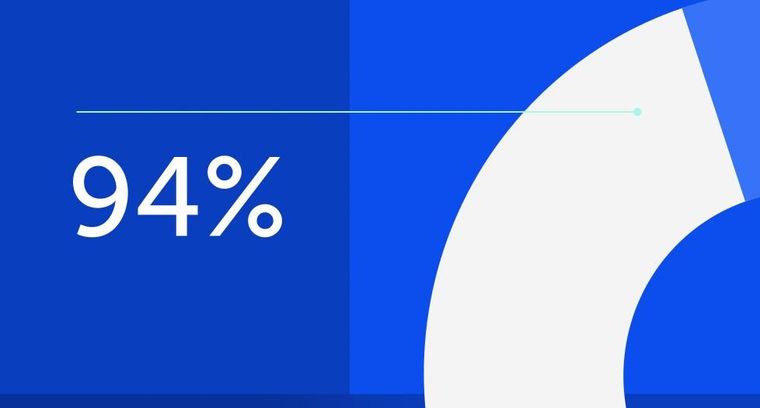
94% of researchers rate our articles as excellent or good
Learn more about the work of our research integrity team to safeguard the quality of each article we publish.
Find out more
ORIGINAL RESEARCH article
Front. Plant Sci., 24 January 2018
Sec. Plant Physiology
Volume 9 - 2018 | https://doi.org/10.3389/fpls.2018.00023
This article is part of the Research TopicHormonal Control of Important Agronomic TraitsView all 31 articles
Phytohormones regulate diverse aspects of plant growth and environmental responses. Recent high-throughput technologies have promoted a more comprehensive profiling of genes regulated by different hormones. However, these omics data generally result in large gene lists that make it challenging to interpret the data and extract insights into biological significance. With the rapid accumulation of theses large-scale experiments, especially the transcriptomic data available in public databases, a means of using this information to explore the transcriptional networks is needed. Different platforms have different architectures and designs, and even similar studies using the same platform may obtain data with large variances because of the highly dynamic and flexible effects of plant hormones; this makes it difficult to make comparisons across different studies and platforms. Here, we present a web server providing gene set-level analyses of Arabidopsis thaliana hormone responses. GSHR collected 333 RNA-seq and 1,205 microarray datasets from the Gene Expression Omnibus, characterizing transcriptomic changes in Arabidopsis in response to phytohormones including abscisic acid, auxin, brassinosteroids, cytokinins, ethylene, gibberellins, jasmonic acid, salicylic acid, and strigolactones. These data were further processed and organized into 1,368 gene sets regulated by different hormones or hormone-related factors. By comparing input gene lists to these gene sets, GSHR helped to identify gene sets from the input gene list regulated by different phytohormones or related factors. Together, GSHR links prior information regarding transcriptomic changes induced by hormones and related factors to newly generated data and facilities cross-study and cross-platform comparisons; this helps facilitate the mining of biologically significant information from large-scale datasets. The GSHR is freely available at http://bioinfo.sibs.ac.cn/GSHR/.
Phytohormones are typically small endogenous compounds regulating every aspect of plant life, from plant growth and development to responses to environmental changes (Santner and Estelle, 2009; Wolters and Jurgens, 2009; Scheres and van der Putten, 2017). Specific phytohormones are synthesized in response to distinct developmental or environmental cues, which are perceived by specific receptors and further activate cascades of signaling pathways, leading to remarkable biochemical and physiological changes (Santner and Estelle, 2009; Wolters and Jurgens, 2009; Scheres and van der Putten, 2017). The major hormones were traditionally classified into two categories, those mainly regulating developmental processes including auxin, brassinosteroid (BR), cytokinin (CK), and gibberellic acid (GA) and strigolactones, and those regulating stress responses, including abscisic acid (ABA), ethylene (ET), jasmonic acid (JA), and salicylic acid (SA). Over the past decades, wide-spread crosstalk was revealed among various phytohormone pathways in response to specific developmental or environmental changes (Depuydt and Hardtke, 2011; Robert-Seilaniantz et al., 2011; Murphy, 2015). Typical examples include the antagonistic role of GA and ABA in controlling seed dormancy (del Carmen Rodríguez-Gacio et al., 2009; Ye and Zhang, 2012; Shu et al., 2016), the interaction between auxin and CK in regulating meristem development (Kuderova and Hejatko, 2009; Su et al., 2011; Azizi et al., 2015). The crosstalk happens on multiple layers of regulation, among which transcriptional control is one major component.
