- 1Key Laboratory of Biology and Genetic Improvement of Oil Crops, Ministry of Agriculture, Oil Crops Research Institute of the Chinese Academy of Agricultural Sciences, Wuhan, China
- 2State Key Laboratory Breeding Base for Zhejiang Sustainable Pest and Disease Control, Zhejiang Academy of Agricultural Sciences, Hangzhou, China
Plant architecture is crucial for rapeseed yield and is determined by plant height (PH), branch initiation height (BIH), branch number (BN) and leaf and inflorescence morphology. In this study, we measured three major factors (PH, BIH, and BN) in a panel of 333 rapeseed accessions across 4 years. A genome-wide association study (GWAS) was performed via Q + K model and the panel was genotyped using the 60 k Brassica Infinium SNP array. We identified seven loci for PH, four for BIH, and five for BN. Subsequently, by determining linkage disequilibrium (LD) decay associated with 38 significant SNPs, we gained 31, 15, and 17 candidate genes for these traits, respectively. We also showed that PH is significantly correlated with BIH, while no other correlation was revealed. Notably, a GA signaling gene (BnRGA) and a flowering gene (BnFT) located on chromosome A02 were identified as the most likely candidate genes associated with PH regulation. Furthermore, a meristem initiation gene (BnLOF2) and a NAC domain transcriptional factor (BnCUC3) that may be associated with BN were identified on the chromosome A07. This study reveals novel insight into the genetic control of plant architecture and may facilitate marker-based breeding for rapeseed.
Introduction
Rapeseed (Brassica napus L., AACC, 2n = 38) is a globally significant oilseed crop used in the production of vegetable oil and oil meals, and ~95% of its total cultivation is in Asia, Europe, and North America. According to a new report from the United States Department of Agriculture (USDA), rapeseed production in 2015/16 is estimated at 66.9 million tons, down 4.7% from the previous year. Harvested area is estimated at 32.9 million hectares, down 5.5% from the previous year (http://www.pecad.fas.usda.gov/). To meet growing demands for both edible products and biofuel, it is critical to increase rapeseed productivity through efficient breeding.
Rapeseed productivity is determined by three components: number of siliques per plant (NSP), number of seeds per silique (NSS), and seed weight per plant (Clarke and Simpson, 1978). Simultaneously, rapeseed plant architecture is a crucial determinant of its yield and is itself determined by plant height (PH) and branch number, as well as inflorescence morphology, which indirectly influences its yield by affecting NSP (Qiu et al., 2006; Chen et al., 2014). Plant height was first selected to improve crop yields: taller plants were more easily affected by lodging and therefore showed decreased yield, while dwarf plants showed resistance to lodging and their yield could be further increased by the use of nitrogen fertilizers. In the late 1960s and early 1970s, the dwarf gene was rapidly adopted in wheat and rice to cultivate new varieties, which greatly increased their yield and precipitated the “Green Revolution” (Peng et al., 1999; Evenson and Gollin, 2003). In addition, most domesticated crops also contain the plant domestication gene TB1 (Doebley et al., 1997; Takeda et al., 2003; Aguilar-Martinez et al., 2007; Lewis et al., 2008). Recently, ideal plant architecture (IPA) has been proposed as a plant type with increased yield potential (Jiao et al., 2010; Miura et al., 2010).
In Arabidopsis and rice, the mechanisms regulating PH are well-known, and many phytohormones, including Gibberellins (GAs), Brassinosteroids (BRs), and Strigolactones (SLs) participate in this process. Genes related to phytohormone biosynthesis and signal transduction contain mutations that affect internode elongation, which in turn regulates PH (Ikeda et al., 2001; Ishikawa et al., 2005; Sun et al., 2010; Clouse, 2011; Jiang et al., 2013; Zhou et al., 2013). During the floral transition, axillary meristems are transformed into branch meristems to form branches (Teo et al., 2014). Phytohormone, including Auxin, Cytokinins (CKs), and SLs biosynthesis, transport, and signaling genes also play important roles in branch formation (Ferguson and Beveridge, 2009; Janssen et al., 2014), as do floral meristem identity genes (i.e., MYB family RAX proteins, ALOG family proteins, other meristem identity genes, flowering time genes, etc.; Liljegren et al., 1999; Hiraoka et al., 2013; Liu et al., 2013). In Arabidopsis, most integrated regulatory networks controlling the formation of branching are regulated by the gene SHOOT MERISTEMLESS (STM) (Long et al., 1996; Lenhard et al., 2002). Despite significant advances in other plants, our understanding of the molecular mechanisms of plant architecture regulation in rapeseed remains limited.
Rapeseed (B. napus) and the model plant Arabidopsis are members of the Brassicaceae. Unlike Arabidopsis thaliana, B. napus is polyphyletic, and is related to B. rapa (AA) and B. oleracea (CC) (Allender and King, 2010). So far, genomes have been sequenced for B. rapa (AA), B. oleracea (CC), and B. napus (AACC) (Wang et al., 2011; Chalhoub et al., 2014; Liu et al., 2014). B. napus is a young allopolyploid and most orthologous genes are duplicated compared to the respective progenitor genomes (Chalhoub et al., 2014). Consequently, obtaining a detailed characterization of each copy is difficult.
Genetic mapping studies have employed bi-parental mapping populations to identify many quantitative trait locus (QTLs) for yield traits in rapeseed. However, most of these QTLs are localized to a large interval (10–20 cM) on chromosomes (Butruille et al., 1999; Quijada et al., 2006; Udall et al., 2006; Chen et al., 2007; Mei et al., 2009; Shi et al., 2009). The genome-wide association study (GWAS) has become a powerful tool to identify multiple related candidate genes regulating important traits in crops (Huang et al., 2010; Brachi et al., 2011; Li H. et al., 2013). This method is performed by scanning a genome using abundant single-nucleotide polymorphisms (SNPs) representing broad genetic variability. Presently, the GWAS in rapeseed has been used to identify loci and candidate genes related to oil content, plant architecture, flowering time, and other yield traits (Schiessl et al., 2015; Li et al., 2016; Liu et al., 2016; Lu et al., 2016; Sun et al., 2016a,b; Sun F. M. et al., 2016; Wang et al., 2016; Xu et al., 2016).
In this study, we report a GWAS for plant architecture traits (PH, BIH, and BN) in rapeseed using the 60 K Brassica Infinium SNP array on a panel with 333 accessions that cover a broad range of genetic diversity. We further identify several possible candidate genes for the three traits underlying these QTLs by LD decays harboring significant SNPs. Although some candidate QTLs are consistent with those found in previous studies, we have also identified novel QTLs for these traits. Moreover, the SNPs and candidate genes in our study may facilitate marker-based breeding to improve plant architecture in order to increase rapeseed yield.
Materials and Methods
Plant Materials and Trait Measurement
The association population used in this study consisted of 333 diverse rapeseed accessions (20 are winter type, 308 are semi-winter type and 5 are unknown) collected as part of a recently published study (Sun F. M. et al., 2016). The rapeseed accessions were grown in two replicates over the course of 4 years (2012–2015) in Wuhan, China (E 114.32°, N 30.52°). Individuals from germplasm populations were genotyped using the 60 K Brassica Infinium SNP array. Accessions were grown in plots of 2 rows, with 10–15 plants in each row. Between eight and ten plants were selected from the center of each row to measure the three traits at maturity: PH, BIH, and BN.
The length of the plant from the base of the stem to the tip of the main inflorescence was noted as PH. The length from the base of the stem to the first primary branch base was recorded as BIH. We also measured the number of primary branches arising from the main shoot (BN). Correlation analyses of PH, BIH, and BN for the association panel were performed in R3.3.0 (Ihaka and Gentleman, 1996).
