- LIPM, Centre National de la Recherche Scientifique, Institut National de la Recherche Agronomique, Université de Toulouse, Castanet-Tolosan, France
The emergence and re-emergence of plant pathogenic microorganisms are processes that imply perturbations in both host and pathogen ecological niches. Global change is largely assumed to drive the emergence of new etiological agents by altering the equilibrium of the ecological habitats which in turn places hosts more in contact with pathogen reservoirs. In this context, the number of epidemics is expected to increase dramatically in the next coming decades both in wild and crop plants. Under these considerations, the identification of the genetic variants underlying natural variation of resistance is a pre-requisite to estimate the adaptive potential of wild plant populations and to develop new breeding resistant cultivars. On the other hand, the prediction of pathogen's genetic determinants underlying disease emergence can help to identify plant resistance alleles. In the genomic era, whole genome sequencing combined with the development of statistical methods led to the emergence of Genome Wide Association (GWA) mapping, a powerful tool for detecting genomic regions associated with natural variation of disease resistance in both wild and cultivated plants. However, GWA mapping has been less employed for the detection of genetic variants associated with pathogenicity in microbes. Here, we reviewed GWA studies performed either in plants or in pathogenic microorganisms (bacteria, fungi and oomycetes). In addition, we highlighted the benefits and caveats of the emerging joint GWA mapping approach that allows for the simultaneous identification of genes interacting between genomes of both partners. Finally, based on co-evolutionary processes in wild populations, we highlighted a phenotyping-free joint GWA mapping approach as a promising tool for describing the molecular landscape underlying plant - microbe interactions.
Introduction
In the last decade the World Health Organization (WHO) reported more than 300 newly infectious diseases that have emerged as threat for human (Jones et al., 2008). In the same trend, a conspicuous burst of plant diseases has been reported since the beginning of this century (Bartoli et al., 2016). Newly pathogenic microbes can spill over form reservoirs or newly variants of a pre-existing pathogen (Elena et al., 2011). Three main driving factors for Emerging Diseases (ED) occurrences are currently considered in both human and plant pathology: (i) genetic and biological factors both acting on the host and the etiological agent (i.e., changes in host susceptibility and microbial adaptation via genomic rearrangements), (ii) ecological and environmental factors (i.e., climate change, perturbations of the ecological niches of the pathogens and changes in host demography), (iii) social, political and economic factors (i.e., land use and international travel; Smolinski et al., 2003). Whatever the factor we consider the common line in ED is the evolutionary potential of microbes that through genetic changes can bypass the host defense system by spreading over new host populations. However, the evolutionary genetic change of the pathogen is not sufficient for the occurrence of ED and ecological perturbations such as habitat modifications are necessary for the newly pathogen to encounter its host. For example, agricultural practices can drastically alter the environment by offering routes for transmission of pathogens (Institute of Medicine, 2003).
As proposed by (Engering et al., 2013), ED caused by microbial agents can be divided in three major groups. The first disease emergence category concerns those pathogens that emerge in a novel host, a common process that is also called host jump. Pathogenic lines with flexible genomes (or high evolvability behavior) and with high environmental survival are much more prone for host jumps (Engering et al., 2013), although interspecies contact rate—that is burst by the ongoing growth in human population and consumption of animal products—is one of the main drivers for host jump occurrence. For example, the oomycete species Phytophthora infestans responsible for the Irish potato famine, experienced several host jumps because of the high rate of genomic rearrangements in non-coding genomic regions (Raffaele et al., 2010). A second type of disease emergence involves mutant pathogenic lines that caused more severe diseases after the acquisition of novel genetic traits. Novel pathogenicity traits can be the result of mutations or horizontal gene transfer and both processes increase pathogen's genetic variability (Bartoli et al., 2016). In light of this, the barley powdery mildew, Blumeria graminis, has increased its pathogenicity and has acquired an obligate biotrophy life-style via retrotransposon proliferation and gene loss (Spanu et al., 2010). However, the environment where the pathogenic populations evolve is of fundamental importance for triggering the evolution of new traits increasing aggressiveness in a pathogen. For example, the intensification of antibiotic utilization in agriculture increased antibiotic resistance gene acquisition as well as virulence in several bacterial pathogens (Jones et al., 2008). Examples are the Escherichia coli O157:H7 in which its aggressiveness increased after the acquisition of the Shiga toxin plasmid, or the rice fungal pathogen Cochiliobolus miyabeanus responsible of Great Bengal Famine of 1943 that gaining non-host specific toxins raised virulence on the rice host (Bruyne et al., 2016). However, in some cases, resistance to antibiotics can also naturally occur in pathogens without the selective pressure of the molecule. For example, in Pseudomonas viridiflava hypermutable variants, resistant to several antibiotics, spontaneously occur in synthetic media, as well in planta, in absence of antibiotics (Bartoli et al., 2015). Also, mutations CYP51 conferring azole resistance in different fungal pathogens is not always related to the utilization of fungicides as demonstrated in Fusarium spp (Parker et al., 2014). Lastly, the geographic expansion (or geographic jump) of a pathogen is also a form of disease emergence that can rapidly lead to disease epidemics (Engering et al., 2013). As already mentioned for host jumps, pathogens with more flexible genomes are obviously more prone to expand their geographic range because they can rapidly respond to the environmental conditions of a new habitat. However, human practices are also responsible for the expansion of pathogens' geographic area. In particular, multiple crop epidemics were caused by multiple introductions pathogen populations from restricted geographic regions, as reported for the worldwide spread of kiwifruit bacterial canker caused by the bacterium Pseudomonas syringae pv. actinidiae originating from China (Kim et al., 2016) and for P. infestans (Kamoun et al., 2015).
