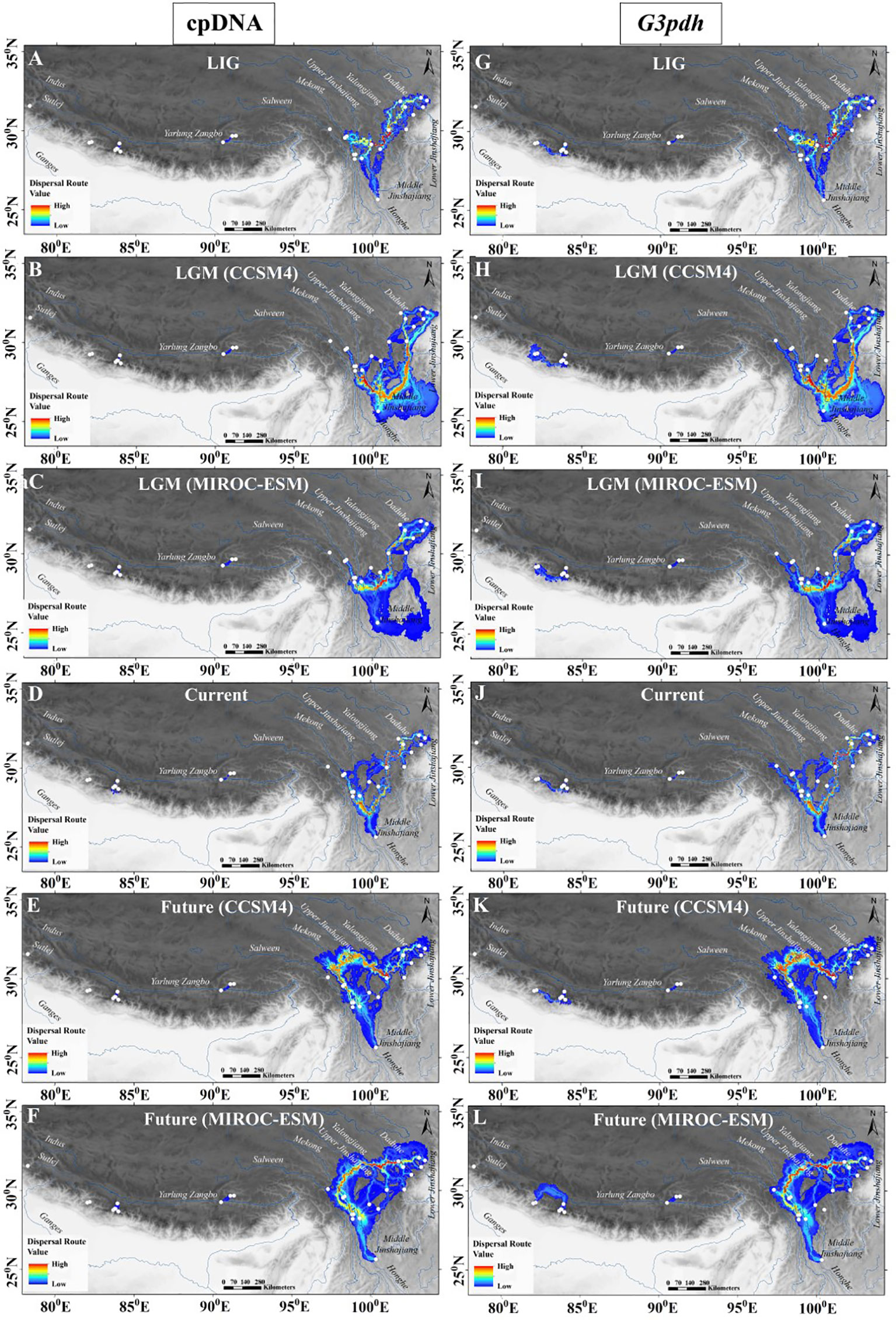
Figure 5 Potential dispersal corridors of M. himalaica in HHM based on cpDNA (A–F) and G3pdh (G–L) for the four periods (LIG, LGM, current, and future). Population genetic connectivity ranges from its highest values in red to its lowest values in blue.
