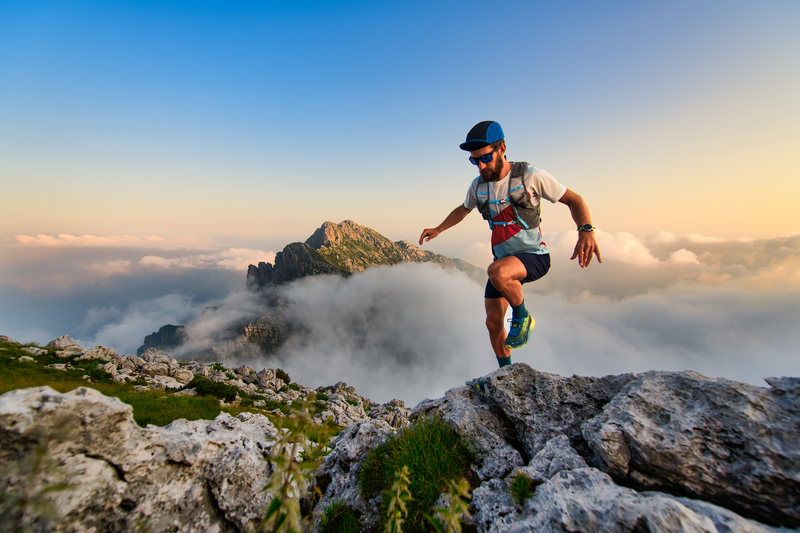
94% of researchers rate our articles as excellent or good
Learn more about the work of our research integrity team to safeguard the quality of each article we publish.
Find out more
ORIGINAL RESEARCH article
Front. Plant Sci. , 16 July 2019
Sec. Plant Systematics and Evolution
Volume 10 - 2019 | https://doi.org/10.3389/fpls.2019.00913
The Sino-Japanese Floristic Region (SJFR) is a key area for plant phylogeographical research, due to its very high species diversity and disjunct distributions of a large number of species and genera. At present, the root cause and temporal origin of the discontinuous distribution of many plants in the Sino-Japanese flora are still unclear. Diabelia (Caprifoliaceae; Linnaeoideae) is a genus endemic to Asia, mostly in Japan, but two recent discoveries in China raised questions over the role of the East China Sea (ECS) in these species' disjunctions. Chloroplast DNA sequence data were generated from 402 population samples for two regions (rpl32-trnL, and trnH-psbA) and 11 nuclear microsatellite loci were screened for 549 individuals. Haplotype, population-level structure, combined analyses of ecological niche modeling, and reconstruction of ancestral state in phylogenies were also performed. During the Last Glacial Maximum (LGM) period after the Tertiary, Diabelia was potentially widely distributed in southeastern China, the continental shelf of the East China Sea and Japan (excluding Hokkaido). After LGM, all populations in China have disappeared except those in Zhejiang which may represent a Glacial refuge. Populations of Diabelia in Japan have not experienced significant bottleneck effects, and populations have maintained a relatively stable state. The observed discontinuous distribution of Diabelia species between China and Japan are interpreted as the result of relatively ancient divergence. The phylogenetic tree of chloroplast fragments shows the characteristics of multi-origin evolution (except for D. sanguinea). STRUCTURE analysis of nuclear Simple Sequence Repeat (nSSR) showed that the plants of the Diabelia were divided into five gene pools: D. serrata, D. spathulata, D. sanguinea, D. ionostachya (D. spathulata var. spathulata-Korea), and populations of D. ionostachya var. ionostachya in Yamagata prefecture, northern Japan. Molecular evidence provides new insights of Diabelia into biogeography, a potential glacial refuge, and population-level genetic structure within species. In the process of species differentiation, ECS acts as a corridor for two-way migration of animals and plants between China and Japan during glacial maxima, providing the possibility of secondary contact for discontinuously distributed species between China and Japan, or as a filter (creating isolation) during glacial minima. The influence of the ECS in speciation and biogeography of Diabelia in the Tertiary remains unresolved in this study. Understanding origins, evolutionary histories, and speciation will provide a framework for the conservation and cultivation of Diabelia.
The northern hemisphere in the early Tertiary period was the source of significant speciation events at medium to high latitudes due to its warm and humid climate (Tiffney, 1985a,b). Beginning in the early Pleistocene, these species began to migrate gradually to low latitudes as the climate became colder (Wolfe, 1971). From the late tertiary to the beginning of the Quaternary, three Glacial (Ice Age) refuge areas developed in East Asia, North America, and southwestern Europe. In East Asia, the only limited glacial cover was formed during the Quaternary glacial period (Qian, 1999). Coupled with a complex geographical environment, a very high number of isolated phylogenetic lineages survived in East Asia (Wen et al., 1998; Wen, 1999; Qiu et al., 2011). The Sino-Japanese flora (SJFR) in East Asia has the largest diversity of temperate plant species in the world (Qiu et al., 2011), and is also a very significant glacial refuge during the Quaternary Ice Age, with significant population fragmentation and subsequent allopatric speciation (Qiu et al., 2011). The Land-bridge islands, formed by the China, continental shelf of the East China Sea and the Japanese archipelago (Whittaker et al., 2007), is an ideal environment for studying the genetic effects of geographic isolation on species formation (Li et al., 2010; Qiu et al., 2011). It differs from two neighboring floristic regions, the Qinghai Tibetan Plateau and the Sino Himalayan regions as its fragmentation is dominated by sea level changes in the East China (Qian and Ricklefs, 2000; Qiu et al., 2011). Palaeobiome reconstructions have demonstrated that the warm deciduous forests of the SJFR extended over vast regions of the mainland (c. 1 million km2) that developed in the East China Sea (ECS) during the Last Glacial Maximum (LGM; c. 21,000 years BP) as well as cool Quaternary periods when ocean levels were c. 85–140 m lower (Millien-Parra and Jaeger, 1999; Wang, 1999; Siddall et al., 2003). Many uncertainties remain about the ECS's role in speciation processes in the SJFR (Qiu et al., 2011; Zhai and Silman, 2012). Some studies have shown that SJFR provides a strong biogeographic barrier, with Kalopanax semptemlobus exhibiting strong genetic differentiation or even species differentiation on both sides of the ECS Qiu et al., 2009a; Sakaguchi et al., 2012. However, yet another study shows no obvious genetic differentiation (e.g., Cercidiphyllum japonicum; Qi et al., 2012). Hence, whether ECS affects the differentiation of discontinuously distributed plants on both sides of the East China Sea remains unclear, so it is necessary to explore the role of the ECS in speciation and species migration through additional case studies. Few studies have examined the very uneven distribution of species diversity between China and Japan, with most studies focusing on species that are widely distributed in Japan (Sakaguchi et al., 2012). Moreover, the most detailed studies only focused on a single species, and few studies have included all the species of a genus or a monophyletic clade (Zhai and Silman, 2012).
Diabelia is a genus in Caprifoliaceae subfamily Linnaeoideae that was recently segregated from Abelia (Landrein, 2010; Wang et al., 2015). Diabelia has been resolved as sister to the genus Dipelta, and together they form a clade with Kolkwitzia (Wang et al., 2015). While the genus is very distinct, species delimitation within Diabelia is controversial and unclear at present. Traditionally, Diabelia included three species with an unusually disjunct distribution pattern in Japan, East China, and Korea (Liang et al., 2004; Zhou and Wen, 2006; Yang et al., 2011; Shin et al., 2012; see Figure 1). Hara (1983) recognized three species primarily based on the number and length of sepals, with additional varieties and forms recognized on the basis of hair types, nectary shape, fusion of nectaries and corolla, and corolla color. Diabelia spathulata (Sieb. & Zucc.) Landrein is distributed in South, central and northern areas of Japan (from Aomori to Saga Prefectures) except Hokaido (Hara, 1983) and three varieties recognized (as Abelia spathulata var. spathulata, var. sanguinea Makino, and var. stenophylla Honda). The second species, D. serrata (Sieb. & Zucc.) Landrein, is widely distributed in southern Japan (from Shiga to Kagoshima Prefectures) and also contains a number of infra-specific varieties and forms (as Abelia serrata var. serrata f. serrata, var. serrata f. obspathulata (Koidz.) Sugimoto, and f. tomentosa (Koidz.) Nakai). These two species also have scattered distributions along the southeast coast of East China (e.g., Sihaishan Mts, Wenzhou, Zhejiang; Liang et al., 2004; Zhou and Wen, 2006). The third taxon, D. tetrasepala (Koidz.) Landrein, is a narrow endemic from Japan (from Fukushima to Fukuoka Prefectures), where its range partly overlaps with both D. spathulata and D. serrata (Hara, 1983). The number of sepals is generally regarded as a key feature to differentiate Diabelia species, i.e., there are five sepals in D. spathulata, two or three sepals in D. serrata and four large plus a reduced sepal in D. ionostachya var. tetrasepala (Koidz.) Landrein (Hara, 1983; Landrein, 2010; Landrein and Farjon, 2019). However, based on recent herbarium studies and field work, we found that the number of sepals is not stable in Diabelia, rather the number of sepals ranges from two to five in D. serrata, from four to five in D. spathulata and there are four large and one reduced sepal in D. ionostachya var. tetrasepala. Landrein and Farjon (2019) have proposed the recognition of four species (i.e., D. serrata, D. spathulata, D. sanguinea, and D. ionostachya (Nakai) Landrein) based on morphological characters (the number of sepals, nectary cushion position and corolla color). Diabelia serrata is distributed in Shikoku, Kyushu and the central and southern regions of Honshu. Whereas, Diabelia ionostachya is mainly distributed in Honshu and Shikoku, but rarely in Kyushu. Diabelia sanguinea grows mainly in central and northeastern Honshu. Diabelia spathulata is widely distributed in Honshu and Shikoku, but rare in Kyushu and Korea. A small number of populations of D. serrata and D. ionostachya are distributed in Zhejiang, China Landrein and Farjon, 2019. This revision maintains the definition of two species recognized by Hara (1983), D. serrata and D. spathulata. Abelia spathulata var. sanguinea is recognized at species level as D. sanguinea (Makino) Landrein. The overlooked name Abelia ionostachya Nakai is recombined in Diabelia and two varieties are recognized, var. stenophylla Landrein and var. tetrasepala. For convenience and uniformity, the samples used in our analyses were assigned names based on Landrein and Farjon's proposed morphology-based taxonomy.
