- 1Department of Agronomy, Iowa State University, Ames, IA, United States
- 2Department of Plant Pathology and Microbiology, Iowa State University, Ames, IA, United States
- 3Crop Genetics Research Unit, United States Department of Agriculture, Agricultural Research Service, Jackson, TN, United States
- 4Department of Botany and Plant Pathology, Purdue University, West Lafayette, IN, United States
- 5Delta Research and Extension Center, Mississippi State University, Stoneville, MS, United States
Charcoal rot (CR) disease caused by Macrophomina phaseolina is responsible for significant yield losses in soybean production. Among the methods available for controlling this disease, breeding for resistance is the most promising. Progress in breeding efforts has been slow due to the insufficient information available on the genetic mechanisms related to resistance. Genome-wide association studies (GWAS) enable unraveling the genetic architecture of resistance and identification of causal genes. The aims of this study were to identify new sources of resistance to CR in a collection of 459 diverse plant introductions from the USDA Soybean Germplasm Core Collection using field and greenhouse screenings, and to conduct GWAS to identify candidate genes and associated molecular markers. New sources for CR resistance were identified from both field and greenhouse screening from maturity groups I, II, and III. Five significant single nucleotide polymorphism (SNP) and putative candidate genes related to abiotic and biotic stress responses are reported from the field screening; while greenhouse screening revealed eight loci associated with eight candidate gene families, all associated with functions controlling plant defense response. No overlap of markers or genes was observed between field and greenhouse screenings suggesting a complex molecular mechanism underlying resistance to CR in soybean with varied response to different environments; but our findings provide useful information for advancing breeding for CR resistance as well as the genetic mechanism of resistance.
Introduction
Soybean [Glycine max (L.) Merrill] is one of the most economically important crops due to its potential as an oilseed crop and major source of plant protein used for both livestock and human consumption. The United States (US) is responsible for 33% of the world production with a record 106.96 million ton in 2015, grown on 33.5 million ha and prices range from $296 to $351 per ton (http://www.usda.gov). However, production can be strongly compromised by abiotic stresses, pests, and pathogens (Hartman et al., 2015).
Charcoal rot (CR), a disease caused by Macrophomina phaseolina, can reduce both yield and seed quality (Smith and Wyllie, 1999). For economically important soybean diseases, it ranked among the top 10 in the US from 1996 to 2014, with an average of 1 million ton of yield loss according to the Extension and Outreach at the University of Illinois (extension.cropsciences.illinois.edu), and sixth in the top eight soybean producing countries in 2006 (Wrather et al., 2010). Charcoal rot is distributed worldwide in the tropics and sub-tropics, as well as in the US north central and southern regions (Wyllie, 1988), and M. phaseolina is known to infect over 500 plant species of economic importance including maize, sorghum (Adeyanju et al., 2015), and sunflower (Pawlowski et al., 2015). Annually, CR is a greater concern in the southern US due to frequent hot and dry conditions that tend to occur during important soybean developmental growth stages.
M. phaseolina is a soil- and seed-borne polyphagous fungus. The abundant production of minute black microsclerotia causes the infected plant tissues to blacken, and therefore, the disease is known as charcoal rot (Sarr et al., 2014). The fungus survives in the soil mainly as microsclerotia that are stimulated by root exudates to germinate and infect host plant material. Limiting soil moisture and higher air and soil temperature increases disease severity (28–35°C; Smith and Wyllie, 1999). The pathogen may move from infected roots to stems, clogging vascular tissues in the tap root (Kaur et al., 2012), and to seed, causing reduced germination, and seedling rots. Aboveground symptoms of CR appear after flowering (soybean growth stage R1), and are particularly evident in soybean fields at the R5 (beginning seed), R6 (full seed), and R7 (beginning maturity) growth stages (Wyllie, 1988). In severe situations, diseased plants may wilt and prematurely die.
Charcoal rot management strategies in soybean include cultural methods, seed-applied fungicide, and biological control, but these have not been effective or widely adopted and have provided limited control (Mengistu et al., 2015). In this scenario, genetic resistance may be the most feasible and sustainable method to manage CR (Mengistu et al., 2007). Complete resistance to M. phaseolina is not reported in any plant species, but identification of partial resistance has been reported in soybean, including moderately resistant cultivars, such as DT97-4290, used as a disease check standard (Paris et al., 2006; Mengistu et al., 2007, 2013; Twizeyimana et al., 2012; Pawlowski et al., 2015). However, investigations into commercially available germplasm and their general response to the fungus have not been widely performed.
Breeding for resistance is difficult because most diseases are quantitatively inherited and controlled by multiple genes; in such a scenario, methodologies that help to elucidate resistance mechanisms, and identify resistant genotypes contribute to increased success in breeding programs (St Clair, 2010). Primarily, breeding efforts have been focused on genetic entries from later maturity groups (MGs; e.g., MG IV and V), which are coincident with predominant CR regions in the southern US; however, the identification of CR in northern latitude soybean growing regions require research and breeding efforts in earlier maturity regions that plant early maturity varieties (typically MG III and earlier). Breeding efforts can be complemented with genome wide association studies (GWAS) as these serve a dual role of disease screening as well as identification of genetic markers and candidate genes.
Genome-wide association studies associate variation across the entire genome with phenotypes (Korte and Farlow, 2013) and are used to identify genetic variations of important traits including disease resistance (Iquira et al., 2015). These GWAS use high-density markers and a population of diverse individuals to provide greater mapping resolution than conventional methodologies, which enables the prediction or identification of putative causal genes, and reduce time and cost for the genetic dissection of traits (Song et al., 2013; Zhang et al., 2015b). In soybean, GWAS have been previously utilized to identify genes associated with resistance to Phytophthora root rot (Sun et al., 2014), soybean cyst nematode (Bao et al., 2014; Vuong et al., 2015), iron deficiency chlorosis (Mamidi et al., 2014; Zhang et al., 2017), sudden death syndrome (Wen et al., 2014; Zhang et al., 2015a,b), Sclerotinia stem rot (Bastien et al., 2014; Iquira et al., 2015; Zhao et al., 2015), and soybean aphid (Chang et al., 2016).
The aims of this study were to (1) identify new sources of CR resistance in 459 diverse soybean plant introduction (PI) lines from MG I, II, and III through field and greenhouse screening, and (2) conduct GWAS to increase our understanding of the resistance mechanisms and identify genetic markers associated with resistance that will contribute to the future selection of genotypes for breeding programs and genetic studies for CR resistance.
Materials and Methods
Two experiments were conducted to identify CR resistance sources and to locate candidate genes and markers related to resistance. The first experiment was a field screening using root and stem severity (RSS; Mengistu et al., 2007) to classify genotypes for resistance. The second experiment was a greenhouse screening utilizing the cut-stem methodology (Twizeyimana et al., 2012) to classify genotypes for resistance based on the area under the disease progress curve (AUDPC).
Plant Material
A collection of 459 soybean PI lines, spanning MG I to III, was obtained from the United States Department of Agriculture (USDA) Soybean Germplasm Collection. Two breeding lines (DT97-4290 provided by the USDA (Paris et al., 2006), and H3LER11017-00-0238 provided by Pioneer) were used as moderately resistant checks, and two others (Pharaoh provided by the USDA (Schmidt et al., 1993), and H3LER11022-00-0037 provided by Pioneer) were used as susceptible checks.
Macrophomina phaseolina Isolate and Culture Maintenance
An isolate of M. phaseolina collected from an Iowa soybean field in 2013 (D. Mueller lab, ISU) was used for all associated studies as outlined below. This isolate will be available to researchers with appropriate permits. From the plate of mycelium obtained from Dr. Mueller's lab, a mycelial plug transfer was made to create inoculum on PDA. We inoculated the fungus onto susceptible plants, then re-isolated the fungus to inoculate the soybean plants (field and greenhouse experiments in this study). The fungus had the same morphological characteristics as the original plate as well as showed similar symptoms on the susceptible plant inoculations. We completed Koch's postulates with the re-isolated pathogen (to inoculate susceptible soybean plants) to confirm its pathogenicity.
