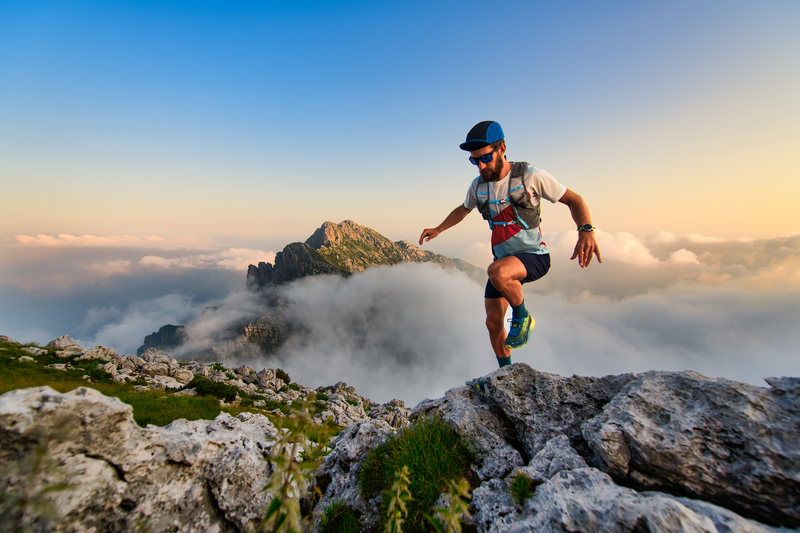
95% of researchers rate our articles as excellent or good
Learn more about the work of our research integrity team to safeguard the quality of each article we publish.
Find out more
MINI REVIEW article
Front. Plant Sci. , 20 June 2017
Sec. Plant Breeding
Volume 8 - 2017 | https://doi.org/10.3389/fpls.2017.01065
This article is part of the Research Topic Omics and systems approaches in grapevine fruit composition to understand responses to environmental factors and agronomical practices View all 26 articles
Vitis vinifera (grapevine) is one of the most important fruit crops, both for fresh consumption and wine and spirit production. The term terroir is frequently used in viticulture and the wine industry to relate wine sensory attributes to its geographic origin. Although, it can be cultivated in a wide range of environments, differences in growing conditions have a significant impact on fruit traits that ultimately affect wine quality. Understanding how fruit quality and yield are controlled at a molecular level in grapevine in response to environmental cues has been a major driver of research. Advances in the area of genomics, epigenomics, transcriptomics, proteomics and metabolomics, have significantly increased our knowledge on the abiotic regulation of yield and quality in many crop species, including V. vinifera. The integrated analysis of multiple ‘omics’ can give us the opportunity to better understand how plants modulate their response to different environments. However, ‘omics’ technologies provide a large amount of biological data and its interpretation is not always straightforward, especially when different ‘omic’ results are combined. Here we examine the current strategies used to integrate multi-omics, and how these have been used in V. vinifera. In addition, we also discuss the importance of including epigenomics data when integrating omics data as epigenetic mechanisms could play a major role as an intermediary between the environment and the genome.
Grapevine is one of the most economically important fruit crops, and it is largely used for wine production (FAO, 2012). Most the chemical compounds that give its unique characteristics to wine are synthesized during berry development (Conde et al., 2007). However, fruit/wine composition is strongly influenced by the interactions between the plant’s genome and the local growing conditions (including the vine management system), and the oenological practices of each winery (Figure 1), which could explain why it is so difficult to replicate a wine from a region outside that area.
FIGURE 1. A conceptual view of some of the terroir factors that contribute to vine balance, fruit composition and wine quality. ∗Vine genome/s refers to the possibility of the scion and rootstock used in a vineyard could be from different varieties/species (Modified from: Smart et al., 1985 and reproduced with permission from the copyright holder).
Terroir is defined as the interactions between the plants, the environment and human factors (Gladstones, 2011) and it is frequently used to relate wine sensory attributes to its geographic origin (Van Leeuwen and Seguin, 2006). Although the relevance of terroir is still under debate (Anesi et al., 2015), a better understanding of how the environment affects grape berry composition can have a significant effect on viticulture. To achieve such an understanding, it is necessary to identify the elements that drive terroir and analyze the interaction between them and the grapevine.
Terroir has been long studied, through the characterization of the different environmental factors affecting berry composition and wine quality, and climate exerts the strongest effect on berry composition (Robinson et al., 2012). Soil physicochemical properties as well have been identified as an influential factor defining the uniqueness of berry composition by vines grown in a specific climate (Cheng et al., 2014; Zerihun et al., 2015). Grapevine microbiome community may play an important role determining wine quality (Burns et al., 2015; Bokulich et al., 2016). Efforts have been made to study the grapevine microbiome landscape in relation to the vegetative growth cycle of the plant (Pinto et al., 2014), the post-harvest treatment of berries (Salvetti et al., 2016) and provenance (Bokulich et al., 2016) (For a review on microbiome analysis see Ibrahim and Kumar, 2017). Less work has been done to elucidate the molecular mechanisms involved in the plant response to terroir. A strategy to better understand the genome and environment interaction is to use ‘omics’ technologies. Omics refers to high throughput technologies that generate a large amount of data for each sample, allowing a deeper insight of the mechanisms regulating biological systems.
