- 1Natural History Museum of Denmark, Faculty of Science, University of Copenhagen, Copenhagen, Denmark
- 2Collaborative Mass Spectrometry Innovation Center, Skaggs School of Pharmacy and Pharmaceutical Sciences, University of California, San Diego, La Jolla, CA, United States
- 3Skaggs School of Pharmacy and Pharmaceutical Sciences, University of California, San Diego, La Jolla, CA, United States
- 4Bioinformatics Group, Department of Plant Sciences, Wageningen University & Research, Wageningen, Netherlands
- 5Comparative Plant and Fungal Biology, Royal Botanic Gardens, Richmond, United Kingdom
- 6Department of Botany, Federal University of Santa Catarina, Florianópolis, Brazil
- 7Department of Biotechnology and Biomedicine, Technical University of Denmark, Lyngby, Denmark
- 8Department of Drug Design and Pharmacology, Faculty of Health and Medical Sciences, University of Copenhagen, Copenhagen, Denmark
- 9Front End Innovation, LEO Pharma A/S, Ballerup, Denmark
- 10Center for Microbiome Innovation, University of California, San Diego, La Jolla, CA, United States
Coevolutionary theory suggests that an arms race between plants and herbivores yields increased plant specialized metabolite diversity and the geographic mosaic theory of coevolution predicts that coevolutionary interactions vary across geographic scales. Consequently, plant specialized metabolite diversity is expected to be highest in coevolutionary hotspots, geographic regions, which exhibit strong reciprocal selection on the interacting species. Despite being well-established theoretical frameworks, technical limitations have precluded rigorous hypothesis testing. Here we aim at understanding how geographic separation over evolutionary time may have impacted chemical differentiation in the cosmopolitan plant genus Euphorbia. We use a combination of state-of-the-art computational mass spectral metabolomics tools together with cell-based high-throughput immunomodulatory testing. Our results show significant differences in specialized metabolite diversity across geographically separated phylogenetic clades. Chemical structural diversity of the highly toxic Euphorbia diterpenoids is significantly reduced in species native to the Americas, compared to Afro-Eurasia. The localization of these compounds to young stems and roots suggest a possible ecological relevance in herbivory defense. This is further supported by reduced immunomodulatory activity in the American subclade as well as herbivore distribution patterns. We conclude that computational mass spectrometric metabolomics coupled with relevant ecological data provide a strong tool for exploring plant specialized metabolite diversity in a chemo-evolutionary framework.
Introduction
Likely as a consequence of niche colonization and adaptive evolution, plants produce an extraordinary variety of molecules. These so-called specialized metabolites are deployed in the response to a multitude of biotic as well as abiotic factors (Bednarek and Osbourn, 2009), for example, to attract pollinators or seed-dispersing animals, in signaling between other plants and symbiotic microorganisms or to combat herbivores or physical stresses such as UV light (Bednarek and Osbourn, 2009; Wink, 2010).
Coevolutionary theory suggests that an arms race between plants and herbivores is the major driving force yielding increased specialized metabolite diversity in plants (Ehrlich and Raven, 1964; Becerra, 2007; Richards et al., 2015). The evolution of a chemically different and biologically more active molecule increases a plant’s fitness by reducing the fitness of its herbivores (Ehrlich and Raven, 1964; Firn and Jones, 2003), and the probability of producing one or more biologically active compounds may increase with plant specialized metabolite diversity (Jones and Firn, 1991). Previous studies have assessed plant specialized metabolite diversity in relation to herbivory and, in agreement with the coevolutionary theory, found a positive relationship between plant chemical diversity, the degree of insect herbivore trophic specialization and diversity (Becerra, 2007; Richards et al., 2015). Furthermore, the geographic mosaic theory of coevolution predicts that coevolutionary interactions vary across geographic scales. Patterns of reciprocal response in coevolving species differ across populations. This variation may be caused by fluctuating biotic and abiotic factors across landscapes, causing species to coevolve in some populations but not in others (Thompson, 2005a,b). For example, detoxification profiles of the parsnip webworm Depressaria pastinacella (Duponchel, 1838) and plant defense profiles of its host, the wild parsnip (Pastinaca sativa L.) were significantly mismatched in the presence of an alternate host plant, the cow parsnip (Heracleum lanatum Michx.). This suggests that the presence of a chemically different alternate host can affect selection intensity and patterns of reciprocal response across landscapes (Zangerl and Berenbaum, 2003). Traits, which are shaped by coevolving interactions become only fixed in a species when strong positive natural selection is present across all populations (Thompson, 2005b). Alternatively, particularly in widespread species, an inevitable consequence of the geographic mosaic of coevolution is the formation of highly divergent populations that have the potential to form new species (Thompson, 2008).
If we assume that the main driving force of plant specialized metabolite diversity is a coevolutionary arms race, and coevolutionary interaction is a rare event and constrained by a geographic mosaic of species interactions, we would expect significant differences in specialized metabolite diversity in plant phylogenetic clades, which have evolved in geographically very distant regions. This would be because we expect geographically very distant regions to vary most in biotic as well as abiotic factors influencing coevolutionary species interaction. While a strong reciprocal selection on the interacting plant–herbivore species might promote plant chemical diversity in one region, the same combination of biotic factors is not likely to be found in another significantly distant geographic region. For example, this could be due to the absence of one of the interacting species.
Previous studies have assessed the role of plant–herbivore interactions in the evolution of plant specialized metabolite diversity (Becerra, 2007; Richards et al., 2015); however, they have not explicitly taken geography into account. Here, we investigate the role of biogeography in the evolution of specialized metabolite diversity in 43 Euphorbia species, representing the genus’ global genetic diversity and biogeographic history across all continents. To do so, we acquired untargeted mass spectrometry data including mass fragmentation (MS/MS) data to assess the specialized metabolome. To annotate and compare the specialized metabolite content across all 43 species, we use a combination of mass spectral molecular networking, in silico annotation tools, substructure recognition, and automated chemical classification leading to the semi-automated annotation of over 1800 specialized metabolites, up to the level of chemical structures, substructures, or subclasses. Furthermore, we assess the possible ecological relevance of plant specialized metabolite diversity through cell-based high-resolution immunomodulatory bioactivity profiling, 3D mass spectral molecular cartography, and herbivore distribution patterns.
Euphorbia is among the most diverse and species-rich plant genera on Earth, exhibiting a near-cosmopolitan distribution and extraordinary chemical diversity among 2,000 species (Horn et al., 2012, 2014; Vasas and Hohmann, 2014). Most recent phylogenetic studies have supported four geographically strongly separated subgeneric clades (Horn et al., 2012). The genus is estimated to have originated in Africa approximately 48 million years ago and expanded to the American continents through two single long-distance dispersal events 30 and 25 million years ago (Figures 1, 2; Zimmermann et al., 2010; Yang and Berry, 2011; Horn et al., 2012, 2014; Yang et al., 2012; Dorsey et al., 2013; Peirson et al., 2013; Riina et al., 2013). The subgenera Athymalus (Neck.) Rchb. and Esula Pers. exhibit a primarily Afro-Eurasian radiation, subg. Euphorbia is diverse in its distribution range and subg. Chamaesyce Raf. comprises the majority of the American radiation of the genus (Horn et al., 2012). The genus’ chemical diversity is characterized by an extraordinary diversity of macro- and polycyclic diterpenoids, biosynthetically derived from a head-to-tail cyclization of the tetraprenyl pyrophosphate precursor (Shi et al., 2008; Vasas and Hohmann, 2014; Appendino, 2016). These compounds are known to play an important ecological role as feeding deterrents and have shown exclusive occurrence and chemotaxonomic relevance in the plant families Euphorbiaceae and Thymelaeaceae (Batra, 1983; Hundsdoerfer et al., 2005a; Huang et al., 2014; Vasas and Hohmann, 2014; Hua et al., 2017). In vitro Euphorbia diterpenoids are known to induce different immunomodulatory responses through the selective modulation of protein kinase C (PKC) including the release of prostaglandins and pro-inflammatory cytokines such as TNF-α, interleukin-6, and interleukin-1β (Smith and Meldrum, 1992; Berman, 2012; Uto et al., 2012; Jian et al., 2018; Zhang et al., 2019).
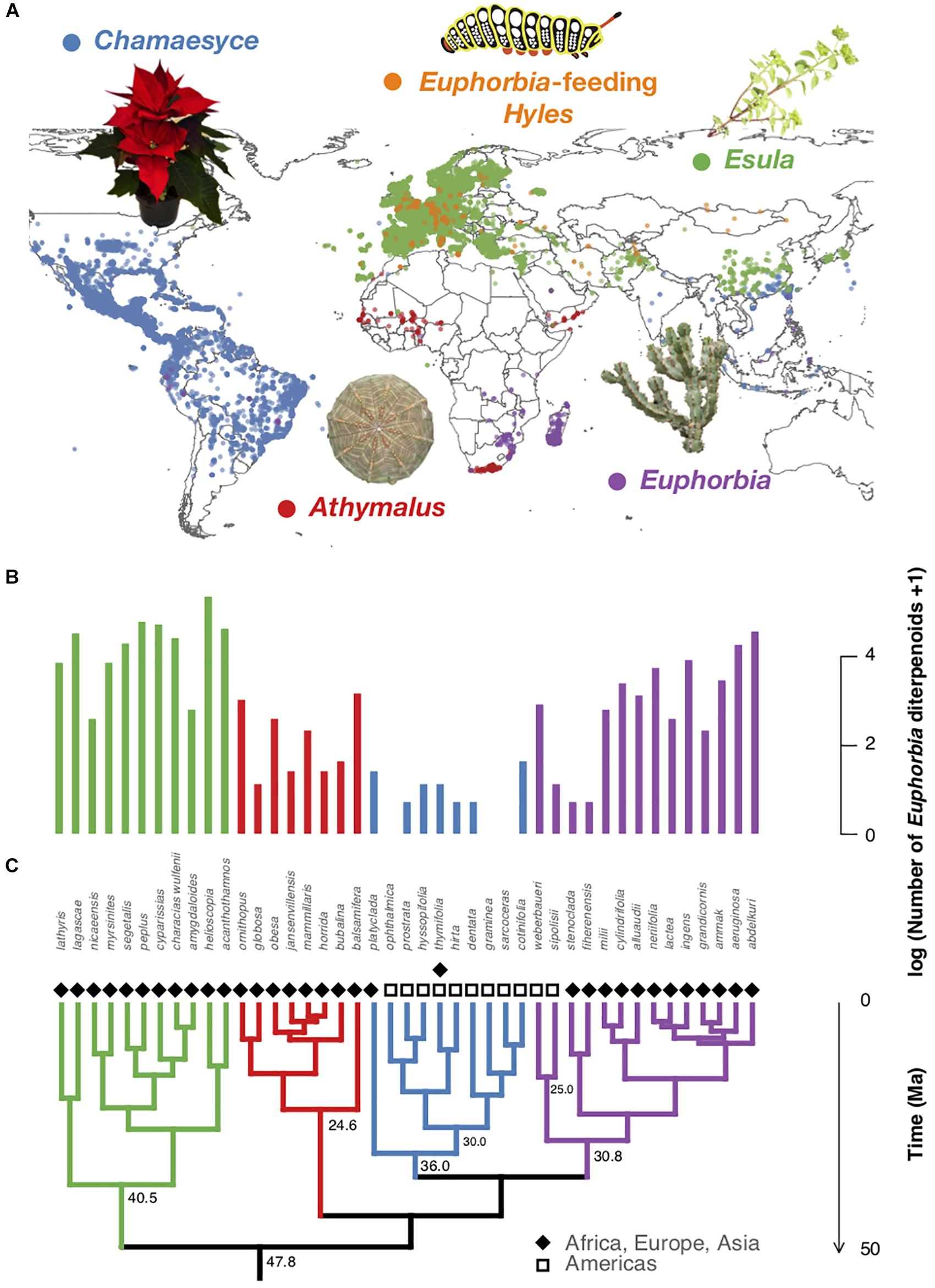
Figure 1. Biogeography, phylogenetic relationships, and diterpenoid production of representative Euphorbia species. (A) Occurrences of Euphorbia species investigated chemically and Euphorbia-feeding Hyles moth larvae retrieved from GBIF and manually restricted to native areas. (B) Number of putatively annotated Euphorbia diterpenoids per species analyzed. (C) Euphorbia phylogenetic tree (50% majority rule consensus tree from Bayesian analysis of 11,587 bp of DNA markers spanning all three plant genomes: chloroplast, mitochondrial, nuclear). Species of subg. Esula exhibit a high number of diterpenoids and co-occur with larvae of Euphorbia-feeding Hyles, whereas the American radiation of subg. Chamaesyce shows reduced Euphorbia diterpenoid production. Subgeneric clades are highlighted with different colors: Athymalus (red), Chamaesyce (blue), Esula (green), and Euphorbia (purple). Photos: Mogens Trolle and Madeleine Ernst.
