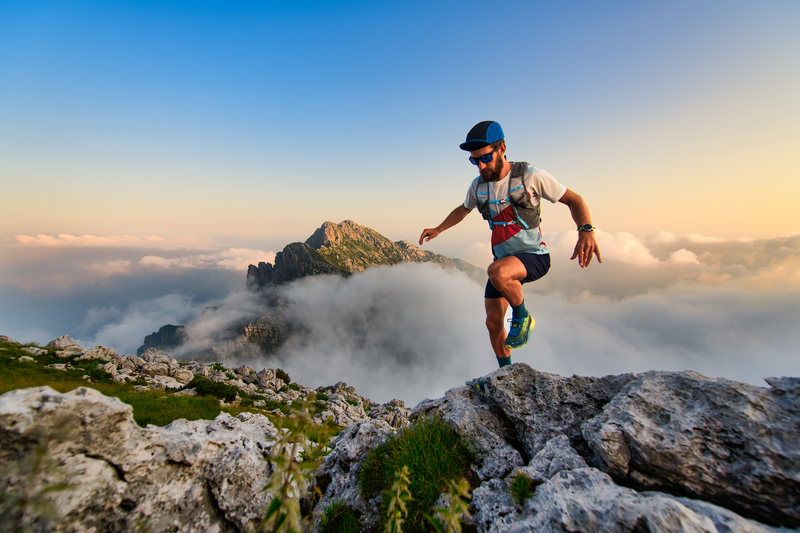
95% of researchers rate our articles as excellent or good
Learn more about the work of our research integrity team to safeguard the quality of each article we publish.
Find out more
ORIGINAL RESEARCH article
Front. Plant Sci. , 09 January 2019
Sec. Plant Breeding
Volume 9 - 2018 | https://doi.org/10.3389/fpls.2018.01968
Fiber quality is an important economic index and a major breeding goal in cotton, but direct phenotypic selection is often hindered due to environmental influences and linkage with yield traits. A genome-wide association study (GWAS) is a powerful tool to identify genes associated with phenotypic traits. In this study, we identified fiber quality genes in upland cotton (Gossypium hirsutum L.) using GWAS based on a high-density CottonSNP80K array and multiple environment tests. A total of 30 and 23 significant single nucleotide polymorphisms (SNPs) associated with five fiber quality traits were identified across the 408 cotton accessions in six environments and the best linear unbiased predictions, respectively. Among these SNPs, seven loci were the same, and 128 candidate genes were predicted in a 1-Mb region (±500 kb of the peak SNP). Furthermore, two major genome regions (GR1 and GR2) associated with multiple fiber qualities in multiple environments on chromosomes A07 and A13 were identified, and within them, 22 candidate genes were annotated. Of these, 11 genes were expressed [log2(1 + FPKM)>1] in the fiber development stages (5, 10, 20, and 25 dpa) using RNA-Seq. This study provides fundamental insight relevant to identification of genes associated with fiber quality and will accelerate future efforts toward improving fiber quality of upland cotton.
Cotton (Gossypium spp.) is the most important natural textile fiber source globally. The genus Gossypium includes approximately 50 species, 45 diploid (2n = 2x = 26) and 5 tetraploid (2n = 4x = 52). The cultivated types of cotton include two diploids, G. arboreum L. and G. herbaceum L., and two tetraploids, G. hirsutum L. and G. barbadense L. Upland cotton (G. hirsutum L.) is the most widely cultivated tetraploid cotton species and accounts for 95% of the world’s cotton production because of its high yield and preferable fiber quality, the chromosomes are often numbered in two sets of 13, A1 through A13 and D1 through D13, the genome sizes is approximately 2,500 Mb in the upland cotton (Wendel and Cronn, 2003; Zhang T. et al., 2015). With changes in spinning technology and diverse uses, the improvement of cotton fiber quality is becoming extremely important (Kohel et al., 2001). Especially in recent years, reform of the supply side structure of cotton has been implemented in China and so fiber quality improvement has become the primary objective of cotton breeding programs (Rong et al., 2007). The identification and characterization of genes associated with fiber quality traits is indispensable for both understanding the genetic basis of phenotypic variation and efficient quality trait improvement.
The fiber quality traits of cotton are complex quantitative traits controlled by quantitative trait loci (QTLs). More than 1000 QTLs associated with fiber quality have been detected by the traditional method based on genetic mapping (Said et al., 2013). Association mapping (AM) based on linkage disequilibrium (LD) is a powerful tool to identify genes associated with agronomic traits, and is convenient because it helps avoid the need to screen large biparental mapping populations (Abdurakhmonov, 2007), and can be detect many natural allelic variations simultaneously in a single study (Myles et al., 2009; Lipka et al., 2015). There has been wide use of AM in QTL mapping for important agricultural traits of cotton (Abdurakhmonov et al., 2009; Mei et al., 2013; Cai et al., 2014; Saeed et al., 2014; Zhao et al., 2014; Li et al., 2016; Nie et al., 2016). However, the markers used in AM did not cover the whole cotton genome, due to low marker density. Recent advances in high-throughput sequencing technologies have enabled rapid and accurate resequencing of a large number of genomes (Chia et al., 2012; Huang et al., 2013; Mace et al., 2013; Aflitos et al., 2014). Recently, genome-wide association study (GWAS) based on high-throughput markers have become a higher-resolution and more cost-effective tool for detecting important QTLs or genes associated with complex traits, and have greatly improved resolution and accuracy of genetic mapping (Wang et al., 2011). Application of GWAS to numerous crop species enabled the detection of many QTLs associated with agronomic traits through a large number of single nucleotide polymorphisms (SNPs) (Li et al., 2013, 2017; Lin et al., 2014; Wen et al., 2014; Zhang J. et al., 2015). In cotton, the upland cotton TM-1 genome sequence was released (Li et al., 2015; Zhang T. et al., 2015); and genomic assessment based on the specific-locus amplified fragment sequencing and genome-wide resequencing have provided opportunities to identify required marker coverage in upland cotton (Su et al., 2016a,b; Fang L. et al., 2017; Ma et al., 2018; Su et al., 2018). The specific-locus amplified fragment sequencing and the resequencing can reveal unknown sequence information, but experimental operation and data analysis are complex; comparatively, array-based SNP detection can be used to genotype many samples within a short period and data analysis is relatively easy (Chen et al., 2014). Two commercial high-density cotton SNP arrays were recently designed and used for GWAS analysis (Hulse-Kemp et al., 2015; Cai et al., 2017; Sun et al., 2017; Li et al., 2018; Liu et al., 2018). To better understand the genetic variation of fiber quality at a natural population level, a diverse panel consisting of 408 upland cotton accessions was genotyped by high-density CottonSNP80K array, which was the latest array developed by Nanjing Agricultural University (Cai et al., 2017). The fiber quality traits were measured in six different environments. A GWAS was performed to identify SNP loci or QTL regions associated with fiber quality traits. The candidate genes controlling fiber quality were further predicted by RNA-Seq analysis. These results will lay the foundation for uncovering the genetic variations of complex traits and for fiber quality improvement through breeding by molecular design and genomic selection.
