- Institute of Crop Sciences, National Key Facility for Crop Gene Resources and Genetic Improvement, Chinese Academy of Agricultural Sciences, Beijing, China
Maize grain yield and related traits are complex and are controlled by a large number of genes of small effect or quantitative trait loci (QTL). Over the years, a large number of yield-related QTLs have been identified in maize and deposited in public databases. However, integrating and re-analyzing these data and mining candidate loci for yield-related traits has become a major issue in maize. In this study, we collected information on QTLs conferring maize yield-related traits from 33 published studies. Then, 999 of these QTLs were iteratively projected and subjected to meta-analysis to obtain metaQTLs (MQTLs). A total of 76 MQTLs were found across the maize genome. Based on a comparative genomics strategy, several maize orthologs of rice yield-related genes were identified in these MQTL regions. Furthermore, three potential candidate genes (Gene ID: GRMZM2G359974, GRMZM2G301884, and GRMZM2G083894) associated with kernel size and weight within three MQTL regions were identified using regional association mapping, based on the results of the meta-analysis. This strategy, combining MQTL analysis and regional association mapping, is helpful for functional marker development and rapid identification of candidate genes or loci.
Introduction
Maize is one of the most important cereal crops in the world and plays an important role in maintaining food security, promoting the development of animal husbandry, and satisfying the demand for industrial raw materials. Thus, improvement of grain yield is consistently one of the most important goals for maize breeders. In maize, grain yield is a complex quantitative trait controlled by many quantitative trait loci (QTL) with a small effect (Wu and Lin, 2006). A better understanding of the genetic architecture and molecular mechanisms of yield-related traits could help improve grain yield in maize.
Linkage mapping is an efficient way to identify genetic loci for complex quantitative traits in maize (Wallace et al., 2014). In the past two decades, many QTLs have been identified for yield and related traits thus far, such as ear-related traits (ERT) and kernel-related traits (http://www.maizegdb.org/). Marker-assisted selection (MAS) is a more efficient selection method for yield and its related traits improvement in the process of crop breeding. The tightly linked markers which are found in the genetic population and related with yield and its related traits should be identified before using in the MAS for crop breeding process (Xu, 2010). However, it is difficult to use these loci in the crop improvement process for the following reasons: (1) the results of QTL mapping for the same trait may vary due to the different populations used in different studies; (2) most QTLs explain just a small proportion of phenotypic variation and are detected only in specific environments; and (3) the confidence intervals for these QTLs are often large and contain hundreds of genes, making it very difficult to determine the candidate gene for the target trait.
QTL meta-analysis is an effective method to identify the genomic hotspot regions that control target traits more frequently and narrow down the confidence intervals of these QTLs to produce the metaQTLs (MQTLs) by integrating information from different mapping populations (Goffinet and Gerber, 2000; Arcade et al., 2004). Chardon et al. (2004) identified 62 MQTLs related to flowering time in maize by synthesizing 313 QTLs from different mapping populations and two important MQTL clusters for flowering time in bins 8.05 and 10.04. Subsequent positional cloning and association mapping analysis showed that Vgt1, which is located in bin 8.05, plays an important role in flowering time and the number of nodes (Salvi et al., 2007, 2011). Another MQTL for flowering time, located in bin 10.04, was found along with the key flowering time gene ZmCCT, which contains a CCT domain (Hung et al., 2012). These successful examples confirm that meta-analysis is a very useful method for predicting candidate genes and developing molecular markers for complex quantitative traits in maize. In fact, previous studies have identified many MQTLs for yield-related traits by integrating different QTL datasets from maize and many maize orthologs of rice yield-related genes using bioinformatic techniques (Semagn et al., 2013; Wang et al., 2013, 2016; Martinez et al., 2016). However, the number of QTLs that have been integrated in previous studies is still low, and methods for effectively mining candidate loci or genes for yield-related traits from these MQTL regions are still not well-developed.
A combination of linkage mapping and association mapping has recently proven to be an efficient method for identifying candidate loci related to yield-related traits in maize. Multiple major QTLs related to kernel size and weight, such as qKS2, qGW4.05, qKL1.07, and qKW7.02, were identified through linkage mapping, and their locations were subsequently narrowed down to very small genomic regions through association mapping (Chen et al., 2016a; Li et al., 2016b; Qin et al., 2016; Zhang et al., 2017). Similar to this strategy, combining QTL meta-analysis and regional association mapping to mine MQTLs and narrow down their associated confidence intervals has been suggested as a quick and effective way to identify candidate functional genes or loci (Daware et al., 2017). Based on this strategy, two potential candidate genes for grain size and weight were successfully identified in rice (Daware et al., 2017). Here, we use this strategy in maize to achieve the following objectives: (1) to synthesize the information on QTLs for grain yield and related traits published between 2000 and 2016; (2) to mine MQTLs across the entire genome through QTL meta-analysis; (3) to identify maize orthologs of rice yield-related genes using a comparative genomics strategy; and (4) to identify candidate genes or loci for kernel-related traits by combining meta-analysis with regional association mapping.
Materials and Methods
QTL Data Collection for Maize Yield and Related Traits
QTL data on maize grain yield (GY) and GY-related traits were collected from 33 studies published between 2000 and 2016 (Table S1). The GY-related traits included two important components: (1) ERT, including ear weight (EW), ear length (EL), ear diameter (ED), cob weight (CW), cob diameter (CD), and kernel row number (KRN); and (2) kernel-related traits (KRT), including kernel length (KL), kernel width (KWI), kernel thickness (KT), kernel number (KN), kernel weight (KW), kernel ratio (KR), and kernel volume (KV) (Table 1). Detailed QTL information and the associated literature on maize grain yield and related traits were collected from three databases, MaizeGDB (http://www.maizegdb.org), Gramene (http://www.gramene.org), and the PubMed web server (http://www.ncbi.nlm.nih.gov/pubmed). In this study, we collected only QTLs that were identified under normal growth conditions.
Meta-Analysis
The collected QTLs were projected onto the “IBM2 2008 Neighbors” maize reference map (http://curation.maizegdb.org/cgi-bin/displaymaprecord.cgi?id=1140201) to generate a consensus map. Then, a meta-analysis was performed to integrate the QTL data from different studies and to refine the confidence intervals. For the meta-analysis of many QTLs, five different models (1-, 2-, 3-, 4-, or N-QTL) with different Akaike information criterion (AIC) values have been proposed using BioMercator software V4 (http://moulon.inra.fr/index.php/en), where the model with the lowest AIC-value is considered optimal. Finally, the consensus QTL presented by the optimum model is regarded as the MQTL (Arcade et al., 2004).
