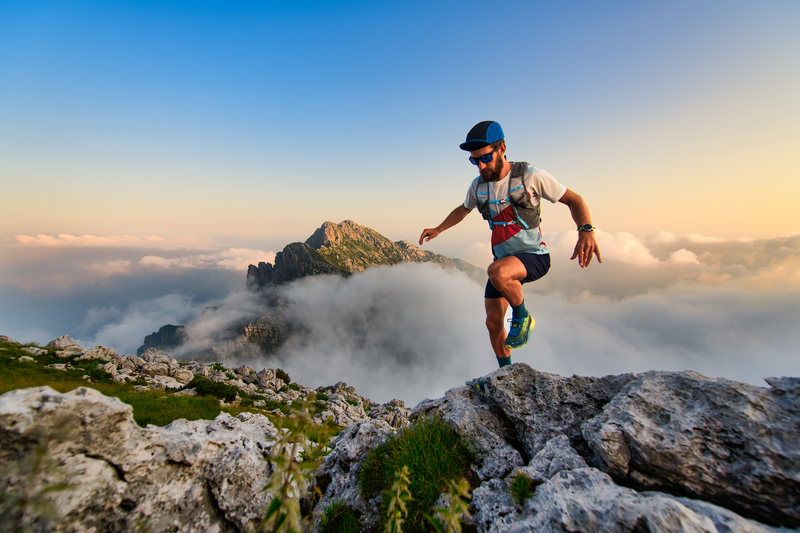
95% of researchers rate our articles as excellent or good
Learn more about the work of our research integrity team to safeguard the quality of each article we publish.
Find out more
ORIGINAL RESEARCH article
Front. Plant Sci. , 05 July 2018
Sec. Plant Breeding
Volume 9 - 2018 | https://doi.org/10.3389/fpls.2018.00895
Combinatorial insect attacks on maize leaves, stems, and kernels cause significant yield losses and mycotoxin contaminations. Several small effect quantitative trait loci (QTL) control maize resistance to stem borers and storage pests and are correlated with secondary metabolites. However, efficient use of QTL in molecular breeding requires a synthesis of the available resistance information. In this study, separate meta-analyses of QTL of maize response to stem borers and storage pests feeding on leaves, stems, and kernels along with maize cell wall constituents discovered in these tissues generated 24 leaf (LIR), 42 stem (SIR), and 20 kernel (KIR) insect resistance meta-QTL (MQTL) of a diverse genetic and geographical background. Most of these MQTL involved resistance to several insect species, therefore, generating a significant interest for multiple-insect resistance breeding. Some of the LIR MQTL such as LIR4, 17, and 22 involve resistance to European corn borer, sugarcane borer, and southwestern corn borer. Eleven out of the 42 SIR MQTL related to resistance to European corn borer and Mediterranean corn borer. There KIR MQTL, KIR3, 15, and 16 combined resistance to kernel damage by the maize weevil and the Mediterranean corn borer and could be used in breeding to reduce insect-related post-harvest grain yield loss and field to storage mycotoxin contamination. This meta-analysis corroborates the significant role played by cell wall constituents in maize resistance to insect since the majority of the MQTL contain QTL for members of the hydroxycinnamates group such as p-coumaric acid, ferulic acid, and other diferulates and derivates, and fiber components such as acid detergent fiber, neutral detergent fiber, and lignin. Stem insect resistance MQTL display several co-localization between fiber and hydroxycinnamate components corroborating the hypothesis of cross-linking between these components that provide mechanical resistance to insect attacks. Our results highlight the existence of combined-insect resistance genomic regions in maize and set the basis of multiple-pests resistance breeding.
Maize (Zea mays) is one of the most essential cultivated food crops worldwide (Kanyamasoro et al., 2012). However, maize production is adversely affected by insect pests (Meihls et al., 2012). Stem borers (SB), and field-to-storage pests are the most devastating on cultivated maize (Demissie et al., 2008; Shiferaw et al., 2011). In Africa, the spotted stem borer (SSB) (Chilo partellus), the African maize stem borer (AMSB) (Busseola fusca), the African pink stem borer (Sesamia calamistis), and the African sugarcane borer (Eldana saccharina) are the SB species attacking maize (Stevens, 2008). Regarding storage pests (SP), the maize weevil (MW) (Sitophilus zeamais), and the Larger grain borer (LGB) (Prostephanus truncatus) are the most challenging to maize storability (Mwololo et al., 2012). In East Africa including Uganda, stem borers, SSB, and AMSB, and storage pests, MW, and LGB are the most abundant insect pest species with SSB being the most competitive species that can displace any indigenous field insect pest within not more than 2 years (Samayoa et al., 2015b). These insect pests account for losses ranging from 20 to 90% starting from the field through to the grain storage period (Nyukuri et al., 2014), with both SB and SP being responsible for contamination of grain with mycotoxins like aflatoxin and fumonisins (Cao et al., 2014). These substantial yield losses and health concerns prompted the use of several control methods aimed at inhibiting insect pest attacks on both maize plants and grains. Chemical control methods (Sylvain et al., 2015) and transgenic resistance conferred by Bacillus thuringiensis (Bt) have limitations such as applicability (Munyiri et al., 2015) and acceptability, and some of the critical pests can develop resistance to both or either insecticides or Bt proteins (Campagne et al., 2013). Besides, environmental factors are a crucial element in plant defensive mechanisms (Stam et al., 2014), and climate change is predicted to negatively impact on plant-insect interaction leading to less fitness of plants coupled with aggravated yield losses (Kissoudis et al., 2014).
Host plant resistance (HPR) is the best integrated-pest management option (García-lara et al., 2010; Murenga et al., 2016) since in its highest level it can reduce plant yield loss from insect pest attacks without the use of controversial methods such as insecticides or transgenic resistance. HPR is the inherent resistance of a plant to biotic stresses conferred by its genetic makeup. Thus to achieve good HPR, the genetic basis of the resistance needs to be understood. Past studies established the polygenic nature of maize resistance to insect pests in general, and SB and SP resistance, in particular, were found to have low to moderate heritability values (Bergvinson, 1999; Kim and Kossou, 2003; Sandoya et al., 2010; Barros et al., 2011). Both significant general and specific combining abilities (GCA, SCA) govern maize resistance to SB (Udaykumar et al., 2013) and SP (Kim and Kossou, 2003; García-lara et al., 2009) implying the importance of both additive and non-additive gene actions coupled with a significant influence of genotype by environment interactions (André et al., 2003; Sandoya et al., 2010; Barros et al., 2011). The development of insect resistant maize lines through conventional means received considerable efforts. Over the years, the International Maize and Wheat Improvement Center (CIMMYT) developed several Africa adapted maize populations resistant to multiple SB or SP (Tefera et al., 2016). However, no report of combined-resistance to both SB and SP is yet available. The nature of inheritance characterizing maize resistance to SB ad SP makes conventional breeding for resistance a challenging task (Murenga et al., 2016). An alternative to this challenge is the use of DNA molecular marker-assisted selection (MAS) to fix resistance genes in susceptible backgrounds of agronomic interest (André et al., 2003).
Therefore, toward the application of MAS in maize breeding, several studies investigated the genomic regions controlling maize resistance to insect pests using family-based quantitative trait loci (QTL) analyses. These studies concerned SP species such as MW (García-lara et al., 2009; Mwololo, 2013; Castro-Álvarez et al., 2015) and LGB (Mwololo, 2013) and SB species such as the European corn borer (ECB) (Schön et al., 1993; Bohn et al., 2000; Cardinal et al., 2001, 2006; Jampatong et al., 2002; Krakowsky et al., 2002; Papst et al., 2004), the sugarcane borer (SCB) (Bohn et al., 1996, 1997; Groh et al., 1998), the Southwestern corn borer (SWCB) (Bohn et al., 1997; Groh et al., 1998; Khairallah et al., 1998; Brooks et al., 2005, 2007), the Mediterranean corn borer (MCB) (Ordas et al., 2009, 2010; Samayoa et al., 2014, 2015a; Jiménez-Galindo et al., 2017), and SSB and AMSB (Munyiri and Mugo, 2017). However, due to the polygenic nature of insect resistance in maize, these studies resulted in the discovery of a plethora of QTL with mainly low phenotypic effects. Furthermore, for MAS to be more efficient than phenotypic selection, several requirements are bound to the used QTL. These criteria pertain to the precision of the positions and the genotypic effects of the QTL, and the QTL explaining a sufficient portion of the genotypic variance, yet most of the QTL detected fall short of these prerequisites (Utz et al., 2000; Chen et al., 2017). Besides, some QTL go undetected due to their small size in the populations under consideration (Bohn et al., 1997). Therefore, a comparative analysis of the genomic regions responsible for maize resistance to insects of similar feeding behaviors across studies could help to better understand the genetics of maize resistance to insects through the reduction of the plethora of reported QTL, and also, to propose the most valuable QTLs to perform MAS (Jiang, 2013).
