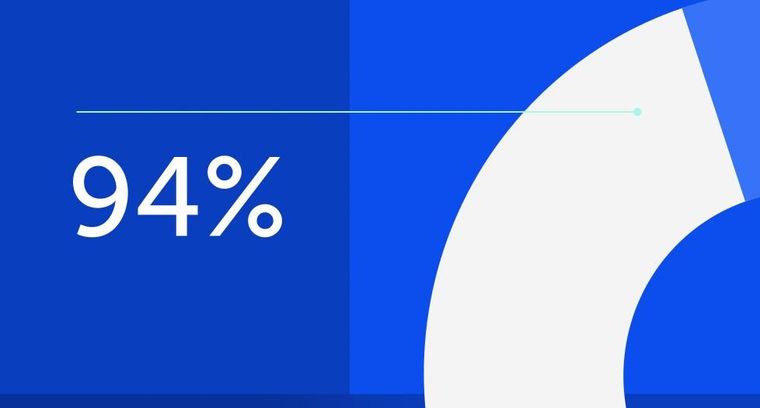
94% of researchers rate our articles as excellent or good
Learn more about the work of our research integrity team to safeguard the quality of each article we publish.
Find out more
ORIGINAL RESEARCH article
Front. Plant Sci., 29 August 2017
Sec. Plant Genetics and Genomics
Volume 8 - 2017 | https://doi.org/10.3389/fpls.2017.01488
The rapidly falling costs and the increasing availability of large DNA sequence data sets facilitate the fast and affordable mining of large molecular markers data sets for comprehensive evolutionary studies. The Brassicaceae (mustards) are an important species-rich family in the plant kingdom with taxa distributed worldwide and a complex evolutionary history. We performed Simple Sequence Repeats (SSRs) mining using de novo assembled transcriptomes from 19 species across the Brassicaceae in order to study SSR evolution and provide comprehensive sets of molecular markers for genetic studies within the family. Moreover, we selected the genus Cochlearia to test the transferability and polymorphism of these markers among species. Additionally, we annotated Cochlearia pyrenaica transcriptome in order to identify the position of each of the mined SSRs. While we introduce a new set of tools that will further enable evolutionary studies across the Brassicaceae, we also discuss some broader aspects of SSR evolution. Overall, we developed 2012 ready-to-use SSR markers with their respective primers in 19 Brassicaceae species and a high quality annotated transcriptome for C. pyrenaica. As indicated by our transferability test with the genus Cochlearia these SSRs are transferable to species within the genus increasing exponentially the number of targeted species. Also, our polymorphism results showed substantial levels of variability for these markers. Finally, despite its complex evolutionary history, SSR evolution across the Brassicaceae family is highly conserved and we found no deviation from patterns reported in other Angiosperms.
The field of evolutionary biology has never seen such an advance as the one promoted by the recent advent and standardization of next-generation sequencing (NGS) techniques. Now, many unsolved questions can be addressed (Nadeau and Jiggins, 2010). Despite that NGS technologies have drastically decreased their costs since their start, it stills remains cost prohibitive for many research programs. Fortunately, as NGS generates enormous amounts of data its analysis can be approached from multiple perspectives targeting different objectives. It is in this context where sharing data proves to be enormously beneficial to the scientific community. Open resources as those provided by the National Center for Biotechnology Information (NCBI Resource Coordinators, 2016), UniProt (The UniProt Consortium, 2007), or TAIR (Huala et al., 2001) are incredibly valuable to the broader research community. Likewise, countless initiatives and researchers are willing to release their data upon request. The sharing of research data yields multiple benefits; it promotes multiple perspectives, helps identify errors, discourages fraud and increases the efficient use of funding resources by avoiding duplicate data collection (Piwowar et al., 2007).
A popular use of NGS data is the discovery of large numbers of molecular markers for population genetic studies (Pashley, 2006; Wöhrmann et al., 2012; Lopez et al., 2015; Tanwar et al., 2017). The accurate assessment of genetic variation within and across species is pivotal in studies addressing topics like evolution, phylogeny and/or conservation and the larger the marker data set used, the more accurate the results and extrapolations are. Among the plethora of available molecular markers for genetic studies Simple Sequence Repeats (SSR) are extremely popular for several reasons. SSRs are known to display high levels of polymorphism, have a multiallelic behavior, are codominant and abundant along the genome (Morgante and Olivieri, 1992; Ritland, 2000); but, their development is still time consuming and relatively expensive (Squirrell et al., 2003). The alternative use of large genomic and/or transcriptomic datasets speeds and cheapens their design process (Lopez et al., 2015). Moreover, classical SSRs are mainly species-specific and markers developed for a taxon are unlikely exchangeable to another (Barbara et al., 2007) while SSRs from the transcribed portion of the genome are highly transferable between related species, and often even genera (Varshney et al., 2005b; Pashley, 2006; Huang et al., 2014) conferring further advantages in comparisons across related taxa. Ultimately, these SSRs can be considered “functional” markers as they represent a portion of the genome expressed under certain circumstances and a putative function can be associated to the sequences containing SSRs by homology search (Andersen and Lübberstedt, 2003). However, this type of marker may also have potential drawbacks. SSRs developed from the transcribed fraction of the genome might display lower levels of variability compared with classical SSRs (Ellis and Burke, 2007) and they might deviate from neutrality impacting the population structure inferences (Varshney et al., 2005a). However, recent studies pointed out that these markers have levels of polymorphism similar to their classical counterparts and, because only a small fraction of the genome might be subjected to recent positive selection, population structure measurements are comparable to those derived from anonymous SSRs (Tiffin and Hahn, 2002; Woodhead et al., 2005). To date, SSRs derived from the transcribed region of the genome have proved their usefulness in phylogenetic studies (Tabbasam et al., 2013), in comparative genetic mapping studies between species (Yu et al., 2004) and in studies assessing levels of genetic diversity (Wöhrmann and Weising, 2011; Olango et al., 2015).
Understanding how evolutionary forces shaped the current patterns of biodiversity worldwide is a challenging but exciting research focus. In the plant kingdom, the Brassicaceae are a fascinating model system for evolutionary studies for many reasons. First, it is one of the most diverse and geographically widespread plant families (Koch and Kiefer, 2006; Lysak and Koch, 2011; Al-Shehbaz, 2012). It compromises ~3,990 species in 52 tribes and more than 325 genera mostly distributed in temperate regions of the world, with scarce representatives in subtropical regions (Koch et al., 2012; Kiefer et al., 2014). Second, it includes many key species because of their economical and/or research value. The first plant with its whole genome sequenced, Arabidopsis thaliana, is a well-known representative of the Brassicaceae and numerous advances of the current knowledge of plant biology have been made from its studies (Meinke et al., 1998; Hohmann et al., 2014; Novikova et al., 2016). Likewise, this family includes many important agricultural and horticultural members like species from the genera Brassica, Camelina, and Raphanus. Third, the Brassicaceae net diversification rates are among the highest inferred for terrestrial plants (Jordon-Thaden et al., 2013; Karl and Koch, 2013; Hohmann et al., 2015). Moreover, recurrent polyploidization has played a significant role in the Brassicaceae evolution with circa half of its taxa hypothesized to have a recent polyploid origin (Franzke et al., 2011; Lysak and Koch, 2011; Hohmann et al., 2015) and the entire family having undergone a unique polyploid event not shared with other Brassicales families (Barker et al., 2009; Edger et al., 2015). Finally, initiatives such as, the BrassiBase knowledge database facilitates and promotes studies targeting the Brassicaceae family by providing publicly available resources (Kiefer et al., 2014). Despite these major advances and resources, multiple aspects of the evolutionary trajectory and phylogeny of this family still remain controversial or unresolved. In this regard, the lack of a sizable data-set of transferable and reliable molecular markers to accurately infer levels of genetic variation and divergence across species has limited the use of the Brassicaceae as an evolutionary model family.
The present study aims to fill this gap by developing a vast number of SSRs with their primers from RNAseq data of 19 Brassicaceae species covering all major evolutionary lineages within the family and an outgroup species from the sister family (Cleome violacea; Cleomaceae). These markers are publicly available in the BrassiBase portal (Kiefer et al., 2014). Our RNAseq dataset results from the fruitful collaboration and sharing of scientific data between researchers and initiatives; data originally used in (Huang et al., 2016) and data provided by the 1,000 plants initiative (1KP; Johnson et al., 2012). RNAseq data of each species was de novo assembled and the resulting transcriptomes went through rigorous quality checks before being mined for SSRs. We tested SSRs polymorphism and transferability in silico across eight Cochlearia species. Also, we annotated the de novo assembled transcriptome for Cochlearia so the sequences containing SSRs could be identified as coding or non-coding and a putative function could be assigned to those SSRs located in coding regions. Finally, we checked for evolutionary patterns of SSR types and motifs across the entire Brassicaceae phylogeny.