Using transcriptomics is possible to study the grape’s complete set of RNA transcripts encoded by the genome using high throughput methods (Hale et al., 2005). Dal Santo et al. (2013) performed gene expression analysis in a single Corvina clone cultivated in 11 different vineyards for three consecutive years. Samples strongly clustered by season, known as a vintage effect, rather than common environmental conditions. However, the genes that showed more variation in expression between years were those involved in secondary metabolism, (mainly the biosynthesis of phenylpropanoids). Only when samples from a single vintage (i.e., 2008) were analyzed, it was observed that 5% of the studied annotated coding genes were differentially regulated under different growing conditions and agronomical practices. Anesi et al. (2015) complemented this study by analyzing the transcriptome and metabolome of the same cultivar. They identified metabolites that could describe a terroir signature for each vineyard. Moreover, it was possible to correlate terroir-sensitive metabolites with changes in the transcript level of genes involved in the biosynthesis of these metabolites. Similar results were obtained by Dal Santo et al. (2016) as they identified a clear correlation between gene expression and accumulation of phenylpropanoids and flavonoids in the variety Garganega grown at four different vineyards. Small RNA profiles have been analyzed to understand the interaction between genotype and environment in the varieties Sangiovese and Cabernet Sauvignon. In silico analysis suggests that microRNAs may be involved in berry development and the accumulation of secondary metabolites (Paim Pinto et al., 2016). Transcriptional analysis of berries from different regions has also shown that transcripts from the abscisic acid (ABA) biosynthesis pathway are among the most terroir sensitive genes (Sun et al., 2015). ABA is a plant hormone that regulates important steps in plant growth and development as well as play a key role in plant biotic and abiotic stress response (Cutler et al., 2010). ABA concentrations affect anthocyanin and flavonol accumulation (Koyama et al., 2010), suggesting a possible mechanism through which the environment affects grape berry composition and wine flavor and aroma.
Metabolomics is defined as the identification and quantification of metabolites using high-throughput techniques (Cevallos-Cevallos et al., 2009). This technology can screen higher numbers of products than more traditional approaches (Pereira et al., 2006; Atanassov et al., 2009; Hong, 2011), while the use of non-targeted metabolomics approaches allows the identification of un characterized metabolites (Panighel et al., 2015). Terroir can be explored by analyzing berry metabolite composition through different analytical methods (For a review in grape and wine metabolomics see Cozzolino, 2016). Son et al. (2009) identified that differences in berry metabolomes associated to environmental regional differences (radiation and rainfall) could explain the observed differences in wine composition. Similar results were obtained by Tarr et al. (2013) who distinguished the metabolic signatures of different grapevine varieties. Metabolomic analysis has also been performed to identify chemical compounds that can be associated to regional wine quality traits (Gambetta et al., 2016, 2017). Roullier-Gall et al. (2014) assessed the metabolomics profiles of two different terroirs, which were just 2 km apart, over three vintages. Although vintage had the greatest effect in the berry’s metabolite composition, differences in fruit chemical composition associated to nearby terroirs could be detected when vintages were individually analyzed. This suggests that subtle geographical differences have a significant effect on grape/wine composition even when variability within vineyards can be relatively high (Mulas et al., 2011).
The aim of integrating multi-omic data is to reduce the gap between data generation and the ability to analyze and understand the biological mechanisms behind an organism’s response to environmental cues. The objective of multi-omic data integration is to combine different types of data to construct a model that can be used to predict complex traits and phenotypes (Figure 2). This approach also allows the identification of biomarkers and of previously unknown relationships between the datasets (Rajasundaram and Selbig, 2016). Through the integration of environmental information with genomic, epigenomic, transcriptomic, and metabolomic data, we hypothesize that it will be possible to better understand the effect of terroir at a molecular level. The use of a multi-omic approach will also help reduce the incidence of false positives generated from single source data sets (Aho, 2013; Ritchie et al., 2015). However, integration of multi-omics data is not a trivial task, because the diversity of characteristics of the data generated from the different high throughput technologies (machine sensitivity, error rate, data structure) makes its combination challenging.
FIGURE 2. Data integration workflow for four omics technologies in addition to environmental data, and fruit and wine quality data (Modified from Wanichthanarak et al., 2015 and reproduced with permission from the copyright holder).
Analysis of large data sets from different origins has been done using two main approaches: network models (NMs) and pathway analysis (PA). Both share the basic idea of storing the data in a clear and meaningful way. NMs use concepts from mathematical graph theory, to represent biological components (e.g., genes) as nodes and their interactions (physical, genetic or functional) as their links (For a review on NM applied to plant biology see Fukushima et al., 2014). NMs are classified as homogeneous or heterogeneous depending on the number of different levels of information integrated (Gligorijević and Pržulj, 2015). Homogenous approaches integrate datasets with the same type of nodes and therefore cannot analyze the connectivity between multiple datasets simultaneously. However, complex biological questions such as the molecular regulation of fruit composition in grapevine are increasingly being addressed through the integration of multiple layers of cellular information (Wong and Matus, 2017), including but not restricted to genomics, transcriptomics, proteomics and metabolomics, using heterogeneous methods. Bayesian networks (BNs) and Kernel-based methods (KBMs) are heterogeneous approaches commonly used for data integration (Zhang, 2009; Gligorijević and Pržulj, 2015). BNs are efficient detecting relationships hidden in noisy datasets but they are computationally demanding (Gligorijević and Pržulj, 2015) and are therefore, better suited for the interrogation of small datasets in hypothesis driven questions (Gligorijević and Pržulj, 2015) (i.e., the analysis of terroir on defined pathways such as those leading to the biosynthesis of metabolites related to fruit quality). KBMs are not as computationally demanding and so can integrate large molecular, structural and phenotypic datasets (Mizrachi et al., 2017), making them ideal for data driven terroir exploratory studies, biomarker discovery or for the reclassification of previously identified drivers of quality (Qi et al., 2008).
On the other hand, pathway analysis requires well documented biochemical pathways where omics data is combined to seek overrepresented groups (Wanichthanarak et al., 2015). For example, multiple co-inertia analysis (MCIA) can detect explanatory omic features even when they are not present in all datasets (Meng et al., 2014), which makes it attractive for the integration of terroir data from different studies. Random Forest implemented for pathway analysis (Pang et al., 2006), can be used to predict fruit/wine quality traits associated to terroir integrating multi-omic and phenotypic data as shown recently for potato (Acharjee et al., 2016).