Identification of Annotated Transcripts and Gene Ontology Analysis
The physical intervals of these MQTLs were identified using the MaizeGDB and Gramene databases. The physical locations of flanking markers for these MQTLs were confirmed on the IBM2 2008 Neighbors map. The annotated transcripts within these MQTL regions were mined in the MaizeGDB database. The physical locations of these MQTL regions were based on genome annotation version AGPv2 of the maize B73 reference map (http://curation.maizegdb.org/). The gene sequences located in the MQTL regions were aligned to the NCBI non-redundant (nr) database with Blastx, using an E-value of < 10−5, with a hit number threshold of 100. The best functional annotations were obtained using this process. With the Nr annotation, we used the Blast2GO program (version: v2.5.0) to obtain the gene ontology (GO) annotation for these genes.
Mining of Homologous Genes in the MQTL Regions
In this study, we collected 25 genes related to grain yield and related traits in rice (Table S3). Homologous genes in maize were identified as follows: (1) the protein sequences of these 25 collected rice genes were obtained from http://www.ricedata.cn/gene; (2) BLASTP (protein-protein BLAST) searches using these protein sequences were performed against the maize “non-redundant protein sequences” database (https://www.ncbi.nlm.nih.gov/). The criteria for these searches included an E-value of < 10−10, identity >60%, and a coverage region >60% to select homologous genes of these rice yield-related genes in maize.
Regional Association Mapping and Expression Analysis of the Candidate Genes
An association mapping panel with 627 maize inbred lines covering highly diverse maize germplasms was applied in this study to perform regional association mapping. The genotypes and phenotypes of this association panel have been provided in our previous reports (Chen et al., 2016a; Qin et al., 2016). We selected SNP markers with minor allele frequencies >0.05 in MQTL regions to perform the analysis. The association analysis was estimated using a mixed linear model (MLM) incorporated in TASSEL V5.0, controlling for population structure (Q) and kinship (K). The first three principal components (PCs), which have been analyzed in previous studies, were used as the covariant variables to control for the existing population structure in the association mapping panel. Significant marker-trait associations were declared at LOD >3. The expression data from different kernel development values of these candidate genes were collected from the MaizeGDB database (http://maizegdb.org/) (Winter et al., 2007; Sekhon et al., 2011).
Results
QTL Collection for Maize Grain Yield and Its Related Traits
A total of 999 QTLs related to GY, ERT, and KRT were collected (Table S1). The number of QTLs per trait ranged from 11 (kernel ratio, KR) to 218 (kernel weight, KW) (Table 1). The 999 collected QTLs were distributed unevenly across the ten chromosomes (Figure 1A). The greatest number of QTLs (182) were located on chromosome 1, while chromosome 6 exhibited the fewest, with 68 (Figure 1A), similar to previous meta-analyses of maize yield QTLs (Wang et al., 2016). A total of 75.08% of the collected QTLs for each trait exhibited an R2 < 10%, implying that the proportion of phenotypic variance explained by each QTL was very small (Figure 1B). These results suggest that grain yield and related traits in maize are mainly controlled by numerous loci of minor effect and display a complex genetic architecture.
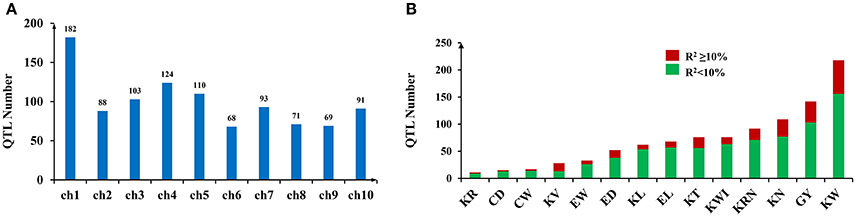
Figure 1. QTL numbers and associated R2-values (variance explained by a single QTL) distributed across the entire genome. (A) QTL numbers distributed on each chromosome. (B) The variance explained by a single QTL in the traits included in this study.
QTL Meta-Analysis
The collected QTLs for maize yield and its related traits were projected onto the target map IBM2 2008 Neighbors via meta-analysis to mine MQTLs and refine QTL intervals. A total of 76 MQTLs were identified according to the models with the lowest AIC-values (Table 2, Figure 2). These MQTLs were distributed unevenly across the genome, with the number per chromosome ranging from 4 on chromosome 4–10 on chromosome 5 (Table 2, Figure 2). These MQTLs were named sequentially from MQTL-1 to MQTL-76 according to their chromosomal locations. MQTL-31 covered just two QTLs, while MQTL-28 interestingly covered 45 QTLs (Table 2). We also found that 60 MQTLs were related to GY, while 4 MQTLs were related to only kernel-related traits (KRT), and MQTL-17, on chromosome 3, was associated with only ERTs (Table 2).
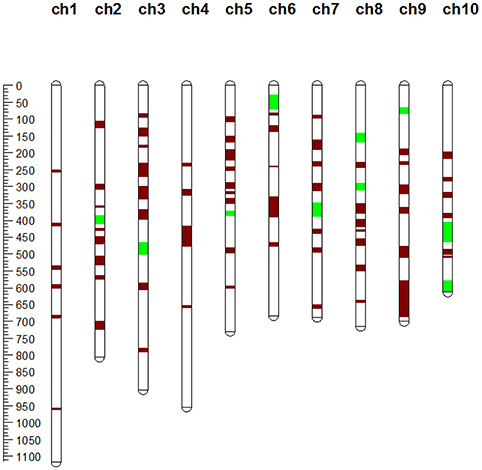
Figure 2. Chromosomal locations of MQTLs identified in this study. The red segments on the chromosome represent MQTLs related to grain yield (GY), ear-related traits (ERT), and kernel-related traits (KRT), while the green segments represent MQTLs related to KRT alone or to GY and KRT simultaneously.