This study uses a set of 19 species (Table 1) covering all major lineages of the Brassicaceae phylogeny (Figure 1). Our data set was kindly provided through two collaborations. Ten out of the nineteen species RNAseq data were provided by Huang et al. (2016). For these RNA extraction, library preparation and sequencing were performed as specified in Huang et al. (2016). The renaming nine species were facilitated by the 1KP initiative. Protocol for RNA extraction, library preparation and sequencing details are described in Johnson et al. (2012).
Figure 1. Brassicaceae lineages included in the present study. Phylogeny adapted from Franzke et al. (2011). Purple stars marks tribes with samples included in this study. Genome size (1 Cx-value) indicates mean tribal value (whole bar = 4.33pg). Percentage of polyploids indicates neopolyploids (auto- and allopolyploids; Hohmann et al., 2014).
Prior to assembly, we filtered and trimmed the raw paired-end reads using Trimmomatic v0.36 (Bolger et al., 2014) based on the following criteria. We checked for remaining adapter sequences in the reads. In Trimmomatic we looked for seed matches allowing a maximum of two mismatches and these seeds were extended and clipped when paired end reads had a score of 30. Moreover, we removed leading and trailing bases of low quality (<20) or Ns. Likewise, we cut and removed the 3′ end if the quality of a 4-base wide sliding window dropped below 15. Finally, we discarded reads that after these steps were shorter that 50 bases long. By implementing this stringent filtering process, we ensured that only high-quality paired-end clean reads were used for the assembly. Finally, we further inspected the clean reads using FastQC v 0.11.5 (http://www.bioinformatics.babraham.ac.uk/projects/) to confirm that they met our quality standards.
To recover full-length transcripts we fed the clean reads to the software Trinity for their de novo assembly (Grabherr et al., 2011). In this regard, Trinity is and efficient and robust method for the de novo reconstruction of transcriptomes from short read sequences using the de Bruijn graph algorithm (Zhao et al., 2011; Honaas et al., 2016; Rana et al., 2016). We conducted all de novo assemblies using the default parameters and we assessed the quality of the de novo assembled transcriptome as it has a large impact on the accuracy of the subsequent analyses. First, we examined the read representation of the assembly by mapping the raw paired-end reads back to the assembly contigs using the bowtie aligner (Langmead et al., 2009). Second, we evaluated the completeness of the assembly in terms of conserved ortholog content with BUSCO (Simão et al., 2015).
For SSRs detection we used QDD v.3.1.2 (Meglecz et al., 2010; Meglécz et al., 2014). We inputted the assembled contigs to QDD3 and screened them for SSRs. Our search was restricted to perfect SSRs with a minimum length of 20 bases. Which, in terms of repeats translates into 10 repeats for di-, seven for tri-, five for tetra- and four for penta- and hexa-nucleotides, respectively. We excluded mononucleotides from the mining criteria because interpreting their polymorphism is challenging. Besides, in order to have enough flanking sequence for primer design, during SSR searches we only took into account contigs larger than 100 bases. For primers' design we used the version of Primer3 embedded in QDD1 (Rozen and Skaletsky, 2000). Our primer parameters were as follows: length of primers ranging from 18 to 23 nucleotides (optimum 20 bases), annealing temperature 55–65°C (optimum 60°C), GC content 30–70% (optimum 50%), and PCR product size from 90 to 320 bases. Also, during the primer search we specified that no other target SSR was allowed in the flanking region. Finally, we only selected those SSRs where their PCR product presented no overlapping with other SSRs sequences.
Because the data was sequenced on two different versions of the Illumina HiSeq we ran an exploratory analysis aiming to identify the impact that the sequencing platform might have over the results output. We evaluated the role of data source in raw reads, number of assembled contigs and number of mined SSRs. Once we identified the influence of the data source we proceeded to investigate the occurrence and frequency of SSR motifs. For this purpose, we imported the QDD output files into RStudio and using a combination of sorting and counting functions we summarized: repeat types, number of repeats, and frequency for each species. Besides, we also sorted out these categories based on phylogenetic lineages trying to look for evolutionary patterns. We chose tabular and graphical representations for results display.
Using Blast2GO (Conesa et al., 2005), a comprehensive software designed for the functional annotation and analysis of gene and protein sequences, we annotated the assembled contigs. We compared all transcripts with various databases aiming to extract the maximum possible information based on sequence and functional similarity. We used the BLASTX algorithm to search for homologous sequences (e-value cut off 1.0E-5) against the UniProtKB (both SwissProt and TrEMBL) and NCBI non-redundant databases and we functionally annotated the transcripts according to the Gene Ontology nomenclature (Gene Ontology, the Gene Ontology Consortium, 2000; http://www.geneontology.org). Moreover, we identified conserved motifs/domains through InterProScan. In order to obtain a comprehensive integrated annotation result, we also retrieved GO terms from the InterProScan ID's and merged them with our blast derived GO annotations. To maximize the information included on the final transcriptome annotation we also conducted a search in the Rfam database and performed an enzyme code and KEGG pathway annotation. First, the Rfam database contains information of non-coding RNAs (Griffiths-Jones, 2003; Nawrocki et al., 2015) and incorporating this information provides a more comprehensive annotation. Second, the Kyoto Encyclopedia of Genes and Genomes (KEGG) database is used extensively to reveal molecular interaction network and metabolic pathways (Kanehisa and Goto, 1999). In the last step, we performed a GO_Slim reduction on GO terms aiming to obtain more precise GO definitions. In all the steps involving Blast2GO we kept the default parameters: InterProScan analysis, Rfam search, enzyme code/KEGG annotation, and GO_Slim. Finally, we identified putative coding regions (Open Reading Frame) in the transcripts using Transdecoder (http://transdecoder.sf.net). The latter uses the information of the BLASTX in order to obtain a more accurate prediction of the ORFs.
We crossed the SSRs mined from the Cochlearia pyrenaica transcriptome with its annotation to identify their putative position within the transcriptome (i.e., coding and non-coding regions). We stablished that a SSR was within coding region when all its bases were within an ORF. Likewise, we considered a SSR as non-coding when it was fully positioned outside the ORF. To perform the in silico transferability and polymorphism analysis we selected 15 SSR which we tested in a total of 14 individuals encompassing eight Cochlearia species (Table 2). We mapped previously filtered high quality genomic reads to the transcripts containing SSRs. For the mapping we used the BWA-MEM algorithm in in BWA v0.7.5a (Li and Durbin, 2010) with default parameters except for the unpaired read pair penalty which was set to 15. Moreover, we removed ambiguously mapped and duplicate reads using samtools v.0.1.18 (Li et al., 2009; Li, 2011) and performed a local realignment around indels with the GATK (McKenna et al., 2010) “IndelRealigner” tool with default settings. We loaded the mappings in Geneious R9 (http://www.geneious.com; Kearse et al., 2012) where the SSRs polymorphism was manually assessed at intra- and inter-species level. Finally, we computed within and across species diversity indexes, as well as a Principal Component Analysis (PCoA) based on standardized genetic distanced between individuals with GenAlex v6.503 (Peakall and Smouse, 2006, 2012).
In this study, we used 19 species' libraries sequenced with Illumina technology. All libraries had the same sequencing depth except for Cochelaria pyrenaica, which was sequenced using two lanes in order to obtain higher coverage of the transcriptome for its annotation. Therefore, the number of reads for C. pyrenaica greatly exceeded those of the other libraries (110,411,546 total reads). For the remaining 18 species, the number of raw reads differed across libraries, ranging from 9,196,502 reads to 26,930,913 paired-reads for C. violacea and Clausia aprica, respectively (Table 3). We obtained high quality clean reads after applying a conservative trimming filter which removed adapter sequences, short reads and low quality reads from raw sequence data. The number of high quality clean sequence varied with a minimum of 8,915,641 reads to a maximum of 25,034,919 reads belonging to Draba ossetica and C. aprica, respectively (Table 3). We carried out the subsequent de novo assembly for each data using the clean reads. As expected, the number of assembled transcripts differed across species. The assembly of Microthlaspi perfoliatum rendered the smallest number of transcripts (35,086) while Macropodium nivale produced the largest assembly in terms of total contigs (97,523). Even if the starting number of clean reads for the de novo assembly was much higher in C. pyrenaica, the number of transcripts that we obtained was not the highest suggesting that other factors, besides number of input reads, might play a role during the assembly including RNA quality (Johnson et al., 2012).