Genetic studies identified various specific transcription factors mediating phytohormone signaling pathways; mutations in these factors resulted in plants that became more sensitive or insensitive to hormone treatments (Moore et al., 2011; Eckardt, 2015; Tsuda and Somssich, 2015). The interaction among factors regulating hormone signaling and factors controlling growth and responses mediates the crosstalk of different hormones in particular conditions. For example, the antagonistic interaction between auxin and CK in controlling root meristem development is achieved via the regulatory circuit between auxin signaling factors SHORT HYPOCOTYL 2 (SHY2/IAA3) and CK signaling regulator ARABIDOPSIS RESPONSE REGULATOR1 (ARR1) (Kuderova and Hejatko, 2009; Su et al., 2011). In another example, the central component regulating dark morphogenesis phytochrome interacting factor (PIF4) actively participated in auxin and BR-mediated pathways (Oh et al., 2012, 2014; Wang et al., 2014; Chaiwanon et al., 2016; Franciosini et al., 2017). To systematically characterize the crosstalk is non-trivial due to the complicated signaling network. The transcription of 100–1000 of genes display significant changes in response to various hormone treatments, and to dissect their common and unique targets is the pre-requisite for a comprehensive understanding of the crosstalk. During the past decades, high-throughput technologies have promoted systematic characterization of the signaling pathways triggered by various phytohormones and related factors. For example, microarray and RNA-seq experiments were performed to profile the transcriptomic changes triggered by phytohormones (Paponov et al., 2008; Garg et al., 2012) while chromatin immunoprecipitation followed by sequencing (ChIP-seq) studies have facilitated the identification of genes regulated by hormone-related factors (Oh et al., 2012, 2014; Song et al., 2016; Zhu et al., 2016). It was revealed that both phytohormones and related factors have wide-spread targets, and much research on phytohormones now involves investigating how plant hormones interact with each other and with related factors to orchestrate plant transcriptomic network (Depuydt and Hardtke, 2011; Eckardt, 2015). Accordingly, it is important to compare the high-throughput data obtained from different studies and from different platforms. However, such comparison is a challenging task for a number of reasons. Firstly, different platforms have different architectures and designs, and the results are not directly comparable (Barnes et al., 2005; Nookaew et al., 2012). For example, the probe intensity obtained from the Affymetrix platform cannot directly be compared to that from the Agilent platform due to different technical procedures such as the probe design, chip fabrication and data analysis (Sah et al., 2010; Del Vescovo et al., 2013); the results obtained from ChIP-seq experiments are not directly comparable to those from RNA-seq studies. Secondly, given the dynamic and flexible effects of phytohormones, similar experiments from different labs or even from different repeats in the same lab may generate data with large variances that are not readily comparable (Lee and Park, 2010; Rivas-San Vicente and Plasencia, 2011). To obtain more robust results, researchers proposed the idea of module-level analysis; this derives its power by focusing on gene sets sharing common biological functions or expression behavior. The basic procedure is to pre-define specific gene sets based on the comprehensive collection of information from dry-lab and wet-lab experiments. For any given gene list, the potential function of these genes could be deduced based on large amounts of prior information. Typical examples include Gene Set Enrichment Analysis (GSEA) and the Database for Annotation, Visualization and Integrated Discovery (DAVID), which are mostly for animals (Dennis et al., 2003; Subramanian et al., 2005), PlantGSEA for plants (Yi et al., 2013), and Comprehensive Annotation of Rice Omics-data (CARMO) for rice (Wang et al., 2015).
Given the vast amount of hormone-related transcriptomic data accumulated in public databases, the ability to make full use of available high-throughput data is of great significance. Here, we present gene set-level analyses of hormone responses in Arabidopsis (GSHR), a web server that provides analyses of phytohormone responses based on the integration of hormone-responsive gene modules. GSHR collected 333 RNA-seq and 1,205 microarray samples from the Gene Expression Omnibus that were organized into 1,368 gene lists regulated by different hormones or hormone-related factors. This gene list-level analysis helps reach more reliable and robust conclusions about which genes under study were affected by what types of plant hormones or related factors; three examples were used to illustrate the power of GSHR in data mining of hormone related information.
1,538 phytohormone-related transcriptomic datasets in Arabidopsis thaliana were collected from Gene Expression Omnibus (GEO1), including 73 studies with 1,205 datasets generated using microarray platforms (Affymetrix or Agilent), and 21 studies with 333 datasets generated by RNA-seq based on Illumina sequencing platform (Supplementary Table 1). All relevant comparisons between datasets of the same study were performed, then we defined the up-regulated and down-regulated genes in one pair comparison respectively as a gene set, finally generating 1,368 gene sets. We extracted the hormones or the transcription factors, mutants, or genes involved in hormone signaling used in the comparison as hormone or hormone-related factors of the gene set.