Discussion
Evolutionary and Demographic History
It was speculated that the ancestor of Mirabilis himalaica might have migrated from North America to the Himalayas either by Beringia or through long-distance dispersal, evolving allopatrically into the extant species (Wang et al., 2019). Mirabilis himalaica diverged from the closest taxa during Early Pliocene c. 5.99 Ma (stem age; 95% HPD: 4.79–8.13 Ma), and the separation of the lineages spanning late Pliocene to early Pleistocene between 3.64–2.33 Ma (Figure 2). Late Miocene QTP uplift might have triggered speciation of M. himalaica in combination with the plentiful environmental alterations in the QTP and adjacent regions (Li et al., 1979). During the recent violent QTP uplifts (c. 3.6–1.5 Ma), intensification of the Asian monsoon (c. 3.6–2.6 Ma) (Li et al., 1979; An et al., 2001; Sun and Wang, 2005) were in progress, involving several mountain ranges in the HHM biodiversity hotspot (Harrison et al., 1992; Royden et al., 2008; Xie et al., 2019). Furthermore, tectonic events and climate change have set off the lineage divergence and rapidly occupying the newly available terrain during the Pleistocene. The uplift process might have coupled with the Pleistocene climatic oscillations leading to habitat diversification, restricted gene flow, and finally differentiation of the species (Xie et al., 2014; Luo et al., 2017; Shahzad et al., 2017; Ren et al., 2017a).
In addition, the glacial cycles of the Quaternary period in the HHM region have likely affected the demographic history of focal species. The results of the neutrality tests and MDA in conjunction with BSPs analysis suggested that M. himalaica did not experienced recent expansion events. Considering the poor ability to seed disperse, associated with the intense altitudinal gradient characterizing the Himalaya. We speculated that there is no large-scale distribution range expansion/contraction, while four lineages of M. himalaica, after diverging from each other survived in situ or experienced restricted regional migration (SDM and LCP) to shape the current phylogeographical pattern and the effective population size of all lineages remained relatively constant throughout the evolutionary period. From SDM analysis, during LGM the species was progressively confined to southwards in the HM, and traces of habitat expansion occurs in WHI, southeastern QTP region (Figures 4C, D) compared to predicted current and LIG distributions. The reason behind the southward movement of populations lies in the fact that the northernmost part of subtropical Central and Northern China was cooler at least 7–10°C and dryer by 200–300 mm/year (Sun and Chen, 1991; Zhou et al., 1991). At the time both steppe and desert vegetation expanded in a west to south directions. The localized cooling caused by glacial advance and later, subsequent warming due to glacial retreat might have exposed new habitats for colonization (Matthews, 1992) in the Northern and Western regions of the HHM. Therefore, our results suggested geological events along with climatic oscillations including monsoonal system and the Quaternary glaciation could have facilitated the formation and divergence of lineage and led to the accumulation of genetic diversity and differentiation of M. himalaica in the HHM region.
Pleistocene Unstable Habitat Connectivity
In this study, we identified the possible dispersal routes of M. himalaica since LIG to the future conditions in the HHM. Despite the adequacy of these mountains as a barrier to dispersal (Oheimb et al., 2013), there are several gorges and passes through the Main Divide, which might have permitted the dispersal of M. himalaica. Consequently, the major River system and the river valleys act as a dispersal corridor or recolonization route within the structured populations. Strikingly, the connectivity of dynamic habitats for M. himalaica was supported by the ensemble SDM prediction, which indicated that the population habitat connectivity also experienced potent change since LIG. Further, population genetic connectivity analysis based on the genetic data and SDM resistance layer similarly indicated that population genetic connectivity was prominent from LIG to current, and later in future conditions too (Figure 5). Population genetic connectivity and historical demographic changes of extant organisms are often associated with cyclical Pleistocene glaciations (Hewitt, 2000); and appears to be an important driver of inconsistency in the population genetic connectivity in M. himalaica. Besides, Pleistocene glaciations likely were restricted to high or middle elevations and did not affect deep slopes or valleys in the south-west mountainous region of China (Qu et al., 2014). As a result, the migration of populations to the deep slopes or valleys might have formulated strong dispersal corridors in the southern Hengduan Mountains during LGM compared to LIG. Our result showed that the corridors shift northward after LGM up to the future, indicating a purely consistent pattern with the spatial distribution. However, the rapid range change during the LIG to the future transition probably contributed to the patterns of genetic connectivity of local populations.