Before SSR search we examined the quality of the de novo assembled transcriptomes using two parameters: the representation of reads and the content of conserved orthologs. In a high-quality transcriptome, the majority of its clean reads are mapped back as proper pairs (~70–80%). Circa 80% of our transcriptomes met this requirement and only four species did not (Brassica nigra, Draba magellanica, Draba chilensis, and Sinapis alba; Table 3). Transcriptomes with highly repetitive content can lead to lower percentage of read pairs mapping uniquely. Thus, in order to determine if we had a low quality or highly repetitive transcriptome, we checked the level of sequence duplication in our samples. When compared with the others, we identified an excess of repetitive content in the clean reads in the four species with low proportions of properly mapped reads. We also noted that these species were sequenced with the old Illumina HiSeq platform and had overrepresented sequences. Moreover, this overrepresentation of sequences was extended to all samples sequenced with the old Illumina platform but none of these overrepresented sequences had a hit with adapters or other sequences involved in the library preparation and/or sequencing processes. When we considered the second quality measurement results, for two of those four species, B. nigra and S. alba, we found a large percentage or orthologs present in their transcriptomes further supporting the repetitive content hypothesis. For the other two, D. magellanica and D. chilensis, the percentage of orthologs we retrieved was lower, around 60%, indicating that these de novo assembled transcriptomes were incomplete. Likewise, two other species, Draba hispida and D. magellanica reported similar low levels of orthologs content. Despite these cases, the overall quality of our de novo assembled transcriptomes was very good. Our quality assessment points toward a bias in relevant aspects for SSRs discovery using RNAseq associated with the choice of sequencing platform.
We used all assembled transcripts as input for the SSR search. In total, we found 23,425 SSRs ranging from 2,524 SSRs in M. nivale to 415 in Draba aizoides (Table 3). The density of SSRs across the de novo assembled transcriptomes showed large variation across species. We found that SSRs were distributed more densely in Noccaea caerulescens and C. violaceae with 1/11.67 kb and 1/11.79 kb, respectively, while in Kernera saxatilis SSRs were more distanced with 1/35.28 kb (Table 3). Even if the total number of SSRs and their density varied greatly, we detected a consistent pattern in the proportion of each repeat type (i.e., dimers, trimmers, tetramers, pentamers, and hexamers) across species (Figure 2). Our analyses showed that dimmers and trimmers accounted for three quarters of the discovered SSRs (30.66 and 44.43%, respectively) while tetramers and pentamers were scarce (4.35% and 8.09%) and hexamers displayed an intermediate frequency (12.33%). As expected, the number of SSRs with primers (2012) dramatically decreased representing 8.6% of the total. C. pyrenaica retained the largest amount of SSRs (160) but, as noted before, this sample was sequenced deeper. Regarding the remaining samples, M. perfoliatum maintained 135 SSRs while D. ossetica had the smallest number, 61 (Table S1). The primer design parameters also impacted the proportions of the different repeat types. Although dimers and trimmers were still the most abundant accounting for 25.94 and 57.36%, respectively, the frequencies of the remaining SSR types changed. Tetramers increased their frequency to 13.19% while pentamers and hexamers decrease their frequency to 2.52 and 2.96% each (Table S1). The latter indicates that the primers search parameters introduced a bias. Hence, we conducted the analyses concerning SSRs evolution across lineages with the whole SSRs data set instead of with the one containing only those SSRs with primers. Interestingly, the average percentage of SSRs for which we successfully designed primers was higher for the samples sequenced with the old HiSeq platform (avg. 14.14 SSRs) than for the ones sequenced with Illumina HiSeq2000 (avg. 7.28 SSRs), even if the starting number of SSRs was higher in the latter.
Figure 2. SSR's types distribution across species. In the x-axis, each type of motif is indicated while the y-axis refers to the percentage of each type of motif in each species. Each species is denoted by a color as shown in the legend.
Regarding the distribution of motifs, in our analyses we only considered those which accounted for at least 5% of the total. We clustered the motifs based on their sequences (Lopez et al., 2015) and the most abundant groups were AG, AAG, and ATG (Figure 3). These three were present in all species and displayed high frequencies. Moreover, we also detected motifs from the AAC, AGG, ACC, and AT groups but in a subset of species and with lower frequency (Figure 3). Overall, AG was the most abundant dimer while AAG was the commonest trimer. We also looked for variations across the phylogenetic lineages in terms of frequency of the different repeats' and motifs' types. We found no differences suggesting the absence of evolutionary patterns specific to each lineage but rather pointing to a conserved and more general scheme that encompasses the whole Brassicaceae family.
Figure 3. SSR's motifs distribution across species. The x-axis indicates species and the y-axis abundance in percentage. Each motif is denoted by a color as shown in the legend. Bars represents those motifs with ≥5% abundance. These motifs represent, depending on the species, between 55 and 75% of the total motifs' frequency.
Since our data set is very heterogeneous, we tested the effect that the number of reads and genome size might have on the de novo assembly, as well as in the amount of mined SSRs and their frequency across the transcriptome. Because of the existing variation in terms of sequencing platform we also considered the effect that this interaction might have had on the aforementioned tests. The sample C. pyrenaica was excluded from these analyses because of its greater sequencing depth. We corroborated that the sequencing platform has a significant impact on the initial number of reads but not on the number of assembled contigs. The overall number of detected SSRs was dependent on the starting number of reads and therefore biased by the sequencing platform but not by the number of contigs. Namely, those species sequenced with the old Illumina HiSeq rendered a smaller number of SSRs. Likewise, the density of SSRs along the transcriptomes was significantly biased by the sequencing platform. Eventually, we also considered the role of DNA content measured as the 1C number. In this regard, DNA content showed marginal significance for the initial number of reads but not in any other aspect. These analyses further confirmed that sequencing platform exerts a significant impact on number and density of mined SSRs.
The de novo assembly of C. pyrenaica generated 51,572 transcripts belonging to 37,394 Trinity genes (Table 2). Based on the representation of reads and ortholog completeness, we established that our transcriptome had a high quality (83.91% properly mapped reads and 94.46% retrieved orthologs). After the homology search a large proportion of our transcripts (79.40%) had a significant hit to closely related species such as, Eutrema salsugineum, Camelina sativa, Brassica napus, A. thaliana, Arabidopsis lyrata, Brassica rapa, Arabis alpine, and Capsella rubella. The remaining 20.60% did not match any known sequence and could be novel transcripts, untranslated regions, non-coding RNA or short sequences not containing a protein domain. The Gene Ontology (GO) categorizations we recovered from the transcripts with a significant hit in the homology search were distributed in the three main GO terms in the following order: biological process (68,585), molecular function (46,819), and cellular component (43,132). In the biological process category, most of the transcripts were assigned to metabolic and cellular process. In the cellular function category, the most abundant classes were cell and cell part, while binding and catalytic activity were the classes with the highest number of assigned transcripts in the molecular function category. We successfully annotated 62% of the de novo assembled sequences. Of the unannotated portion (~38%), 7% had GO mappings, 10% had Blast hits and 21% had neither GO mapping nor Blasts hits. Within that 21%, we assigned 11 sequences with a significant hit for non-coding RNA in the Rfam database. Five sequences belonged to snoRNAs, two were labeled as plant_SRP and the remaining three were tRNA, SSU-rRNA and miRNA. Eventually, to identify active metabolic pathways, we assigned the transcripts with retrieved GO terms to KEGG pathways and enzyme commission (EC) numbers. We retrieved a total of 6 EC classes with transferases (3255) and hydrolases (2191) as the most abundant. We included the EC numbers in KEEG pathways identifying biosynthesis of antibiotics (697 sequences with 158 enzymes) and purine metabolism (512 sequences wit 47 enzymes) as the most represented pathways. Other well-characterized pathways were starch and sucrose metabolism (298 sequences with 31 enzymes), pyrimidine metabolism (236 sequences with 27) and amino sugar and nucleotide sugar metabolism (221 sequences with 35 enzymes). Finally, we added the ORF prediction to the annotation results with 24,487 complete ORFs, 3393 partial ORFs and 3911 internal ORFs. Overall, we provide a high quality and well annotated transcriptome which represents a valuable tool for future studies aiming to disentangle the evolutionary history of the plant genus Cochlearia.