In silico Mapping and Linkage Disequilibrium (LD) Analysis
The probe sequences of 52,157 SNPs were used to perform a BLASTN search through the B. napus genome database (Chalhoub et al., 2014). Only top blast-hits with an e-value threshold of e−15 were used. Some SNPs were excluded due to a match in BLAST with multiple loci with identical scores. SNPs that showed a minor allele frequency (MAF) of <5% or that showed a maximal missing frequency of >2% were excluded (Brachi et al., 2011), remaining 32,297 SNPs identified, covering all 19 chromosomes of the B. napus genome and providing approximately one SNP per 25 kb. An estimate of the linkage disequilibrium (LD) was calculated using squared allele frequency correlations (r2) between all pairs of SNPs using TASSEL 5.2.28 (Bradbury et al., 2007).
Genome-Wide Association Study
All the SNPs were used to calculate the principal component analysis (PCA) matrix using the GCTA tool, and a subset of 3,571 SNPs (MAF ≥ 0.2) evenly distributed across the whole genome (every 150 k) were selected to perform population structure (Q) and relative kinship analysis (K). STRUCTURE v2.3.4 was used to calculate a Q matrix, the putative number of genetic groups (k-value) setting from 1 to 10 with five independent runs (Pritchard et al., 2000). The length of the burn-in period and the number of Markov Chain Monte Carlo (MCMC) replications after burn-in were set to 50,000 and 100,000 iterations, respectively. The relative kinship K matrix was performed by the SPAGeDi software package (Hardy and Vekemans, 2002; Yang et al., 2011). The Δk method was used to determine the most likely number of groups or subpopulations, as described by Evanno et al. (2005).
Association analysis was performed using TASSEL 5.2.28 (Bradbury et al., 2007), using a mixed linear model (MLM) to calculate the association in all analyses, incorporating Q matrix/PCA and kinship data (K) (Zhao et al., 2011). The MLM was applied using default settings (P3D for variance component analysis and compression set to optimum level). For MLM (Q + K), the significance threshold for significantly associated markers was set to p ≤ 4.06 × 10−4 [−log10 (p-value) = 3.39].
Candidate Gene Mining
To identify candidate genes, local LD decay was calculated using TASSEL5.2.28 to capture flanking regions of up to 450 kb on either side of significant SNPs, with a cut-off value of r2 = 0.2. Gene sequences that correspond to putative orthologs in A. thaliana were based on GO annotations (http://www.arabidopsis.org/index.jsp). The genes with GO terms for auxin, GA, IAA, SL, CK, and flowering time were highlighted, and the closest one of these genes was considered as the most likely candidate.
Results
Phenotypic Variation among Accessions for PH, BIH, and BN
Extensive phenotypic variations for PH, BIH, and BN were observed in the 333 accessions that were grown over the course of 4 years (2012–2015). PH varied from 86.2 to 206.0 cm, with 1.6 to 2.4-fold variations across the 4 years (Figure 1A, Table 1). BIH varied from 6.9 to 122.0 cm, with 5.9 to 17.3-fold variations across the 4 years (Figure 1B, Table 1). BN varied from 1.7 to 15.0, with 3.5 to 8.6-fold variations across the 4 years (Figure 1C, Table 1).
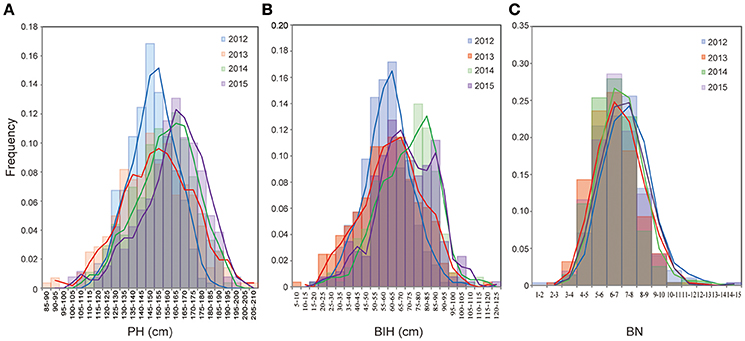
Figure 1. Frenquency distribution of PH (A), BIH (B), and BN (C) in the association panel of 333 accessions in 4 years (2012-2015). PH, plant height; BIH, branch initation height; BN, branch number.
In addition, we assessed these three traits for any significant correlation. PH and BIH were significantly correlated across the 4 years with a Pearson's correlation coefficient of 0.3 to 0.8 (Table S1). This suggests that these two traits may show genetic linkage or that some genes have pleiotropic roles in controlling these phenotypes. However, no other significant correlations were found in our accession panel, between the PH and BN, or between BN and BIH (Table S1).
SNP Quality Control, Performance, and in silico Mapping
SNP genotyping was performed using the Brassica 60 K SNP array. We blasted the SNP probe sequences to the B. napus genome database (http://www.Genoscope.cns.fr/brassicanapus/) and a total of 34,292 SNP markers (65.7%) were selected to genotype the panel of 333 accessions (Table 2). The C04 linkage group had the most SNPs (3,182), with a marker density of one per 20.89 kb, whereas the C05 linkage group had the least SNPs (1,085), with a marker density of one per 39.8 kb (Table 2). Altogether, the overall mapping results demonstrate the high quality of the genotyping in this study.
Linkage Disequilibrium
We calculated LD in 333 accessions using the parameter r2 with the 34,292 SNP markers. In this analysis, three chromosomes (A08, C02, and C07) showed strong LD, with distances ranging from 1,300 to 1,710 kb; while others exhibited modest LD, with distances ranging from 100 to 825 kb when r2 = 0.2 (Table 2). The average LD decay of chromosomes A and C were of 190 and 700 kb respectively, while the average LD decay of the whole genome (A + C chromosomes) was of 450 kb when r2 = 0.2 (Sun F. M. et al., 2016; Figure 2A).
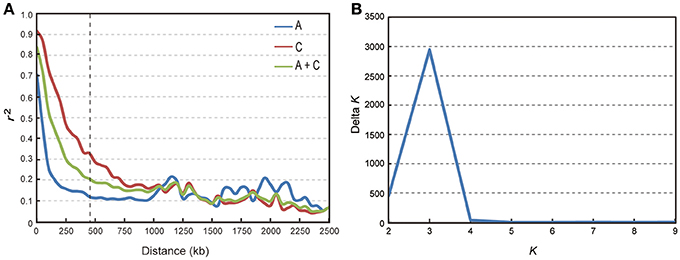
Figure 2. Analysis of LD decay and the population structure of 333 rapeseed accessions. (A) Subgenome and genome distribution of r2-value (linkage disequilibrium decay, LD) estimated from 333 rapeseed accessions. (B) Calculation of Δ K based on the value of Ln P(D) between successive K-values.
Genome-Wide Association Study
We performed GWAS with mixed linear modeling (MLM) across 4 years. For the groups-populations, we created a Q matrix (k = 2) for all 333 accessions, in order to obtain the highest Δ k-value (Figure 2B). In addition, minor differences were observed among the PCA, Q, K, Q + K, and PCA + K models, and the Q + K model is an appropriate model (Figure 3). Thus, the Q + K model was used for GWAS. As in other GWAS studies, we set a threshold of −log10 P = 3.39 as a significant association (Figure S1; Benjamini and Hochberg, 1995; Wang et al., 2016). Furthermore, QQ plots of PH in 2012 and 2013 were not linear. As a result, the SNPs showing the 50 highest −log10 P-values in each year were compiled for the PH trait for these 2 years to identify potentially significant SNP clusters (Figure 4; Ueda et al., 2015). Overall, for all traits analyzed, a total of 158 SNPs associated with PH, BIH, and BN were identified (Table S2). Furthermore, LD blocks (450 kb) harboring the repeating SNPs across the 4 years (and detected in at least two of those years) were identified as regions containing putative candidate loci. This led to the selection of 38 SNPs (Table 3), which in turn could be merged into seven, four, and five loci for PH, BIH, and BN, respectively (Figures 4–6, Table 3, Figures S2–S4).