RNA-seq reads with MAPQ < 20 were filtered followed by adapter trimming using trim galore2 with default settings. The cleaned reads were mapped to Col-0 genome (Araport11) using STAR (Dobin et al., 2013), and the number of reads in each gene was counted. Differentially expressed genes were calculated via DEseq (Anders and Huber, 2010) with the combined criteria: |log2(fold change)| >1, p value<0.05.
Microarray data from Affymetrix platform were pre-processed with Bioconductor package affy (Gautier et al., 2004; Gentleman et al., 2004), and gcrma function was used for background correction and normalization (Wu and Irizarry, 2004). Microarray data from Agilent platform were normalized using quantile normalization (Bolstad et al., 2003). In the case of genes with multiple probes, the probe with the largest expression intensity was kept. For both platforms, linear model in Bioconductor package limma (Smyth, 2005) was applied for identifying differentially expressed genes with combined criteria:|log2 (fold change)| >1 and p value<0.01. Comparisons resulting in >10 differentially expressed genes were kept.
Fisher’s exact test (Routledge, 2005) was used for the enrichment analysis, which is displayed as follows:
where N is the total number of genes as background. Different N is used for different analysis. For comparison of gene sets from transcriptomic data, there are 24,327 genes in total; and the numbers for KEGG, gene ontology and InterPro domain analysis are 4,797, 30,468 and 15,816, respectively. n is the number of input genes. K is the total number of genes in one gene set and k strands for the overlapped genes between input and the pre-defined gene sets. For multiple testing correction, the adjusted P values calculated by FDR, Bonferroni correction and Benjamini and Hochberg method were provided.
The enriched fold change is the fraction of input genes involved in the given gene set divided by the fraction of all annotated genes involved in the gene set. This is commonly used for functional term enrichment analysis, e.g., DAVID bioinformatics Resources: https://david.ncifcrf.gov/term2term.jsp. The calculation formula is:
The values of N, n, k and K are descripted as above and presented in the download file, corresponding to PopTotal, ListTotal, Count and PopHits columns, respectively.
We selected 22,090 genes present in both microarray and RNA-seq datasets for construction of co-expression network. log2(fold change) of each gene from all pair-wise comparisons were collected, and the function rcorr in R package Hmisc (Harrell, 2017) was used to calculated the Pearson correlation coefficients and p-values between a pair of genes. The genes pairs with coefficients above 0.70 were used to construct the co-expression network.
The GSHR was constructed on Apache HTTP Server based on Linux system, MySQL was used for storage and operation of the database, the web interface is supported by PHP and JavaScript scripts. We used Python for statistical analysis and data processing, cluster analysis was performed in R. The JavaScript Library d3.js was used for co-expression network visualization. All scripts are available upon request.
GSHR integrates thousands of hormone-related transcriptomic data sets and offers a user-friendly interface for gene list-level analyses of user input gene lists (Figure 1). GSHR accepts gene lists with Arabidopsis thaliana Genbank IDs (Figure 2A) and returns the hormones and hormone-related factors potentially regulating the input gene list, ranked by statistical significance. Detailed descriptive and statistical information about the comparisons is also presented (Figures 2B–D). For those hormones regulated input gene lists, further functional exploration could be directly performed on the resulting page, including hierarchical clustering of expression pattern in response to related hormones, co-expression network, enrichment analyses of pathways, gene ontology terms and InterPro domains. The purpose is to facilitate a more comprehensive understanding about the input gene list, and helping pinpointing essential genes of interest from the input (Figure 2E and Supplementary Figure 1). The manual page of the website provides detailed guidelines.
FIGURE 2. Usage and features of GSHR. (A) Input page for submitting an Arabidopsis gene list. (B) Summary of the number of input genes and matched terms in the database. (C) Detailed annotation of input gene list. (D) Summary page of the transcriptomic comparisons closely relevant to the input gene list. The hormones or factors associated with the comparisons are listed in the 1st and 2nd columns. (E) Optional functional analyses of selected genes from the summary page shown in (D) including clustering and visualization of the expression pattern, co-expression network, enrichment analysis of pathways (KEGG), functions (Gene Ontology, GO) and domains (InterPro).
To illustrate the usage of GSHR, we used genes up-regulated by phosphate starvation in Arabidopsis thaliana roots as input (Sun et al., 2016). The output given by GSHR showed that a significant proportion of the input genes were regulated by ABA, auxin and ET (Figure 3A), indicating that these hormones were potentially involved in plant responses to phosphate deficiency. In support of the results, ABA-responsive genes were induced when cultured in low level of phosphate (Ciereszko and Kleczkowski, 2002; Chiou and Lin, 2011). In addition, low phosphorus enhanced the sensitivity of root response to auxin and ET in Arabidopsis, resulting in root architecture changes (Lopez-Bucio et al., 2002; Ma et al., 2003). Following identification of the relevant hormones, the enriched gene sets could be selected for further functional analyses. Clustering and visualization were provided to characterize their expression responses to selected hormones. The heatmap suggested that the selected gene sets were preferentially up-regulated by IAA and ABA, and both up and down-regulated genes were observed by treatment of ET (Figure 3B). In addition, genes with high degree in the co-expression network are good candidates of key factors linking signaling pathways between phosphate signaling and hormone responses (Figure 3C). Enriched GO terms include ET biosynthesis and ABA signaling pathway as expected (Figure 3D).