We identified a total of 2738 SSRs in the species C. pyrenaica (Table 3) and from those 160 fulfilled our primer search criteria. Trimers were the most common type of SSR followed by dimers, both before and after primer search (Table S1). Motifs from the AG group were the most abundant (22.21%) followed by the group AAG (19.44%; Figure 3). We crossed the 160 SSRs with primers with the transcriptome's annotation showing that 74 were located within ORF and 86 outside (Table 4). When analyzed in detail, the SSRs were not equally distributed across the transcriptome. We found that trimers clearly dominated within ORFs (>85%) while dimers and tetramers contributed the most (>82%) outside ORFs.
Table 4. ORF location and type distribution of SSRs used for the in silico polymorphism test in Cochlearia species.
For the in silico polymorphism evaluation, we randomly selected 16 SSRs which were we tested in 13 individuals from seven species of the genus Cochlearia (Table 2). Our species data set included six individuals from C. pyrenaica, two individuals of C. groenlandica and one individual of each of the remaining five species. Regarding the SSRs, eight were located within ORFs (seven trimers and one hexamer) and eight outside ORFs (three dimers, two dimers and two tetramers; Table 5). We mapped genomic reads of the different Cochlearia species to the C. pyrenaica transcriptome and selected those mapped regions with the transcripts containing SSRs. In order to find the expected PCR product, we performed a search of the SSR for both primers, forward and reverse. By identifying the whole PCR product, we could also detect presence of introns or other elements that might modify the expected fragment length. In this regard, none of the 16 selected SSRs deviated from the expected length. Moreover, the primers' sequences were conserved in all species indicating that these SSRs are fully transferable across all tested species in the genus Cochlearia.
Once the PCR product was identified we scored the SSRs. Because of possible PCR inaccuracies, we only considered an allele as “true” if it was present in at least two reads. One sample, Cpyr_0699 had no reads mapped to two SSR-containing contigs and therefore those were coded as missing alleles (Table 6). As expected for diploid species, the maximum number of alleles we found per SSR was two (Table 6). Despite the reduced number of individuals, and with the only purpose to be used as guidance, we computed several diversity measurements. Overall, mean expected heterozygosity (He) was 0.210 (SE = 0.023) and the mean percentage of polymorphic loci was 40.63% (SE = 9.67%). In terms of species' diversity our only sample of C. sessilifolia was monomorphic for all scored SSRs and thus, the polymorphism at species level was zero. The highest percentage of polymorphic loci was 87.50% in C. pyrenaica followed by C. groenlandica (56.25%). These two species were the ones with more than one individual per species scored. In the case of C. pyrenaica we analyzed six individuals and its genetic diversity can be considered as realistic measurement (He = 0.512, SE = 0.068). For the remaining one-individual-per-species samples polymorphism ranged from 25.00 to 56.25%. Because of the reduced n per species these measurements cannot be considered an accurate representation of the species' diversity. SSRs in coding regions are expected to be more conserved thus, we tested if they displayed lower levels of polymorphism than those in non-coding regions. We re-run the diversity analysis for the SSRs within ORFs (coding) vs. those outside the ORFs (non-coding). In the first case of coding regions, the He recorded was 0.230 (SE = 0.033) and mean polymorphism of 45.31% (SE = 10.28%). Interestingly, in non-coding SSRs the total mean He was 0.189 (SE = 0.033) and mean polymorphism of 35.94% (SE = 10.96%). Finally, the first two axes of the PCoA calculated for the individuals based on their genetic distance explained 51.24% (coord.1 accounted for 32.27% and coord.2 18.97%). The six individuals of C. pyrenaica formed a dispersed cluster while the two individuals of C. groenlandica grouped tightly together (Figure 4). The PCoA also revealed a geographical sub-grouping where most individuals from Artic and Alpine areas clustered together (Alaska, Iceland, Norway, and alpine Austria). Our transferability and polymorphism analyses showed that this is a suitable approach for developing large sets of SSRs for population studies within and across related species.
Table 6. SSR polymorphism in species from the genus Cochlearia. Alleles scored in each sample per SSR (indicated by length in bp).
Figure 4. Standardized distance PCoA for Cochlearia samples. Each color represents a species from the genus Cochlearia and the country of origin is displayed next to the sample symbol. Both coordinates explain 51.24% of the variability (coord.1 accounted for 32.27% and coord.2 18.97%).
The Brassicaceae is a captivating model for evolutionary studies. This family is one of the biggest in the plant kingdom with taxa distributed worldwide including model species and economically relevant crops. Besides, it shows a complex history with high rates of speciation and recurrent polyploid events distributed across its phylogeny at both ancient and more recent time scales. Nevertheless, there is still more to learn from this family. In the present study, we developed a large amount of SSRs with their respective primers (2012) in 19 Brassicaceae species from their de novo assembled transcriptomes. Besides, we used eight species from the genus Cochlearia to test their transferability and polymorphism in silico. In this genus, we went one step further and also annotated its transcriptome. Overall, we provide a new set of tools for evolutionary studies in the family Brassicaceae and we discuss its implications.
During the design of an experiment, choices like the sampling strategy or the selection of a particular molecular tool can deeply impact the outcome. In studies using NGS technologies, the chosen sequencing platform could be one of these determinant factors. However, there is no clear consensus regarding how strongly its influence can be. In some cases, the sequencing platform resulted in little or no after-affect (Solonenko et al., 2013; Tremblay et al., 2015). In contrast, other studies demonstrated a significant bias in relevant aspects like the G = C content of the data among others (Benjamini and Speed, 2012; Salipante et al., 2014). Our results strongly support that the sequencing platform exerts a strong bias on the results of studies using NGS data for SSR mining in aspects like overall number of mined SSRs or SSR density suggesting caution when data from different sequencing platforms are combined.
The frequency and distribution of SSRs from the transcribed regions of the genome can vary greatly among studies as their discovery depends on aspects such as, mining criteria, sequencing platform, or the completeness of the inputted transcriptome (Aggarwal et al., 2006; Blair and Hurtado, 2013). In an effort to increase polymorphism and avoid fluctuations in the length of the PCR product not associated with the SSR per se, we choose conservative criteria and only perfect SSRs with at least 20 bp with no other SSRs in the flanking or primer regions were considered (Blair and Hurtado, 2013). Hence, if we had used more relaxed parameters for the searching, the amount of obtained SSRs could have been larger. Overall, we found 23,425 SSRs in 19 Brassicaceae species. Those 19 species covered all lineages of the Brassicaceae phylogeny projecting a comprehensive picture of the family. The overall number of SSRs and their density along the de novo assembled transcriptomes varied largely across species. These changes have been attributed in some cases to genome size (Qiu et al., 2010). However, in our case these changes seem to be caused by platform sequencing rather than genome size. In fact, when we considered only the data set generated by Illumina HiSeq2000 and looked for correlations between the 1C number with the number of SSRs or the SSRs density per species, no clear pattern emerged. Despite the fact that the sequencing platform influenced the overall amount of mined SRRs and their density, the general distribution of the different SSR types and motives remained stable. Trimers, followed by dimers, were the most abundant motives accounting for ~75% while tetra-, penta- and hexa-nucleotides had lower frequencies in all studied species. This pattern has been previously reported for numerous angiosperm taxa (Kantety et al., 2002; Morgante et al., 2002; Victoria et al., 2011). Likewise, the most common dimer motif was AG/CT while the rarest was the CG/GC as in similar studies with Arabidopsis, rice and several other angiosperms (Kantety et al., 2002; Morgante et al., 2002; Victoria et al., 2011; Lopez et al., 2015). The high amount of GA/CT motifs is likely to be related to high levels of the translated amino acid products of these motifs (Kantety et al., 2002; Qiu et al., 2010). Among the trimers, our results also fitted the expectations for angiosperms with AAG/CTT as the most abundant group followed by the ATG/CAT group and the rarest was AAT/TTA (Morgante et al., 2002; Victoria et al., 2011). Few other trinucleotide motives had a frequency of at least 5% but none of them was consistently found across all studied species as the two previous ones were. Finally, besides the motives found in each and all studied species, no motif was represented in a particular phylogenetic lineage.