Most of these multi-omics analysis approaches are pipelines that perform task sequences which share statistical methods (Bersanelli et al., 2016). Correlation analyses are the most common approaches performed to find relationships between the omics data. Simple correlation analyses, like Pearson or Spearman correlation, are widely used for multi-omics data integration (Rajasundaram et al., 2014; Rajasundaram and Selbig, 2016). Partial least square/projections to latent structures (PLS) and its extension, orthogonal partial least square (OPLS) (Tobias, 1995) have also been used for data integration from multi-omics results. Even though their predictive power is similar, OPLS results are much easier to interpret and outliers are quickly detected. OPLS can be used as a discriminate analysis (OPLS-DA), to identify differences between the overall data properties while removing systematic variation (Kirwan et al., 2012). However, these methods provided little insight when they are used in complex biological systems (highly multicollinear systems) (Wanichthanarak et al., 2015).
Modifications to these methods have been implemented to facilitate the interpretation of the data, for example, sparse PLS (sPLS) (Chun and Keleş, 2010) can better predict phenotypes through multi-omics data integration than previous methods (Rajasundaram et al., 2014). Orthogonal 2PLS (O2PLS), capable of dealing with unrelated systematic variation between datasets (Bouhaddani et al., 2016), has been successfully used for data integration of transcriptomics and metabolomics results from aspen under different light treatments (Bylesjö et al., 2007). Srivastava et al. (2013) used orthogonal projections to latent structures (OnPLS), an extension of O2PLS, to integrate transcriptomics, proteomics, and metabolomics data to construct a model that could identify biological relevant events in the oxidative stress response in poplar.
In plant science, most of data integration of omics results comes from model plants; however, there is an increase in publications on multi-omics data integration in V. vinifera. One of the first publications in multi-omics data integration in V. vinifera was the work of Zamboni et al. (2010). Integrating transcriptome, proteome and metabolome data, they identified stage specific biomarkers for berry development. Data integration was performed using two strategies, one hypothesis driven (i.e., a hypothesis was tested) and the other hypothesis free (i.e., discovery driven), in both cases principal component analysis (PCA), O2PLS and O2PLS-DA were used.
Using five different omics technologies and correlation analysis (PCA and Pearson correlation) together with biochemical pathway analysis (KEGG, PlantCyC and VitisCyC), Ghan et al. (2015) could differentiate biochemical characteristics from five different cultivars. Moreover, Anesi et al. (2015) studied the terroir effect in V. vinifera cultivar Corvina in seven different sites over a 3 years period using metabolome and transcriptome data. Using correlation analyses (PCA, PLS-DA and O2PLS-DA) they could identify a terroir signature in the berry metabolome composition for each growing site. Network analyses have been recently adopted to integrate grapevine multi omics results (Wong and Matus, 2017). For example, Palumbo et al. (2014) using network-based methods, identified “fight-club” nodes (genes with negatively correlated profiles) that may be relevant for the control of berry transition between development and ripening.
There are also online resources available that can help analyze omic data from V. vinifera. For example, VitisNet (Grimplet et al., 2009, 2012) offers manually annotated molecular networks (16,000 genes and 247 networks) where omics data can be loaded to visualize changes in the transcriptome, proteome and metabolome for a given experiment. VTCdb (Wong et al., 2013) is a gene co-expression database for V. vinifera that allows exploring transcription regulation. With more than 29,000 genes (95% of the predicted grapevine transcriptome) to query co-expression networks, VTCdb offers the possibility to analyze the transcriptional network of grapevine development, metabolism and stress response. VitisCyc (Naithani et al., 2014) is a grapevine metabolic pathway database that also allows omics data to be uploaded (transcriptome, proteome and metabolome) and to analyze changes in metabolic networks in each experiment. VESPUCCI (Moretto et al., 2016) is a manually annotated gene expression compendium exploratory tool that can be used to investigate grapevine’s gene expression patterns.
Epigenetics is the study of heritable phenotypes that occur through modifications that alter DNA activity without modifying its basic nucleotide structure (Feil and Fraga, 2012). Many epigenetic mechanisms, acting in an interactive and redundant fashion (Grant-Downton and Dickinson, 2005; Berger et al., 2009), have been described to date, with DNA methylation probably being the best-studied of all (Rapp and Wendel, 2005). DNA methylation affects chromatin condensation in a rapid and reversible manner (Grativol et al., 2012). In turn, the regional level of chromatin condensation affects the transcriptional state of nearby genomic features such as genes and transposable elements (Zhang et al., 2006). Global changes in DNA methylation associated to local environments can be analyze using a myriad of methods (Kurdyukov and Bullock, 2016). Bisulfite modification of genomic DNA combined with whole genome sequencing (BS-Seq) is the gold standard for methylation analysis because it can assess an entire methylome with single base resolution (Krueger et al., 2012). However, due to their lower cost, other approaches such as next generation sequencing following the capture of the methylated fraction of the genome or its fragmentation using methylation sensitive restriction enzymes (Bock et al., 2010; Li et al., 2010; Kitimu et al., 2015) are better suited to study large number of samples. Both generate quantitative and qualitative information of the methylation status of a reduced but significant representation of the total genome.
Environmental signals are one of the elements that can have a major effect in modifying the DNA methylation patterns leading to gene expression changes that ultimately affect the plant phenotype (Feil and Fraga, 2012). The idea that the environment could modify the epigenetic status, and these modifications passed to the offspring (Tricker et al., 2013) or maintained as epigenetic memory on long lived organisms (Latzel et al., 2016), has attracted attention from scientists studying mechanisms involved in adaptation to local environments (Consuegra and Rodríguez López, 2016) and how these could be used to enhance crop performance (Rodríguez López and Wilkinson, 2015). There are many reported examples of how the environment affects the epigenome in natural environments and how epigenetic variations in plant populations could help to overcome the lack of genetic diversity (Fonseca Lira-Medeiros et al., 2010; Verhoeven et al., 2010).