Furthermore, we found that several yield-related MQTLs tended to show a clustered distribution in the maize genome. One of these clustered regions was detected in the genomic region from 38.17 to 69.15 Mb on maize chromosome 5 and consisted of two MQTLs (MQTL-33 and MQTL-34) (Table 2). Two MQTLs (MQTL-39 to MQTL-40) were clustered in a 28.5-Mb region on maize chromosome 6 (Table 2). Other clusters were found on maize chromosome 7 (from 9.78 to 34.19 Mb), chromosome 8 (from 146.89 to 163.31 Mb), and chromosome 9 (from 16.24 to 22.68 Mb) (Table 2). Five MQTLs (from MQTL-72 to MQTL-76) were clustered in a 16.24-Mb genomic region on maize chromosome 10 (Table 2).
Identification of Annotated Transcripts and Go analysis in MQTL Regions
Among the 76 MQTLs, 44 MQTLs with an interval of less than 5 Mb were selected for GO analysis of annotated transcripts. A total of 4,501 genes were identified in the 44 MQTL regions, whose detailed gene IDs are presented in Table S2. Multiple genes associated with yield-related traits in maize were found to be located in MQTL intervals. Fea2 (Bommert et al., 2013) and Ub2 (Chuck et al., 2014), which are important genes in the control of kernel row numbers in maize, were located in the MQTL-27 and MQTL-5 regions, respectively. The UBL1 gene, which plays an important role in kernel and seedling development by influencing pre-mRNA splicing (Li et al., 2016a), was located in MQTL-37. Three genes related to maize kernel development, emp2 (Fu et al., 2002), ZmZHOUPI (Grimault et al., 2015), and ZmReas1 (Qi et al., 2016), were located in the MQTL-11, MQTL-9, and MQTL-43 regions, respectively. According to the two-level WEGO classification, 2,257 genes were annotated, which were divided into three categories (cellular component, molecular function, and biological pathway) and further divided into 48 classes (Figure 3). We found that more than one thousand genes were related to the cell, cell part, metabolic process, cellular process, organelle, binding, and catalytic activity terms (Figure 3).
Homologous Gene Mining in MQTL Regions
To identify candidate genes related to grain yield in the MQTL regions, we collected 25 genes that were functionally characterized as being associated with grain yield and its components from the rice genome (Table S3). Homologous genes were not found in the maize genome for four of these rice genes (APG, GW5, PGL1, qGL3), while eight of the rice genes (An-1, Bsg1, D2, DEP1, GIF1, GW2, LP, SMG1, SRS3, and SRS5) exhibited more than one homologous gene, and the other genes exhibited a single homologous gene in maize (Table S3). Finally, 11 MQTL regions containing 11 maize orthologs of 10 rice genes related to grain yield and its related traits were identified in this study.
MQTL-19 contained ZmGS5 (Gene ID: GRMZM2G13815), an ortholog of the well-characterized rice yield gene GS5 (Table 3). In rice, GS5 encodes a serine carboxypeptidase family protein that controls grain size and weight by regulating grain width and filling (Li et al., 2011). One candidate gene (Gene ID: GRMZM2G097275), homologous to OsSPL14, was identified in the MQTL-35 region (Table 3). OsSPL14 is a member of the SBP (squamosa promoter-binding-like transcription activator) family, and can improve the grain yield by regulating plant architecture (Miura et al., 2010). GN1a encodes a cytokinin dehydrogenase 2 protein and regulates grain number to improve grain yield in rice (Ashikari et al., 2005). A GN1a homolog (Gene ID: GRMZM2G325612) was identified in maize in the MQTL-54 region (Table 3). A homologous gene of D61 (Gene ID: GRMZM2G048294), which encodes a brassinosteroid receptor kinase and can regulate plant height to produce small grains in rice (Morinaka et al., 2006), was identified in the MQTL-58 region (Table 3). Homologs of other grain yield-related genes, such as LP1, SRS5, Ghd7, and DEP1, were also identified in MQTL regions (Table 3). These homologs of yield-related genes may play important roles in ear and kernel development in maize.
Regional Association Analysis Underlying KRT MQTL Regions
In our study, a total of 10 MQTL regions were found to be related to KRT alone or to both GY and KRT (Table 2). These 10 MQTL regions were selected to implement a regional association analysis. The integration of SNP genotyping data from the 10 selected MQTL regions with phenotype information (10-kernel length, 10KL; 10-kernel width, 10KW; 100-kernel weight, HKW) for all 627 accessions successfully revealed significant associations for four MQTL regions (MQTL-10, MQTL-39, MQTL-49, and MQTL-73).
MQTL-10 Region
We selected SNP markers in an interval (chr2: 62924210~149111857) corresponding to the MQTL-10 region in the maize genome. Using a mixed linear model, we identified two, two and one SNPs associated with variation in kernel length, kernel width, and hundred kernel weight, respectively (Table 4, Figure 4A). These significant SNP loci explained 2.72–3.80% of the observed phenotypic variation in kernel size and weight in this association mapping panel (Table 4). We also identified one SNP (PZE-102096886) located in the GRMZM2G359974 gene that was associated with the variation in kernel weight and 10-kernel width (Figure 4A). Comparisons of these significant P-values with the P-value distribution of 600 randomly chosen SNPs from this region suggested that these significant associations were not due to false positives (Figure 4B).
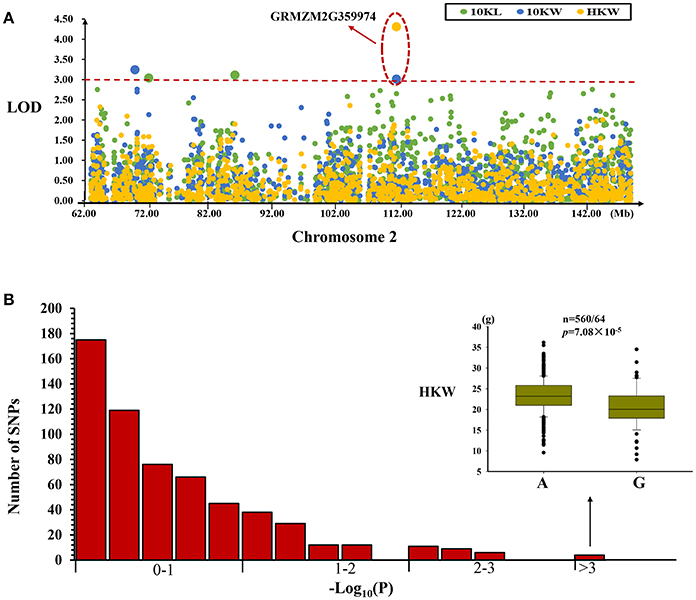
Figure 4. Regional association analysis of the MQTL-10 region. (A) Association analysis between the SNPs located within the MQTL-10 region and kernel size and weight. The green, blue, and yellow circles represent different SNPs, whereas the red line represents the significance threshold. An LOD >3 indicates that a SNP is significantly related to kernel size or weight. One SNP located in the gene (GRMZM2G359974) was found to be associated with kernel width and weight. (B) Permutation test for the identified significantly associated SNP markers. Comparison of the most highly significant SNP (n = 560/64, P = 7.08 × 10−5) with the association results for 600 randomly selected SNPs for the same trait. These results suggested that the significant association was a real association and was not caused by a false positive.