Response to Climate Change and Implications for Conservation
According to the forecasted future potential distribution, the focal species shift north-westward losing their potential suitability in hilly and lower mountainous regions by 2070 (Figures 4E, F). This extensive elevational shift might be due to global warming which combined with other biotic/abiotic factors fabricating unsuitable habitats at lower elevations. The annual mean warming rates during the period 1901–2020 was 0.19°C/decade (Ren et al., 2017b; Krishnan et al., 2019) over the Hindu Kush Himalaya, which is expected to increase further in future. In addition, the rate of warming for the Himalaya has been reported approximately three times (i.e. 1.5 °C/year) than the global average (i.e. 0.06 °C/year) (Shrestha et al., 2012). This increased warming rate at Himalaya region may prompt a significant issue for montane species. Likewise, the northward elevational shift of the species in the future does not ensure an increase in plant production itself. Eventually, high mountains serve as a geographical blockade and obstruct the species to relocate upwards due to the climatic condition atop the summit (Salick et al., 2009; Rana et al., 2017; Rana et al., 2019), because of summit trap phenomena (Pertoldi and Bach, 2007). This finally leads to the extinction of species due to no habitat for survival atop the mountain. Future distributions show the north-westward elevational shift of the species habitat and the dispersal corridor as well, which represents more credible conservation priority areas in the north-west higher elevational region of the HHM for M. himalaica. Geo-climatic variables are by all account not the only component to cause species habitat or population destruction because in present world anthropogenic factors are equally contributing to the cause. In this way, predicted expansion regions could be prioritized to protect the species from serious destruction of genetic diversity.
Data Availability Statement
The haplotype sequence data have been deposited in GenBank with accessions: petL-psbE: MK792906–MK792916; rps16-trnK: MK792917–MK792925; trnS-trnG: MK792926–MK792935; rps16 intron: MK803108–MK803115; G3pdh: MK803090–MK803107. The matrix containing the geographic and climatic information used for species distribution modelling and the aligned sequence data matrix for both the markers were deposited in Dryad dataset (https://doi.org/10.5061/dryad.0zpc866t8).
Author Contributions
HR and HS planned and designed the research. HR and SR carried out sampling and performed experiments. HR and DL analyzed data. HR and DL conceived the manuscript with the support of SR and HS.
Conflict of Interest
The authors declare that the research was conducted in the absence of any commercial or financial relationships that could be construed as a potential conflict of interest.
Acknowledgments
The present work is financially supported by the Second Tibetan Plateau Scientific Expedition and Research (STEP) program (2019QZKK0502), the Key Projects of the Joint Fund of the National Natural Science Foundation of China (U1802232), the Major Program of the National Natural Science Foundation of China (31590823), and the National Natural Science Foundation of China (31600170). Authors acknowledge Mr. Alexander Robert O'Neill (Native English speaker, USA) for grammar and language correction. Authors further thank Ms. Song Minshu for her help with the experiments, as well as Dr. Tao Deng and Mr. Ek Bahadur Rana for providing the samples.
Supplementary Material
The Supplementary Material for this article can be found online at: https://www.frontiersin.org/articles/10.3389/fpls.2019.01721/full#supplementary-material
Supplementary Figure S1 | Distributions of the FCT values for the indicated number of groups (K) of M. himalaica populations based on cpDNA (solid line) and G3pdh (dotted line) sequences.
Supplementary Figure S2 | Mismatch distribution of chloro/haplotypes of cpDNA/G3pdh in M. himalaica. The continuous lines with box represent observed distributions, whereas dotted lines with box represent simulated distributions under models of Demographic expansion (A and C) and spatial expansion (B and D) for cpDNA and G3pdh sequences, respectively (Rogers and Harpending, 1992).
Supplementary Figure S3 | Historical demographic trends of the whole population sample of M. himalaica by Bayesian skyline plot (BSP) based on each marker and the combined (cpDNA and G3pdh) data. The x-axis in the plot represents time-scale before present, and the y-axis represents the estimated effective population size. Estimates of means are joined by a solid line, and the shaded range delineates the 95% HPD limits.
Supplementary Table S1 | Details of population localities including population codes, voucher, geographic origins, coordinates (latitude and longitude), and altitudes of M. himalaica.
Supplementary Table S2 | Pearson’s correlation (r) matrix performed among the 19 bioclimatic variables.
Supplementary Table S3 | Result generated by Principal Components (PC) for the subset of explanatory variables, representing Eigenvalue and its percent.
Supplementary Table S4 | Result of Model accuracy assessment for different General Circulation models (GCMs) under an ensemble distribution modelling using Biomod2 in R-programming language.