FIGURE 3. The resulting page summarizing relevant hormones and functional terms enriched for phosphate deficiency-induced genes. The most relevant hormones and factors (A), the clustering and visualization of the expression pattern in response to abscisic acid (ABA) and auxin (IAA) and ethylene (ET) (B), the co-expression network (C) and the enriched GO terms (D).
Cadmium is a toxic non-biodegradable heavy metal which negatively affect plant growth and development (Bucker-Neto et al., 2017). We used a set of cadmium-induced gene from Arabidopsis root as input to search for hormones involved in plant response to cadmium stress (Lopez-Martin et al., 2008). The GSHR identified SA, ABA, GA and JA as top enriched plant hormones (Figure 4A). This result is consistent with previous studies. SA influenced the absorption and transport of cadmium and acted as a signaling molecule activating cadmium-tolerant genes (Liu et al., 2016); JA could alleviate negative impacts of cadmium stress by increasing activities of antioxidant enzymes in soybean (Keramat et al., 2009); GA reduced nitric oxide accumulation in roots and suppressed cadmium uptake in Arabidopsis to alleviate cadmium toxicity (Zhu et al., 2012); ABA concentration was increased when exposed to cadmium, which induced transient MAP kinase activity thereby increasing cadmium tolerance (Burnett et al., 2000; Bucker-Neto et al., 2017). Further KEGG and GO enrichment results of selected gene sets indicated that these genes mainly related to plant circadian rhythm, starch and sucrose metabolism, ABA and stress response (Figures 4B,C), which were also reported to be involved in cadmium responses (Devi et al., 2007; Maistri et al., 2011).
FIGURE 4. The resulting page summarizing relevant hormones and functional terms enriched for cadmium stress response. The most relevant hormones and factors (A), the enriched pathways (B), and GO terms (C).
Besides differentially expressed genes from transcriptomic studies, GSHR accepts gene lists obtained from any source including different types of high-throughput data. Here, PIF4 target genes identified via ChIP-seq experiment (Pedmale et al., 2016) were used as input to illustrate this application of GSHR. AUXIN RESISTANT 2 (AXR2)- and BRASSINOSTEROID INSENSITIVE 1 (BRI1)-regulated genes were among the most enriched gene sets (Figure 5A). AXR2 belongs to the Auxin/Indole Acetic Acid protein family essential for mediating auxin signaling (Nagpal et al., 2000) that was also reported to be involved in the BR signaling network (Nemhauser et al., 2004; Nakamura et al., 2006). BRI1 is a leucine-rich repeat receptor kinase involved in BR signal transduction (Wang et al., 2001; Kinoshita et al., 2005). Consistent with the enrichment result, recent genetic and high-throughput studies revealed that PIF4 is closely associated with auxin- and BR-mediated signaling pathways. (Oh et al., 2012, 2014; Wang et al., 2014; Franciosini et al., 2017). PIF4 targets a significant proportion of the genes targeted by AUXIN RESPONSE FACTOR 6 (ARF6) and BRASSINAZOLE-RESISTANT 1 (BZR1), the core mediators of auxin and BR signaling, respectively (Chaiwanon et al., 2016). Input genes could be further clustered according to their expression changes in arx2 and bri1 mutants (Figure 5B).
FIGURE 5. The result pages summarizing the transcriptomic comparisons relevant to PIF4 target genes. The expression enrichment result (A) and the clustering analysis of the genes regulated by AXR2 and BRI1 (B).
GSHR provides data-driven analysis to search for hormones and hormone-related factors affecting transcription of input genes. The purpose of this platform is to link previously generated transcriptomic information to newly generated data, thereby helping to underpin biological insights from omics data and provide clues for subsequent research. This is different from traditional plant hormone databases that have mostly focused on providing comprehensive hormone-related information for individual genes (Peng et al., 2009; Jiang et al., 2011).
Focusing on gene sets facilitates comparison across different platforms and different experiments. However, pair-wise comparison generates multiple similar gene sets resulted from comparisons from similar experiments. The resulting page could be more concise via further clustering or removing redundant results. We choose to provide all pair-wise comparison results because different growth conditions and different way of treatments may affect non-identical gene sets, and all these information may be informative and helpful to users. With the rapid accumulation of high-throughput data, annotations, and references, the gene sets in GSHR will be updated annually.