The inoculation method was adapted from Mengistu et al. (2007). Sorghum (Sorghum bicolor L.) seed (400 mL by volume) was soaked for 24–48 h in 4 L of distilled water. The liquid was decanted, and seed were equally divided and put into autoclavable bags. The autoclave cycle consisted of 121°C for 30 min, and samples were autoclaved twice. The autoclaved sorghum seed were put in bags containing 1.8 kg of sorghum grain and 1-week-old culture plugs of M. phaseolina (grown on PDA) were placed into each bag to inoculate the sorghum at a rate of 1 plate of fungus per 1.8 kg of seed. The bags were closed and incubated at 30°C for 2 weeks while periodically shaking the bag. After 2 weeks, the sorghum seed were completely colonized by the fungus and were darkened with microsclerotia. These seed were removed from the sealed bags to allow air drying, and were then stored in sealed plastic containers at 4°C until use.
Field Experiment
Four hundred sixty five soybean genotypes including 459 PI lines, four maturity checks (MN1410, LD02-4485, IA3023, and IA4005), one moderately resistant check (H3LER11017-00-0238, provided by Pioneer), and one susceptible check (H3LER11022-00-0037, provided by Pioneer), were grown near Muscatine, IA, in 1.52 m long single rows with 0.76 m row to row distance and 0.91 m alleyways. Maturity and disease checks were spaced every 100 and every 50 entries, respectively. Genotypes were arranged in a randomized complete block design (RCBD) with three replications. During field planting, the planter was calibrated to apply 3 g of charcoal rot-infected sorghum seed per linear 0.3 m in furrow with the soybean seed at a rate of 8 seeds/0.3 m using a 4-row planter (Almaco Company, Nevada, IA).
Stem collection and charcoal rot ratings were based on the evaluation of root and stem severity rating (RSS; Mengistu et al., 2007). For each replication, five plants of each genotype were randomly harvested between the R7 and R8 growth stage from each row. Stem and top of the tap root portion of each plant was obtained by gently uprooting each plant and clearing it of the soil and other debris. Each plant stem was longitudinally split using a sharp knife and ratings were given on a scale of 1–5 (Figures 1A,B).
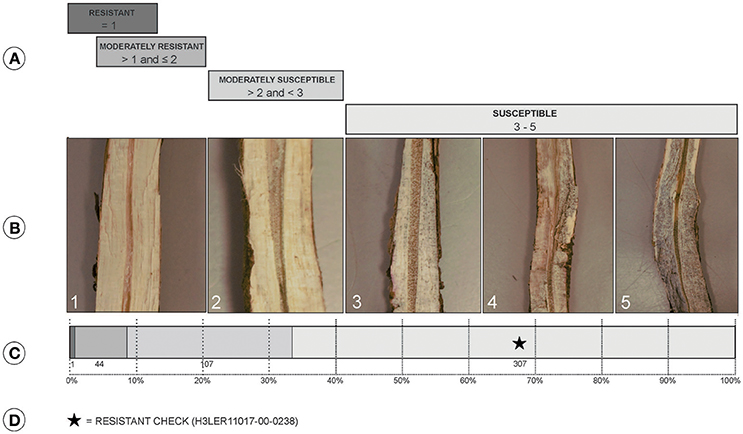
Figure 1. (A) Classes of charcoal rot resistance where resistant (values of 1), moderately resistant (values >1 and ≤2), moderately susceptible (values >2 and <3), and susceptible (values 3–5). (B) Split stem showing symptoms associated with the scores: 1, no microsclerotia visible in vascular tissue; 2, very few microsclerotia visible and vascular tissue is not discolored; 3, microsclerotia partially covering the vascular tissue and there is minimal discoloration; 4, numerous microsclerotia in the tissue and also visible under the outside epidermis, and discolored vascular tissue; 5, darkened vascular tissue due to high numbers of microsclerotia both inside and outside of the stem. (C) Distribution of the 459 PI lines and checks for resistance classification. (D) Class of location of the resistant check in accessions distribution.
Greenhouse Screening
A total of 463 soybean genotypes were evaluated including 459 PI lines and four checks, two resistant and two susceptible checks repeated twice per block. The genotypes were arranged in a randomized complete block design (RCBD) with four replications. Two plants of each genotype in cups made for an experimental unit in each block. Plants were grown in 0.24 L styrofoam cups, with holes in the bottom for drainage, filled with soilless mix (Sunshine Mix, LC1; Sun Gro Horticulture Inc., Agawam, MA) and topped with pellets of slow-release fertilizer (Osmocote Plus 19-9-12, 23 g per cup) spread over the surface of each cup. Seeds were over sown, three seeds per cup, in each pot and thinned to two plants 10 days post-emergence. All experiments were conducted in a greenhouse maintained at 30°C day and 22°C night temperatures, and the room was supplemented with high-pressure 400 W sodium lights to ensure the 16-h photo period. Plants were watered manually to avoid plant wilting. The cut-stem inoculation technique was used to classify genotypes for resistance (Twizeyimana et al., 2012). Briefly, soybean plants were grown to the V2 growth stage and a razor sharp blade was used to cut 40 mm above the unifoliate node. From the growing margin of a 4-day old culture of M. phaseolina on PDA, a mycelial plug was obtained using a 200 μL pipette tip (Fisher Scientific). The pipette tip with mycelial plug was immediately placed over the stem cut by the razor blade and ensuring the agar was in embedded in the stem. Ratings were based on the recorded measurements of lesion length. The details of measurements are provided in Pawlowski et al. (2015) with the modification that the length of cut-stem at the time of inoculation was 40 mm instead of 25 mm used in their publication. Measurements were taken the third day after inoculation (dai), and followed every 3 days, for a total of 5 ratings (3, 6, 9, 12, and 15 dai). The area under the disease progress curve (AUDPC) for each entry was calculated to estimate the disease resistance and select superior PI lines (Jeger and Viljanen-Rollinson, 2001). AUDPC was used for GWAS using greenhouse data.
Statistical Analyses
The model for the phenotypic trait was Yij = μ + gi + bj + eij, where μ is the total mean, gi is the genetic effect of the ith genotype, bj is the block effect, and eij is a random error following N(0, ). Broad sense heritability was calculated as H2 = /[ + /r], where is the genotypic variance, is the error variance, and r is the number of replications. The estimation of variance components was performed by R software with all effects considered to be random.
Genotyping and Quality Control
The SNP dataset of the association panel was prepared by a previous study using the Illumina Infinium SoySNP50K BeadChip and was retrieved from the SoyBase (https://soybase.org/; Sonah et al., 2015). Of the 42,180 SNPs available for the association panel, 60 SNPs failed to anchor to the reference genome sequence and were excluded from further analyses. Individual markers with missing rates >10% were omitted, and the remaining missing data were imputed using BEAGLE version 3.3.1 with default parameter settings (Browning and Browning, 2007, 2009). SNPs with a minor allele frequency (MAF) <5% after imputation were also omitted for further analyses. Finally, 35,683 SNPs were used for GWAS.
Marker Distribution and Linkage Disequilibrium Estimation
The Glyma.Wm.82.a2 reference genome was used to obtain chromosome physical lengths (bp) through SoyBase (www.soybase.org) which were used to calculate genome-wide inter-marker distance and chromosome-wide densities. Pairwise linkage disequilibrium (LD) between markers was measured using the squared correlation coefficient (r2) between alleles with the R package, synbreed (Wimmer et al., 2012). The r2 was calculated separately for euchromatic and heterochromatic regions due to the variability of recombination. Only significant r2 values (P < 0.001), calculated according to Remington et al. (2001), were considered informative. The chromosomal distance where the average r2 dropped to half of its maximum value was used to estimate the LD decay rate of the population (Huang et al., 2010).
Genome-Wide Association Analysis
To minimize the effect of environmental variation, best linear unbiased predictors (BLUPs) of individual lines were calculated using the R package, lme4 (Bates et al., 2014) for further analysis. The association analysis was conducted by using the genome assessment and prediction integrated tool (GAPIT) R package as descried in previous studies (Zhang et al., 2010; Lipka et al., 2012). No population structure was involved in the mixed linear model as a covariant as suggested by the Bayesian information criteria test.