Supplementary Table S5 | cpDNA sequence polymorphisms detected in the combined region of M. himalaica individuals from 29 populations, identifying 29 chlorotypes (C1–C29).
Supplementary Table S6 | Polymorphisms detected in the low-copy nuclear gene (G3pdh) region of M. himalaica individuals from 29 populations, identifying 18 haplotypes (H1–H18).
Supplementary Table S7 | List of variables used as inputs to generate ensemble distribution models for Ecological Niche Modelling of M. himalaica.
Supplementary Method S1 | Details on methods.
References
An, Z. S., Kutzbach, J. E., Prell, W. L., Porter, S. C. (2001). Evolution of Asian monsoons and phased uplift of the Himalaya-Tibetan Plateau since Late Miocene times. Nature 411, 62–66. doi: 10.1038/35075035
Avise, J. C. (2000). Phylogeography: the history and formation of species (Cambridge, MA: Harvard University Press).
Avise, J. C. (2009). Phylogeography: retrospect and prospect. J. Biogeogr. 36, 3–15. doi: 10.1111/j.1365-2699.2008.02032.x
Bandelt, H. J., Forster, P., Rohl, A. (1999). Median-joining networks for inferring intraspecific phylogenies. Mol. Biol. Evol. 16, 37–48. doi: 10.1093/oxfordjournals.molbev.a026036
Brown, J. L., Yoder, A. D. (2015). Shifting ranges and conservation challenges for lemurs in the face of climate change. Ecol. Evol. 5, 1131–1142. doi: 10.1002/ece3.1418
Brown, J. L., Bennett, J. R., French, C. M. (2017). SDMtoolbox 2.0: the next generation Python-based GIS toolkit for landscape genetic, biogeographic and species distribution model analyses. PeerJ 5, e4095. doi: 10.7717/peerj.4095
Chan, L. M., Brown, J. L., Yoder, A. D. (2011). Integrating statistical genetic and geospatial methods brings new power to phylogeography. Mol. Phylogenet. Evol. 59, 523–537. doi: 10.1016/j.ympev.2011.01.020
Christe, C., Caetano, S., Aeschimann, D., Kropf, M., Diadema, K., Naciri, Y. (2014). The intraspecific genetic variability of silicious and calcareous Gentiana species is shaped by contrasting demographic and re-colonization process. Mol. Phylogenet. Evol. 70, 323–336. doi: 10.1016/j.ympev.2013.09.022
Clark, A. G. (1990). Inference of haplotypes from PCR-amplified samples of diploid populations. Mol. Biol. Evol. 7, 111–122. doi: 10.1093/oxfordjournals.molbev.a040591
Drummond, A. J., Rambaut, A. (2007). BEAST: Bayesian evolutionary analysis by sampling trees. BMC Evol. Biol. 7, 214. doi: 10.1186/1471-2148-7-214
Drummond, A. J., Rambaut, A., Shapiro, B., Pybus, O. G. (2005). Bayesian coalescent inference of past population dynamics from molecular sequences. Mol. Biol. Evol. 22 (5), 1185–1192. doi: 10.1093/molbev/msi103
Drummond, A. J., Suchard, M. A., Xie, D., Rambaut, A. (2012). Bayesian phylogenetics with BEAUti and the BEAST 1.7. Mol. Biol. Evol. 29 (8), 1969–1973. doi: 10.1093/molbev/mss075
Dupanloup, I., Schneider, S., Excoffier, L. (2002). A simulated annealing approach to define the genetic structure of populations. Mol. Ecol. 11, 2571– 2581. doi: 10.1046/j.1365-294X.2002.01650.x
Dupont-Nivet, G., Lippert, P. C., Van Hinsbergen, D. J., Meijers, M. J., Kapp, P. (2010). Palaeolatitude and age of the Indo–Asia collision: palaeomagnetic constraints. Geophys. J. Int. 182, 1189–1198. doi: 10.1111/j.1365-246X.2010.04697.x
Excoffier, L., Lischer, H. E. (2010). Arlequin suite ver 3.5: a new series of programs to perform population genetics analyses under Linux and Windows. Mol. Ecol. Resour. 10, 564–567. doi: 10.1111/j.1755-0998.2010.02847.x
Favre, A., Packert, M., Pauls, S. U., Jahnig, S. C., Uhl, D., Michalak, I., et al. (2015). The role of the uplift of the Qinghai-Tibetan Plateau for the evolution of Tibetan biotas. Biol. Rev. Camb. Philos. Soc. 90, 236–253. doi: 10.1111/brv.12107
Felsenstein, J. (1988). Phylogenies from molecular sequences: inference and reliability. Annu. Rev. Genet. 22, 521–565. doi: 10.1146/annurev.ge.22.120188.002513
Forester, B. R., DeChaine, E. G., Bunn, A. G. (2013). Integrating ensemble species distribution modelling and statistical phylogeography to inform projections of climate change impacts on species distributions. Divers. Distrib. 19, 1480–1495. doi: 10.1111/ddi.12098
Fox, J., Weisberg, S. (2011). An R Companion to Applied Regression, second ed (Thousand Oaks, CA: Sage).
