- 1Graduate School of Life and Environmental Sciences, University of Tsukuba, Tsukuba, Japan
- 2Central Research Institute for Feed and Livestock, National Federation of Agricultural Co-operative Associations, Tsukuba, Japan
- 3RIKEN Center for Sustainable Resource Science, Yokohama, Japan
- 4Graduate School of Pharmaceutical Sciences, Chiba University, Chiba, Japan
- 5Keystone Technology, Yokohama, Japan
- 6Graduate School of Environment and Information Sciences, Yokohama National University, Yokohama, Japan
Vegetables produce metabolites that affect their taste and nutritional value and compounds that contribute to human health. The quality of vegetables grown in plant factories under hydroponic cultivation, e.g., their sweetness and softness, can be improved by controlling growth factors including the temperature, humidity, light source, and fertilizer. However, soil is cheaper than hydroponic cultivation and the visual phenotype of vegetables grown under the two conditions is different. As it is not clear whether their metabolite composition is also different, we studied leaf lettuce raised under the hydroponic condition in practical plant factory and strictly controlled soil condition. We chose two representative cultivars, “black rose” (BR) and “red fire” (RF) because they are of high economic value. Metabolite profiling by comprehensive gas chromatography-mass spectrometry (GC-MS) and liquid chromatography-mass spectrometry (LC-MS) resulted in the annotation of 101 metabolites from 223 peaks detected by GC-MS; LC-MS yielded 95 peaks. The principal component analysis (PCA) scatter plot showed that the most distinct separation patterns on the first principal component (PC1) coincided with differences in the cultivation methods. There were no clear separations related to cultivar differences in the plot. PC1 loading revealed the discriminant metabolites for each cultivation method. The level of amino acids such as lysine, phenylalanine, tryptophan, and valine was significantly increased in hydroponically grown leaf lettuce, while soil-cultivation derived leaf lettuce samples contained significantly higher levels of fatty-acid derived alcohols (tetracosanol and hexacosanol) and lettuce-specific sesquiterpene lactones (lactucopicrin-15-oxalate and 15-deoxylactucin-8-sulfate). These findings suggest that the metabolite composition of leaf lettuce is primarily affected by its cultivation condition. As the discriminant metabolites reveal important factors that contribute to the nutritional value and taste characteristics of leaf lettuce, we performed comprehensive metabolite profiling to identify metabolite compositions, i.e., metabolite signature, that directly improve its quality and value.
Introduction
For the stable supply of vegetables, plant factories use hydroponic cultivation that controls vegetable growth and development under closed environments by regulating important factors for plant growth such as the temperature, humidity, light, growing medium, and plant nutrition (Kozai, 2013). Many use artificial lighting for the cultivation of leafy vegetables and herbs. Because light-emitting diodes (LEDs) suppress heat, their energy requirement is lower than of other light sources such as fluorescent lamps. As plant factories involve higher initial financial investments and running costs than soil cultivation (Tokimasa and Nishiura, 2015), their cultivation conditions must be optimized to yield economically viable horticultural outputs.
Leaf lettuce (Lactuca sativa L. var crispa) is a popular leafy vegetable grown in plant factories. Optimization of hydroponic cultivation conditions has focused on increasing its yield (Touliatos et al., 2016) and on the accumulation of functional nutrients (Miyagi et al., 2017). Different light intensities influence the composition of important phytochemicals such as anthocyanins, carotenoids, chlorophylls, phenolics, and ascorbic acid in baby leaf lettuce (Li and Kubota, 2009; Samuoliene et al., 2013). Moreover, the soilless cultivation can be more efficient in term of nutrient requirements for vegetables growth than that of soil culture (Rouphael et al., 2004; Palermo et al., 2012). Although the metabolite composition of leaf lettuce grown under hydroponic cultivation has been investigated, a comparison of the primary and secondary metabolite profiles in lettuce grown under hydroponic- and soil cultivation conditions is still needed.
Metabolomics has been used to identify the effect of different cultivation environments on plants and for the quality evaluation of agricultural products (Kusano et al., 2011b; Zeng et al., 2014; Sung et al., 2015; Garcia et al., 2016; Ya-Qin et al., 2017). According to Lisec et al. (2006), plants can produce approximately 200,000 metabolites and specific metabolites are produced in different plant species (Kusano et al., 2011b, 2014a,b), even in cultivars and ecotypes (Sulpice et al., 2009). As mass spectrometry (MS)-based metabolomics yields highly sensitive results and facilitates high-throughput data acquisition, it has been combined with various types of analytical separation techniques, including gas chromatography (GC), liquid chromatography (LC), and capillary electrophoresis (CE) (Gowda and Djukovic, 2014). Since primary metabolites contribute to taste, integrated comprehensive GC-MS analysis and sensory evaluation were applied to determine the taste characteristics of green tea grown under various artificial light conditions, and bidimensional GC could provide extended metabolic phenotyping of natural variants in rice (Kusano et al., 2007; Miyauchi et al., 2017). LC-MS-based metabolite profiling aimed at detecting secondary metabolites including functional ingredients in foods and plants (Xie et al., 2008; Ma et al., 2013) revealed that environmental factors affected the accumulation of flavonoids in tea (Zhang et al., 2014). Comprehensive LC-MS analysis combined with ultra-high-performance LC (UHPLC) and quadrupole high-resolution time-of-flight MS (qTOF-MS) was used for secondary metabolite profiling to evaluate the quality of three artichoke cultivars and their commercial products (Farag et al., 2013). Furthermore, CE-MS-based metabolomics to analyze charged metabolites, i.e., amino acids and organic acids, has been utilized for quality control of agricultural products such as edamame beans, pork meat, and sake (rice spirit) (Sugimoto et al., 2010, 2012; Muroya et al., 2014). Additionally, the use of nuclear magnetic resonance (NMR) spectroscopy in plant metabolomics study also complements analytical technique choices in providing robust and reproducible metabolic data (Sobolev et al., 2005; Pereira et al., 2014; Sekiyama et al., 2017).
Metabolite profiling is a promising analytical method for the elucidation of taste characteristics and for the functional evaluation of agricultural products because unlike targeted analysis, it returns a variety of component profiles for many samples. Moreover, multi-MS-based metabolite profiling yields wider coverage for the detection of a large variety of metabolites than single chromatographic techniques combined with MS (Arbona et al., 2009; Lee et al., 2014; Sung et al., 2015). It not only detects variations in taste-related metabolites elicited by differences in cultivation conditions, but also contributes to the identification of quantitative changes in specialized metabolites in vegetables. Taken together, collection of metabolite alterations data of leaf lettuce from different cultivation systems is very important for clarifying their quality.
We performed GC-MS- and LC-MS analysis for the comprehensive capture of cultivation-specific- and taste-related metabolites in leaf lettuce (Figure 1). To differentiate the metabolite composition, including primary and secondary metabolites, of two leaf lettuce cultivars, black rose (BR) and red fire (RF), grown under hydroponic or fertilized soil conditions, including light sources and nutrient, we performed integrated MS-based metabolite profiling to capture metabolite signature for BR and RF, and thus to establish their quality indexes.
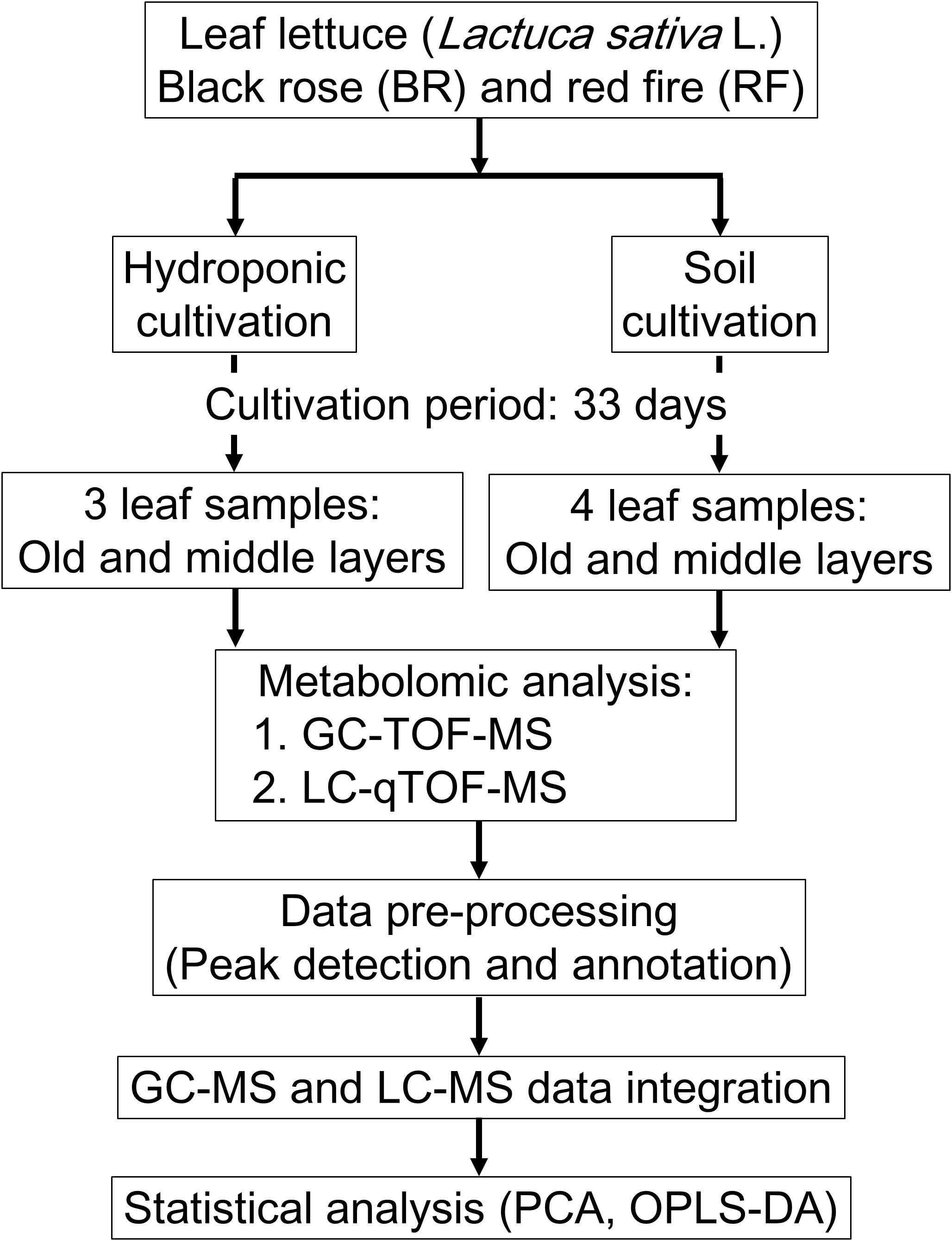
FIGURE 1. Workflow for the metabolomic evaluation of leaf lettuce grown under hydroponic- and soil cultivation methods. The leaf samples from hydroponic and soil cultivations were 3 and 4 biological replicates, respectively.