On the other hand, previous studies explored the biochemical basis of maize resistance to insects including SB and SP. Meihls et al. (2013) reported the concentration of insect resistance-related QTL in some bins such as at the top of chromosome 1, the bottom of chromosome 2, and on chromosome 7 and that only 10% of maize bins are known to be involved in some insect resistance (Meihls et al., 2012). Moreover, stem boring resistance QTL co-localize with several QTL of defense chemicals in 51 bins (Meihls et al., 2012). Cell wall components (CWC), especially fiber and hydroxycinnamates provide maize resistance to feeding by several stem borers (Cardinal and Lee, 2005; Krakowsky et al., 2007; Santiago et al., 2016). Fiber components such as acid detergent fiber (ADF), neutral detergent fiber (NDF), and lignin, and hydroxycinnamates such a p-coumaric acid (p-CA), ferulic acid (FA), and diferulic acid (DiFA), which are byproducts of the phenylpropanoids pathway, are involved in maize resistance to ECB, SCB, SWCB, and MCB both in leaves and stems (Santiago et al., 2013, 2017). Besides, the hydroxycinnamates and several other cell wall bound constituents are also associated with maize kernels resistance to MW (García-lara et al., 2010; Castro-Álvarez et al., 2015). Co-localizations of QTL for insect resistance and CWC have often been reported (Cardinal and Lee, 2005; Krakowsky et al., 2007; Santiago et al., 2016).
However, the accumulations and involvement of biochemical compounds in plants resistance to insects is complex and varies highly from one genotype to another, one plant tissue to another, and even the same tissue, from one developmental stage to another (Santiago et al., 2013). Therefore, for each maize tissue, a Meta-QTL analysis of QTL identified for maize resistance to insects and QTL for CWC would allow a better understanding of the genetic and biochemical basis of resistance. Meta-analysis of QTL is a means for refining the positions of QTL on a consensus map developed from the integration of individual maps or their projection on a reference map to accurately detect consensus QTL across studies, genetic backgrounds, and environments (Sosnoswki and Joets, 2012). It generates useful information for molecular breeding and cloning and presents an efficient way of investigating genetic correlation among traits (Wang et al., 2016). Furthermore, QTL meta-analysis helps in mitigating some of the weaknesses of individual QTL that hinder their efficiency in MAS. In the context of maize, this approach holds promise for the identification of MQTL across germplasms of various genetic and geographical backgrounds since the pan-genome theory implies that virtually all the lines share a significant portion of the genomic regions containing almost all the genes (Morgante et al., 2007). On that note, the ultimate goal of this study was to conduct a comparative mapping of maize resistance to SB and SP along with CWC QTL to identify tissue-specific resistance genomic regions for use in multiple insect pest resistance molecular breeding. To achieve this goal, we used the IBM2 2008 Neighbors (www.maizegdb.org) genetic linkage map which allows an increase in QTL resolution (Lee et al., 2002) as a reference first to conduct individual meta-analyses of QTL to identify tissue-specific meta-QTL (MQTL) for leaf, stem, and kernel resistance, and secondly, investigate combined resistance genomic regions.
We surveyed published QTL experiments on maize CWC and resistance to ECB, SCB, SWCB, MCB, MW, and on CWC on Google Scholar (https://scholar.google.com/), PubMed (https://www.ncbi.nlm.nih.gov/pubmed/), on the MaizeGDB Locus + QTL data center (http://www.maizegdb.org/data_center/locus), and the Gramene database QTL data center (http://archive.gramene.org/qtl/) (Table 1, Supplementary Material Presentation 1: Maps and QTL files). From all the experiments that were later considered for analysis, we either downloaded the maps from the MaizeGDB (http://www.maizegdb.org/data_center/locus) or, when not available, we generated them using the published maps (Supplementary Material Presentation 1: Maps and QTL files). When marker coordinates were unavailable, we used the Adobe reader distance measurement tool (https://helpx.adobe.com/acrobat/using/grids-guides-measurements-pdfs.html) to measure the intervals of the different markers on each chromosome for each map relative to the first marker positioned at the zero coordinate. Then, we used the provided scale to convert the distances from inches to centiMorgans (cM). However, due to non-availability of maps both from the online databases and the publications, we could not include some of the QTL experiments (Groh et al., 1998; Brooks et al., 2005, 2007). We also did not consider experiments for which the maps were built using single nucleotide polymorphism (SNP) markers (Orsini et al., 2012; Mwololo, 2013; Samayoa et al., 2015a; Munyiri and Mugo, 2017), because of a lack of shared markers with the other maps. Also, due to lack of similar markers with the consensus map, from the experiment by Méchin et al. (2001), we could only project chromosome 7 containing one QTL. For each of the maps, the information recorded included the population size and type, and the mapping function. Regarding the QTL data, parameters included were the QTL name, trait, LOD score of the QTL, the percentage of phenotypic variance explained by each QTL (R2), QTL most likely position and its confidence interval (CI) start and end. Some of the publications did not provide information on the R2 and the LOD scores (Khairallah et al., 1998; Fontaine et al., 2003; Papst et al., 2004; Samayoa et al., 2014). Where only the likelihood ratio statistics (LRS) was available (Papst et al., 2004), the LRS of each QTL was used to compute its LOD score using the formula: (Liu, 1997). Also, the individual LOD scores of the QTL were used to estimate R2 using the formula: R2 = 1−10(−2LOD/N), where N is the population size (Van and McHale, 2017). The CIs of the QTL were transformed at 95% using the following formulas: , where K = 530 for F2 and F3 populations, and K = 163 for recombinant inbred lines (RILs) and inter-mated RILs (IRILs) (Darvasi and Soller, 1997).
The data from each experiment was checked to reduce overlapped QTL by considering only the one with the highest R2 to avoid bias in the meta-analysis by over-representing the same QTL (Truntzler et al., 2010; Sosnoswki and Joets, 2012). The QTL experiments included in this analysis encompassed population from temperate (USA and Europe), sub-tropical and tropical regions. Moreover, each of the populations used for QTL mapping of maize response to the MW had at least one of the parental lines containing African pedigree (García-lara et al., 2009; Castro-Álvarez et al., 2015).
We loaded the different maps along with the QTL data (Supplementary Material Presentation 1: Maps and QTL files) in BioMercator 4.2 (Arcade et al., 2004) which integrates each QTL file with its corresponding map and check for common markers (at least 2) between each pair of maps included in the analysis to allow integration of the maps. However, maps displayed different sets of markers, and we could not compile them directly. Therefore, we used the high-density genetic linkage map of more than 1500 markers, the IBM2 2008 Neighbors (www.maizegdb.org, Supplementary Material Presentation 1: IBM2 2008 Neighbors) as a reference map and iteratively projected the experimental maps. The iterative map compilation tool implemented in BioMercator 4.2 allowed for the projection of QTL and loci from the individual genetic maps to the reference map. Common markers between homologous chromosomes were used to compute a specific ratio for each interval between pairs of shared markers, and a global ratio was implemented to project the remaining markers located above or below the first interval of shared markers and below the last interval of shared markers, respectively. In that process, the software automatically discarded inverted markers. Finally, BioMercartor used a homothetic function to project the QTL (Sosnoswki and Joets, 2012). We compiled the maps by starting with the maps showing the highest similarity with the reference map to avoid having some markers or QTL CIs spanning beyond the scope of the reference map and generating negative coordinates. When for a particular map, some markers or QTL still fell beyond the zero coordinate of the reference map, we discarded them from the original maps or QTL files, respectively (Jiang et al., 2016).