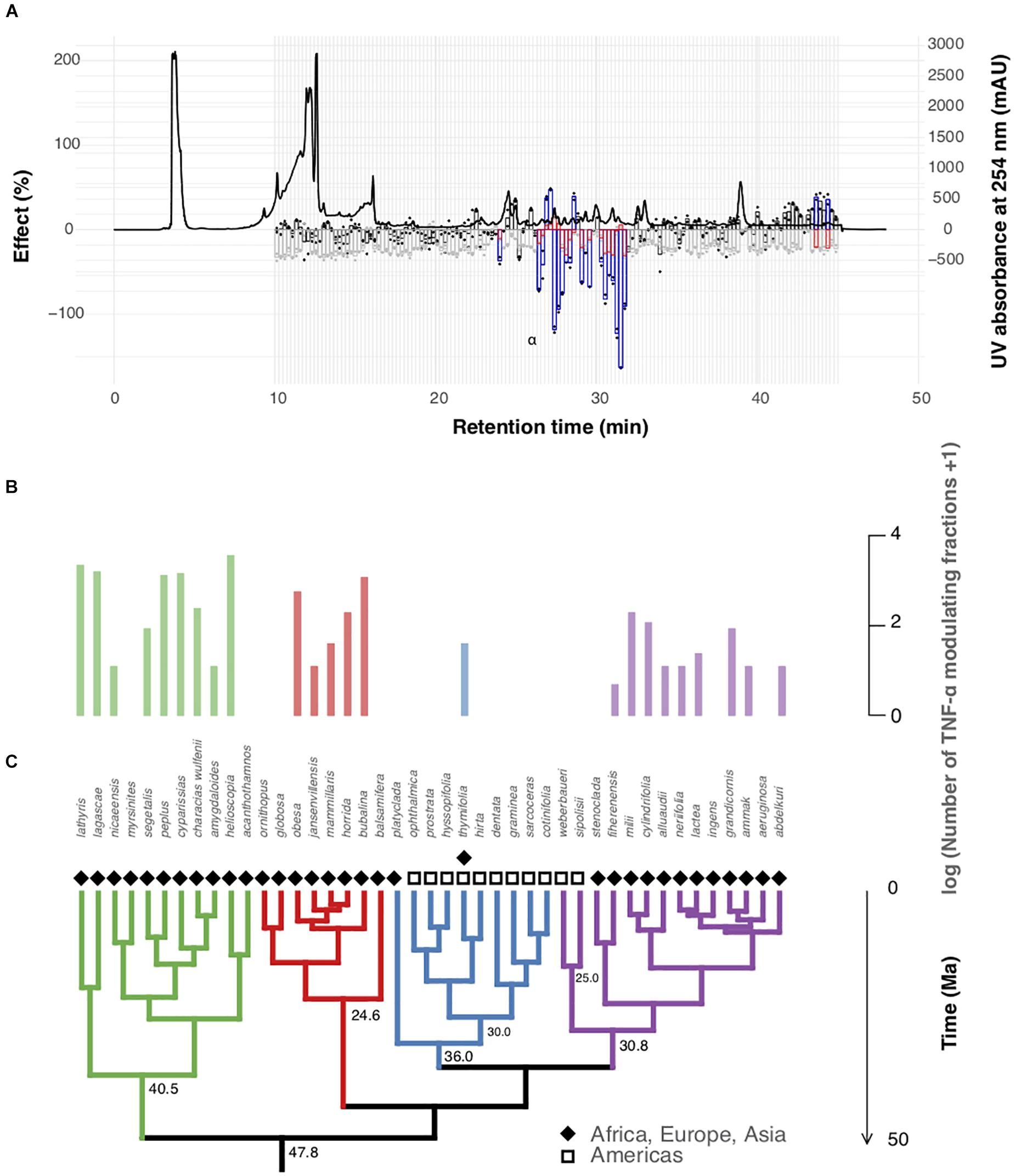
Figure 2. Pro-inflammatory potential of representative Euphorbia species. (A) TNF-α modulating properties of fractions of the crude extract of Euphorbia peplus. The effect of the fractionated extract on TNF-α modulation (black/blue) and cell viability (gray/red) is plotted against the HPLC chromatogram at 254 nm. Fractions with significant modulation of TNF-α are highlighted in blue, whereas fractions significantly modulating cell viability are highlighted in red. 100% effect corresponds to 100% inhibition of TNF-α release and cell viability, respectively. (B) Number of fractions significantly modulating TNF-α without having a significant effect on cell viability per species analyzed. (C) Euphorbia phylogenetic tree (50% majority rule consensus tree from Bayesian analysis of 11,587 bp of DNA markers spanning all three plant genomes: chloroplast, mitochondrial, nuclear). Species of subg. Esula exhibit a high number of TNF-α modulating fractions and co-occur with larvae of Euphorbia-feeding Hyles (Figure 1), whereas the American radiation of subg. Chamaesyce shows reduced TNF-α modulating activity. Subgeneric clades are highlighted with different colors: Athymalus (red), Chamaesyce (blue), Esula (green), and Euphorbia (purple).
Following coevolutionary and geographic mosaic theory, we hypothesize that (1) there are significant differences in specialized metabolite diversity across Euphorbia phylogenetic clades, which is (2) correlated with geographical separation through evolutionary time with highest differences observed between phylogenetic clades having evolved in the American versus the Afro-Eurasian continents, where genetic interchange is least likely, and (3) specialized metabolite diversity in Euphorbia may reflect a role in herbivory defense, as predicted by herbivore–plant coevolutionary hotspots.
Materials and Methods
Collection of Plant Material
Forty-three Euphorbia species (Supplementary Data S1) were collected from the greenhouses of the Living Collections of the Botanical Garden, Natural History Museum of Denmark, University of Copenhagen. Live plants were sampled for xerophytic species, whereas herbaceous perennials were grown from seeds originating from the seed bank of the Botanical Garden or collections performed in southern Brazil (species 11–14 and 16–18). Euphorbia myrsinites L. and E. amygdaloides L. were purchased as live plants from Jespers Planteskole, Holstebro A/S, Denmark and Kridtvejs Planter, Denmark, respectively, and kept in the greenhouse of the Botanical Garden with the other herbaceous species until harvest. To obtain representative samples of the specialized metabolite profile in the living plants, the plants were sampled as whole intact specimens with the roots included. Over six specimens or as many as available from the collections were sampled for each species (Supplementary Data S1). Some tree species or rare species could not be sampled as whole plants, in these cases, branches of representative parts of the living specimen were sampled (Supplementary Data S1). The fresh plant material was then stored at −20°C until extract preparation. Voucher specimens were prepared for species with enough material available from the Living Collections and deposited at Herbarium C, Natural History Museum of Denmark, Copenhagen. Taxonomic species identity was confirmed for all species sampled based on the voucher specimens, the living specimens in the greenhouse or photographs.
Collection of Plant Material for 3D Mass Spectral Molecular Cartography
Individual plant parts from two to three specimens of one representative species of each subgeneric clade of Euphorbia were sampled (E. horrida Boiss., subg. Athymalus, three specimens; E. hirta L., subg. Chamaesyce, two specimens; E. lathyris L., subg. Esula, two specimens; E. milii Des Moul., subg. Euphorbia, two specimens). Approximately 200 mg fresh plant material of each individual plant part was collected in 1.5 ml Eppendorf tubes and flash frozen under liquid nitrogen. The samples were stored at −80°C until further analysis.
Extract Preparation
Individual specimens of the same species were pooled, and the frozen plant material was disrupted with a pestle and mortar in liquid nitrogen and approximately 75 g was extracted with 975 ml ethyl acetate (VWR Chemicals, HiPerSolv Chromanorm) under sonication (Fisher Scientific) at 40°C during 2 h. The extracts were filtered and evaporated to dryness on a rotary evaporator. The dried extracts were then resuspended in acetonitrile (VWR Chemicals, HiPerSolv Chromanorm), extracted under sonication (Fisher Scientific) at 40°C during 15 min, filtered, and evaporated to dryness. Description of the extract preparation for 3D mass spectral molecular cartography is provided in the Supplementary Methods.
LC–MS/MS Analysis
Extracts were transferred to a 96-well plate (Falcon, 96-well plates, 0.34 ml, polypropylene) and dried with a vacuum centrifuge. Samples were redissolved in 3/7 vol/vol methanol (Fisher Scientific, HPLC Grade)/acetonitrile (Fisher Scientific, Optima LC/MS) to a concentration of 10 mg/ml, in a volume of 150 μl, with a 100 mM concentration of ammonium formate, sealed with Zone-Free Sealing Film (Excel Scientific), and centrifuged for 30 min at 2,000 r.p.m. at 4°C. Ammonium formate was used to promote the ionization of diterpene esters as ammonium adducts rather than sodiated adducts, thus inducing a richer MS/MS fragmentation pattern as described by Vogg et al. (1999). MS analysis was performed on a qTOF Maxis II (Bruker Daltonics) mass spectrometer with an electrospray ionization (ESI) source, controlled by OTOF control and Hystar. Additional descriptions of the liquid chromatography tandem mass spectrometry (LC–MS/MS) analysis as well as a description of the LC–MS/MS analysis for 3D mass spectral molecular cartography is provided in the Supplementary Methods.