Materials and Methods
Plant Materials and Growth Condition
Two cultivars of L. sativa L. cv. BR and RF were grown for 33 days under hydroponic or soil conditions. The soil was treated with optimized concentrations of liquid fertilizer. The BR and RF seeds were purchased from Kaneko Seed Co. (Japan) and Takii Seed Co., Ltd. (Japan), respectively.
For hydroponic cultivation of BR and RF lettuce we applied the methods used by Keystone Technology Inc. (Japan). The conditions were: temperature, 22°C; 16 h light/8 h dark cycle (16 l/8 days); photosynthetic photon flux density (PPFD), 150 μmol m-2s-1 from light-emitting diode (LED) light (peak wavelength: blue = 460 nm, green = 525 nm, red = 660 nm). For soil cultivation the growth-chamber (LPH-350S, Nippon Medical and Chemical Instruments Co., Ltd., Japan) conditions were: temperature, 24°C; 16 l/8 days; PPFD, 140 μmol m-2 s-1 from white LED light, 2–3 times per week watering (Tamoi et al., 2017). The leaves of each plant were counted starting with the smallest leaf and the leaf growth stages were recorded as “young,” “middle” and “old” (Kusano et al., 2011a). The collected leaf samples were as follows: hydroponic cultivation; 2 cultivars × 2 sampling positions × 3 biological replicates, soil cultivation; 2 cultivars × 2 sampling positions × 4 biological replicates. In total, there were 28 leaf samples (Figure 1). Leaf samples were collected in bulk from 4 sites on leaves in the middle- and old stage using an 8-mm leaf punch disk (Fujiwara Scientific Company Co., Ltd., Japan) and immediately frozen in liquid nitrogen. Samples were stored at -80°C until analysis.
Metabolite Profiling by GC-TOF-MS
For the extraction of 25-mg leaf samples (fresh weight) we used 1 ml methanol/chloroform/H2O (3:1:1, v/v/v) containing 10 stable isotope references. The extraction was performed for 10 min at 15 Hz and 4°C using a mixer mill (Retsch MM301, Retsch GmbH, Germany) (Kusano et al., 2007). The mixture was then centrifuged, the supernatant was placed in a glass insert vial, and evaporated to dryness in a vacuum concentrator (Savant SPD2010 SpeedVac, Thermo Fisher Scientific Inc., United States). The dry extracts were derivatized using 30 μl of methoxylamine hydrochloride-HCl (20 mg/ml in pyridine) for 23 h at room temperature, then for 1 h in 30 μl of N-methyl-N-trimethylsilyltrifluoroacetamide (MSTFA) at 37°C with shaking; heptane (30 μl) was added to the extract mixture.
The extract (1 μl) was injected in into an Agilent 6890N GC instrument (Agilent Technologies, United States) via a CTC CombiPAL autosampler (CTC Analytics, Switzerland) for primary metabolite profiling. For separation we used an Rxi-5 Sil MS column [RESTEK, United States, inner diameter (ID), 30 m × 0.25 mm; film thickness, 0.25 μm]. Helium was the carrier gas delivered at a constant flow rate of 1 ml/min. The initial GC oven temperature was set at 80°C for 2 min, raised to 320°C at a rate of 30°C/min, and then held constant for 3.5 min. Data acquisition was performed on a Pegasus IV TOF MS instrument (LECO Corp., United States); the acquisition rate and range were 30 spectra/s and m/z 60–800, respectively. Alkane standard mixtures (C8–C20 and C21–C40, Sigma-Aldrich, Japan) were used for calculating the retention index (RI).
Metabolite Profiling by LC-TOF-MS
The dried samples were extracted (7 min, 18 Hz, 4°C) with 150 μl of 80% MeOH and zirconia beads containing 2.5 μM lidocaine and 10-Camphorsulfonic acid per mg dried weight using a mixer mill. Zirconia beads were used to increase extraction efficiency in particularly for cationic compounds, e.g., anthocyanins. After centrifugation, the supernatant was filtered using an Oasis HLB μElution plate (Waters Co., United States) (Nakabayashi et al., 2014). Extract (1 μl) was injected in into a Waters Acquity UPLC instrument coupled with a Waters Xevo G2 QTOF-MS instrument for metabolite profiling. The analytical conditions were LC column: Acquity-bridged ethyl hybrid (BEH) C18 (ID, 100 mm × 2.1 mm; 1.7 μm particle diameter; Waters); solvent system: solvent A (water including 0.1% formic acid); solvent B (acetonitrile including 0.1% formic acid); gradient program: 99.5% A/0.5% B, 0 min; 99.5% A/0.5% B, 0.1 min; 20% A/80% B, 10 min; 0.5% A/99.5% B, 10.1 min; 0.5% A/99.5% B, 12.0 min; 99.5% A/0.5% B, 12.1 min; 99.5% A/0.5% B, 15.0 min; flow rate: 0.3 ml/min at 0 min, 0.3 ml/min at 10 min, 0.4 ml/min at 10.1 min, 0.4 ml/min at 14.4 min, and 0.3 ml/min at 14.5 min; column temperature: 40°C; MS detection: capillary voltage, +3.0 keV; cone voltage, 25.0 V; source temperature, 120°C; desolvation temperature, 450°C; cone gas flow, 50 l/h; desolvation gas flow, 800 l/h; collision energy: 6 V; mass range: m/z 50–1500; scan duration: 0.1 s; interscan delay: 0.014 s; data acquisition: centroid mode; polarity: positive/negative; Lockspray (leucine enkephalin); scan duration: 1.0 s; interscan delay: 0.1 s. MS/MS data were acquired in ramp mode under the following analytical conditions: (1) MS: mass range, m/z 50–1500; scan duration, 0.1 s; inter-scan delay, 0.014 s; data acquisition, centroid mode; polarity, positive/negative; and (2) MS/MS: mass range, m/z 50–1500; scan duration, 0.02 s; inter-scan delay, 0.014 s; data acquisition, centroid mode; polarity, positive/negative; collision energy, ramped from 10–50 V. In this mode, MS/MS spectra of the top 10 ions (>1000 counts) in an MS scan were automatically obtained. If the ion intensity was below 1000, we did not perform MS/MS data acquisition but moved on to the next top 10 ions. Data acquisition was with Progenesis CoMet (Nonlinear Dynamics). Peak normalization was with lidocaine (positive mode) and 10-camphorsulfonic acid (negative mode).
Data Pre-processing
Non-processed data (NetCDF format) from GC-TOF-MS were exported to MATLAB (Mathworks, United States); custom scripts were used for data normalization, baseline correction, and subsequent analysis. Lastly, processed data obtained from hyphenated data analysis (HDA) were identified or annotated using an in-house metabolite library in PRIMe (Platform for RIKEN Metabolomics1), and the library in the Golm Metabolome Database (GMD) (Jonsson et al., 2005, 2006). Peaks were normalized with the cross-contribution compensating multiple standard normalization (CCMN) method (Redestig et al., 2009). Chemical assignment of data acquired from LC-TOF-MS was performed using reported MS- or MS/MS data (Wu and Prior, 2005; Abu-Reidah et al., 2013) and the KNApSAcK database (keyword: Lactuca sativa). The m/z values were set as monoisotopic masses [(M+H)+ or (M-H)-] under a tolerance match limit of 0.01 Da.
Statistical Analysis
To determine the effect of the different cultivation conditions on the metabolite profile of leaf lettuce we performed multivariate statistical analysis including principal component analysis (PCA) and orthogonal partial least square-discriminant analysis (OPLS-DA) of the unit variance scaled and log-10-transformed data matrix obtained from GC- and UPLC-TOF-MS using SIMCA-P 14 (Umetrics, Sweden). Significant metabolites in leaf lettuce were evaluated based on their differing variable importance in projection (VIP) values calculated with OPLS-DA and Q-values adjusted via the false discovery rate (FDR) approach (Benjamini and Hochberg, 1995) using R package samr2. The effect of the different cultivation methods on the resulting common metabolites in the cultivars was visualized on the Venn diagram (VENNY ver.2.13).