Fu, Y. X. (1997). Statistical tests of neutrality of mutations against population growth, hitchhiking and background selection. Genetics 147 (2), 915–925.
Guindon, S., Gascuel, O. (2003). A simple, fast and accurate algorithm to estimate large phylogenies by maximum likelihood. Syst. Biol. 52 (5), 696–704. doi: 10.1080/10635150390235520
Guisan, A., Zimmermann, N. E. (2000). Predictive habitat distribution models in ecology. Ecol. Model. 135, 147–186. doi: 10.1016/s0304-3800(00)00354-9
Harpending, H. (1994). Signature of ancient population growth in a low-resolution mitochondrial DNA mismatch distribution. Hum. Biol. 66, 591–600.
Harrison, T. M., Copeland, P., Kidd, W., Yin, A. (1992). Raising Tibet. Science 255, 1663–1670. doi: 10.1126/science.255.5052.1663
Hewitt, G. (2000). The genetic legacy of the Quaternary ice ages. Nature 405, 907–913. doi: 10.1038/35016000
Jian, H. Y., Tang, K. X., Sun, H. (2015). Phylogeography of Rosa soulieana (Rosaceae) in the Hengduan Mountains: refugia and ‘melting' pots in the Quaternary climate oscillations. Plant Syst. Evol. 301 (7), 1819–1830. doi: 10.1007/s00606-015-1195-0
Kearse, M., Moir, R., Wilson, A., Stones-Havas, S., Cheung, M., Sturrock, S., et al. (2012). Geneious basic: an integrated and extendable desktop software platform for the organization and analysis of sequence data. Bioinformatics 28 (12), 1647–1649. doi: 10.1093/bioinformatics/bts199
Krishnan, R., Shrestha, A. B., Ren, G., Rajbhandari, R., Saeed, S., Sanjay, J., et al. (2019). “Unravelling climate change in the Hindu kush himalaya: rapid warming in the mountains and increasing extremes,” in The Hindu Kush Himalaya Assessment. Eds. Wester, P., Mishra, A., Mukherji, A., Shrestha, A. (Cham: Springer). doi: 10.1007/978-3-319-92288-1_3
Larkin, J. L., Maehr, D. S., Hoctor, T. S., Orlando, M. A., Whitney, K. (2004). Landscape linkages and conservation planning for the black bear in west-central Florida. Anim. Conserv. 7, 23–34. doi: 10.1017/S1367943003001100
Li, J. J., Fang, X. M. (1999). Uplift of the Tibetan Plateau and environmental changes. Sci. Bull. 44 (23), 2117–2124. doi: 10.1007/BF03182692
Li, J. J., Wen, S. X., Zhang, Q. S., Wang, F. B., Zheng, B. X., Li, B. Y. (1979). A discussion on the period, amplitude and type of the uplift of the Qinghai‐Xizang (Tibetan) Plateau. Sci. Sin. 22, 1314–1328.
Librado, P., Rozas, J. (2009). DnaSP v5: a software for comprehensive analysis of DNA polymorphism data. Bioinformatics 25, 1451–1452. doi: 10.1093/bioinformatics/btp187
Liu, J. Q., Wang, Y. J., Wang, A. L., Hideaki, O., Abbott, R. J. (2006). Radiation and diversification within the Ligularia-Cremanthodium-Parasenecio complex (Asteraceae) triggered by uplift of the Qinghai-Tibetan Plateau. Mol. Phylogenet. Evol. 38, 31–49. doi: 10.1016/j.ympev.2005.09.010
Lu, D. Q., Gilbert, M. G., Wu, Z. Y., Raven, P. H. (2003). “Nyctaginaceae,” in Flora of China, vol. 5. (Beijing & St. Louis: Science Press & Missouri Botanical Garden Press), 432–433.