We conducted separate meta-analyses of damage resistance and CWC QTL discovered in each tissue attacked by SB and SP, namely leaves, stems, and kernels to identify tissue-specific MQTL we named leaf insect resistance (LIR), stem insect resistance (SIR) and kernel insect resistance (KIR). The QTL choice option of the BioMercator 4.2 (Arcade et al., 2004) was used in each of the analyses to select QTL reported for the tissue under consideration. For each meta-analysis, procedures followed two steps to determine the number of “real QTL” present on each chromosome from the QTL projected to the reference map by clustering all the QTL of each chromosome and refining the CIs of the QTL (Sosnoswki and Joets, 2012). In Meta-analysis step 1 of 2 (Veyrieras et al., 2007), QTL on each chromosome of the reference map were clustered assuming a normal distribution of QTL locations around their true locations and the reported CI and R2-values were used to derive their variances. The software used the Akaike information criterion (AIC), corrected Akaike information criterion (AICc and AIC3), Bayesian information criterion (BIC), and approximate weight of evidence (AWE) to determine the most likely MQTL models on each chromosome. The lowest value obtained from the five criteria became the number of “real QTL” on the chromosome and was used in Meta-analysis step 2 of 2 to generate MQTL with their positions, CIs, and percentage of the membership of the original QTL to each MQTL (Veyrieras et al., 2007).
For each MQTL, we recorded the right and left flanking markers on the IBM2 2008 Neighbors (www.maizegdb.org) reference map and determined their physical positions on the maize B73 reference map version 2 (www.maizegdb.org) using the locus pair lookup tool (Andorf et al., 2010). When a flanking marker was not physically mapped, we used the next closest outer marker. We used the same procedure to determine the physical positions of the QTL from the insect resistance mapping experiments (Groh et al., 1998; Brooks et al., 2007) that we could not include in the meta-analyses. We then compared the physical positions of the QTL determined by their flanking markers with those of the meta-QTL to investigate possible co-localizations. For the experiments which used SNPs (Samayoa et al., 2015a,b; Jiménez-Galindo et al., 2017), we used the physical positions of the significant SNPs where available.
We downloaded 302 QTL from 28 publications consisting of 32 experiments conducted on 21 populations derived from 17 crosses. Mapping populations comprised 10 recombinant inbred lines (RILs), one inter-mated recombinant inbred lines (IRILs), four F3s and six F2s developed from 19 crosses (Table 1). We successfully projected 383 QTL on the IBM2 2008 Neighbors reference map (www.maizegdb.org), of which, 152 were for insect resistance and 221 for CWC (Table 2). The individual maps projection to the reference map resulted in a consensus map of 16681 markers density (7980.637 cM) (Supplementary Material Presentation 1: map_CKM2_map and map_CKM2_QTL). The QTL spread on all the ten chromosomes with chromosome 1 having the highest numbers (57 QTL) and chromosome 8 with the lowest number (28) (Table 2, Figure 1).
Figure 1. Distribution of the different tissue-specific MQTL LIR, Leaf insect resistance; SIR, Stem insect resistance; KIR, Kernel insect resistance, along with the original insect resistance QTL measured in each tissue.
We identified 42 stem insect resistance (SIR), 42 leaf insect resistance (LIR), and 20 kernel insect resistance (KIR) MQTL (Tables 2, 3, Supplementary Material Presentation 1: MQTL summary). Each chromosome displays at least one of each of the tissue-specific resistance MQTL type (Figure 1) and shows a smaller number of the real QTL compared to the original QTL composition (Table 2). Chromosomes 1 contains the highest number of LIR MQTL, four MQTL, and chromosome 10 has the lowest, one MQTL. For SIR MQTL, chromosomes 2 and 5 have the highest, six MQTL each, and the lowest, one MQTL, on chromosome 4. Chromosomes 4 and 10 have each three KIR MQTL, and chromosomes 5 and 8 have each 1 KIR MQTL (Figure 1). The 95% confidence intervals (95% CI) for LIR MQTL vary from 7.71 cM for LIR10 to 140.45 cM for LIR7 with and an average of 48.04 cM. Meta-QTL for SIR have 95% CI varying from 4.73 for SIR11 to 191.02 cM for SIR16 and average at 26.30 cM. Regarding KIR MQTL, the 95% CI vary from 4.21 cM for KIR17 to 231.05.96 cM for KIR1 averaging at 24.42 (Table 3).
Twenty-three SIR MQTL and 13 LIR MQTL involve at least two stem borer species with LIR4 and 22 combining three QTL for resistance to three different SB species (ECB, SCB, and SWCB). Regarding KIR MQTL, KIR3, 15, and 16 combine QTL for MW resistance with resistance to kernel damage by MCB (Table 4). Only KIR18 combines QTL for resistance to MW from both of the two studies conducted for response to MW (García-lara et al., 2009; Castro-Álvarez et al., 2015). Resistance to ECB is involved in most of the LIR, and SIR MQTL and several of these are specific to this insect. Most of the LIR, SIR, and KIR MQTL involve at least one CWC QTL except five LIR, five KIR, and four KIR QTL. Quantitative trait loci for fiber components and hydroxycinnamtes were co-evaluated only in stems, and the former were the only group measured in leaves and the latter were only measured in the kernels. Regarding SIR MQTL, fiber components and hydroxycinnamates are co-involved in 19 MQTL, while 14 MQTL contain only fiber components and three involve hydroxycinnamates alone (Table 4). Hydroxycinnamate QTL are involved in 14 KIR MQTL.
Although in this study more than half of the total populations included in the meta-analysis were of advanced generations (Table 1), most of the MQTL feature a combination of primary and advanced populations. Furthermore, most of the QTL experiments included in the analysis used the line B73 as a parent for their bi-parental populations (Table 1), and as a result, the majority of LIR, SIR, and KIR MQTL contain at least one original QTL identified from a population parented by B73. Also, most of the MQTL identified in this study were representative of temperate, and to some extent, subtropical and tropical maize populations (Tables 1, 4).
We sorted out the different tissue-specific MQTL by their genetic and physical position which revealed 14 regions showing overlaps among MQTL for resistance in different tissues of which, seven involve KIR MQTL, and three combine all the resistance categories (Table 3).
We compared the physical positions of the QTL for insect resistance experiments that we failed to consider in the meta-analysis with those of the declared MQTL and located them in several MQTL taking into consideration of the tissues involved (Table 3). We estimated the percentages of genetic coverage of the MQTL for KIR, LIR, and SIR and that of their projected QTL by summed up the 95% CI in each case while correcting for overlaps in the case of the QTLs, and computing their percentages against the genetic size of the reference map following the formula:
To compute the percentage of genetic coverage reduction achieved by the meta-analysis, we used the formula:
Thus, the meta-analysis allowed reduction of the genetic coverage from 33.86 to 13.91%, 50.43 to 14.45%, and 69.65 to 13.84%, for KIR, LIR, and SIR, respectively, amounting to a reduction from QTL to MQTL coverage of 58.91, 71.35, and 80.12%, respectively (Table 3). We also estimated the genome coverage of the KIR, LIR, and SIR MQTL by adding up all the differences between the end and start physical positions of the MQTL in each class and computing their percentages against the physical length of the B73 version 2 which is 2,066,432,718 base pairs (bp) (https://genomevolution.org/coge/OrganismView.pl) using the formula:
Thus, the genome coverage for the different types of resistance is 21.25, 17.07, and 25.03%, for KIR, LIR, and SIR, respectively.