Results
Experimental Design
To investigate the effect of different cultivation conditions, cultivars, and leaf positions on the metabolite composition of leaf lettuce, we studied BR and RF cultivars raised for 33 days under the strictly controlled soil and hydroponic conditions (Table 1 and Figure 1). As metabolite profiles are largely affected the extent of visual phenotypes (Fiehn et al., 2000), we optimized soil condition for minimizing differences of visual phenotypes when compared to lettuce phenotypes harvested from the practical plat factory. The same type of liquid fertilizer was applied under both conditions and the light intensity was almost the same. Different concentration of the fertilizer was used for each cultivation because appearance and growth was different when we used the similar concentration for both cultivations (data not shown). Comparison of their phenotypes showed that the BR and RF lettuce leaves exposed to soil and hydroponic conditions were distinct (Figure 2). Soil-based cultivation yielded smaller colored leaves than hydroponic farming. Soil-grown BR and RF leaves were of the typical green color with dark- (BR) and bright-red pigments (RF) at the tip. For GC- and LC-MS, tissue samples from leaves in the old- and middle growth stage were collected from the same plant using an 8-mm leaf disk. The metabolites obtained by GC- and LC-MS were identified or annotated, and the metabolite profile data were then integrated for data interpretation by multivariate statistical analysis.
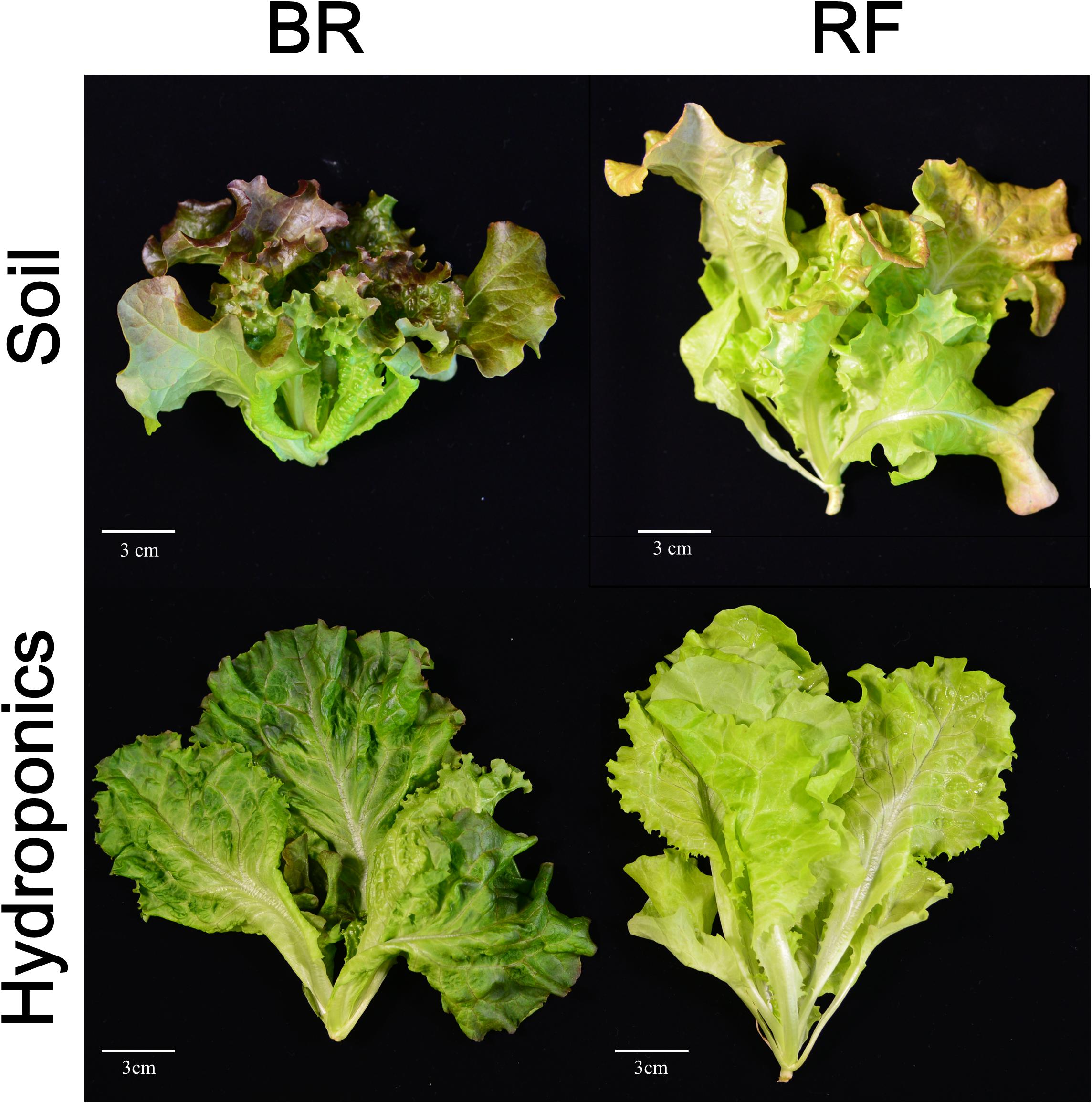
FIGURE 2. Black rose (BR) and red fire (RF) lettuce leaves grown under the two cultivation conditions. White bar, 3 cm.
Metabolite Profiling of Leaf Lettuce Grown Under Different Cultivation Conditions
To investigate metabolite changes, including primary and secondary metabolites, in BR and RF lettuce grown under different cultivation conditions, we harvested 28 leaf samples from each cultivar for comprehensive GC- and LC-MS analyses. GC-MS detected 223 peaks; 101 were identified- or provisionally identified primary metabolites. Positive- and negative-ionization mode-LC-MS followed by an MS/MS library search made it possible to annotate 2 of 30- and 30 of 65-detected compounds, respectively (Supplementary Table 1). Unsupervised multivariate statistical analysis by PCA was then performed to visualize the extent of metabolite changes elicited in the cultivars, under the different cultivation conditions, and in the leaf position.
In detail, “cultivation conditions” could differentiate on the first principal component, PC1 (22.9%), in which soil-based cultivation was recorded in the positive quadrant and hydroponic in the negative (Figure 3). Except for samples obtained from old-layer BR leaves grown hydroponically (H-BR), the plots of the other leaves were not clearly separated. This observation suggests that there is little difference in the metabolite composition of the cultivars and of leaves sampled at different growth stages. We next identified the discriminant metabolites in leaf lettuce by OPLS-DA. The score scatter plot in Figure 4A shows that the samples were clearly separated by the cultivation condition [PredComp1 = 20.5%, overall predictive performance of the model = R2 (Y): 0.99%, Q2 (cum) = 0.92%]. Therefore, the cultivation conditions had more impact on metabolite changes than the cultivar-type or the leaf growth stage.
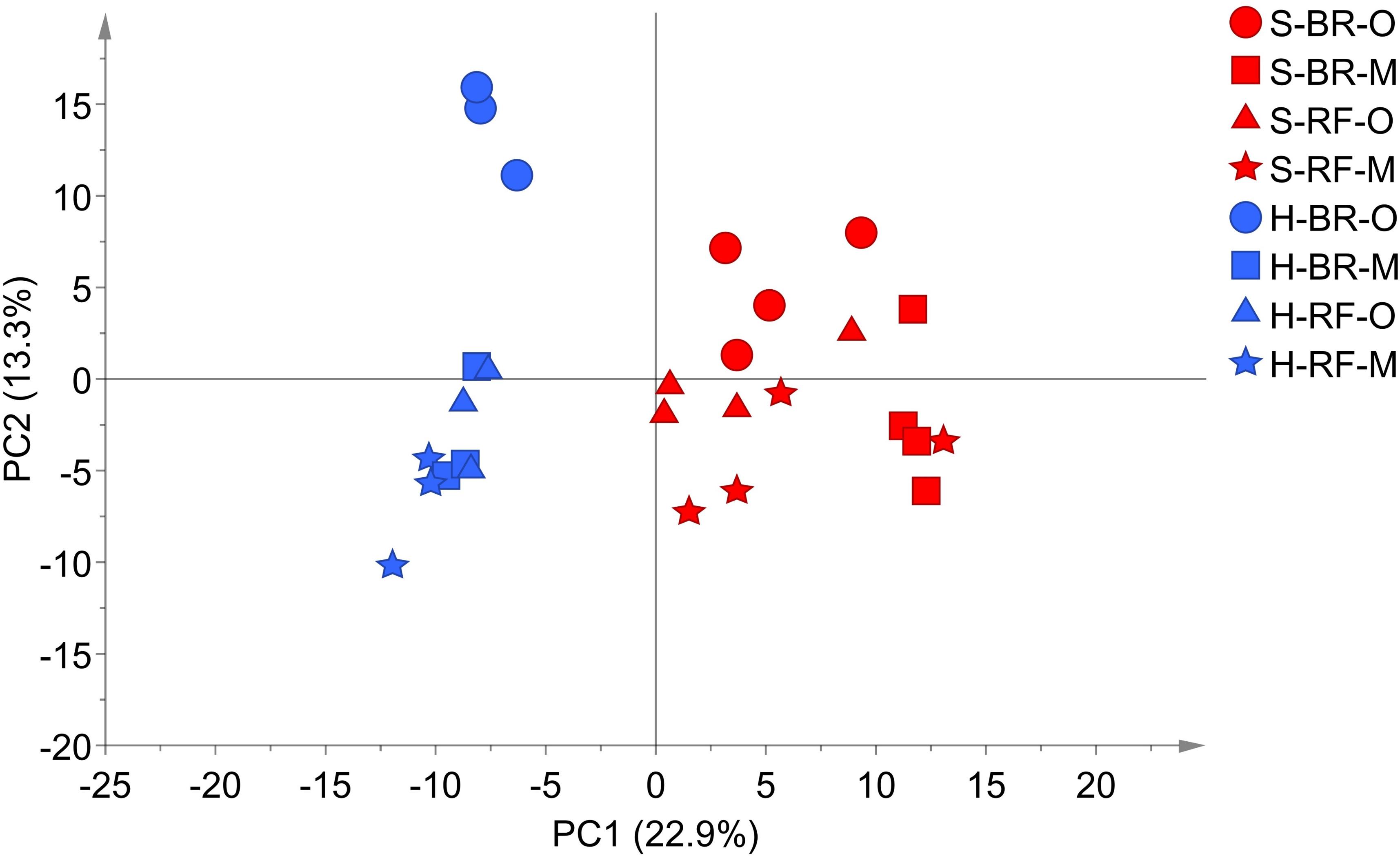
FIGURE 3. PCA score scatter plot of metabolites derived from black rose (BR) and red fire (RF) lettuce leaves (O, old layer; M, middle layer) grown under hydroponic (H) and soil (S) cultivation conditions. The leaf samples from hydroponic and soil cultivations were 3 and 4 biological replicates, respectively. The normalized data matrix (318 peak areas × 28 leaf samples) obtained from GC and LC-MS was used.