One of the most well-known examples in which the environment affects the phenotype through epigenetic modifications is vernalization (Feil and Fraga, 2012). Through this process, plants in temperate regions mitigate the deleterious effects of low winter temperatures on flower and fruit development by breaking dormancy only after having been exposed to a cold period (Kumar et al., 2016). Unusual environmental conditions during dormancy such as high winter temperatures have been shown to exert a negative effect on fruit quality and yield on perennial crops requiring a vernalization period (Sugiura et al., 2012). Recent work in apple shows how methylation and expression levels of key genes involved in flowering and fruit set are modified by the level of chill received during bud dormancy (Kumar et al., 2016), indicating that the environmentally induced changes observed in fruit quality could be regulated by DNA methylation.
Together these studies suggest that the environment can have a long lasting phenotypic effect in plants through epigenetic changes without the need for genetic variation, and that epigenetic mechanisms could be working as intermediaries between environmental variation and the plant genome, and in this way, potentially contributing to plant phenotypic plasticity. Moreover, this mechanism could give plant populations a way of adapting to the local growing conditions (Platt et al., 2015; González et al., 2016). However, to our knowledge, almost all epigenetic studies done in V. vinifera have focused on the identification of commercial clones (Imazio et al., 2002; Schellenbaum et al., 2008; Ocaña et al., 2013) and on the assessment of in vitro culture on the epigenome (Baránek et al., 2015), there is, therefore, a lack of information of how the environment affects a grapevine’s epigenome and to what extent this interaction affects fruit quality. Until now, there are no studies looking at the epigenome to understand the control of gene expression in V. vinifera and how environmental signals can change the regulation of metabolic pathways through epigenetic modifications. In our view, the inclusion of epigenomic data on the analysis of the terroir effect will not only increase the resolution of analysis but will also help us to understand the regulatory mechanisms behind the observed differences.
There is no doubt that the elements affecting grapevine growth and fruit composition are complex and multifarious. While the concept of terroir is widely discussed, the underlying mechanisms remain somewhat enigmatic. However, with the recent parallel development of omics technologies and of statistical approaches for their integration, we are reaching a point where it may be possible to overcome this challenge. The geographic delimitation of a terroir is the first challenge to overcome before its molecular characterization. This delimitation could be achieved 1. Empirically, based in the number of significantly different environmental subregions present in the study or/and 2. based on the traditionally defined wine regions. Moreover, the masking effect that environmental inter-annual variations can have over single year measurements demands the incorporation of data from multiple seasons to be able to determine terroirs with enough confidence. Ideally such seasons should be, from a weather perspective, variable within the range characteristic for the region of study to be able to capture its “normal terroir.”
Understanding how the genome, environment and viticulture practices interact to affect fruit quality will allow us the opportunity to implement agricultural practices aimed to obtain the desired fruit characteristics for every climate/cultivar combination (Jones and Davis, 2000), leading to more efficient use of resources and better management of vineyards. In addition, grape growers can maximize the terroir effect on the grapevine to highlight the uniqueness of their vineyards ultimately increasing their industrial competitiveness. We propose that the integration of multi-omic and environmental datasets will contribute to a better understanding of the drivers of the terroir effect in grapevine. Moreover, multiple dataset data integration will increase our understanding of the molecular mechanisms involved in the regulation of multifactorial genome by environment interactions. Finally, it is increasingly recognized that plants are involved in complex interactions their soil and epiphytic microbiomes, which can affect their phenotype (Mueller and Sachs, 2015). The ‘omics’ era gives us the ability to explore the nature and consequences of biotic/abiotic interactions and so, a future challenge will be to bring the concept of the holobiont (the plant host plus its microbiomes) into the analysis of terroir and its effect on grapevine growth and fruit composition.
PF, TC, CC, and CR designed and wrote the manuscript.
The authors declare that the research was conducted in the absence of any commercial or financial relationships that could be construed as a potential conflict of interest.
PF was supported by Australian Grape and Wine Authority (AGWA) and University of Adelaide Graduate Research Scholarships. CR is supported by a University of Adelaide Research Fellowship. This study was funded through a Pilot Program in Genomic applications in Agriculture and Environment Sectors jointly supported by the University of Adelaide and the Australian Genome Research Facility Ltd.
Acharjee, A., Kloosterman, B., Visser, R. G. F., and Maliepaard, C. (2016). Integration of multi-omics data for prediction of phenotypic traits using random forest. BMC Bioinformatics 17(Suppl. 5), 180. doi: 10.1186/s12859-016-1043-4
Aho, K. A. (2013). Foundational and Applied Statistics for Biologists Using R. Illustrated. Boca Raton, FL: CRC Press.