In this study, a total of 408 upland cotton accessions were selected, comprising 201 accessions that originated from the Northwestern Inland Region (NIR), 88 that originated from the Yellow River region (YRR), 63 from the Yangtze River Region (YtRR), 29 from the northern specific early maturation region (NSEMR) and 27 introduced from abroad (Supplementary Table S1). All 408 accessions were planted at Shihezi (SHZ) in North Xinjiang (85.94°E, 44.27°N) and at Korla (KRL) in South Xinjiang (86.06°E, 41.68°N) for 2013, 2014, and 2015, denoted SHZ13, KRL13, SHZ14, KRL14, SHZ15, and KRL15, respectively. The field experiments were designed so that each accession was grown in a plot with 40–45 plants in two rows, with 0.10 m between plants in rows and 0.45 m between rows. The field experiments used a randomized complete block design with three replications in each environment. The field management conformed to local practices.
The fiber quality traits were measured by the HFT9000 Automatic Fiber Determination System (Premier Inc, Coimbatore, India) at the Key Laboratory of China Northwestern Inland Region, Ministry of Agriculture, China. Fiber quality traits were the upper half mean fiber length (FL, mm), fiber strength (FS, cN/tex), fiber micronaire (FM), fiber uniformity (FU, %) and fiber elongation (FE, %). Software SPSS 19.0 was used to calculate basic statistics and Pearson correlations between traits. Analysis of variance (ANOVA) and broad-sense heritability (h2 B) were calculated using Statistical Analysis System (SAS8.0, SAS Institute 1999). The best linear unbiased prediction (BLUP) values for the five fiber quality across the six environments were estimated using the R program, and were used for subsequent GWAS.
Total DNA was extracted from young leaves of each accession as described by Paterson et al. (1993). A genome-wide CottonSNP80K genotyping array containing 77,774 SNPs (Cai et al., 2017) was applied to genotype the 408 accessions using the Illumina Infinium platform according to the manufacturer’s protocol (Illumina Inc., San Diego, CA, United States). All SNP genotype data were treated with raw data normalization, clustering and genotype calling by Illumina Genome Studio Genotyping Module (Illumina). The SNPs with a minor allele frequency (MAF) < 0.05 and missing rates ≥ 0.30 were removed to avoid problems of spurious LD and false positive associations. Finally, 48,072 high-quality SNPs were used for GWAS analysis (Supplementary Table S2).
The STRUCTURE version 2.2 (Pritchard et al., 2000) was used to assess the population structure among 408 accessions, based on Bayesian MCMC set to 10,000, burn-in set to a running time of 100,000 and K-value set to 1–9, with the rest of the parameters using software default settings. The most likely number of clusters (K) was selected by comparing the logarithmic probabilities of LnP(D) and ΔK data as previously described by Evanno et al. (2005). Nei’s genetic distances among the 408 accessions were calculated with Phylip software (version 3.69) (Felsenstein, 1989), and a phylogenetic tree was constructed with Dendroscope software (Huson et al., 2007). Principal component analysis (PCA) using EIGENSTRAT software (Price et al., 2006) was used to further verify population structure. The LD parameter (r2) between linked SNPs was estimated using Haploview software (Barrett et al., 2005). The core parameters were set as follows: mingeno 0.6, minmaf 0.05, and hwcutoff 0.001. The LD decay rate was measured as the chromosomal distance at which the average pairwise correlation coefficient dropped to half of its maximum value (Huang et al., 2010). The LD decay map was drawn using the R program.
The BLUPs for the six environments and the phenotypic values of five fiber quality traits for each environment were used in GWAS with the Efficient Mixed-Model Association Expedited program (EMMAx), the threshold adopted was the Bonferroni P ≤ 1/n [1/48,072 = 2.08 × 10-5, -log10(P) ≥ 4.68] (Cai et al., 2017; Sun et al., 2017) to determine significant associations, where n is the number of SNP markers used in the association analysis. Manhattan plots were performed using the R software package “qqman”. For the significant marker–trait associations, elite haplotypes were identified according to the breeding objectives of the target trait. The formula for calculating phenotypic effect (ai) of alleles was described by Zhang et al. (2013). For FL, FS, FU and FE, ai > 0 indicated an elite allele; and for FM, ai < 0 indicated an elite allele. Box plots for phenotypic differences of traits among different haplotypes were constructed using SPSS19.0.
According to the GWAS-associated SNP markers, candidate genes were identified within 500 kb upstream and downstream of peak SNPs. Gene functions were predicted based on the expression profiling data in different TM-1 tissues (the SRA database: PRJNA248163; Zhang T. et al., 2015): root, stem, leaf, calycle, fiber-5 DPA (fiber at 5 days post-anthesis), fiber-10 DPA, fiber-20 DPA and fiber-25 DPA1. Normalized fragments per kilobase of transcript per million fragments mapped (FPKM) values were calculated to predict the expression levels of these candidate genes. The calculation method for FPKM was as follows: FPKM = cDNA fragments/Mapped Reads (Millions) × Transcript Length (Kb). Further gene annotations were performed from by gene ontology (GO) items on the cotton website2.
Fiber quality performance in six environments and the BLUPs in the 408 accessions are presented in Supplementary Table S3 and Supplementary Figure S1. The FL, FS, FM, FU, and FE were in the ranges of 21.20–36.70 mm, 23.70–43.60 cN/tex, 2.60–6.00, 78.90–88.40, and 5.90–7.60%, respectively. The ANOVA showed that the effects of genotype (G) and genotype × environment (G × E) interactions were significant (P < 0.01) on the five traits except for FU and FE (P < 0.05). The h2 B values for the five traits were in the range of 69.54–91.05%, with the maximum h2 B for FL. The correlations between the five fiber quality traits using the BLUP results showed that FL was significantly positively correlated with FS, FU, and FE, and a positive correlation was also found for FS with FU and FE. There were significant negative correlations of FM with FL and FS (Supplementary Table S4). These results demonstrated that cotton fiber quality traits were highly related to each other, and provide meaningful theoretical knowledge for cotton fiber quality breeding.