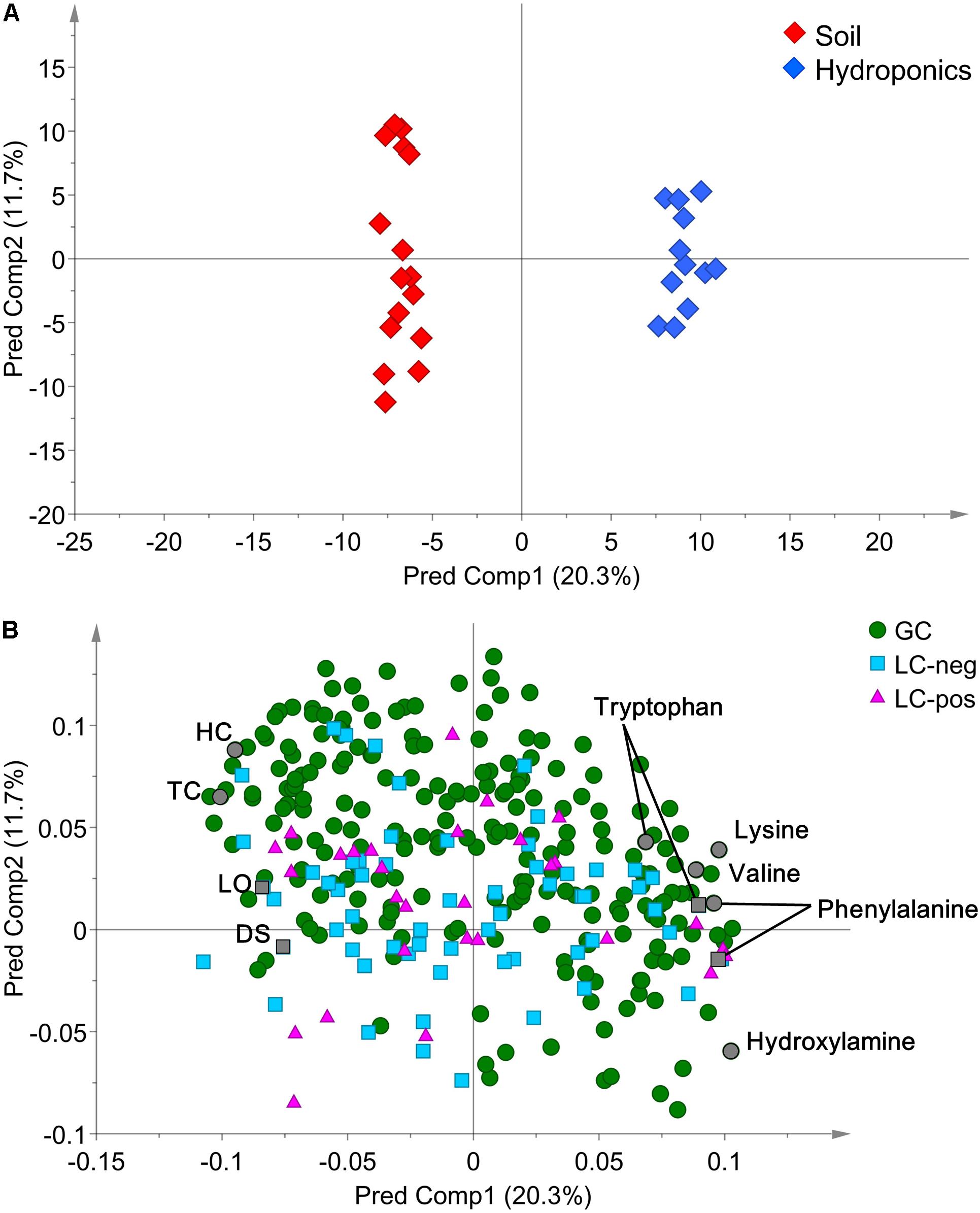
FIGURE 4. (A) OPLS-DA score scatter- and (B) loading plots of metabolites derived from 12 hydroponically- and 16 soil-grown lettuce leaves (number of samples: hydroponic cultivation; 2 cultivars × 2 sampling positions × 3 biological replicates, soil cultivation; 2 cultivars × 2 sampling positions × 4 biological replicates). The normalized data matrix (318 peak areas × 28 leaf samples) obtained by GC and LS-MS was used. HC, 1-hexacosanol; TC, 1-tetracosanol; LO, lactucopicrin-15-oxalate; DS, 15-deoxylactucin-8-sulfate.
Discriminant Metabolites in Soil- and Hydroponic-Cultivated Leaf Lettuce
The discriminant metabolites for our quality index of leaf lettuce were screened from all 318 metabolites based on the selection criteria of VIP>1.0 and FDR<0.05 (Figure 4B and Supplementary Table 2). We then compared changes in the metabolite profiles of hydroponically- and soil-grown lettuce. Leaves harvested after soil cultivation contained more typical lettuce sesquiterpene lactones and fatty acid-derived alcohols [log2-fold change (FC) ranging from -3.37 to -1.45] than hydroponically grown leaf lettuce which contained more amino acids (log2-FC ranging from 0.84 to 4.25).
In both cultivars we looked for metabolites that differed when they were grown under the two different conditions. We extracted discriminant metabolites that showed FDR<0.05 and |log2-FC| >1; our findings are presented in a Venn diagram (Figure 5 and Supplementary Tables 3, 4). When GC-MS and LC-MS detected the same metabolite, we selected the metabolite peak with the higher VIP value. The Venn diagram of metabolites that accumulated under hydroponic conditions (log2-FC>1) shows that common metabolites in BR and RF were amino acids (lysine, phenylalanine, pyroglutamate, tryptophan, and tyrosine) and phosphoric acid (Figure 5A). Soil-specific metabolites (log2-FC<-1) were sugars, organic acids, sesquiterpenes, and fatty acid-derived alcohols. Common metabolites in soil-grown BR and RF were arabinose, sucrose, myo-inositol, β-sitosterol, 1-hexacosanol, 1-tetracosanol, cystine, galactonic acid, galacturonic acid, and hydroxybenzoic acid (Figure 5B). The cultivar-specific metabolites in BR leaves grown hydroponically were amino acids (isoleucine, leucine and valine), caffeoylmalic acid, and coniferoside; in RF leaves grown hydroponically they were 2-propenoic acid and glutamate (Figure 5A). BR-specific metabolites obtained from plants grown in soil were sugars (erythritol and raffinose), organic acids (2-oxoglutaric, glutaric, and shikimic acids), and sesquiterpenes (lactucopicrin-15-oxalate and 15-deoxylactucin-8-sulfate); RF-specific metabolites included organic acids (glyceric and suberic acids) and phenolics (caffeoyltartaric-p-coumaroyl and p-coumaroylquinic acids) (Figure 5B). The different cultivation systems did not significantly alter the content of pigment and flavonol compounds in leaf lettuce, while these compounds tended to be accumulated in BR leaves (Figure 6). The application of the two cultivation methods could significantly differentiate (p < 0.05) taste-related compounds that might influence the sensory acceptance of lettuce, including glutamate (umami) (Halpern, 2000; Hounsome et al., 2008; Kurihara, 2009), sucrose (sweetness), and lactucopicrin-15-oxalate (bitterness) (Figure 6). Thoroughly, RF leaves from hydroponic cultivation had significantly more glutamate content than that of soil-cultivated RF leaves. Then again, both BR and RF leaves grown hydroponically had significantly less sucrose and lactucopicrin-15-oxalate levels than leaves from soil cultivation.