Microsatellite distribution is known to be a function of the dynamics and history of genome evolution (Morgante et al., 2002). The dominance of trinucleotides responds to the coding nature of the transcriptome as selection and evolution benefit the presence of repeat types which maintain the coding frame (Wang et al., 1994; Gao et al., 2003). Yet, a large number of dinucleotides was also found suggesting that there is a considerable amount of non-coding regions like introns and UTR which might benefit from polymorphism affecting the regulation of transcription expression levels (Gingeras, 2007). Overall, this congruency with previous studies in angiosperms in the frequency pattern of the various SSR repeat types and motives points to the conserved nature of SSR evolution despite the complex and rich evolutionary history of the Brassicaceae family.
The final amount of available SSRs with their respective primers was significantly smaller compared with the total number of mined SSRs as expected from our strict mining criteria. Our data set comprehends 2012 SSRs with their respective primers ranging from 61 to 160 per species. After primer search the general SSR frequency pattern stayed fairly stable with mean percentage of trimers of 59.25 followed by dimers with 25.10% and the remaining repeat types displaying lower frequencies. However, the not-so-common repeat types experienced some changes. In this regard, tetramers went from a mean 5.11 to 12.61% while penta- and hexamers changed from 8.76 to 2.11% and 13.17 to 2.10%, respectively. Primer search, as mining criteria, have an enormous impact on the final size of the data set. Here we opted for a more conservative approach to ensure the stability, polymorphism and transferability of our SSRs instead of aiming for a larger number of mined SSRs which might lead to lower accuracy of the PCR products derived from our SSRs.
The genus Cochlearia is a non-model system in the Brassicaceae with no prior genome information. This lack of genomic resources hinders the study of its evolutionary history. Thus, besides the SSR discovery, we annotated the de novo assembled transcriptome of C. pyrenaica in an effort to provide more comprehensive resources for future studies. Circa 80% of the transcripts had a positive hit on the protein databases indicating that most of our transcripts coded proteins. The latter was probably facilitated by the annotated genomes from close relatives of the Brassicaceae family such as, A. thaliana or E. salsugineum. For those transcripts without any hit during the annotation process we ran a Rfam search. Only 11 transcripts had a match. Therefore, most of our unidentified transcripts are probably lacking conserved functions or might belong to uncharacterized genes. Overall, based on our quality measurements and the large proportion of annotated transcripts, we are confident of the accuracy and completeness of our de novo assembled and annotated C. pyrenaica transcriptome.
The GO terms association provides an important resource for the identification of gene roles (Ashburner et al., 2000). Overall, we assigned 159,836 GO terms and classified them into the three main categories (biological process, cellular component and molecular function). Within the biological process, several GO terms (e.g., cold adaptation and salt tolerance) are of special relevance for the evolutionary history of Cochlearia and open a new horizon for future studies. This genus is known to inhabit a wide range of environments (salt marshes, high alpine environments, and artic regions among others) but the mechanisms behind these adaptations which might have mediated the expansion of the genus are unknown (Koch et al., 1998, 1999; Koch, 2012). Besides, several pathways we detected that in the C. pyrenaica transcriptome are putatively associated with adaptation to these environments. Namely, starch and sucrose metabolism are known to play a role during cold acclimation (Beck et al., 2007; Peng et al., 2015). Likewise, the identification of enzyme codes in the present study is likely to be helpful for understanding the metabolic activities of the contrasting species in this interesting and emblematic genus.
We selected 14 individuals of eight diploid Cochlearia species to conduct a performance test addressing polymorphism and transferability of our SSRs. These species are very interesting because of their complex polyploid and reticulate evolutionary history which spans a few 100, 000 years only (Koch et al., 1998, 1999; Koch, 2002, 2012). Because of their association with conserved parts of the genome, SSRs derived from the transcribed region are often considered to display low levels of polymorphism (Ellis and Burke, 2007). However, numerous studies contrasting SSR from the anonymous and transcribed regions of the genome showed that this premise does not hold true as they show comparable levels of polymorphism (Tiffin and Hahn, 2002; Woodhead et al., 2005). Our within-species measurements cannot be considered an accurate reference as we only had more than one individual per species in C. groenlandica (n = 2) and C. pyrenaica (n = 6). Still, our data set involved a comprehensive group of the genus representatives making it suitable to test transferability and polymorphism across species. We considered a SSR transferable across species when both primers were found in the genomic reads. Based on this criterion all 16 SSRs were 100% transferable across species. Even if our search parameters allowed for two bases difference between the primer and targeted sequence, all primers had an identical match suggesting full transferability across species. We found no deviation from the expected inferred PCR product indicating absence of non-transcribed introns (Lopez et al., 2015). However, deviation cannot be completely disregarded for all mined SSRs as we tested only a small fraction.
The expected heterozygosity detected for C. pyrenaica falls within the expected for short-lived perennial plants (Nybom, 2004) supporting the adequacy of these type of markers in studies addressing genetic variation within species. When we considered all species, the expected inter-species heterozygosity decreased (He = 0.230, SE = 0.033). The latter could be explained by presence of several individuals with reduced polymorphism. Life history traits are known to have a strong impact on the species' genetic variation and population structure (Nybom, 2004) and our lower inter-species measurement can also be a consequence of contrasting features of the species included in our data set. The computation of FST was impeded by the low number of individuals per species. Thus, we performed a PCoA as an approximation of genetic structure. In the PCoA individuals from the same species appeared tightly clustered for C. groenlandica while for C. pyrenaica the cluster was more dispersed. Interestingly, the two individuals of arctic C. groenlandica were grouped with arctic C. sessilifolia from Alaska, arctic C. islandica from Iceland and alpine C. excelsa from Austria forming an arctic/alpine environmental deme. We also observed a geographical clustering in C. pyrenaica where individuals from central Europe and UK are closer together compared with individuals from Spain. Surprisingly the individual from Slovakia was closer to the Spanish accession that to the Central European ones. Another striking detail was the position of the Canadian sample which, due to environmental conditions, we expected to be grouped with the other Arctic/Alpine samples. Instead, it was positioned in the Central European deme. These two anomalies could be attributed to the limited number of samples and SSRs used for this test. Because of the latter we cannot make general assumptions but, based on our PCoA, it seems reasonable to say that this approach for SSRs development can also be regarded as an alternative to classical SSRs when inferring species' genetic structure.
Finally, the annotated transcriptome of C. pyrenaica allowed us to identify the location of the SSRs in coding and non-coding regions. Based on the premise that coding parts are conserved, we expected SSRs from these to be less polymorphic. Interestingly, we found that SSRs located within ORF displayed higher levels of diversity than their non-coding counterparts. Our data set comprehends multiple species distributed worldwide which inhabit contrasting environments and are likely to be adapted to their local environments. Thus, the higher diversity observed in the SSRs from coding regions may be attributed to adaptation and selection processes. Overall, we have established a significant genomic data resource for the genus Cochlearia providing a comprehensive annotated transcriptome and a large set of polymorphic molecular markers suitable for genetic studies which are transferable between species.
Our main goal in this study was to identify genic-markers that can be immediately available in evolutionary studies across the Brassicaceae family. Among various molecular markers we choose SSRs because they are extensively and successfully used for genetics and plant breeding applications (Hiremath et al., 2012). Overall, we identified 2012 SSR markers with their respective primers for 19 Brassicaceae species which are publicly available in the BrassiBase portal (Kiefer et al., 2014). As shown in our transferability test with the genus Cochlearia these transcriptome-based markers are fully transferable within the genus. Even more, several studies have found that transferability can go beyond the within-genus level (Varshney et al., 2005b) increasing exponentially the number of targeted species. Our polymorphism test supports previous studies indicating that these markers have similar variation levels as classical SSRs (Tiffin and Hahn, 2002; Woodhead et al., 2005). Also, we found that the SSRs within coding regions harbored larger variability than the non-coding ones suggesting that these markers might be suitable to detect functional adaptive variation across populations and/or species. Additionally, we delivered a high quality annotated transcriptome for C. pyrenaica which facilitates future evolutionary studies in this fascinating genus. Our results showed that sequencing platform has a significant impact on the SSRs discovery outcome and we recommend that when combining different data sets this bias should not be taken lightly. Finally, SSRs' evolutionary patterns across Brassicaceae lineages are highly conserved despite its complex evolutionary history and all our findings were in agreement with previous studies conducted in other Angiosperms.