The 408 cotton accessions were genotyped using the CottonSNP80K Illumina Infinium SNP array at Beijing Compass Biotechnology Co. Ltd. (Beijing, China). After removing MAF < 0.05 and missing rates ≥ 0.30, a total of 48,072 SNPs of high genotyping quality (48,072/77,774, 61.81%) were used for subsequent GWAS (Supplementary Table S5 and Figure 1A). The 48,072 SNPs covered all 26 chromosomes, with 25,444 and 22,628 SNPs in the A and D subgenomes, respectively. The highest number of SNPs was identified on chromosome A08 (3408 SNPs) and the least on chromosome D04 (910 SNPs). The average SNP density was approximately one SNP per 42.88 kb, with the highest density on chromosome D07 and the least on chromosome A02 (one SNP per 69.31 and 22.89 kb, respectively). For these SNPs, 84.55% of the adjacent SNP distances were < 50 kb and only 0.92% were > 500 kb (Figure 1B).
Figure 1. Description of SNPs and genetic diversity of 408 cotton accessions. (A) Genetic diversity of 408 cotton genomes. The serial numbers of 26 chromosomes are represented by different colors. From the outer to the inner circle, the curves depict SNP density (the number of SNPs per 100-kb window) and the level of genetic differentiation (the FST values per 100-kb window) between G1 and G2, respectively. (B) Proportion of the 48,072 SNPs categorized by the adjacent SNP distances. “d” represents the distance between two adjacent SNPs.
Evaluation of the population structure of the 408 cotton accessions by STRUCTURE showed that the LnP(D) value corresponding to each putative K kept increasing with K-value and did not show an inflection point (Figure 2A). The ΔK value showed a maximum likelihood at K = 2 (Figure 2B), suggesting that the 408 cotton accessions could be divided into two major subgroups (Supplementary Table S6 and Figure 2C): G1 and G2. Each subgroup was composed of accessions from different ecological zones, and was unrelated to geographical distribution (Figure 2D). Furthermore, phylogenetic analysis (Figure 2E) and PCA (Figure 2F) also agreed well with the clustering results in STRUCTURE. Moreover, the genetic diversity across the 26 chromosomes (Figure 1A and Supplementary Table S7), shown by FST values of the two subgroups of 0.094, indicated a low level of subpopulation differentiation or a low level of genetic diversity during cotton domestication. The decay of LD with physical distance between SNPs was approximately 0.50 Mb, with r2 = 0.03 at half of the maximum value (Supplementary Figure S2).
Figure 2. Analysis of population structure of 408 upland cotton accessions. (A) Mean LnP(D) values plotted as the number of subgroups; (B) ΔK values plotted as the number of subgroups; (C) population structure based on STRUCTURE when K = 2; (D) distribution of accessions in different cotton regions of two subgroups. Red and green represent G1 and G2, respectively; (E) NJ tree based on Nei’s genetic distances; (F) principal component analysis.
Based on the genotypic data of 48,072 high-quality SNPs, phenotypic mean values of different environments and the BLUPs, the GWAS was performed to identify the associated loci in 408 cotton accessions. A total of 90 significant SNPs were identified for the five fiber quality traits in SHZ and KRL for 2013–2015 (Supplementary Table S8). The numbers of loci associated with FL, FS, FM, FU, and FE were 29, 17, 8, 22, and 14, respectively. To select major loci among all significant SNPs, the SNPs with the lowest P-values (the highest log10P) were maintained within a 1.0-Mb window according to the average LD decay rate in the panel. Finally, 30 significant SNPs were identified for these five traits in different environments (Supplementary Table S8). For FL, six peak SNPs were distributed on chromosomes A05, A07, A13, and D11, and 93 candidate genes were predicted within 1.0 Mb (±500 kb of the peak SNP) (Supplementary Table S8 and Supplementary Figure S3). For FS, seven peak SNPs were distributed on chromosomes A04, A07, A13, D11 and D13, and 129 candidate genes predicted within 1.0 Mb (Supplementary Table S8 and Supplementary Figure S4). For FM, three peak SNPs were distributed on chromosomes A12, D03 and D11, and 38 candidate genes predicted within 1.0 Mb (Supplementary Table S8 and Supplementary Figure S5). For FU, six peak SNPs were distributed on chromosomes A07, A08, A09, D03, and D13, and 108 candidate genes predicted within 1.0 Mb (Supplementary Table S8 and Supplementary Figure S6). For FE, eight peak SNPs were distributed on chromosomes A01, A07, A09, A11, A13, and D09, and 90 candidate genes predicted within 1.0 Mb (Supplementary Table S8 and Supplementary Figure S7). We also found that more candidate genes for fiber quality were predicted in the A (302) than the D subgenome (156). Moreover, 23 significant SNPs were detected across the BLUPs, and 164 candidate genes were predicted in the CottonSNP80K array (Supplementary Table S9). Most importantly, seven SNPs were the same loci for 30 significant SNPs and 23 SNPs across the BLUPs (Figure 3), and 128 candidate genes were predicted within 1.0 Mb (Table 1 and Supplementary Tables S10, S11). Furthermore, we observed that the expression of 91 genes of them were expressed [log2(1 + FPKM) > 1] in the fiber development stages (5, 10, 20, and 25 dpa) using RNA-Seq. Gene ontology (GO) analysis indicated that the functions of most genes were binding and enzymatic activity belong to molecular functions category. This information provides a convenient way to rapidly identify candidate genes associated with fiber quality.
Figure 3. GWAS of the FL (A), FS (B), FM (C), FU (D), and FE (E) in the BLUPs using EMMAX. The horizontal line indicates the threshold (4.68). Seven SNPs (the same loci between 30 significant SNPs and 23 SNPs across the BLUPs) are indicated by the red arrow in the Manhattan plots.
Table 1. Summary of seven significant peak SNPs associated with fiber quality traits and candidate genes within 500 kb either side of the SNP locus.