Figure 1. Species range, geographical distribution of haplotypes, and phylogenetic relationship among four Diabelia species. Geographical distribution of 37 chloroplast haplotypes in sampled locations. Shading indicates the native range of each species (Hara, 1983; Landrein and Farjon, 2019). Four haplotypes (H13, H25, H28, and H29) are multiple species shared haplotypes, of which H25 is shared haplotype of D. ionostachya, D. sanguinea, and D. spathulata. H13 is the shared haplotype for D. ionostachya and D. serrata; H28 is the shared haplotype for D. ionostachya and D. sanguinea; H29 is the shared haplotype for D. ionostachya and D. spathulata, respectively.
The small fruits (achenes) of Diabelia are likely to be wind dispersed (Zhou and Wen, 2006) and might be easily blown across the ECS by strong winds associated with typhoons or large storms. The sister lineage of Diabelia is Dipelta, which is endemic to southwest China (e.g., Shaanxi, Gansu, and Yunnan province), and while Diabelia is mainly distributed in Japan, its origin and subsequent dispersal patterns remain uncertain (Wang et al., 2015).
In the present investigation, we assessed the phylogenetic relationships and the phylogeographic history of Diabelia taxa to determine when these species diverged, and which processes (dispersal or vicariance) most likely led to the current disjunct distribution of D. ionostachya and D. serrata across the ECS. We utilized population-level sampling to determine the relations between species and potential hybrid populations in Diabelia. Our objectives were (i) to refine the timeframe in which Diabelia populations diverged by fitting both datasets (nSSR and cpDNA) to an Isolation with Migration (IM) model; (ii) to model the species' potential distributions in response to past climatic changes (LGM) and to the present day using Ecological Niche Modeling (ENM); (iii) Through a comprehensive analysis of nSSR and cpDNA data we aimed to determine whether the current biogeography of Diabelia is due to the spread from China or Japan by long-distance dispersal (e.g., typhoons) or during the LGM. Finally, we questioned whether D. ionostachya var. tetrasepala could be a hybrid of D. serrata (calyx with 2–3 sepals) and D. spathulata or D. ionostachya (calyx with five sepals). We used these data to assess infra-specific variation within Diabelia species.
We collected leaf samples from 602 Diabelia individuals from 69 sites throughout the range of this genus in Japan (64 populations), East China (Zhejiang, four populations) and Korea (one population, Figure 1; Table 1). Chloroplast (cp) DNA data were generated from 402 samples (representing all 69 sites) and nSSR data was generated from 549 individuals (Table 2). Samples were selected to provide a representation of both geographic and morphological diversity within the genus. As some specimens were not fertile at the time of collection, or their identity was not recorded previously, morphological data for key reproductive organ traits could not be assessed.
Table 2. Genetic characteristics of 59 Diabelia populations from China, Japan, and Korean surveyed for chloroplast (cp) DNA sequences and nSSR variation.
Total genomic DNA was extracted from silica-dried leaf tissue using the modified cetyltrimethyl ammonium bromide (CTAB) protocol of Doyle and Doyle (1987). We amplified 11 nuclear microsatellite loci that were isolated from three species of Diabelia by Zhao et al. (2017). We selected two cpDNA intergenic spacer (IGS) regions (i.e., rpl32-trnL, trnH-psbA) which showed consistent amplification and have also been reported to be the most variable markers for subfamily Linnaeoideae (Wang et al., 2015). These markers allowed sampling of a larger number of individuals and testing of relationships between populations. The primers of these two chloroplast DNA regions are described in Dong et al. (2012) and Wang et al. (2015). Each polymerase chain reaction amplification for cpDNA and microsatellites was carried out in a 25 μL volume with the following reagents: Taq polymerase buffer, 10 ng total genomic DNA, 0.1–1 μl each of both forward and reverse primers, 12.5 μl 2 × Taq PCR MasterMix (Jinbaite, Biotechnology Co., Beijing, China), and ddH2O was added to make up the total volume of 25 μl. The thermal cycling conditions were 3 min at 95°C, followed by 43 cycles of 30 s at 95°C, 30 s at 54–58°C, and 1 min at 68°C, with a final extension of 20 min at 68°C.
The final PCR products (chloroplast DNA and microsatellite sequences) were purified with PEG8000 (Polyethylene Glycol) and sequenced using ABI Prism BigDye Terminator Cycle Sequencing Kits v. 3.1 on an ABI 3730xl DNA Sequencer (Life Technologies, 5791 Van Allen Way, Carlsbad, California 92008, USA) following the manufacturer's instructions.
GeneMarker 2.6.4 (LIZ500; Hulce et al., 2011) was used for Genotyping analysis. GenAlEx v. 6.1 (Peakall and Smouse, 2006) was used for estimating the standard genetic diversity parameters at both population and species levels for: percentage of polymorphic loci when the most common allele had a frequency of <0.99 (%P99); mean number of alleles per locus (A); observed heterozygosity (Ho); unbiased expected heterozygosity or Nei (1978) gene diversity (He); genetic diversity indices of the populations of Diabelia; sum of squared variation within populations; and source of variation. GenePop v.4.2.2 (Raymond and Rousset, 1995b) was used for checking genotypic linkage disequilibrium between pairs of loci at the population level and across all populations, but also for calculating possible deviations from Hardy-Weinberg (H–W) equilibrium within each population and for each locus per population. For both calculations, GenePop used a Fisher's exact test following the Markov chain (MC) algorithm (Raymond and Rousset, 1995a). The frequency of null alleles was estimated by following the expectation maximization (EM) algorithm (Dempster et al., 1977) in Free NA (Chapuis and Estoup, 2006). Free NA was also used to estimate FST values between pairs of populations and species (with and without the method of excluding (ENA) correction for null alleles; Chapuis and Estoup, 2006). Pairwise genetic distance between populations was calculated using two algorithms, Nei (1972) standard genetic distance (DS) and Roger's (1972) distance (DR). These distance matrices were converted into UPGMA (unweighted pair-group method using arithmetic averages) dendrograms (after 1,000 bootstrap replicates) employing the programs Populations v.1.2.30 (Langella, 1999) and TreeView v.1.6 (Page, 1996).
The genetic structure was assessed through five different methods:
First, STRUCTURE v. 2.3.4 (Pritchard et al., 2000), a widely-employed clustering software that is based on a Bayesian algorithm, was used. On the basis of preliminary runs, K was run from 1 to 20 (10 independent runs were run for each K value) assuming an admixture model with correlated allele frequencies, and with a priori grouping of individuals into populations (but not into species). The length of the burn-in period and the MCMC runs were set to 50,000 and 500,000, respectively. The most likely value of K was determined both by choosing the smallest K after the log probability of data [ln Pr(X|K)] values reached a plateau (Pritchard et al., 2000) and by the ΔK statistic of (Evanno et al., 2005) with the aid of Structure Harvester v. 0.6.94 (Earl, 2012). For the most likely K, Clumpp v. 1.1.2b (Jakobsson and Rosenberg, 2007) was used to combine the results of the 10 independent runs of the best K. To plot the output result produced by Clumpp, we used the program Distruct v. 1.1.
Second, a molecular variance analysis (AMOVA) was run with the aid of GenAlex and Arlequin, calculating the fixation indices and percentage of molecular variance at four hierarchical levels and the percentage of molecular variance at five hierarchical levels (based on the structure results of nSSR; R1, R2, R3, R4, R5): (i) among species; (ii) among populations; (iii) calculation of the sum of squared variation within populations; and (iv) intraspecific variance. Similar to the first level, several possible combinations of populations were used, and based on the STRUCTURE analysis the clusters were divided into five geographic regions: (A), West Japan; (B), South Japan (including Nara and Wakayama); (C), Southeast Japan (including Ibaraki, Chiba, Kanagawa); (D), Northwest Japan (including Akita, Yamagata, Niigata), China (Wenzhou) and Korea (Gyeongsangnam-do); and (E), Northeast Japan (including Fukui, Shiga, Kyoto); where the study populations occurred (Japan, Korea, and China), and the four species (D. serrata, D. spathulata, D. ionostachya, and D. sanguinea). Third, a Principal Coordinates Analysis (PCoA) at the population level was carried out with GenAlEx. Fourth, putative genetic barriers between populations were detected with the software Barrier v. 2.2 (Manni et al., 2004); significance of identified barriers was tested by bootstrapping 1,000 Nei's genetic distance DA (Nei and Takezaki, 1983) matrices that were previously obtained with Microsatellite Analyzer (MSA) v. 4.05 software (Dieringer and Schlötterer, 2003). Fifth, a Mantel test (1,000 permutations) between the pairwise population matrix of Nei Genetic Identity and the matrix of the log-transformed geographical distances was carried out using the software GenAlEx.