The assembled transcripts used for the SSR mining and further analysis are available in Dryad (https://doi.org/10.5061/dryad.35k67). Likewise, the file containing the annotated transcriptome can be found at Dryad (https://doi.org/10.5061/dryad.35k67). Finally, the SSR with their respective primer and all accompanying information can be found at the Brassibase (XXX) and Dryad (https://doi.org/10.5061/dryad.35k67).
LL and MK designed the study and set up the analysis. LL processed and analyzed the data. EW, PE, and JP made relevant contributions to the data analysis process. LL drafted the manuscript and MK, EW, PE, and JP critically revised the manuscript. All authors read and approved the final version of the manuscript.
Research was supported by grants awarded to LLP from Deputación de A Coruña (Becas de Investigacion-2014) and Heidelberg University (COS) internship. The project was funded by Deutsche Forschungsgemeinschaft (DFG) to MK (KO2302-16/1).
The authors declare that the research was conducted in the absence of any commercial or financial relationships that could be construed as a potential conflict of interest.
We thank the 1KP initiative as well as the authors of Huang et al. (2016) for kindly providing access to their data.
The Supplementary Material for this article can be found online at: http://journal.frontiersin.org/article/10.3389/fpls.2017.01488/full#supplementary-material
Aggarwal, R. K., Hendre, P. S., Varshney, R. K., Bhat, P. R., Krishnakumar, V., and Singh, L. (2006). Identification, characterization and utilization of EST-derived genic microsatellite markers for genome analyses of coffee and related species. Theor. Appl. Genet. 114, 359–372. doi: 10.1007/s00122-006-0440-x
Al-Shehbaz, I. A. (2012). A generic and tribal synopsis of the Brassicaceae (Cruciferae). Taxon 61, 931–954. Available online at: www.jstor.org/stable/41679341
Andersen, J. R., and Lübberstedt, T. (2003). Functional markers in plants. Trends Plant Sci. 8, 554–560. doi: 10.1016/j.tplants.2003.09.010
Ashburner, M., Ball, C. A., Blake, J. A., Botstein, D., Butler, H., Cherry, J. M., et al. (2000). Gene ontology: tool for the unification of biology. Nat. Genet. 25, 25–29. doi: 10.1038/75556
Barbara, T., Palma Silva, C., Paggi, G. M., Bered, F., Fay, M. F., and Lexer, C. (2007). Cross-species transfer of nuclear microsatellite markers: potential and limitations. Mol. Ecol. 16, 3759–3767. doi: 10.1111/j.1365-294X.2007.03439.x
Barker, M. S., Vogel, H., and Schranz, M. E. (2009). Paleopolyploidy in the Brassicales: analyses of the cleome transcriptome elucidate the history of genome duplications in Arabidopsis and other brassicales. Genome Biol. Evol. 1, 391–399. doi: 10.1093/gbe/evp040
Beck, E. H., Fettig, S., Knane, C., Hartig, K., and Bhattarai, T. (2007). Specific and unspecific responses of plants to cold and drought stress. J. Biosci. 32, 501–510. doi: 10.1007/s12038-007-0049-5
Benjamini, Y., and Speed, T. P. (2012). Summarizing and correcting the GC content bias in high-throughput sequencing. Nucleic Acids Res. 40:e72. doi: 10.1093/nar/gks001
Blair, M. W., and Hurtado, N. (2013). EST-SSR markers from five sequenced cDNA libraries of common bean (Phaseolus vulgaris L.) comparing three bioinformatic algorithms. Mol. Ecol. Resour. 13, 688–695. doi: 10.1111/1755-0998.12099
Bolger, A. M., Lohse, M., and Usadel, B. (2014). Trimmomatic: a flexible trimmer for Illumina sequence data. Bioinformatics 30, 2114–2120. doi: 10.1093/bioinformatics/btu170
Conesa, A., Gotz, S., Garcia-Gomez, J. M., Terol, J., Talon, M., and Robles, M. (2005). Blast2GO: a universal tool for annotation, visualization and analysis in functional genomics research. Bioinformatics 21, 3674–3676. doi: 10.1093/bioinformatics/bti610
Edger, P. P., Heidel-Fischer, H. M., Bekaert, M. L., Rota, J., Glckner, G., Platts, A. E., et al. (2015). The butterfly plant arms-race escalated by gene and genome duplications. Proc. Natl. Acad. Sci. U.S.A. 112, 8362–8366. doi: 10.1073/pnas.1503926112
Ellis, J. R., and Burke, J. M. (2007). EST-SSRs as a resource for population genetic analyses. Heredity 99, 125–132. doi: 10.1038/sj.hdy.6801001
Franzke, A., Lysak, M. A., Al-Shehbaz, I. A., Koch, M. A., and Mummenhoff, K. (2011). Cabbage family affairs: the evolutionary history of Brassicaceae. Trends Plant Sci. 16, 108–116. doi: 10.1016/j.tplants.2010.11.005
Gao, L., Tang, J., Li, H., and Jia, J. (2003). Analysis of microsatellites in major crops assessed by computational and experimental approaches. Mol. Breed. 12, 245–261. doi: 10.1023/A:1026346121217
Gingeras, T. R. (2007). Origin of phenotypes: genes and transcripts. Genome Res. 17, 682–690. doi: 10.1101/gr.6525007
Grabherr, M. G., Haas, B. J., Yassour, M., Levin, J. Z., Thompson, D. A., Amit, I., et al. (2011). Full-length transcriptome assembly from RNA-seq data without a reference genome. Nat. Biotechnol. 29, 644–652. doi: 10.1038/nbt.1883
Griffiths-Jones, S. (2003). Rfam: an RNA family database. Nucleic Acids Res. 31, 439–441. doi: 10.1093/nar/gkg006
Hiremath, P. J., Kumar, A., Penmetsa, R. V., Farmer, A., Schlueter, J. A., Chamarthi, S. K., et al. (2012). Large-scale development of cost-effective SNP marker assays for diversity assessment and genetic mapping in chickpea and comparative mapping in legumes. Plant Biotechnol. J. 10, 716–732. doi: 10.1111/j.1467-7652.2012.00710.x
Hohmann, N., Schmickl, R., Chiang, T.-Y., Lučanová, M., Kolář, F., Marhold, K., et al. (2014). Taming the wild: resolving the gene pools of non-model Arabidopsis lineages. BMC Evol. Biol. 14:224. doi: 10.1186/s12862-014-0224-x
Hohmann, N., Wolf, E. M., Lysak, M. A., and Koch, M. A. (2015). A time-calibrated road map of brassicaceae species radiation and evolutionary history. Plant Cell 27, 2770–2784. doi: 10.1105/tpc.15.00482
Honaas, L. A., Wafula, E. K., Wickett, N. J., Der, J. P., Zhang, Y., Edger, P. P., et al. (2016). Selecting superior de novo transcriptome assemblies: lessons learned by leveraging the best plant genome. PLoS ONE 11:e0146062. doi: 10.1371/journal.pone.0146062
Huala, E., Dickerman, A. W., Garcia-Hernandez, M., Weems, D., Reiser, L., LaFond, F., et al. (2001). The Arabidopsis Information Resource (TAIR): a comprehensive database and web-based information retrieval, analysis, and visualization system for a model plant. Nucleic Acids Res. 29, 102–105. doi: 10.1093/nar/29.1.102
Huang, C.-H., Sun, R., Hu, Y., Zeng, L., Zhang, N., Cai, L., et al. (2016). Resolution of brassicaceae phylogeny using nuclear genes uncovers nested radiations and supports convergent morphological evolution. Mol. Biol. Evol. 33, 394–412. doi: 10.1093/molbev/msv226
Huang, D., Zhang, Y., Jin, M., Li, H., Song, Z., Wang, Y., et al. (2014). Characterization and high cross-species transferability of microsatellite markers from the floral transcriptome of Aspidistra saxicola (Asparagaceae). Mol. Ecol. Resour. 14, 569–577. doi: 10.1111/1755-0998.12197
Johnson, M. T. J., Carpenter, E. J., Tian, Z., Bruskiewich, R., Burris, J. N., Carrigan, C. T., et al. (2012). Evaluating methods for isolating total RNA and predicting the success of sequencing phylogenetically diverse plant transcriptomes. PLoS ONE 7:e50226. doi: 10.1371/journal.pone.0050226