Based on the seven significant peak SNPs, each SNP locus could be classified into three alleles; according to cotton quality breeding objectives, for FL, FS, FU, and FE, the alleles with positive effects are elite alleles. For FM, the alleles with negative effects were elite alleles. The effects of elite alleles for the five traits directly related to fiber quality are summarized in Table 2. These findings indicated that the phenotypic characteristics with elite alleles displayed a significantly average values than the other non-included elite alleles (Supplementary Figure S8), and further confirmed that there were major QTLs controlling fiber quality in the regions adjacent to the seven associated SNP loci. In addition, three SNP loci (TM21110, TM21111, and TM47488) were associated with FL, and six SNP loci (TM21110, TM21111, TM21292, TM47488, TM77174, and TM81816) were associated with FS, without taking into account the effect of interactions among these loci, with the accumulation of more elite alleles in accessions, with the larger average phenotypic value significant increased (Figure 4). These results indicated that the genetic control of fiber quality generally had additive effects in cotton. Consequently, increasing the frequency of elite alleles would significantly improve cotton fiber quality.
Figure 4. Build-up effect analysis for different numbers of elite alleles. (A) FL, fiber length; (B) FS, fiber strength. The X-axis represents the number of elite alleles carried by the accessions and the Y-axis represents trait mean value. Different lowercase letters above the plots represent Duncan’s multiple comparison at P < 0.05.
Gene linkage and pleiotropy are common phenomena in crop breeding. In this study, out of the seven significant peak SNPs, three showed signal linkage or pleiotropy associated with multiple traits in the six environments. In particular, two major genome regions including 11 and five loci (GR1 and GR2) on chromosomes A07 and A13 were associated with multiple fiber qualities in multiple environments (Figure 5). The TM21110 and TM21111 loci on chromosome A07 were associated with both FL and FS in KRL13, KRL14 and the BLUPs. Moreover, the correlation between FL and FS (r = 0.848, P < 0.01) was significant in the BLUPs (Supplementary Table S4). The TM47488 locus on chromosome A13 was closely related to FL, FS and FE in SHZ13, SHZ14, KRL13, KRL14 and the BLUPs. These associated loci may explain the correlations among fiber qualities: between FL and FS (r = 0.848, P < 0.01), FL and FE (r = 0.829, P < 0.01) and FS and FE (r = 0.748, P < 0.01) in the BLUPs (Supplementary Table S4).
Figure 5. Physical map of SSR markers identified by electronic PCR based on the reference genome sequence (Zhang T. et al., 2015). The SSR markers linked to fiber quality QTLs from previous studies. The unit of physical distance for the chromosomes is Mb; gray columnar graph represents SSR markers or QTLs from previous studies in the region adjacent to the associated SNP loci identified in this study. (A) The first major genome region (GR1) on chromosome A07; (B) The second major genome region (GR2) on chromosome A13.
The first major genome region of 1.70 Mb (GR1, within 70,365,245–72,067,994 bp) on chromosome A07 including 11 loci (TM21097, TM21099, TM21108, TM21110, TM21111, TM21134, TM21135, TM21170, TM21256, TM21273, and TM21292) was associated with FL, FS, and FE in different environments and the BLUPs (Supplementary Table S8), An LD block analysis of the 11 loci showed that these markers had high levels of LD (Figure 5A). Using the CottonSNP80K array information, 16 candidate genes were annotated within the GR1, and their functional annotations are summarized in Table 3. RNA-Seq analysis from eight upland cotton TM-1 tissues (Zhang T. et al., 2015) of the 16 genes showed that six (Gh_A07G1723, Gh_A07G1727, Gh_A07G1731, Gh_A07G1742, Gh_A07G1751, and Gh_A07G1752) were expressed [log2 (1 + FPKM) > 1] in the fiber. Notably, Gh_A07G1723 and Gh_A07G1752 was preferentially expressed in fiber of 5 DPA, and Gh_A07G1731 was mainly expressed in fiber of 10 DPA (Table 3 and Supplementary Table S11). The second major genome region of 65.09 kb (GR2, within 74,977,977–75,043,070 bp) on chromosome A13, including five loci (TM47482, TM47488, TM47490, TM47491, and TM47492), were associated with FL, FS and FE in different environments and the BLUPs (Supplementary Table S8), An LD block analysis of the five loci showed that these markers had high levels of LD (Figure 5B). Use of the CottonSNP80K array information allowed six genes to be annotated within the GR2 region, and their functional annotations are summarized in Table 3. RNA-Seq analysis showed that of the five genes (Gh_A13G1614, Gh_A13G1615, Gh_A13G1616, Gh_A13G1619, and Gh_A13G1622) expressed [log2(1 + FPKM) > 1] in the fiber, which Gh_A13G1615 was mainly expressed in fiber of 20 DPA (Table 3 and Supplementary Table S11). Generally, these results suggested that the above candidate genes played important roles in different stages of fiber development.
Natural fiber products are favored by the majority of consumers because of moisture absorption, air permeability and warmth retention. Cotton fiber is the most important raw material in the textile industry. Its quality is directly related to the quality and grade of the textile products, and improving fiber quality has always been the primary objective of cotton breeding programs. In this study, a GWAS was performed based on a large natural population of upland cotton and the high-density CottonSNP80K array. First of all, five of the most important fiber quality traits of breeding were accurately measured in six different environments. Phenotypic trait analysis based on the BLUP results preferably eliminated environmental effects and improved the accuracy of predicting complex quantitative traits. This work provided accurate phenotypic data for association analysis. Furthermore, we conducted population structure analysis and LD estimation based on the high-density CottonSNP80K array. The panel was classified into two groups based on three different analysis methods (Figure 2). The clustering results showed no obvious correspondence with geographical distribution, similar to previous results (Wang et al., 2016; Sun et al., 2017). The FST values were calculated between G1 and G2, and were lower than in maize (Jiao et al., 2012) and hexaploid wheat (Cavanagh et al., 2013). These results supported the narrow diversity of upland cotton cultivars and that most were derived from the same ancestral strain (Fang L. et al., 2017). We also found that the approximate LD decay was 500 kb in this panel (Supplementary Figure S2), consistent with previous studies in which upland cotton had a long-range LD decay distance from ∼500 to ∼800 kb (Cai et al., 2017; Sun et al., 2017), which provided a good reference for further selecting of candidate genes. Eventually, we found that two major genome regions including 16 SNP loci were associated with multiple fiber qualities in multiple environments, and 22 candidate genes were annotated. This study provided numerous new genomic resources for further understanding the genetic basis of fiber quality and so to improve upland cotton.