Anesi, A., Stocchero, M., Dal Santo, S., Commisso, M., Zenoni, S., Ceoldo, S., et al. (2015). Towards a scientific interpretation of the terroir concept: plasticity of the grape berry metabolome. BMC Plant Biol. 15, 191. doi: 10.1186/s12870-015-0584-4
Atanassov, I., Hvarleva, T., Rusanov, K., Tsvetkov, I., and Atanassov, A. (2009). Wine metabolite profiling: possible application in winemaking and grapevine breading in Bulgaria. Biotechnol. Biotechnol. Equip. 23, 1449–1452. doi: 10.2478/V10133-009-0011-9
Baránek, M., Èechová, J., Raddová, J., Holleinová, V., Ondrušíková, E., and Pidra, M. (2015). Dynamics and reversibility of the DNA methylation landscape of grapevine plants (Vitis vinifera) stressed by in vitro cultivation and thermotherapy. PLoS ONE 10:e0126638. doi: 10.1371/journal.pone.0126638
Berger, S. L., Kouzarides, T., Shiekhattar, R., and Shilatifard, A. (2009). An operational definition of epigenetics. Genes Dev. 23, 781–783. doi: 10.1101/gad.1787609
Bersanelli, M., Mosca, E., Remondini, D., Giampieri, E., Sala, C., Castellani, G., et al. (2016). Methods for the integration of multi-omics data: mathematical aspects. BMC Bioinformatics 17(Suppl. 2), S15. doi: 10.1186/s12859-015-0857-9
Bock, C., Tomazou, E. M., Brinkman, A. B., Müller, F., Simmer, F., Gu, H., et al. (2010). Quantitative comparison of genome-wide DNA methylation mapping technologies. Nat. Biotechnol. 28, 1106–1114. doi: 10.1038/nbt.1681
Bokulich, N. A., Collins, T. S., Masarweh, C., Allen, G., Heymann, H., Ebeler, S. E., et al. (2016). Associations among wine grape microbiome, metabolome, and fermentation behavior suggest microbial contribution to regional wine characteristics. MBio 7:e00631-16. doi: 10.1128/mBio.00631-16
Bouhaddani, S. E., Houwing-Duistermaat, J., Salo, P., Perola, M., Jongbloed, G., and Uh, H.-W. (2016). Evaluation of O2PLS in Omics data integration. BMC Bioinformatics 17(Suppl. 2), S11. doi: 10.1186/s12859-015-0854-z
Burns, K. N., Kluepfel, D. A., Strauss, S. L., Bokulich, N. A., Cantu, D., and Steenwerth, K. L. (2015). Vineyard soil bacterial diversity and composition revealed by 16S rRNA genes: differentiation by geographic features. Soil Biol. Biochem. 91, 232–247. doi: 10.1016/j.soilbio.2015.09.002
Bylesjö, M., Eriksson, D., Kusano, M., Moritz, T., and Trygg, J. (2007). Data integration in plant biology: the O2PLS method for combined modeling of transcript and metabolite data. Plant J. 52, 1181–1191. doi: 10.1111/j.1365-313X.2007.03293.x
Cevallos-Cevallos, J. M., Reyes-De-Corcuera, J. I., Etxeberria, E., Danyluk, M. D., and Rodrick, G. E. (2009). Metabolomic analysis in food science: a review. Trends Food Sci. Technol. 20, 557–566. doi: 10.1016/j.tifs.2009.07.002
Cheng, G., He, Y.-N., Yue, T.-X., Wang, J., and Zhang, Z.-W. (2014). Effects of climatic conditions and soil properties on Cabernet Sauvignon berry growth and anthocyanin profiles. Molecules 19, 13683–13703. doi: 10.3390/molecules190913683
Chun, H., and Keleş, S. (2010). Sparse partial least squares regression for simultaneous dimension reduction and variable selection. J. R. Stat. Soc. Ser. B. Stat. Methodol. 72, 3–25. doi: 10.1111/j.1467-9868.2009.00723.x
Conde, C., Silva, P., Fontes, N., Dias, A. C. P., Tavares, R. M., Sousa, M. J., et al. (2007). Biochemical changes throughout grape berry development and fruit and wine quality. Food 1, 1–22. doi: 10.1186/s12864-016-2660-z
Consuegra, S., and Rodríguez López, C. M. (2016). Epigenetic-induced alterations in sex-ratios in response to climate change: an epigenetic trap? Bioessays 38, 950–958. doi: 10.1002/bies.201600058
Cozzolino, D. (2016). Metabolomics in grape and wine: definition, current status and future prospects. Food Anal. Method 9, 2986–2997. doi: 10.1007/s12161-016-0502-x
Cutler, S. R., Rodriguez, P. L., Finkelstein, R. R., and Abrams, S. R. (2010). Abscisic acid: emergence of a core signaling network. Annu. Rev. Plant Biol. 61, 651–679. doi: 10.1146/annurev-arplant-042809-112122
Dal Santo, S., Fasoli, M., Negri, S., D’Incà, E., Vicenzi, N., Guzzo, F., et al. (2016). Plasticity of the berry ripening program in a white grape variety. Front. Plant Sci. 7:970. doi: 10.3389/fpls.2016.00970
Dal Santo, S., Tornielli, G. B., Zenoni, S., Fasoli, M., Farina, L., Anesi, A., et al. (2013). The plasticity of the grapevine berry transcriptome. Genome Biol. 14:r54. doi: 10.1186/gb-2013-14-6-r54
FAO (2012). FAOSTAT. Available at: http://faostat.fao.org/site/339/default.aspx [accessed August 3, 2016].
Feil, R., and Fraga, M. F. (2012). Epigenetics and the environment: emerging patterns and implications. Nat. Rev. Genet. 13, 97–109. doi: 10.1038/nrg3142
Fonseca Lira-Medeiros, C., Parisod, C., Fernandes, R. A., Mata, C. S., Cardoso, M. A., and Ferreira, P. C. (2010). Epigenetic variation in mangrove plants occurring in contrasting natural environment. PLoS ONE 5:e10326. doi: 10.1371/journal.pone.0010326
Fukushima, A., Kanaya, S., and Nishida, K. (2014). Integrated network analysis and effective tools in plant systems biology. Front. Plant Sci. 5:598. doi: 10.3389/fpls.2014.00598
Gambetta, J. M., Cozzolino, D., Bastian, S. E. P., and Jeffery, D. W. (2016). Towards the creation of a wine quality prediction index: correlation of Chardonnay juice and wine compositions from different regions and quality levels. Food Anal. Method 9, 2842–2855. doi: 10.1007/s12161-016-0467-9
Gambetta, J. M., Schmidtke, L. M., Wang, J., Cozzolino, D., Bastian, S. E. P., and Jeffery, D. W. (2017). Relating expert quality ratings of Australian chardonnay wines to volatile composition and production method. Am. J. Enol. Vitic. 68, 39–48. doi: 10.5344/ajev.2016.16058
Ghan, R., Van Sluyter, S. C., Hochberg, U., Degu, A., Hopper, D. W., Tillet, R. L., et al. (2015). Five omic technologies are concordant in differentiating the biochemical characteristics of the berries of five grapevine (Vitis vinifera L.) cultivars. BMC Genomics 16, 946. doi: 10.1186/s12864-015-2115-y
Gladstones, J. (2011). “Introduction and definition of terroir,” in Wine, Terroir and Climate Change, ed. M. Deves (Kent Town, SA: Wakefield Press), 1–4.