Jordon-Thaden, I. E., Al-Shehbaz, I. A., and Koch, M. A. (2013). Species richness of the globally distributed, arctic–alpine genus Draba L. (Brassicaceae). Alp. Bot. 123, 97–106. doi: 10.1007/s00035-013-0120-9
Kanehisa, M., and Goto, S. (1999). KEGG: kyoto encyclopedia of genes and genomes. Nucleic Acids Res. 28, 27–30. doi: 10.1093/nar/28.1.27
Kantety, R. V., La Rota, M., Matthews, D. E., and Sorrells, M. E. (2002). Data mining for simple sequence repeats in expressed sequence tags from barley, maize, rice, sorghum and wheat. Plant Mol. Biol. 48, 501–510. doi: 10.1023/A:1014875206165
Karl, R., and Koch, M. A. (2013). A world-wide perspective on crucifer speciation and evolution: phylogenetics, biogeography and trait evolution in tribe Arabideae. Ann. Bot. 112, 983–1001. doi: 10.1093/aob/mct165
Kearse, M., Moir, R., Wilson, A., Stones-Havas, S., Cheung, M., Sturrock, S., et al. (2012). Geneious basic: an integrated and extendable desktop software platform for the organization and analysis of sequence data. Bioinformatics 28, 1647–1649. doi: 10.1093/bioinformatics/bts199
Kiefer, M., Schmickl, R., German, D. A., Mandakova, T., Lysak, M. A., Al-Shehbaz, I. A., et al. (2014). Brassibase: introduction to a novel knowledge database on brassicaceae evolution. Plant Cell Physiol. 55:e3. doi: 10.1093/pcp/pct158
Koch, M. A. (2002). Genetic differentiation and speciation in prealpine cochlearia: allohexaploid Cochlearia bavarica Vogt (Brassicaceae) compared to its diploid ancestor Cochlearia pyrenaica DC. in Germany and Austria. Plant Syst. Evol. 232, 35–49. doi: 10.1007/s006060200025
Koch, M. A. (2012). Mid-Miocene divergence of Ionopsidium and Cochlearia and its impact on the systematics and biogeography of the tribe Cochlearieae (Brassicaceae). Taxon 61, 76–92. Available online at: http://www.jstor.org/stable/23210316
Koch, M. A., Huthmann, M., and Hurka, H. (1998). Isozymes, speciation and evolution in the polyploid complex Cochlearia L. Bot. Acta 111, 411–425. doi: 10.1111/j.1438-8677.1998.tb00727.x
Koch, M. A., and Kiefer, C. (2006). Molecules and migration: biogeographical studies in cruciferous plants. Plant Syst. Evol. 259, 121–142. doi: 10.1007/s00606-006-0416-y
Koch, M. A., Kiefer, M., German, D., Ihsan, A.-S. A., Franzke, A., Mummenhoff, K., et al. (2012). BrassiBase: Tools and biological resources to study characters and traits in the Brassicaceae – version 1.1. Taxon 61, 1001–1009. Available online at: www.jstor.org/stable/41679346
Koch, M. A., Mummenhoff, K., and Hurka, H. (1999). Cochlearia (Brassicaceae) and allied genera based on nuclear ribosomal ITS DNA sequence analysis contradict traditional concepts of their evolutionary relationship. Plant Syst. Evol. 216, 207–230. doi: 10.1007/BF01084399
Langmead, B., Trapnell, C., Pop, M., and Salzberg, S. L. (2009). Ultrafast and memory-efficient alignment of short DNA sequences to the human genome. Genome Biol. 10:R25. doi: 10.1186/gb-2009-10-3-r25
Li, H. (2011). A statistical framework for SNP calling, mutation discovery, association mapping and population genetical parameter estimation from sequencing data. Bioinformatics 27, 2987–2993. doi: 10.1093/bioinformatics/btr509
Li, H., and Durbin, R. (2010). Fast and accurate long-read alignment with Burrows-Wheeler transform. Bioinformatics 26, 589–595. doi: 10.1093/bioinformatics/btp698
Li, H., Handsaker, B., Wysoker, A., Fennell, T., Ruan, J., Homer, N., et al. (2009). The Sequence Alignment/Map format and SAMtools. Bioinformatics 25, 2078–2079. doi: 10.1093/bioinformatics/btp352
Lopez, L., Barreiro, R., Fischer, M., and Koch, M. A. (2015). Mining microsatellite markers from public expressed sequence tags databases for the study of threatened plants. BMC Genomics 16:781. doi: 10.1186/s12864-015-2031-1
Lysak, M. A., and Koch, M. A. (2011). “Phylogeny, genome, and karyotype evolution of crucifers (Brassicaceae),” in Genetics and Genomics of the Brassicaceae, eds R. Schmidt, and I. Bancroft (New York, NY: Springer), 1–31.
McKenna, A., Hanna, M., Banks, E., Sivachenko, A., Cibulskis, K., Kernytsky, A., et al. (2010). The genome analysis toolkit: a mapreduce framework for analyzing next-generation DNA sequencing data. Genome Res. 20, 1297–1303. doi: 10.1101/gr.107524.110
Meglecz, E., Costedoat, C., Dubut, V., Gilles, A., Malausa, T., Pech, N., et al. (2010). QDD: a user-friendly program to select microsatellite markers and design primers from large sequencing projects. Bioinformatics 26, 403–404. doi: 10.1093/bioinformatics/btp670
Meglécz, E., Pech, N., Gilles, A., Dubut, V., Hingamp, P., Trilles, A., et al. (2014). QDD version 3.1: a user-friendly computer program for microsatellite selection and primer design revisited: experimental validation of variables determining genotyping success rate. Mol. Ecol. Resour. 14, 1302–1313. doi: 10.1111/1755-0998.12271
Meinke, D. W., Cherry, J. M., Dean, C., Rounsley, S. D., and Koornneef, M. (1998). Arabidopsis thaliana: a model plant for genome analysis. Genome 282, 662–682. doi: 10.1126/science.282.5389.662
Morgante, M., Hanafey, M., and Powell, W. (2002). Microsatellites are preferentially associated with nonrepetitive DNA in plant genomes. Nat. Genet. 30, 194–200. doi: 10.1038/ng822
Morgante, M., and Olivieri, A. M. (1992). PCR-amplified microsatellites as markers in plant genetics. Plant J. 3, 175–182. doi: 10.1111/j.1365-313X.1993.tb00020.x
Nadeau, N. J., and Jiggins, C. D. (2010). A golden age for evolutionary genetics? Genomic studies of adaptation in natural populations. Trends Genet. 26, 484–492. doi: 10.1016/j.tig.2010.08.004
Nawrocki, E. P., Burge, S. W., Bateman, A., Daub, J., Eberhardt, R. Y., Eddy, S. R., et al. (2015). Rfam 12.0: updates to the RNA families database. Nucleic Acids Res. 43, D130–D137. doi: 10.1093/nar/gku1063
NCBI Resource Coordinators (2016). Database resources of the National Center for Biotechnology Information. Nucleic Acids Res. 44, D7–D19. doi: 10.1093/nar/gkv1290
Novikova, P. Y., Hohmann, N., Nizhynska, V., Tsuchimatsu, T., Ali, J., Muir, G., et al. (2016). Sequencing of the genus Arabidopsis identifies a complex history of nonbifurcating speciation and abundant trans-specific polymorphism. Nat. Publishing Group 48, 1077–1082. doi: 10.1038/ng.3617
Nybom, H. (2004). Comparison of different nuclear DNA markers for estimating intraspecific genetic diversity in plants. Mol. Ecol. 13, 1143–1155. doi: 10.1111/j.1365-294X.2004.02141.x
Olango, T. M., Tesfaye, B., Pagnotta, M. A., Pè, M. E., and Catellani, M. (2015). Development of SSR markers and genetic diversity analysis in enset (Ensete ventricosum (Welw.) Cheesman), an orphan food security crop from Southern Ethiopia. BMC Genet. 16:98. doi: 10.1186/s12863-015-0250-8
Pashley, C. H. (2006). EST databases as a source for molecular markers: lessons from helianthus. J. Hered. 97, 381–388. doi: 10.1093/jhered/esl013
Peakall, R., and Smouse, P. E. (2006). genalex 6: genetic analysis in excel. Population genetic software for teaching and research. Mol. Ecol. Notes 6, 288–295. doi: 10.1111/j.1471-8286.2005.01155.x