The threshold for significant associations was determined by the empirical significance level of P < 0.001. To access the empirical significance of SNPs, a total of 1,000 permutations of genome-wide association was performed as previously described (Zhang et al., 2015a). For each iteration, the phenotype values and kinship matrix (K) in the MLM remained unchanged while genotypes of each SNP were permuted. The threshold was set at the lowest P-value of the SNP-trait association that did not meet the empirical significance level.
Prediction of Putative Candidate Genes
Genes annotated in Glyma1.1, Glmy1.0, and NCBI RefSeq gene models, available through SoyBase aligning to the Glyma.Wm.82.a2 reference genome (www.soybase.org), were used as the source of candidate genes. The significant SNPs in LD r2 > 0.7 with the peak SNP were clustered to form the candidate region of the quantitative trait locus (QTL). The peak SNP is defined as the SNP with the lowest P-value within the region defined above. The prediction of candidate genes resulted from the following priorities: (i) genes of known function related to soybean disease resistance, (ii) genes of known function as orthologs related to disease resistance in Arabidopsis, and (iii) genes pinpointed by the peak SNPs.
Results
Field Screening
The RSS method, used in field screening, enabled identification of accessions with better disease resistance than the resistant checks (Figure 1). The mean score was 3.5 with a standard deviation of 1.05. PI379559D had the lowest score of 1.0 and classified as resistance as per the RSS classification (Paris et al., 2006; Mengistu et al., 2007). Twenty-six accessions had a score of 5.0, and the moderately resistant check, H3LER11017-00-0238, had a score of 4.0. Moderately resistant accessions present scores ranging from values >1 and ≤2, and for this experiment 44 accessions met these criteria. However, 90% of the accessions were classified as moderately susceptible and susceptible, with values >2 and ≤5.
Greenhouse Screening
Significant differences among the genotypes were observed (P < 0.001). The mean AUDPC value was 470 with a standard deviation of 124. PI603444A had the highest AUDPC value of 1,036, and PI567241 had the lowest AUDPC value of 270. The moderately resistant checks, DT97-4290 and H3LER11017-00-0238, had AUDPC values of 359 and 382, respectively, and the susceptible checks, Pharaoh and H3LER11022-00-0037, had a value range of 465 and 567, respectively.
Accessions with better resistance than the resistant checks were identified in the greenhouse screening (Figure 2). Among the 459 accessions, 51 (11%) exhibited AUDPC values less than the resistant checks. All accessions, even the most resistant, developed a lesion, which indicated that infection had occurred but that the plant was able to stop fungal development.
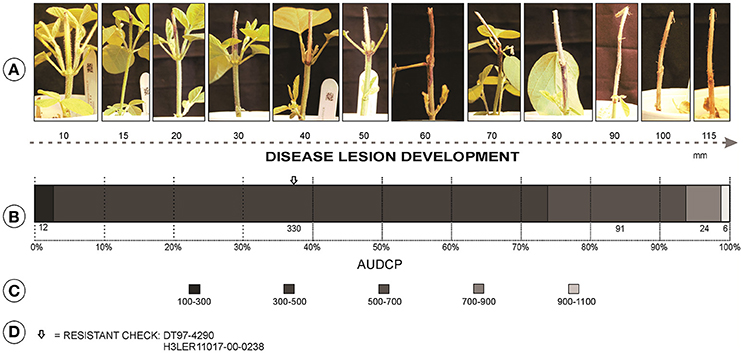
Figure 2. (A) Variation of disease lesion length (mm) for charcoal rot from disease screening in greenhouse. (B) Distribution of the 459 PI lines and checks for resistance classification based on AUDPC. (C) Classes of distribution of the AUDPC. (D) Class of location of the resistant checks in the distribution of the accessions.
Genotyping and Quality Control
For field screening, 155 (34%) accessions exhibited better CR resistance than the resistant check, and for greenhouse screening, 30 (7%) accessions were more resistant than the resistant checks. Table 1 shows the top 20 genotypes selected from maturity groups I–III for disease resistance from each experiment (field and greenhouse screening). Heritability values ranged from 0.60 to 0.58 for greenhouse and field screening, respectively (Supplementary Table 1). The field and greenhouse screening had a low but significant negative correlation (rfg = −0.12). Among all the accessions selected for the field and the greenhouse, 22 (5%) were common between field and greenhouse experiments (Table 2) showing a good level of resistance in both and included accessions PI379559D and PI603594 with the lowest RSS and AUDPC scores, respectively.
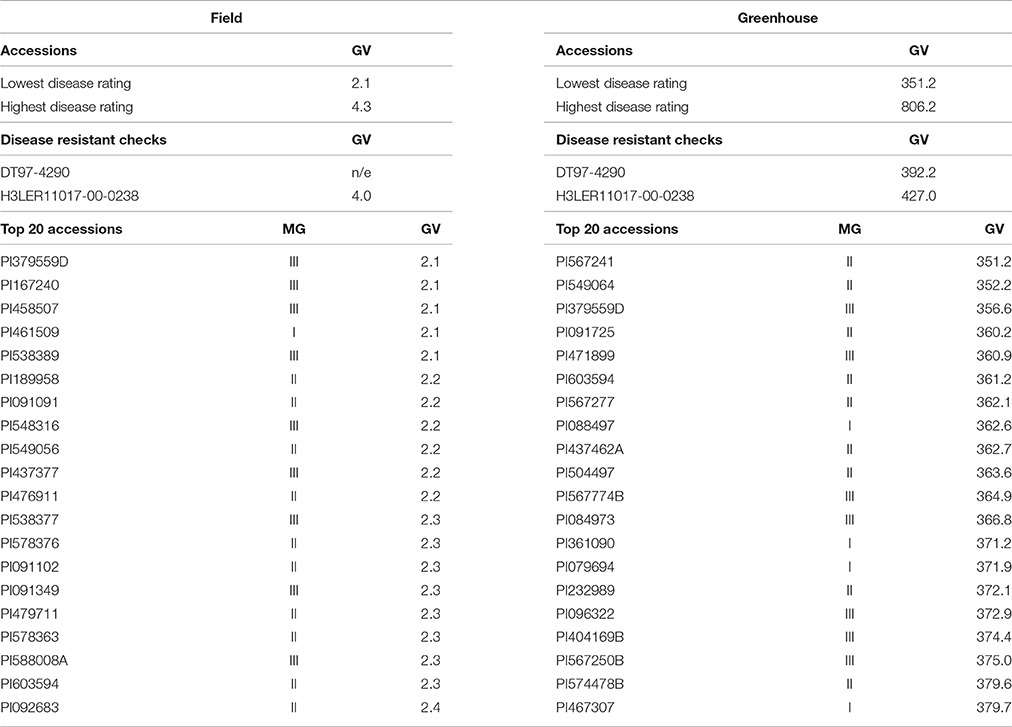
Table 1. Genotypic value (GV) and maturity group (MG) of the top 20 plant introduction lines selected for charcoal rot resistance that were better than the resistant checks, variation of GV for low and high ratings, and GV for the resistant checks for field and greenhouse screening for charcoal rot resistance.
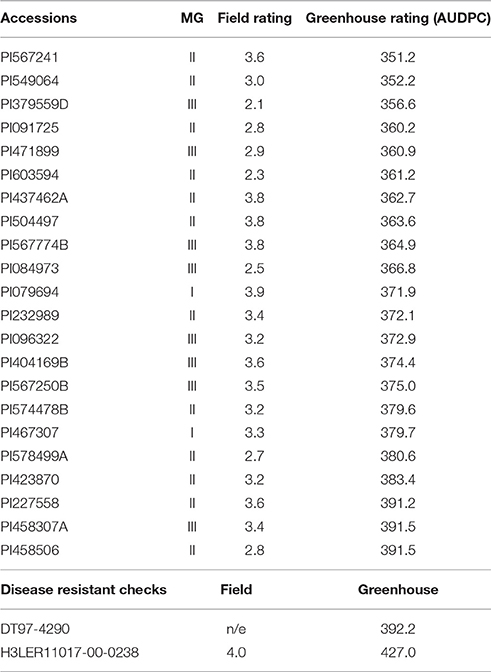
Table 2. Selection of soybean genotypes with better charcoal rot resistance than the resistant checks for both field and greenhouse experiments using 459 plant introduction accessions from the USDA germplasm bank.