XR and JL constructed the web server. XR, MQ, YW, and JC collected and processed public data. XR and YZ wrote the manuscript.
This work was supported by grants from National Natural Science Foundation of China (31570319 and 31770285), and sponsored by CAS Center for Excellence in Molecular Plant Sciences and Shanghai Institutes for Biological Sciences, Chinese Academy of Sciences.
The authors declare that the research was conducted in the absence of any commercial or financial relationships that could be construed as a potential conflict of interest.
The reviewer BS and handling Editor declared their shared affiliation.
The Supplementary Material for this article can be found online at: https://www.frontiersin.org/articles/10.3389/fpls.2018.00023/full#supplementary-material
Anders, S., and Huber, W. (2010). Differential expression analysis for sequence count data. Genome Biol. 11:R106. doi: 10.1186/gb-2010-11-10-r106
Azizi, P., Rafii, M. Y., Maziah, M., Abdullah, S. N. A., Hanafi, M. M., Latif, M. A., et al. (2015). Understanding the shoot apical meristem regulation: a study of the phytohormones, auxin and cytokinin, in rice. Mech. Dev. 135(Suppl. C), 1–15. doi: 10.1016/j.mod.2014.11.001
Barnes, M., Freudenberg, J., Thompson, S., Aronow, B., and Pavlidis, P. (2005). Experimental comparison and cross-validation of the Affymetrix and Illumina gene expression analysis platforms. Nucleic Acids Res. 33, 5914–5923. doi: 10.1093/nar/gki890
Bolstad, B. M., Irizarry, R. A., Astrand, M., and Speed, T. P. (2003). A comparison of normalization methods for high density oligonucleotide array data based on variance and bias. Bioinformatics 19, 185–193. doi: 10.1093/bioinformatics/19.2.185
Bucker-Neto, L., Paiva, A. L. S., Machado, R. D., Arenhart, R. A., and Margis-Pinheiro, M. (2017). Interactions between plant hormones and heavy metals responses. Genet. Mol. Biol. 40(1 Suppl. 1), 373–386. doi: 10.1590/1678-4685-gmb-2016-0087
Burnett, E., Desikan, R., Moser, R. C., and Neill, S. (2000). ABA activation of an MBP kinase in Pisum sativum epidermal peels correlates with stomatal responses to ABA. J. Exp. Bot. 51, 197–205. doi: 10.1093/jexbot/51.343.197
Chaiwanon, J., Wang, W., Zhu, J.-Y., Oh, E., and Wang, Z.-Y. (2016). Information integration and communication in plant growth regulation. Cell 164, 1257–1268. doi: 10.1016/j.cell.2016.01.044
Chiou, T. J., and Lin, S. I. (2011). Signaling network in sensing phosphate availability in plants. Annu. Rev. Plant Biol. 62, 185–206. doi: 10.1146/annurev-arplant-042110-103849
Ciereszko, I., and Kleczkowski, L. A. (2002). Effects of phosphate deficiency and sugars on expression of rab18 in Arabidopsis: hexokinase-dependent and okadaic acid-sensitive transduction of the sugar signal. Biochim. Biophys. Acta 1579, 43–49. doi: 10.1016/S0167-4781(02)00502-X
del Carmen Rodríguez-Gacio, M., Matilla-Vázquez, M. A., and Matilla, A. J. (2009). Seed dormancy and ABA signaling: the breakthrough goes on. Plant Signal. Behav. 4, 1035–1048. doi: 10.4161/psb.4.11.9902
Del Vescovo, V., Meier, T., Inga, A., Denti, M. A., and Borlak, J. (2013). A cross-platform comparison of affymetrix and Agilent microarrays reveals discordant miRNA expression in lung tumors of c-Raf transgenic mice. PLOS ONE 8:e78870. doi: 10.1371/journal.pone.0078870
Dennis, G., Sherman, B. T., Hosack, D. A., Yang, J., Gao, W., Lane, H. C., et al. (2003). DAVID: database for annotation, visualization, and integrated discovery. Genome Biol. 4:R60. doi: 10.1186/gb-2003-4-9-r60