Presently, many QTL related to cotton fiber qualities have been mapped, and some fiber quality QTL hotspots have been discovered by a comparative meta-analysis (Said et al., 2015). Similarly, a few associated SNPs with fiber quality have been detected via GWAS in upland cotton (Sun et al., 2017; Li et al., 2018; Liu et al., 2018; Ma et al., 2018). In this study, We compared the GR1 and GR2 on chromosomes A07 and A13 detected in our GWAS (Figure 5) with SSRs and SNPs associated with QTLs for the fiber quality traits identified in previous studies by electronic PCR based on the reference genome sequence (Zhang T. et al., 2015). In the GR1 (Figure 5A), previous researchers found 10 QTLs (Chen et al., 2008; Lacape et al., 2010; Sun et al., 2012; Wang et al., 2013; Zhang et al., 2012; Fang X. et al., 2017) associated with fiber quality within or adjacent regions through linkage analysis and QTL mapping, Liu et al. (2018) detected 10 SNP loci associated with FS in the GR1 region by GWAS, and two SNP loci were the same loci with this study (TM21110 and TM21135), Sun et al. (2017) and Ma et al. (2018) have identified a number of cluster_A07 SNPs for FS are distributed in genome region A07: 71.99–72.25 Mbp, There was partial overlap with GR1 (70.37–72.07 Mbp) identified in this study. These results further indicated that the GR1 is an important hotspot controlling fiber quality. In the GR2 (Figure 5B), previous researchers also found two QTLs (Lacape et al., 2010; Wang et al., 2011) associated with fiber quality in the adjacent regions through linkage analysis, Fang L. et al. (2017) detected three SNP loci associated with fiber quality in the GR2 adjacent region by GWAS, these associated QTLs or SNPs were excluded in the GR2 of the previous reports. Therefore, the GR2 may be novel hotspot controlling fiber quality.
Cotton fiber development is a complex process involving initiation, elongation (primary cell wall synthesis), secondary cell wall synthesis and maturation (Kim and Triplett, 2001). Previous research indicated that many genes regulate different fiber developmental phases. In our study, 22 candidate genes in GR1 and GR2 on chromosomes A07 and A13 were annotated, and 11 genes showed higher expression in different periods of fiber development (Table 3). For example, gene Gh_A07G1723 in GR1 belonged to the Arabidopsis heat shock protein 90-like, and this protein serves as a molecular chaperone with important roles in plant cellular signal transduction pathway and influences plant growth and development (Ishiguro et al., 2002; Sangster et al., 2007). Interestingly, of four genes (Gh_A07G1748, Gh_A07G1749, Gh_A07G1750, and Gh_A07G1751) reported in GR1 of upland cotton, one is engaged in mediating a combination of cell elongation and SCW biosynthesis during cotton fiber development (Fang X. et al., 2017). In addition, two genes (Gh_A13G1606 and Gh_A13G1624) in GR2 neighboring regions had higher expression levels for fiber-20 DPA and fiber-5 DPA than in other tissues (Supplementary Figure S9), Gh_A13G1606 on chromosome A13, a homologous of AT4G32330 locus for protein WAVE-DAMPENED 2-like 6 (WVD2) in Arabidopsis, was identified as an activation-tagged allele that can cause plant organ stockiness, which is critical for the organization of plant microtubules (Perrin et al., 2007). The Gh_A13G1624, a homolog of Arabidopsis At4g25140, belongs to the S12E family of ribosomal proteins and plays an important role in intracellular protein biosynthesis (Maguire and Zimmermann, 2001; Khairulina et al., 2010). However, the biological validation of these candidate genes in developing cotton fibers needs further research.
In this study, based on a high-density CottonSNP80K array, a total of 30 and 23 significant SNPs associated with five fiber quality traits were identified across the 408 cotton accessions in six environments and the BLUP, respectively. Among these SNPs, seven loci were the same, and 128 candidate genes were predicted in a 1-Mb region. Most importantly, two major genome regions (GR1 and GR2) associated with multiple fiber qualities in multiple environments on chromosomes A07 and A13 were identified, and 22 candidate genes were annotated. This study provides fundamental insight relevant to identification of genes associated with fiber quality and will accelerate future efforts toward improving fiber quality of upland cotton.
TZ and HC designed and supervised the research. CD, JW, YY, XZ, XM, and BL conducted the field trials to evaluate the traits. CD, JW, LJ, GM, ZH, and ZS performed the data analysis. CD and JW wrote the manuscript. All of the authors read and approved the manuscript.
This research was funded by The Science Technology and Achievement Transformation Project of The Xinjiang Production and Construction Corps (2016AC002) and The National Natural Science Foundation of China (31260340).
The authors declare that the research was conducted in the absence of any commercial or financial relationships that could be construed as a potential conflict of interest.
The Supplementary Material for this article can be found online at: https://www.frontiersin.org/articles/10.3389/fpls.2018.01968/full#supplementary-material
FIGURE S1 | Frequency distributions based on the BLUPs of the five fiber quality traits in six environments. (A) fiber length (FL); (B) fiber strength (FS); (C) fiber micronaire value (FM); (D) fiber uniformity (FU); and (E) fiber elongation (FE).
FIGURE S2 | Average LD decay estimated in 408 cotton accessions.
FIGURE S3 | Manhattan plots showing the GWAS for FL in six environments. The horizontal line indicates the threshold (–log10P > 4.68).
FIGURE S4 | Manhattan plots showing the GWAS for FS in six environments. The horizontal line indicates the threshold (–log10P > 4.68).
FIGURE S5 | Manhattan plots showing the GWAS for FM in six environments. The horizontal line indicates the threshold (–log10P > 4.68).
FIGURE S6 | Manhattan plots showing the GWAS for FU in six environments. The horizontal line indicates the threshold (–log10P > 4.68).
FIGURE S7 | Manhattan plots showing the GWAS for FE in six environments. The horizontal line indicates the threshold (–log10P > 4.68).
FIGURE S8 | Boxplots depicting the genetic effects of SNPs with significant associations with fiber quality traits. (A) Boxplot diagram depicting the genetic effect of three significant SNPs to FL. (B) Boxplot diagram depicting the genetic effect of six significant SNPs to FS. (C) Boxplot diagram depicting the genetic effect of one significant SNP to FM. (D) Boxplot diagram depicting the genetic effect of one significant SNP to FE. The box shows the lower quartile, median and upper quartile values, and the whiskers show the range of phenotypic variation in the population.
FIGURE S9 | Expression levels of two genes associated with fiber quality traits. (A) Gh_A13G1606, (B) Gh_A13G1624.
TABLE S1 | The ecological origins information of the 408 Upland cotton accessions.
TABLE S2 | The Genotyping data of 408 materials from high-density array.
TABLE S3 | Descriptive statistics and ANOVA for five fiber quality traits.
TABLE S4 | Correlation analysis of five fiber quality traits.