High-Resolution TNF-α Modulation Profiling
Euphorbia diterpenoids are known to induce different immunomodulatory responses in vitro through the selective modulation of PKC including the release of prostaglandins and pro-inflammatory cytokines such as TNF-α, interleukin-6, and interleukin-1β (Smith and Meldrum, 1992; Berman, 2012; Uto et al., 2012; Jian et al., 2018; Zhang et al., 2019). Both vertebrates and invertebrates produce cytokines, and it is likely that they exhibit similar functions both in insect as well as mammalian cells (Adamo, 2008). To assess the immunomodulatory capacity of compounds or compound groups in Euphorbia, we chose to measure in vitro TNF-α release from peripheral blood mononuclear cells (PBMCs). TNF-α was chosen above other cytokines because of its key role in initiating local inflammatory responses (Monaco et al., 2014; Sedger and McDermott, 2014; Kalliolias and Ivashkiv, 2016). First, we subjected the same 43 plant extracts we had previously characterized chemically to high-resolution microplate-based extract collection, resulting in 144 individual fractions per extract. Then we used all the individual fractions to treat human peripheral blood mononuclear cells (hPBMCs), which are activated to release TNF-α by stimulation with anti-CD3- and anti-CD28-coated beads. Finally, we assessed the modulation of TNF-α by evaluating the level of TNF-α released in culturing media in the HPLC trace (Wubshet et al., 2013; Supplementary Data S2 and Supplementary Figures S2–S45). Detailed description of the high-resolution microplate-based extract collection as well as the TNF-α inhibition assay is provided in the Supplementary Methods.
Mass Spectral Molecular Networking
Liquid chromatography tandem mass spectrometry data of the pooled extracts were converted to mzML data, and lock mass correction was performed using Compass Data Analysis (Bruker Daltonics). MassFuser merged mzML files (for a detailed description, see Supplementary Methods) were then processed using Optimus v1.1.0, a processing workflow for LC–MS/MS untargeted metabolomics based on OpenMS algorithms (Röst et al., 2016; Protsyuk et al., 2018)1 with parameters set to: MS polarity mode positive, m/z tolerance 30 ppm, noise threshold 20,000, half of MS/MS isolation window: 0.02 Da, RT tolerance of MS/MS acquisition 5 s, RT tolerance 20 s, enable re-integration of missing features off, enable pose clustering alignment off, intensity factor compared to blanks 3, minimal occurrence number 1, presence of MS/MS on, variation in pooled QC runs and replicates off, enable feature normalization off, save as MGF, MS1 noise threshold 250, and MS2 noise threshold 150. Features detected in blank samples were discarded from the samples using the Optimus Workflow. Subsequently, the ms2.mgf output file was uploaded to the Global Natural Products Social (GNPS) Molecular Networking web server2 and submitted to network analysis using the following settings: Precursor ion mass tolerance 0.02 Da, fragment ion mass tolerance 0.02 Da, min pairs Cos 0.5, min matched fragment ions 6, Network TopK 10, minimum cluster size 1, maximum connected component size 200, and Run MS Cluster off. The molecular networks were visualized with Cytoscape version 3.4.0 (Shannon et al., 2003). The data are publicly accessible at http://gnps.ucsd.edu under the MassIVE accession no. MSV000081082 and network exploration options and views are available and networking parameters described at: https://gnps.ucsd.edu/ProteoSAFe/status.jsp?task=26326c233918419f8dc80e8af984cdae. Description of the mass spectral molecular networking analysis for 3D mass spectral molecular cartography is provided in the Supplementary Methods.
Integration of in silico Annotation, Automated Chemical Classification, and Substructure Recognition With Mass Spectral Molecular Networks
In silico structure annotation was performed by submitting the preprocessed ms1-2.mgf output file of the pooled extracts from Optimus to Sirius+CSI:FingerID (Dührkop et al., 2015; Böcker and Dührkop, 2016) with m/z tolerance set to 20 ppm. Additionally, data were submitted to Network Annotation Propagation (NAP) (da Silva et al., 2018). For NAP, both [M+NH4]+ and [M+H]+ adducts were searched with m/z tolerance set to 15 ppm and parameters described at: https://proteomics.ucsd.edu/ProteoSAFe/status.jsp?task=184a80db74334668ae1d0c0f852cb77c and https://proteomics2.ucsd.edu/ProteoSAFe/status.jsp?task=2cfddd3b8b1e469181a13e7d3a867a6f.
We matched a custom database of molecular structures against our samples’ preprocessed mass spectral data using both Sirius+CSI:FingerID and NAP. This database was compiled manually from literature (Shi et al., 2008; Vasas and Hohmann, 2014) and the dictionary of natural products (DNP)3. Subsequently, in silico structure matches from Sirius+CSI:FingerID and NAP were submitted to automated chemical classification using ClassyFire4 (Djoumbou Feunang et al., 2016) and consensus classifications at each hierarchical level of the chemical taxonomy per mass spectral molecular subnetwork were calculated. Consensus classifications and molecular structures were then visualized on the molecular networks using Cytoscape version 3.4.0 (Shannon et al., 2003). Substructure recognition of the crude extracts was performed by submitting the preprocessed ms2.mgf output file from Optimus to MS2LDA (van der Hooft et al., 2016; Wandy et al., 2018). Data and parameters used are publically accessible at http://ms2lda.org/basicviz/short_summary/390/. Subsequently substructures (Mass2Motifs) were mapped on the nodes of the mass spectral molecular network, and Mass2Motifs shared among different nodes were mapped on the edges connecting the nodes and visualized using Cytoscape version 3.4.0. Description of the integration of in silico annotation, automated chemical classification, and substructure recognition with mass spectral molecular networks for 3D mass spectral molecular cartography is provided in the Supplementary Methods.
3D Modeling and Visualization
We converted approximately 250 photos taken from one to three specimens of one representative species of each subgeneric clade of Euphorbia into high-definition 3D meshes using Autodesk Remake5. The 3D meshes were then exported to .obj format and edited using Meshmixer6. Point coordinates for sampled plant parts were added using Meshlab (Cignoni et al., 2008), and the 3D Models were exported to .stl format. Representative samples were taken of individual plant parts (e.g., roots, upper and lower leaves and stems, fruits), rather than exact sample locations (e.g., three to five representative pieces of one inflorescence were taken and pooled together for LC–MS/MS analysis, the entire inflorescence was subsequently mapped with the pooled LC–MS data). The 3D mass spectral mapping thus shows data obtained from representative pools of individual plant parts, rather than being accurate point representatives of plant parts sampled. Point coordinates and LC–MS data were combined into a .csv file and were then mapped on the 3D Models using ’ili7 (Bouslimani et al., 2015; Floros et al., 2017). URL links of cartographical snapshots, which can be opened in a web browser can be found in Supplementary URL S1, Links 1–15. Each URL will open the ’ili web application, and load data, settings, and visualizations as shown in Figure 5 and Supplementary Figures S3–S9. Additionally, the visualization is interactive, and the models can be rotated, visualization parameters changed, and other molecules selected by their retention time and m/z value (Protsyuk et al., 2018).
Calculation of the Chemical Structural Compositional Similarity and Chemogram
To assess specialized metabolite diversity in relation to the evolutionary history of Euphorbia, we calculated the chemical structural and compositional similarity (CSCS) per Euphorbia subgeneric clade (Sedio et al., 2017). CSCS provides a more accurate estimate of phytochemical diversity compared to traditional dissimilarity measures (e.g., Bray-Curtis) by integrating the cosine scores retrieved from the mass spectral molecular network analysis and thus also accounting for chemical structural similarity among specialized metabolites detected by mass spectrometry (Sedio, 2017; Sedio et al., 2017). To illustrate chemical similarity among species we created a chemogram by using the pairwise chemical structural and compositional dissimilarities (CSCDs), corresponding to 1-CSCS, as input for a hierarchical cluster analysis with the complete agglomeration method.
Phylogenetic Hypothesis and Comparative Methods
We produced a phylogenetic hypothesis of Euphorbia compiling DNA sequences from 10 markers spanning all three plant genomes (cp accD, cp rbcL-accD, cp ndhF, cp rbcL, cp rpl16, cp trnL-F, mt nad1B-C, mt rps3, nu EMB2765, nu ITS) of a publicly available dataset (Horn et al., 2012, 2014). Our matrix included sequences (11,587 base pairs) of 38 out of the 43 Euphorbia species investigated chemically. We produced a Bayesian phylogenetic hypothesis using parameters described in Horn et al. (2014). Subsequently, we added missing species (E. sarcoceras O.L.M. Silva and Cordeiro, E. hyssopifolia L., E. ophthalmica Pers., E. prostrata Aiton, E. thymifolia L.) based on their subgeneric classification (Yang et al., 2012; da Silva and Cordeiro, 2016) using the bind.tip function of the R package phytools (Revell, 2012) to the 50% majority rule consensus tree. We performed a phylogenetic generalized least squares (PGLS) regression analysis using the pgls function of the R package caper (Orme et al., 2013) to test whether the number of putatively annotated molecules within a chemical subclass and the number of TNF-α modulating fractions are significantly associated over evolutionary time. PGLS is a modification of the generalized least squares regression, taking into account non-independence of species sharing an evolutionary history by weighing the generalized least squares regression by the amount of expected correlation between species based on their phylogenetic relationships (Symonds and Blomberg, 2014). We only assessed chemical subclasses containing at least five molecules and non-zero values for at least 20 species out of the 43 species within the phylogenetic tree. Supplementary Table S1 shows chemical subclasses and associated p-values. Figure 1 and Supplementary Figure S12 show the number of TNF-α modulating fractions versus the number of putatively annotated molecules within the chemical subclasses with significant p-values (Euphorbia diterpenoids, all diterpenoids, glycosylglycerols) across the phylogenetic tree. The best fit was observed for the Euphorbia diterpenoids (Figure 1), with species of the American clade within subg. Chamaesyce being almost entirely deprived of these compounds, corresponding to the TNF-α modulating properties of these species.
Species Occurrences of Sampled Euphorbia and Euphorbia-Feeding Hyles
We downloaded species occurrences of sampled Euphorbia species and Euphorbia-feeding Hyles from the Global Biodiversity Information Facility (GBIF8). Before mapping species occurrences, we reduced the datasets to the native distributions of the respective species (Meerman, 1993; Hundsdoerfer et al., 2005b; Govaerts et al., 2014). Species names of Euphorbia-feeding Hyles were retrieved from Hundsdoerfer et al. (2009). URL links to GBIF species occurrence downloads are found below:
Species of subg. Euphorbia: GBIF.org (5 January 2018) GBIF Occurrence Download https://doi.org/10.15468/dl.llmwkb.
Species of subg. Esula: GBIF.org (5 January 2018) GBIF Occurrence Download https://doi.org/10.15468/dl.avy89i.
Species of subg. Chamaesyce: GBIF.org (5 January 2018) GBIF Occurrence Download https://doi.org/10.15468/dl.9ntc6n.
Species of subg. Athymalus: GBIF.org (5 January 2018) GBIF Occurrence Download https://doi.org/10.15468/dl.ug6cnm.
Hyles euphorbiae: GBIF.org (5 January 2018) GBIF Occurrence Download https://doi.org/10.15468/dl.cgwykr.
Other Euphorbia-feeding Hyles species: GBIF.org (24 January 2018) GBIF Occurrence Download https://doi.org/10.15468/dl.ahwr3w.