Depuydt, S., and Hardtke, C. S. (2011). Hormone signalling crosstalk in plant growth regulation. Curr. Biol. 21, R365–R373. doi: 10.1016/j.cub.2011.03.013
Devi, R., Munjral, N., Gupta, A. K., and Kaur, N. (2007). Cadmium induced changes in carbohydrate status and enzymes of carbohydrate metabolism, glycolysis and pentose phosphate pathway in pea. Environ. Exp. Bot. 61, 167–174. doi: 10.1016/j.envexpbot.2007.05.006
Dobin, A., Davis, C. A., Schlesinger, F., Drenkow, J., Zaleski, C., Jha, S., et al. (2013). STAR: ultrafast universal RNA-seq aligner. Bioinformatics 29, 15–21. doi: 10.1093/bioinformatics/bts635
Eckardt, N. A. (2015). The Plant Cell reviews dynamic aspects of plant hormone signaling and crosstalk. Plant Cell 27, 1–2. doi: 10.1105/tpc.115.136291
Franciosini, A., Rymen, B., Shibata, M., Favero, D. S., and Sugimoto, K. (2017). Molecular networks orchestrating plant cell growth. Curr. Opin. Plant Biol. 35, 98–104. doi: 10.1016/j.pbi.2016.11.010
Garg, R., Tyagi, A. K., and Jain, M. (2012). Microarray analysis reveals overlapping and specific transcriptional responses to different plant hormones in rice. Plant Signal. Behav. 7, 951–956. doi: 10.4161/psb.20910
Gautier, L., Cope, L., Bolstad, B. M., and Irizarry, R. A. (2004). affy—analysis of Affymetrix GeneChip data at the probe level. Bioinformatics 20, 307–315. doi: 10.1093/bioinformatics/btg405
Gentleman, R. C., Carey, V. J., Bates, D. M., Bolstad, B., Dettling, M., Dudoit, S., et al. (2004). Bioconductor: open software development for computational biology and bioinformatics. Genome Biol. 5:R80. doi: 10.1186/gb-2004-5-10-r80
Harrell, F. E. Jr. (2017). Package ‘Hmisc’. R Foundation for Statistical Computing. Available at: http://biostat.mc.vanderbilt.edu/Hmisc
Jiang, Z., Liu, X., Peng, Z., Wan, Y., Ji, Y., He, W., et al. (2011). AHD2.0: an update version of Arabidopsis Hormone Database for plant systematic studies. Nucleic Acids Res. 39, D1123–D1129. doi: 10.1093/nar/gkq1066
Keramat, B., Manouchehri Kalantari, K., and Javad Arvin, M. (2009). Effects of methyl jasmonate in regulating cadmium induced oxidative stress in soybean plant (Glycine max L.). Afr. J. Microbiol. Res. 5, 240–244.
Kinoshita, T., Cano-Delgado, A., Seto, H., Hiranuma, S., Fujioka, S., Yoshida, S., et al. (2005). Binding of brassinosteroids to the extracellular domain of plant receptor kinase BRI1. Nature 433, 167–171. doi: 10.1038/nature03227
Kuderova, A., and Hejatko, J. (2009). Spatiotemporal aspect of cytokinin-auxin interaction in hormonal regulation of the root meristem. Plant Signal. Behav. 4, 156–157. doi: 10.4161/psb.4.2.7685
Lee, S., and Park, C.-M. (2010). Modulation of reactive oxygen species by salicylic acid in Arabidopsis seed germination under high salinity. Plant Signal. Behav. 5, 1534–1536. doi: 10.4161/psb.5.12.13159
Liu, Z., Ding, Y., Wang, F., Ye, Y., and Zhu, C. (2016). Role of salicylic acid in resistance to cadmium stress in plants. Plant Cell Rep. 35, 719–731. doi: 10.1007/s00299-015-1925-3
Lopez-Bucio, J., Hernandez-Abreu, E., Sanchez-Calderon, L., Nieto-Jacobo, M. F., Simpson, J., and Herrera-Estrella, L. (2002). Phosphate availability alters architecture and causes changes in hormone sensitivity in the Arabidopsis root system. Plant Physiol. 129, 244–256. doi: 10.1104/pp.010934