Gene flow was estimated with two time-frameworks. First, we estimated recent (i.e., within the recent 2–3 generations) migration rates between the clusters identified with STRUCTURE; between the three geographical regions where the populations under this study occurred; and between the four species using a Bayesian assignment test with the software BayesAss v. 3.0 (Wilson and Rannala, 2003). A total of 2 × 107 MCMC iterations were run, with a burn-in of 2 × 106 iterations and a sampling frequency of 2,000; while the mixing parameters deltaA, deltaF, and deltaM were set to 0.40, 0.60, and 0.20. The convergence and stability of the MCMC algorithm were checked by visual inspection of plotted posterior parameter estimates using the software Tracer v. 1.6 (Rambaut et al., 2014). Second, historical mutation-scaled migration rates (M = m/μ, where m is migration rate and μ is mutation rate per generation) were estimated by using MIGRATE-N v. 3.6.4 (Beerli and Felsenstein, 2001) with the same sampling scheme as that of BayesAss. Ten replicates were run under a Brownian motion model, assuming a constant mutation rate for all the loci. With a Bayesian approach, a long chain with 20,000 genealogies to sample was run, with a sampling increment of 100 (thereby generating 2,000,000 genealogies for each replicate); the burn-in was set to 20,000. A static heating scheme was chosen (temperatures were specified to 1.00, 1.50, 3, and 1 × 106), with uniform prior distribution both for Θ and M (min: 0; max: 100; delta: 10). The effective number of migrants per generation (Nm) among populations was estimated using the formula 4 Nm = ΘM (Beerli and Felsenstein, 2001). Total immigration and emigration rates for each population were obtained by summing values of Nm. The analyses were carried out at the CIPRES bioinformatic facility (Miller et al., 2010).
DnaSP v. 5 (Librado and Rozas, 2009) was used to determine haplotypes (based on two chloroplast fragments rpl32-trnL and trnH-psbA from 402 individuals; Figure S1).
We edited the sequences and assembled them with Sequencher v. 4.7 (Gene Codes Corporation, Ann Arbor, Michigan, USA). We aligned the newly generated sequences and sequences from GenBank with Clustal W implemented in MEGA X version 10.0.5 software (Kumar et al., 2018) and they are further manually adjusted with Se-Al v. 2.0 (Rambaut, 1996). Prior to concatenating the dataset of each marker, incongruence length difference (ILD) tests were performed on two datasets. We concatenated the datasets with SequenceMatrix (Vaidya et al., 2011). All the sequences identified in the present study were deposited in GenBank (Table S1). Phylogenetic analyses were independently performed for the two chloroplast markers (psbA-trnH and rpl32-trnL), representative sample sequences of haplotypes are shown in Figure 2. As Dipelta is a sister genus of Diabelia (Wang et al., 2015), we took Dipelta as an outgroup for the phylogenetic tree construction in this study.
Figure 2. Maximum Likelihood tree inferred from RAxML for 37 haplotypes in Diabelia based on concatenated sequences of chloroplast DNA (trnH-psbA, rpl32-trnL) with Dipelta yunnanensis as the outgroup. Numbers associated with branches are ML bootstrap support values. Population migration direction inferred (a, b, and c) from haplotype phylogenetic tree of Diabelia.
The evolutionary model (best of fit) that was most appropriate for all the data was selected according to the corrected Akaike Information Criterion (AICc) implemented in jModelTest (Santorum et al., 2014). The probabilistic analysis was conducted using RAxML (v. 8) (Stamatakis, 2014) for maximum likelihood (ML) using the default parameters with bootstrap support of 1,000 pseudo-replicates and MrBayes v. 3 (Huelsenbeck and Ronquist, 2001) for Bayesian inference with 5 × 105 generations with two runs and four chains following the substitution matrix assessed as mentioned above. Both analyses were performed on the CIPRES Science Gateway website (Miller et al., 2010) and cladograms were edited with the program TreeGraph2 beta v. 2.0 (Stöver and Kai, 2010).
Ecological niche modeling (ENM) was performed to evaluate the potential distribution of the four extant species of Diabelia under present climatic conditions and projected back to the Last Glacial Maximum (LGM, ca. 21,000 year BP). We employed the maximum entropy algorithm, as implemented in MaxEnt v. 3.3 (Phillips et al., 2006). The current distribution information for the four Diabelia species was obtained from Hara (1983) and Landrein and Farjon (2019). Data were based on specimens deposited in the main Chinese herbaria (through the Chinese Virtual Herbarium platform; www.cvh.ac.cn), from the collection records of Laboratory for Plant Systematics in Chiba University (bean.bio.chiba-u.jp/eng/index.php; Table S2). In total, after removing duplicate records within each pixel (2.5 arc-min, ca. 5 km), we obtained 67, 67, 69, and 31 records for D. serrata, D. spathulata, D. ionostachya, and D. sanguinea, respectively. A set of 19 bioclimatic variables at 2.5 arc-min resolution covering the distribution range (and neighboring areas) for all four species under current conditions (1950–2000) were downloaded from the WorldClim website (www.worldclim.org; Hijmans et al., 2005). After a correlation analysis of the bioclimatic variables within the study area (with the help of SDM toolbox v. 1.1b; Brown, 2014, we selected a smaller set of 13 (relatively) uncorrelated variables: mean diurnal range (bio2), isothermality (bio3), temperature annual range (BIO5-BIO6) (bio7), mean temperature of the wettest quarter (bio8), mean temperature of the driest quarter (bio9), mean temperature of the warmest quarter (bio10), mean temperature of coldest quarter(bio11), annual precipitation (bio12), precipitation of wettest month (bio13), precipitation of the driest month (bio14), precipitation seasonality (bio15), and precipitation of the warmest quarter (bio18), precipitation of coldest quarter (bio19). The selection of variables from pairs or groups of highly correlated (r ≥ 0.9) variables were made based on their relative contribution to the model (percent contribution, permutation importance jackknife of regularized gaining train), making sure that the most influential variables for the two outgroup species were selected.
The distribution model under current conditions was projected to the LGM using palaeoclimatic layers simulated by the Community Climate System Model version 4 (CCSM4; Gent et al., 2011), the Model for Interdisciplinary Research on Climate Earth System Model (MIROC-ESM; Watanabe et al., 2011), and the New Earth System Model of Max Planck Institute for Meteorology (MPI-ESM; http://www.mpimet.mpg.de/en/science/models/mpi-esm/). Replicate runs (20) of MaxEnt (using the “subsample” method) were performed to ensure reliable results. Model performance was assessed using the area under the curve (AUC) of the receiver operating characteristic plot, with 25% of the localities randomly selected to test the model. AUC scores may range between 0.5 (randomness) and 1 (exact match), with those above 0.9 indicating good performance of the model (Swets, 1988). The MaxEnt jackknife analysis was used to evaluate the relative importance of the 13 bioclimatic variables employed, based on their gain values when used in isolation. To convert the continuous value projection to a binary presence/absence distribution, we applied the maximum sensitivity plus specificity logistic threshold, which is very robust with all types of data (Liu et al., 2016). All ENM predictions were visualized in ArcGIS v.10.2 (ESRI, Redlands, CA, USA).
The total alignment of the two chloroplast regions (trnH-psbA, rpl32-trnL) surveyed across the 402 individuals of Diabelia was 1,127 bp long. Thirty-seven haplotypes (H1–37) were identified across the 51 populations of Diabelia (Figure 1). Four haplotypes (H13, H25, H28, and H29) were found to be shared by multiple species. H25 was shared haplotype of D. ionostachya, D. sanguinea, and D. spathulata. H13 was a shared haplotype between D. ionostachya and D. serrata. H28 was a shared haplotype between D. ionostachya and D. sanguinea, while H29 was the shared haplotype between D. ionostachya and D. spathulata (Figure 1).
As currently defined, Diabelia serrata has 18 haplotypes, mainly distributed in the southwest of Japan, with diversity ranging from 0.282 to 0.75 (cpDNA, Table 2). We found 15 haplotypes in D. spathulata, mainly distributed in central and southern Japan, and the diversity ranged from 0.25 to 0.667 (cpDNA, Table 2). There were three haplotypes (H25, H28, and H30, Figure 1) in D. sanguinea, mainly distributed in the northeast of Japan (e.g., Akita, Toyama), and the diversity ranged from 0 to 0.087 (cpDNA, Table 2). There were six haplotypes (H13, H23, H25, H28, H29, and H37) in D. ionostachya, distributed in Wenzhou (China) and Saitama (Honshu), and the diversity ranged from 0 to 0.083 (Figure 1; Table 2).
Based on the two cpDNA regions (rpl32-trnL, trnH-psbA), D. serrata formed a monophyletic group (BS = 88, PP = 0.54, Figure 2; Figure S1) with the inclusion of one sample of D. ionostachya var. tetrasepala (H13). The first diverging clade included D. serrata in Kagoshima (Table 1, KAG3; haplotype H17), and Zhejiang (Table 1, WEN2; haplotype H16, Figure 1). Diabelia spathulata, D. ionostachya, and D. sanguinea were not monophyletic in these analyses, with intermixed samples forming a series of clades that were sequentially sister to D. serrata, though the exact relationships between some clades are only weakly supported (Figure 2; Figure S1).
Diabelia ionostachya var. wenzhouensis Landrein from China was also weakly supported as sister to D. spathulata in Japan (Figure 2; Figure S1, Bootstrap value (BS) = 56, PP = 0.68). Diabelia spathulata in Nara, Ehime, Aichi and Kochi Prefectures (H7, H18, H33, and H34) were found to be sister to D. ionostachya var. wenzhouensis (H37), sharing a common ancestor. Diabelia spathulata showed a pattern of ancient divergence between populations. The earliest diverging clade of D. spathulata in Japan is distributed in Kochi (Figure 2, D. spathulata var. spathulata Kochi6 (H18). Diabelia spathulata from Korea formed a clade with D. ionostachya var. ionostachya from Yamagata (BS = 88, PP = 1) (Figure 2), likely representing a dispersal event between Japan and Korea.