Luo, D., Yue, J. P., Sun, W. G., Xu, B., Li, Z. M., Comes, H. P., et al. (2016). Evolutionary history of the subnival flora of the Himalaya-Hengduan Mountains: first insights from comparative phylogeography of four perennial herbs. J. Biogeogr. 43, 31–43. doi: 10.1111/jbi.12610
Luo, D., Xu, B., Li, Z. M., Sun, H. (2017). The ‘Ward Line-Mekong-Salween Divide' is an important floristic boundary between the eastern Himalaya and Hengduan Mountains: evidence from the phylogeographical structure of subnival herbs Marmoritis complanatum (Lamiaceae). Bot. J. Linn. Soc 185, 482–496. doi: 10.1093/botlinnean/box067
Mantel, N. (1967). The detection of disease clustering and a generalized regression approach. Cancer Res. 27, 209–220.
Matthews, J. A. (1992). The Ecology of Recently-Deglaciated Terrain (Cambridge University Press, Cambridge). doi: 10.1002/jqs.3390080213
Meng, H. H., Su, T., Gao, X. Y., Li, J. I., Jiang, X. L., Sun, H., et al. (2017). Warm-cold colonization: response of oaks to uplift of the Himalaya-Hengduan Mountains. Mol. Ecol. 26, 3276–3294. doi: 10.1111/mec.14092
Mulch, A., Chamberlain, C. P. (2006). Earth science-The rise and growth of Tibet. Nature 439, 670–671. doi: 10.1038/439670a
Nei, M., Li, W. H. (1979). Mathematical model for studying genetic variation in terms of restriction endonucleases. Proc. Natl. Acad. Sci. U.S.A. 76, 5269–5273. doi: 10.1073/pnas.76.10.5269
Nei, M., Tajima, F. (1981). DNA polymorphism detectable by Restriction Endonucleases. Genetics 97 (1), 145–163.
Nei, M. (1975). Molecular population genetics and evolution (North-Holland Pub. Co., Amsterdam, Netherlands).
Oheimb, P. V., Albrecht, C., Riedel, F., Bössneck, U., Zhang, H., Wilke, T. (2013). Testing the role of the Himalayan Mountains as a dispersal barrier in freshwater gastropods (Gyraulus spp.). Biol. J. Linn. Soc. 109, 526–534. doi: 10.1111/bij.12068
Otto-Bliesner, B. L., Marshall, S. J., Overpeck, J. T., Miller, G. H., Hu, A., CAPE Last Interglacial Project Members (2006). Simulating Arctic climate warmth and icefield retreat in the Last Interglaciation. Science 311 (5768), 1751–1753. doi: 10.1126/science.1120808
Peakall, R., Smouse, P. E. (2012). GenAlEx 6.5: Genetic analysis in Excel. Population genetic software for teaching and research-an update. Bioinformatics 28, 2537–2539. doi: 10.1093/bioinformatics/bts460
Pertoldi, C., Bach, L. A. (2007). Evolutionary aspects of climate-induced changes and the need for multidisciplinarity. J. Therm. Biol. 32, 118–124. doi: 10.1016/j.jtherbio.2007.01.011
Pons, O., Petit, R. J. (1996). Measuring and testing genetic differentiation with ordered versus unordered alleles. Genetics 144, 1237–1245.
Posada, D., Buckley, T. (2004). Model selection and model averaging in phylogenetics: advantages of Akaike information criterion and Bayesian approaches over likelihood ratio tests. Syst. Biol. 53, 793–808. doi: 10.1093/bioinformatics/14.9.817
Qiu, Y. X., Fu, C. X., Comes, H. P. (2011). Plant molecular phylogeography in China and adjacent regions: tracing the genetic imprints of Quaternary climate and environmental change in the world's most diverse temperate flora. Mol. Phylogenet. Evol. 59, 225–244. doi: 10.1016/j.ympev.2011.01.012
Qu, Y. H., Ericson, P. G. P., Quan, Q., Song, G., Zhang, R. Y., Gao, B., et al. (2014). Long-term isolation and stability explain high genetic diversity in the Eastern Himalaya. Mol. Ecol. 23, 705–720. doi: 10.1111/mec.12619
R Development Core Team. (2016). R v3.4.1: A language and environment for statistical computing (Vienna, Austria: R Foundation for Statistical Computing).