Peakall, R., and Smouse, P. E. (2012). GenAlEx 6.5: genetic analysis in excel. Population genetic software for teaching and research-an update. Bioinformatics 28, 2537–2539. doi: 10.1093/bioinformatics/bts460
Peng, X., Teng, L., Yan, X., Zhao, M., and Shen, S. (2015). The cold responsive mechanism of the paper mulberry: decreased photosynthesis capacity and increased starch accumulation. BMC Genomics 16:898. doi: 10.1186/s12864-015-2047-6
Piwowar, H. A., Day, R. S., and Fridsma, D. B. (2007). Sharing detailed research data is associated with increased citation rate. PLoS ONE 2:e308. doi: 10.1371/journal.pone.0000308
Qiu, L., Yang, C., Tian, B., Yang, J.-B., and Liu, A. (2010). Exploiting EST databases for the development and characterization of EST-SSR markers incastor bean (Ricinus communis L.). BMC Plant Biol. 10:278. doi: 10.1186/1471-2229-10-278
Rana, S. B., Zadlock, F. J., Zhang, Z., Murphy, W. R., and Bentivegna, C. S. (2016). Comparison of de novo transcriptome assemblers and k-mer strategies using the killifish, Fundulus heteroclitus. PLoS ONE 11:e0153104. doi: 10.1371/journal.pone.0153104
Ritland, K. (2000). Marker-inferred relatedness as a tool for detecting heritability in nature. Mol. Ecol. 1195–1204. doi: 10.1046/j.1365-294x.2000.00971.x
Rozen, S., and Skaletsky, H. (2000). Primer3 on the WWW for general users and for biologist programmers. Methods Mol. Biol. 132, 365–386. doi: 10.1385/1-59259-192-2:365
Salipante, S. J., Kawashima, T., Rosenthal, C., Hoogestraat, D. R., Cummings, L. A., Sengupta, D. J., et al. (2014). Performance comparison of illumina and ion torrent next-generation sequencing platforms for 16S rRNA-based bacterial community profiling. Appl. Environ. Microbiol. 80, 7583–7591. doi: 10.1128/AEM.02206-14
Simão, F. A., Waterhouse, R. M., Ioannidis, P., Kriventseva, E. V., and Zdobnov, E. M. (2015). BUSCO: assessing genome assembly and annotation completeness with single-copy orthologs. Bioinformatics 31, 3210–3212. doi: 10.1093/bioinformatics/btv351
Solonenko, S. A., Ignacio-Espinoza, J. C., Alberti, A., Cruaud, C., Hallam, S., Konstantinidis, K., et al. (2013). Sequencing platform and library preparation choices impact viral metagenomes. BMC Genomics 14:320. doi: 10.1186/1471-2164-14-320
Squirrell, J., Hollingsworth, P. M., Woodhead, M., Russell, J., Lowe, A. J., Gibby, M., et al. (2003). How much effort is required to isolate nuclear microsatellites from plants? Mol. Ecol. 12, 1339–1348. doi: 10.1046/j.1365-294X.2003.01825.x
Tabbasam, N., Zafar, Y., and Mehboob-ur-Rahman (2013). Pros and cons of using genomic SSRs and EST-SSRs for resolving phylogeny of the genus Gossypium. Plant Syst. Evol. 300, 559–575. doi: 10.1007/s00606-013-0891-x
Tanwar, U. K., Pruthi, V., and Randhawa, G. S. (2017). RNA-Seq of Guar (Cyamopsis tetragonoloba, L. Taub.) Leaves: de novo transcriptome assembly, functional annotation and development of genomic resources. Front. Plant Sci. 8:91. doi: 10.3389/fpls.2017.00091
The UniProt Consortium (2007). The Universal Protein Resource (UniProt). Nucleic Acids Res. 36, D190–D195. doi: 10.1093/nar/gkm895
Tiffin, P., and Hahn, M. W. (2002). Coding sequence divergence between two closely related plant species: Arabidopsis thaliana and Brassica rapa ssp. pekinensis. J. Mol. Evol. 54, 746–753. doi: 10.1007/s00239-001-0074-1
Tremblay, J., Singh, K., Fern, A., Kirton, E. S., He, S., Woyke, T., et al. (2015). Primer and platform effects on 16S rRNA tag sequencing. Front. Microbiol. 6:771. doi: 10.3389/fmicb.2015.00771
Varshney, R. K., Graner, A., and Sorrells, M. E. (2005a). Genic microsatellite markers in plants: features and applications. Trends Biotechnol. 23, 48–55. doi: 10.1016/j.tibtech.2004.11.005
Varshney, R. K., Sigmund, R., Börner, A., Korzun, V., Stein, N., Sorrells, M. E., et al. (2005b). Interspecific transferability and comparative mapping of barley EST-SSR markers in wheat, rye and rice. Plant Sci. 168, 195–202. doi: 10.1016/j.plantsci.2004.08.001
Victoria, F. C., da Maia, L. C., and de Oliveira, A. C. (2011). In silico comparative analysis of SSR markers in plants. BMC Plant Biol. 11:15. doi: 10.1186/1471-2229-11-15
Wang, Z., Weber, J. L., Zhong, G., and Tanksley, S. D. (1994). Survey of plant short tandem DNA repeats. Theor. Appl. Genet. 88, 1–6. doi: 10.1007/BF00222386
Wöhrmann, T., Barros Pinangé, D. S., Krapp, F., Benko-Iseppon, A.-M., Huettel, B., and Weising, K. (2012). Development of 15 nuclear microsatellite markers in the genus Dyckia (Pitcairnioideae; Bromeliaceae) using 454 pyrosequencing. Conserv. Genet. Resour. 5, 81–84. doi: 10.1007/s12686-012-9738-y
Wöhrmann, T., and Weising, K. (2011). In silico mining for simple sequence repeat loci in a pineapple expressed sequence tag database and cross-species amplification of EST-SSR markers across Bromeliaceae. Theor. Appl. Genet. 123, 635–647. doi: 10.1007/s00122-011-1613-9
Woodhead, M., Russell, J., Squirrell, J., Hollingsworth, P. M., Mackenzie, K., Gibby, M., et al. (2005). Comparative analysis of population genetic structure in Athyrium distentifolium (Pteridophyta) using AFLPs and SSRs from anonymous and transcribed gene regions. Mol. Ecol. 14, 1681–1695. doi: 10.1111/j.1365-294X.2005.02543.x
Yu, J.-K., Dake, T. M., Singh, S., Benscher, D., Li, W., Gill, B., et al. (2004). Development and mapping of EST-derived simple sequence repeat markers for hexaploid wheat. Genome 47, 805–818. doi: 10.1139/g04-057
Keywords: Brassicaceae, RNAseq/transcriptome, SSRs, molecular resources, data mining, sequencing platform
Citation: Lopez L, Wolf EM, Pires JC, Edger PP and Koch MA (2017) Molecular Resources from Transcriptomes in the Brassicaceae Family. Front. Plant Sci. 8:1488. doi: 10.3389/fpls.2017.01488
Received: 16 June 2017; Accepted: 11 August 2017;
Published: 29 August 2017.
Edited by:
Xiaowu Wang, Biotechnology Research Institute (CAAS), ChinaReviewed by:
Liangsheng Zhang, Fujian Agriculture and Forestry University, ChinaCopyright © 2017 Lopez, Wolf, Pires, Edger and Koch. This is an open-access article distributed under the terms of the Creative Commons Attribution License (CC BY). The use, distribution or reproduction in other forums is permitted, provided the original author(s) or licensor are credited and that the original publication in this journal is cited, in accordance with accepted academic practice. No use, distribution or reproduction is permitted which does not comply with these terms.
*Correspondence: Marcus A. Koch, bWFyY3VzLmtvY2hAY29zLnVuaS1oZWlkZWxiZXJnLmRl
†Present Address: Lua Lopez, Department of Biology, Penn State University, State College, PA, United States
Disclaimer: All claims expressed in this article are solely those of the authors and do not necessarily represent those of their affiliated organizations, or those of the publisher, the editors and the reviewers. Any product that may be evaluated in this article or claim that may be made by its manufacturer is not guaranteed or endorsed by the publisher.
Research integrity at Frontiers
Learn more about the work of our research integrity team to safeguard the quality of each article we publish.