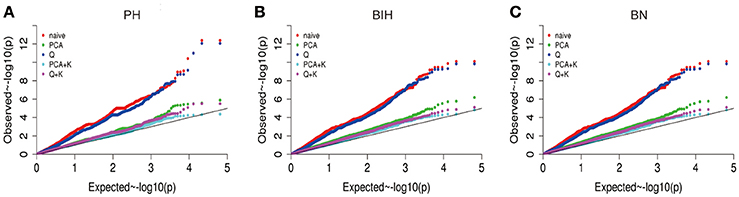
Figure 3. Quantile-quantile plots of estimated −log10 (p-value) for PH (A), BIH (B), and BN (C) using five models. PH, plant height; BIH, branch initation height; BN, branch number.
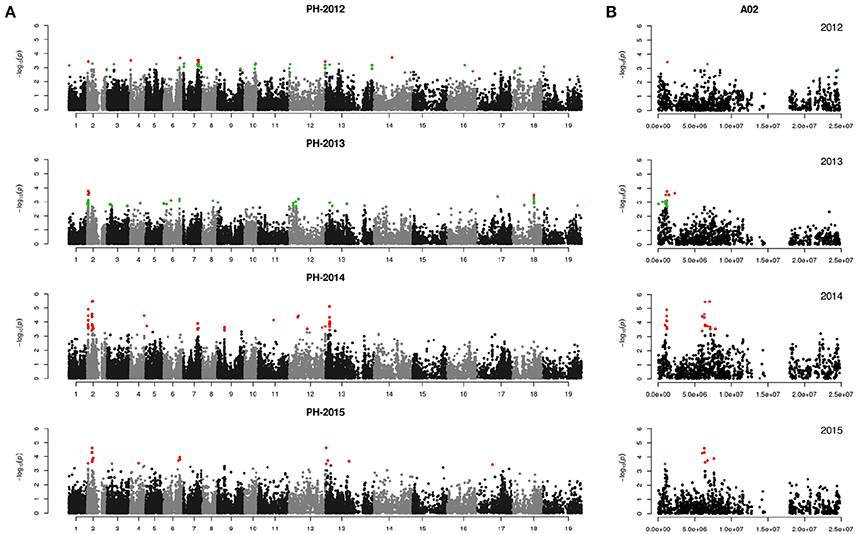
Figure 4. Genome-wide association study of plant height in the panel of 333 accessions. (A) Manhattan plot of the MLM for plant height in 4 years. (B) Main locus on chromosome A02 for plant height regulation. Red color plots are identified by the Q+K model, green color plots are identified by the top 50 method.
For PH, we detected nine GWAS peak SNPs in the seven loci located on chromosomes A02, A06, C03, and A07, and five of which were detected in at least 2 years (Figure 4, Table 3). These seven peak SNPs explained 35.56% of the total phenotypic variance, with the largest contribution (R2 ~9.79%) from SNP Bn-A02-p9505646 (Table 3). For BIH, four peak SNPs were detected on chromosomes A02, A07, A08, and A09, and three of these SNPs were detected in at least 2 years (Figure 5, Table 3). These three SNPs explained 27.96% of the phenotypic variance (Table 3). SNP Bn-A02-p9505646 was detected in both PH and BIH traits and it accounted for 9.36% of the total phenotypic variance in BIH (Table 3). For BN, five peak SNPs were detected on chromosomes A01, A03, A07, C04, and C09 (Figure 6), two of which were detected in at least 3 years, with the largest contribution (R2 ~11.54%) from SNP Bn-A07-p21413042 (Table 3).
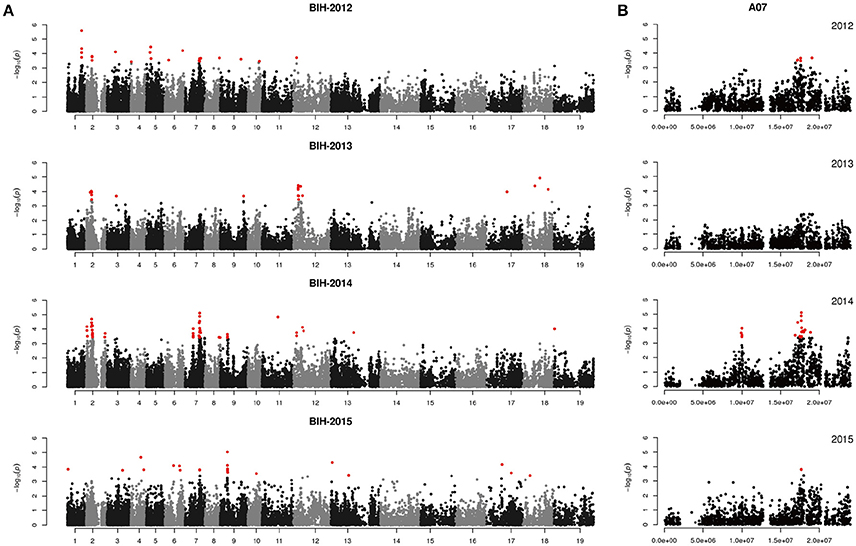
Figure 5. Genome-wide association study of branch initiation height in the panel of 333 accessions. (A) Manhattan plot of the MLM for branch initiation height in 4 years. (B) Main locus on chromosome A07 for branch initiation height regulation.
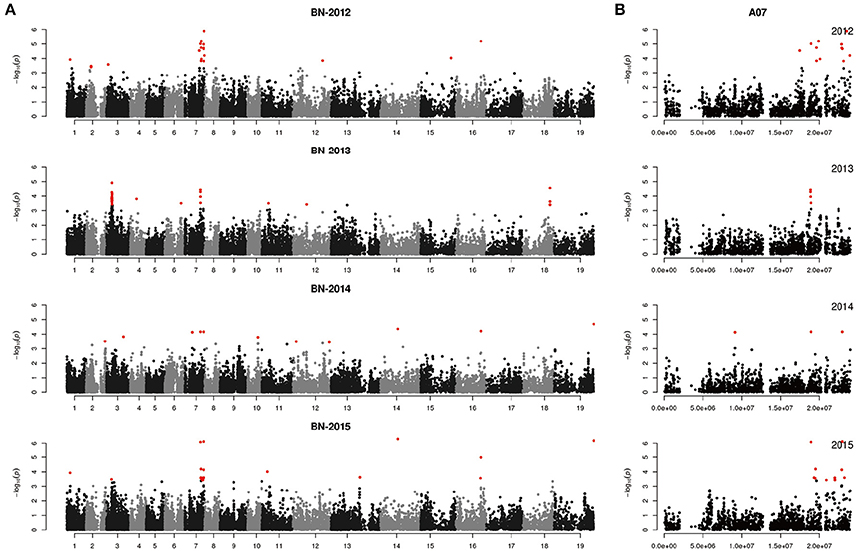
Figure 6. Genome-wide association study of branch number in the panel of 333 accessions. (A) Manhattan plot of the MLM for branch number in 4 years. (B) Main locus on chromosome A07 for branch number regulation.