Rambaut, A., Drummond, A. J., Xie, D., Baele, G., Suchard, M. A. (2018). Posterior summarisation in Bayesian phylogenetics using Tracer 1.7. Syst. Biol. 67 (5), 901–904. doi: 10.1093/sysbio/syy032
Rambaut, A. (2012). FigTree, tree figure drawing tool v.1.4.0 (University of Edinburgh: Institute of Evolutionary Biology). [WWW document] URL http://tree.bio.ed.ac.uk/software/figtree/[accessed 20 June 2018].
Rana, S. K., Rana, H. K., Ghimire, S. K., Shrestha, K. K., Ranjitkar, S. (2017). Predicting the impact of climate change on the distribution of two threatened Himalayan medicinal plants of Liliaceae in Nepal. J. Mt. Sci. 14, 558–570. doi: 10.1007/s11629-015-3822-1
Rana, S. K., Luo, D., Rana, H. K., O'Neill, A. R., Sun, H. (2019). Geoclimatic factors influence the population genetic connectivity of Incarvillea arguta (Bignoniaceae) in the Himalaya–Hengduan Mountains biodiversity hotspot. J. Syst. Evol. (Published online) doi: 10.1111/jse.12521
Ren, G. P., Mateo, R. G., Liu, J. Q., Suchan, T., Alvarez, N., Guisan, A., et al. (2017a). Genetic consequences of Quaternary climatic oscillations in the Himalayas: Primula tibetica as a case study based on restriction site-associated DNA sequencing. New Phytol. 213, 1500–1512. doi: 10.1111/nph.14221
Ren, Y. Y., Ren, G. Y., Sun, X. B., Shrestha, A. B., You, Q. L., Zhan, Y. J., et al. (2017b). Observed changes in surface air temperature and precipitation in the Hindu Kush Himalayan region over the last 100-plus years. Adv. Clim. Change. Res. 8 (3), 148–156. doi: 10.1016/j.accre.2017.08.001
Rogers, A. R., Harpending, H. (1992). Population-growth makes waves in the distribution of pairwise genetic-differences. Mol. Biol. Evol. 9, 552–569. doi: 10.1093/oxfordjournals.molbev.a040727
Ronquist, F., Teslenko, M., Mark, P. V. D., Ayres, D., Darling, A., Höhna, S., et al. (2012). MrBayes 3.2: efficient Bayesian phylogenetic inference and model choice across a large model space. Syst. Biol. 61 (3), 539–542. doi: 10.1093/sysbio/sys029
Royden, L. H., Burchfiel, B. C., van der Hils, T. R. D. (2008). The geological evolution of the Tibetan plateau. Science 321, 1054–1058. doi: 10.1126/science.1155371
Salick, J., Zhendong, F., Byg, A. (2009). Eastern Himalayan alpine plant ecology, Tibetan ethnobotany, and climate change. Global Environ. Change 19, 147–155. doi: 10.1016/j.gloenvcha.2009.01.008
Shahzad, K., Jia, Y., Chen, F. L., Zeb, U., Li, Z. H. (2017). Effects of mountain uplift and climatic oscillations on phylogeography and species divergence in four endangered Notopterygium Herbs. Front. Plant Sci. 8, 19–29. doi: 10.3389/fpls.2017.01929
Shrestha, U. B., Gautam, S., Bawa, K. S. (2012). Widespread climate change in the Himalayas and associated changes in local ecosystems. PloS One 7, e36741. doi: 10.1371/journal.pone.0036741
Spellenberg, R., Flora of North America Editorial Committee (2003). “Nyctaginaceae,” in Flora of North America north of Mexico (New York: Oxford University Press), 14–74.
Sun, X. J., Wang, P. X. (2005). How old is the Asian monsoon system? Palaeobotanical records from China. Palaeogeogr. Palaeoclimatol. Palaeoecol. 222, 181–222. doi: 10.1016/j.palaeo.2005.03.005
Sun, X. J., Chen, Y. S. (1991). Palynological records of the last 11,000 years in China. Quat. Sci. Rev. 10, 537–544. doi: 10.1016/0277-3791(91)90047-X
Swofford, D. L. (2002). PAUP*: Phylogenetic analysis using parsimony (and other methods) v. 4.0b10 (Sunderland, Massachusetts, USA: Sinauer Associates).
Tajima, F. (1989). Statistical method for testing the neutral mutation hypothesis by DNA polymorphism. Genetics 123, 585–595.