Code and Data Availability
All scripts used for data analysis were written in R version 3.3.29. Data, code, and material required to understand and assess the conclusions of this research are publicly available at https://github.com/DorresteinLaboratory/supplementary-GlobalEuphorbiaStudy. LC–MS/MS data are publicly accessible on GNPS under the MassIVE accession nos. MSV000081082 and MSV000081083. Bayesian trees used for analysis were deposited on dryad10.
Results
To assess specialized metabolite diversity in relation to the evolutionary and biogeographic history of Euphorbia, we subjected extracts of 43 Euphorbia species to LC–MS/MS, created mass spectral molecular networks through GNPS Molecular Networking (Watrous et al., 2012; Wang et al., 2016), and calculated the CSCS for all Euphorbia subgeneric clades (Sedio et al., 2017). Our data show significantly higher chemical similarity among species of the predominantly American subg. Chamaesyce compared to the mean chemical similarity among species of the remaining subgeneric clades mostly native to Afro-Eurasia (Figure 3). The only species clustered in the chemogram (sharing high chemical similarity) are eight out of nine species representing the American radiation within subg. Chamaesyce (Figure 3D).
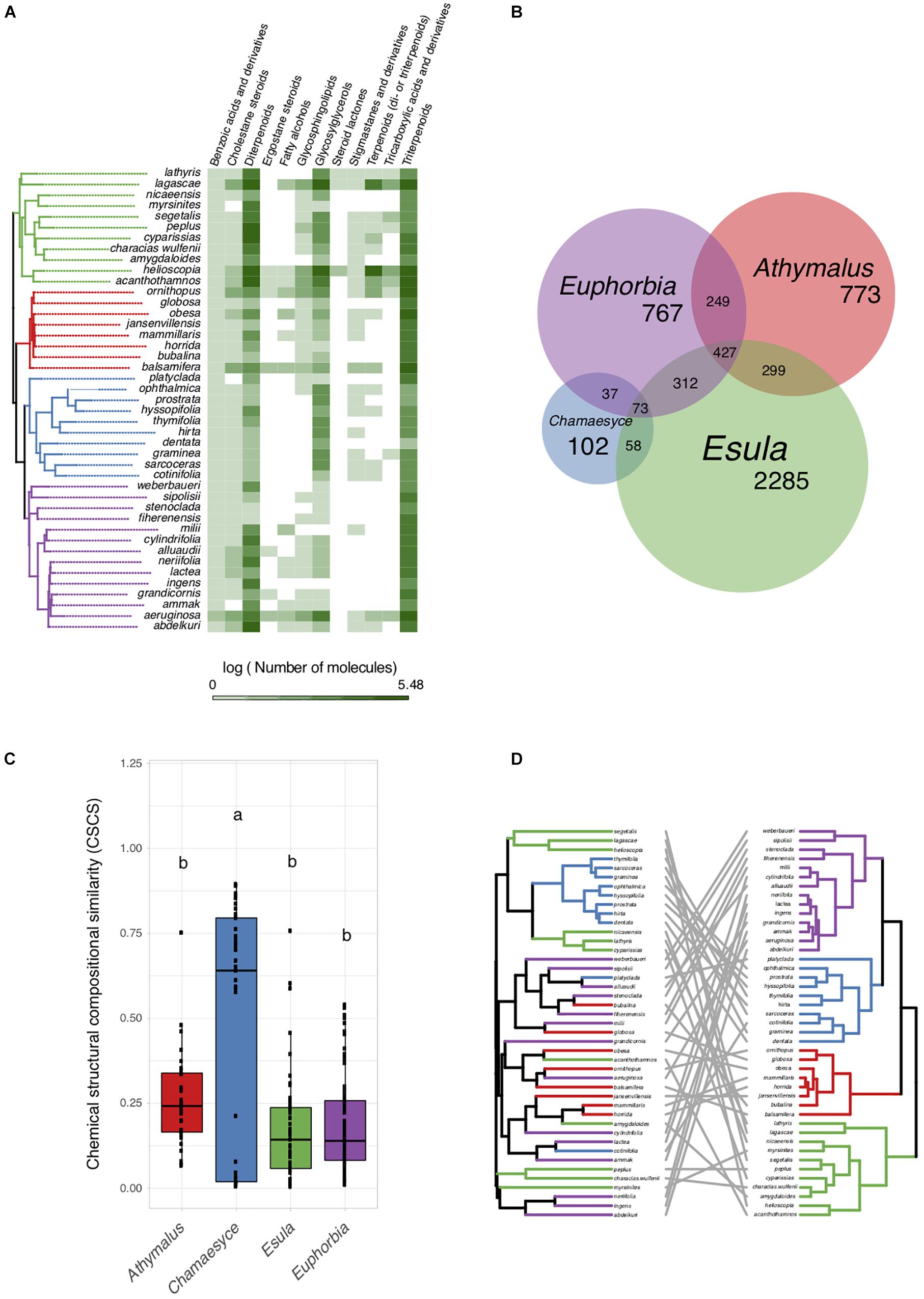
Figure 3. Specialized metabolite diversity in Euphorbia. (A) Distribution of specialized metabolite classes on the Euphorbia phylogenetic tree (50% majority rule consensus tree from Bayesian analysis of 11,587 bp of DNA markers spanning all three plant genomes: chloroplast, mitochondrial, nuclear). Chemical classes of Euphorbia specialized metabolites were putatively identified using a mass spectrometry based workflow combining mass spectral molecular networking, in silico annotation, automated chemical classification, and substructure recognition. (B) Molecular features representing individual mass spectral molecular network nodes shared across species of Euphorbia subgeneric clades. (C) Chemical similarity among Euphorbia subgeneric clades assessed using the chemical structural compositional similarity. Compared to subgenera Athymalus, Esula, and Euphorbia, subg. Chamaesyce exhibits very few chemically distinct features and high chemical structural compositional similarity. (D) Euphorbia chemogram (left) and phylogenetic tree (right). The chemogram was generated using hierarchical cluster analysis on the pair-wise chemical structural and compositional dissimilarities of the tandem mass spectrometry data of the crude extracts using the complete agglomeration method. Phylogeny and chemogram show low overlap, suggesting that closely related Euphorbia species differ considerably in their chemistry.
To further understand the chemo-evolutionary relationships of Euphorbia at a molecular level we putatively identified major specialized metabolite classes by combining mass spectral molecular networking with in silico annotation tools (Dührkop et al., 2015; Böcker and Dührkop, 2016; da Silva et al., 2018), substructure recognition (van der Hooft et al., 2016; Wandy et al., 2018), and automated chemical classification through ClassyFire (Djoumbou Feunang et al., 2016). This allowed us to putatively identify chemical classes within the mass spectral molecular networks, as well as chemical subclasses within molecular families (Figure 4 and Supplementary Figure S2). Automated chemical classification revealed annotated compound classes for over 30% of the compounds detected, corresponding to a level 3 metabolite identification according to the Metabolomics Standard Initiative’s reporting standards (Sumner et al., 2007; Supplementary Figures S1, S2 and Supplementary Notes S1). The genus Euphorbia is known for producing an extraordinary diversity of macro- and polycyclic diterpenoids, biosynthetically derived from a head-to-tail cyclization of the tetraprenyl pyrophosphate precursor (Shi et al., 2008; Vasas and Hohmann, 2014). To distinguish these diterpenoids from diterpenoids biosynthetically derived from a cascade cyclization, we here refer to them as Euphorbia diterpenoids. Many known Euphorbia diterpenoids as well as other metabolite classes were revealed. Out of six major structural classes of Euphorbia specialized metabolites (sesquiterpenoids, diterpenoids, cerebrosides, phenolics, flavonoids, and triterpenoids including steroids) (Shi et al., 2008; Vasas and Hohmann, 2014), four are found in our mass spectral molecular networks (i.e., diterpenoids; triterpenoids including cholestane and ergostane steroids; steroid lactones and stigmastanes; and glycosylglycerols corresponding to cerebrosides). Additionally, in silico structure annotation suggests the presence of tricarboxylic and benzoic acids and derivatives as well as fatty alcohols and glycosphingolipids (Figure 3A). Among the Euphorbia diterpenoids, we observe different skeletal types within the same molecular families [two or more connected components of a graph) (Figure 4)]. Many Euphorbia diterpenoid backbone skeletons are isomeric, and their respective fragmentation spectra are highly similar (Nothias-Scaglia et al., 2015). Nonetheless, we were able to distinguish different diterpene spectral fingerprints within a molecular family by mapping Mass2Motifs on the mass spectral molecular networks. Mass2Motifs correspond to common patterns of mass fragments and neutral losses, which are extracted using unsupervised substructure discovery of the MS/MS data through MS2LDA (van der Hooft et al., 2016; Wandy et al., 2018). Consistent with the low chemical diversity exhibited by subg. Chamaesyce and previous observations of Euphorbia diterpenoids exhibiting anti-herbivore biological activity (Batra, 1983; Hundsdoerfer et al., 2005a; Huang et al., 2014; Hua et al., 2017), we find very few to no Euphorbia diterpenoids in representatives of the American radiation of subg. Chamaesyce (Figure 1).