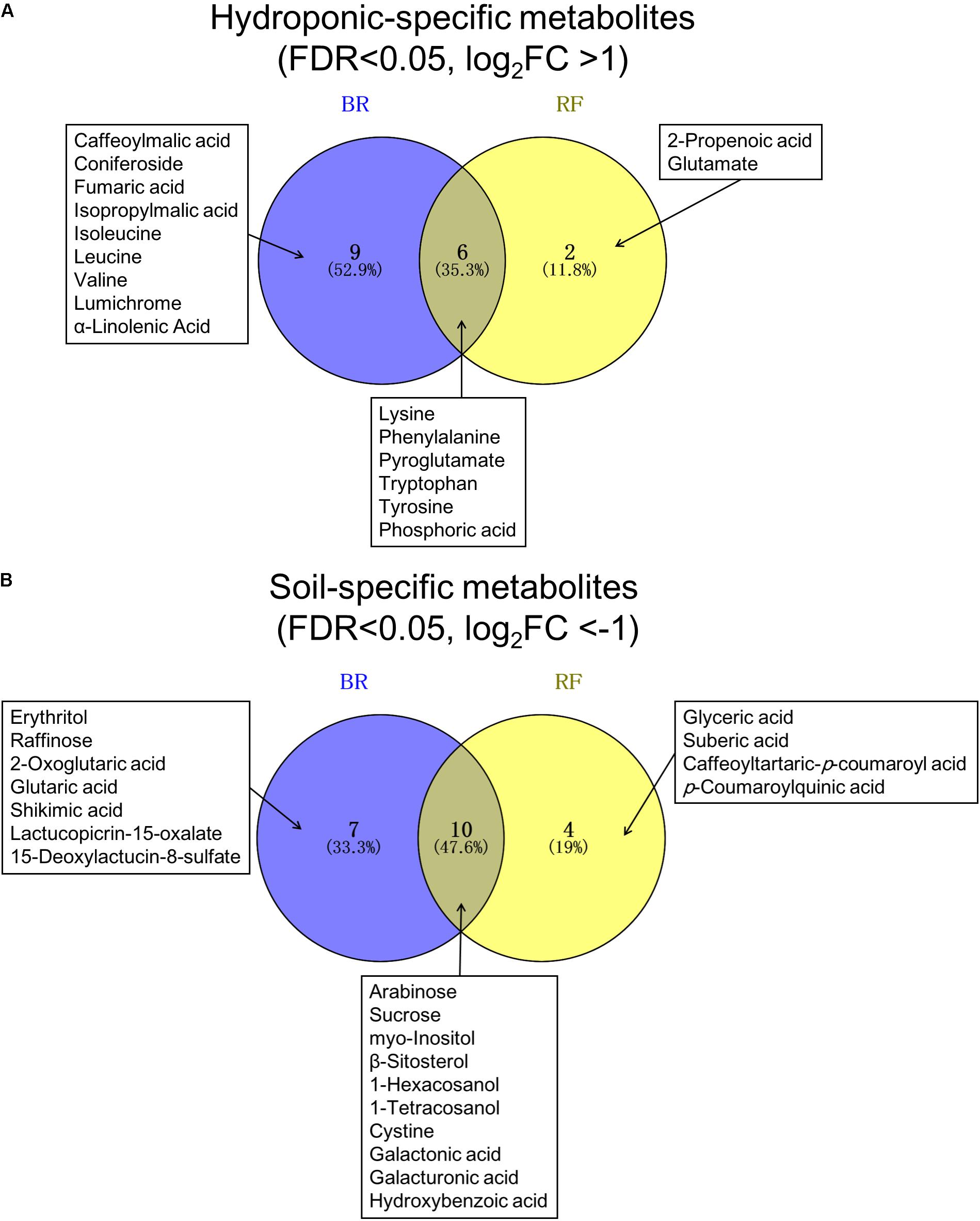
FIGURE 5. Venn diagram of (A) hydroponic-specific- and (B) soil-specific metabolites in black rose (BR) and red fire (RF) lettuce leaves. The false discovery rate (FDR) and log2-fold changes (FC) were calculated with soil cultivation as the control condition. Significant metabolites of the two lettuce cultivars were identified using a threshold of FDR<0.05 and | log2-FC| > 1.
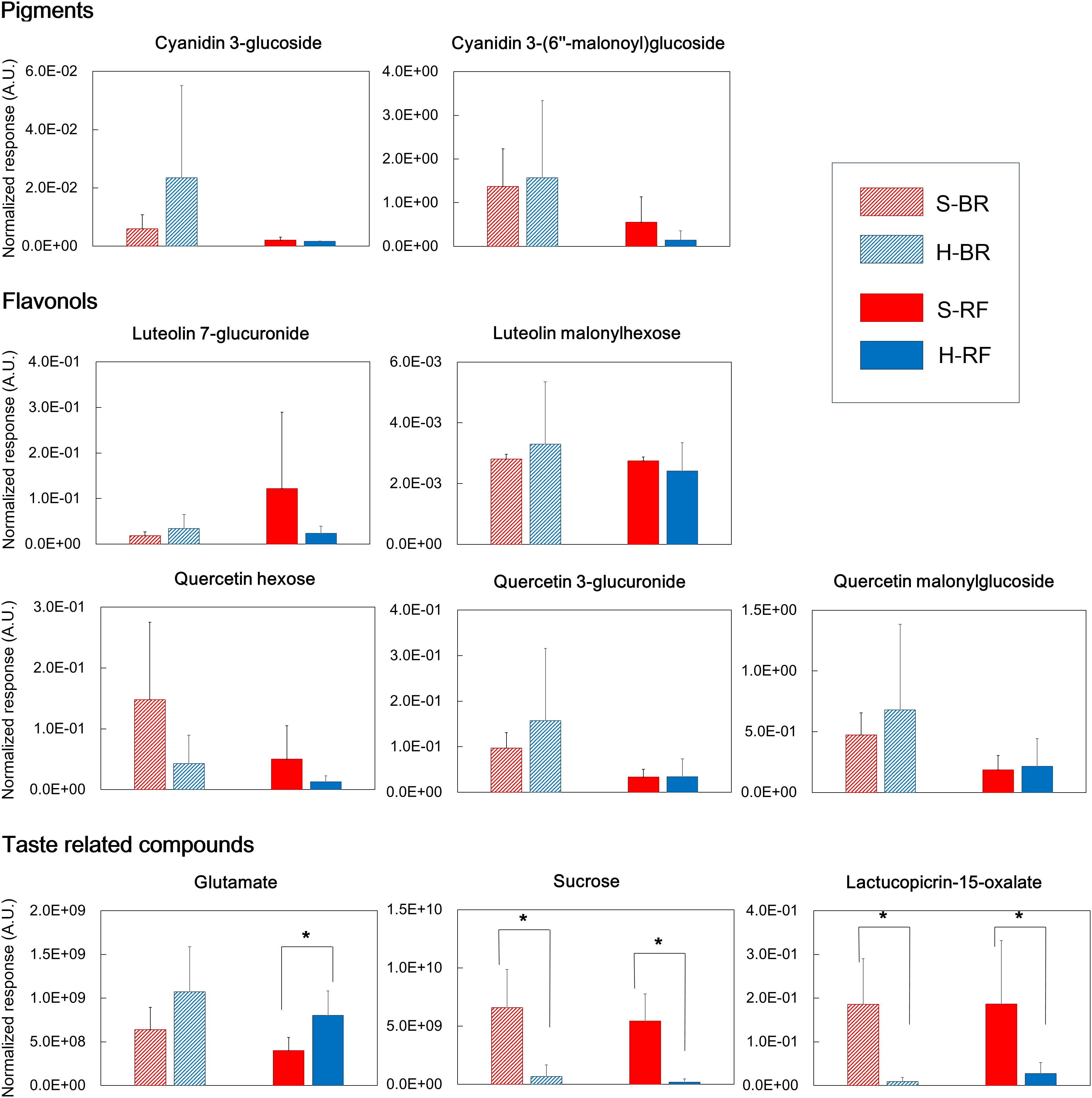
FIGURE 6. Quality index metabolites, represented by pigments, flavonols, and taste-related compounds, of Black rose (BR) and red fire (RF) lettuce leaves grown under hydroponic (H) and soil (S) cultivation conditions (number of samples: 6 × H-BR, 6 × H-RF, 8 × S-BR and 8 × S-RF). (∗indicates significant difference at p < 0.05 analyzed by Student’s t-test).
Discussion
Effect of Cultivation Conditions on Leaf Lettuce Growth
The leaves of BR and RF lettuce grown for 33 days in soil or under hydroponic conditions exhibited different morphological characteristics, i.e., their color and shape, but similar size (Figure 2). It has been reported that metabolite profiles largely affect from differences that is considered to be derived from difference of growth stages as well as visible phenotypes (Kusano et al., 2011a; Clevenger et al., 2015; Li et al., 2016). Soil-cultivated leaves had more reddish appearance than leaves grown hydroponically. Under both growth conditions the BR leaves were reddish and more darkly green than RF leaves, suggesting leaf pigmentation is affected by cultivar characteristics (Caldwell and Britz, 2006). According to Stutte et al. (2009) and Son and Oh (2013), the combination of red and blue LED light yields red leaf lettuce while the sole exposure to red LED light failed to yield pigmented leaves. These differences in appearance, including the leaf morphology and color, may influence sensorial preferences and the acceptance RF and BR lettuce (McWatters et al., 2002). As plant pigmentation reflects the level of chlorophyll and other secondary metabolites such as carotenoid and anthocyanin, we hypothesized that environmental- and cultivar-related factors impact the primary and secondary metabolite composition associated with metabolic pathways (Sass-Kiss et al., 2005; Caldwell and Britz, 2006). Therefore, we used comprehensive GC- and LC-MS-based metabolic profiling to identify specific metabolites related with the BR and RF lettuce phenotype except for chlorophylls and carotenoids. Because the MS-based metabolomics platform cannot detect these compounds.