Lopez-Martin, M. C., Becana, M., Romero, L. C., and Gotor, C. (2008). Knocking out cytosolic cysteine synthesis compromises the antioxidant capacity of the cytosol to maintain discrete concentrations of hydrogen peroxide in Arabidopsis. Plant Physiol. 147, 562–572. doi: 10.1104/pp.108.117408
Ma, Z., Baskin, T. I., Brown, K. M., and Lynch, J. P. (2003). Regulation of root elongation under phosphorus stress involves changes in ethylene responsiveness. Plant Physiol. 131, 1381–1390. doi: 10.1104/pp.012161
Maistri, S., DalCorso, G., Vicentini, V., and Furini, A. (2011). Cadmium affects the expression of ELF4, a circadian clock gene in Arabidopsis. Environ. Exp. Bot. 72, 115–122. doi: 10.1016/j.envexpbot.2011.02.010
Moore, J. W., Loake, G. J., and Spoel, S. H. (2011). Transcription dynamics in plant immunity. Plant Cell 23, 2809–2820. doi: 10.1105/tpc.111.087346
Nagpal, P., Walker, L. M., Young, J. C., Sonawala, A., Timpte, C., Estelle, M., et al. (2000). AXR2 encodes a member of the Aux/IAA protein family. Plant Physiol. 123, 563–574. doi: 10.1104/pp.123.2.563
Nakamura, A., Nakajima, N., Goda, H., Shimada, Y., Hayashi, K., Nozaki, H., et al. (2006). Arabidopsis Aux/IAA genes are involved in brassinosteroid-mediated growth responses in a manner dependent on organ type. Plant J. 45, 193–205. doi: 10.1111/j.1365-313X.2005.02582.x
Nemhauser, J. L., Mockler, T. C., and Chory, J. (2004). Interdependency of brassinosteroid and auxin signaling in Arabidopsis. PLOS Biol. 2:E258. doi: 10.1371/journal.pbio.0020258
Nookaew, I., Papini, M., Pornputtapong, N., Scalcinati, G., Fagerberg, L., Uhlén, M., et al. (2012). A comprehensive comparison of RNA-Seq-based transcriptome analysis from reads to differential gene expression and cross-comparison with microarrays: a case study in Saccharomyces cerevisiae. Nucleic Acids Res. 40, 10084–10097. doi: 10.1093/nar/gks804
Oh, E., Zhu, J. Y., Bai, M. Y., Arenhart, R. A., Sun, Y., and Wang, Z. Y. (2014). Cell elongation is regulated through a central circuit of interacting transcription factors in the Arabidopsis hypocotyl. Elife 3:e03031. doi: 10.7554/eLife.03031
Oh, E., Zhu, J. Y., and Wang, Z. Y. (2012). Interaction between BZR1 and PIF4 integrates brassinosteroid and environmental responses. Nat. Cell Biol. 14, 802–809. doi: 10.1038/ncb2545
Paponov, I. A., Paponov, M., Teale, W., Menges, M., Chakrabortee, S., Murray, J. A. H., et al. (2008). Comprehensive transcriptome analysis of auxin responses in Arabidopsis. Mol. Plant 1, 321–337. doi: 10.1093/mp/ssm021
Pedmale, U. V., Huang, S. S., Zander, M., Cole, B. J., Hetzel, J., Ljung, K., et al. (2016). Cryptochromes interact directly with PIFs to control plant growth in limiting blue light. Cell 164, 233–245. doi: 10.1016/j.cell.2015.12.018
Peng, Z. Y., Zhou, X., Li, L., Yu, X., Li, H., Jiang, Z., et al. (2009). Arabidopsis Hormone Database: a comprehensive genetic and phenotypic information database for plant hormone research in Arabidopsis. Nucleic Acids Res. 37, D975–D982. doi: 10.1093/nar/gkn873
Rivas-San Vicente, M., and Plasencia, J. (2011). Salicylic acid beyond defence: its role in plant growth and development. J. Exp. Bot. 62, 3321–3338. doi: 10.1093/jxb/err031
Robert-Seilaniantz, A., Grant, M., and Jones, J. D. G. (2011). Hormone crosstalk in plant disease and defense: more than just jasmonate-salicylate antagonism. Annu. Rev. Phytopathol. 49, 317–343. doi: 10.1146/annurev-phyto-073009-114447
Routledge, R. (2005). “Fisher’s exact test,” in Encyclopedia of Biostatistics, eds P. Armitage and T. Colton (Hoboken, NJ: John Wiley & Sons).