Combinatorial insect attacks on leaves, stems, and kernels severely limit maize yield, and QTL identification was intended to serve as a basis for genetic improvement through marker-assisted breeding programs. However, several factors inherent to experimental and statistical procedures limit the efficient use of QTL (Jiang, 2013). Insect resistance being polygenic and controlled mostly by several small effect QTL, an efficient way of making the QTL information useful in molecular breeding is through a meta-analysis (Wang et al., 2016). Several studies reported the correlations between CWC and insect resistance, especially SB and SP (Santiago et al., 2013). However, the accumulation of CWC in maize varies substantially between tissues and even within the same tissue over time. Hence their involvement in insect resistance varies accordingly (Santiago et al., 2013). Co-localizations of QTL for resistance to different insect species prompted the investigation of MQTL involving multiple insect resistance that would assist in breeding programs for multiple resistance to pests. In this study, QTL for maize resistance to SB and SP, and for maize CWC discovered in leaves, stems, and kernels were separately meta-analyzed using the IBM2 2008 Neighbors (www.maizegdb.org) as a reference map to identify significant MQTL for insect resistance in different maize tissues with potential use in multiple pests' resistance molecular breeding.
Although the number of original QTL in each tissue was substantially reduced, the resulting MQTL are relatively large constituting a limitation for their introgression using MAS. Large CIs resulting from a meta-analysis is not seldom for studies conducted in similar conditions (Jin et al., 2015; Luo et al., 2015; Zhao et al., 2015; Jiang et al., 2016). In fact, the IBM 2 2008 neighbors is a result of intermating lines from a bi-parental cross between B73 and Mo17, and as such, has a size increase of nearly four-fold in the genetic map distance, but also it substantially increases resolution up to 91% (Lee et al., 2002). Therefore, map projection of the original QTL on the reference map results in an increase of the CIs for the individual QTL as a result of the homothetic rescaling of the QTL CI (Sosnoswki and Joets, 2012) leading to a similar increase of the CIs of the MQTL. Also, the increase of MQTL CIs is caused by the fact that the 95% CIs of the QTL, considered conservative and more comprehensive of the real span of the individual QTL (Truntzler et al., 2010), are mostly larger than the original CIs due to low QTL R2. Despite these increases in the size of the CIs, the meta-analysis though BioMercator (Arcade et al., 2004) permitted reduction of the genome size covered by the QTL from 33.86 to 13.91%, 50.43 to 14.45%, and 69.65 to 13.84%, for KIR, LIR, and SIR, respectively. Similar results were obtained by Truntzler et al. (2010) who meta-analyzed maize CWC and digestibility and succeeded to refine QTL CIs and reduce the QTL genome size coverage from 68 to 28%. However, the genome coverage based on the physical coordinates of the MQTL is higher than that based on the genetic map reaching around 17, 21, and 25% of the total maize genome for LIR, KIR, and SIR, respectively. This difference between the genetic and genomic coverage of the MQTL is because the locus pair lookup tool (Andorf et al., 2010) provides a range estimate of physical coordinates of the MQTL's flanking makers which results in wider physical lengths of the MQTL CIs.
Plants co-evolved with insects and developed an array of resistance mechanisms to thwart herbivore attacks through direct or indirect defense mechanisms (War et al., 2012). Consequently, plant-insect interaction is the primary driving force of plants' evolution, especially the development and conservation of a diverse range of defense metabolites and their underlying genes (Kliebenstein, 2014). The results of this study corroborate the significant role played by plant chemicals with the involvement of at least one CWC QTL in the majority of the identified insect resistance MQTL and confirms earlier correlations (Groh et al., 1998; Papst et al., 2004; Cardinal and Lee, 2005; Krakowsky et al., 2007; García-lara et al., 2010; Santiago et al., 2016). Also, several co-localizations between fiber and hydroxycinnamates, and between hydroxycinnamates and sugars occur within the SIR and KIR MQTL, respectively. However, a co-localization between two or more QTL does not necessarily mean they control the same phenotypes since a QTL is a genomic region that can contain several genes that could be playing different functions. In fact, QTL co-localizations result from mainly two reasons. One reason is gene pleiotropism whereby genes under the MQTL regions regulate the production of CWC conferring a protective function against several insects through the fortification of the maize cell wall and antibiosis or antixenosis (Smith and Clement, 2015). It could also be due to tight-linkage of QTL/genes not resolved by the meta-analysis. Ferulates and p-coumarates are reported to form several structures through cross-linking and binding with fiber components such as hemicellulose and lignin which in turn act as a barrier to leaf, stem, and kernel feeding by insects (Santiago et al., 2013, 2017), which, in this study could explain the co-localization of QTL for these components in most SIR MQTL. Besides, 14 chromosomal regions contain overlaps between MQTL for resistance in different tissues of which, seven involve KIR MQTL and three combine all the resistance categories suggesting possible common resistance components in these MQTL. Transcription factors that regulate plant secondary metabolism genes are mostly tissue-specific but also can be ubiquitous (Pichersky and Gang, 2000; Vom Endt et al., 2002; LeClere et al., 2007). Furthermore, plants can use defense mechanisms specific to one tissue or condition to respond to stresses in another compartment through the production of new specific enzymes that could be functional variations of existing ones and arise from genes routinely expressed in specific conditions (Pichersky and Gang, 2000). Thus, the overlaps between different tissue-specific MQTL are worth further investigations to test the hypothesis of common resistance mechanisms across tissues.
Despite its importance in trait genetic architecture analysis, QTL meta-analysis for maize resistance to insect-related stresses has been only reported for ear rot rates and mycotoxin contaminations due to Aspergillus flavus, Fusarium graminearum, and F. verticillioides (Xiang et al., 2010; Mideros et al., 2014). This study is the first report of QTL meta-analyses on maize resistance to insect herbivory and corroborates the polygenic nature of maize resistance to SB and SP (Kliebenstein, 2014). Most LIR and SIR MQTL discovered involve multiple insect resistance confirming correlations among resistance mechanisms to diverse stem borer species (Thome et al., 1992). Some of LIR MQTL like LIR4, 17, and 22 involve resistance to ECB, SCB, and SWCB. Regarding QTL experiments for stem insect resistance, only ECB and MCB were involved. Therefore, multiple insect resistance MQTL were related to these two insect pests, for instance, SIR2-5, 10, 20, 26, 34, 34, and 38. Furthermore, the kernel insect resistance MQTL, KIR3, 15, and KIR18 involve QTL for resistance to kernel damage by MCB and MW and could be good candidates for reducing maize grain damage and mycotoxin contamination attributable to insect pests. These multiple-insect resistance tissue-specific MQTL, when used together in a MAS scheme, could help in sustainably improving maize resistance to a broad range of insect pest species.
The omnipresence of ECB QTL in most of the MQTL identified in this study might be due solely to the fact that more experiments, thus more QTL for ECB were included (Table 1). It is probable that if more experiments were previously conducted on other SB and SP species as required for polygenic traits (Collard et al., 2005; Ordas et al., 2009), the meta-analyses would have generated more valuable MQTL. Thus, a comprehensive review of stem borer resistance-related mapping experiments provided by Meihls et al. (2012) located the different QTL discovered in the maize bins. A comparison between the bin locations of the MQTL from our study with that of the QTL in the experiments we could not include in the meta-analysis (Groh et al., 1998; Brooks et al., 2007; Samayoa et al., 2015a,b; Jiménez-Galindo et al., 2017) shows co-localization in the same or adjacent bins. These co-localizations were further illustrated by locating some of these QTL (Groh et al., 1998; Brooks et al., 2007; Samayoa et al., 2015a,b; Jiménez-Galindo et al., 2017) within the corresponding tissue-specific MQTL based on the physical positions of their flanking markers. However, several other QTL from these studies did not fall within the CIs of the MQTL corroborating the probability of the existence of more tissue-specific MQTL. Furthermore, only KIR18 combines QTL discovered from both of the MW resistance QTL mapping experiments (García-lara et al., 2009; Castro-Álvarez et al., 2015). The lack of QTL co-localizations between these two studies necessitates conducting more QTL experiments on MW resistance on diverse maize background to confirm the discovered QTL and identify additional MW resistance genomic regions (Castro-Álvarez et al., 2015). In this meta-analysis, no QTL experiment conducted in Africa could be included, yet maize resistance to local insects, especially stem borer species could be having a different genetic basis due to the co-evolutionary and environment-dependent nature of plant-insect interactions (War et al., 2012; Kliebenstein, 2014). Therefore, more QTL discovery studies for resistance to local stem borers such as Busseola fusca and Chilo partellus need to be conducted in addition to the already available ones (Munyiri and Mugo, 2017) to allow more comprehensive comparative mappings to be carried out. These recommendations also hold for other parts of the world such as Central and South-America, and Asia maize germplasms and stem borer and storage pest species.