Gligorijević, V., and Pržulj, N. (2015). Methods for biological data integration: perspectives and challenges. J. R. Soc. Interface 12:112. doi: 10.1098/rsif.2015.0571
González, A. P., Chrtek, J., Dobrev, P. I., Dumalasová, V., Fehrer, J., Mráz, P., et al. (2016). Stress-induced memory alters growth of clonal offspring of white clover (Trifolium repens). Am. J. Bot. 103, 1567–1574. doi: 10.3732/ajb.1500526
Grant-Downton, R. T., and Dickinson, H. G. (2005). Epigenetics and its implications for plant biology. 1. The epigenetic network in plants. Ann. Bot. 96, 1143–1164. doi: 10.1093/aob/mci273
Grativol, C., Hemerly, A. S., and Ferreira, P. C. G. (2012). Genetic and epigenetic regulation of stress responses in natural plant populations. Biochim. Biophys. Acta 1819, 176–185. doi: 10.1016/j.bbagrm.2011.08.010
Grimplet, J., Cramer, G. R., Dickerson, J. A., Mathiason, K., Van Hemert, J., and Fennell, A. Y. (2009). VitisNet: “Omics” integration through grapevine molecular networks. PLoS ONE 4:e8365. doi: 10.1371/journal.pone.0008365
Grimplet, J., Van Hemert, J., Carbonell-Bejerano, P., Díaz-Riquelme, J., Dickerson, J., Fennell, A., et al. (2012). Comparative analysis of grapevine whole-genome gene predictions, functional annotation, categorization and integration of the predicted gene sequences. BMC Res. Notes 5:213. doi: 10.1186/1756-0500-5-213
Hale, W. G., Saunders, V. A., and Margham, J. P. (2005). Collins Dictionary of Biology, 3rd Edn. London: Collins.
Hong, Y.-S. (2011). NMR-based metabolomics in wine science. Magn. Reson. Chem. 49(Suppl. 1), S13–S21. doi: 10.1002/mrc.2832
Ibrahim, K. S., and Kumar, N. S. (2017). “Methods for microbiome analysis,” in Translational Bioinformatics and Its Application, eds D.-Q. Wei, Y. Ma, W. C. S. Cho, Q. Xu, and F. Zhou (Dordrecht: Springer), 269–298. doi: 10.1007/978-94-024-1045-7_12
Imazio, S., Labra, M., Grassi, F., Winfield, M., Bardini, M., and Scienza, A. (2002). Molecular tools for clone identification: the case of the grapevine cultivar ‘Traminer.’ Plant Breed. 121, 531–535. doi: 10.1046/j.1439-0523.2002.00762.x
Jones, G. V., and Davis, R. E. (2000). Climate influences on grapevine phenology, grape composition, and wine production and quality for Bordeaux, France. Am. J. Enol. Vitic. 51, 249–261.
Kirwan, G. M., Johansson, E., Kleemann, R., Verheij, E. R., Wheelock,ÅM., Goto, S., et al. (2012). Building multivariate systems biology models. Anal. Chem. 84, 7064–7071. doi: 10.1021/ac301269r
Kitimu, S. R., Taylor, J., March, T. J., Tairo, F., Wilkinson, M. J., and Rodríguez López, C. M. (2015). Meristem micropropagation of cassava (Manihot esculenta) evokes genome-wide changes in DNA methylation. Front. Plant Sci. 6:590. doi: 10.3389/fpls.2015.00590
Koyama, K., Sadamatsu, K., and Goto-Yamamoto, N. (2010). Abscisic acid stimulated ripening and gene expression in berry skins of the Cabernet Sauvignon grape. Funct. Integr. Genomics 10, 367–381. doi: 10.1007/s10142-009-0145-8
Krueger, F., Kreck, B., Franke, A., and Andrews, S. R. (2012). DNA methylome analysis using short bisulfite sequencing data. Nat. Methods 9, 145–151. doi: 10.1038/nmeth.1828
Kumar, G., Rattan, U. K., and Singh, A. K. (2016). Chilling-Mediated DNA methylation changes during dormancy and its release reveal the importance of epigenetic regulation during winter dormancy in apple (Malus × domestica Borkh.). PLoS ONE 11:e0149934. doi: 10.1371/journal.pone.0149934
Kurdyukov, S., and Bullock, M. (2016). DNA methylation analysis: choosing the right method. Biology 5:e3. doi: 10.3390/biology5010003
Latzel, V., Rendina González, A. P., and Rosenthal, J. (2016). Epigenetic memory as a basis for intelligent behavior in clonal plants. Front. Plant Sci. 7:1354. doi: 10.3389/fpls.2016.01354
Li, N., Ye, M., Li, Y., Yan, Z., Butcher, L. M., Sun, J., et al. (2010). Whole genome DNA methylation analysis based on high throughput sequencing technology. Methods 52, 203–212. doi: 10.1016/j.ymeth.2010.04.009
Meng, C., Kuster, B., Culhane, A. C., and Gholami, A. M. (2014). A multivariate approach to the integration of multi-omics datasets. BMC Bioinformatics 15:162. doi: 10.1186/1471-2105-15-162
Mizrachi, E., Verbeke, L., Christie, N., Fierro, A. C., Mansfield, S. D., Davis, M. F., et al. (2017). Network-based integration of systems genetics data reveals pathways associated with lignocellulosic biomass accumulation and processing. Proc. Natl. Acad. Sci. U.S.A. 114, 1195–1200. doi: 10.1073/pnas.1620119114
Moretto, M., Sonego, P., Pilati, S., Malacarne, G., Costantini, L., Grzeskowiak, L., et al. (2016). VESPUCCI: exploring patterns of gene expression in grapevine. Front. Plant Sci. 7:633. doi: 10.3389/fpls.2016.00633
Mueller, U. G., and Sachs, J. L. (2015). Engineering microbiomes to improve plant and animal health. Trends Microbiol. 23, 606–617. doi: 10.1016/j.tim.2015.07.009