Metabolomic Evaluation of the Quality of Leaf Lettuce Grown Under Different Cultivation Conditions
We demonstrate that the cultivation condition exerts greater influence on the metabolite composition of leaf lettuce than the cultivar type or the leaf position. Environmental factors of cultivation systems can contribute to the elicitation of taste- and nutrition-related compounds in various plants (Ohashi-Kaneko et al., 2007; Zhang et al., 2014). Okazaki et al. (2008), who examined the influence of the primary metabolite composition and of nitrogen availability in two spinach cultivars grown under different inorganic nitrogen concentrations, found that the efficiency of their nitrogen use was not the same.
We varied the light source intensity and the liquid fertilizer concentration in our study of RF and BR lettuce grown hydroponically and in soil. Miyagi et al. (2017) reported that red LED radiation combined with high concentrations of carbon dioxide and liquid fertilizer increased the biomass and the level of some amino acids in head lettuce grown hydroponically. Nitrogen, the main component of liquid fertilizer, impacts the plant metabolism. We found higher concentrations of amino acids (leucine, isoleucine, tryptophan, tyrosine, and phenylalanine) in the leaves of hydroponically- than soil-grown BR and RF lettuce. This phenomenon might also be affected by the applied nutritional values in both cultivation systems wherein more nitrogen nutrition was provided for hydroponic condition than in soil cultivation (Table 1). Due to the elicitation of higher amino acid levels, hydroponic cultivation may result in stronger “umami” perception than soil cultivation (Morris et al., 2007; Wang et al., 2016). On the other hand, the accumulation of sugars, fatty acid-derived alcohols, and β-sitosterol was lower in hydroponically- than soil-grown lettuce leaves. Our findings agree with earlier reports that the accumulation of sugars may be affected by environmental factors or stress such as light- and nutrient-conditions (Okazaki et al., 2008; Rosa et al., 2009; Miyagi et al., 2017). The level of leaf epidermal wax compounds such fatty acid-derived alcohols and β-sitosterol might be decreased in lettuce leaves grown in a hydroponic culture system (Baker et al., 1979). Also, the three-times shorter shelf life of hydroponically grown leaf lettuce may be attributable to lower dehydration (Manzocco et al., 2011), suggesting that the decreased level of fatty acids and sterols we observed in hydroponically grown lettuce contributed to the change in its leaf epidermal composition. Additionally, increased levels of fatty acids were considered to reflect stress of lettuce in soil (Yang and Ohlrogge, 2009).
To evaluate the effect of environmental growth conditions on RF and BR lettuces, we compared their metabolite profiles and extract metabolite signature for developing a quality index. Compared with hydroponically grown lettuce, the accumulation of sesquiterpene lactones and phenolics was markedly greater in BR- and RF-lettuce leaves harvested after soil cultivation. Seo et al. (2009) reported that the correlation between mineral nutrients intake and producing of sesquiterpene lactones in leaf lettuce. Exposure to short-wavelength light (blue LED and UV-B treatments) elicits an increase in phenolics, flavonoids, and the pigment content in lettuce leaves (Ouzounis et al., 2015) and in Arabidopsis thaliana (Kusano et al., 2011c) and our light conditions may have exerted a similar effect. Caldwell and Britz (2006) suggested that differences in the photosensitivity trait among cultivars changes the metabolite composition in leafy vegetables. The higher level of phenolics in soil- than hydroponically grown RF lettuce may increase its antioxidant and antimutagenic effects but render it more bitter-tasting and more astringent (Ren et al., 2001; Altunkaya et al., 2016; Filippo D’Antuono et al., 2016). BR-specific metabolites such as sesquiterpene lactones found in soil-grown plants may also enhance its bitter taste (Chadwick et al., 2016; Filippo D’Antuono et al., 2016). On the other hand, the sugar components in these soil-grown lettuces may mask their bitter taste and enrich their taste complexity (Beck et al., 2014; Chadwick et al., 2016).
Conclusion
Our study shows that the two cultivation methods greatly affect the metabolite profile of RF and BR leaf lettuce, including the metabolites responsible for their taste and their functional ingredients (e.g., amino acids and phenolic compounds). These metabolites may contribute to the overall quality and sensorial perception of leaf lettuce grown under hydroponic and soil cultivation methods (Manzocco et al., 2011; Mampholo et al., 2016). Metabolomic elucidations for lettuce have been conducted using various analytical techniques, including GC-MS, LC-MS, CE-MS, and NMR analyses (Pereira et al., 2014; Garcia et al., 2016; Zhao et al., 2016; Hurtado et al., 2017; Miyagi et al., 2017; Kitazaki et al., 2018). These techniques enabled to detect most of nutritional and pigment metabolites in the evaluated lettuce. Thus, metabolomic data can be used as an important basis for further detailed assessment of cultivation systems and horticulture products not only for lettuce, but for other vegetables. As the next step, it will be needed to evaluate which growth factor(s) are essential to affect phytochemical accumulation as well as yield in plant factories. We document that integrated metabolic profiling is a powerful tool for the comprehensively evaluation of the quality of leaf lettuce. Studies are underway to examine the effect of the light properties and of the liquid fertilizer concentration on the quality and nutritional value of leaf lettuce.
Author Contributions
MK initiated the study conception. MK, KS, and SO designed the analysis. YT performed the statistical analysis and drafted the manuscript. TM, RN, and MKo worked on data acquisition and analysis. MK supervised the study. SO and NW created and provided the samples. YT and MK wrote the manuscript and interpreted biological meaning of the results.
Funding
This research was partly supported by the “Sustainable Food Security Research Project” in the form of an operational grant from the National University Corporation.
Conflict of Interest Statement
The authors declare that the research was conducted in the absence of any commercial or financial relationships that could be construed as a potential conflict of interest.
Acknowledgments
We thank Drs. Thomas Moritz, Pär Jonsson, and Hans Stenlund (Umeå Plant Science Centre) for their assistance with data analysis and Dr. Yonathan Asikin (University of Tsukuba) for helpful comments on the manuscript.
Supplementary Material
The Supplementary Material for this article can be found online at: https://www.frontiersin.org/articles/10.3389/fpls.2018.00665/full#supplementary-material
Footnotes
- ^ http://prime.psc.riken.jp
- ^ https://cran.r-project.org/web/packages/samr/index.html
- ^ http://bioinfogp.cnb.csic.es/tools/venny/
References
Abu-Reidah, I. M., Contreras, M. M., Arráez-Román, D., Segura-Carretero, A., and Fernández-Gutiérrez, A. (2013). Reversed-phase ultra-high-performance liquid chromatography coupled to electrospray ionization-quadrupole-time-of-flight mass spectrometry as a powerful tool for metabolic profiling of vegetables: Lactuca sativa as an example of its application. J. Chromatogr. A 1313, 212–227. doi: 10.1016/j.chroma.2013.07.020
Altunkaya, A., Gökmen, V., and Skibsted, L. H. (2016). pH dependent antioxidant activity of lettuce (L. sativa) and synergism with added phenolic antioxidants. Food Chem. 190, 25–32. doi: 10.1016/j.foodchem.2015.05.069
Arbona, V., Iglesias, D. J., Talón, M., and Gómez-Cadenas, A. (2009). Plant phenotype demarcation using nontargeted LC-MS and GC-MS metabolite profiling. J. Agric. Food Chem. 57, 7338–7347. doi: 10.1021/jf9009137
Baker, E. A., Bukovac, M. J., and Flore, J. A. (1979). Ontogenetic variations in the composition of peach leaf wax. Phytochemistry 18, 781–784. doi: 10.1016/0031-9422(79)80013-8
Beck, T. K., Jensen, S., Bjoern, G. K., and Kidmose, U. (2014). The masking effect of sucrose on perception of bitter compounds in brassica vegetables. J. Sens. Stud. 29, 190–200. doi: 10.1111/joss.12094
Benjamini, Y., and Hochberg, Y. (1995). Controlling the false discovery rate: a practical and powerful approach to multiple testing. J. R. Stat. Soc. Series B Stat. Methodol. 57, 289–300.