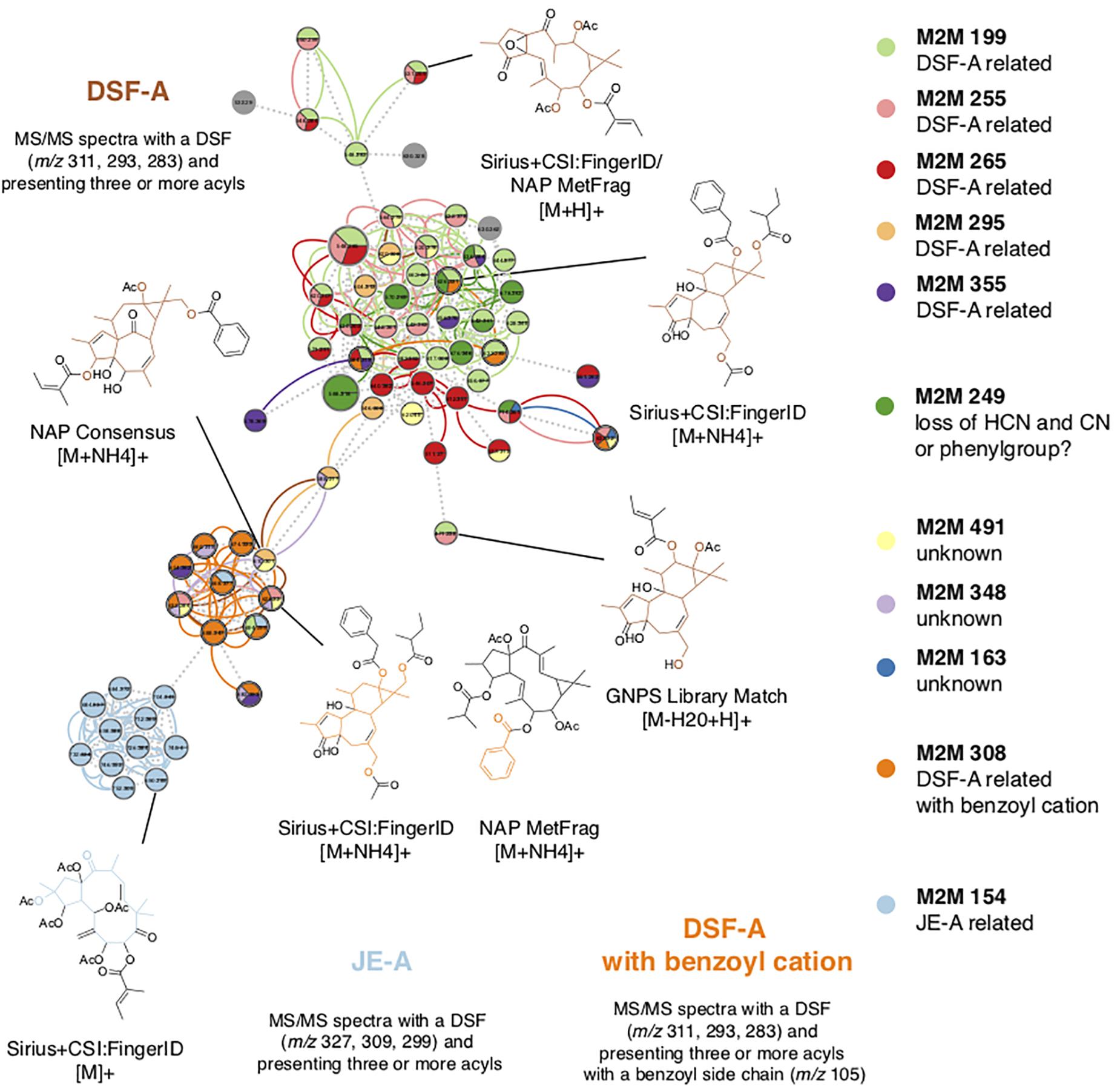
Figure 4. Putative identification of chemical compound classes. We putatively identified compound classes within the mass spectral molecular networks by combining in silico annotation with automated chemical classification and substructure recognition (MS2LDA). Euphorbia diterpenoids exhibit many isoforms; therefore, different diterpene backbone skeletons were found within the same molecular family. Matching substructures (Mass2Motifs) associated with diterpenoid substructures obtained from matches to reference spectra and in silico structure annotation enabled the identification of different diterpene spectral fingerprints clustered within one molecular family. Node size represents the total ion current (TIC) of all samples analyzed, edge colors represent different substructures (Mass2Motifs) that are shared across different nodes, and dotted lines connecting the nodes represent the cosine score. M2M, Mass2Motif; DSF-A, diterpene spectral fingerprint type A; JE-A, Jatrophane ester type A.
Assessing intra-specimen distribution of specialized metabolites can reveal possible ecological functions. Specialized metabolites allocated for the defense against herbivory, for example, can be highly compartmentalized and enriched only in specialized structures such as secretory ducts or laticifers found in roots or stems, as a strategy used by the plants to avoid autotoxicity (Engelberth, 2015). Furthermore, young plant parts may be enriched in defense molecules as a consequence of higher nutritional quality and increased rate of herbivory (Coley and Aide, 1991; Coley and Barone, 1996; Brenes-Arguedas et al., 2006; Kursar et al., 2009). To understand where the Euphorbia diterpenoids are produced within the plants, we dissected four species representing the four subgeneric clades into approximately 20 sections (Supplementary Figures S10, S11). Mass spectrometric investigation revealed that diterpenoids are primarily found in the roots, in representatives of subgenera Euphorbia and Athymalus E. milii var. hislopii (N.E.Br.) Ursch & Leandri and E. horrida; Figure 5 and Supplementary Figures S3, S6–S9). In the European subg. Esula (E. lathyris), diterpenoid production is also pronounced in other plant parts, such as the young stems (Figure 4 and Supplementary Figure S5). Consistent with the lower chemical diversity reported above, diterpenoid production is reduced or absent from most sections throughout the whole plant in E. hirta, a representative of the American clade within subg. Chamaesyce (Figure 5 and Supplementary Figures S4, S10). Compartmentalization of the diterpenoids to mainly young stems and roots could suggest possible functions as anti-feeding molecules (McKey, 1979; Batra, 1983; Hundsdoerfer et al., 2005a; Huang et al., 2014; Hua et al., 2017).
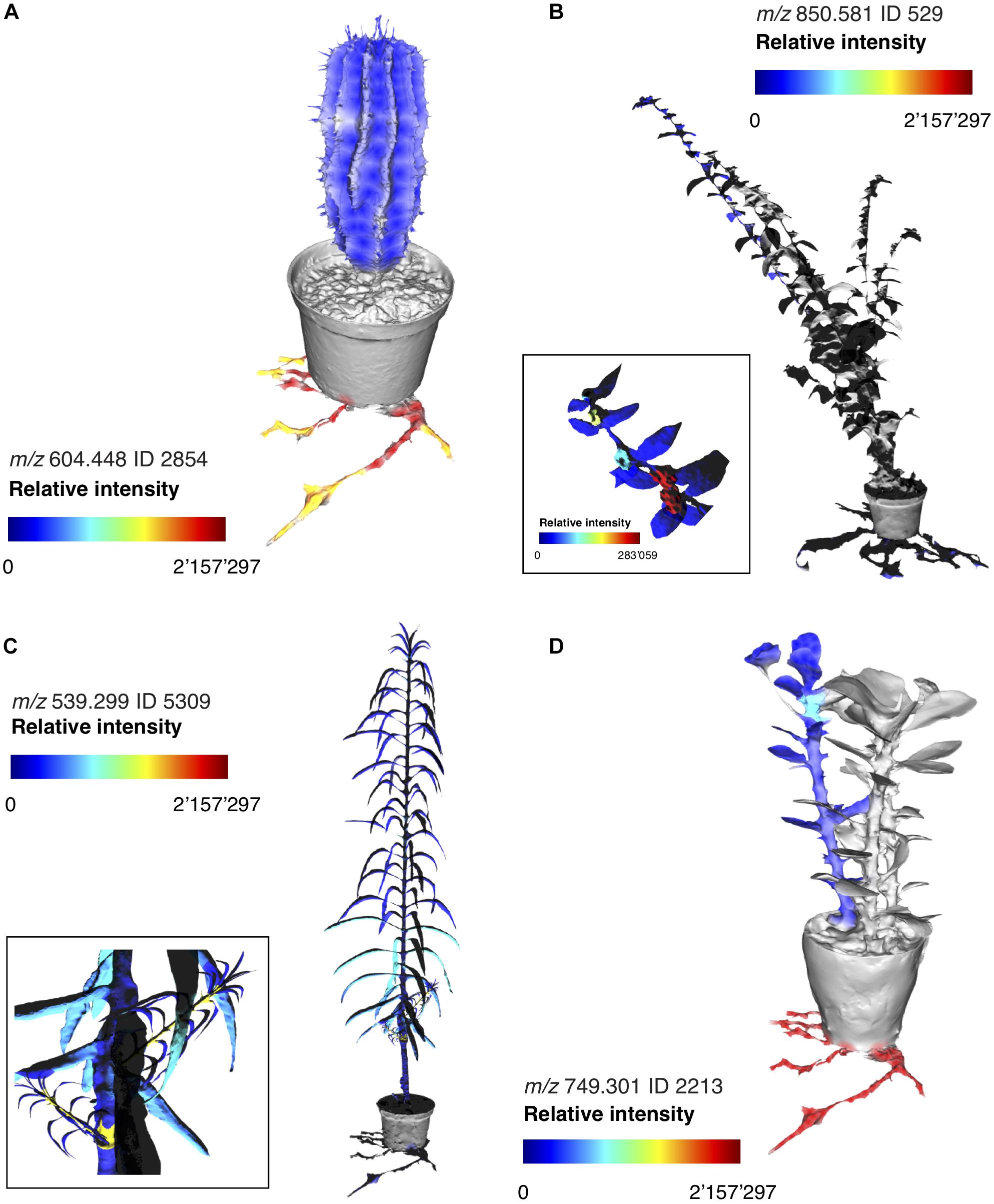
Figure 5. Molecular maps of selected Euphorbia diterpenoids across representatives of each subgeneric clade. Relative intensity of LC–MS molecular features annotated as Euphorbia diterpenoids through spectral matching (A) Euphorbia horrida, subg. Athymalus, (B) Euphorbia hirta, subg. Chamaesyce, (C) Euphorbia lathyris, subg. Esula, and (D) Euphorbia milii var. hislopii, subg. Euphorbia. For interactive cartographical snapshots, see Supplementary URL S1, Links 1–15. The 3D images are for illustrative purposes only and do not represent exact locations of sample collection.
As anti-herbivore activity cannot be directly tested from the past continental transition and data on Euphorbia herbivory at a global scale are very scarce, we set out to test a bioactivity that could reflect a chemical defense mechanism. One strategy of defense invoked by plants to overcome their sessile habit is through local immunomodulatory and inflammatory effects on herbivores. Previous studies (Huang et al., 2014; Hua et al., 2017) have reported anti-feeding activity of selected Euphorbia diterpenoids against generalist plant-feeding insects, Spodoptera exigua (Hübner, 1808) and Helicoverpa armigera (Hübner, 1808). In vitro, Euphorbia diterpenoids are known to induce different immunomodulatory responses through the selective modulation of PKC including the release of prostaglandins and pro-inflammatory cytokines such as TNF-α, interleukin-6, and interleukin-1β (Smith and Meldrum, 1992; Berman, 2012; Uto et al., 2012; Jian et al., 2018; Zhang et al., 2019). Here, we evaluated the pro-inflammatory potential of the crude Euphorbia extracts by measuring the capacity of small extract fractions, corresponding to compounds or compound groups in each of the 43 Euphorbia species that modulate in vitro TNF-α release from PBMCs. Both vertebrates and invertebrates produce cytokines, and assuming they may exhibit similar functions both in insect as well as in mammalian cells (Adamo, 2008), this assay setup may be considered an approximation of potential anti-herbivore activity, independent of the species, as Euphorbia may experience attack by both vertebrates and invertebrates (Batra, 1983; Loutit et al., 1987; Hundsdoerfer et al., 2005a; Huang et al., 2014; Hua et al., 2017). Figure 2 and Supplementary Figures S2–S45 in Supplementary Data S2 show TNF-α modulating properties of all 43 Euphorbia fractionated extracts, where fractions with significant modulation of TNF-α without having a significant effect on cell viability (Supplementary Methods S8) are highlighted in blue. Several fractions with significant modulation of TNF-α are found in representatives of subgenera Athymalus, Esula, and Euphorbia (Supplementary Figures S2–S9, S20–S45 in Supplementary Data S2), whereas only one out of nine representatives (Euphorbia thymifolia; Supplementary Figure S19 in Supplementary Data S2) of the American subg. Chamaesyce is found to contain immunomodulatory compounds or compound groups. To address the role of Euphorbia diterpenoids involved with TNF-α modulating properties, we tested for correlation between the number of TNF-α modulating fractions and the number of molecules within the previously annotated chemical classes using PGLS regression analysis. The association between the number of TNF-α modulating fractions and the number of Euphorbia diterpenoids was found significant (p-value: 0.02) (Figures 1B,C, Supplementary Figure S12, and Supplementary Table S1), supporting our hypothesis of a possible ecological function of Euphorbia diterpenoids as immunomodulatory defense molecules.