The maize pan-genome theory supports the commonality of a substantial portion of the maize genome, containing almost all the genes, in all lines (Morgante et al., 2007). Maize experiences several simultaneously or subsequently occurring abiotic and biotic stress, and such stress events lead to the generalization of fitness phenotypes across environments among other adaptive strategies (Anderson et al., 2013). A generalization of insect resistance across populations of diverse genetic and geographic origins would allow developing multiple pest resistance by taking advantage of available data. This meta-analysis allowed us to investigate the commonality of the genetic basis maize resistance to insects of geographically diverse environments among both genetically and geographically diverse maize populations. However, QTL studies have mostly been conducted using temperate materials from North America and Europe. The genetic variability in other germplasms such as African and Central and Southern American and Asian materials have been poorly explored, hence, underrepresented in the meta-QTL identified in this study. Therefore, the current MQTL study might not have comprehensively uncovered all possible genomic regions involved in maize resistance to stem borers and storage pests. Nonetheless, we identified several consensus QTL, and as per the pan-genome theory, we can assume that the MQTL identified are inclusive of most of the insect resistance genes (Morgante et al., 2007) contained in European and North American germplasm. Also, most of the MQTL identified in this study are representative of all the geographical diversity and the different recombination levels of the host plant populations used in the original QTL mapping experiments. The diversity in these MQTL implies a commonality of the genomic regions responsible for multiple pest resistance across populations and generations. Conducting QTL mapping studies in other regions of the world and including them in a more comprehensive meta-analysis would help to better understand the extent of this convergence of resistance genomic regions in maize.
Insect resistance QTL in maize have low R2 due to the influence of low to moderate heritabilities (García-lara et al., 2009), and several QTL are involved with usually small effects and large CI in controlling the trait (Jiang, 2013), especially for maize resistance to SP (García-lara et al., 2009). Low heritabilities and large CIs imply low efficacy in MAS (Ordas et al., 2009). However, in this meta-analysis, the MQTL's CIs and genome coverage are still large, which could be solved by conducting more QTL discovery studies using more precise methods. Nonetheless, the MQTL identified here can help in efficiently achieving multiple pest resistance by accumulating into commercially preferred but susceptible lines through molecular breeding approaches. Furthermore, the overlaps observed among MQTL from different tissues prompts the investigation of combined resistance across maize tissues and insect pest species which is feasible through multi-trait association mapping (Stich et al., 2008) among other methods.
The polygenic nature of combined resistance highlighted in this study implies that the most effective methods for molecular breeding of multiple-insect resistant lines would be marker-assisted gene pyramiding or marker-assisted recurrent selection (Jiang, 2013) and could be combined with phenotypic selection for better breeding progress when dealing with insect resistance with low heritability (Collard and Mackill, 2008). The MQTL identified in the current study cannot be readily utilized in regions, for instance, the sub-Saharan Africa, whose germplasms was limitedly included in the meta-analysis. In a bid to accelerate breeding, regional genome-wide association studies (Chen et al., 2017) could be conducted to investigate resistance trait-related SNPs/INDELs within the MQTL identified in this study as a confirmatory step before use in multiple insect pests' resistance molecular breeding.
AB conceived and developed the study with the help of PR, SK, LM, MO, TO, and DK. AB run the analyses with inputs from DO, SA, LM, and DK. The manuscript was drafted by AB and was critically reviewed by all the authors with final approval by PR, SK, MO, LM, and TO.
The authors are thankful for the scholarship received from the Intra-ACP Academic mobility for Crop Scientists for Africa Agriculture (CSAA) project. Support was also received from the capacity building competitive grant training the next generation of scientists provided by Carnegie Cooperation of New York through the Regional Universities Forum for Capacity Building in Agriculture (RUFORUM: RU/2016/Intra-ACP/RG/001).
The authors declare that the research was conducted in the absence of any commercial or financial relationships that could be construed as a potential conflict of interest.
The reviewer AB and handling Editor declared their shared affiliation.
The authors would like to thank Yannick De Oliveira from the BioMercator google group support team (https://groups.google.com/forum/#!forum/biomercator) for valuable advice during analysis. Fruitful discussion with Dr. Patrick Ongom from the Department of Agricultural Production of Makerere University is acknowledged.
The Supplementary Material for this article can be found online at: https://www.frontiersin.org/articles/10.3389/fpls.2018.00895/full#supplementary-material
Anderson, J. T., Rushworth, C. A., and Prasad, K. (2013). The evolution of quantitative traits in complex environments. Heredity 112, 4–12. doi: 10.1038/hdy.2013.33
Andorf, C. M., Lawrence, C. J., Harper, L. C., Schaeffer, M. L., Campbell, D. A., and Sen, T. Z. (2010). The Locus Lookup tool at MaizeGDB: identification of genomic regions in maize by integrating sequence information with physical and genetic maps. Bioinformatics 26, 434–436. doi: 10.1093/bioinformatics/btp556
André, A. M., van Rensburg, J. B. J., and Labuschagne, M. T. (2003). Inheritance of resistance in maize to the African stalk borer, Busseola fusca (Fuller) (Lepidoptera: Noctuidae). South Afr. J. Plant Soil 20, 64–71. doi: 10.1080/02571862.2003.10634910
Arcade, A., Labourdette, A., Falque, M., Mangin, B., Chardon, F., Charcosset, A., et al. (2004). BioMercator: integrating genetic maps and QTL towards discovery of candidate genes. Bioinformatics 20, 2324–2326. doi: 10.1093/bioinformatics/bth230
Barriere, Y., Thomas, J., and Denoue, D. (2008). QTL mapping for lignin content, lignin monomeric composition, p -hydroxycinnamate content, and cell wall digestibility in the maize recombinant inbred line progeny F838 x F286. Plant Sci. 175, 585–595. doi: 10.1016/j.plantsci.2008.06.009
Barros, J., Malvar, R. A., Butrón, A., and Santiago, R. (2011). Combining abilities in maize for the length of the internode basal ring, the entry point of the Mediterranean corn borer larvae. Plant Breed. 130, 268–270. doi: 10.1111/j.1439-0523.2010.01789.x
Bohn, M., Khairallah, M. M., Gonz, D., Hoisington, D. A., Utz, H. F., Deutsch, J. A., et al. (1997). QTL mapping in tropical maize. II. Comparison of genomic regions for resistance to Diatraea spp. Crop Sci. 37, 1892–1902. doi: 10.2135/cropsci1997.0011183X003700060038x
Bohn, M., Khairallah, M. M., Gonzalez-de-Leon, D., Hoisington, D. A., Utz, H. F., Deutsch, J. A., et al. (1996). Mapping in tropical maize : I. genomic regions affecting leaf feeding resistance to sugarcane borer and other traits originating from the Caribbean. Crop Sci. 36, 1352–1361. doi: 10.2135/cropsci1996.0011183X003600050045x
Bohn, M., Schulz, B., Kreps, R., Klein, D., and Melchinger, A. E. (2000). QTL mapping for resistance against the European corn borer (Ostrinia nubilalis H.) in early maturing European dent germplasm. Theor. Appl. Genet. 101, 907–917. doi: 10.1007/s001220051561
Brooks, T. D., Bushman, B. S., Williams, W. P., McMullen, M. D., and Buckley, P. M. (2007). Genetic basis of resistance to fall armyworm (Lepidoptera: Noctuidae) and southwestern corn borer (Lepidoptera: Crambidae) leaf-feeding damage in maize. J. Econ. Entomol. 100, 1470–1475. doi: 10.1093/jee/100.4.1470
Brooks, T. D., Willcox, M. C., Williams, W. P., and Buckley, P. M. (2005). Quantitative trait loci conferring resistance to fall armyworm and southwestern corn borer leaf feeding damage. Crop Sci. 45, 2430–2434. doi: 10.2135/cropsci2004.0656
Campagne, P., Kruger, M., Pasquet, R., Le Ru, B., and Van den Berg, J. (2013). Dominant Inheritance of Field-Evolved Resistance to Bt Corn in Busseola fusca. PLoS ONE 8:69675. doi: 10.1371/journal.pone.0069675
Cao, A., Santiago, R., Ramos, A. J., Souto, X. C., Aguín, O., Malvar, R. A., et al. (2014). Critical environmental and genotypic factors for Fusarium verticillioides infection, fungal growth and fumonisin contamination in maize grown in northwestern Spain. Int. J. Food Microbiol. 177, 63–71. doi: 10.1016/j.ijfoodmicro.2014.02.004
Cardinal, A. J., and Lee, M. (2005). Genetic relationships between resistance to stalk-tunneling by the European corn borer and cell-wall components in maize population B73xB52. Theor. Appl. Genet. 111, 1–7. doi: 10.1007/s00122-004-1831-5
Cardinal, A. J., Lee, M., and Moore, K. J. (2003). Genetic mapping and analysis of quantitative trait loci affecting fiber and lignin content in maize Theor. Appl. Genet. 106, 866–874. doi: 10.1007/s00122-002-1136-5
Cardinal, A. J., Lee, M., Guthrie, W. D., Bing, J., Austin, D. F., Veldboom, L. R., et al. (2006). Mapping of factors for resistance to leaf-blade feeding by European corn borer (Ostrinia nubilalis) in maize. Maydica 51, 93–102.