MQTL-39 Region
Two significant SNPs associated with HKW and 10KW were identified in this region, while explained 2.80 and 1.81% of the observed phenotypic variation, respectively (Table 4). A significant SNP (SYN11458) related to kernel weight variation was located in the GRMZM2G301884 gene (Figure 5A). For SYN11458, two alleles (A/G) were present in the association panel, with the G allele being associated with a higher kernel weight (Figure 5B). The PZE-106021411 SNP, located in an intergenic region, was significantly associated with kernel width. Two alleles for this SNP (A/G) were present in this panel, with the A allele being associated with greater kernel width (Table 4, Figure 5C).
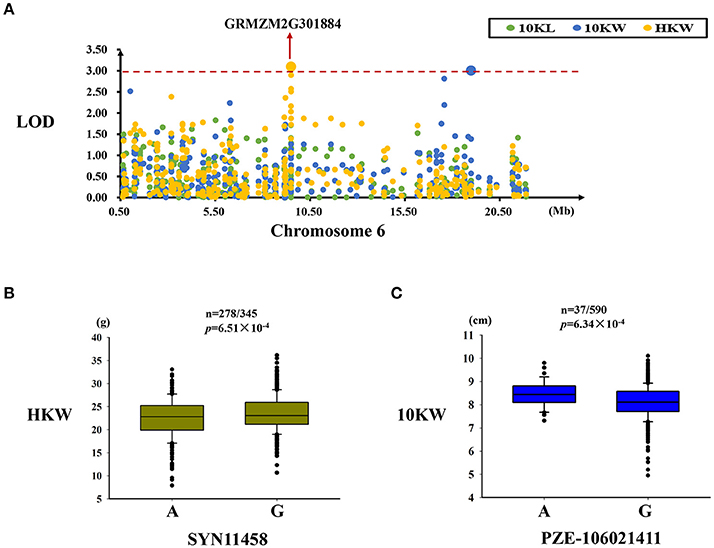
Figure 5. Regional association analysis of the MQTL-39 region. (A) Association analysis between the SNPs located in the MQTL-39 region and kernel size and weight. A SNP (SYN11458) associated with kernel weight was found in this gene (GRMZM2G301884), and another SNP (PZE-106021411) associated with kernel width was identified in the intergenic region. (B) The SYN11458 SNP was significantly related to kernel weight (n = 278/345, P = 6.51 × 10−4). SYN11458 has two alleles (A and G), and the G allele is associated with a greater kernel weight. (C) The SNP PZE-106021411 was significantly related to kernel width (n = 37/590, P = 6.34 × 10−4). PZE-106021411 had two alleles (A and G), and the A allele was associated with a wider kernel. The red dashed line represent the significance threshold (LOD = 3).
MQTL-49 Region
Only one significant SNP (SYN35079) located in the GRMZM2G083894 gene was found to be associated with kernel width in this region (Figure 6A). This SNP explained 2.85% of the variation in kernel width and exhibited two alleles (A/G) in this association panel (Table 4). Significant differences in kernel width were found between these two alleles of SYN35079 (Figure 6B).
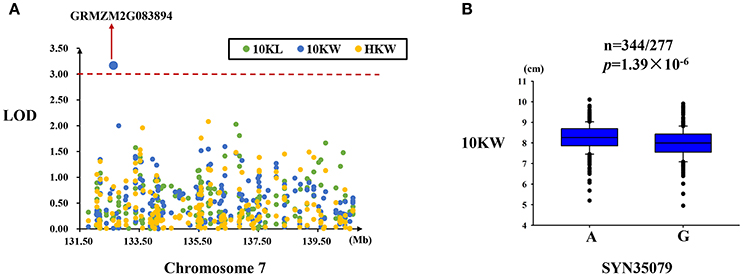
Figure 6. Regional association analysis of the MQTL-49 region. (A) Association analysis between the SNPs located in the MQTL-49 region and kernel size and weight. Only one SNP (SYN35079) was found to be associated with kernel width in this gene (GRMZM2G083894). (B) The SNP SYN35079 was significantly related to kernel width (n = 344/277, P = 1.39 × 10−6). SYN35079 had two alleles (A and G), and the A allele was associated with a wider kernel. The red dashed line represent the significance threshold (LOD = 3).
MQTL-73 Region
One SNP (PZE-110091041), located in the intergenic region, was significantly associated with hundred kernel weight (Figure 7A). This SNP explained 3.01% of the variation in kernel weight, and the T allele of this SNP was associated with a higher kernel weight (Table 4, Figure 7B).
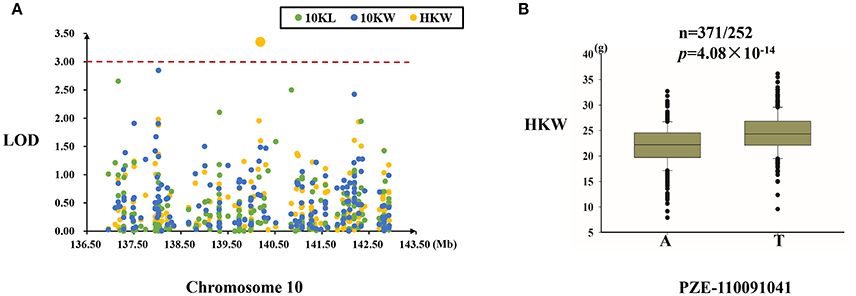
Figure 7. Regional association analysis of the MQTL-73 region. (A) Association analysis between the SNPs located in the MQTL-73 region and kernel size and weight. Only one SNP (PZE-110091041) was found to be associated with kernel weight in the intergenic region. (B) The PZE-110091041 SNP was significantly related to kernel weight (n = 371/252, P = 4.08 × 10−14). PZE-110091041 had two alleles (A and T), and the T allele was associated with a greater kernel weight. The red dashed line represent the significance threshold (LOD = 3).