Mulas, G., Galaffu, M. G., Pretti, L., Nieddu, G., Mercenaro, L., Tonelli, R., et al. (2011). NMR analysis of seven selections of Vermentino grape berry: metabolites composition and development. J. Agric. Food Chem. 59, 793–802. doi: 10.1021/jf103285f
Naithani, S., Raja, R., Waddell, E. N., Elser, J., Gouthu, S., Deluc, L. G., et al. (2014). VitisCyc: a metabolic pathway knowledgebase for grapevine (Vitis vinifera). Front. Plant Sci. 5:644. doi: 10.3389/fpls.2014.00644
Ocaña, J., Walter, B., and Schellenbaum, P. (2013). Stable MSAP markers for the distinction of Vitis vinifera cv Pinot noir clones. Mol. Biotechnol. 55, 236–248. doi: 10.1007/s12033-013-9675-3
Paim Pinto, D. L., Brancadoro, L., Dal Santo, S., De Lorenzis, G., Pezzotti, M., Meyers, B. C., et al. (2016). The influence of genotype and environment on small RNA profiles in grapevine berry. Front. Plant Sci. 7:1459. doi: 10.3389/fpls.2016.01459
Palumbo, M. C., Zenoni, S., Fasoli, M., Massonnet, M., Farina, L., Castiglione, F., et al. (2014). Integrated network analysis identifies fight-club nodes as a class of hubs encompassing key putative switch genes that induce major transcriptome reprogramming during grapevine development. Plant Cell 26, 4617–4635. doi: 10.1105/tpc.114.133710
Pang, H., Lin, A., Holford, M., Enerson, B. E., Lu, B., Lawton, M. P., et al. (2006). Pathway analysis using random forests classification and regression. Bioinformatics 22, 2028–2036. doi: 10.1093/bioinformatics/btl344
Panighel, A., De Rosso, M., Dalla Vedova, A., and Flamini, R. (2015). Putative identification of new p-coumaroyl glycoside flavonoids in grape by ultra-high performance liquid chromatography/high-resolution mass spectrometry. Rapid Commun. Mass Spectrom. 29, 357–366. doi: 10.1002/rcm.7115
Pereira, G. E., Gaudillere, J. P., van Leeuwen, C., Hilbert, G., Maucourt, M., Deborde, C., et al. (2006). 1H NMR metabolite fingerprints of grape berry: comparison of vintage and soil effects in Bordeaux grapevine growing areas. Anal. Chim. Acta 563, 346–352. doi: 10.1016/j.aca.2005.11.007
Pinto, C., Pinho, D., Sousa, S., Pinheiro, M., Egas, C., and Gomes, A. C. (2014). Unravelling the diversity of grapevine microbiome. PLoS ONE 9:e85622. doi: 10.1371/journal.pone.0085622
Platt, A., Gugger, P. F., Pellegrini, M., and Sork, V. L. (2015). Genome-wide signature of local adaptation linked to variable CpG methylation in oak populations. Mol. Ecol. 24, 3823–3830. doi: 10.1111/mec.13230
Qi, Y., Suhail, Y., Lin, Y.-Y., Boeke, J. D., and Bader, J. S. (2008). Finding friends and enemies in an enemies-only network: a graph diffusion kernel for predicting novel genetic interactions and co-complex membership from yeast genetic interactions. Genome Res. 18, 1991–2004. doi: 10.1101/gr.077693.108
Rajasundaram, D., Runavot, J.-L., Guo, X., Willats, W. G. T., Meulewaeter, F., and Selbig, J. (2014). Understanding the relationship between cotton fiber properties and non-cellulosic cell wall polysaccharides. PLoS ONE 9:e112168. doi: 10.1371/journal.pone.0112168
Rajasundaram, D., and Selbig, J. (2016). More effort - more results: recent advances in integrative “omics” data analysis. Curr. Opin. Plant Biol. 30, 57–61. doi: 10.1016/j.pbi.2015.12.010
Rapp, R. A., and Wendel, J. F. (2005). Epigenetics and plant evolution. New Phytol. 168, 81–91. doi: 10.1111/j.1469-8137.2005.01491.x
Ritchie, M. D., Holzinger, E. R., Li, R., Pendergrass, S. A., and Kim, D. (2015). Methods of integrating data to uncover genotype-phenotype interactions. Nat. Rev. Genet. 16, 85–97. doi: 10.1038/nrg3868
Robinson, A. L., Adams, D. O., Boss, P. K., Heymann, H., Solomon, P. S., and Trengove, R. D. (2012). Influence of geographic origin on the sensory characteristics and wine composition of Vitis vinifera cv. Cabernet Sauvignon wines from Australia. Am. J. Enol. Vitic. 63, 467–476. doi: 10.5344/ajev.2012.12023
Rodríguez López, C. M., and Wilkinson, M. J. (2015). Epi-fingerprinting and epi-interventions for improved crop production and food quality. Front. Plant Sci. 6:397. doi: 10.3389/fpls.2015.00397
Roullier-Gall, C., Lucio, M., Noret, L., Schmitt-Kopplin, P., and Gougeon, R. D. (2014). How subtle is the “terroir” effect? Chemistry-related signatures of two “climats de Bourgogne”. PLoS ONE 9:e97615. doi: 10.1371/journal.pone.0097615
Salvetti, E., Campanaro, S., Campedelli, I., Fracchetti, F., Gobbi, A., Tornielli, G. B., et al. (2016). Whole-Metagenome-Sequencing-Based community profiles of Vitis vinifera L. cv. Corvina berries withered in two post-harvest conditions. Front. Microbiol. 7:937. doi: 10.3389/fmicb.2016.00937
Schellenbaum, P., Mohler, V., Wenzel, G., and Walter, B. (2008). Variation in DNA methylation patterns of grapevine somaclones (Vitis vinifera L.). BMC Plant Biol. 8:78. doi: 10.1186/1471-2229-8-78
Smart, R. E., Robinson, J. B., Due, G. R., and Brien, C. J. (1985). Canopy microclimate modification for the cultivar Shiraz. I. Definition of canopy microclimate. Vitis 24, 17–24.