Caldwell, C. R., and Britz, S. J. (2006). Effect of supplemental ultraviolet radiation on the carotenoid and chlorophyll composition of green house-grown leaf lettuce (Lactuca sativa L.) cultivars. J. Food Compost. Anal. 19, 637–644. doi: 10.1016/j.jfca.2005.12.016
Chadwick, M., Gawthrop, F., Michelmore, R. W., Wagstaff, C., and Methven, L. (2016). Perception of bitterness, sweetness and liking of different genotypes of lettuce. Food Chem. 197, 66–74. doi: 10.1016/j.foodchem.2015.10.105
Clevenger, J. P., Van Houten, J., Blackwood, M., Rodríguez, G. R., Jikumaru, Y., Kamiya, Y., et al. (2015). Network analyses reveal shifts in transcript profiles and metabolites that accompany the expression of SUN and an elongated tomato fruit. Plant Physiol. 168, 1164–1178. doi: 10.1104/pp.15.00379
Farag, M. A., El-ahmady, S. H., Elian, F. S., and Wessjohann, L. A. (2013). Metabolomics driven analysis of artichoke leaf and its commercial products via UHPLC–q-TOF-MS and chemometrics. Phytochemistry 95, 177–187. doi: 10.1016/j.phytochem.2013.07.003
Fiehn, O., Kopka, J., Dormann, P., Altmann, T., Trethewey, R. N., and Willmitzer, L. (2000). Metabolite profiling for plant functional genomics. Nat. Biotechnol. 18, 1157–1161. doi: 10.1038/81137
Filippo D’Antuono, L., Ferioli, F., and Manco, M. A. (2016). The impact of sesquiterpene lactones and phenolics on sensory attributes: an investigation of a curly endive and escarole germplasm collection. Food Chem. 199, 238–245. doi: 10.1016/j.foodchem.2015.12.002
Garcia, C. J., García-Villalba, R., Garrido, Y., Gil, M. I., and Tomás-Barberán, F. A. (2016). Untargeted metabolomics approach using UPLC-ESI-QTOF-MS to explore the metabolome of fresh-cut iceberg lettuce. Metabolomics 12, 1–13. doi: 10.1007/s11306-016-1082-x
Gowda, G. A. N., and Djukovic, D. (2014). Overview of Mass spectrometry-based metabolomics: opportunities and challenges. Methods Mol. Biol. 1198, 3–12. doi: 10.1007/978-1-4939-1258-2
Halpern, B. P. (2000). Glutamate and the flavor of foods. J. Nutr. 130, 921–926. doi: 10.1093/jn/130.4.910S
Hounsome, N., Hounsome, B., Tomos, D., and Edwards-Jones, G. (2008). Plant metabolites and nutritional quality of vegetables. J. Food Sci. 73, 48–65. doi: 10.1111/j.1750-3841.2008.00716.x
Hurtado, C., Parastar, H., Matamoros, V., Piña, B., Tauler, R., and Bayona, J. M. (2017). Linking the morphological and metabolomic response of Lactuca sativa L exposed to emerging contaminants using GC × GC-MS and chemometric tools. Sci. Rep. 7, 18–26. doi: 10.1038/s41598-017-06773-0
Jonsson, P., Johansson, A. I., Gullberg, J., Trygg, J., Aa, J., Grung, B., et al. (2005). High-throughput data analysis for detecting and identifying differences between samples in GC/MS-based metabolomic analyses. Anal. Chem. 77, 5635–5642. doi: 10.1021/ac050601e
Jonsson, P., Johansson, E. S., Wuolikainen, A., Lindberg, J., Schuppe-Koistinen, I., Kusano, M., et al. (2006). Predictive metabolite profiling applying hierarchical multivariate curve resolution to GC-MS data - A potential tool for multi-parametric diagnosis. J. Proteome Res. 5, 1407–1414. doi: 10.1021/pr0600071
Kitazaki, K., Fukushima, A., Nakabayashi, R., Okazaki, Y., Kobayashi, M., Mori, T., et al. (2018). Metabolic reprogramming in leaf lettuce grown under different light quality and intensity conditions using narrow-band LEDs. Sci. Rep. 8:7914. doi: 10.1038/s41598-018-25686-0
Kozai, T. (2013). Resource use efficiency of closed plant production system with artificial light: concept, estimation and application to plant factory. Proc. Jpn. Acad. Ser. B 89, 447–461. doi: 10.2183/pjab.89.447
Kurihara, K. (2009). Glutamate: from discovery as a food flavor to role as a basic taste (umami). Am. J. Clin. Nutr. 90, 1–3. doi: 10.3945/ajcn.2009.27462D
Kusano, M., Baxter, I., Fukushima, A., Oikawa, A., Okazaki, Y., Nakabayashi, R., et al. (2014a). Assessing metabolomic and chemical diversity of a soybean lineage representing 35 years of breeding. Metabolomics 261–270. doi: 10.1007/s11306-014-0702-6
Kusano, M., Fukushima, A., Kobayashi, M., Hayashi, N., Jonsson, P., Moritz, T., et al. (2007). Application of a metabolomic method combining one-dimensional and two-dimensional gas chromatography-time-of-flight/mass spectrometry to metabolic phenotyping of natural variants in rice. J. Chromatogr. B 855, 71–79. doi: 10.1016/j.jchromb.2007.05.002
Kusano, M., Jonsson, P., Fukushima, A., Gullberg, J., Sjöström, M., Trygg, J., et al. (2011a). Metabolite signature during short-day induced growth cessation in populus. Front. Plant Sci. 2:29. doi: 10.3389/fpls.2011.00029
Kusano, M., Redestig, H., Hirai, T., Oikawa, A., and Matsuda, F. (2011b). Covering chemical diversity of genetically-modified tomatoes using metabolomics for objective substantial equivalence assessment. PLoS One 6:e16989. doi: 10.1371/journal.pone.0016989
Kusano, M., Tohge, T., Fukushima, A., Kobayashi, M., Hayashi, N., Otsuki, H., et al. (2011c). Metabolomics reveals comprehensive reprogramming involving two independent metabolic responses of Arabidopsis to UV-B light. Plant J. 67, 354–369. doi: 10.1111/j.1365-313X.2011.04599.x
Kusano, M., Yang, Z., Okazaki, Y., Nakabayashi, R., Fukushima, A., and Saito, K. (2014b). Using metabolomic approaches to explore chemical diversity in rice. Mol. Plant 8, 58–67. doi: 10.1016/j.molp.2014.11.010
Lee, J., Jung, Y., Shin, J. H., Kim, H. K., Moon, B. C., Ryu, D. H., et al. (2014). Secondary metabolite profiling of curcuma species grown at different locations using GC/TOF and UPLC/Q-TOF MS. Molecules 19, 9535–9551. doi: 10.3390/molecules19079535
Li, L., Zhao, J., Zhao, Y., Lu, X., Zhou, Z., Zhao, C., et al. (2016). Comprehensive investigation of tobacco leaves during natural early senescence via multi-platform metabolomics analyses. Sci. Rep. 6:37976. doi: 10.1038/srep37976
Li, Q., and Kubota, C. (2009). Effects of supplemental light quality on growth and phytochemicals of baby leaf lettuce. Environ. Exp. Bot. 67, 59–64. doi: 10.1016/j.envexpbot.2009.06.011
Lisec, J., Schauer, N., Kopka, J., Willmitzer, L., and Fernie, A. R. (2006). Gas chromatography mass spectrometry – based metabolite profiling in plants. Nat. Protoc. 1, 387–396. doi: 10.1038/nprot.2006.59
Ma, C., Dastmalchi, K., Flores, G., Wu, S. B., Pedraza-Peñalosa, P., Long, C., et al. (2013). Antioxidant and metabolite profiling of North American and neotropical blueberries using LC-TOF-MS and multivariate analyses. J. Agric. Food Chem. 61, 3548–3559. doi: 10.1021/jf400515g
Mampholo, B. M., Maboko, M. M., Soundy, P., and Sivakumar, D. (2016). Phytochemicals and overall quality of leafy lettuce (Lactuca sativa L.) varieties grown in closed hydroponic system. J. Food Qual. 39, 805–815. doi: 10.1111/jfq.12234
Manzocco, L., Foschia, M., Tomasi, N., Maifreni, M., Dalla Costa, L., Marino, M., et al. (2011). Influence of hydroponic and soil cultivation on quality and shelf life of ready-to-eat lamb’s lettuce (Valerianella locusta L. Laterr). J. Sci. Food Agric. 91, 1373–1380. doi: 10.1002/jsfa.4313
McWatters, L. H., Chinnan, M. S., Walker, S. L., Doyle, M. P., and Lin, C. M. (2002). Consumer acceptance of fresh-cut iceberg lettuce treated with 2% hydrogen peroxide and mild heat. J. Food Prot. 65, 1221–1226.