A total of 549 individuals from 66 populations across the range of the genus were genotyped with nSSR (some representative individuals were selected). All the 11 surveyed microsatellites were polymorphic across populations. Significant linkage disequilibrium was detected in 158 out of 1,207 locus–locus comparisons (about 13%), although only nine persisted after the implementation of Bonferroni's correction (Rice, 1989). A significant deviation from H–W equilibrium expectations (P < 0.05) was observed for 117 of 549 validity tests, mostly showing a higher than expected frequency of homozygosity. The values of null allele frequency at all loci were very low, averaging 0.051 [range = 0.027 (locus 8)−0.114 (locus 7)]; low percentages of null alleles are not expected to cause significant biases in genetic diversity estimates (cf. Dakin and Avise, 2004; Orsini et al., 2008). The differences between the “raw” values of FST (one of the most sensitive parameters when null alleles occur; Chapuis and Estoup, 2006; Chapuis et al., 2008) and those after correcting for the presence of null alleles in our dataset were negligible (<3%). Nevertheless, there was a positive correlation between the frequency of null alleles and FIS values per locus within (N = 356, R2 = 0.709, P = 0.000) and across populations (N = 11, R2 = 0.701, P = 0.001), indicating that the significant positive deviations from H–W equilibrium can be at least partly attributed to the presence of null alleles.
A total of 187 alleles were detected within the study system, ranging from 10 (locus 9) to 25 (locus 11) and averaging 17 alleles per locus (Table 2). The number of polymorphisms was only slightly higher for populations of D. sanguinea and D. ionostachya compared to D. serrata and D. spathulata, both for allelic richness and for heterozygosity (Table 3), even though sample numbers for D. sanguinea and D. ionostachya were lower. Indeed, polymorphism values only increased moderately for parameter A (number of alleles) when only populations with N > 10 were considered (Table 2). The most variable populations belonged to D. ionostachya and are distributed in the Northeast region and Tokushima. However, the richest populations in terms of alleles were D. spathulata from Fukui (SP-FUK1) (highest values of A and TA; Total number of alleles) and D. spathulata from Yamagata (SP-YAM2) (the population with most private alleles), whereas the population with the largest value of He was also SP-FUK1. Populations outside Japan showed low genetic diversity, especially D. serrata (the only non-Japanese population) (WEN) (He = 0.273) (Table 2).
Table 3. Analysis of molecular variance (AMOVA) and gene-frequency of Diabelia within species, areas and total based on nSSR and chloroplast DNA variation.
The genetic differentiation within populations of Diabelia based on FST was significant [mean FST (66 populations) = 0.419; Table 4]. The FST values for individual species were also higher (mean FST; D. serrata = 0.288; D. spathulata = 0.358; D. sanguinea = 0.388, D. ionostachya = 0.558. The difference between the lowest and the highest FST was 0.27 (D. serrata, FST = 0.288; D. ionostachya, FST = 0.558) showing low interspecific differentiation. The “among-species” component was also low (8.9%) in AMOVA (Table 4). According to STRUCTURE results of the nSSR data, the mean FST (66 populations) was found to be 0.437 based on AMOVA analysis. The Fst value of each gene-pool was lower than the four species concept (R1 = 0.075; R2 = 0.217; R3 = 0.474; R4 = 0.234; R5 = 0.217, Figure 3). The difference between the lowest and highest FST value was 0.399. The gene pool differentiation was also very high (22.7%; Table 4). The Mantel test showed a low P-value (0.010), indicating a significant correlation between genetic differentiation and geographical distance, thereby suggesting that there is an isolation-by-distance across all studied populations. The greatest variation was found among all the populations of D. serrata (1568), followed by the populations of D. spathulata (1082), D. sanguinea (762), and D. ionostachya (710), respectively. The population of D. spathulata from Fukui (SP-FUK1) had the highest mutation value (240), followed by the population of D. sanguinea from Tochigi (SA-TOC; 137) and the population of D. serrata from Kagoshima (SR-KAG2; 136). At the population level, the four species were found to be diverging at different rates, and D. ionostachya was more variable than other species (Tables 3, 4).
Table 4. F-statistics of the five gene-pool (the result of STRUCTURE-nSSR) and four species within Diabelia.
Figure 3. Results of STRUCTURE analysis for all individuals of Diabelia studied based on nSSR data. Assignment of individuals to genetic clusters at K = 5.
The results of STRUCTURE analyses for all individuals based on the nSSR markers are presented in Figure 3; Figure S2. According to Evanno's approach and plot of mean posterior probability values of each K (Figure S3), K = 5 was the most likely number of genetic clusters for 0.5 million generations. The genetic clustering was highly different from K = 1 to K = 25. However, K = 9 was the most likely number of genetic clusters for 1 million generations. The genetic clustering was highly different from K = 1 to K = 20. Comparing the two graphs of K = 5 and 9 (Figure S2), the cluster diagram of the 1 million generation of K = 9 is more complex than that of the 500,000 generations, perhaps the results of K = 9, in theory, will be more refined, but more refined results also require greater sampling to support these analyses and it would be more persuasive to have at least 1,000 samples of the data in the 1 million generations of K = 9, as our analyzed sample set is too small to give accurate results. Secondly, with more iterations, uncertainty factors may be also magnified and produce inaccurate results. In addition, the results of the 1 million generations of K = 9 does not reflect morphological trait diversity. In contrast, the results of the 500,000 generations K = 5 take into account the size of the cluster and the gene pool in the PCoA (Figure S2), identifying four large clusters and a small red cluster representing an unusual gene pool in the northernmost part of Japan. This small cluster may represent a relatively primitive area of diversity; however, this is difficult to assess as it is represented by relatively few samples. In summary, it is better to merge the possible additional lineages represented by K = 9 into five larger lineages, consistent with the results of our phylogenetic analyses (Figure S2).
The distribution of all Diabelia populations in five distinctive lineages are presented in Figure 3. Diabelia serrata is a distinct lineage (R5; Figure 2). However, Diabelia spathulata (R4) as well as D. ionostachya var. ionostachya (R1; Figure 2). D. ionostachya var. wenzhouensis, D. ionostachya var. tetrasepala and D. spathulata var. spathulata from Korea form another distinct lineage (R3; Figure 2). The result of this structure (K = 5) does not match current taxonomic concepts (Hara, 1983; Landrein, 2010). Samples of D. sanguinea (R2; Figure 2) were often admixed with D. spathulata or D. ionostachya. R1 is mainly located in the Yamagata area of northwestern Japan, Southward in Fukushima, Toyama, Tukui, and nearby regions, and hence there is a degree of introgression. R2 is mainly located in Miyagi, Fukushima, Tochigi area of northeastern Japan, south in Saitama, Tokyo, Shizuoka, and Shiga Prefectures and shows minor introgression. R3 is relatively scattered, occurring in Chinese and Korean populations, but mainly in the northeastern part of Japan in Fukushima Prefecture. Populations in Japan's Tokushima, Kochi, Shimane Prefectures showed low introgression. R4 is mainly located in the central part of Honshu Prefecture, Japan, to the eastern Pacific coast and to Ibaraki Prefecture with scattered populations in the north of Tochigi, Fukushima, Miyagi, and nearby Prefectures show minor introgression. R5 is primarily found in Shikoku and Kyushu Prefectures in southern Japan, and the central region of Honshu, with a limited occurrence in Zhejiang, China. It should be noted that due to the limited number of samples in some clades, the conclusion of K = 5 taxonomic entities requires further investigation with more detailed sampling.
The AUC scores averaged across 10 runs were higher for the four species (mean ± SD, 0.997 ± 0.001 for D. serrata, 0.997 ± 0.002 for D. spathulata, 0.998 ± 0.001 for D. sanguinea, and 0.996 ± 0.001 for D. ionostachya), which supported the predictive power of the model. According to the MaxEnt jackknife tests of variable importance, the precipitation variables were more informative for the model than the temperature ones under the present climate conditions (bio 14, bio 15, bio 18, and bio 19 were invariably very informative for the four species), although bio9 (mean temperature of driest quarter) was also important for all the models. The present-day distributional predictions for the four species were largely congruent with the known occurrences, although additional areas appeared as suitable (cutline 0.1–1 areas in Figure 4; Figure S4), including some areas in eastern and south-eastern China, and for D. spathulata, a thin coastal strip in South Korea. Projections of the species niche to the LGM climate produced considerably different maps of the probability of occurrence at the LGM compared to the present time. In general, with the two LGM models, large areas appeared as suitable in mainland China for all species, although the suitability of the exposed East China Sea shelf and Japan greatly varied depending on the model and the species (Figure 4; Figure S4). For four species of Diabelia, the distribution of the two LGM models is very similar; species had a potentially continuous range through most of south-eastern/eastern mainland China, and the contiguous exposed East China Sea shelf (Figure 4; Figure S4). For the CCSM and MIROC model (MIROC model simulates somewhat warmer and dryer LGM conditions compared to CCSM within the study area; mean annual temperature, −22.2 to 26.4 °C (average, 3.6°C) for CCSM and −11.0 to 25.4°C (average, 8.5°C) for MIROC; annual precipitation, 70–5,531 mm/year (average, 921 mm/year) for CCSM and 58–3,982 mm/year (average, 549 mm/year for MIROC). The distribution density and range of Diabelia under the MIROC model were slightly lower than the distribution density and range under the CCSM model, but the differences were not significant. The potential range for Diabelia species in mainland China would have been much smaller, while habitats would have remained stable across most of the current distribution area within Japan (Figure 4; Figure S4).
Figure 4. Comparison of potential distributions as probability of occurrence for Diabelia serrata, D. spathulata, D. sanguinea, and D. ionostachya (Landrein and Farjon, 2019), at the CCSM climatic scenarios of the Last Glacial Maximum (LGM, ca. 21,000 years BP). The maximum training sensitivity plus specificity logistic threshold has been used to discriminate between suitable (cutline 0.1–1 area) and unsuitable habitat. The darker color indicates a higher probability of occurrence.