Genome-Wide Association Analysis and Prediction of Putative Candidate Genes
A total of 19 SNPs associated with charcoal rot resistance were identified across chromosomes 4, 14, and 18 for field experiments, and chromosomes 6, 8, 9, 12, 18, and 20 for greenhouse experiments (Supplementary Figure S1). Based on the results of GWAS and genes annotated in SoyBase (www.soybase.org), four putative candidate genes were predicted for four of the six loci associated with field RSS scores. They are orthologs of Arabidopsis genes related to stress response, antimicrobial properties, and cell wall functions (Table 3). For the greenhouse AUDPC, a total of 11 putative candidate genes were predicted for eight of the 30 loci associated with the trait. Three of them are orthologs of Arabidopsis genes related to cell defense components and disease plant resistance proteins (Table 3).
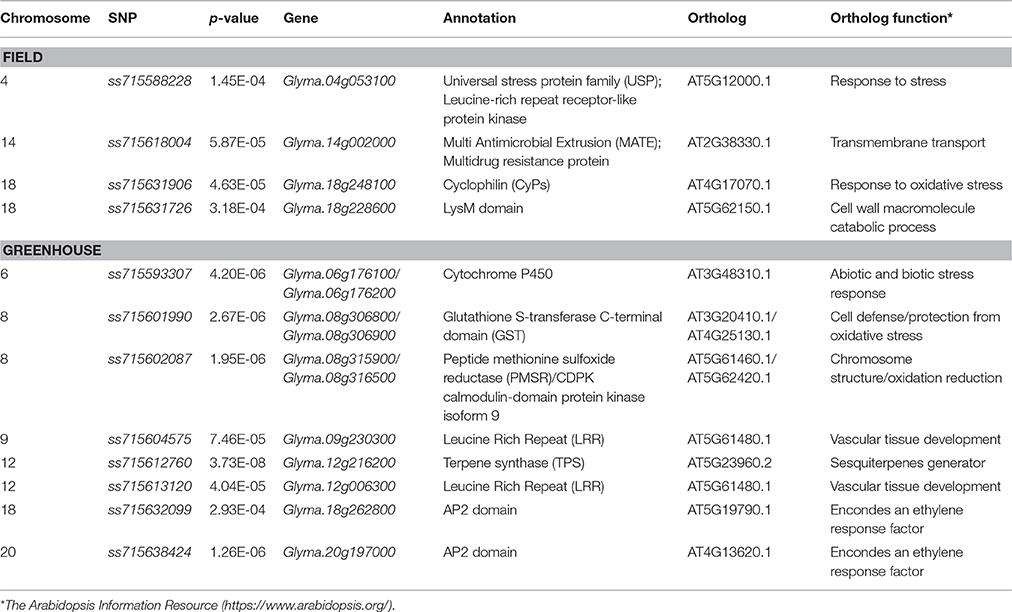
Table 3. SNPs significantly associated with charcoal rot resistance through GWAS and predicted candidate genes for field and greenhouse screening.
Field screening identified four putative candidate genes (Table 3). The one, Glyma.04g053100, on chromosome 4 was identified at 6.4 kb downstream of ss715588228. Glyma.04g053100 belongs to a universal stress protein family (USP) protein and is homologous to the AT5G12000.1 that encodes a Leucine-rich repeat receptor-like protein kinase response to stress (Table 3). On chromosome 14, the candidate gene Glyma.14g002000 was identified at 6.9 kb away from the peak SNP ss715618004. It encodes a multidrug resistance protein and is homologous to the AT4G38380.1, which encodes a transporter of plant metabolites related to defense signals (Table 3). On chromosome 18, two gene candidates, Glyma.18g248100 and Glyma.18g228600, were found at the proximity of the peak SNP ss715631906 and ss715631726, respectively. The former is homologous to Arabidopsis AT4G17070.1 encoding a peptidyl-prolyl cis-trans isomerase and is related to the response to oxidative stress and pathogen infection. The latter produces a protein containing the LysM domain, which is related to cell wall catabolism and fungal pathogen defense in Arabidopsis (Table 3).
Greenhouse study identified eight loci across six chromosomes (Table 3). On chromosome 6, two candidate genes were identified at 24.5 and 14.8 kb upstream of the peak SNP ss715593307. Both encode a Cytochrome P450 protein involved in biosynthetic reactions including defensive compounds such as terpenoids. On chromosome 8, two loci were identified, each with two candidate genes. Glyma.08g306800 and Glyma.08g306900 at the proximity of ss715601990 encoding a Glutathione S-transferase, proposed to be involved in the synthesis of stress-related proteins and defense components. The second locus was targeted by two SNPs in high LD (r2 > 0.7). Two genes located at 21.3 kb downstream and 35.8 kb upstream of the leading SNP ss715602087 were identified. Glyma.08g315900 and Glyma.08g316500 encode a peptide methionine sulfoxide reductase (PMSR) and a Calmodulin-domain Protein Kinase (CDPK), respectively, and both are related to abiotic and biotic stress responses. On chromosome 9, Glyma.09g230300 was identified 15.6 kb downstream of the lead SNP ss715604575. It encodes a leucine rich repeat (LRR) protein that is involved in disease resistance mechanisms. The candidate gene, Glyma.12g006300, was identified in a region consisting of 16 significant SNPs in high LD (r2 > 0.7) on chromosome 12. It also encodes a LRR type protein and is related to disease defense components and stress related responses. On chromosome 18, putative candidate gene Glyma.18g262800 is located 2.9 kb downstream of the peak SNP ss715632099 and encodes an AP2 domain-containing inductor of ethylene-responsive element (ERE) that is associated with plant disease defense. Similarly, Glyma.20g197000 encoding an AP2 domain-containing protein was found at the close proximity of the peak SNP ss715638424 on chromosome 20.
Discussion
Charcoal rot is an important fungal disease; however, limited information is available on the resistance of earlier maturity soybean accessions to this disease in the US. From both field and greenhouse screenings in the current research, new sources of charcoal rot resistance were identified for MGs I to III among the accessions that performed better than or similar to the resistant checks. Pawlowski et al. (2015) reported three PI lines from maturity groups I, II, and III exhibiting partial resistance to M. phaseolina in a greenhouse screening of 81 genotypes using the cut-stem technique; however, there was no overlap with the genotypes from this study. The present study identifies previously unreported sources of charcoal rot resistance from early MGs (I, II, and III), contributing to the development of CR resistant cultivars adapted to northern soybean growing regions in the US, and complements the screening of soybean genotypes in the later maturities (MG IV and beyond; Mengistu et al., 2007). The identification of these accessions, together with the heritability value, reinforces the existence of disease expression repeatability and usefulness to breeding programs. Utilization of field and greenhouse testing for charcoal rot resistance allowed the identification and selection of soybean accessions with better disease resistance than the resistant check in both field and greenhouse environments.
The field and greenhouse screening allowed the comparison of different environments in an attempt to understand the mechanisms of resistance involved in each and to verify correlation between expression of resistance in field and greenhouse environments. The correlation between field and greenhouse experiments was significant, but showed a low negative value (rfg = −0.12). Charcoal rot resistance has been screened primarily through field evaluations (Bristow and Wyllie, 1984; Pearson et al., 1984; Smith and Carvil, 1997; Mengistu et al., 2007). However, with the development of new inoculation methodologies, some screenings have been evaluated in controlled environments of greenhouse and growth chamber (Bristow and Wyllie, 1984; Surrette et al., 2006; Twizeyimana et al., 2012). No researchers screened plants under both field and greenhouse conditions, although Twizeyimana et al. (2012) had reported a comparable result for the cut-stem methodology with previously reported field results. It is important to highlight that these comparable results compared 16 PI lines pre-screened for resistance and did not represent a true correlation of a heterogeneous population consisting of variable resistance responses.