Allele-Specific SNP Markers Correlated with Physical Traits (PH, BIH, and BN)
The GWAS identified several significant SNP markers in the regulation of three traits (Table 3). To find useful SNP markers for marker-based breeding, we estimated the allelic effect of the peak SNPs across these three traits. The marker Bn-A02-p9505646 (G/A) of the GG allele showed the largest contribution (R2 ~9.79%) to PH regulation, measuring 21.5 cm more than those of the AA allele (P < 0.05; Figure 7A). Apart from the Bn-A02-p9505646 marker, individuals with the CC allele marker Bn-A09-p9669847 showed the largest contribution to BIH regulation (R2 ~9.87%) and measured 11.4 cm more than those of the AA allele (P < 0.05; Figure 7B). The average BN of individuals with the AA allele with marker Bn-A03-p7326202 showed the higher contribution (R2~8.61%) and was significantly greater than GG alleles on chromosome A3 (Figure 7C). These SNP markers may permit marker-based breeding for rapeseed plant architecture.
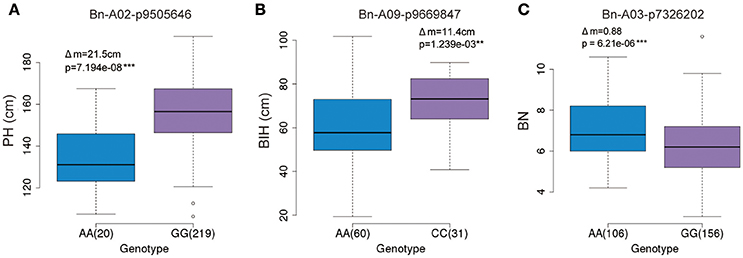
Figure 7. Allelic effects of associated SNPs for three traits. (A) Phenotypic difference between AA and GG with marker Bn-A02-p9505646 for plant height in the panel. (B) Phenotypic difference between AA and CC with marker Bn-A09-p9669847 for branch initiation height in the panel. (C) Phenotypic difference between AA and GG with marker Bn-A03-p7326202 for branch number in the panel.
Identification of Candidate Genes
We searched for candidate genes from the genomic regions with significant SNPs according to GO annotations from the Arabidopsis database (http://www.arabidopsis.org/index.jsp). Notably, 31, 15, and 17 candidate genes for PH, BIH, and BN were located in each region, respectively (Table S3).
For PH, the main QTL was detected on chromosome A02, located at 6.0~6.7 M. From the peak SNP Bn-A02-p9607756 [−log10 (p) = 5.47], two candidate genes associated with GA signaling and flowering time repression were located at 77.2 and 29.4 kb, respectively (Table 4). They encode proteins GAI-DELLA (BnaA02g12260D) and FT (BnaA02g12130D), which may be involved in PH formation (Wang and Li, 2008; Salas Fernandez et al., 2009). A BR biosynthesis gene CYP450 (BnaA02g02150D), a gibberellin-regulated protein GASA4 (BnaA02g02560D), and two cell wall biosynthesis proteins (BnaA02g02700D and BnaA02g03310D) were identified up- and down-stream of the peak SNP of Bn-A02-p3760177 [−log10 (p) = 4.90] (Table S3). In addition, we also identified four candidate genes for PH regulation on chromosomes A06 and A07 (Table 4).
For BIH, the C2H2 zinc finger protein, TCP 12, and SOB five-like 2 (BnaA02g13870D, BnaA02g14010D, and BnaA02g14060D) were also identified in the candidate region around the peak SNP Bn-A02-p9505646 [−log10 (p) = 4.70] on chromosome A02 (Table 4). On chromosome A07, a CLAVATA3 protein and a LOF2 transcription factor (BnaA07g24210D and BnaA07g24240D) were identified downstream from the peak SNP Bn-A07-p15758978) [−log10 (p) = 4.88] (Table 4). Their homologs in Arabidopsis are also involved in meristem initiation and maintenance (Lee et al., 2009). In addition, on chromosome A09, we found a peak SNP Bn-A09-p9669847 with the highest probability of marker-trait association, with −log10 (p) = 5.04, and with the largest contribution to BIH variation, with R2 = 9.87%. Notably, a MATE efflux family protein (BnaA09g14730D) involved in determining the rate of organ initiation was identified close to the peak SNP (Table 4).
For BN, most candidate genes around peak SNPs were associated with Auxin, CK, and SL (Table S2). Five candidate genes, ARR11, TCP12, ARF8, LOF2, and CUC3, were identified up- and downstream of the peak SNP Bn-A07-p16996070 [−log10 (p) = 6.08] (Table 4). Another peak SNP Bn-A01-p4070059 [−log10 (p) = 3.93] contained two candidate genes (YUCC8 and IAA11). On chromosome C09, a WOX5 gene (BnaC09g49730D) was located 0.45 M from the peak SNP Bn-scaff_28053_1-p60542 [−log10 (p) = 6.18] (Table 4).
Discussion
In our study, a MLM was used to calculate the association in the GWAS analysis, while incorporating a Q matrix and kinship data to control the false discovery rate, this model was an improvement relative to the naïve GLM (Figure 3; Larsson et al., 2013). Although it has been suggested that PCA or PCA + K models may reduce false positives in GWAS analyses (Yu et al., 2006; Zhu et al., 2008; Li et al., 2016), many studies have used the Q + K model because it permits control of the false discovery rate (Cai et al., 2014; Sun F. M. et al., 2016; Wang et al., 2016). In our assays, even the QQ plots of PH deviated from the expected distribution, while the Manhattan plots showed clearly defined peaks, indicating that MLM might be the best approach to detect the main QTLs for our traits (Figures 4–6, Figure S1). According to previous studies, we defined the SNPs which less than the p-value of 4.06 × 10−4 [−log10 (p-value) = 3.39, Q + K] or in the top 50 SNPs in PH control as the candidate SNPs (Ueda et al., 2015; Wang et al., 2016). The LD decay is the key factor for mining candidate genes in a GWAS study (Yu and Buckler, 2006). We observed that LD decay in the A- and C-subgenomes is different in our panel. The LD decay in the A- subgenome was generally shorter than those in the C- subgenome, while the LD decays on A08, C02, and C07 were beyond 1.3 M. Our results for LD decay rates are similar to those found in recent GWAS studies on rapeseed (Qian et al., 2014; Liu et al., 2016), and therefore, we hold that the LD decay in our study is reliable to identify candidate genes.
Novel Genetic Control of Three Plant Architecture Traits (PH, BIH, and BN) in Rapeseed
GWAS was used to detect QTLs of PH, BIH, and BN, which are main factors regulating plant architecture in rapeseed. According to the marker-trait association SNPs, we identified seven QTLs for PH, four for BIH, and five for BN (Table 3). In the PH candidate loci, these QTLs were distributed on six chromosomes and some QTL regions were similar to those identified in previous studies. Among them, the QTLs at 1.2 and 6.4 M on chromosome A02 overlapped regions detected by Udall et al. (2006); Li X. N. et al. (2013), and Sun et al. (2016b). The QTL at 18.0 M on chromosome A07 was close to the QTLs detected by Udall et al. (2006) and Li et al. (2016). The QTL near the top of chromosome C03 was also detected by Ding et al. (2012) and Shi et al. (2009). Additionally, the QTL at 6.2 M on chromosome A03 was found by Mei et al. (2009) and Li X. N. et al. (2013), and also corresponds closely to the findings of Li et al. (2016). The QTL at 45.8 M on chromosome C02 corresponds closely to the QTL on the bottom of C02 detected by Udall et al. (2006). These results indicate that these QTLs were stable as they were detected by different map populations and in different environments using distinct methods of analysis, and they may also have been selected in rapeseed breeding. Notably, the QTL at 19.7 M of A06, which was detected in 2 years has not been found in previous studies, and may be a novel QTL.