In terms of genetic diversity, the geographic distribution of Diabelia species is highly discontinuous (Figure 1). However, populations of Diabelia species still show similar or even higher diversity at haplotype (cpDNA) and nucleotide levels (Ht = 0.926; πt = 0.0072; Tables 2, 3) than that of other endemic populations of China and Japan [e.g., Croomia japonica and C. heterosepala, (Li et al., 2008); Dysosma versipellis, (Qiu et al., 2009a)]. Diabelia species have high microsatellite genetic diversity (He = 0.736; Tables 2, 3). High genetic diversity usually means a very long evolutionary history (Huang et al., 2001; Qiu et al., 2009b). Wang et al. (2015) estimated chronogram of Linnaeoideae and its relatives based on nine plastid markers data, infers that the species differentiation in Diabelia occurred in the Miocene (5–23 Ma), when the genus may have been widely distributed in southern Asia. However, after the Quaternary Period climate shocks and more recent environmental constraints, the main distribution of Diabelia in Asia was confined to Japan. The main distribution in southern China (during the LGM period) had disappeared except in Zhejiang, and a large number of populations are likely to have gone extinct (Qiu et al., 2011; Qi et al., 2012; Sakaguchi et al., 2012; Zhai et al., 2012). In the phylogenetic tree based on chloroplast markers, Diabelia shows a typical multi-origin distribution pattern (Figure 2; Figure S5). Therefore, the high genetic variability of Diabelia may also be due to the inheritance of variation from ancestral populations. Based on the genetic diversity analysis of microsatellite markers, we found relatively low genetic variation at the population level (mean He = 0.385, Table 3). Meanwhile, the microsatellite analysis of Diabelia revealed a high degree of genetic differentiation (Fst = 0.419; Table 4). Similar to Diabelia, Cercidiphyllum, a Chinese-Japanese discontinuous species, has similar genetic characteristics (Qiu et al., 2011; Qi et al., 2012; Sakaguchi et al., 2012; Zhai et al., 2012).
The above population genetic characteristics may reflect certain biological characteristics of Diabelia. In Japan, the main distribution area, Diabelia population-level habitat fragmentation due to climatic variation may have driven current disjunctions (Fu and Jin, 1992). Through bottleneck analysis, we found that only four of the 36 Japanese populations with more than five individuals had experienced recent bottlenecks under the IAM model, indicating that Japanese populations had not experienced serious bottleneck effects during recent climatic variations, which was similar to the previous distribution pattern (ENM, Figure 4). In terms of breeding system, although Diabelia has relatively small winged seeds that can be dispersed by wind (Hufford, 2004), its small size, growth at particular altitudes and undergrowth habitats may significantly reduce the likelihood of dispersal over long distances. Based on the above hypothesis, although Diabelia is widely distributed in Japan and has not experienced serious bottleneck effects in recent history, it shows low genetic diversity and high genetic differentiation among populations. The population genetic history of Diabelia suggests that interruption of gene flow and genetic drift are the main factors driving existing population structures in Japan.
The glacial and interglacial fluctuations caused by Quaternary climate shocks have a great influence on the distribution of species (Comes and Kadereit, 1998; Hewitt, 2000). Most plant distributions undergo expansion and contraction, leading to population migration or extinction, followed by geographical isolation, differentiation and subsequent population expansion (e.g., Liu et al., 2013). Wang et al. (2015) estimated that species differentiation in Diabelia occurred in the Miocene (5–23 Ma), suggesting that extant populations likely differentiated well before the LGM. Compared with the LGM, the Tertiary climate and the relative location simulation of the ECS, China and Japan are not developed enough to predict the role of the ECS in the species differentiation of Diabelia. Although the phylogenetic tree shows that the populations of China and Japanese have significant genetic differentiation (Figure 2). The climate shocks in the LGM period may have had a significant impact on the recent history of Diabelia at a population level compared with the previous period of species differentiation (Qiu et al., 2011; Qi et al., 2012; Sakaguchi et al., 2012, 2017; Zhai et al., 2012). In the haplotype network, the primitive haplotypes associated with Diabelia are determined by inserting an outgroup (Figure 1, Dipelta yunnanensis BOP012201). In the haplotype network in Diabelia, haplotype H23, H29, H36, H37 were found to be connected with haploidy of external groups (Figure 1). It was found that the distribution of ancestral haploidy was very scattered in geographical location, in Zhejiang Province of China, Gyeongsangnam-do of Korea, and Yamagata County of Japan, respectively, suggesting that Diabelia had an extensive distribution in southeast China and Japan (excluding Hokkaido) during the LGM. The predicted results of ENM in LGM also support this conclusion. The distribution records of Diabelia in China are all from one area in Zhejiang Province. In addition, plants growing in glacial refugial areas are generally expected to exhibit corresponding ecological characteristics, such as low dispersal capacity, niche specialization (Hopper, 2009) and harbor unique haplotypes and a relatively higher level of genetic diversity than populations not influenced by glaciation (Comes and Kadereit, 1998; Petit et al., 2003). The populations in Zhejiang Province, which have not been dispersed to other areas, have unique haplotype (H23, H37, Figure 1) and high genetic diversity (WEN5, cp-π: 0.111; Table 2). Another group (WEN4) is represented by only two individuals which do not allow for meaningful assessment. During the Quaternary period, although China's subtropical region experienced severe climatic shocks during the glacial and interglacial periods, large areas of the region remained unfrozen during the LGM period (Axelrod et al., 1996) with many refuge zones for ancient and relict species (e.g., Wang et al., 2009). After the LGM, Diabelia populations in southeastern China may have experienced a large-scale population extinction event, and Zhejiang may be a glacial refuge for Diabelia ionostachya var. wenzhouensis. Our data support the Chinese population as more divergent than other lineages, and it is not the result of a recent dispersal from Japan. At the same time, our ENM results also provide strong evidence for survival of Diabelia in southern China during the LGM period (Figure 4; Figure S4). Similar to the population in China, the population in South Korea also had primitive haplotypes (H36; Figure 1), but did not exhibit high genetic diversity and high genetic differentiation as would be expected for a refugial population (Hewitt, 2000). The degree of genetic diversity was very low (GYE, 0; Table 1), and Bottleneck analysis shows that Korean Diabelia population has experienced a very serious bottleneck effect in its recent history (Table S3). The genetic relationship between the two populations, though far apart from each other, was very close, and both are derived from a common ancestor in our phylogenetic analysis (Figure 2). During the LGM period, it appears there was no population of Diabelia in the Korean Peninsula based on ENM (Figure 4). The population in Korea is more likely the result of very recent long-distance transmission across the Tsushima Strait from northern Japan. Korean populations are related to D. ionostachya not D. spathulata which is accordance with the previous conclusion by Shin et al. (2012).
The altitudinal ranges of species in Diabelia are relatively narrow, but could potentially have expanded significantly during the LGM. Diabelia sanguinea is a higher altitude species (Landrein and Farjon, 2019). In China and East Asia, although most areas were not covered by ice sheets, violent fluctuations in the Quaternary Ice Age also affected the distribution and evolutionary history of flora and fauna in the region (Axelrod et al., 1996). The genealogy of five different alpine plants in Japan was studied by Fujii and Senni (2006), and it was inferred that alpine plants had shelters for warm interglacial periods in the alpine areas in the middle of Honshu Island. Several studies of the endemic Fagus crenata (altitude 600–1,900 m) in Japan have shown that its current distribution pattern formed after the Ice Age by the migration of multiple shelters from the Sea of Japan and the Pacific Rim (Tomaru et al., 1998; Fujii et al., 2002; Okaura and Harada, 2002). Most pine fir sanctuaries are located along the southwest Pacific coast, spreading northward after the Ice Age (Ikeda et al., 2006; Tsumura, 2006). The study on the phylogeography of Potentilla matsumurae (altitude 700–3,100 m) further confirmed the inference that the alpine mountains in the middle of Honshu Island were refugees of the interglacial age (Fujii and Senni, 2006). Fujii and Senni (2006) found that Japan's alpine plants have experienced a different evolutionary history from European alpine plants during the interglacial periods because of the expansion of forests, so they retreated to refugia, both in the mountains, and at low altitudes (Fujii et al., 1997, 1999; Fujii and Senni, 2006; Ikeda et al., 2006; Sakaguchi et al., 2012, 2017; Zhai et al., 2012). Therefore, although the species were formed before LGM, the current altitude range of the four species might still have been influenced by the extreme climate in LGM.