Cardinal, A. J., Lee, M., Sharopova, N., Woodman-clikeman, W. L., and Long, M. J. (2001). Genetic Mapping and Analysis of quantitative trait loci for resistance to stalk tunneling by the European Corn Borer in maize. Crop Sci. 41, 835–845. doi: 10.2135/cropsci2001.413835x
Castro-Álvarez, F. F., Manilal, Á., Bergvinson, D. J., and García-Lara, S. (2015). Genetic mapping of QTL for maize weevil resistance in a RIL population of tropical maize. Theor. Appl. Genet. 128, 411–419. doi: 10.1007/s00122-014-2440-6
Chen, L., An, Y., Li, Y., Li, C., Shi, Y., Song, Y., et al. (2017). Candidate Loci for yield-related traits in maize revealed by a combination of MetaQTL analysis and regional association mapping. Front. Plant Sci. 8:2190. doi: 10.3389/fpls.2017.02190
Collard, B. C., and Mackill, D. J. (2008). Marker-assisted selection : an approach for precision plant breeding in the twenty-first century. Phil. Trans. R. Soc. B. 363, 557–572. doi: 10.1098/rstb.2007.2170
Collard, B. C. Y., Jahufer, M. Z. Z., Brouwer, J. B., and Pang, E. C. K. (2005). An introduction to markers, quantitative trait loci (QTL) mapping and marker-assisted selection for crop improvement: the basic concepts. Euphytica 142, 169–196. doi: 10.1007/s10681-005-1681-5
Courtial, A., Méchin, V., Reymond, M., Grima-Pettenati, J., and Barrière, Y. (2014). Colocalizations between several QTLs for cell wall degradability and composition in the F288 × F271 Early Maize RIL progeny raise the question of the nature of the possible underlying determinants and breeding targets for biofuel capacity. Bioenergy Res. 7, 142–156. doi: 10.1007/s12155-013-9358-8
Courtial, A., Thomas, J., Reymond, M., Méchin, V., Grima-Pettenati, J., and Barrière, Y. (2013). Targeted linkage map densification to improve cell wall related QTL detection and interpretation in maize. Theor. Appl. Genet. 126, 1151–1165. doi: 10.1007/s00122-013-2043-7
Darvasi, A., and Soller, M. (1997). A simple method to calculate resolving power and confidence interval of QTL map location. Behav. Genet. 27, 125–132. doi: 10.1023/A:1025685324830
Demissie, G., Tefera, T., and Tadesse, A. (2008). Importance of husk covering on field infestation of maize by Sitophilus zeamais Motsch (Coleoptera: Curculionidea) at Bako, Western Ethiopia. African J. Biotechnol. 7, 3777–3782.
Fontaine, A. S., Bout, S., Barrière, Y., and Vermerris, W. (2003). Variation in Cell wall composition among forage maize (Zea mays L.) inbred lines and its impact on digestibility: analysis of neutral detergent fiber composition by pyrolysis-gas chromatography-mass spectrometry. J. Agri. Food Chem. 51, 8080–8087. doi: 10.1021/jf034321g
García-lara, S., Burt, A. J., Arnason, J. T., and Bergvinson, D. J. (2010). QTL mapping of tropical maize grain components associated with maize weevil resistance. Crop Sci. 50, 815–825. doi: 10.2135/cropsci2009.07.0415
García-lara, S., Khairallah, M. M., Vargas, M., and Bergvinson, D. J. (2009). Mapping of QTL Associated with Maize Weevil resistance in tropical maize. Crop Sci. 49, 139–149. doi: 10.2135/cropsci2007.06.0326
Groh, S., Gonzalez-de-Leon, D., Khairallah, M. M., Jiang, C., Bergvinson, D., Bohn, M., et al. (1998). QTL Mapping in Tropical Maize: III. genomic regions for resistance to Diatraea spp. and associated traits in two RIL populations. Crop Sci. 38, 1062–1072. doi: 10.2135/cropsci1998.0011183X003800040030x
Hazen, S. P., Hawley, R. M., Davis, G. L., Henrissat, B., and Walton, J. D. (2003). Quantitative trait loci and comparative genomics of cereal cell wall composition. Plant Physiol. 132, 263–271. doi: 10.1104/pp.103.020016
Jampatong, C., McMullen, M. D., Barry, B. D., Darrah, L. L., Byrne, P. F., and Kross, H. (2002). Quantitative trait loci for first- and second-generation european corn borer resistance derived from the Maize Inbred Mo47. Crop Sci. 42, 584–593. doi: 10.2135/cropsci2002.0584
Jiang, G. L. (2013). “Molecular markers and marker-assisted breeding in plants,” in Plant Breeding from Laboratories to Fields (IntechOpen), 45–83.
Jiang, Q., Tang, D., Hu, C., Qu, J., and Liu, J. (2016). Combining meta-QTL with RNA-seq data to identify candidate genes of kernel row number trait in maize. Maydica 61, 1–9.
Jiménez-Galindo, J. C., Ordás, B., Butron, A., Samayoa, L. F., and Malvar, R. A. (2017). QTL Mapping for yield and resistance against Mediterranean corn borer in maize. Front. Plant Sci. 8:698. doi: 10.3389/fpls.2017.00698
Jin, T., Chen, J., Zhu, L., Zhao, Y., Guo, J., and Huang, Y. (2015). Comparative mapping combined with homology-based cloning of the rice genome reveals candidate genes for grain zinc and iron concentration in maize. BMC Genetics 16:1. doi: 10.1186/s12863-015-0176-1
Kanyamasoro, M. G., Karungi, J., Asea, G., and Gibson, P. (2012). Determination of the heterotic groups of maize inbred lines and the inheritance of their resistance to the maize weevil. African Crop Sci. J. 20(Supplement S1), 99–104.