TABLE S5 | Genome-wide SNP distributions on the 26 chromosomes of the 408 upland cotton accessions.
TABLE S6 | Subpopulation arrangement of the 403 upland cotton accessions.
TABLE S7 | Levels of genetic differentiation on the 26 chromosomes.
TABLE S8 | The summary of significant SNPs and candidate genes for fiber quality traits in six environments.
TABLE S9 | The summary of significant SNPs and candidate genes for fiber quality traits in the BLUPs.
TABLE S10 | Hundred and twenty eight candidate genes with fiber quality traits.
TABLE S11 | Transcriptome sequencing data of 128 candidate genes from 8 upland cotton (TM-1) tissues.
Abdurakhmonov, I. Y. (2007). “Exploiting genetic diversity,” in Proceedings of the World Cotton Research Conference -4, ed. D. Ethridge (Lubbock, TX: El Paso Times).
Abdurakhmonov, I. Y., Saha, S., Jenkins, J. N., Buriev, Z. T., Shermatov, S. E., Scheffler, B. E., et al. (2009). Linkage disequilibrium based association mapping of fiber quality traits in G. hirsutum L. variety germplasm. Genetica 136, 401–417. doi: 10.1007/s10709-008-9337-8
Aflitos, S., Schijlen, E., de Jong, H., de Ridder, D., Smit, S., Finkers, R., et al. (2014). Exploring genetic variation in the tomato (Solanum section Lycopersicon) clade by whole-genome sequencing. Plant J. 80, 136–148. doi: 10.1111/tpj.12616
Barrett, J. C., Fry, B., Maller, J., and Daly, M. J. (2005). Haploview: analysis and visualization of LD and haplotype maps. Bioinformatics 21, 263–265. doi: 10.1093/bioinformatics/bth457
Cai, C., Ye, W., Zhang, T., and Guo, W. (2014). Association analysis of fiber quality traits and exploration of elite alleles in upland cotton cultivars/accessions (Gossypium hirsutum L.). J. Integr. Plant Biol. 56, 51–62. doi: 10.1111/jipb.12124
Cai, C., Zhu, G., Zhang, T., and Guo, W. (2017). High-density 80K SNP array is a powerful tool for genotyping G. hirsutum accessions and genome analysis. BMC Genomics 18:654. doi: 10.1186/s12864-017-4062-2
Cavanagh, C. R., Chao, S., Wang, S., Huang, B., Stephen, S., Kiani, S., et al. (2013). Genome-wide comparative diversity uncovers multiple targets of selection for improvement in hexaploid wheat landraces and cultivars. Proc. Natl. Acad. Sci. U.S.A. 110, 8057–8062. doi: 10.1073/pnas.1217133110
Chen, H., Xie, W., He, H., Yu, H., Chen, W., Li, J., et al. (2014). A high-density SNP genotyping array for rice biology and molecular breeding. Mol. Plant 7, 541–553. doi: 10.1093/mp/sst135
Chen, L., Zhang, Z., Hu, M., Wang, W., Zhang, J., Liu, D., et al. (2008). Genetic linkage map construction and QTL mapping for yield and fiber quality in upland cotton (Gossypium hirsutum L.). Acta Agronom. Sin. 34, 1199–1205. doi: 10.3724/SP.J.1006.2008.01199
Chia, J. M., Song, C., Bradbury, P. J., Costich, D., de Leon, N., Doebley, J., et al. (2012). Maize HapMap2 identifies extant variation from a genome in flux. Nat. Genet. 44, 803–807. doi: 10.1038/ng.2313
Evanno, G., Regnauts, S., and Goudet, J. (2005). Detecting the number of clusters of individuals using the software STRUCTURE: a simulation study. Mol. Ecol. 14, 2611–2620. doi: 10.1111/j.1365-294X.2005.02553.x
Fang, L., Wang, Q., Hu, Y., Jia, Y., Chen, J., Liu, B., et al. (2017). Genomic analyses in cotton identify signatures of selection and loci associated with fiber quality and yield traits. Nat. Genet. 49, 1089–1098. doi: 10.1038/ng.3887
Fang, X., Liu, X., Wang, X., Wang, W., Liu, D., Zhang, J., et al. (2017). Fine-mapping qFS07.1 controlling fiber strength in upland cotton (Gossypium hirsutum L.) Theor. Appl. Genet. 130, 795–806. doi: 10.1007/s00122-017-2852-1
Huang, X., Lu, T., and Han, B. (2013). Resequencing rice genomes: an emerging new era of rice genomics. Trends Genet. 29, 225–232. doi: 10.1016/j.tig.2012.12.001
Huang, X., Wei, X., Sang, T., Zhao, Q., Feng, Q., Zhao, Y., et al. (2010). Genome-wide association studies of 14 agronomic traits in rice landraces. Nat. Genet. 42, 961–967. doi: 10.1038/ng.695
Hulse-Kemp, A. M., Lemm, J., Plieske, J., Ashrafi, H., Buyyarapu, R., Fang, D. D., et al. (2015). Development of a 63K SNP array for cotton and high-density mapping of intraspecific and interspecific populations of Gossypium spp. G3 5, 1187–1209. doi: 10.1534/g3.115.018416
Huson, D. H., Richter, D. C., Rausch, C., Dezulian, T., Franz, M., and Rupp, R. (2007). Dendroscope: an interactive viewer for large phylogenetic trees. BMC Bioinformatics 8:460. doi: 10.1186/1471-2105-8-460
Ishiguro, S., Watanabe, Y., Ito, N., Nonaka, H., Takeda, N., Sakai, T., et al. (2002). SHEPHRD is the Arabidopsis Grp94 responsible for the formation of functional CLAVATA proteins. EMBO J. 21, 898–908. doi: 10.1093/emboj/21.5.898
Jiao, Y., Zhao, H., Ren, L., Song, W., Zeng, B., Guo, J., et al. (2012). Genome-wide genetic changes during modern breeding of maize. Nat. Genet. 44, 812–815. doi: 10.1038/ng.2312
Khairulina, J., Graifer, D., Bulygin, K., Ven’yaminovam, A., Frolova, L., and Karpova, G. (2010). Eukaryote-specific motif of ribosomal protein S15 neighbors A site codon during elongation and termination of translation. Biochimie 92, 820–825. doi: 10.1016/j.biochi.2010.02.031