And, we found that the inbred lines which harbor the positive allele in the linkage population also have the SNP allele can increase the corresponding trait (Table S6). HBqkwid2 is a QTL related with kernel width and found by using the RIL population from the cross of Huangzaosi and Huobai and a positive effect from Huangzaosi (Li et al., 2013). Using regional association mapping, we identified an SNP (A/G) significantly related with kernel width, and Huangzaosi has an “A” allele, which can increase kernel width. The MQTL-73 region contains a hundred kernel weight QTL (Zqkwei10), which is found in the RIL populations from Huangzaosi and Zheng58, and the positive effect from Zheng58 can increase the hundred kernel weight (Li et al., 2013). A significant SNP (A/T) was identified using regional association mapping in this region; Zheng58 contains the “T” allele and can increase the kernel weight, and Huangzaosi contains the “A” allele and can decrease the kernel weight.
Expression Pattern Analysis of Candidate Genes
The combination of meta-analysis and regional association mapping indicated that three genes (GRMZM2G359974, GRMZM2G301884, and GRMZM2G083894) may play important roles in maize kernel development. The expression data for the three candidate genes were collected from the MaizeGDB database (http://www.maizegdb.org/). We found that these genes were all expressed at different stages of maize kernel development. For instance, GRMZM2G359974, which encodes a transferase family protein, was highly expressed in endosperm-20 DAP, while GRMZM2G301884 was highly expressed at the early stage of grain development, and GRMZM2G083894, which encodes an AN1-like zinc finger protein, was expressed at different levels in different stages of kernel development in maize (Figure S1). Therefore, these genes should be considered the most promising candidates for involvement in maize kernel development for further functional validation.
Discussion
Meta-Analysis of Yield-Related Trait QTLs in Maize
In maize, most important agronomic traits, such as grain yield, ERT, and kernel-related traits, are complex, quantitative and controlled by numerous small effect QTLs. With increasing numbers of identified QTLs, researchers tend to compare the results of QTL analysis from different backgrounds and environments (Peng et al., 2011; Li et al., 2013). However, the results of QTL mapping are often inconsistent due to different population types, population sizes, mapping methods and genetic backgrounds. Therefore, QTLs localized to inconsistent genomic regions should be investigated further to validate these QTLs and verify their precise positions. Integrating QTL data from different studies and using meta-analysis to identify MQTL regions will pave the way for further fine mapping of QTLs and gene cloning (Wang et al., 2013, 2016; Martinez et al., 2016). In this study, 999 QTLs for maize yield and related traits were collected from different populations (Table S1). Through meta-analysis, a total of 76 maize yield MQTLs were identified in this study (Table 2, Figure 2). Compared with previous studies, we identified many MQTL regions that were highly consistent across different studies (Table S4). We also identified nine genomic regions for yield-related traits that had been identified in previous studies (Wang et al., 2013, 2016; Martinez et al., 2016) (Table S4). Intriguingly, we found that a major QTL (qGW4.05) for maize kernel size and weight that we recently fine-mapped was located in the MQTL-27 region on maize chromosome 4. On the same chromosome, a QTL for maize grain yield, qYPP4-1, was recently identified using a high-density genetic map, which was placed in the MQTL-28 region by our meta-analysis (Chen et al., 2016b). These results suggest that some MQTL regions identified in our study can serve as major QTLs for fine mapping and gene cloning of important yield genes.
Homologous Gene Cloning of Maize Yield and Related Traits
In crops with large and complex genomes, such as maize and wheat, homology-based cloning methods are useful tools for identifying important genes related to complex traits. In recent years, many crop genome sequences have been released based on the wide application of next-generation sequencing technologies (Liu et al., 2015). These public genome sequences aid in the identification of conserved genomic regions and key genes in different crops.
With the numerous grain yield-related genes identified in rice, many homologous genes related to grain yield have been cloned in maize based on a comparative genomics strategy. In maize, multiple genes related to kernel size and weight have been identified, and their function has been validated through linkage analysis and association mapping analysis (Li et al., 2010a,b; Liu et al., 2015). ZmGS5, a maize homolog of the rice grain size and weight gene GS5 was identified in the MQTL-19 region in this study (Table 3). Previous studies have demonstrated that brassinosteroids such as brd2, dwf11, and d61 play an important role in seed development in rice. One gene homologous to D61 was identified in MQTL-58 (Table 3). The dwarf d61 mutant of rice exhibits smaller grains and a lower grain weight due to defects in brassinolide metabolism (Morinaka et al., 2006). The members of the SBP gene family are important transcription factors in plants, involved in plant growth and development. OsSPL14, which encodes an SBP transcription factor, controls shoot branching in the vegetative stage, and a higher grain yield can be achieved in rice via over-expression of this gene in the reproductive stage (Miura et al., 2010). One candidate gene (GRMZM2G097275) was identified in the MQTL-35 region, which was homologous to OsSPL14 (Table 3). The rice gene GN1a, which encodes a cytokinin oxidase, can increase grain number and improve grain yield. When the expression of GN1a is reduced, the cytokinin accumulates in inflorescence meristems, and the number of reproductive organs is increased (Ashikari et al., 2005). Furthermore, GRMZM2G325612, a homolog of GN1a, was mapped to the MQTL-54 region (Table 3). The homologs of the 10 genes in the MQTL regions have been shown to improve grain yield in rice, suggesting their possible roles in maize. As in previous studies, multiple methods of analysis, such as expression pattern analysis, phylogenetic analysis, linkage analysis, candidate gene mapping analysis, and transgenic analysis, should be used to validate the function of homologous genes. These homologous genes in MQTL regions should to be validated in the future using a number of the techniques mentioned above.