The lack of correlation between field and greenhouse experiments for disease assessment is commonly reported in literature (Kim and Diers, 2000; Hoffman et al., 2002; Hartman et al., 2014). Environmental factors, including rainfall and temperature, and plant maturity have major effect on the severity of CR and must be considered when correlating greenhouse and field studies that evaluate a diverse maturity set of soybean accessions (Pawlowski et al., 2015). Several issues with greenhouse and field disease experiments, such as control of environmental conditions, physiological differences between genotypes, resistance mechanisms, uniform concentrations of inoculum, and inoculation location on the plant should be considered to help standardize and correlate future research efforts. Plants in field tests are indirectly inoculated by placing inoculum with the seed during planting, and the initial inoculum content of soil is generally not quantified. Furthermore, the rating methodologies for field and greenhouse screening may be of influence because one rating is subjective based on visual symptoms (RSS), and the other is directly quantified by measuring the extent of necrosis (cut-stem method). Another issue is related to the plant growth stage and physiology. A plant's response to abiotic and biotic stresses involves complex signaling pathways that depend on numerous genes, proteins, and metabolites, reflecting in differences in the resistance mechanism, which may also vary across life stages (Radwan et al., 2013). In the current studies, disease evaluation occurred at the V2 growth stage in the greenhouse screening, and R7 growth stage for the field screening. Response differences due to artificial wounding (in cut stem method) and growth stage may relate to the expression of different genes for disease reaction mechanisms, and environmental conditions for field settings can generate genotype by environment interactions.
Few accessions showed better performance than the checks for both field and greenhouse screening (22 accessions from 459), and need careful development of strategies for selection. Although greenhouse screening is considered faster and less laborious, the correlation between resistance observed in the greenhouse vs. in field screening is not consistently related. Therefore, field screening, which better represents the final environment for crops, should still be considered in the screening process. However, it should be considered that the available methods for CR assessment are few and new, and still under processes of improvement. In this scenario, further investigation for field and greenhouse methods which correlate well in classifying genotypes for CR resistance are needed and recommended to enable secure and reliable selection and classification of resistant genotypes. The interest in greenhouse screening still exists because it is less time and resource intensive'; however, only after a greenhouse screening methodology that is positively correlated with field expression is developed, can controlled (indoor) condition screening be effectively used as a pre-screening prior to a more thorough field screening.
M. phaseolina is known as a generalist because no specific resistance genes are reported in any of its hosts. There is no complete resistance reported for this pathogen among the host species probably because resistance is quantitatively controlled. The current research is the first study to our knowledge to use a genomic tool (i.e., GWAS) to identify genes related to CR resistance in soybean in order to better understand the pathway of genetic expression and association with resistance. Five gene families were associated with the resistance response for the field screening. Among them, the USP family and the cyclophilins (CyPs) are both related to stress response. USPs are small cytoplasmic proteins which have been related to pathogen-plant interaction. Lenman et al. (2008) reported that the USP were the first plant protein to phosphorylate in response to the inoculum presence when inoculating Arabidopsis plants with Phytophthora spores. CyPs are ubiquitous proteins, that are induced by various stresses including temperature (low or heat shock), light, salt stress, physical injury, and pathogens (Marivet et al., 1994). In diseased plants, cyclophilin may function as a “chaperon-like molecule” that decrease the risk of proteolytic degradation or help avoid accumulation during stress.
One of the genes identified is a characterized member of the multi antimicrobial extrusion (MATE) protein which has been reported to be involved in many physiological functions. Among these functions are alkaloid accumulation, flavonoid accumulation, and plant hormone signaling, all directly related to defense responses against herbivores and pathogens (Shitan, 2016). MATE transporters are associated with plant disease resistance, transporting compounds such as salicylic acid (SA) that plays an essential role in plant innate immune signaling and in disease resistance. Initiation of plant antimicrobial defenses in response to attempted microbial infection relies on a molecular dialog between the interacting organisms. LysMs genes have been associated in the recognition of carbohydrate patterns commonly related with microbial surfaces and in microbial infection immunity (Willmann et al., 2011). Wan et al. (2008) demonstrated that plant cells can perceive chitin fragments that lead to gene induction and defense responses by a LysM receptor-like kinase1 (LysM RLK1) in Arabidopsis thaliana, indicating that LysM RLK1 is essential for chitin signaling and plant innate immunity.
For the greenhouse screening, eight gene families were related to the mechanism of response to CR resistance. Among them, a cytochrome P450 was identified on chromosome 6, which is widely reported for its function in plant defense, including soybean cyst nematode and soybean rust (Irmisch et al., 2015; Wan et al., 2015; Langenbach et al., 2016). Three candidate genes were proposed on chromosome 8. Glutathione S-transferases (GSTs) has been connected in reactions linked to secondary metabolism and response to pathogen, including its importance in regulation of jasmonic acid (Han et al., 2013) and has been reported in multiple species, for example, Populus tomentosa (Liao et al., 2014) and Lilium regale Wilson (Han et al., 2016). Peptide methionine sulfoxide reductase (PMSR) acts as an antioxidant, repairing proteins damaged from oxidative stress and a novel defensive role against attack by pathogens Phytophthora capsici and P. infestans on pepper (Capsicum annuum) via regulation of the cellular levels of reactive oxygen and defense-related genes (Oh et al., 2010). Calcium-dependent protein kinases (CPKs or CDPKs) are regulated by various external stimuli including temperature stress (heat or cold), water stress, salinity, wounding, and biotic stress. NtCDPK2 has been reported to be activated by race-specific pathogen elicitation (Cf-9/Avr9) and abiotic stress in tobacco (Romeis et al., 2000). On chromosome 12 a strong association was detected and leucine rich repeat (LRR) and Terpene synthase (TPS) related candidate genes were identified. LRR proteins plays a crucial role in the plant's defense against pathogens, for their “immune” functions and recognition of non-self-molecules, including from GWAS in soybean (Li et al., 2016). Genome-wide identification and evolutionary analysis of LRR genes in soybean reported 467 putative LRR-receptor like kinase (LRR-RLK) genes in the soybean genome (Zhou et al., 2016). Terpene synthase (TPS) act as signaling molecules that induce defenses against tissue damage from wounds and microbes infected sites. Recent research demonstrated a terpene synthase 24 (OsTPS24) encoding a jasmonate-responsive monoterpene synthase that produces an antibacterial γ-terpinene against pathogens in rice (Yoshitomi et al., 2016). On chromosome 18 and 20, the APETALA2/ethylene-responsive element binding protein (AP2/EREBP) plays various roles in plant growth and development, and in response to stresses as pathogen infection, drought, temperature, and salinity (Zhang et al., 2013; Tang et al., 2016). It has been reported that the ethylene pathway was activated in response to the pathogen attack in several crop species (Dong et al., 2015; Wang et al., 2015; Agarwal et al., 2016; Hong et al., 2016).
Genome wide association studies have been reported as a very successful approach to investigating SNP-disease associations and deciphering the trait genetic architecture. The results reported in this study suggest that CR has a complex genetic architecture, with a polygenic background, classified as a quantitative trait. Due to its quantitative nature, information generated in this study is more suitable applied to genomic selection approaches rather than marker assisted selection (MAS), as MAS is inadequate for improving quantitatively inherited, i.e., polygenic traits. Genomic selection can improve genetic value estimations and selection accuracy for breeding for CR resistance. The information related to the candidate genes and markers reported are the first report for CR disease in soybean and require validation of marker-trait association in bi-parental mapping populations. Additionally, it is highly necessary that the genes are submitted to validation studies through gene silencing and expression studies.
It is important to highlight that CR disease is a disease commonly observed in the southern soybean production region of the US, especially during years when hot and dry conditions persist (Romero Luna et al., 2017). The disease has been spreading to the northern soybean growing regions of the US and have been reported in northern states including Iowa (Yang and Navi, 2005), Michigan (Baird et al., 2010), Minnesota (Elaraby et al., 2003), North Dakota (Bradley and Río, 2003), and Wisconsin (Birrenkott et al., 1984). More information about this disease and its evolution through the production regions is needed in order to develop resistant cultivars. Additional research is needed to determine if resistance mechanisms are the same or different in northern and southern adapted germplasm.