The challenge of understanding and predicting ED occurrences is even more relevant in the climate change context which is likely to favor conditions for pathogens' development and dispersal (Bergot et al., 2004; Garrett et al., 2006; Tylianakis et al., 2008). Consequently, climate change scenarios predict an increase in the number of epidemics in the next coming decades (Bergot et al., 2004; Evans et al., 2008). However, the relationships between climate change and ED occurrences is also related to the life histories and the infection processes of the pathogens. For example, pathogens living in highly fluctuating environments (such as epiphytes) should be more favored under a climatic change scenario than pathogens strictly adapted to more constant habitats (such as obligate endophytes). Taking into account the environmental drivers of the pathogen/host variability that is the central theme for ED occurrence, there is an increasing need to better identify and understand the genetic and molecular mechanisms underlying pathogen virulence and plant resistance in the ecological conditions where both pathogens and hosts evolve. To our opinion, this ecological genomics approach can favor the identification of novel, durable, and sustainable means to prevent crop diseases.
To date, traditional linkage mapping based on genetic map, has been achieved in the identification of the genetic basis underlying phenotypic variation in both plants and pathogenic microbes. Traditional linkage mapping refers to a diversity of experimental populations ranging from F2 populations to the more recently developed multiparent advanced generation intercross (MAGIC) lines (Kover et al., 2009). Because Recombinant Inbred Lines (RILs) are almost completely homozygous, they can be replicated within an experiment and/or among several environmental conditions, thereby making RIL families the most popular experiment populations for traditional linkage mapping, at least in plants (Bergelson and Roux, 2010). While few genetic markers are required for a complete genome scan, traditional linkage mapping presents several drawbacks including (i) coarse mapping, (ii) genetic diversity that is limited to the parental lines of the segregating populations, and (iii) the impossibility to distinguish between pleiotropic and physically close genes (Bergelson and Roux, 2010). To address those problems, the method of genome-wide association (GWA) mapping emerged through the recent development of next-generation sequencing (NGS) technologies. Taking advantage of recombination events that have accumulated over thousands of generations (Mitchell-Olds and Schmitt, 2006; Nordborg and Weigel, 2010), GWA mapping uses natural linkage disequilibrium (LD) to identify polymorphisms that are associated with phenotypic variation. Although its power is reduced to detect rare alleles or weak-effect alleles in GWA mapping populations, this issue is counterbalanced by the greater advantage of fine mapping (down to the gene level) and common alleles associated with phenotypic variation at the species level (Bergelson and Roux, 2010).
The aim of the present review is to summarize recent progresses on the identification of QTLs underlying plant-pathogen interactions, through the use of GWA mapping. We discussed the main experimental and methodological difficulties that need to be addressed in the future to obtain a thorough overview of the genetic architecture underlying plant-pathogen interactions. We also introduced the emerging joint GWA mapping approach that allows the simultaneous identification of intergenomic epistatic QTLs underlying the molecular landscape of plant-pathogen interactions. In this context, we highlighted the benefits of the joint GWA mapping approach, in particular when performed in absence of phenotyping.