For the BN candidate loci, only one QTL was located at 6.6 M on chromosome A03, as shown previously in other studies (Shi et al., 2011; Li et al., 2016). Interestingly, we found that all the other four QTLs were detected in a rapeseed accession that has a stable BN but increased branching. In our study, most of the accessions are varieties cultivated from different areas, we therefore deduced that the four QTLs might have converged or have been artificially selected in rapeseed breeding. However, several QTLs of PH and BN regulation reported in other studies were not detected in this study. This may be ascribed to insufficient numbers of rapeseed accessions in GWAS or to diversity of climate. In addition, all QTLs might not have been detected due to an insufficient density of SNPs in some genomic regions.
Previously, only one study identified QTLs associated with the height of the lowest primary effective branch (HPB) using two populations in rapeseed, and it identified 10 QTLs distributed on chromosomes A02, A07, A08, C04, C06, and C07 (Chen et al., 2007). In our study, BIH was measured from the ground level up to the base of the lowest primary effective branch, and four QTLs were detected in our natural population. One of these QTLs was located at 18.0 M on chromosome A08, which corresponds to the QTL detected on the bottom of the same chromosome by Chen et al. (2007). Two more QTLs were found on corresponding chromosomes A02 and A07. However, their specific regions did not overlap. To verify the reliability of these QTLs, further investigations of this trait must be undertaken.
In our study, the QTLs at 6.3 M on chromosome A02 and at 18.0 M on chromosome A07 influenced PH and BIH, and a significant correlation was found between these two traits during our correlation assay. In the A02 QTL regions, PH and BIH traits shared three SNPs and the two traits were also co-localized, which suggests pleiotropic regulation of a single gene or the existence of closely linked genes. In contrast, in the A07 QTLs, the SNP associated with the PH QTL was separated from the related SNP associated with the BIH QTL by about 700 kb, while the LD (r2) between the two SNPs was 0.27, indicating that they are separate QTLs. In addition, QTLs related to PH on chromosome A03 and A07 were found close to the QTLs related to BN on the same chromosomes, at a distance of 500 kb and 1 M, respectively. The QTL at 17.7 M on chromosome A07 related to BIH regulation was close to the QTL related to BN, at ~1.3 M. These distances are also beyond the average LD decay and the LD (r2) between the two peak SNPs for each group were relatively low, suggesting that they are separate QTLs.
Candidate Genes For PH and BN Regulation
In Arabidopsis, rice, and other plant species, BR, IAA, GA, and SL biosynthesis and signaling pathways are known to regulate PH, while mutations in most of these genes cause dwarf phenotypes (Wang and Li, 2008; Sun et al., 2010; Clouse, 2011). Additionally, genes involved in flowering time also play a role in PH regulation, such as DTH8 and Ghd7 in rice (Xue et al., 2008; Wei et al., 2010), and Flt-2L in wheat (Chen et al., 2009). In rapeseed, Mei et al. (2009) found an overlap between a flowering time locus and PH QTLs. Moreover, in some genes regulating cell wall formation, like cellulose synthase genes, mutations affecting cell elongation lead to dwarf phenotypes (Tanaka et al., 2003). Therefore, we defined PH regulation candidate genes in rapeseed based on similarity to orthologs of the QTL regions. Using the average LD decay, 5 genes (16.7%) are related to BR biosynthesis or signaling pathways, 6 genes (20%) are related to GA, 2 genes (6.7%) are associated with Auxin, 4 genes (13.3%) are involved in cell wall formation, 4 genes (13.3%) are related to flowering time regulation, and 2 gene (6.7%) are related to trichome branching development in Arabidopsis (Bischoff et al., 2010; Table S3). Notably, the RGA gene, encoding a DELLA protein, displays a signal close to Bn-A02-p9505646, which itself is close to the Bn-A02-p9610453 marker identified in Sun et al. (2016b). Furthermore, we found that some flowering time QTLs (BnFT) are also located in the same region of chromosome A02 (Xu et al., 2016). In Arabidopsis and rice, most studies showed that FLOWERING LOCUS T (FT) and the rice FT homolog HEADINGDATE3a (Hd3a) gene affected flowering and PH (Kardailsky et al., 1999; Kobayashi et al., 1999; Tamaki et al., 2007). In addition, overexpressed the FT in tobacco also exhibited the dwarf phenotype (Lewis and Kernodle, 2009). Thus, we deduce that BnRGA (BnaA02g12260D) and BnFT (BnaA02g12130D) are main candidate genes on chromosome A02 that are involved in PH regulation.
Several transcription factors (TF) and hormones (Auxin, CKs, and SLs) also affect shoot outgrowth in plant architecture (Yang and Jiao, 2016). We compared these orthologs to our main candidate genes for BN. Four genes (23.5%) are related to CK biosynthesis or signaling pathways, 6 genes (35.3%) are related to IAA, 3 genes (17.6%) are associated with flowering or flower identity formation, and one gene is involved in the SL signaling pathway. Many recent studies report that SL is functionally important in BN and PH regulation; however, only one candidate gene was identified in our GWAS. This may be due to a low density of SNP markers, or due to the candidate genes being farther away from the QTL locus than the average LD decay, or due to the confounding of the population structure. In Arabidopsis, LATERAL ORGAN FUSION2 (LOF2) encodes a MYB-domain transcription factor that functions in both lateral organ separation and axillary meristem formation, in part through interaction with CUC2, CUC3, and STM (Raman et al., 2008; Lee et al., 2009). In novel loci on chromosome A07, genes BnLOF2 (BnaA07g26170D) and BnCUC3 (BnaA07g28210D) were identified as the most likely candidate genes and may play roles in both organ separation and axillary meristem formation.
Although several candidate genes were identified following the GWAS, the function of these genes remains ambiguous. In the future, it is necessary to isolate target genes by cloning these putative genes or by developing mapping populations for the QTLs, and to illustrate their functional role in relative trait regulation by transformation experiments.
Author Contributions
MZ, WH, and HW conceived and designed the research; HL performed GWAS; XW, MT, HY, XL, JL, and XS characterized the agronomic traits; MZ, CP, HL, and JX analyzed the data; and MZ wrote the manuscript. All authors read and approved the final manuscript.
Conflict of Interest Statement
The authors declare that the research was conducted in the absence of any commercial or financial relationships that could be construed as a potential conflict of interest.
Acknowledgments
This study was supported by the National High Technology Research and Development Program of China (2013AA102602), the National Key Basic Research Program of China (2015CB150200), the National Key Research and Development Program of China (2016YFD0101007) and the Natural Science Foundation of Hubei province (2016CFB286).
Supplementary Material
The Supplementary Material for this article can be found online at: http://journal.frontiersin.org/article/10.3389/fpls.2017.01246/full#supplementary-material
Figure S1. Quantile-quantile plots of the estimated –log10 (p-value) for PH, BIH, and BN, using Q+K models.
Figure S2. Close-up of the other five loci on chromosomes for PH regulation.
Figure S3. Close-up of the other three loci on chromosomes for BIH regulation.
Figure S4. Close-up of the other four loci on chromosomes for BN regulation.
Table S1. Correlation analysis among the three traits.
Table S2. Significant-SNP for PH, BIH, and BN identified by the MLM.