Kim, H. J., and Triplett, B. A. (2001). Cotton fiber growth in planta and in vitro. Models for plant cell elongation and cell wall biogenesis. Plant Physiol. 127, 1361–1366. doi: 10.1104/pp.010724
Kohel, R. J., Yu, J., Park, Y. H., and Lazo, G. R. (2001). Molecular mapping and characterization of traits controlling fiber quality in cotton. Euphytica 121, 163–172. doi: 10.1023/A:1012263413418
Lacape, J. M., Llewellyn, D., Jacobs, J., Arioli, T., Becker, D., Calhoun, S., et al. (2010). Meta-analysis of cotton fiber quality QTLs across diverse environments in a Gossypium hirsutum×G. barbadense RIL population. BMC Plant Biol. 10:132. doi: 10.1186/1471-2229-10-132
Li, C., Ai, N., Zhu, Y., Wang, Y., Chen, X., Li, F., et al. (2016). Association mapping and favourable allele exploration for plant architecture traits in upland cotton (Gossypium hirsutum L.) accessions. J. Agric. Sci. 154, 567–583. doi: 10.1017/S0021859615000428
Li, C., Fu, Y., Sun, R., Wang, Y., and Wang, Q. (2018). Single-locus and multi-locus genome-wide association studies in the genetic dissection of fiber quality traits in upland cotton (Gossypium hirsutum L.). Front. Plant Sci. 9:1083. doi: 10.3389/fpls.2018.01083
Li, F., Fan, G., Lu, C., Xiao, G., Zou, C., Kohel, R. J., et al. (2015). Genome sequence of cultivated upland cotton (Gossypium hirsutumTM-1) provides insights into genome evolution. Nat. Biotech. 33, 524–530. doi: 10.1038/nbt.3208
Li, G., Bai, G., Carver, B. F., Elliott, N. C., Bennett, R. S., Wu, Y., et al. (2017). Genome-wide association study reveals genetic architecture of coleoptile length in wheat. Theor. Appl. Genet. 130, 391–401. doi: 10.1007/s00122-016-2820-1
Li, H., Peng, Z., Yang, X., Wang, W., Fu, J., Wang, J., et al. (2013). Genome-wide association study dissects the genetic architecture of oil biosynthesis in maize kernels. Nat. Genet. 45, 43–50. doi: 10.1038/ng.2484
Lin, T., Zhu, G., Zhang, J., Xu, X., Yu, Q., Zheng, Z., et al. (2014). Genomic analyses provide insights into the history of tomato breeding. Nat. Genet. 46, 1220–1226. doi: 10.1038/ng.3117
Lipka, A. E., Kandianis, C. B., Hudson, M. E., Yu, J., Drnevich, J., Bradbury, P. J., et al. (2015). From association to prediction: statistical methods for the dissection and selection of complex traits in plants. Curr. Opin. Plant Biol. 24, 110–118. doi: 10.1016/j.pbi.2015.02.010
Liu, R., Gong, J., Xiao, X., Zhang, Z., Li, J., Liu, A., et al. (2018). GWAS analysis and QTL identification of fiber quality traits and yield components in upland cotton using enriched high-density SNP markers. Front. Plant Sci. 9:1067. doi: 10.3389/fpls.2018.01067
Ma, Z., He, S., Wang, X., Sun, J., Zhang, Y., Zhang, G., et al. (2018). Resequencing a core collection of upland cotton identifies genomic variation and loci influencing fiber quality and yield. Nat. Genet. 50, 803–813. doi: 10.1038/s41588-018-0119-7
Mace, E. S., Tai, S., Gilding, E. K., Li, Y., Prentis, P. J., Bian, L., et al. (2013). Whole-genome sequencing reveals untapped genetic potential in Africa’s indigenous cereal crop sorghum. Nat. Commun. 4:2320. doi: 10.1038/ncomms3320
Maguire, B. A., and Zimmermann, R. A. (2001). The ribosome in focus. Cell 104, 813–816. doi: 10.1016/S0092-8674(01)00278-1
Mei, H., Zhu, X., and Zhang, T. (2013). Favorable QTL alleles for yield and its components identified by association mapping in Chinese upland cotton cultivars. PLoS One 8:e82193. doi: 10.1371/journal.pone.0082193
Myles, S., Peiffer, J., Brown, P. J., Ersoz, E. S., Zhang, Z., Costich, D. E., et al. (2009). Association mapping: critical considerations shift from genotyping to experimental design. Plant Cell 21, 2194–2202. doi: 10.1105/tpc.109.068437
Nie, X., Huang, C., You, C., Li, W., Zhao, W. X., Shen, C., et al. (2016). Genome-wide SSR-based association mapping for fiber quality in nation-wide upland cotton inbreed cultivars in China. BMC Genomics 17:352. doi: 10.1186/s12864-016-2662-x
Paterson, A. H., Brubaker, C. L., and Wendel, J. F. (1993). A rapid method for extraction of cotton (Gossypium spp) genomic DNA suitable for RFLP or PCR analysis. Plan Mol. Biol. Rep. 11, 122–127. doi: 10.1007/BF02670470
Perrin, R. M., Wang, Y., Yuen, C. Y., Will, J., and Masson, P. H. (2007). WVD2 is a novel microtubule-associated protein in Arabidopsis thaliana. Plant J. 49, 961–971. doi: 10.1111/j.1365-313X.2006.03015.x
Price, A. L., Patterson, N. J., Plenge, R. M., Weinblatt, M. E., Shadick, N. A., and Reich, D. (2006). Principal components analysis corrects for stratification in genome-wide association studies. Nat. Genet. 38, 904–909. doi: 10.1038/ng1847
Pritchard, J. K., Stephens, M., and Donnelly, P. (2000). Inference of population structure using multilocus genotype data. Genetics 155, 945–959.
Rong, J., Feltus, F. A., Waghmare, V. N., Pierce, G. J., Chee, P. W., Draye, X., et al. (2007). Meta-analysis of polyploid cotton QTL shows unequal contributions of subgenomes to a complex network of genes and gene clusters implicated in lint fiber development. Genetics 176, 2577–2588. doi: 10.1534/genetics.107.074518
Saeed, M., Guo, W., and Zhang, T. (2014). Association mapping for salinity tolerance in cotton (Gossypium hirsutum L.) germplasm from US and diverse regions of China. Aust. J. Crop Sci. 8, 338–346.