Son, H.-S., Hwang, G.-S., Kim, K. M., Ahn, H.-J., Park, W.-M., Van Den Berg, F., et al. (2009). Metabolomic studies on geographical grapes and their wines using 1H NMR analysis coupled with multivariate statistics. J. Agric. Food Chem. 57, 1481–1490. doi: 10.1021/jf803388w
Srivastava, V., Obudulu, O., Bygdell, J., Löfstedt, T., Rydén, P., Nilsson, R., et al. (2013). OnPLS integration of transcriptomic, proteomic and metabolomic data shows multi-level oxidative stress responses in the cambium of transgenic hipI- superoxide dismutase Populus plants. BMC Genomics 14:893. doi: 10.1186/1471-2164-14-893
Sugiura, T., Sumida, H., Yokoyama, S., and Ono, H. (2012). Overview of recent effects of global warming on agricultural production in Japan. Jpn. Agr. Res. Q. 46, 7–13. doi: 10.6090/jarq.46.7
Sun, R., He, F., Lan, Y., Xing, R., Liu, R., Pan, Q., et al. (2015). Transcriptome comparison of Cabernet Sauvignon grape berries from two regions with distinct climate. J. Plant Physiol. 178, 43–54. doi: 10.1016/j.jplph.2015.01.012
Tarr, P. T., Dreyer, M. L., Athanas, M., Shahgholi, M., Saarloos, K., and Second, T. P. (2013). A metabolomics based approach for understanding the influence of terroir in Vitis Vinifera L. Metabolomics 9, 170–177. doi: 10.1007/s11306-013-0497-x
Tobias, R. D. (1995). “An introduction to partial least squares regression,” in Proceedings of the Twentieth Annual SAS Users Group International Conference, (Cary, NC: SAS Institute Inc.), 1250–1257.
Tricker, P. J., López, C. M. R., Gibbings, G., Hadley, P., and Wilkinson, M. J. (2013). Transgenerational, dynamic methylation of stomata genes in response to low relative humidity. Int. J. Mol. Sci. 14, 6674–6689. doi: 10.3390/ijms14046674
Van Leeuwen, C., and Seguin, G. (2006). The concept of terroir in viticulture. J. Wine Res. 17, 1–10. doi: 10.1080/09571260600633135
Verhoeven, K. J. F., Jansen, J. J., van Dijk, P. J., and Biere, A. (2010). Stress-induced DNA methylation changes and their heritability in asexual dandelions. New Phytol. 185, 1108–1118. doi: 10.1111/j.1469-8137.2009.03121.x
Wanichthanarak, K., Fahrmann, J. F., and Grapov, D. (2015). Genomic, proteomic, and metabolomic data integration strategies. Biomark Insights 10(Suppl. 4), 1–6. doi: 10.4137/BMI.S29511
Wong, D. C. J., and Matus, J. T. (2017). Constructing integrated networks for identifying new secondary metabolic pathway regulators in grapevine: recent applications and future opportunities. Front. Plant Sci. 8:505. doi: 10.3389/fpls.2017.00505
Wong, D. C. J., Sweetman, C., Drew, D. P., and Ford, C. M. (2013). VTCdb: a gene co-expression database for the crop species Vitis vinifera (grapevine). BMC Genomics 14:882. doi: 10.1186/1471-2164-14-882
Zamboni, A., Di Carli, M., Guzzo, F., Stocchero, M., Zenoni, S., Ferrarini, A., et al. (2010). Identification of putative stage-specific grapevine berry biomarkers and omics data integration into networks. Plant Physiol. 154, 1439–1459. doi: 10.1104/pp.110.160275
Zerihun, A., McClymont, L., Lanyon, D., Goodwin, I., and Gibberd, M. (2015). Deconvoluting effects of vine and soil properties on grape berry composition. J. Sci. Food Agric. 95, 193–203. doi: 10.1002/jsfa.6705
Zhang, A. (2009). “Data fusion in the analysis of protein interaction networks,” in Protein Interaction Networks: Computational Analysis (New York, NY: Cambridge University Press), 243–257. Available at: http://assets.cambridge.org/97805218/88950/frontmatter/9780521888950_frontmatter.pdf
Keywords: multi-omics, environment, Vitis vinifera, data integration, epigenetics, transcriptomics, metabolomics
Citation: Fabres PJ, Collins C, Cavagnaro TR and Rodríguez López CM (2017) A Concise Review on Multi-Omics Data Integration for Terroir Analysis in Vitis vinifera. Front. Plant Sci. 8:1065. doi: 10.3389/fpls.2017.01065
Received: 08 February 2017; Accepted: 02 June 2017;
Published: 20 June 2017.
Edited by:
Giovanni Battista Tornielli, University of Verona, ItalyReviewed by:
Pablo Carbonell-Bejerano, Instituto de las Ciencias de la Vid y del Vino (ICVV), CSIC, SpainCopyright © 2017 Fabres, Collins, Cavagnaro and Rodríguez López. This is an open-access article distributed under the terms of the Creative Commons Attribution License (CC BY). The use, distribution or reproduction in other forums is permitted, provided the original author(s) or licensor are credited and that the original publication in this journal is cited, in accordance with accepted academic practice. No use, distribution or reproduction is permitted which does not comply with these terms.
*Correspondence: Carlos M. Rodríguez López, Y2FybG9zLnJvZHJpZ3VlemxvcGV6QGFkZWxhaWRlLmVkdS5hdQ==
Disclaimer: All claims expressed in this article are solely those of the authors and do not necessarily represent those of their affiliated organizations, or those of the publisher, the editors and the reviewers. Any product that may be evaluated in this article or claim that may be made by its manufacturer is not guaranteed or endorsed by the publisher.
Research integrity at Frontiers
Learn more about the work of our research integrity team to safeguard the quality of each article we publish.