Sah, S., McCall, M. N., Eveleigh, D., Wilson, M., and Irizarry, R. A. (2010). Performance evaluation of commercial miRNA expression array platforms. BMC Res. Notes 3:80. doi: 10.1186/1756-0500-3-80
Santner, A., and Estelle, M. (2009). Recent advances and emerging trends in plant hormone signalling. Nature 459, 1071–1078. doi: 10.1038/nature08122
Scheres, B., and van der Putten, W. H. (2017). The plant perceptron connects environment to development. Nature 543, 337–345. doi: 10.1038/nature22010
Shu, K., Liu, X.-D., Xie, Q., and He, Z.-H. (2016). Two faces of one seed: hormonal regulation of dormancy and germination. Mol. Plant 9, 34–45. doi: 10.1016/j.molp.2015.08.010
Smyth, G. K. (2005). “Limma: linear models for microarray data,” in Bioinformatics and Computational Biology Solutions Using R and Bioconductor, eds R. Gentleman, V. J. Carey, W. Huber, R. A. Irizarry, and S. Dudoit (New York, NY: Springer), 397–420. doi: 10.1007/0-387-29362-0_23
Song, L., Huang, S.-S. C., Wise, A., Castanon, R., Nery, J. R., Chen, H., et al. (2016). A transcription factor hierarchy defines an environmental stress response network. Science 354:aag1550. doi: 10.1126/science.aag1550
Su, Y. H., Liu, Y. B., and Zhang, X. S. (2011). Auxin-cytokinin interaction regulates meristem development. Mol. Plant 4, 616–625. doi: 10.1093/mp/ssr007
Subramanian, A., Tamayo, P., Mootha, V. K., Mukherjee, S., Ebert, B. L., Gillette, M. A., et al. (2005). Gene set enrichment analysis: a knowledge-based approach for interpreting genome-wide expression profiles. Proc. Natl. Acad. Sci. U.S.A. 102, 15545–15550. doi: 10.1073/pnas.0506580102
Sun, L., Song, L., Zhang, Y., Zheng, Z., and Liu, D. (2016). Arabidopsis PHL2 and PHR1 Act redundantly as the key components of the central regulatory system controlling transcriptional responses to phosphate starvation. Plant Physiol. 170, 499–514. doi: 10.1104/pp.15.01336
Tsuda, K., and Somssich, I. E. (2015). Transcriptional networks in plant immunity. New Phytol. 206, 932–947. doi: 10.1111/nph.13286
Wang, J., Qi, M., Liu, J., and Zhang, Y. (2015). CARMO: a comprehensive annotation platform for functional exploration of rice multi-omics data. Plant J. 83, 359–374. doi: 10.1111/tpj.12894
Wang, W., Bai, M.-Y., and Wang, Z.-Y. (2014). The brassinosteroid signaling network—a paradigm of signal integration. Curr. Opin. Plant Biol. 21, 147–153. doi: 10.1016/j.pbi.2014.07.012
Wang, Z. Y., Seto, H., Fujioka, S., Yoshida, S., and Chory, J. (2001). BRI1 is a critical component of a plasma-membrane receptor for plant steroids. Nature 410, 380–383. doi: 10.1038/35066597
Wolters, H., and Jurgens, G. (2009). Survival of the flexible: hormonal growth control and adaptation in plant development. Nat. Rev. Genet. 10, 305–317. doi: 10.1038/nrg2558
Wu, Z., and Irizarry, R. A. (2004). Preprocessing of oligonucleotide array data. Nat. Biotechnol. 22, 656–658. doi: 10.1038/nbt0604-656b
Ye, N., and Zhang, J. (2012). Antagonism between abscisic acid and gibberellins is partially mediated by ascorbic acid during seed germination in rice. Plant Signal. Behav. 7, 563–565. doi: 10.4161/psb.19919
Yi, X., Du, Z., and Su, Z. (2013). PlantGSEA: a gene set enrichment analysis toolkit for plant community. Nucleic Acids Res. 41, W98–W103. doi: 10.1093/nar/gkt281
Zhu, J. Y., Oh, E., Wang, T., and Wang, Z. Y. (2016). TOC1-PIF4 interaction mediates the circadian gating of thermoresponsive growth in Arabidopsis. Nat. Commun. 7, 13692. doi: 10.1038/ncomms13692
Keywords: GSHR, Arabidopsis thaliana, web-based platform, hormone, transcriptome, gene set-level, cross-study, cross-platform
Citation: Ran X, Liu J, Qi M, Wang Y, Cheng J and Zhang Y (2018) GSHR, a Web-Based Platform Provides Gene Set-Level Analyses of Hormone Responses in Arabidopsis. Front. Plant Sci. 9:23. doi: 10.3389/fpls.2018.00023
Received: 29 June 2017; Accepted: 08 January 2018;
Published: 24 January 2018.
Edited by:
Diwakar Shukla, University of Illinois at Urbana–Champaign, United StatesReviewed by:
Manpreet Singh Katari, New York University, United StatesCopyright © 2018 Ran, Liu, Qi, Wang, Cheng and Zhang. This is an open-access article distributed under the terms of the Creative Commons Attribution License (CC BY). The use, distribution or reproduction in other forums is permitted, provided the original author(s) or licensor are credited and that the original publication in this journal is cited, in accordance with accepted academic practice. No use, distribution or reproduction is permitted which does not comply with these terms.
*Correspondence: Yijing Zhang, emhhbmd5aWppbmdAc2licy5hYy5jbg==
Disclaimer: All claims expressed in this article are solely those of the authors and do not necessarily represent those of their affiliated organizations, or those of the publisher, the editors and the reviewers. Any product that may be evaluated in this article or claim that may be made by its manufacturer is not guaranteed or endorsed by the publisher.
Research integrity at Frontiers
Learn more about the work of our research integrity team to safeguard the quality of each article we publish.