Conclusion
The current study is the first effort to apply GWAS to understand and explain the genetic mechanisms underlying resistance to charcoal rot in soybean. We identified 5 and 8 loci for field and greenhouse screening, respectively, which were associated with candidate genes involved in controlling the plant defense response. However, the lack of overlap in significant SNP and candidate gene in field and greenhouse screenings, indicates that different mechanisms are involved in disease resistance expression in field and greenhouse. This research serves as a basis for the identification of candidate genes involved in CR resistance and unravels the complexity of this resistance. In the materials studied in our study, due to the prevalence of smaller effect loci controlling CR, genomic selection is an attractive strategy. The genetic entries identified in this paper will be useful for improving charcoal rot resistance in soybean.
Author Contributions
AKS and AS formulated research problem and designed the approaches, AKS and AS directed field efforts and phenotyping; SC and RC collected phenotypic data; SC, JZ, RC, AKS analyzed the data. All authors contributed to the preparation and development of manuscript.
Funding
The authors are grateful to CAPES/Cnpq for the scholarship support to SC (PhD student, Universidade Federal de Viçosa) for a visiting fellowship at Iowa State University. Research funding support from the United Soybean Board through “Improved Management of Charcoal Rot and Stem Canker” USB Project, USDA-CRIS IOW04314 project (Crop Genetic Improvement and Adaptation Using Gene Discovery, Phenotypic Prediction, and Systems Engineering), Monsanto Chair in Soybean Breeding (AKS), Baker Center for Plant Breeding at ISU (AKS) and Iowa State University is gratefully appreciated.
Conflict of Interest Statement
The authors declare that the research was conducted in the absence of any commercial or financial relationships that could be construed as a potential conflict of interest.
Acknowledgments
Support from members of Singh Soybean Breeding and Genetics Group for field experimentation is appreciated. We sincerely thank Jae Brungardt, Brian Scott, and Stith Wiggs for research experimentation. We thank Jennifer Hicks for editing the manuscript.
Supplementary Material
The Supplementary Material for this article can be found online at: http://journal.frontiersin.org/article/10.3389/fpls.2017.01626/full#supplementary-material
References
Adeyanju, A., Little, C., Yu, J. M., and Tesso, T. (2015). Genome-wide association study on resistance to stalk rot diseases in grain sorghum. G3 5, 1165–1175. doi: 10.1534/g3.114.016394
Agarwal, G., Garg, V., Kudapa, H., Doddamani, D., Pazhamala, L. T., Khan, A. W., et al. (2016). Genome-wide dissection of AP2/ERF and HSP90 gene families in five legumes and expression profiles in chickpea and pigeonpea. Plant Biotechnol. J. 14, 1563–1577. doi: 10.1111/pbi.12520
Baird, R. E., Wadl, P. A., Allen, T., McNeill, D., Wang, X. W., Moulton, J. K., et al. (2010). Variability of United States isolates of Macrophomina phaseolina based on simple sequence repeats and cross genus transferability to related genera within Botryosphaeriaceae. Mycopathologia 170, 169–180. doi: 10.1007/s11046-010-9308-3
Bao, Y., Vuong, T., Meinhardt, C., Tiffin, P., Denny, R., Chen, S., et al. (2014). Potential of association mapping and genomic selection to explore PI 88788 derived soybean cyst nematode resistance. Plant Genome 7, 1–13. doi: 10.3835/plantgenome2013.11.0039
Bastien, M., Sonah, H., and Belzile, F. (2014). Genome wide association mapping of Sclerotinia sclerotiorum resistance in soybean with a genotyping-by-sequencing approach. Plant Genome 7, 1–13. doi: 10.3835/plantgenome2013.10.0030
Bates, D., Maechler, M., Bolker, B., and Walker, S. (2014). lme4: Linear Mixed-Effects Models Using Eigen and S4. R. Package Version 1. Available online at: http://CRAN.R-project.org/package=lme4
Birrenkott, G., Mengistu, A., and Grau, C. (1984). First report of charcoal rot caused by Macrophomina phaseolina on soybeans in Wisconsin. Plant Dis. 68:628. doi: 10.1094/PD-68-628c
Bradley, C., and Río, L. D. (2003). First report of charcoal rot on soybean caused by Macrophomina phaseolina in North Dakota. Plant Dis. 87, 601–601. doi: 10.1094/PDIS.2003.87.5.601B
Bristow, W. E., and Wyllie, T. (1984). Reaction of soybean cultivars to Macrophomina phaseolina as seedlings in the growth chamber and field. Trans. Missouri Acad. Sci. 19, 10.
Browning, B. L., and Browning, S. R. (2007). Efficient multilocus association testing for whole genome association studies using localized haplotype clustering. Genet. Epidemiol. 31, 365–375. doi: 10.1002/gepi.20216
Browning, B. L., and Browning, S. R. (2009). A unified approach to genotype imputation and Haplotype-phase inference for large data sets of Trios and unrelated individuals. Am. J. Human Genet. 84, 210–223. doi: 10.1016/j.ajhg.2009.01.005
Chang, H. X., Brown, P. J., Lipka, A. E., Domier, L. L., and Hartman, G. L. (2016). Genome-wide association and genomic prediction identifies associated loci and predicts the sensitivity of tobacco ringspot virus in soybean plant introductions. BMC Genomics 17:153. doi: 10.1186/s12864-016-2487-7
Dong, L., Cheng, Y., Wu, J., Cheng, Q., Li, W., Fan, S., et al. (2015). Overexpression of GmERF5, a new member of the soybean EAR motif-containing ERF transcription factor, enhances resistance to Phytophthora sojae in soybean. J. Exp. Bot. 66, 2635–2647. doi: 10.1093/jxb/erv078
Elaraby, M., Kurle, J., and Stetina, S. (2003). First report of charcoal rot (Macrophomina phaseolina) on soybean in Minnesota. Plant Dis. 87, 202–202. doi: 10.1094/PDIS.2003.87.2.202C
Han, Q., Chen, R., Yang, Y., Cui, X., Ge, F., Chen, C., et al. (2016). A glutathione S-transferase gene from Lilium regale Wilson confers transgenic tobacco resistance to Fusarium oxysporum. Sci. Hortic. 198, 370–378. doi: 10.1016/j.scienta.2015.11.047
Han, Y., Mhamdi, A., Chaouch, S., and Noctor, G. (2013). Regulation of basal and oxidative stress-triggered jasmonic acid-related gene expression by glutathione. Plant Cell Environ. 36, 1135–1146. doi: 10.1111/pce.12048
Hartman, G. L., Chang, H. X., and Leandro, L. F. (2015). Research advances and management of soybean sudden death syndrome. Crop Protect. 73, 60–66. doi: 10.1016/j.cropro.2015.01.017
Hartman, Y., Hooftman, D. A., Uwimana, B., Schranz, M. E., Van De Wiel, C. C., Smulders, M. J., et al. (2014). Abiotic stress QTL in lettuce crop-wild hybrids: comparing greenhouse and field experiments. Ecol. Evol. 4, 2395–2409. doi: 10.1002/ece3.1060
Hoffman, D. D., Diers, B. W., Hartman, G. L., Nickell, C. D., Nelson, R. L., Pedersen, W. L., et al. (2002). Selected soybean plant introductions with partial resistance to Sclerotinia sclerotiorum. Plant Dis. 86:980. doi: 10.1094/PDIS.2002.86.9.971