Finally, to explore the possibility of Euphorbia diterpenoids being produced as a consequence of local plant–herbivore coevolutionary hotspots, we searched for known Euphorbia herbivores in the literature. Several hawk moth species of the genus Hyles were found to be highly specialized herbivores of Euphorbia (Batra, 1983; Hundsdoerfer et al., 2009). They have been shown to exhibit host specificity and to tolerate the highly toxic Euphorbia diterpenoids, which they reuse as a defense strategy against their own predators by regurgitating plant material from the gut (Batra, 1983; Hundsdoerfer et al., 2005a; Huang et al., 2014; Hua et al., 2017). Native species distribution data suggest a close co-occurrence of Euphorbia-feeding Hyles species with the chemically highly diverse and biologically active European species of subg. Esula (Figure 1A), and an absence in the American habitats of the chemically less diverse and biologically little active representatives of subg. Chamaesyce. This finding supports the hypothesis of co-evolutionary hotspots driving chemical defense diversity. However, further evidence linking specialized metabolite profiles with Hyles herbivory, tolerance mechanisms, or other possible mammalian or insect herbivory and distribution would be needed to draw a strong conclusion.
Discussion
Following coevolutionary theory, we hypothesized that specialized metabolite diversity would show significant phylogenetic structure in Euphorbia, which could be driven by the chemical structural diversity of metabolites potentially used in the defense against herbivory. Furthermore, as a consequence of herbivore–plant coevolutionary hotspots, we would observe significant differences in specialized metabolite diversity across phylogenetic clades geographically separated through evolutionary time.
Our results show that specialized metabolite diversity as well as the number of Euphorbia diterpenoids with previously reported feeding-deterrent properties (Batra, 1983; Hundsdoerfer et al., 2005a; Huang et al., 2014; Hua et al., 2017) is significantly reduced in phylogenetic clades native to the Americas, compared to species native to the Afro-Eurasian continents. The localization of these compounds to young stems and roots as well as reduced immunomodulatory activity in species of the American subclade further support their possible ecological function as immunomodulatory defense molecules. Furthermore, these findings could suggest chemo-evolutionary adaptation to reduced herbivory pressure in the Americas. High specialized metabolite diversity, number of defensive molecules and immunomodulatory activity, on the other hand, suggests a coevolutionary arms race in species native to the Afro-Eurasian continents. Euphorbia originated in Africa and only later expanded to the Americas (Zimmermann et al., 2010; Yang and Berry, 2011; Horn et al., 2012, 2014; Yang et al., 2012; Dorsey et al., 2013; Peirson et al., 2013; Riina et al., 2013). Thus, if the maintenance of the chemical coevolutionary defensive traits come at a cost, species of the American subclade are indeed most likely to have lost this trait, as maintenance would require an equally specialized herbivore in the newly occupied habitats. Our results are consistent with this hypothesis with species of the American radiation of subg. Chamaesyce exhibiting least chemical structural diversity, none to very few Euphorbia diterpenoids as well as immunomodulatory activity.
If Euphorbia specialized metabolite diversity is a consequence of herbivore–plant coevolutionary hotspots, we would expect specialized Euphorbia herbivores to geographically co-occur with chemically highly diverse and biologically active Euphorbia species. Native species distribution data suggest a close co-occurrence of Euphorbia-feeding Hyles species with the chemically highly diverse and biologically active European species of subg. Esula (Figure 1A). On the other hand, Euphorbia-feeding Hyles are not native to the American habitats, where chemically less diverse and biologically little active representatives of subg. Chamaesyce occur (Ernst et al., 2018, Preprint). This supports the hypothesis that specialized metabolite diversity in Euphorbia may be a consequence of herbivore–plant coevolutionary hotspots. However, our data also suggest that African members of subg. Athymalus and subg. Euphorbia occurring in Southern Africa and Madagascar, outside of the distribution range of Euphorbia-feeding Hyles, produce a high diversity of feeding deterrent diterpenoids. We speculate that previously not described (or extinct) generalist or specialist Euphorbia-feeding herbivores occur (or occurred) in these regions, which contributed to maintaining adaptive pressure. The black rhinoceros, Diceros bicornis (Linnaeus, 1758) distributed in the southern African subregion, for example, was found to feed often and extensively on African Euphorbia species of subg. Euphorbia (Loutit et al., 1987). Anecdotal evidence of the lack of specialized herbivores in the Americas also corroborate with our results. A single hawk moth species, Hyles euphorbiae, was only introduced recently to North America (Batra, 1983), where it was used as a host-specific enemy and biological control for the reportedly highly invasive European species of subg. Esula (E. cyparissias L. and E. esula L.), lacking herbivores in the newly occupied habitats. Furthermore, although the poinsettia (E. pulcherrima Willd. ex Klotzsch), a very well-known ornamental plant and American representative of subg. Chamaesyce, is notorious for its “extreme toxicity” among the general public, toxicity remains unconfirmed in the clinic, as 92.4% of patients exposed to the plant did not develop adverse effects (Krenzelok et al., 1996). This corroborates with our results of the low chemical diversity, diterpenoid content, and immunomodulatory activity found in representatives of subg. Chamaesyce.
Research exploring the geographic variation of plant–herbivore co-evolution can increase our understanding of the processes that generate and maintain plant chemical diversity across landscapes (Dyer et al., 2018). Here we assessed the evolution of specialized metabolite diversity in relation to the biogeographic history of the cosmopolitan genus Euphorbia. Although extensive genetic studies would be necessary to reveal evidence for the coevolutionary relationship across Euphorbia species and its herbivores, our study reveals for the first time potential evolutionary drivers of specialized metabolite diversity at a global scale.
Plant specialized metabolite diversity is an important driver of many aspects of biodiversity, among others shaping trophic interactions, species diversity, and ecological coexistence (Richards et al., 2015; Sedio, 2017). The evolutionary origins and ecological consequences of specialized metabolite diversity have been addressed by several well-established hypotheses in chemical ecology; however, technical limitations in assessing chemical structures in high-throughput have so far precluded rigorous hypothesis testing (Bednarek and Osbourn, 2009; Richards et al., 2015; Allard et al., 2016; Sedio, 2017; Dyer et al., 2018). Consequently, most previous studies have either focused on broad chemical classes of compounds (Ayres et al., 1997; Agrawal et al., 2009; Kursar et al., 2009), a small number of well-characterized molecules (Ayres et al., 1997; Becerra, 1997, 2007; Kursar et al., 2009), or a large quantity of unidentified chemical entities (Richards et al., 2015; Sedio et al., 2017). Here we performed the semi-automated putative identification of over 30% of the metabolites detected up to the level of chemical structures or subclasses. This corresponds to more than five times as many identified metabolites when compared to most recent studies using mass spectral metabolomics techniques to investigate plant specialized metabolite diversity (Sedio et al., 2017). Compared to previous studies, our approach thus represents a significant technical advance and reveals an unprecedented insight into plant chemical diversity in Euphorbia. Computational mass spectrometric metabolomics tools introduced here are likely to revolutionize our understanding of evolutionary and ecological mechanisms underlying plant chemical diversity in the future.
Code and Data Availability
The datasets generated for this study can be found in https://massive.ucsd.edu/, MSV000081082, and MSV000081083.
Author Contributions
ME, L-FN, CS-L, OG, HS, NN, DS, PD, and NR designed the study. ME and KM-S collected and sampled Euphorbia species from the Botanical Garden at the Natural History Museum of Denmark. GH and L-AF collected seeds of Brazilian Euphorbia species and performed taxonomic circumscription and identification. ME prepared the plant extracts. L-FN performed LC-MS/MS analysis of the pooled Euphorbia extracts. ME performed LC-MS/MS analysis for the 3D mass spectral molecular cartography. ME and L-FN performed mass spectral molecular networking analysis. JvdH performed MS2LDA analysis. L-FN, JvdH, and ME annotated Mass2Motifs on MS2LDA. ME and JvdH developed and performed the (semi-)automated annotation workflow by combining mass spectral molecular networking with MS2LA, in silico annotation and ClassyFire. RS developed dereplication strategies through NAP and provided support for data analysis. NN, PL, DS, and ME designed the high-resolution bioactivity study. ME performed the high-resolution bioactivity study and analyzed data together with DS, NN, and PL. ME generated the phylogenetic hypothesis, performed comparative analyses and wrote all scripts used for data analysis. ME wrote the manuscript together with L-FN, NR, and PD. All authors discussed the results and commented on the manuscript.
Funding
This work was supported by the Marie Curie Actions of the 7th European Community Framework Programme: FP7/2007-2013/, REA grant agreement no. 606895-MedPlant to NR and a grant from the Aase and Ejnar Danielsens Foundation to ME, and the MSCA-IF-2016 no. 704786 to L-FN. The TNF-α experiments were conducted at and partly funded by LEO Pharma A/S. LC–MS/MS analyses were funded through the Center for Computational Mass Spectrometry P41 GM103484, R01 GM107550, and the NIH grant on reuse of metabolomics data R03 CA211211. This manuscript has been released as a pre-print at https://www.biorxiv.org/content/10.1101/323014v1.
Conflict of Interest Statement
The authors declare that the research was conducted in the absence of any commercial or financial relationships that could be construed as a potential conflict of interest.
Acknowledgments
The authors thank Ivan Protsyuk and Theodore Alexandrov (EMBL) for their support in using the KNIME workflow Optimus and MassFuser; Arife Önder for technical assistance with fractionation; Martin Aarseth-Hansen and Gry Bastholm for assistance with collecting plant material and co-workers of LEO Pharma A/S for technical assistance with biological assays. Kevin Nguyen, Brandon Ross, and Kirandeep Chhokar (UCSD) are thanked for assistance with preparing plant extracts for the 3D mass spectral molecular cartography.
Supplementary Material
The Supplementary Material for this article can be found online at: https://www.frontiersin.org/articles/10.3389/fpls.2019.00846/full#supplementary-material
Footnotes
- ^ https://github.com/MolecularCartography/Optimus
- ^ http://gnps.ucsd.edu
- ^ http://dnp.chemnetbase.com
- ^ http://classyfire.wishartlab.com/
- ^ https://remake.autodesk.com/
- ^ http://www.meshmixer.com/
- ^ https://github.com/MolecularCartography/ili
- ^ https://www.gbif.org
- ^ http://www.R-project.org/
- ^ https://doi.org/10.5061/dryad.t513409
References
Adamo, S. A. (2008). “6 - Bidirectional connections between the immune system and the nervous system in insects,” in Insect Immunology, ed. N. E. Beckage (San Diego, CA: Academic Press), 129–149. doi: 10.1016/b978-012373976-6.50008-2
Agrawal, A. A., Salminen, J. P., and Fishbein, M. (2009). Phylogenetic trends in phenolic metabolism of milkweeds (Asclepias): evidence for escalation. Evolution 63, 663–673. doi: 10.1111/j.1558-5646.2008.00573.x
Allard, P. M., Péresse, T., Bisson, J., Gindro, K., Marcourt, L., Pham, V. C., et al. (2016). Integration of molecular networking and in-silico MS/MS fragmentation for natural products dereplication. Anal. Chem. 88, 3317–3323. doi: 10.1021/acs.analchem.5b04804
Appendino, G. (2016). “Ingenane diterpenoids,” in Progress in the Chemistry of Organic Natural Products, eds A. D. Kinghorn, H. Falk, S. Gibbons, and J. Kobayashi (Cham, CH: Springer International Publishing), 1–90. doi: 10.1007/978-3-319-33172-0_1
Ayres, M. P., Clausen, T. P., MacLean, S. F., Redman, A. M., and Reichardt, P. B. (1997). Diversity of structure and antiherbivore activity in condensed tannins. Ecology 78, 1696–1712. doi: 10.1890/0012-9658(1997)078
Batra, S. W. T. (1983). Establishment of Hyles Euphorbiae (L.) (Lepidoptera: Sphingidae) in the United States for control of the weedy spurges Euphorbia esula L. and E. cyparissias L. J. N. Y. Entomol. Soc. 91, 304–311.