Combining Regional Association Mapping and MQTL Results to Mine Candidate Genes for Kernel Size and Weight
Association mapping and linkage mapping are two effective strategies for dissection of the genetic basis of complex quantitative traits in crops. These two methods each have particular advantages, such as a higher relative power and lower false positive rate for linkage mapping and a relatively higher mapping resolution for association analysis (Sneller et al., 2009). In recent years, the combination of linkage and association mapping strategies has been widely used in crops. One strategy is to construct integrated mapping populations such as MAGIC (multiparent advanced generation inter-crosses) and NAM (nested association mapping) populations (Buckler et al., 2009; Meng et al., 2016). Based on these types of populations, many complex quantitative traits have been resolved, such as flowering time, plant height, and disease resistance (Buckler et al., 2009; Peiffer et al., 2014; Ding et al., 2015). Another strategy is to first determine confidence intervals related to target traits based on linkage mapping analysis. Then, genome-wide association mapping or regional association mapping methods can be used to narrow down the confidence intervals of major QTLs and identify candidate genes or loci. Many major QTLs related to kernel size and weight have been finely mapped to candidate genes in maize (Chen et al., 2016a; Li et al., 2016b; Qin et al., 2016) or rapeseed (Li et al., 2014).
Combining meta-analysis and regional association mapping can rapidly identify candidate genes associated with complex agronomic traits, such as grain size and weight, in rice (Daware et al., 2017). In this study, we identified three candidate genes (GRMZM2G359974, GRMZM2G301884, and GRMZM2G083894) for kernel size and weight by combining meta-analysis and regional association mapping (Table 4, Figures 4–6). One gene (GRMZM2G359974) located in MQTL-10 region encoding a transferase family protein was found to be significantly associated with kernel width and weight through regional association analysis (Table 4, Figure 4). GRMZM2G083894, which encodes an AN1-like zinc finger domain protein, was found to be associated with kernel width and exhibited different expression levels in different stages of kernel development (Table 4, Table S4). The three genes (GRMZM2G359974, GRMZM2G301884, and GRMZM2G083894) are identified by combined the meta analysis and regional association mapping. And, we found the three genes were all expressed at different stages of maize kernel development. To date, many GWAS studies have been done for maize yield and its related traits, but these results are very different for many reasons such as the mapping population, the phenotype variation and the population structure. The three genes identified in our study were near by the significant SNPs with GWAS for kernel size and weight traits through combining 10 RIL populations (Liu et al., 2017). For example, GRMZM2G359974 were significant associated with kernel width and weight in this study and a SNP which located on 0.9 Mb upstream of this gene were also found significantly associated with kernel width by GWAS (Liu et al., 2017), and a SNP associated with kernel width was located on 1.5 Mb upstream of GRMZM2G083894. GRMZM2G083894 were located in the QTL interval which is found by using the RIL population from the cross of B73 and BY804 (Liu et al., 2017). Based on these results, these three candidate genes should be considered the most promising candidates related to kernel size and weight in maize for further functional validation.
Conclusion
Grain yield and related traits are complex quantitative traits controlled by numerous QTLs of small effect in maize. The integration and meta-analysis of a large set of QTLs provides valuable information for QTL fine mapping and key genes for cloning. In this study, we collected 999 QTLs related to yield and related traits and identified 76 MQTLs across the maize genome. Based on a comparative genomic strategy, several maize orthologs of rice yield-related genes were identified in the MQTL regions. We then mined the candidate genes or loci for kernel size and weight through regional association mapping based on the results of the meta-analysis. Consequently, three potential candidate genes associated with kernel size and weight within three MQTL regions were identified in this study. These results confirmed that combining meta-analysis and regional association mapping is helpful for functional marker development and rapid determination of candidate genes or loci, and the candidate loci identified in this study contribute to our understanding of the genetic architecture of grain yield and related traits in maize.
Author Contributions
TW and YuL: conceived and designed the study and carried out all the experiments; LC, YA, YoL, CL, DZ, YuS, and YaS: perform the field experiment and the evaluation of the phenotype: LC, YA, YoL, and CL: carried out the analysis. LC and YA: wrote the manuscript. All authors have read and approved the final version of the manuscript.
Conflict of Interest Statement
The authors declare that the research was conducted in the absence of any commercial or financial relationships that could be construed as a potential conflict of interest.
Acknowledgments
This research was supported by the National Natural Science Foundation (91335206, 91735306), the Ministry of Science and Technology of China (2014CB138200, 2013BAD01B02), and the CAAS Innovation Program.
Supplementary Material
The Supplementary Material for this article can be found online at: https://www.frontiersin.org/articles/10.3389/fpls.2017.02190/full#supplementary-material
Figure S1. Expression levels of candidate genes identified through regional association mapping during the different stages of maize kernel development.
Table S1. QTL information collected in this study.
Table S2. Annotated transcripts located in MQTL regions.
Table S3. Genes related to grain yield and associated traits in rice and homologous genes in maize.
Table S4. Comparison of meta-analysis results between this study and previous studies (Wang et al., 2013, 2016; Martinez et al., 2016).
Table S5. The MQTL region location based on B73 ref V4.
Table S6. Compared the positive allele between the linkage and association population.