Khairallah, M. M., Bohn, M., Jiang, C., Deutsch, J. A., Jewell, D. C., Mihm, J. A., et al. (1998). Molecular mapping of QTL for southwestern corn borer resistance, plant height and flowering in tropical maize. Plant Breed. 117, 309–318. doi: 10.1111/j.1439-0523.1998.tb01947.x
Kim, S. K., and Kossou, D. K. (2003). Responses and genetics of maize germplasm resistant to the maize weevil Sitophilus zeamais Motschulsky in West Africa. J. Stor. Prod. Res. 39, 489–505. doi: 10.1016/S0022-474X(02)00056-5
Kissoudis, C., van de Wiel, C., Visser, R. G., and van der Linden, G. (2014). Enhancing crop resilience to combined abiotic and biotic stress through the dissection of physiological and molecular crosstalk. Front. Plant Sci. 5:207. doi: 10.3389/fpls.2014.00207
Kliebenstein, D. J. (2014). Quantitative genetics and genomics of plant resistance to insects. Ann. Plant Rev. 47, 235–262. doi: 10.1002/9781118829783.ch7
Krakowsky, M. D., Brinkman, M. J., Woodman-Clikeman, W. L., and Lee, M. (2002). Genetic components of resistance to stalk tunneling by the European corn borer in maize. Crop Sci. 42, 1309–1315. doi: 10.2135/cropsci2002.1309
Krakowsky, M. D., Lee, M., and Coors, J. G. (2005). Quantitative trait loci for cell-wall components in recombinant inbred lines of maize (Zea mays L.) I: stalk tissue. Theor. Appl. Genet. 111, 337–346. doi: 10.1007/s00122-005-2026-4
Krakowsky, M. D., Lee, M., and Coors, J. G. (2006). Quantitative trait loci for cell wall components in recombinant inbred lines of maize (Zea mays L.) II: leaf sheath tissue. Theor. Appl. Genet. 112, 717–726. doi: 10.1007/s00122-005-0175-0
Krakowsky, M. D., Lee, M., and Holland, J. B. (2007). Genotypic correlation and multivariate QTL analyses for cell wall components and resistance to stalk tunneling by the European corn borer in maize. Crop Sci. 47, 485–490. doi: 10.2135/cropsci2006.05.0283
Krakowsky, M. D., Lee, M., Long, M. J., and Sharopova, N. (2004). QTL Mapping of resistance to stalk tunneling by the European Corn Borer in RILs of maize population B73 × De811. Crop Sci. 44, 274–282. doi: 10.2135/cropsci2004.2740
LeClere, S., Schmelz, E. A., and Chourey, P. S. (2007). Phenolic compounds accumulate specifically in maternally-derived tissues of developing maize kernels. Cereal Chem. 84, 350–356. doi: 10.1094/CCHEM-84-4-0350
Lee, M., Sharopova, N., Beavis, W. D., Grant, D., Katt, M., Blair, D., et al. (2002). Expanding the genetic map of maize with the intemated B73 × Mo17 (IBM) population. Plant Mol. Biol. 48, 453–461. doi: 10.1023/A:1014893521186
Liu, B. H. (1997). Statistical Genomics: Linkage, Mapping, and QTL Analysis. Boca Raton, FL: CRC Press.
Luo, B., Tang, H., Liu, H., Shunzong, S., Zhang, S., Wu, L., et al. (2015). Mining for low-nitrogen tolerance genes by integrating meta-analysis and large-scale gene expression data from maize. Euphytica 206, 117–131. doi: 10.1007/s10681-015-1481-5
Méchin, V., Argillier, O., Hébert, Y., Guingo, E., Moreau, L., Charcosset, A., et al. (2001). Genetic analysis and QTL mapping of cell wall digestibility and lignification in silage maize. Crop Sci. 41, 690–697. doi: 10.2135/cropsci2001.413690x
Meihls, L. N., Handrick, V., Glauser, G., Barbier, H., Kaur, H., Haribal, M. M., et al. (2013). Natural variation in maize aphid resistance is associated with 2,4-Dihydroxy-7-Methoxy-1,4-Benzoxazin-3-one glucoside methyltransferase activity. Plant Cell 25, 2341–2355. doi: 10.1105/tpc.113.112409
Meihls, L. N., Kaur, H., and Jander, G. (2012). Natural variation in maize defense against insect herbivores. Cold Spring Harbor Symp. Quant. Biol. 77, 269–283. doi: 10.1101/sqb.2012.77.014662
Mideros, S. X., Warburton, M. L., Jamann, T. M., Windham, G. L., Williams, W. P., and Nelson, R. J. (2014). Quantitative trait loci influencing mycotoxin contamination of maize: analysis by linkage mapping, characterization of near-isogenic lines, and meta-analysis. Crop Sci. 54, 127–142. doi: 10.2135/cropsci2013.04.0249
Morgante, M., De Paoli, E., and Radovic, S. (2007). Transposable elements and the plant pan-genomes. Curr. Opin. Plant Biol. 10, 149–155. doi: 10.1016/j.pbi.2007.02.001
Munyiri, S. W., and Mugo, S. N. (2017). Quantitative trait loci for resistance to spotted and African maize stem borers (Chilo partellus and Busseola fusca) in a tropical maize (Zea mays L.) population. Afr. J. Biotechnol. 16, 1579–1589. doi: 10.5897/AJB2017.15991
Munyiri, S. W., Mugo, S. N., and Mwololo, J. K. (2015). Mechanisms and levels of resistance in hybrids, open pollinated varieties and landraces to Chilo partellus maize stem borers. Int. Res. J. Agric. Sci. Soil Sci., 5, 81–90. doi: 10.14303/irjas.2015.029
Murenga, M., Derera, J., Mugo, S., and Tongoona, P. (2016). A review of genetic analysis and response to selection for resistance to Busseola fusca and Chilo partellus, stem borers in tropical maize germplasm: a Kenyan perspective. Maydica 61.
Mwololo, J. K. (2013). Resistance in Tropical Maize To the Maize Weevil and Larger Grain Borer. Ph.D. thesis, Makerere University.
Mwololo, J. K., Okori, P., Mugo, S., Tefera, T., Yoseph, B., Otim, M., et al. (2012). Phenotypic and genotypic variation in tropical maize inbred lines for resistance to the maize weevil and larger grain borer. Int. J. Agri. Sci. Res. 2, 41–52.
Nyukuri, R. W., Wanjala, F. M., Kirui, S. C., and Cheramgoi, E. (2014). Damage of stem borer species to Zea mays L., sorghum bicolor 1. and three refugia graminae. Adv. Agri. Biol. 1, 6–11. doi: 10.14303/ajfst.2014.012
Ordas, B., Malvar, R. A., Santiago, R., and Butron, A. (2010). QTL mapping for Mediterranean corn borer resistance in European flint germplasm using recombinant inbred lines. BMC Genomics 11:174. doi: 10.1186/1471-2164-11-174
Ordas, B., Malvar, R. A., Santiago, R., Sandoya, G., Romay, M. C., and Butron, A. (2009). Mapping of QTL for resistance to the Mediterranean corn borer attack using the intermated B73 3 Mo17 (IBM) population of maize. Theor. Appl. Genet. 119, 1451–1459. doi: 10.1007/s00122-009-1147-6
Orsini, E., Krchov, L. M., Uphaus, J., and Melchinger, A. E. (2012). Mapping of QTL for resistance to first and second generation of European corn borer using an integrated SNP and SSR linkage map. Euphytica 183, 197–206. doi: 10.1007/s10681-011-0441-y
Papst, C., Bohn, M., Utz, H. F., Melchinger, A. E., Klein, D., and Eder, J. (2004). QTL mapping for European corn borer resistance (Ostrinia nubilalis Hb.), agronomic and forage quality traits of testcross progenies in early-maturing European maize (Zea mays L.) germplasm. Theor. Appl. Genet. 108, 1545–1554. doi: 10.1007/s00122-003-1579-3
Pichersky, E., and Gang, D. R. (2000). Genetics and biochemistry of secondary metabolites in plants: an evolutionary perspective. Trends Plant Sci. 5, 439–445. doi: 10.1016/S1360-1385(00)01741-6
Riboulet, C., Fabre, F., Dknoue, D., Martinant, J. P., Lefevre, B., and Barrière, Y. (2008). QTL mapping and candidate gene research for lignin content and cell wall digestibility in a top-cross of a flint maize recombinant inbred line progeny harvested at silage stage. Maydica 53, 1–9.
Roussel, V., Gibelin, C., Fontaine, A. S., and Barriere, Y. (2002). Genetic analysis in recombinant inbred lines of early dent forage maize. II. QTL mapping for cell wall constituents and cell wall digestibility from per se value and top cross experiments. Maydica 47, 9–20.