External morphological differences and similar environmental niche among Diabelia populations in different regions are fascinating. Based on the phylogenetic relationships inferred using chloroplast loci and the result of STRUCTURE analysis (nSSR, Figure 3), only D. serrata was found to be a monophyletic group, and consistent with proposed taxonomic boundaries (Landrein and Farjon, 2019). The phylogenetic definition of all other species is at odds with taxonomic boundaries proposed based on morphology alone. There may be several reasons for this: Firstly, the phylogenetic tree used only two chloroplast fragments, with limited mutation sites, and the resulting phylogenetic gene trees may not reflect an accurate species tree for the genus. A second possibility is that populations have diverged, but retained ancestral polymorphisms shared by wider taxa (Sakaguchi et al., 2017), obscuring monophyletic species relationships in the phylogenetic trees. Thirdly, environmentally driven genetic adaptations associated with specific ecotypes may be directly linked to phenotypic plasticity, which can obscure taxonomic boundaries based on morphological traits. Genetic-based adaptive evolution plays a decisive role in expressed morphological traits (Hirano et al., 2017; Sakaguchi et al., 2017). Therefore, the morphological traits expressed in Diabelia populations may be primarily based on selective amplification of genetically-determined traits in different geographical habitats. In addition, co-occurring Diabelia species can readily hybridize, and we also found a large number of gene infiltration events between species in our analyses of nSSR structure (Figure 3). In the actual sample observation process, we found that hybridization may produce intermediate morphological traits that differ from their parents (D. serrata sepal range from 1 to 4; D. sanguinea has many flower colors such as red, pink, or dark-pink; Landrein and Farjon, 2019). This may also be one of the reasons for variation of individual morphological characters within a species. We have speculated that D. ionostachya var. tetrasepala (calyx with five sepals and the adaxial sepal is reduced in size) results from hybridization between D. serrata (calyx with 2–3 sepals) and D. spathulata (calyx with five sepals). In results of nSSR structure, D. ionostachya var. tetrasepala individuals from R4, R5, gene infiltration is low (Figure 3). So it is impossible to accurately determine that D. ionostachya var. tetrasepala is the result of hybridization between D. serrata and D. spathulata. Intermediate individuals produced by interspecific hybridization do make it difficult to classify species accurately in the field. Additional data may enable the underlying discordance between taxonomic concepts and our molecular data to be correctly interpreted.
This study presents the first comprehensive analyses of the phylogeny and biogeography of taxa within Diabelia using phylogeographic data. Furthermore, the role of the ECS in speciation and species migration was explored with Diabelia as a case study. Combining chloroplast, nSSR with ecological niche modeling, we assessed phylogeny and population dynamics in Diabelia. Based on our results, a distinct population of D. ionostachya was identified in the Yamagata prefecture of northern Japan. The divergence of Diabelia species dates back to the mid to late Tertiary. Diabelia presents a typical multi-origin pattern of species evolution. Diabelia species in China represent primitive populations and the isolating function of the East China Sea in Diabelia speciation remains unresolved.
The datasets generated for this study can be found in Tables S1–S4.
H-FW, SL, and SS conceived the ideas and designed methodology. SS and MM collected the materials. K-KZ, HL, and W-XM constructed the species phylogeny cladogram. K-KZ, W-XM, Z-XZ, and TY analyzed the data. H-FW, SL, and RB led the writing of the manuscript. All authors contributed critically to the drafts and gave final approval for publication. All authors contribute equally to the work.
This study was funded by the National Science Foundation of China (31660055 and 31660074) and a start-up fund from Hainan University [kyqd1633 and kyqd1840 (ZR)] and the China Scholarship Council (Grant No. 201907565012), supporting H-FW's research visit to the Smithsonian Institution, Washington, DC, United States. It was also supported by the grants of Basic Research Program, Shenzhen Municipal Government of China (No. JCYJ20150831201123287, No. JCYJ20160331150739027), and the Guangdong Provincial Key Laboratory of Genome Read and Write (Grant No. 2017B030301011).
The authors declare that the research was conducted in the absence of any commercial or financial relationships that could be construed as a potential conflict of interest.
We sincerely thank two reviewers for their constructive suggestions to our initial version of the manuscript, Shuichi Nemoto for extending help in plant materials collection and Jordi López-Pujol and Sonia Herrando-Moraira for their partial help in data analyses.
The Supplementary Material for this article can be found online at: https://www.frontiersin.org/articles/10.3389/fpls.2019.00913/full#supplementary-material
Figure S1. Maximum Likelihood tree (RAxML) for 402 individuals of in Diabelia based on concatenated sequences of chloroplast DNA (trnH-psbA, rpl32- trnL) with seven species (Kolkwitzia amabilis_ BOP012293; Abelia chinensis_BOP012223; Dipelta yunnanensis_BOP012201; Linnaea borealis_ BOP012344; Vesalea floribunda_BOP022783; Zabelia buddleioides_BOP012222; Zabelia dielsii_BOP012228) as outgroups.
Figure S2. Principal coordinate analysis (PCoA) and structural analysis of 59 populations of Diabelia based on nSSR data using codominant genetic distances.
Figure S3. Structural analysis (nSSR) of 549 individuals based on 69 populations of Diabelia. (a) ΔK statistics calculated; (b) Plot of mean posterior probability values of each K.
Figure S4. Comparison of potential distributions as probability of occurrence for Diabelia serrata, D. spathulata, D. sanguinea, and D. ionostachya (Landrein and Farjon, 2019), at present and at two climatic scenarios (MIROC, MPI) of the Last Glacial Maximum (LGM, ca. 21,000 years BP). The maximum training sensitivity plus specificity logistic threshold has been used to discriminate between suitable (cutline 0.1–1 areas) and unsuitable habitat. The darker color indicates a higher probability of occurrence.
Figure S5. Population coordinate maps for Ecological Niche Modeling (ENM) analysis of each species (Diabelia).
Table S1. GenBank Information for voucher specimens for DNA sequencing (rpl32-trnL and trnH-psbA).
Table S2. Longitudinal and latitudinal information of Diabelia sampling sites.
Table S3. Probabilities from tests (Wilcoxon' s) for mutation drift equilibrium in 40 populations of Diabelia under the infinite allele model (IAM), stepwise mutation model (SMM) and two-phase model (TPM) for nSSR data.
Table S4. The bottleneck effect data analyses of nSSR data.
Axelrod, D. I., Al-Shehbaz, I., and Raven, P. H. (1996). “History of the modern flora of China,” in Floristic Characteristics and Diversity of Eastern Asian Plants, eds A. Zhang, S. Wu (New York, NY: Springer, 43–55.
Beerli, P., and Felsenstein, J. (2001). Maximum likelihood estimation of a migration matrix and effective population sizes in n subpopulations by using a coalescent approach. Proc. Natl. Acad. Sci. USA. 98, 4563–4568. doi: 10.1073/pnas.081068098
Brown, J. L. (2014). SDM toolbox: a python-based GIS toolkit for landscape genetic, biogeographic and species distribution model analyses. Methods Ecol. Evol. 5, 694–700. doi: 10.1111/2041-210X.12200
Chapuis, M.-P., and Estoup, A. (2006). Microsatellite null alleles and estimation of population differentiation. Mol. Biol. Evol. 24, 621–631. doi: 10.1093/molbev/msl191
Chapuis, M. P., Lecoq, M., Michalakis, Y., Loiseau, A., Sword, G., Piry, S., et al. (2008). Do outbreaks affect genetic population structure? A worldwide survey in Locusta migratoria, a pest plagued by microsatellite null alleles. Mol. Ecol. 17, 3640–3653. doi: 10.1111/j.1365-294X.2008.03869.x
Comes, H. P., and Kadereit, J. W. (1998). The effect of Quaternary climatic changes on plant distribution and evolution. Trends Plant Sci. 3, 432–438.
Dakin, E., and Avise, J. (2004). Microsatellite null alleles in parentage analysis. Heredity 93:504. doi: 10.1038/sj.hdy.6800545
Dempster, A. P., Laird, N. M., and Rubin, D. B. (1977). Maximum likelihood from incomplete data via the EM algorithm. J. R. Stat. Soc. Series B Methodol. 39, 1–38. doi: 10.1111/j.2517-6161.1977.tb01600.x
Dieringer, D., and Schlötterer, C. (2003). Microsatellite analyser (MSA): a platform independent analysis tool for large microsatellite data sets. Mol. Ecol. Notes 3, 167–169. doi: 10.1046/j.1471-8286.2003.00351.x
Dong, W., Liu, J., Yu, J., Wang, L., and Zhou, S. (2012). Highly variable chloroplast markers for evaluating plant phylogeny at low taxonomic levels and for DNA barcoding. PLoS ONE 7:e35071. doi: 10.1371/journal.pone.0035071
Doyle, J. J., and Doyle, J. L. (1987). A rapid NA isolation procedure for small quantities of fresh leaf tissue. Phy. Bull. 19, 11–15.
Earl, D. A. (2012). Structure harvester: a website and program for visualizing structure output and implementing the Evanno method. Conserv. Genet. Resour. 4, 359–361. doi: 10.1007/s12686-011-9548-7
Evanno, G., Regnaut, S., and Goudet, J. (2005). Detecting the number of clusters of individuals using the software structure: a simulation study. Mol. Ecol. 14, 2611–2620. doi: 10.1111/j.1365-294X.2005.02553.x
Fu, L. K., and Jin, J. M. (1992). China plant red data book? rare and endangered plants. Beijing: SciencePress.
Fujii, N., and Senni, K. (2006). Phylogeography of Japanese alpine plants: biogeographic importance of alpine region of Central Honshu in Japan. Taxon 55, 43–52. doi: 10.2307/25065527
Fujii, N., Tomaru, N., Okuyama, K., Koike, T., Mikami, T., Ueda, K., et al. (2002). Chloroplast DNA phylogeography of Fagus crenata (Fagaceae) in Japan. Plant. Syst. Evol. 232, 21–33. doi: 10.1007/s006060200024
Fujii, N., Ueda, K., Watano, Y., and Shimizu, T. (1997). Intraspecific sequence variation of chloroplast DNA in Pedicularis chamissonis Steven (Scrophulariaceae) and geographic structuring of the Japanese “Alpine” plants. J. Plant. Res. 110, 195–207. doi: 10.1007/BF02509308
Fujii, N., Ueda, K., Watano, Y., and Shimizu, T. (1999). Further analysis of intraspecific sequence variation of chloroplast DNA in Primula cuneifolia Ledeb (Primulaceae): implications for biogeography of the Japanese alpine flora. J. Plant. Res. 112, 87–95. doi: 10.1007/PL00013866
Gent, P. R., Danabasoglu, G., Donner, L. J., Holland, M. M., Hunke, E. C., Jayne, S. R., et al. (2011). The community climate system model version 4. J. Clim. 24, 4973–4991. doi: 10.1175/2011JCLI4083.1
Hara, H. (1983). A revision of Caprifoliaceae of Japan with reference to allied plants in other districts and the Adoxaceae. Ginkgoana 5, 1–336.