Said, J. I., Lin, Z., Zhang, X., Song, M., and Zhang, J. (2013). A comprehensive meta QTL analysis for fiber quality, yield, yield related and morphological traits, drought tolerance, and disease resistance in tetraploid cotton. BMC Genomics 14:776. doi: 10.1186/1471-2164-14-776
Said, J. I., Song, M., Wang, H., Lin, Z., Zhang, X., Fang, D. D., et al. (2015). A comparative meta-analysis of QTL between intraspecific Gossypium hirsutum and interspecific G. hirsutum × G. barbadens populations. Mol. Genet. Genomics 290, 1003–1025. doi: 10.1007/s00438-014-0963-9
Sangster, T. A., Bahrami, A., Wilczek, A., Watanabe, E., Schellenberg, K., Mclellan, C., et al. (2007). Phenotypic diversity and altered environmental plasticity in Arabidopsis thaliana with reduced Hsp90 levels. PLoS One 2:e648. doi: 10.1371/journal.pone.0000648
Su, J., Fan, S., Li, L., Wei, H., Wang, C., Wang, H., et al. (2016a). Detection of favorable QTL alleles and candidate genes for lint percentage by GWAS in Chinese upland cotton. Front. Plant Sci. 7:1576. doi: 10.3389/fpls.2016.01576
Su, J., Ma, Q., Li, M., Hao, F., and Wang, C. (2018). Multi-Locus genome-wide association studies of fiber-quality related traits in Chinese early-maturity upland cotton. Front. Plant Sci. 9:1169. doi: 10.3389/fpls.2018.01169
Su, J., Pang, C., Wei, H., Li, L., Liang, B., Wang, C., et al. (2016b). Identification of favorable SNP alleles and candidate genes for traits related to early maturity via GWAS in upland cotton. BMC Genomics 17:687. doi: 10.1186/s12864-016-2875-z
Sun, F., Zhang, J., Wang, S., Gong, W., Shi, Y., Liu, A., et al. (2012). QTL mapping for fiber quality traits across multiple generations and environments in upland cotton. Mol. Breed. 30, 569–582. doi: 10.1007/s11032-011-9645-z
Sun, Z., Wang, X., Liu, Z., Gu, Q., Zhang, Y., Li, Z., et al. (2017). Genome-wide association study discovered genetic variation and candidate genes of fibre quality traits in Gossypium hirsutum L. Plant Biotechnol. J. 15, 982–996. doi: 10.1111/pbi.12693
Wang, F., Xu, Z., Sun, R., Gong, Y., Liu, G., Zhang, J., et al. (2013). Genetic dissection of the introgressive genomic components from Gossypium barbadense L. that contribute to improved fiber quality in Gossypium hirsutum L. Mol Breed. 32, 547–562. doi: 10.1007/s11032-013-9888-y
Wang, L., Wang, A., Huang, X., Zhao, Q., Dong, G., Qian, Q., et al. (2011). Mapping 49 quantitative trait loci at high resolution through sequencing-based genotyping of rice recombinant inbred lines. Theor. Appl. Genet. 122, 327–340. doi: 10.1007/s00122-010-1449-8
Wang, Y., Zhou, Z., Wang, X., Cai, X., Li, X., Wang, C., et al. (2016). Genome-wide association mapping of glyphosate-resistance in Gossypium hirsutum races. Euphytica 209, 209–221. doi: 10.1007/s10681-016-1663-9
Wen, Z., Tan, R., Yuan, J. Z., Bales, C., Du, W., Zhang, S., et al. (2014). Genome-wide association mapping of quantitative resistance to sudden death syndrome in soybean. BMC Genomics 15:809. doi: 10.1186/1471-2164-15-809
Wendel, J. F., and Cronn, R. C. (2003). Polyploidy and the evolutionary history of cotton. Adv. Agron. 78, 139–186. doi: 10.1016/S0065-2113(02)78004-8
Zhang, J., Song, Q., Cregan, P. B., Nelson, R. L., Wang, X., Wu, J., et al. (2015). Genomewide association study for flowering time, maturity dates and plant height in early maturing soybean (Glycine max) germplasm. BMC Genomics 16:217. doi: 10.1186/s12864-015-1441-4
Zhang, K., Zhang, J., Ma, J., Tang, S., Liu, D., Teng, Z., et al. (2012). Genetic mapping and quantitative trait locus analysis of fiber quality traits using a three-parent composite population in upland cotton (Gossypium hirsutum L.). Mol. Breed. 29, 335–348. doi: 10.1007/s11032-011-9549-y
Zhang, T., Hu, Y., Jiang, W., Fang, L., Guan, X., Chen, J., et al. (2015). Sequencing of allotetraploid cotton (Gossypium hirsutum L. acc. TM-1) provides a resource for fiber improvement. Nat. Biotech. 33, 531–537. doi: 10.1038/nbt.3207
Zhang, T., Qian, N., Zhu, X., Chen, H., Wang, S., Mei, H., et al. (2013). Variations and transmission of QTL alleles for yield and fiber qualities in upland cotton cultivars developed in China. PLoS One 8:e57220. doi: 10.1371/journal.pone.0057220
Keywords: upland cotton, fiber quality, genome-wide association study, SNP genotyping array, candidate genes
Citation: Dong C, Wang J, Yu Y, Ju L, Zhou X, Ma X, Mei G, Han Z, Si Z, Li B, Chen H and Zhang T (2019) Identifying Functional Genes Influencing Gossypium hirsutum Fiber Quality. Front. Plant Sci. 9:1968. doi: 10.3389/fpls.2018.01968
Received: 30 July 2018; Accepted: 18 December 2018;
Published: 09 January 2019.
Edited by:
Nicolas Rispail, Spanish National Research Council (CSIC), SpainReviewed by:
Jun Zhang, Shandong Academy of Agricultural Sciences, ChinaCopyright © 2019 Dong, Wang, Yu, Ju, Zhou, Ma, Mei, Han, Si, Li, Chen and Zhang. This is an open-access article distributed under the terms of the Creative Commons Attribution License (CC BY). The use, distribution or reproduction in other forums is permitted, provided the original author(s) and the copyright owner(s) are credited and that the original publication in this journal is cited, in accordance with accepted academic practice. No use, distribution or reproduction is permitted which does not comply with these terms.
*Correspondence: Hong Chen, eGpjaGVuaDA3MjhAMTYzLmNvbQ== Tianzhen Zhang, Y290dG9uQHpqdS5lZHUuY24=
†These authors have contributed equally to this work
Disclaimer: All claims expressed in this article are solely those of the authors and do not necessarily represent those of their affiliated organizations, or those of the publisher, the editors and the reviewers. Any product that may be evaluated in this article or claim that may be made by its manufacturer is not guaranteed or endorsed by the publisher.
Research integrity at Frontiers
Learn more about the work of our research integrity team to safeguard the quality of each article we publish.