References
Arcade, A., Labourdette, A., Falque, M., Mangin, B., Chardon, F., Charcosset, A., et al. (2004). Biomercator: integrating genetic maps and QTL towards discovery of candidate genes. Bioinformatics 20, 2324–2326. doi: 10.1093/bioinformatics/bth230
Ashikari, M., Sakakibara, H., Lin, S., Yamamoto, T., Takashi, T., Nishimura, A., et al. (2005). Cytokinin oxidase regulates rice grain production. Science 309, 741–745. doi: 10.1126/science.1113373
Bommert, P., Nagasawa, N. S., and Jackson, D. (2013). Quantitative variation in maize kernel row number is controlled by the fasciated ear2 locus. Nat. Genet. 45, 334–337. doi: 10.1038/ng.2534
Buckler, E. S., Holland, J. B., Bradbury, P. J., Acharya, C. B., Brown, P. J., Browne, C., et al. (2009). The genetic architecture of maize flowering time. Science 325, 714–718. doi: 10.1126/science.1174276
Chardon, F., Virlon, B., Moreau, L., Falque, M., Joets, J., Decousset, L., et al. (2004). Genetic architecture of flowering time in Maize as inferred from quantitative trait loci meta-analysis and synteny conservation with the rice genome. Genetics 168, 2169–2185. doi: 10.1534/genetics.104.032375
Chen, L., Li, Y., Li, C., Xun, W., Weiwei, Q., Xin, L., et al. (2016a). Fine-mapping of qGW4.05, a major QTL for kernel weight and size in maize. BMC Plant Biol. 16:81. doi: 10.1186/s12870-016-0768-6
Chen, L., Li, C., Li, Y., Song, Y., Zhang, D., Wang, T., et al. (2016b). Quantitative trait loci mapping of yield and related traits using a high-density genetic map of maize. Mol Breed. 36:134. doi: 10.1007/s11032-016-0545-0
Chuck, G. S., Brown, P. J., Meeley, R., and Hake, S. (2014). Maize SBP-box transcription factors unbranched2 and unbranched3 affect yield traits by regulating the rate of lateral primordia initiation. Proc. Natl. Acad. Sci. U.S.A. 111, 18775–18780. doi: 10.1073/pnas.1407401112
Daware, A. V., Srivastava, R., Singh, A. K., and Tyagi, A. K. (2017). Regional association analysis of metaQTLs delineates candidate grain size genes in rice. Front. Plant Sci. 8:807. doi: 10.3389/fpls.2017.00807
Ding, J., Ali, F., Chen, G., Li, H., Mahuku, G., and Yang, N. (2015). Genome-wide association mapping reveals novel sources of resistance to northern corn leaf blight in maize. BMC Plant Biol. 15:206. doi: 10.1186/s12870-015-0589-z
Fu, S., Meeley, R., and Scanlon, M. J. (2002). EMPTY PERICARP2 encodes a negative regulator of the heat shock response and is required for maize embryogenesis. Plant Cell 14, 3119–3132. doi: 10.1105/tpc.006726
Goffinet, B., and Gerber, S. (2000). Quantitative trait loci: a meta-analysis. Genetics 155, 463–473. Available online at: http://www.genetics.org/content/155/1/463
Grimault, A., Gendrot, G., Chamot, S., Widiez, T., Rabillé, H., Gérentes, M., et al. (2015). ZmZHOUPI, an endosperm-specific basic helix–loop–helix transcription factor involved in maize seed development. Plant J. 84:574–586. doi: 10.1111/tpj.13024
Hung, H. Y., Shannonb, L. M., Tian, F., Bradbury, P. J., Chen, C., Flint-Garcia, S. A., et al. (2012). ZmCCT and the genetic basis of day-length adaptation underlying the postdomestication spread of maize. Proc. Natl. Acad. Sci. U.S.A. 109, 1913–1921. doi: 10.1073/pnas.1203189109
Li, C., Li, Y., Peng, B., Liu, C., Liu, Z., Yang, Z., et al. (2013). Quantitative trait loci mapping for yield components and kernel-related traits in multiple connected RIL populations in maize. Euphytica 193, 303–316. doi: 10.1007/s10681-013-0901-7
Li, N., Shi, J., Wang, X., Liu, G., and Wang, H. (2014). A combined linkage and regional association mapping validation and fine mapping of two major pleiotropic QTLs for seed weight and silique length in rapeseed (Brassica napus L.). BMC Plant Biol. 14:114. doi: 10.1186/1471-2229-14-114
Li, J., Fu, J., Chen, Y., Fan, K., He, C., Zhang, Z., et al. (2016a). The U6 biogenesis-like 1 plays an important role in maize kernel and seedling development by affecting the 3′ end processing of U6 snRNA. Mol. Plant. 10, 470–482. doi: 10.1016/j.molp.2016.10.016
Li, Q., Li, L., Yang, X., Warburton, M. L., Bai, G., Dai, J. R., et al. (2010a). Relationship, evolutionary fate and function of two maize co-orthologs of rice GW2 associated with kernel size and weight. BMC Plant Biol. 10:143. doi: 10.1186/1471-2229-10-143
Li, Q., Yang, X., Bai, G., Warburton, M. L., Mahuku, G., Gore, M., et al. (2010b). Cloning and characterization of a putative GS3 ortholog involved in maize kernel development. Theor. Appl. Genet. 120, 753–763. doi: 10.1007/s00122-009-1196-x
Li, X., Li, Y. X., Chen, L., Wu, X., Qin, W., Song, Y., et al. (2016b). Fine mapping of qkw7, a major QTL for kernel weight and kernel width in maize, confirmed by the combined analytic approaches of linkage and association analysis. Euphytica 210, 221–232. doi: 10.1007/s10681-016-1706-2
Li, Y., Fan, C., Xing, Y., Jiang, Y., Luo, L., Sun, L., et al. (2011). Natural variation in GS5 plays an important role in regulating grain size and yield in rice. Nat. Genet. 43, 1266–1269. doi: 10.1038/ng.977
Liu, E., Liu, Y., Wu, G., Zeng, S., Thi, T. G. T., Liang, L., et al. (2016). Identification of a candidate gene for panicle length in rice (Oryza sativa L.) via association and linkage analysis. Front. Plant Sci. 7:596. doi: 10.3389/fpls.2016.00596
Liu, J., Deng, M., Guo, H., Raihan, S., Luo, J., Xu, Y., et al. (2015). Maize orthologs of rice GS5 and their trans-regulator are associated with kernel development. J. Integr. Plant Biol. 57, 943–953. doi: 10.1111/jipb.12421
Liu, J., Huang, J., Guo, H., Lan, L., Wang, H., Xu, Y., et al. (2017). The conserved and unique genetic architecture of kernel size and weight in maize and rice. Plant Physiol. 175, 774–785. doi: 10.1104/pp.17.00708
Luo, J., Liu, H., Zhou, T., Gu, B., Huang, X., Shangguan, Y., et al. (2013). An-1 encodes a basic helix-loop-helix protein that regulates awn development, grain size, and grain number in rice. Plant Cell 25, 3360–3376. doi: 10.1105/tpc.113.113589
Martinez, A. K., Soriano, J. M., Tuberosa, R., Koumproglou, R., Jahrmann, T., and Salvi, S. (2016). Yield QTLome distribution correlates with gene density in maize. Plant Sci. 242, 300–309. doi: 10.1016/j.plantsci.2015.09.022