Hewitt, G. (2000). The genetic legacy of the Quaternary ice ages. Nature 405, 907–913. doi: 10.1038/35016000
Hijmans, R. J., Cameron, S. E., Parra, J. L., Jones, P. G., and Jarvis, A. (2005). Very high resolution interpolated climate surfaces for global land areas. Int. J. Climatol. 25, 1965–1978. doi: 10.1002/joc.1276
Hirano, M., Sakaguchi, S., and Takahashi, K. (2017). Phenotypic differentiation of the Solidago virgaurea complex along an elevational gradient: insights from a common garden experiment and population genetics. Ecol. Evol. 7, 6949–6962. doi: 10.1002/ece3.3252
Hopper, S. D. (2009). OCBIL theory: towards an integrated understanding of the evolution, ecology and conservation of biodiversity on old, climatically buffered, infertile landscapes. Plant Soil 322, 49–86.
Huang, Y., Street-Perrott, F. A., Metcalfe, S. E., Brenner, M., Moreland, M., Freeman, K. H., et al. (2001).Climate change as the dominant control on glacial-interglacial variations in C3 and C4 plant abundance. Science 293, 1647–1651. doi: 10.1126/science.1060143
Huelsenbeck, J. P., and Ronquist, F. (2001). MrBayes: Bayesian inference of phylogenetic trees. Bioinformatics 17, 754–755. doi: 10.1093/bioinformatics/17.8.754
Hufford, L. (2004). “Hydrangeoideae-Philadelpheae,” in The Families and Genera of Vascular Plants, Vol. 6, ed K. Kubitzki (Berlin; Heidelberg: Springer-Verlag), 202–215.
Hulce, D., Li, X., Snyder-Leiby, T., and Liu, C. J. (2011). GeneMarker® genotyping software: tools to Increase the statistical power of DNA fragment analysis. J. Biomol. Tech. 22, S35–S36.
Ikeda, H., Senni, K., Fujii, N., and Setoguchi, H. (2006). Refugia of Potentilla matsumurae (Rosaceae) located at high mountains in the Japanese archipelago. Mol. Ecol. 15, 3731–3740. doi: 10.1111/j.1365-294X.2006.03054.x
Jakobsson, M., and Rosenberg, N. A. (2007). CLUMPP: a cluster matching and permutation program for dealing with label switching and multimodality in analysis of population structure. Bioinformatics 23, 1801–1806. doi: 10.1093/bioinformatics/btm233
Kumar, S., Stecher, G., Li, M., Knyaz, C., and Tamura, K. (2018). MEGA X: molecular evolutionary genetics analysis across computing platforms. Mol. Biol. Evol. 35, 1547–1549. doi: 10.1093/molbev/msy096
Landrein, S. (2010). Diabelia, a new genus of tribe Linnaeeae subtribe Linnaeinae (Caprifoliaceae). Phytotaxa 3, 34–38. doi: 10.11646/phytotaxa.3.1.4
Langella, O. (1999). Populations 1.2.28: A Population Genetic Software. Available online at: http://www.pge.cnrs-gif.fr/bioinfo/populations/index.php (accessed April 16, 2018).
Li, E. X., Sun, Y., Qiu, Y. X., Guo, J. T., Comes, H. P., and Fu, C. X. (2008). Phylogeography of two East Asian species in Croomia (Stemonaceae) inferred from chloroplast DNA and ISSR fingerprinting variation. Mol. Phylogenet. Evol. 49, 702–714. doi: 10.1016/j.ympev.2008.09.012
Li, J. W., Yeung, C. K. L., Tsai, P. I., Lin, R. C., Yeh, C. F., Yao, C. T., et al. (2010). Rejecting strictly allopatric speciation on a continental island: prolonged postdivergence gene flow between Taiwan (Leucodioptron taewanus, Passeriformes Timaliidae) and Chinese (L. canorum canorum) hwameis. Mol. Ecol. 19, 494–507. doi: 10.1111/j.1365-294X.2009.04494.x
Liang, Z. S., Tsuneo, F., and Wen, J. (2004). Species relationships in Abelia sect. Abelia (Caprifoliaceae) in East Asia and verification of distribution of A. serrata in China: evidence from AFLP analysis. Acta Bot. Yunnan 26, 405–412. doi: 10.1088/1009-0630/6/5/011
Librado, P., and Rozas, J. (2009). DnaSP v5: a software for comprehensive analysis of DNA polymorphism data. Bioinformatics 25, 1451–1452. doi: 10.1093/bioinformatics/btp187
Liu, C., Newell, G., and White, M. (2016). On the selection of thresholds for predicting species occurrence with presence-only data. Ecol. Evol. 6, 337–348. doi: 10.1002/ece3.1878
Liu, J., Moller, M., Provan, J., Gao, L. M., Poude, R. C., and Li, D. Z. (2013). Geological and ecological factors drive cryptic speciation of yewsina biodiversity hotspot. New Phytol. 199, 1093–1108. doi: 10.1111/nph.12336
Manni, F., Guerard, E., and Heyer, E. (2004). Geographic patterns of (genetic, morphologic, linguistic) variation: how barriers can be detected by using Monmonier's algorithm. Hum. Biol. 173–190. doi: 10.1353/hub.2004.0034
Miller, M. A., Pfeiffer, W., and Schwartz, T. (2010). “Creating the CIPRES science gateway for inference of large phylogenetic trees,” in Gateway Computing Environments Workshop (New Orleans, LA), 1–8. doi: 10.1109/GCE.2010.5676129
Millien-Parra, V., and Jaeger, J. J. (1999). Island biogeography of the Japanese terrestrial mammal assemblages: an example of a relict fauna. J. Bio. 26, 959–972. doi: 10.1046/j.1365-2699.1999.00346.x
Nei, M. (1978). Estimation of average heterozygosity and genetic distance from a small number of individuals. Genetics 89, 583–590.
Nei, M., and Takezaki, N. (1983). “Estimation of genetic distances and phylogenetic trees from DNA analysis,” in Proceedings of the 5th World Congress on Genetics Applied to Livestock Production (Guelph, ON), 405–412.
Okaura, T., and Harada, K. (2002). Phylogeographical structure revealed by chloroplast DNA variation in Japanese Beech (Fagus crenata Blume). Heredity 88, 322–329. doi: 10.1038/sj.hdy.6800048
Orsini, L., Corander, J., Alasentie, A., and Hanski, I. (2008). Genetic spatial structure in a butterfly metapopulation correlates better with past than present demographic structure. Mol. Ecol. 17, 2629–2642. doi: 10.1111/j.1365-294X.2008.03782.x
Page, R. D. (1996). TreeView: an application to display phylogenetic trees on personal computers. Bioinformatics 12, 357–358. doi: 10.1093/bioinformatics/12.4.357
Peakall, R., and Smouse, P. E. (2006). GENALEX 6: genetic analysis in Excel. Population genetic software for teaching and research. Mol. Ecol. Notes 6, 288–295. doi: 10.1111/j.1471-8286.2005.01155.x
Petit, R. J., Aguinagalde, I., Beaulieu, J. L., Bittkau, C., Brewer, S., Cheddadi, R., et al. (2003). Glacial refugia: hotspots but not melting pots of genetic diversity. Science 300, 1563–1565. doi: 10.1126/science.1083264
Phillips, S. J., Anderson, R. P., and Schapire, R. E. (2006). Maximum entropy modeling of species geographic distributions. Ecol. Model. 190, 231–259. doi: 10.1016/j.ecolmodel.2005.03.026
Pritchard, J. K., Stephens, M., and Donnelly, P. (2000). Inference of population structure using multilocus genotype data. Genetics 155, 945–959.
Qi, X. S., Chen, C., Comes, H. P., Sakaguchi, S., Liu, Y. H., Tanaka, N., et al. (2012). Molecular data and ecological niche modelling reveal a highly dynamic evolutionary history of the East Asian Tertiary relict Cercidiphyllum (Cercidiphyllaceae). New Phytol. 196, 617–630. doi: 10.1111/j.1469-8137.2012.04242.x
Qian, H. (1999). Spatial pattern of vascular plant diversity in North America north of Mexico and its floristic relationship with Eurasia. Ann. Bot. 83, 271–283. doi: 10.1006/anbo.1998.0816
Qian, H., and Ricklefs, R. E. (2000). Large-scale processes and the Asian bias in species diversity of temperate plants. Nature 407, 180–182. doi: 10.1038/35025052
Qiu, Y. X., Fu, C. X., and Comes, H. P. (2011). Plant molecular phylogeography in China and adjacent regions: tracing the genetic imprints of Quaternary climate and environmental change in the world's most diverse temperate flora. Mol. Phylogenet. Evol. 59, 225–244. doi: 10.1016/j.ympev.2011.01.012
Qiu, Y. X., Guan, B. C., Fu, C. X., and Comes, H. P. (2009a). Did glacials and/or interglacials promote allopatric incipient speciation in East Asian temperate plants? Phylogeographic and coalescent analyses on refugial isolation and divergence in Versipellis. Mol. Phylogenet. Evol. 51, 281–293. doi: 10.1016/j.ympev.2009.01.016
Qiu, Y. X., Sun, Y., Zhang, X. P., Lee, J., Fu, C. X., and Comes, H. P. (2009b). Molecular phylogeography of East Asian Kirengeshoma (Hydrangeaceae) in relation to Quaternary climate change and landbridge configurations. New Phytol. 183, 480–495. doi: 10.1111/j.1469-8137.2009.02876.x
Rambaut, A. (1996). Se-Al: Sequence Alignment Editor, Version 2.0 a11. Available online at: http://treebioedacuk/software/seal/ (accessed September 19, 2019).