Table S3. Putative candidate genes for PH, BIH, and BN.
References
Aguilar-Martinez, J. A., Poza-Carrion, C., and Cubas, P. (2007). Arabidopsis BRANCHED1 acts as an integrator of branching signals within axillary buds. Plant Cell 19, 458–472. doi: 10.1105/tpc.106.048934
Allender, C. J., and King, G. J. (2010). Origins of the amphiploid species Brassica napus L. investigated by chloroplast and nuclear molecular markers. BMC Plant Biol. 10:54. doi: 10.1186/1471-2229-10-54
Benjamini, Y., and Hochberg, Y. (1995). Controlling the false discovery rate - a practical and powerful approach to multiple testing. J. R. Statist. Soc. 57, 289–300.
Bischoff, V., Nita, S., Neumetzler, L., Schindelasch, D., Urbain, A., Eshed, R., et al. (2010). TRICHOME BIREFRINGENCE and its homolog AT5G01360 encode plant-specific DUF231 proteins required for cellulose biosynthesis in Arabidopsis. Plant Physiol. 153, 590–602. doi: 10.1104/pp.110.153320
Brachi, B., Morris, G. P., and Borevitz, J. O. (2011). Genome-wide association studies in plants: the missing heritability is in the field. Genome Biol. 12:232. doi: 10.1186/gb-2011-12-10-232
Bradbury, P. J., Zhang, Z., Kroon, D. E., Casstevens, T. M., Ramdoss, Y., and Buckler, E. S. (2007). TASSEL: software for association mapping of complex traits in diverse samples. Bioinformatics 23, 2633–2635. doi: 10.1093/bioinformatics/btm308
Butruille, D. V., Guries, R. P., and Osborn, T. C. (1999). Linkage analysis of molecular markers and quantitative trait loci in populations of inbred backcross lines of Brassica napus L. Genetics 153, 949–964.
Cai, G. Q., Yang, Q. Y., Yi, B., Fan, C. C., Edwards, D., Batley, J., et al. (2014). A complex recombination pattern in the genome of allotetraploid Brassica napus as revealed by a high-density genetic map. PLoS ONE 9:e109910. doi: 10.1371/journal.pone.0109910
Chalhoub, B., Denoeud, F., Liu, S. Y., Parkin, I. A. P., Tang, H. B., Wang, X. Y., et al. (2014). Early allopolyploid evolution in the post-Neolithic Brassica napus oilseed genome. Science 345, 950–953. doi: 10.1126/science.1253435
Chen, A., Baumann, U., Fincher, G. B., and Collins, N. C. (2009). Flt-2L, a locus in barley controlling flowering time, spike density, and plant height. Funct. Integr. Genomics 9, 243–254. doi: 10.1007/s10142-009-0114-2
Chen, B. Y., Xu, K., Li, J., Li, F., Qiao, J. W., Li, H., et al. (2014). Evaluation of yield and agronomic traits and their genetic variation in 488 global collections of Brassica napus L. Genet. Resour. Crop Evol. 61, 979–999. doi: 10.1007/s10722-014-0091-8
Chen, W., Zhang, Y., Liu, X., Chen, B., Tu, J., and Tingdong, F. (2007). Detection of QTL for six yield-related traits in oilseed rape (Brassica napus) using DH and immortalized F(2) populations. Theor. Appl. Genet. 115, 849–858. doi: 10.1007/s00122-007-0613-2
Clarke, J. M., and Simpson, G. M. (1978). Influence of irrigation and seeding rates on yield and yield components of Brassica napus cv. tower. Canad. J. Plant Sci. 58, 731–737. doi: 10.4141/cjps78-108
Ding, G., Zhao, Z., Liao, Y., Hu, Y., Shi, L., Long, Y., et al. (2012). Quantitative trait loci for seed yield and yield-related traits, and their responses to reduced phosphorus supply in Brassica napus. Ann. Bot. 109, 747–759. doi: 10.1093/aob/mcr323
Doebley, J., Stec, A., and Hubbard, L. (1997). The evolution of apical dominance in maize. Nature 386, 485–488. doi: 10.1038/386485a0
Evanno, G., Regnaut, S., and Goudet, J. (2005). Detecting the number of clusters of individuals using the software STRUCTURE: a simulation study. Mol. Ecol. 14, 2611–2620. doi: 10.1111/j.1365-294X.2005.02553.x
Evenson, R. E., and Gollin, D. (2003). Assessing the impact of the green revolution, 1960 to 2000. Science 300, 758–762. doi: 10.1126/science.1078710
Ferguson, B. J., and Beveridge, C. A. (2009). Roles for Auxin, Cytokinin, and Strigolactone in regulating shoot branching. Plant Physiol. 149, 1929–1944. doi: 10.1104/pp.109.135475
Hardy, O. J., and Vekemans, X. (2002). SPAGEDi: a versatile computer program to analyse spatial genetic structure at the individual or population levels. Mol. Ecol. Notes 2, 618–620. doi: 10.1046/j.1471-8286.2002.00305.x
Hiraoka, K., Yamaguchi, A., Abe, M., and Araki, T. (2013). The Florigen Genes, F. T., and TSF modulate lateral shoot outgrowth in Arabidopsis thaliana. Plant Cell Physiol. 54, 352–368. doi: 10.1093/pcp/pcs168
Huang, X. H., Wei, X. H., Sang, T., Zhao, Q. A., Feng, Q., Zhao, Y., et al. (2010). Genome-wide association studies of 14 agronomic traits in rice landraces. Nat. Genet. 42, U961–U976. doi: 10.1038/ng.695
Ihaka, R., and Gentleman, R. (1996). R: A language for data analysis and graphics. J. Comput. Graph. Statist. 5, 299–314. doi: 10.1080/10618600.1996.10474713
Ikeda, A., Ueguchi-Tanaka, M., Sonoda, Y., Kitano, H., Koshioka, M., Futsuhara, Y., et al. (2001). slender rice, a constitutive gibberellin response mutant, is caused by a null mutation of the SLR1 gene, an ortholog of the height-regulating gene GAI/RGA/RHT/D8. Plant Cell 13, 999–1010. doi: 10.1105/tpc.13.5.999
Ishikawa, S., Maekawa, M., Arite, T., Onishi, K., Takamure, I., and Kyozuka, J. (2005). Suppression of tiller bud activity in tillering dwarf mutants of rice. Plant Cell Physiol. 46, 79–86. doi: 10.1093/pcp/pci022
Janssen, B. J., Drummond, R. S. M., and Snowden, K. C. (2014). Regulation of axillary shoot development. Curr. Opin. Plant Biol. 17, 28–35. doi: 10.1016/j.pbi.2013.11.004
Jiang, L., Liu, X., Xiong, G. S., Liu, H. H., Chen, F. L., Wang, L., et al. (2013). DWARF 53 acts as a repressor of strigolactone signalling in rice. Nature 504, 401–410. doi: 10.1038/nature12870
Jiao, Y., Wang, Y., Xue, D., Wang, J., Yan, M., Liu, G., et al. (2010). Regulation of OsSPL14 by OsmiR156 defines ideal plant architecture in rice. Nat. Genet. 42, 541–544. doi: 10.1038/ng.591
Kardailsky, I., Shukla, V. K., Ahn, J. H., Dagenais, N., Christensen, S. K., Nguyen, J. T., et al. (1999). Activation tagging of the floral inducer FT. Science 286, 1962–1965. doi: 10.1126/science.286.5446.1962
Kobayashi, Y., Kaya, H., Goto, K., Iwabuchi, M., Araki, T. (1999). A pair of related genes with antagonistic roles in mediating flowering signals. Science 286, 1960–1962. doi: 10.1126/science.286.5446.1960
Larsson, S. J., Lipka, A. E., and Buckler, E. S. (2013). Lessons from Dwarf8 on the strengths and weaknesses of structured association mapping. PLoS Genet. 9:e1003246. doi: 10.1371/journal.pgen.1003246
Lee, D. K., Geisler, M., and Springer, P. S. (2009). LATERAL ORGAN FUSION1 and LATERAL ORGAN FUSION2 function in lateral organ separation and axillary meristem formation in Arabidopsis. Development 136, 2423–2432. doi: 10.1242/dev.031971
Lenhard, M., Jurgens, G., and Laux, T. (2002). The WUSCHEL and SHOOTMERISTEMLESS genes fulfil complementary roles in Arabidopsis shoot meristem regulation. Development 129, 3195–3206.