Hong, K., Gong, D., Zhang, L., Hu, H., Jia, Z., Gu, H., et al. (2016). Transcriptome characterization and expression profiles of the related defense genes in postharvest mango fruit against Colletotrichum gloeosporioides. Gene 576, 275–283. doi: 10.1016/j.gene.2015.10.041
Huang, X., Wei, X., Sang, T., Zhao, Q., Feng, Q., Zhao, Y., et al. (2010). Genome-wide association studies of 14 agronomic traits in rice landraces. Nat. Genet. 42, 967. doi: 10.1038/ng.695
Iquira, E., Humira, S., and Francois, B. (2015). Association mapping of QTLs for sclerotinia stem rot resistance in a collection of soybean plant introductions using a genotyping by sequencing (GBS) approach. BMC Plant Biol. 15:5. doi: 10.1186/s12870-014-0408-y
Irmisch, S., Zeltner, P., Handrick, V., Gershenzon, J., and Kollner, T. G. (2015). The maize cytochrome P450 CYP79A61 produces phenylacetaldoxime and indole-3-acetaldoxime in heterologous systems and might contribute to plant defense and auxin formation. BMC Plant Biol. 15:128. doi: 10.1186/s12870-015-0526-1
Jeger, M. J., and Viljanen-Rollinson, S. L. H. (2001). The use of the area under the disease-progress curve (AUDPC) to assess quantitative disease resistance in crop cultivars. Theor. Appl. Genet. 102, 32–40. doi: 10.1007/s001220051615
Kaur, S., Dhillon, G. S., Brar, S. K., Vallad, G. E., Chand, R., and Chauhan, V. B. (2012). Emerging phytopathogen Macrophomina phaseolina: biology, economic importance and current diagnostic trends. Crit. Rev. Microbiol. 38, 136–151. doi: 10.3109/1040841X.2011.640977
Kim, H. S., and Diers, B. W. (2000). Inheritance of partial resistance to sclerotinia stem rot in soybean. Crop Sci. 40:61. doi: 10.2135/cropsci2000.40155x
Korte, A., and Farlow, A. (2013). The advantages and limitations of trait analysis with GWAS: a review. Plant Methods 29:9. doi: 10.1186/1746-4811-9-29
Langenbach, C., Schultheiss, H., Rosendahl, M., Tresch, N., Conrath, U., and Goellner, K. (2016). Interspecies gene transfer provides soybean resistance to a fungal pathogen. Plant Biotechnol. J. 14, 699–708. doi: 10.1111/pbi.12418
Lenman, M., Sörensson, C., and Andreasson, E. (2008). Enrichment of phosphoproteins and phosphopeptide derivatization identify universal stress proteins in elicitor-treated arabidopsis. Mol. Plant Microbe Interact. 21:1284. doi: 10.1094/MPMI-21-10-1275
Li, L., Guo, N., Niu, J., Wang, Z., Cui, X., Sun, J., et al. (2016). Loci and candidate gene identification for resistance to Phytophthora sojae via association analysis in soybean [Glycine max (L.) Merr]. Mol. Genet. Genomics 291, 1095–1103. doi: 10.1007/s00438-015-1164-x
Liao, W., Ji, L., Wang, J., Chen, Z., Ye, M., Ma, H., et al. (2014). Identification of glutathione S-transferase genes responding to pathogen infestation in Populus tomentosa. Funct. Integr. Genomics 14, 517–529. doi: 10.1007/s10142-014-0379-y
Lipka, A. E., Tian, F., Wang, Q., Peiffer, J., Li, M., Bradbury, P. J., et al. (2012). GAPIT: genome association and prediction integrated tool. Bioinformatics 28, 2397–2399. doi: 10.1093/bioinformatics/bts444
Mamidi, S., Lee, R. K., Goos, J. R., and McClean, P. E. (2014). Genome-wide association studies identifies seven major regions responsible for iron deficiency chlorosis in soybean (Glycine max). PLoS ONE 9:e107469. doi: 10.1371/journal.pone.0107469
Marivet, J., Margis-Pinheiro, M., Frendo, P., and Burkard, G. (1994). Bean cyclophilin gene expression during plant development and stress conditions. Plant Mol. Biol. 26, 1181–1189. doi: 10.1007/BF00040698
Mengistu, A., Arelli, P., Bond, J., Nelson, R., Rupe, J., Shannon, G., et al. (2013). Identification of soybean accessions resistant to Macrophomina phaseolina by field screening and laboratory validation. Plant Health Progr. doi: 10.1094/PHP-2013-0318-01-RS. Available online at: http://www.plantmanagementnetwork.org/php/2013.asp
Mengistu, A., Ray, J. D., Smith, J. R., and Paris, R. L. (2007). Charcoal rot disease assessment of soybean genotypes using a colony-forming unit index. Crop Sci. 47:2453. doi: 10.2135/cropsci2007.04.0186
Mengistu, A., Wrather, A., and Rupe, J. C. (2015). “Charcoal rot,” in Compendium of Soybean Diseases and Pests, eds G. L. Hartman, J. C. Rupe, E. F. Sikora, L. L. Domier, J. A. Davis, and K. L. Steffey (St. Paul, MN: American Phytopathological Society), 67–69.
Oh, S. K., Baek, K. H., Seong, E. S., Joung, Y. H., Choi, G. J., Park, J. M., et al. (2010). CaMsrB2, pepper methionine sulfoxide reductase B2, is a novel defense regulator against oxidative stress and pathogen attack. Plant Physiol. 154, 245–261. doi: 10.1104/pp.110.162339
Paris, R. L., Mengistu, A., Tyler, J. M., and Smith, J. R. (2006). Registration of soybean germplasm line DT97–4290 with moderate resistance to charcoal rot. Crop Sci. 46:2324. doi: 10.2135/cropsci2005.09.0297
Pawlowski, M. L., Hill, C. B., and Hartman, G. L. (2015). Resistance to charcoal rot identified in ancestral soybean germplasm. Crop Sci. 55:1230. doi: 10.2135/cropsci2014.10.0687
Pearson, C., Schwenk, F., Crowe, F., and Kelley, K. (1984). Colonization of soybean roots by Macrophomina phaseolina. Plant Dis. 68, 1086–1088. doi: 10.1094/PD-69-1086
Radwan, O., Rouhana, L. V., Hartman, G. L., and Korban, S. S. (2013). Genetic mechanisms of host–pathogen interactions for charcoal rot in soybean. Plant Mol. Biol. Rep. 32, 617–629. doi: 10.1007/s11105-013-0686-9
Remington, D. L., Thornsberry, J. M., Matsuoka, Y., Wilson, L. M., Whitt, S. R., Doebley, J., et al. (2001). Structure of linkage disequilibrium and phenotypic associations in the maize genome. Proc. Natl. Acad. Sci. U.S.A. 98, 11479–11484. doi: 10.1073/pnas.201394398
Romeis, T., Piedras, P., and Jones, J. D. G. (2000). Resistance gene-dependent activation of a calcium-dependent protein kinase in the plant defense response. Plant Cell 12, 803–815. doi: 10.1105/tpc.12.5.803
Romero Luna, M. P., Mueller, D., Mengistu, A., Singh, A. K., Hartman, G. L., and Wise, K. A. (2017). Advancing our understanding of charcoal rot in soybeans. J. Integr. Pest Manage. 8, 1–8. doi: 10.1093/jipm/pmw020
Sarr, M., Ndiaye, M., Groenewald, J., and Crous, P. (2014). Genetic diversity in Macrophomina phaseolina, the causal agent of charcoal rot. Phytopathol. Mediterr. 53, 250–268. doi: 10.14601/Phytopathol_Mediterr-13736
Schmidt, M. E., Myers, O., and Gibson, P. T. (1993). Registration of pharaoh soybean. Crop Sci. 33, 210–211. doi: 10.2135/cropsci1993.0011183X003300010054x
Shitan, N. (2016). Secondary metabolites in plants: transport and self-tolerance mechanisms. Biosci. Biotechnol. Biochem. 80, 1283–1293. doi: 10.1080/09168451.2016.1151344
Smith, G., and Carvil, O. (1997). Field screening of commercial and experimental soybean cultivars for their reaction to Macrophomina phaseolina. Plant Dis. 81, 363–368. doi: 10.1094/PDIS.1997.81.4.363
Smith, G., and Wyllie, T. (1999). “Charcoal rot,” in Compendium of Soybean Disease, 4th Edn, ed St. Paul (Minnesota, MN: American Phytopathological Society), 29–31.