Rambaut, A., Suchard, M., Xie, D., and Drummond, A. (2014). Tracer v1. 6. Available online at: http://beast.bio.ed.ac.uk/Tracer (accessed August 02, 2018).
Raymond, M., and Rousset, F. (1995a). An exact test for population differentiation. Evolution 49, 1280–1283. doi: 10.1111/j.1558-5646.1995.tb04456.x
Raymond, M., and Rousset, F. (1995b). GENEPOP (Version 1.2): Population genetics software for exact tests and ecumenicism. J. Heredity 86, 248–249. doi: 10.1093/oxfordjournals.jhered.a111573
Rice, W. R. (1989). Analyzing tables of statistical tests. Evolution 43, 223–225. doi: 10.1111/j.1558-5646.1989.tb04220.x
Roger, J. (1972). Measure of genetic similarity and genetic distance. Studies in genetics VII. Univ. Texas Pub. 7213, 145–153.
Sakaguchi, S., Horie, K., Ishikawa, N., Nagano, A. J., Yasugi, M., Kudoh, H., et al. (2017). Simultaneous evaluation of the effects of geographic, environmental and temporal isolation in ecotypic populations of Solidago virgaurea. New Phytol. 216, 1268–1280. doi: 10.1111/nph.14744
Sakaguchi, S., Qiu, Y. X., Liu, Y. H., Qi, X. S., Kim, S. H., Han, J., et al. (2012). Climate oscillation during the Quaternary associated with landscape heterogeneity promoted allopatric lineage divergence of a temperate tree Kalopanax septemlobus (Araliaceae) in East Asia. Mol. Ecol. 21, 3823–3838. doi: 10.1111/j.1365-294X.2012.05652.x
Santorum, J. M., Darriba, D., Taboada, G. L., and Posada, D. (2014). jmodeltest.org: selection of nucleotide substitution models on the cloud. Bioinformatics 30, 1310–1311. doi: 10.1093/bioinformatics/btu032
Shin, H. T., Yi, M. H., Shin, J. S., Lee, B. C., and Yoon, J. W. (2012). Distribution of rare plants - Ulsan, Busan, Yangsan. J. Korean Nat. 5, 145–153. doi: 10.7229/jkn.2012.5.2.145
Siddall, M., Rohling, E. J., Almogilabin, A., Ch, H., Meischner, D., Schmelzer, I., et al. (2003). Sea-level fluctuations during the last glacial cycle. Nature 423, 853–858. doi: 10.1038/nature01690
Stamatakis, A. (2014). RAxML version 8: a tool for phylogenetic analysis and post-analysis of large phylogenies. Bioinformatics 30, 1312–1313. doi: 10.1093/bioinformatics/btu033
Stöver, B. C., and Kai, F. M. (2010). TreeGraph 2: combining and visualizing evidence from different phylogenetic analyses. BMC Bio. 11:7. doi: 10.1186/1471-2105-11-7
Swets, J. A. (1988). Measuring the accuracy of diagnostic systems. Science 240, 1285–1293. doi: 10.1126/science.3287615
Tiffney, B. H. (1985a). The Eocene North Atlantic land bridge: its importance in Tertiary and modern phytogeography of the Northern Hemisphere. J. Arnold Arboretum 66, 243–273. doi: 10.5962/bhl.part.13183
Tiffney, B. H. (1985b). Perspectives on the origin of the floristic similarity between eastern Asia and eastern North America. J. Arnold Arboretum 66, 73–94. doi: 10.5962/bhl.part.13179
Tomaru, N., Takahashi, M., Tsumura, Y., Takahashi, M., and Ohba, K. (1998). Intraspecific variation and phylogeographic patterns of Fagus crenata (Fagaceae) mitochondrial DNA. Amer J. Bot. 85, 629–636. doi: 10.2307/2446531
Tsumura, Y. (2006). The phylogeographic structure of Japanese coniferous species as revealed by genetic markers. Taxon 55, 53–66. doi: 10.2307/25065528
Vaidya, G., Lohman, D. J., and Meier, R. (2011). Sequence matrix concatenation software for the fast assembly of multi-gene datasets with character set and codon information. Cladistics 27, 171–180. doi: 10.1111/j.1096-0031.2010.00329.x
Wang, H. F., Landrein, S., Dong, W. P., Nie, Z. L., Kondo, K., Funamoto, T., et al. (2015). Molecular phylogeny and biogeographic diversification of Linnaeoideae (Caprifoliaceae s. l.) disjunctly distributed in Eurasia, North America and Mexico. PLoS ONE 10:e0116485. doi: 10.1371/journal.pone.0116485
Wang, J., Gao, P., Kang, M., Lowe, A. J., and Huang, H. (2009). Refugia within refugia: the case study of a canopy tree Eurycorymbus cavaleriei in subtropical China. J. Biogeogr. 36, 2156–2164.
Wang, P. (1999). Response of Western Pacific marginal seas to glacial cycles: paleoceanographic and sedimentological features. Marine Geol. 156, 5–39. doi: 10.1016/S0025-3227(98)00172-8
Watanabe, S., Hajima, T., Sudo, K., Nagashima, T., Takemura, T., Okajima, H., et al. (2011). MIROC-ESM 2010: model description and basic results of CMIP5-20c3m experiments. Geosci. Model Dev. 4, 845–872. doi: 10.5194/gmd-4-845-2011
Wen, J. (1999). Evolution of eastern Asian and eastern North American disjunct distributions in flowering plants. Ann. Rev. Ecol. Syst. 30, 421–455. doi: 10.1146/annurev.ecolsys.30.1.421
Wen, J., Shi, S. H., Jansen, R., and Zimmer, E. (1998). Phylogeny and biogeography of Aralia sect. Aralia (Araliaceae). Am. J. Bot. 85, 866–875. doi: 10.2307/2446422
Whittaker, R. J., Fernández-Palacios, J. M., Matthews, T. J., Borregaard, M. K., and Triantis, K. A. (2007). Island biogeography: Taking the longview of nature' s laboratories. Science 357:eaam8326. doi: 10.1126/science.aam8326
Wilson, G. A., and Rannala, B. (2003). Bayesian inference of recent migration rates using multilocus genotypes. Genetics 163, 1177–1191. doi: 10.1093/eurpub/13.1.11
Wolfe, J. A. (1971). Tertiary climatic fluctuations and methods of analysis of Tertiary floras. Palaeogeogr. Palaeoclimatol. Palaeoecol. 9, 27–57. doi: 10.1016/0031-0182(71)90016-2
Yang, Q., Landrein, S., Osborne, J., and Borosova, R. (2011). “Caprifoliaceae,” in Flora of China, Vol. 19 (Cucurbitaceae through Valerianaceae, with Annonaceae and Berberidaceae), eds Z. Y. Wu, P. H. Raven, and D. Y. Hong (Beijing; St. Louis, MO: Science Press; Missouri Botanical Garden Press, 616–641.
Zhai, S. -N., Hans, P. C., Koh, N., Hai-Fei, Y., and Ying-Xiong, Q. (2012). Late pleistocene lineage divergence among populations of Neolitseasericea (Lauraceae) across a deep sea-barrier in the Ryukyu Islands. J. Biogeogr. 39, 1347–1360.
Zhai, S. N., and Silman, M. R. (2012). Late Pleistocene lineage divergence among populations of Neolitsea sericea (Lauraceae) across a deep sea-barrier in the Ryukyu Islands. J. Bio. 39, 1347–1360. doi: 10.1111/j.1365-2699.2012.02685.x
Zhao, K. K., Wang, H. F., Sakaguchi, S., Landrein, S., Isagi, Y., Maki, M., et al. (2017). Development and characterization of EST-SSR markers in an East Asian temperate plant genus Diabelia (Caprifoliaceae). Plant Spec. Biol. 32, 247–251. doi: 10.1111/1442-1984.12143
Keywords: Diabelia, Caprifoliaceae, phylogeography, ecological niche modeling, Sino-Japanese disjunct distribution
Citation: Zhao K-K, Landrein S, Barrett RL, Sakaguchi S, Maki M, Mu W-X, Yang T, Zhu Z-X, Liu H and Wang H-F (2019) Phylogeographic Analysis and Genetic Structure of an Endemic Sino-Japanese Disjunctive Genus Diabelia (Caprifoliaceae). Front. Plant Sci. 10:913. doi: 10.3389/fpls.2019.00913
Received: 10 April 2019; Accepted: 27 June 2019;
Published: 16 July 2019.
Edited by:
Stefan Wanke, Dresden University of Technology, GermanyReviewed by:
Jun Ying Lim, University of Amsterdam, NetherlandsCopyright © 2019 Zhao, Landrein, Barrett, Sakaguchi, Maki, Mu, Yang, Zhu, Liu and Wang. This is an open-access article distributed under the terms of the Creative Commons Attribution License (CC BY). The use, distribution or reproduction in other forums is permitted, provided the original author(s) and the copyright owner(s) are credited and that the original publication in this journal is cited, in accordance with accepted academic practice. No use, distribution or reproduction is permitted which does not comply with these terms.
*Correspondence: Hua-Feng Wang, d2FuZ2h1YWZlbmcyMDEyQGZveG1haWwuY29t; Huan Liu, bGl1aHVhbkBnZW5vbWljcy5jbg==
Disclaimer: All claims expressed in this article are solely those of the authors and do not necessarily represent those of their affiliated organizations, or those of the publisher, the editors and the reviewers. Any product that may be evaluated in this article or claim that may be made by its manufacturer is not guaranteed or endorsed by the publisher.
Research integrity at Frontiers
Learn more about the work of our research integrity team to safeguard the quality of each article we publish.