Samayoa, L. F., Butron, A., and Malvar, R. A. (2014). QTL mapping for maize resistance and yield under infestation with Sesamia nonagrioides. Mol. Breed. 34, 1331–1344. doi: 10.1007/s11032-014-0119-y
Samayoa, L. F., Malvar, R. A., Mcmullen, M. D., and Butrón, A. (2015a). Identification of QTL for resistance to Mediterranean corn borer in a maize tropical line to improve temperate germplasm. BMC Plant Biol. 15:265. doi: 10.1186/s12870-015-0652-9
Samayoa, L. F., Malvar, R. A., Olukolu, B. A., Holland, J. B., and Butrón, A. (2015b). Genome-wide association study reveals a set of genes associated with resistance to the Mediterranean corn borer (Sesamia nonagrioides L.) in a maize diversity panel. BMC Plant Biol. 15:35. doi: 10.1186/s12870-014-0403-3
Sandoya, G., Butrón, A., Santiago, R., Alvarez, A., and Malvar, R. A. (2010). Indirect response to selection for improving resistance to the Mediterranean corn 2 borer (Sesamia nonagrioides Lef) in maize. Euphytica 176, 231–237. doi: 10.1007/s10681-010-0232-x
Santiago, R., Barros-Rios, J., and Malvar, R. (2013). Impact of cell wall composition on maize resistance to pests and diseases. Int. J. Mol. Sci. 14, 6960–6980. doi: 10.3390/ijms14046960
Santiago, R., Cao, A., Butrón, A., López-Malvar, A., Rodríguez, V. M., Sandoya, G. V., et al. (2017). Defensive changes in maize leaves induced by feeding of Mediterranean corn borer larvae. BMC Plant Biol. 7, 305–312. doi: 10.1186/s12870-017-0991-9
Santiago, R., Malvar, R. A., Barros-Rios, J., Samayoa, L. F., and Butrón, A. (2016). Hydroxycinnamate Synthesis and Association with Mediterranean Corn Borer Resistance. J. Agri. Food Chem. 64, 539–551. doi: 10.1021/acs.jafc.5b04862
Schön, C. C., Lee, M., Melchinger, A. E., Guthrie, W. D., and Woodman, W. L. (1993). Mapping and characterization of quantitative trait loci affecting resistance against second-generation European corn borer in maize with the aid of RFLPs. Heredity 70, 648–659. doi: 10.1038/hdy.1993.93
Shiferaw, B., Prasanna, B. M., Hellin, J., and Bänziger, M. (2011). Crops that feed the world 6. Past successes and future challenges to the role played by maize in global food security. Food Security 3, 307–327. doi: 10.1007/s12571-011-0140-5
Smith, C. M., and Clement, S. L. (2015). Molecular bases of plant resistance to arthropods. Ann. Rev. Entomol. 57, 309–328. doi: 10.1146/annurev-ento-120710-100642
Stam, J. M., Kroes, A., Li, Y., Gols, R., Loon, J. J. A., Van, P., et al. (2014). Plant interactions with multiple insect herbivores: from community to genes. Ann Rev. Plant Biol. 65, 689–713. doi: 10.1146/annurev-arplant-050213-035937
Stevens, R. (2008). Prospects for using marker-assisted breeding to improve maize production in Africa. J. Sci. Food Agric. 755, 745–755. doi: 10.1002/jsfa.3154
Stich, B., Piepho, H.-P., Schulz, B., and Melchinger, A. E. (2008). Multi-trait association mapping in sugar beet (Beta vulgaris L.). Theor. Appl. Genet. 117, 947–954. doi: 10.1007/s00122-008-0834-z
Sylvain, N. M., Manyangarirwa, W., Tuarira, M., and Onesime, M. K. (2015). Effect of lepidopterous stemborers, Busseola fusca (Fuller) and Chilo partellus (Swinhoe) on maize (Zea mays L) yield: a review. Int. J. Innovat. Res. Dev. 4.
Tefera, T., Mugo, S., and Beyene, Y. (2016). Developing and deploying insect resistant maize varieties to reduce pre-and post-harvest food losses in Africa. Food Security 8, 211–220. doi: 10.1007/s12571-015-0537-7
Thome, C. R., Smith, M. E., and Mihm, J. A. (1992). Leaf feeding resistance to multiple insect species in a maize diallel. Crop Sci. 32, 1460–1463. doi: 10.2135/cropsci1992.0011183X003200060030x
Truntzler, M., Barrière, Y., Sawkins, M. C., Lespinasse, D., Betran, J., Charcosset, A., et al. (2010). Meta-analysis of QTL involved in silage quality of maize and comparison with the position of candidate genes. Theor. Appl. Genet. 121, 1465–1482. doi: 10.1007/s00122-010-1402-x
Udaykumar, K., Wali, M. C., Deepa, M., Laxman, M., and Gangashetty, P. (2013). Combining ability studies for yield and its related traits in newly derived inbred lines of maize (Zea Mays L.). Mol. Plant Breed. 4, 71–76. doi: 10.5376/mpb.2013.04.0008
Utz, H. F., Melchinger, A. E., and Schön, C. C. (2000). Bias and sampling error of the estimated proportion of genotypic variance explained by quantitative trait loci determined from experimental data in maize using cross validation and validation with independent samples. Genetics 154, 1839–1849.
Van, K., and McHale, L. K. (2017). Meta-analyses of QTLs associated with protein and oil contents and compositions in soybean [Glycine max (L.) Merr.] Seed. Int. J. Mol. Sci. 18:6. doi: 10.3390/ijms18061180
Veyrieras, J.-B., Goffinet, B., and Charcosset, A. (2007). MetaQTL: a package of new computational methods for the meta-analysis of QTL mapping experiments. BMC Bioinform. 8:49. doi: 10.1186/1471-2105-8-49
Vom Endt, D., Kijne, J. W., and Memelink, J. (2002). Transcription factors controlling plant secondary metabolism: what regulates the regulators? Phytochemistry 61, 107–114. doi: 10.1016/S0031-9422(02)00185-1
Wang, Y., Xu, J., Deng, D., Ding, H., Bian, Y., Yin, Z., et al. (2016). A comprehensive meta-analysis of plant morphology, yield, stay-green, and virus disease resistance QTL in maize (Zea mays L.). Planta 243, 459–471. doi: 10.1007/s00425-015-2419-9
War, A. R., Paulraj, M. G., Ahmad, T., Buhroo, A. A., Hussain, B., Ignacimuthu, S., et al. (2012). Mechanisms of plant defense against insect herbivores. Plant Signal. Behav. 7, 1306–1320. doi: 10.4161/psb.21663
Xiang, K., Zhang, Z. M., Reid, L. M., Zhu, X. Y., Yuan, G. S., and Pan, G. T. (2010). A meta-analysis of QTL associated with ear rot resistance in maize. Maydica 55, 281–290.
Keywords: maize, stem borers, storage pests, cell wall constituents, tissue-specific meta-QTL, multiple-insect resistance, marker-assisted selection
Citation: Badji A, Otim M, Machida L, Odong T, Kwemoi DB, Okii D, Agbahoungba S, Mwila N, Kumi F, Ibanda A, Mugo S, Kyamanywa S and Rubaihayo P (2018) Maize Combined Insect Resistance Genomic Regions and Their Co-localization With Cell Wall Constituents Revealed by Tissue-Specific QTL Meta-Analyses. Front. Plant Sci. 9:895. doi: 10.3389/fpls.2018.00895
Received: 01 November 2017; Accepted: 07 June 2018;
Published: 05 July 2018.
Edited by:
Rosa Ana Malvar, Misión Biológica de Galicia (MBG)-CSIC, SpainReviewed by:
Rogelio Santiago, University of Vigo, SpainCopyright © 2018 Badji, Otim, Machida, Odong, Kwemoi, Okii, Agbahoungba, Mwila, Kumi, Ibanda, Mugo, Kyamanywa and Rubaihayo. This is an open-access article distributed under the terms of the Creative Commons Attribution License (CC BY). The use, distribution or reproduction in other forums is permitted, provided the original author(s) and the copyright owner(s) are credited and that the original publication in this journal is cited, in accordance with accepted academic practice. No use, distribution or reproduction is permitted which does not comply with these terms.
*Correspondence: Arfang Badji, YXJiYWQyMDA5QGxpdmUuZnI=
Disclaimer: All claims expressed in this article are solely those of the authors and do not necessarily represent those of their affiliated organizations, or those of the publisher, the editors and the reviewers. Any product that may be evaluated in this article or claim that may be made by its manufacturer is not guaranteed or endorsed by the publisher.
Research integrity at Frontiers
Learn more about the work of our research integrity team to safeguard the quality of each article we publish.