Thuiller, W., Georges, D., Engler, R. (2014). Biomod2: ensemble platform for species distribution modelling. R package version 3. 1–64, [WWW document] URL http://CRAN.R-project.org/package = biomod2. [accessed 20 August 2018].
Wang, Y. L., Yan, G. Q. (2014). Molecular Phylogeography and Population Genetic Structure of O. longilobus and O. taihangensis (Opisthopappus) on the Taihang Mountains. PloS One 9 (8), e104773. doi: 10.1371/journal.pone.0104773
Wang, I. J., Savage, W. K., Bradley, H. S. (2009). Landscape genetics and least-cost path analysis reveal unexpected dispersal routes in the California tiger salamander (Ambystoma californiense). Mol. Ecol. 18, 1365–1374. doi: 10.1111/j.1365-294X.2009.04122.x
Wang, S. L., Li, L., Ci, X. Q., Conran, J. G., Li, J. (2019). Taxonomic status and distribution of Mirabilis himalaica (Nyctaginaceae). J. Syst. Evol. 57 (5), 431–439. doi: 10.1111/jse.12466
Xie, H., Ash, J. E., Linde, C. C., Cunningham, S., Nicotra, A. (2014). Himalayan-Tibetan plateau uplift drives divergence of polyploid poppies: Meconopsis Viguier (Papaveraceae). PloS One 9 (6), e99177. doi: 10.1371/journal.pone.0099177
Xie, C., Xie, D. F., Zhong, Y., Guo, X. L., Liu, Q., Zhou, S. D., et al. (2019). The effect of Hengduan Mountains Region (HMR) uplift to environmental changes in the HMR and its eastern adjacent area: tracing the evolutionary history of Allium section Sikkimensia (Amaryllidaceae). Mol. Phylogenet. Evol. 130, 380–396. doi: 10.1016/j.ympev.2018.09.011
Ye, Z., Yuan, J. J., Li, M., Damgaard, J., Chen, P. P., Zheng, C. G., et al. (2018). Geological effects influence population genetic connectivity more than Pleistocene glaciations in the water strider Metrocoris sichuanensis (Insecta: Hemiptera: Gerridae). J. Biogeogr. 45, 690–701. doi: 10.1111/jbi.13148
Yin, A., Harrison, T. M. (2000). Geologic evolution of the Himalayan-Tibetan orogen. Annu. Rev. Earth Planet. Sci. 28, 211–280. doi: 10.1146/annurev.earth.28.1.211
Yu, H. B., Zhang, Y. L., Liu, L. S., Qi, W., Li, S. C., Hu, Z. G. (2015). Combining the least cost path method with population genetic data and species distribution models to identify landscape connectivity during the late Quaternary in Himalayan hemlock. Ecol. Evol. 5, 5781–5791. doi: 10.1002/ece3.1840
Yu, H. B., Zhang, Y. L., Wang, Z. F., Liu, L. S., Chen, Z., Qi, W. (2017). Diverse range dynamics and dispersal routes of plants on the Tibetan Plateau during the late Quaternary. PloS One 12 (5), e0177101. doi: 10.1371/journal.pone.0177101
Keywords: climate change, dispersal corridors, ensemble species distribution modelling, genetic connectivity, Mirabilis himalaica, phylogeography
Citation: Rana HK, Luo D, Rana SK and Sun H (2020) Geological and Climatic Factors Affect the Population Genetic Connectivity in Mirabilis himalaica (Nyctaginaceae): Insight From Phylogeography and Dispersal Corridors in the Himalaya-Hengduan Biodiversity Hotspot. Front. Plant Sci. 10:1721. doi: 10.3389/fpls.2019.01721
Received: 01 April 2019; Accepted: 06 December 2019;
Published: 31 January 2020.
Edited by:
Jeremy B. Yoder, California State University, Northridge, United StatesReviewed by:
Juan Francisco Ornelas, Instituto de Ecología (INECOL), MexicoMario Fernández-Mazuecos, Real Jardín Botánico (RJB), Spain
Copyright © 2020 Rana, Luo, Rana and Sun. This is an open-access article distributed under the terms of the Creative Commons Attribution License (CC BY). The use, distribution or reproduction in other forums is permitted, provided the original author(s) and the copyright owner(s) are credited and that the original publication in this journal is cited, in accordance with accepted academic practice. No use, distribution or reproduction is permitted which does not comply with these terms.
*Correspondence: Hang Sun, sunhang@mail.kib.ac.cn
†These authors have contributed equally to this work