Becerra, J. X. (1997). Insects on plants: macroevolutionary chemical trends in host use. Science 276, 253–256. doi: 10.1126/science.276.5310.253
Becerra, J. X. (2007). The impact of herbivore–plant coevolution on plant community structure. Proc. Natl. Acad. Sci. U.S.A. 104, 7483–7488. doi: 10.1073/pnas.0608253104
Bednarek, P., and Osbourn, A. (2009). Plant-microbe interactions: chemical diversity in plant defense. Science 324, 746–748. doi: 10.1126/science.1171661
Berman, B. (2012). New developments in the treatment of actinic keratosis: focus on ingenol mebutate gel. Clin. Cosmet. Investig. Dermatol. 20, 111–122. doi: 10.2147/CCID.S28905
Birmingham, A., Selfors, L. M., Forster, T., Wrobel, D., Kennedy, C. J., Shanks, E., et al. (2009). Statistical methods for analysis of high-throughput RNA interference screens. Nat. Methods 6, 569–575. doi: 10.1038/nmeth.1351
Bouslimani, A., Porto, C., Rath, C. M., Wang, M., Guo, Y., Gonzalez, A., et al. (2015). Molecular cartography of the human skin surface in 3D. Proc. Natl. Acad. Sci. U.S.A. 112, E2120–E2129. doi: 10.1073/pnas.1424409112
Böcker, S., and Dührkop, K. (2016). Fragmentation trees reloaded. J. Cheminform. 8:5. doi: 10.1186/s13321-016-0116-8
Brenes-Arguedas, T., Horton, M. W., Coley, P. D., Lokvam, J., Waddell, R. A., Meizoso-O’Meara, B. E., et al. (2006). Contrasting mechanisms of secondary metabolite accumulation during leaf development in two tropical tree species with different leaf expansion strategies. Oecologia 149, 91–100. doi: 10.1007/s00442-006-0423-2
Cignoni, P., Callieri, M., Corsini, M., Dellepiane, M., Ganovelli, F., and Ranzuglia, G. (2008). “Meshlab: an open-source mesh processing tool,” in Proceedings of the Eurographics Italian Chapter Conference, eds V. Scarano, R. D. Chiara, and U. Erra (Genova: The Eurographics Association).
Coley, P., and Aide, T. M. (1991). “Comparison of herbivory and plant defenses in temperate and tropical broad-leaved forests,” in Plant-Animal Interactions: Evolutionary Ecology in Tropical and Temperate Regions, eds P. W. Price, T. Lewinsohn, G. W. Fernandes, and W. W. Benson (New York, NY: Wiley & Sons), 25–49.
Coley, P. D., and Barone, J. A. (1996). Herbivory and plant defenses in tropical forests. Annu. Rev. Ecol. Syst. 27, 305–335. doi: 10.1146/annurev.ecolsys.27.1.305
da Silva, O. L. M., and Cordeiro, I. (2016). Euphorbia sarcoceras, a new species of Euphorbia sect. Alectoroctonum from Brazil. Syst. Bot. 40, 962–967. doi: 10.3389/fpls.2018.00660
da Silva, R. R., Wang, M., Nothias, L. F., van der Hooft, J. J. J., Caraballo-Rodríguez, A. M., Fox, E., et al. (2018). Propagating annotations of molecular networks using in silico fragmentation. PLoS Comput. Biol. 14:e1006089. doi: 10.1371/journal.pcbi.1006089
Djoumbou Feunang, Y., Eisner, R., Knox, C., Chepelev, L., Hastings, J., Owen, G., et al. (2016). ClassyFire: automated chemical classification with a comprehensive, computable taxonomy. J. Cheminform. 8:61.
Dorsey, B. L., Haevermans, T., Aubriot, X., Morawetz, J. J., Riina, R., Steinmann, V. W., et al. (2013). Phylogenetics, morphological evolution, and classification of Euphorbia subgenus Euphorbia (Euphorbiaceae). TAXON 62, 291–315. doi: 10.12705/622.1
Dührkop, K., Shen, H., Meusel, M., Rousu, J., and Böcker, S. (2015). Searching molecular structure databases with tandem mass spectra using CSI: fingerid. Proc. Natl. Acad. Sci. U.S.A. 112, 12580–12585. doi: 10.1073/pnas.1509788112
Dyer, L. A., Philbin, C. S., Ochsenrider, K. M., Richards, L. A., Massad, T. J., Smilanich, A. M., et al. (2018). Modern approaches to study plant–insect interactions in chemical ecology. Nat. Rev. Chem. 2, 50–64.
Ehrlich, P. R., and Raven, P. H. (1964). Butterflies and plants: a study in coevolution. Evolution 18, 586–608. doi: 10.1111/j.1558-5646.1964.tb01674.x
Engelberth, J. (2015). “23: Biotic interactions,” in Plant Physiology and Development, eds L. Taiz, E. Zeiger, I. M. Møller, and A. Murphy (Sunderland, MA: Sinauer Associates, Incorporated), 693–729.
Ernst, M., Nothias, L. F., van der Hooft, J. J. J., Silva, R. R., Saslis-Lagoudakis, C. H., Grace, O. M., et al. (2018). Did a plant-herbivore arms race drive chemical diversity in Euphorbia? bioRxivGoogle Scholar
Firn, R. D., and Jones, C. G. (2003). Natural products - a simple model to explain chemical diversity. Nat. Prod. Rep. 20, 382–391.
Floros, D. J., Petras, D., Kapono, C. A., Melnik, A. V., Ling, T. J., Knight, R., et al. (2017). Mass spectrometry based molecular 3D-cartography of plant metabolites. Front. Plant Sci. 8:429. doi: 10.3389/fpls.2017.00429
Govaerts, R., Dransfield, J., Zona, S., Hodel, D. R., and Henderson, A. (2014). World Checklist of Euphorbiaceae. Sydney: Royal Botanic Gardens.
Horn, J. W., van Ee, B. W., Morawetz, J. J., Riina, R., Steinmann, V. W., Berry, P. E., et al. (2012). Phylogenetics and the evolution of major structural characters in the giant genus Euphorbia L. (Euphorbiaceae). Mol. Phylogenet. Evol. 63, 305–326. doi: 10.1016/j.ympev.2011.12.022
Horn, J. W., Xi, Z., Riina, R., Peirson, J. A., Yang, Y., Dorsey, B. L., et al. (2014). Evolutionary bursts in Euphorbia (Euphorbiaceae) are linked with photosynthetic pathway. Evolution 68, 3485–3504. doi: 10.1111/evo.12534
Hua, J., Liu, Y., Xiao, C. J., Jing, S. X., Luo, S. H., and Li, S. H. (2017). Chemical profile and defensive function of the latex of Euphorbia peplus. Phytochemistry 136, 56–64. doi: 10.1016/j.phytochem.2016.12.021
Huang, C. S., Luo, S. H., Li, Y. L., Li, C. H., Hua, J., Liu, Y., et al. (2014). Antifeedant and antiviral diterpenoids from the fresh roots of Euphorbia jolkinii. Nat. Prod. Bioprospect. 4, 91–100. doi: 10.1007/s13659-014-0009-3
Hundsdoerfer, A. K., Kitching, I. J., and Wink, M. (2005a). The phylogeny of the hyles euphorbiae complex (Lepidoptera: Sphingidae): molecular evidence from sequence data and ISSR-PCR fingerprints. Org. Divers. Evol. 5, 173–198. doi: 10.1016/j.ode.2004.11.012
Hundsdoerfer, A. K., Tshibangu, J. N., Wetterauer, B., and Wink, M. (2005b). Sequestration of phorbol esters by aposematic larvae of Hyles Euphorbiae (Lepidoptera: Sphingidae): Chemoecology 15, 261–267. doi: 10.1007/s00049-005-0321-9
Hundsdoerfer, A. K., Rubinoff, D., Attieì, M., Wink, M., and Kitching, I. J. (2009). A revised molecular phylogeny of the globally distributed hawkmoth genus Hyles (Lepidoptera: Sphingidae), based on mitochondrial and nuclear DNA sequences. Mol. Phylogenet. Evol. 52, 852–865. doi: 10.1016/j.ympev.2009.05.023
Jian, B., Zhang, H., and Liu, J. (2018). Structural diversity and biological activities of diterpenoids derived from Euphorbia fischeriana Steud. Molecules 23:935. doi: 10.3390/molecules23040935
Jones, C. G., and Firn, R. D. (1991). On the evolution of plant secondary chemical diversity. Philos. Trans. R. Soc. Lond. B Biol. Sci. 333, 273–280. doi: 10.1098/rstb.1991.0077
Kalliolias, G. D., and Ivashkiv, L. B. (2016). TNF biology, pathogenic mechanisms and emerging therapeutic strategies. Nat. Rev. Rheumatol. 12, 49–62. doi: 10.1038/nrrheum.2015.169
Krenzelok, E. P., Jacobsen, T., and Aronis, J. M. (1996). Poinsettia exposures have good outcomes just as we thought. Am. J. Emerg. Med. 14, 671–674. doi: 10.1016/s0735-6757(96)90086-8
Kursar, T. A., Dexter, K. G., Lokvam, J., Pennington, R. T., Richardson, J. E., Weber, M. G., et al. (2009). The evolution of antiherbivore defenses and their contribution to species coexistence in the tropical tree genus Inga. Proc. Natl. Acad. Sci. U.S.A. 106, 18073–18078. doi: 10.1073/pnas.0904786106
Loutit, B. D., Louw, G. N., and Seely, M. K. (1987). First approximation of food preferences and the chemical composition of the diet of the desert-dwelling black rhinoceros, Diceros bicornis L. Madoqua 15, 35–54.
McKey, D. (1979). “The distribution of secondary compounds within plants,” in Herbivores: Their Interaction with Secondary Plant Metabolites, eds G. A. Rosenthal and D. H. Janzen (Cambridge, MA: Academic Press), 56–134.
Meerman, J. C. (1993). Relationships within the Hyles Euphorbiae-complex: a numerical taxonomy approach (Lepidoptera: Sphingidae). Entomol. Gaz. 44, 205–209.