Lewis, J. M., Mackintosh, C. A., Shin, S., Gilding, E., Kravchenko, S., Baldridge, G., et al. (2008). Overexpression of the maize Teosinte Branched1 gene in wheat suppresses tiller development. Plant Cell Rep. 27, 1217–1225. doi: 10.1007/s00299-008-0543-8
Lewis, R. S., and Kernodle, S. P. (2009). A method for accelerated trait conversion in plant breeding. Theor. Appl. Genet. 118, 1499–1508. doi: 10.1007/s00122-009-0998-1
Li, F., Chen, B. Y., Xu, K., Gao, G. Z., Yan, G. X., Qiao, J. W., et al. (2016). A genome-wide association study of plant height and primary branch number in rapeseed (Brassica napus). Plant Sci. 242, 169–177. doi: 10.1016/j.plantsci.2015.05.012
Li, H., Peng, Z. Y., Yang, X. H., Wang, W. D., Fu, J. J., Wang, J. H., et al. (2013). Genome-wide association study dissects the genetic architecture of oil biosynthesis in maize kernels. Nat. Genet. 45, U43–U72. doi: 10.1038/ng.2484
Li, X. N., Ramchiary, N., Dhandapani, V., Choi, S. R., Hur, Y., Nou, I. S., et al. (2013). Quantitative trait loci mapping in Brassica rapa revealed the structural and functional conservation of genetic loci governing morphological and yield component traits in the A, B, and C subgenomes of Brassica species. DNA Res. 20, 1–16. doi: 10.1093/dnares/dss029
Liljegren, S. J., Gustafson-Brown, C., Pinyopich, A., Ditta, G. S., and Yanofsky, M. F. (1999). Interactions among APETALA1, LEAFY, and TERMINAL FLOWER1 specify meristem fate. Plant Cell 11, 1007–1018. doi: 10.1105/tpc.11.6.1007
Liu, C., Teo, Z. W. N., Bi, Y., Song, S. Y., Xi, W. Y., Yang, X. B., et al. (2013). A conserved genetic pathway determines inflorescence architecture in Arabidopsis and Rice. Dev. Cell 24, 612–622. doi: 10.1016/j.devcel.2013.02.013
Liu, S., Fan, C. C., Li, J. N., Cai, G. Q., Yang, Q. Y., Wu, J., et al. (2016). A genome-wide association study reveals novel elite allelic variations in seed oil content of Brassica napus. Theor. Appl. Genet. 129, 1203–1215. doi: 10.1007/s00122-016-2697-z
Liu, S., Liu, Y., Yang, X., Tong, C., Edwards, D., Parkin, I. A., et al. (2014). The Brassica oleracea genome reveals the asymmetrical evolution of polyploid genomes. Nat. Commun. 5:3930. doi: 10.1038/ncomms4930
Long, J. A., Moan, E. I., Medford, J. I., and Barton, M. K. (1996). A member of the KNOTTED class of homeodomain proteins encoded by the STM gene of Arabidopsis. Nature 379, 66–69. doi: 10.1038/379066a0
Lu, K., Xiao, Z. C., Jian, H. J., Peng, L., Qu, C. M., Fu, M. L., et al. (2016). A combination of genome-wide association and transcriptome analysis reveals candidate genes controlling harvest index-related traits in Brassica napus. Sci. Rep. 6, 36452. doi: 10.1038/srep36452
Mei, D. S., Wang, H. Z., Hu, Q., Li, Y. D., Xu, Y. S., and Li, Y. C. (2009). QTL analysis on plant height and flowering time in Brassica napus. Plant Breed. 128, 458–465. doi: 10.1111/j.1439-0523.2008.01528.x
Miura, K., Ikeda, M., Matsubara, A., Song, X. J., Ito, M., Asano, K., et al. (2010). OsSPL14 promotes panicle branching and higher grain productivity in rice. Nat. Genet. 42, 545–549. doi: 10.1038/ng.592
Peng, J., Richards, D. E., Hartley, N. M., Murphy, G. P., Devos, K. M., Flintham, J. E., et al. (1999). ‘Green revolution’ genes encode mutant gibberellin response modulators. Nature 400, 256–261. doi: 10.1038/22307
Pritchard, J. K., Stephens, M., and Donnelly, P. (2000). Inference of population structure using multilocus genotype data. Genetics 155, 945–959.
Qian, L. W., Qian, W., and Snowdon, R. J. (2014). Sub-genomic selection patterns as a signature of breeding in the allopolyploid Brassica napus genome. BMC Genomics 15:1170. doi: 10.1186/1471-2164-15-1170
Qiu, D., Morgan, C., Shi, J., Long, Y., Liu, J., Li, R., et al. (2006). A comparative linkage map of oilseed rape and its use for QTL analysis of seed oil and erucic acid content. Theor. Appl. Genet. 114, 67–80. doi: 10.1007/s00122-006-0411-2
Quijada, P. A., Udall, J. A., Lambert, B., and Osborn, T. C. (2006). Quantitative trait analysis of seed yield and other complex traits in hybrid spring rapeseed (Brassica napus L.): 1. Identification of genomic regions from winter germplasm. Theor. Appl. Genet. 113, 549–561. doi: 10.1007/s00122-006-0323-1
Raman, S., Greb, T., Peaucelle, A., Blein, T., Laufs, P., and Theres, K. (2008). Interplay of miR164, CUP-SHAPED COTYLEDON genes and LATERAL SUPPRESSOR controls axillary meristem formation in Arabidopsis thaliana. Plant J. 55, 65–76. doi: 10.1111/j.1365-313X.2008.03483.x
Salas Fernandez, M. G., Becraft, P. W., Yin, Y., and Lubberstedt, T. (2009). From dwarves to giants? Plant height manipulation for biomass yield. Trends Plant Sci. 14, 454–461. doi: 10.1016/j.tplants.2009.06.005
Schiessl, S., Iniguez-Luy, F., Qian, W., and Snowdon, R. J. (2015). Diverse regulatory factors associate with flowering time and yield responses in winter-type Brassica napus. BMC Genomics 16:s737. doi: 10.1186/s12864-015-1950-1
Shi, J. Q., Li, R. Y., Qiu, D., Jiang, C. C., Long, Y., Morgan, C., et al. (2009). Unraveling the complex trait of crop yield with quantitative trait loci mapping in Brassica napus. Genetics 182, 851–861. doi: 10.1534/genetics.109.101642
Shi, J., Li, R., Zou, J., Long, Y., and Meng, J. (2011). A dynamic and complex network regulates the heterosis of yield-correlated traits in rapeseed (Brassica napus L.). PLoS ONE 6:e21645. doi: 10.1371/journal.pone.0021645
Sun, C. M., Wang, B. Q., Wang, X. H., Hu, K. N., Li, K. D., Li, Z. Y., et al. (2016a). Genome-wide association study dissecting the genetic architecture underlying the branch angle trait in rapeseed (Brassica napus L.). Sci. Rep. 6:33673. doi: 10.1038/srep33673
Sun, C. M., Wang, B. Q., Yan, L., Hu, K. N., Liu, S., Zhou, Y. M., et al. (2016b). Genome-Wide association study provides insight into the genetic control of plant height in rapeseed (Brassica napus L.). Front. Plant Sci. 7:1102. doi: 10.3389/fpls.2016.01102