Meng, L., Guo, L., Ponce, K., Zhao, X., and Ye, G. (2016). Characterization of three rice multiparent advanced generation intercross (MAGIC) populations for quantitative trait loci identification. Plant Genome 9, 1–14. doi: 10.3835/plantgenome2015.10.0109
Miura, K., Ikeda, M., Matsubara, A., Song, X. J., Ito, M., Asano, K., et al. (2010). OsSPL14 promotes panicle branching and higher grain productivity in rice. Nat. Genet. 42, 545–549. doi: 10.1038/ng.592
Morinaka, Y., Sakamoto, T., Inukai, Y., Agetsuma, M., Kitano, H., Ashikari, M., et al. (2006). Morphological alteration caused by brassinosteroid insensitivity increases the biomass and grain production of rice. Plant Physiol. 141, 924–931. doi: 10.1104/pp.106.077081
Peiffer, J. A., Romay, M. C., Gore, M. A., Flintgarcia, S. A., Zhang, Z., Millard, M. J., et al. (2014). The genetic architecture of maize height. Genetics 196, 1337–1356. doi: 10.1534/genetics.113.159152
Peng, B., Li, Y., Wang, Y., Liu, C., Liu, Z., Tan, W., et al. (2011). QTL analysis for yield components and kernel-related traits in maize across multi-environments. Theor. Appl. Genet. 122, 1305–1320. doi: 10.1007/s00122-011-1532-9
Qi, W., Zhu, J., Wu, Q., Wang, Q., Li, X., Yao, D., et al. (2016). Maize rea1 mutant stimulates ribosome use efficiency and triggers distinct transcriptional and translational responses. Plant Physiol. 170, 971–988. doi: 10.1104/pp.15.01722
Qin, W., Li, Y. X., Wu, X., Li, X., Chen, L., Shi, Y., et al. (2016). Fine mapping of qKL1.07, a major QTL for kernel length in maize. Mol. Breed. 36:8. doi: 10.1007/s11032-015-0419-x
Ren, D., Rao, Y., Wu, L., Xu, Q., Li, Z., Yu, H., et al. (2016). The pleiotropic abnormal flower and dwarf1 affects plant height, floral development and grain yield in rice. J. Integr. Plant Biol. 58, 529–539. doi: 10.1111/jipb.12441
Salvi, S., Corneti, S., Bellotti, M., Carraro, N., Sanguineti, M. C., Castelletti, S., et al. (2011). Genetic dissection of maize phenology using an intraspecific introgression library. BMC Plant Biol. 11:4. doi: 10.1186/1471-2229-11-4
Salvi, S., Sponza, G., Morgante, M., Tomes, D., Niu, X., Fengler, K. A., et al. (2007). Conserved noncoding genomic sequences associated with a flowering-time quantitative trait locus in maize. Proc. Natl. Acad. Sci. U.S.A. 104, 11376–11381. doi: 10.1073/pnas.0704145104
Segami, S., Kono, I., Ando, T., Yano, M., Kitano, H., Miura, K., et al. (2012). Small and round seed 5 gene encodes alpha-tubulin regulating seed cell elongation in rice. Rice 5:4. doi: 10.1186/1939-8433-5-4
Sekhon, R. S., Lin, H., Childs, K. L., Hansey, C. N., Buell, C. R., De, L. N., et al. (2011). Genome-wide atlas of transcription during maize development. Plant J. 66, 553–563. doi: 10.1111/j.1365-313X.2011.04527.x
Semagn, K., Beyene, S. K., Beyene, Y., Warburton, M. L., Tarekegne, A., Mugo, S., et al. (2013). Meta-analyses of QTL for grain yield and anthesis silking interval in 18 maize populations evaluated under water-stressed and well-watered environments. BMC Genomics 14:313. doi: 10.1186/1471-2164-14-313
Sneller, C. H., Mather, D. E., and Crepieux, S. (2009). Analytical approaches and population types for finding and utilizing QTL in complex plant populations. Crop Sci. 49, 363–380. doi: 10.2135/cropsci2008.07.0420
Sun, H., Qian, Q., Wu, K., Luo, J., Wang, S., Zhang, C., et al. (2014). Heterotrimeric G proteins regulate nitrogen-use efficiency in rice. Nat. Genet. 46, 652–656. doi: 10.1038/ng.2958
Wallace, J. G., Larsson, S. J., and Buckler, E. S. (2014). Entering the second century of maize quantitative genetics. Heredity 112, 30–38. doi: 10.1038/hdy.2013.6
Wang, Y., Huang, Z., Deng, D., Ding, H., Zhang, R., Wang, S., et al. (2013). Meta-analysis combined with syntenic metaQTL mining dissects candidate loci for maize yield. Mol. Breed. 31, 601–614. doi: 10.1007/s11032-012-9818-4
Wang, Y., Xu, J., Deng, D., Ding, H., Bian, Y., Yin, Z., et al. (2016). A comprehensive meta-analysis of plant morphology, yield, stay-green, and virus disease resistance QTL in maize (Zea mays L.). Planta 243, 459–471. doi: 10.1007/s00425-015-2419-9
Winter, D., Vinegar, B., Nahal, H., Ammar, R., Wilson, G. V., Provart, N. J., et al. (2007). An “Electronic Fluorescent Pictograph” browser for exploring and analyzing large-scale biological data sets. PLoS ONE 2:e718. doi: 10.1371/journal.pone.0000718
Wu, R., and Lin, M. (2006). Functional mapping - how to map and study the genetic architecture of dynamic complex traits. Nat. Rev. Genet. 7, 229–237. doi: 10.1038/nrg1804
Xue, W., Xing, Y., Weng, X., Zhao, Y., Tang, W., Wang, L., et al. (2008). Natural variation in Ghd7 is an important regulator of heading date and yield potential in rice. Nat. Genet. 40, 761–767. doi: 10.1038/ng.143
Keywords: grain yield, kernel size and weight, metaQTL, regional association mapping, maize
Citation: Chen L, An Y, Li Y, Li C, Shi Y, Song Y, Zhang D, Wang T and Li Y (2017) Candidate Loci for Yield-Related Traits in Maize Revealed by a Combination of MetaQTL Analysis and Regional Association Mapping. Front. Plant Sci. 8:2190. doi: 10.3389/fpls.2017.02190
Received: 03 August 2017; Accepted: 12 December 2017;
Published: 22 December 2017.
Edited by:
Soren K. Rasmussen, University of Copenhagen, DenmarkReviewed by:
Roberto Tuberosa, Università di Bologna, ItalyHongjun Liu, Shandong Agricultural University, China
Copyright © 2017 Chen, An, Li, Li, Shi, Song, Zhang, Wang and Li. This is an open-access article distributed under the terms of the Creative Commons Attribution License (CC BY). The use, distribution or reproduction in other forums is permitted, provided the original author(s) or licensor are credited and that the original publication in this journal is cited, in accordance with accepted academic practice. No use, distribution or reproduction is permitted which does not comply with these terms.
*Correspondence: Tianyu Wang, wangtianyu@263.net
Yu Li, liyu03@caas.cn
†These authors have contributed equally to this work.