Miyagi, A., Uchimiya, H., and Kawai-Yamada, M. (2017). Synergistic effects of light quality, carbon dioxide and nutrients on metabolite compositions of head lettuce under artificial growth conditions mimicking a plant factory. Food Chem. 218, 561–568. doi: 10.1016/j.foodchem.2016.09.102
Miyauchi, S., Yonetani, T., Yuki, T., Tomio, A., Bamba, T., and Fukusaki, E. (2017). Quality evaluation of green tea leaf cultured under artificial light condition using gas chromatography/mass spectrometry. J. Biosci. Bioeng. 123, 197–202. doi: 10.1016/j.jbiosc.2016.07.017
Morris, W. L., Ross, H. A., Ducreux, L. J. M., Bradshaw, J. E., Bryan, G. J., and Taylor, M. A. (2007). Umami compounds are a determinant of the flavor of potato (Solanum tuberosum L.). J. Agric. Food Chem. 55, 9627–9633. doi: 10.1021/jf0717900
Muroya, S., Oe, M., Nakajima, I., Ojima, K., and Chikuni, K. (2014). CE-TOF MS-based metabolomic profiling revealed characteristic metabolic pathways in postmortem porcine fast and slow type muscles. Meat Sci. 98, 726–735. doi: 10.1016/j.meatsci.2014.07.018
Nakabayashi, R., Yonekura-Sakakibara, K., Urano, K., Suzuki, M., Yamada, Y., Nishizawa, T., et al. (2014). Enhancement of oxidative and drought tolerance in Arabidopsis by overaccumulation of antioxidant flavonoids. Plant J. 77, 367–379. doi: 10.1111/tpj.12388
Ohashi-Kaneko, K., Takese, M., Kon, N., Fujiwara, K., and Kurata, K. (2007). Effect of light quality on growth and vegetable quality in leaf lettuce, spinach and komatsuna. Environ. Control Biol. 45, 189–198. doi: 10.2525/ecb.45.189
Okazaki, K., Oka, N., Shinano, T., Osaki, M., and Takebe, M. (2008). Differences in the metabolite profiles of spinach (Spinacia oleracea L.) leaf in different concentrations of nitrate in the culture solution. Plant Cell Physiol. 49, 170–177. doi: 10.1093/pcp/pcm173
Ouzounis, T., Razi Parjikolaei, B., Frette, X., Rosenqvist, E., and Ottosen, C. O. (2015). Predawn and high intensity application of supplemental blue light decreases the quantum yield of PSII and enhances the amount of phenolic acids, flavonoids, and pigments in Lactuca sativa. Front. Plant Sci. 6:19. doi: 10.3389/fpls.2015.00019
Palermo, M., Paradiso, R., Pascale, S., and Fogliano, V. (2012). Hydroponic cultivation improves the nutritional quality of soybean and its products. J. Agric. Food Chem. 55, 9627–9633. doi: 10.1021/jf203275m
Pereira, S. I., Figueiredo, P. I., Barros, A. S., Dias, M. C., Santos, C., Duarte, I. F., et al. (2014). Changes in the metabolome of lettuce leaves due to exposure to mancozeb pesticide. Food Chem. 154, 291–298. doi: 10.1016/j.foodchem.2014.01.019
Redestig, H., Fukushima, A., Stenlund, H., Moritz, T., Arita, M., Saito, K., et al. (2009). Compensation for systematic cross-contribution improves normalization of mass spectrometry based metabolomics data. Anal. Chem. 81, 7974–7980. doi: 10.1021/ac901143w
Ren, H., Endo, H., and Hayashi, T. (2001). Antioxidative and antimutagenic activities and polyphenol content of pesticide-free and organically cultivated green vegetables using water-soluble chitosan as a soil modifier and leaf surface spray. J. Sci. Food Agric. 81, 1426–1432. doi: 10.1002/jsfa.955
Rosa, M., Prado, C., Podazza, G., Interdonato, R., González, J. A., Hilal, M., et al. (2009). Soluble sugars-metabolism, sensing and abiotic stress a complex network in the life of plants. Plant Signal. Behav. 4, 388–393. doi: 10.4161/psb.4.5.8294
Rouphael, Y., Colla, G., Battistelli, A., Moscatello, S., Rea, E., Proietti, S., et al. (2004). Yield, water requirement, nutrient uptake and fruit quality of zucchini squash grown in soil and closed soilless culture. J. Hort. Sci. Biotechnol. 79, 423–431. doi: 10.1080/14620316.2004.11511784
Samuoliene, G., Brazaityte, A., Sirtautas, R., Viršile, A., Sakalauskaite, J., Sakalauskiene, S., et al. (2013). LED illumination affects bioactive compounds in romaine baby leaf lettuce. J. Sci. Food Agric. 93, 3286–3291. doi: 10.1002/jsfa.6173
Sass-Kiss, A., Kiss, J., Milotay, P., Kerek, M. M., and Toth-Markus, M. (2005). Differences in anthocyanin and carotenoid content of fruits and vegetables. Food Res. Int. 38, 1023–1029. doi: 10.1016/j.foodres.2005.03.014
Sekiyama, Y., Okazaki, K., Kikuchi, J., and Ikeda, S. (2017). NMR-based metabolic profiling of field-grown leaves from sugar beet plants harbouring different levels of resistance to cercospora leaf spot disease. Metabolites 7:4. doi: 10.3390/metabo7010004
Seo, M. W., Yang, D. S., Kays, S. J., Lee, G. P., and Park, K. W. (2009). Sesquiterpene lactones and bitterness in Korean leaf lettuce cultivars. HortScience 44, 246–249.
Sobolev, A. P., Brosio, E., Gianferri, R., and Segre, A. L. (2005). Metabolic profile of lettuce leaves by high-field NMR spectra. Magn. Reson. Chem. 43, 625–638. doi: 10.1002/mrc.1618
Son, K. H., and Oh, M. M. (2013). Leaf shape, growth, and antioxidant phenolic compounds of two lettuce cultivars grown under various combinations of blue and red light-emitting diodes. HortScience 48, 988–995.
Stutte, G. W., Edney, S., and Skerritt, T. (2009). Photoregulation of bioprotectant content of red leaf lettuce with light-emitting diodes. HortScience 44, 79–82.
Sugimoto, M., Goto, H., Otomo, K., Ito, M., Onuma, H., Suzuki, A., et al. (2010). Metabolomic profiles and sensory attributes of edamame under various storage duration and temperature conditions. J. Agric. Food Chem. 58, 8418–8425. doi: 10.1021/jf101471d
Sugimoto, M., Kaneko, M., Onuma, H., Sakaguchi, Y., Mori, M., Abe, S., et al. (2012). Changes in the charged metabolite and sugar profiles of pasteurized and unpasteurized Japanese sake with storage. J. Agric. Food Chem. 60, 2586–2593. doi: 10.1021/jf2048993
Sulpice, R., Pyl, E., Ishihara, H., Trenkamp, S., Steinfath, M., Witucka-wall, H., et al. (2009). Starch as a major integrator in the regulation of plant growth. Proc. Natl. Acad. Sci. U.S.A. 106, 10348–10353. doi: 10.1073/pnas.0903478106
Sung, J., Lee, S., Lee, Y., Ha, S., Song, B., Kim, T., et al. (2015). Metabolomic profiling from leaves and roots of tomato (Solanum lycopersicum L.) plants grown under nitrogen, phosphorus or potassium-deficient condition. Plant Sci. 241, 55–64. doi: 10.1016/j.plantsci.2015.09.027
Tamoi, M., Ishida, K., Eguchi, M., Harada, K., Kijihana, I., and Shigeoka, S. (2017). Growth and photosynthetic characteristics of arabidopsis and tobacco plants grown under LED lights “PLANT FLEC” (in Japanese with English summary). Shokubutsu Kankyo Kogaku 29, 96–103. doi: 10.2525/shita.29.96
Tokimasa, M., and Nishiura, Y. (2015). Automation in plant factory with labor-saving conveyance system. Environ. Control Biol. 53, 101–105. doi: 10.2525/ecb.53.101
Touliatos, D., Dodd, I. C., and Mcainsh, M. (2016). Vertical farming increases lettuce yield per unit area compared to conventional horizontal hydroponics. Food Energy Secur. 5, 184–191. doi: 10.1002/fes3.83
Wang, L., Xu, B., Li, L., Zhang, M., Feng, T., Wang, J., et al. (2016). Enhancement of umami taste of hydrolyzed protein from wheat gluten by β-cyclodextrin. J. Sci. Food Agric. 96, 4499–4504. doi: 10.1002/jsfa.7665
Wu, X., and Prior, R. L. (2005). Identification and characterization of anthocyanins by high-performance liquid chromatography-electrospray ionization-tandem mass spectrometry in common foods in the United States: vegetables, nuts, and grains. J. Agric. Food Chem. 53, 3101–3113. doi: 10.1021/jf0478861
Xie, G. X., Ni, Y., Su, M. M., Zhang, Y. Y., Zhao, A. H., Gao, X. F., et al. (2008). Application of ultra-performance LC-TOF MS metabolite profiling techniques to the analysis of medicinal Panax herbs. Metabolomics 4, 248–260. doi: 10.1007/s11306-008-0115-5
Yang, Z., and Ohlrogge, J. B. (2009). Turnover of fatty acids during natural senescence of Arabidopsis, brachypodium, and switchgrass and in arabidopsis -oxidation mutants. Plant Physiol. 150, 1981–1989. doi: 10.1104/pp.109.140491
Ya-Qin, W., Li-Ping, H., Guang-Min, L., De-Shuang, Z., and Hong-Ju, H. (2017). Evaluation of the nutritional quality of Chinese kale (Brassica alboglabra Bailey) using UHPLC-quadrupole-orbitrap MS/MS-based metabolomics. Molecules 22:E1262. doi: 10.3390/molecules22081262
Zeng, W., Hazebroek, J., Beatty, M., Hayes, K., Ponte, C., Maxwell, C., et al. (2014). Analytical method evaluation and discovery of variation within maize varieties in the context of food safety: transcript profiling and metabolomics. J. Agric. Food Chem. 62, 2997–3009. doi: 10.1021/jf405652j
Zhang, Q., Shi, Y., Ma, L., Yi, X., and Ruan, J. (2014). Metabolomic analysis using ultra-performance liquid chromatography-quadrupole-time of flight mass spectrometry (UPLC-Q-TOF MS) uncovers the effects of light intensity and temperature under shading treatments on the metabolites in tea. PLoS One 9:e112572. doi: 10.1371/journal.pone.0112572
Keywords: leaf lettuce, plant factory, hydroponic system, soil cultivation, metabolomics
Citation: Tamura Y, Mori T, Nakabayashi R, Kobayashi M, Saito K, Okazaki S, Wang N and Kusano M (2018) Metabolomic Evaluation of the Quality of Leaf Lettuce Grown in Practical Plant Factory to Capture Metabolite Signature. Front. Plant Sci. 9:665. doi: 10.3389/fpls.2018.00665
Received: 15 February 2018; Accepted: 30 April 2018;
Published: 27 June 2018.
Edited by:
Andreia Figueiredo, Universidade de Lisboa, PortugalReviewed by:
Francisco A. Tomas-Barberan, Consejo Superior de Investigaciones Científicas (CSIC), SpainJosep Maria Bayona, Consejo Superior de Investigaciones Científicas (CSIC), Spain
Luigi Lucini, Università Cattolica del Sacro Cuore, Italy
Copyright © 2018 Tamura, Mori, Nakabayashi, Kobayashi, Saito, Okazaki, Wang and Kusano. This is an open-access article distributed under the terms of the Creative Commons Attribution License (CC BY). The use, distribution or reproduction in other forums is permitted, provided the original author(s) and the copyright owner are credited and that the original publication in this journal is cited, in accordance with accepted academic practice. No use, distribution or reproduction is permitted which does not comply with these terms.
*Correspondence: Miyako Kusano, kusano.miyako.fp@u.tsukuba.ac.jp