Monaco, C., Nanchahal, J., Taylor, P., and Feldmann, M. (2014). Anti-TNF therapy: past, present and future. Int. Immunol. 27, 55–62. doi: 10.1093/intimm/dxu102
Nothias-Scaglia, L. F., Schmitz-Afonso, I., Renucci, F., Roussi, F., Touboul, D., Costa, J., et al. (2015). Insights on profiling of phorbol, deoxyphorbol, ingenol and jatrophane diterpene esters by high performance liquid chromatography coupled to multiple stage mass spectrometry. J. Chromatogr. A 1422, 128–139. doi: 10.1016/j.chroma.2015.09.092
Orme, D., Freckleton, R., Thomas, G., Petzoldt, T., Fritz, S., Isaac, N., et al. (2013). caper: Comparative Analyses of Phylogenetics and Evolution in R. R package version 0.5.2. Available at: http://CRAN.Rproject.org/package=caperGoogle Scholar
Peirson, J. A., Bruyns, P. V., Riina, R., Morawetz, J. J., and Berry, P. E. (2013). A molecular phylogeny and classification of the largely succulent and mainly African Euphorbia subg. Athymalus (Euphorbiaceae). TAXON 62, 1178–1199. doi: 10.12705/626.12
Pluskal, T., Castillo, S., Villar-Briones, A., and Orešič, M. (2010). MZmine 2: modular framework for processing, visualizing, and analyzing mass spectrometry-based molecular profile data. BMC Bioinformatics 11:395. doi: 10.1186/1471-2105-11-395
Protsyuk, I., Melnik, A. V., Nothias, L. F., Rappez, L., Phapale, P., Aksenov, A. A., et al. (2018). 3D molecular cartography using LC–MS facilitated by optimus and ’ili software. Nat. Protoc. 13, 134–154. doi: 10.1038/nprot.2017.122
Revell, L. J. (2012). phytools: an R package for phylogenetic comparative biology (and other things). Methods Ecol. Evol. 3, 217–223. doi: 10.1111/j.2041-210x.2011.00169.x
Richards, L. A., Dyer, L. A., Forister, M. L., Smilanich, A. M., Dodson, C. D., Leonard, M. D., et al. (2015). Phytochemical diversity drives plant–insect community diversity. Proc. Natl. Acad. Sci. U.S.A. 112, 10973–10978. doi: 10.1073/pnas.1504977112
Riina, R., Peirson, J. A., Geltman, D. V., Molero, J., Frajman, B., Pahlevani, A., et al. (2013). A worldwide molecular phylogeny and classification of the leafy spurges, Euphorbia subgenus Esula (Euphorbiaceae). TAXON 62, 316–342. doi: 10.12705/622.3
Röst, H. L., Sachsenberg, T., Aiche, S., Bielow, C., Weisser, H., Aicheler, F., et al. (2016). OpenMS: a flexible open-source software platform for mass spectrometry data analysis. Nat. Methods 13, 741–748. doi: 10.1038/nmeth.3959
Sedger, L. M., and McDermott, M. F. (2014). TNF and TNF-receptors: from mediators of cell death and inflammation to therapeutic giants - past, present and future. Cytokine Growth Factor Rev. 25, 453–472. doi: 10.1016/j.cytogfr.2014.07.016
Sedio, B. E. (2017). Recent breakthroughs in metabolomics promise to reveal the cryptic chemical traits that mediate plant community composition, character evolution and lineage diversification. New Phytol. 214, 952–958. doi: 10.1111/nph.14438
Sedio, B. E., Rojas Echeverri, J. C., Boya, P. C. A., and Wright, S. J. (2017). Sources of variation in foliar secondary chemistry in a tropical forest tree community. Ecology 98, 616–623. doi: 10.1002/ecy.1689
Shannon, P., Markiel, A., Ozier, O., Baliga, N. S., Wang, J. T., Ramage, D., et al. (2003). Cytoscape: a software environment for integrated models of biomolecular interaction networks. Genome Res. 13, 2498–2504. doi: 10.1101/gr.1239303
Shi, Q. W., Su, X. H., and Kiyota, H. (2008). Chemical and pharmacological research of the plants in genus Euphorbia. Chem. Rev. 108, 4295–4327.
Smith, S. E., and Meldrum, B. S. (1992). The protein kinase C activators, phorbol 12-myristate,13- acetate and phorbol 12,13-dibutyrate, are convulsant in the pico-nanomolar range in mice. Eur. J. Pharmacol. 213, 133–135. doi: 10.1016/0014-2999(92)90242-v
Sumner, L. W., Amberg, A., Barrett, D., Beale, M. H., Beger, R., Daykin, C. A., et al. (2007). Proposed minimum reporting standards for chemical analysis chemical analysis working group (CAWG) metabolomics standards initiative (MSI). Metabolomics 3, 211–221. doi: 10.1007/s11306-007-0082-2
Symonds, M. R. E., and Blomberg, S. P. (2014). “A primer on phylogenetic generalised least squares,” in Modern Phylogenetic Comparative Methods and Their Application in Evolutionary Biology: Concepts and Practice, ed. L. Z. Garamszegi (Berlin: Springer), 105–130. doi: 10.1007/978-3-662-43550-2_5
Thompson, J. N. (2005a). Coevolution: the geographic mosaic of coevolutionary arms races. Curr. Biol. 15, R992–R994.
Thompson, J. N. (2005b). The Geographic Mosaic of Coevolution, Interspecific Interactions. Chicago, IL: University of Chicago Press.
Thompson, J. N. (2008). Coevolution, Cryptic Speciation, and the Persistence of Interactions. Oakland, CA: University of California Press, 216–224.
Uto, T., Qin, G. W., Morinaga, O., and Shoyama, Y. (2012). 17-Hydroxy-jolkinolide B, a diterpenoid from Euphorbia fischeriana, inhibits inflammatory mediators but activates heme oxygenase-1 expression in lipopolysaccharide-stimulated murine macrophages. Int. Immunopharmacol. 12, 101–109. doi: 10.1016/j.intimp.2011.10.020
van der Hooft, J. J. J., Wandy, J., Barrett, M. P., Burgess, K. E. V., and Rogers, S. (2016). Topic modeling for untargeted substructure exploration in metabolomics. Proc. Natl. Acad. Sci. U.S.A. 113, 13738–13743. doi: 10.1073/pnas.1608041113
Vasas, A., and Hohmann, J. (2014). Euphorbia diterpenes: isolation, structure, biological activity, and synthesis (2008-2012). Chem. Rev. 114, 8579–8612. doi: 10.1021/cr400541j
Vogg, G., Achatz, S., Kettrup, A., and Sandermann, H. (1999). Fast, sensitive and selective liquid chromatographic-tandem mass spectrometric determination of tumor-promoting diterpene esters. J. Chromatogr. A 855, 563–573. doi: 10.1016/s0021-9673(99)00728-1
Wandy, J., Zhu, Y., van der Hooft, J. J. J., Daly, R., Barrett, M. P., and Rogers, S. (2018). Ms2lda.org: web-based topic modelling for substructure discovery in mass spectrometry. Bioinformatics 34, 317–318. doi: 10.1093/bioinformatics/btx582
Wang, M., Carver, J. J., Phelan, V. V., Sanchez, L. M., Garg, N., Peng, Y., et al. (2016). Sharing and community curation of mass spectrometry data with global natural products social molecular networking. Nat. Biotechnol. 34, 828–837. doi: 10.1038/nbt.3597
Watrous, J., Roach, P., Alexandrov, T., Heath, B. S., Yang, J. Y., Kersten, R. D., et al. (2012). Mass spectral molecular networking of living microbial colonies. Proc. Natl. Acad. Sci. U.S.A. 109, E1743–E1752. doi: 10.1073/pnas.1203689109
Wink, M. (2010). “Introduction: biochemistry, physiology and ecological functions of secondary metabolites,” in Annual Plant Reviews: Biochemistry of Plant Secondary Metabolism, Vol. 40, ed. M. Wink (Hoboken, NJ: Wiley-Blackwell), 1–19. doi: 10.1002/9781444320503.ch1
Wubshet, S. G., Schmidt, J. S., Wiese, S., and Staerk, D. (2013). High-resolution screening combined with HPLC-HRMS-SPE-NMR for identification of potential health-promoting constituents in sea aster and searocket – new nordic food ingredients. J. Agric. Food Chem. 61, 8616–8623. doi: 10.1021/jf402949y
Yang, Y., and Berry, P. E. (2011). Phylogenetics of the Chamaesyce clade (Euphorbia, Euphorbiaceae): reticulate evolution and long-distance dispersal in a prominent C4 lineage. Am. J. Bot. 98, 1486–1503. doi: 10.3732/ajb.1000496
Yang, Y., Riina, R., Morawetz, J. J., Haevermans, T., Aubriot, X., and Berry, P. E. (2012). Molecular phylogenetics and classification of Euphorbia subgenus Chamaesyce (Euphorbiaceae). TAXON 61, 764–789. doi: 10.1002/tax.614005
Zangerl, A. R., and Berenbaum, M. R. (2003). Phenotype matching in wild parsnip and parsnip web- 696 worms: causes and consequences. Evolution 57, 806–815. doi: 10.1111/j.0014-3820.2003.tb00292.x
Zhang, C. Y., Wu, Y. L., Zhang, P., Chen, Z. Z., Li, H., and Chen, L. X. (2019). Anti-inflammatory lathyrane diterpenoids from Euphorbia lathyris. J. Nat. Prod. 28, 756–764. doi: 10.1021/acs.jnatprod.8b00600
Zhang, J. H., Chung, T. D. Y., and Oldenburg, K. R. (1999). A simple statistical parameter for use in evaluation and validation of high throughput screening assays. J. Biomol. Screen. 4, 67–73. doi: 10.1177/108705719900400206
Keywords: computational metabolomics, coevolution, Euphorbia, specialized metabolites, immunomodulatory testing
Citation: Ernst M, Nothias L-F, van der Hooft JJJ, Silva RR, Saslis-Lagoudakis CH, Grace OM, Martinez-Swatson K, Hassemer G, Funez LA, Simonsen HT, Medema MH, Staerk D, Nilsson N, Lovato P, Dorrestein PC and Rønsted N (2019) Assessing Specialized Metabolite Diversity in the Cosmopolitan Plant Genus Euphorbia L. Front. Plant Sci. 10:846. doi: 10.3389/fpls.2019.00846
Received: 26 April 2019; Accepted: 13 June 2019;
Published: 02 July 2019.
Edited by:
Dorothea Tholl, Virginia Tech, United StatesReviewed by:
Wajid Waheed Bhat, Michigan State University, United StatesMarco Kai, University of Rostock, Germany
Copyright © 2019 Ernst, Nothias, van der Hooft, Silva, Saslis-Lagoudakis, Grace, Martinez-Swatson, Hassemer, Funez, Simonsen, Medema, Staerk, Nilsson, Lovato, Dorrestein and Rønsted. This is an open-access article distributed under the terms of the Creative Commons Attribution License (CC BY). The use, distribution or reproduction in other forums is permitted, provided the original author(s) and the copyright owner(s) are credited and that the original publication in this journal is cited, in accordance with accepted academic practice. No use, distribution or reproduction is permitted which does not comply with these terms.
*Correspondence: Madeleine Ernst, ernst.made@gmail.com; Pieter C. Dorrestein, pdorrestein@ucsd.edu; Nina Rønsted, nronsted@snm.ku.dk
†Present address: Madeleine Ernst, Department of Congenital Disorders, Statens Serum Institut, Copenhagen, Denmark