Sun, F. M., Liu, J., Hua, W., Sun, X. C., Wang, X. F., and Wang, H. Z. (2016). Identification of stable QTLs for seed oil content by combined linkage and association mapping in Brassica napus. Plant Sci. 252, 388–399. doi: 10.1016/j.plantsci.2016.09.001
Sun, Y., Fan, X. Y., Cao, D. M., Tang, W., He, K., Zhu, J. Y., et al. (2010). Integration of brassinosteroid signal transduction with the transcription network for plant growth regulation in Arabidopsis. Dev. Cell 19, 765–777. doi: 10.1016/j.devcel.2010.10.010
Takeda, T., Suwa, Y., Suzuki, M., Kitano, H., Ueguchi-Tanaka, M., Ashikari, M., et al. (2003). The OsTB1 gene negatively regulates lateral branching in rice. Plant J. 33, 513–520. doi: 10.1046/j.1365-313X.2003.01648.x
Tamaki, S., Matsuo, S., Wong, H. L., et al. (2007). Hd3a protein is a mobile flowering signal in rice. Science 316, 1033–1036. doi: 10.1126/science.1141753
Tanaka, K., Murata, K., Yamazaki, M., Onosato, K., Miyao, A., and Hirochika, H. (2003). Three distinct rice cellulose synthase catalytic subunit genes required for cellulose synthesis in the secondary wall. Plant Physiol. 133, 73–83. doi: 10.1104/pp.103.022442
Teo, Z. W. N., Song, S. Y., Wang, Y. Q., Liu, J., and Yu, H. (2014). New insights into the regulation of inflorescence architecture. Trends Plant Sci. 19, 158–165. doi: 10.1016/j.tplants.2013.11.001
Udall, J. A., Quijada, P. A., Lambert, B., and Osborn, T. C. (2006). Quantitative trait analysis of seed yield and other complex traits in hybrid spring rapeseed (Brassica napus L.): 2. Identification of alleles from unadapted germplasm. Theor. Appl. Genet. 113, 597–609. doi: 10.1007/s00122-006-0324-0
Ueda, Y., Frimpong, F., Qi, Y. T., Matthus, E., Wu, L. B., Holler, S., et al. (2015). Genetic dissection of ozone tolerance in rice (Oryza sativa L.) by a genome-wide association study. J. Exp. Bot. 66, 293–306. doi: 10.1093/jxb/eru419
Wang, N., Chen, B. Y., Xu, K., Gao, G. Z., Li, F., Qiao, J. W., et al. (2016). Association mapping of flowering time QTLs and insight into their contributions to rapeseed growth habits. Front. Plant Sci. 7:0338. doi: 10.3389/fpls.2016.00338
Wang, X., Wang, H., Wang, J., Sun, R., Wu, J., Liu, S., et al. (2011). The genome of the mesopolyploid crop species Brassica rapa. Nat. Genet. 43, 1035–1039. doi: 10.1038/ng.919
Wang, Y., and Li, J. (2008). Molecular basis of plant architecture. Annu. Rev. Plant Biol. 59, 253–279. doi: 10.1146/annurev.arplant.59.032607.092902
Wei, X. J., Xu, J. F., Guo, H. N., Jiang, L., Chen, S. H., Yu, C. Y., et al. (2010). DTH8 suppresses flowering in rice, influencing plant height and yield potential simultaneously. Plant Physiol. 153, 1747–1758. doi: 10.1104/pp.110.156943
Xu, L. P., Hu, K. N., Zhang, Z. Q., Guan, C. Y., Chen, S., Hua, W., et al. (2016). Genome-wide association study reveals the genetic architecture of flowering time in rapeseed (Brassica napus L.). DNA Res. 23, 43–52. doi: 10.1093/dnares/dsv035
Xue, W. Y., Xing, Y. Z., Weng, X. Y., Zhao, Y., Tang, W. J., Wang, L., et al. (2008). Natural variation in Ghd7 is an important regulator of heading date and yield potential in rice. Nat. Genet. 40, 761–767. doi: 10.1038/ng.143
Yang, J. A., Lee, S. H., Goddard, M. E., and Visscher, P. M. (2011). GCTA: a tool for genome-wide complex trait analysis. Am. J. Hum. Genet. 88, 76–82. doi: 10.1016/j.ajhg.2010.11.011
Yang, M., and Jiao, Y. (2016). Regulation of axillary meristem initiation by transcription factors and plant hormones. Front. Plant Sci. 7:183. doi: 10.3389/fpls.2016.00183
Yu, J. M., Pressoir, G., Briggs, W. H., Bi, I. V., Yamasaki, M., Doebley, J. F., et al. (2006). A unified mixed-model method for association mapping that accounts for multiple levels of relatedness. Nat. Genet. 38, 203–208. doi: 10.1038/ng1702
Yu, J., and Buckler, E. S. (2006). Genetic association mapping and genome organization of maize. Curr. Opin. Biotech. 17, 155–160. doi: 10.1016/j.copbio.2006.02.003
Zhao, K., Tung, C. W., Eizenga, G. C., Wright, M. H., Ali, M. L., Price, A. H., et al. (2011). Genome-wide association mapping reveals a rich genetic architecture of complex traits in Oryza sativa. Nat. Commun. 2:467. doi: 10.1038/ncomms1467
Zhou, F., Lin, Q., Zhu, L., Ren, Y., Zhou, K., Shabek, N., et al. (2013). D14-SCF(D3)-dependent degradation of D53 regulates strigolactone signalling. Nature 504, 406–410. doi: 10.1038/nature12878
Keywords: Brassica napus, plant height, branch initiation height, branch number, GWAS, QTLs
Citation: Zheng M, Peng C, Liu H, Tang M, Yang H, Li X, Liu J, Sun X, Wang X, Xu J, Hua W and Wang H (2017) Genome-Wide Association Study Reveals Candidate Genes for Control of Plant Height, Branch Initiation Height and Branch Number in Rapeseed (Brassica napus L.). Front. Plant Sci. 8:1246. doi: 10.3389/fpls.2017.01246
Received: 10 May 2017; Accepted: 30 June 2017;
Published: 18 July 2017.
Edited by:
Maoteng Li, Huazhong University of Science and Technology, ChinaReviewed by:
Shengwu Hu, Northwest A&F University, ChinaSarah-Veronica Schiessl, University of Giessen, Germany
Copyright © 2017 Zheng, Peng, Liu, Tang, Yang, Li, Liu, Sun, Wang, Xu, Hua and Wang. This is an open-access article distributed under the terms of the Creative Commons Attribution License (CC BY). The use, distribution or reproduction in other forums is permitted, provided the original author(s) or licensor are credited and that the original publication in this journal is cited, in accordance with accepted academic practice. No use, distribution or reproduction is permitted which does not comply with these terms.
*Correspondence: Hanzhong Wang, d2FuZ2h6QG9pbGNyb3BzLmNu
†These authors have contributed equally to this work.