Sonah, H., O'donoughue, L., Cober, E., Rajcan, I., and Belzile, F. (2015). Identification of loci governing eight agronomic traits using a GBS-GWAS approach and validation by QTL mapping in soya bean. Plant Biotechnol. J. 13, 211–221. doi: 10.1111/pbi.12249
Song, Q., Hyten, D. L., Jia, G., Quigley, C. V., Fickus, E. W., Nelson, R. L., et al. (2013). Development and evaluation of SoySNP50K, a high-density genotyping array for soybean. PLoS ONE 8:e54985. doi: 10.1371/journal.pone.0054985
St Clair, D. A. (2010). Quantitative disease resistance and quantitative resistance loci in breeding. Ann. Rev. Phytopathol. 48, 247–268. doi: 10.1146/annurev-phyto-080508-081904
Sun, J., Li, L., Zhao, J., Huang, J., Yan, Q., Xing, H., et al. (2014). Genetic analysis and fine mapping of RpsJS, a novel resistance gene to Phytophthora sojae in soybean [Glycine max (L.) Merr]. Theor. Appl. Genet. 127, 913–919. doi: 10.1007/s00122-014-2266-2
Surrette, S. B., Meints, P., and Trevathan, L. (2006). Evaluation of two methods to infect soybean with Macrophomina phaseolina (Deuteromycota) under controlled environmental conditions. J. Miss Acad. Sci. 51, 134–139. Available online at: http://msacad.org/journal/april06journal/mas06a.pdf#page=16
Tang, Y., Qin, S., Guo, Y., Chen, Y., Wu, P., Chen, Y., et al. (2016). Genome-wide analysis of the AP2/ERF gene family in physic nut and overexpression of the JcERF011 gene in rice increased its sensitivity to salinity stress. PLoS ONE 11:e0150879. doi: 10.1371/journal.pone.0150879
Twizeyimana, M., Hill, C. B., Pawlowski, M., Paul, C., and Hartman, G. L. (2012). A cut-stem inoculation technique to evaluate soybean for resistance toMacrophomina phaseolina. Plant Dis. 96, 1210–1215. doi: 10.1094/PDIS-02-12-0126-RE
Vuong, T. D., Sonah, H., Meinhardt, C. G., Deshmukh, R., Kadam, S., Nelson, R. L., et al. (2015). Genetic architecture of cyst nematode resistance revealed by genome-wide association study in soybean. BMC Genomics 16:593. doi: 10.1186/s12864-015-1811-y
Wan, J., Vuong, T., Jiao, Y., Joshi, T., Zhang, H., Xu, D., et al. (2015). Whole-genome gene expression profiling revealed genes and pathways potentially involved in regulating interactions of soybean with cyst nematode (Heterodera glycines Ichinohe). BMC Genomics 16:148. doi: 10.1186/s12864-015-1316-8
Wan, J., Zhang, X. C., Neece, D., Ramonell, K. M., Clough, S., Kim, S. Y., et al. (2008). A LysM receptor-like kinase plays a critical role in chitin signaling and fungal resistance in Arabidopsis. Plant Cell 20, 471–481. doi: 10.1105/tpc.107.056754
Wang, Y., Wang, H., Ma, Y., Du, H., Yang, Q., and Yu, D. (2015). Identification of transcriptional regulatory nodes in soybean defense networks using transient co-transactivation assays. Front. Plant Sci. 6:915. doi: 10.3389/fpls.2015.00915
Wen, Z., Tan, R., Yuan, J., Bales, C., Du, W., Zhang, S., et al. (2014). Genome-wide association mapping of quantitative resistance to sudden death syndrome in soybean. BMC Genomics 15:11. doi: 10.1186/1471-2164-15-809
Willmann, R., Lajunen, H. M., Erbs, G., Newman, M.-A., Kolb, D., Tsuda, K., et al. (2011). Arabidopsis lysin-motif proteins LYM1 LYM3 CERK1 mediate bacterial peptidoglycan sensing and immunity to bacterial infection. Proc. Natl. Acad. Sci.U.S.A. 108, 19824–19829. doi: 10.1073/pnas.1112862108
Wimmer, V., Albrecht, T., Auinger, H. J., and Schon, C. C. (2012). Synbreed: a framework for the analysis of genomic prediction data using R. Bioinformatics 28, 2086–2087. doi: 10.1093/bioinformatics/bts335
Wrather, A., Shannon, G., Balardin, R., Carregal, L., Escobar, R., Gupta, G. K., et al. (2010). Effect of diseases on soybean yield in the top eight producing countries in 2006. Plant Health Prog. doi: 10.1094/PHP-2010-0125-01-RS. Available online at: http://www.plantmanagementnetwork.org/php/2010.asp
Wyllie, T. D. (1988). “Charcoal rot of soybean: Current status,” in Soybean Diseases of the North Central Region, eds T. D. Wyllie and D. H. Scott. (St. Paul, MN: American Phytopathological Society), 106–113.
Yang, X. B., and Navi, S. S. (2005). First report of charcoal rot epidemics caused by Macrophomina phaseolina in soybean in Iowa. Plant Dis. 89, 526–526. doi: 10.1094/PD-89-0526B
Yoshitomi, K., Taniguchi, S., Tanaka, K., Uji, Y., Akimitsu, K., and Gomi, K. (2016). Rice terpene synthase 24 (OsTPS24) encodes a jasmonate-responsive monoterpene synthase that produces an antibacterial gamma-terpinene against rice pathogen. J. Plant Physiol. 191, 120–126. doi: 10.1016/j.jplph.2015.12.008
Zhang, F., Du, Z., Huang, L., Cruz, C. V., Zhou, Y., and Li, Z. (2013). Comparative transcriptome profiling reveals different expression patterns in Xanthomonas oryzae pv. oryzae strains with putative virulence-relevant genes. PLoS ONE 8:e64267. doi: 10.1371/journal.pone.0064267
Zhang, J. P., Naik, H. S., Assefa, T., Sarkar, S., Reddy, R. V. C., Singh, A., et al. (2017). Computer vision and machine learning for robust phenotyping in genome-wide studies. Sci. Rep. 7:44048. doi: 10.1038/srep44048
Zhang, J., Singh, A., Mueller, D. S., and Singh, A. K. (2015a). Genome-wide association and epistasis studies unravel the genetic architecture of sudden death syndrome resistance in soybean. Plant J. 84, 1124–1136. doi: 10.1111/tpj.13069
Zhang, J., Song, Q., Cregan, P. B., Nelson, R. L., Wang, X., Wu, J., et al. (2015b). Genome-wide association study for flowering time, maturity dates and plant height in early maturing soybean (Glycine max) germplasm. BMC Genomics 16:217. doi: 10.1186/s12864-015-1441-4
Zhang, Z., Ersoz, E., Lai, C. Q., Todhunter, R. J., Tiwari, H. K., Gore, M. A., et al. (2010). Mixed linear model approach adapted for genome-wide association studies. Nat. Genet. 42, 355–360. doi: 10.1038/ng.546
Zhao, X., Han, Y., Li, Y., Liu, D., Sun, M., Zhao, Y., et al. (2015). Loci and candidate gene identification for resistance to Sclerotinia sclerotiorum in soybean (Glycine max L. Merr.) via association and linkage maps. Plant J. 82, 245–255. doi: 10.1111/tpj.12810
Keywords: GWAS, Macrophomina phaseolina, soybean, breeding, resistance, charcoal rot
Citation: Coser SM, Chowda Reddy RV, Zhang J, Mueller DS, Mengistu A, Wise KA, Allen TW, Singh A and Singh AK (2017) Genetic Architecture of Charcoal Rot (Macrophomina phaseolina) Resistance in Soybean Revealed Using a Diverse Panel. Front. Plant Sci. 8:1626. doi: 10.3389/fpls.2017.01626
Received: 02 May 2017; Accepted: 05 September 2017;
Published: 21 September 2017.
Edited by:
Laurent Gentzbittel, National Polytechnic Institute of Toulouse, FranceReviewed by:
Harsh Raman, NSW Department of Primary Industries, AustraliaValeria Terzi, Consiglio per la Ricerca in Agricoltura e l'analisi dell'economia Agraria, Italy
Chris Little, Kansas State University, United States
Copyright © 2017 Coser, Chowda Reddy, Zhang, Mueller, Mengistu, Wise, Allen, Singh and Singh. This is an open-access article distributed under the terms of the Creative Commons Attribution License (CC BY). The use, distribution or reproduction in other forums is permitted, provided the original author(s) or licensor are credited and that the original publication in this journal is cited, in accordance with accepted academic practice. No use, distribution or reproduction is permitted which does not comply with these terms.
*Correspondence: Asheesh K. Singh, singhak@iastate.edu
Arti Singh, arti@iastate.edu
†Present Address: Sara M. Coser, Monsanto Company, São José dos Campos, Brazil