GWA Studies of Pathogen Resistance in Plants
In this review, we only considered GWAS based on a substantial number of genetic markers covering the whole plant species genome, thereby allowing to obtain an unbiased picture of the genetic architecture driving disease resistance. Also, we focused on studies where plants were directly challenged with a pathogen species. We therefore not considered GWAS of autoactive hypersensitive response (Olukolu et al., 2014). Based on these criteria, we identified a total of 35 studies reporting the identification of genomic regions associated with natural variation of plant response to pathogen infection in 34 pathosystems (Table 1). Despite the limited number of GWAS of disease resistance, important observations can be still drawn. Firstly, although the first GWAS of disease resistance—on Arabidopsis thaliana interacting with the bacterial pathogen P. syringae—was published in 2005 (Aranzana et al., 2005), almost half of the GWAS (~48.6%) have been published in the last 2 years. The recent burst of GWAS is directly linked to the development of the NGS technologies that occurred in the last decade. NGS technologies provide sufficient numbers of genetic markers to fine-map genes underlying natural variation of complex traits (Bergelson and Roux, 2010). The 35 GWAS identified here used Single Nucleotide Polymorphisms (SNPs) as genetic markers for mapping, because of their high frequency across the genomes and the development of SNP-tilling arrays containing probe sets for tens (even hundreds) of thousands of SNPs. While SNP markers remain highly popular, they represent only a fraction of the available genetic polymorphisms. The access to structural variants such as copy number variation (CNV) and insertions-deletions (indels) is already facilitated by Single Molecule Real-Time (SMRT) sequencing technologies such as the Pacific Biosciences (PacBio) and Oxford Nanopore systems (Goodwin et al., 2016; Lee et al., 2016). In addition, because epigenetic variation can account for a non-negligible fraction (up to 30%) of the variation in complex traits (Roux et al., 2011), epigenome characterization at a single-base-pair resolution for hundreds of plant lines has already started for a limited number of plant species. For example, a high-quality single-base resolution genome-wide methylome was recently reported for 1,107 natural A. thaliana accessions (Kawakatsu et al., 2016). While combining different types of genomic markers with epigenomic diversity should help the access to causal variations and to tease apart the relative effect of genetic variants from epigenetic variants, the inclusion of additional information ever lead to an increase of false-positive associations between phenotype and polymorphic markers due to the problem of large dimensionality. The reduction of false-positive rate requests the development of statistical methods taking into account the diversity of polymorphic markers and the inherent kinships among plant lines, potentially reflecting different aspects of the demographic history of the plant species considered.
Secondly, the 35 plant GWAS are not evenly distributed across the three classes of pathogenic organisms considered in this review (Table 1). Two thirds of the GWAS reported the mapping of resistance QTLs to fungal pathogens, whereas the remaining GWAS were split between bacterial pathogens (n = 9) and oomycete pathogens (n = 6). The greater number of GWAS designed to identify genes decreasing the detrimental effect of fungal infection on plants is in line with fungal pathogens being the most widespread and rapidly spreading crop pathogens, despite their restricted host range in comparison with bacterial and oomycete pathogens (Bebber et al., 2014). Although plants are simultaneously and/or sequentially attacked by a range of pathogens, whether in natural environments or in crop fields (Kniskern et al., 2007; Davila Olivas et al., 2016; Roux and Bergelson, 2016), GWAS reporting genomic regions associated with plant responses to multiple pathogen attacks remain however scarce. In a first attempt, by measuring resistance to three fungal leaf diseases (i.e., southern leaf blight caused by Cochliobolus heterostrophus, gray leaf spot caused by Cercospora zeae-maydis (Czm) and northern leaf blight caused by Cercospora zeina) on 253 maize inbred lines genotyped for only 858 SNPs (i.e., 0.0015% SNP sites in maize), Wisser et al. (2011) performed a multivariate analysis and identified a glutathione S-transferase (GST) associated with modest levels of resistance to all three diseases. The recent development of statistical tools to perform multi-trait GWA mapping would undoubtedly facilitate the identification of the pleiotropic genetic determinants underlying multi-pathogen response (Korte et al., 2012; Thoen et al., 2016). In the era of metagenomics, describing multiple pathogen infections can be facilitated by the description of the pathobiota (defined as the complex of microorganisms with the potential to cause disease on the plant host; Kamada et al., 2013) based on high throughput sequencing of amplicons of housekeeping genes with deep taxonomic resolution (such as gyrB gene marker for bacteria), allowing to distinguish pathogenic from commensal Operational Taxonomic Units (OTUs) within a given microbe genus (Barret et al., 2015). A metagenomic approach can help to estimate the relative fraction of microbial pathogens and can also have the advantage of describing multiple pathogen infections at very early disease stages. Under these considerations, the next challenge in tracing the plant genomic regions associated with disease occurrence concerns the developed of GWAS on the whole pathobiota. While a recent GWA study reported the identification of genomic regions associated with descriptors (i.e., species richness, α-diversity, β-diversity, presence/absence of OTUs) of leaf microbial community in A. thaliana (Horton et al., 2014), to our knowledge, no GWAS on descriptors of pathobiota communities have been published so far. Altogether, mapping genomic regions associated with plant response to multiple pathogen infections can help to better elucidate the pathways that ultimately enable a plant to fine-tune its defense against different aggressors, thereby shedding some light on downstream components of the complex signaling network leading to resistance that cannot be revealed by classical mono-pathogen infection approaches.
Thirdly, the 35 GWAS involved only 11 plant species distributed across three botanical families, i.e., Brassicaceae (A. thaliana and Brassica napus), Fabaceae (Glycine max, Medicago sativa, Medicago truncatula, Pisum sativum) and Poaceae (Hordeum vulgare, Oryza sativa, Setaria italica, Sorghum bicolor, and Zea mays; Table 1). Because of the economic and environmental cost of crop pathogens, the majority of GWAS (~62.8%) was conducted on crop species to speed up the identification of new sources of disease resistance. The remaining GWAS were conducted on the two model plant species A. thaliana (~33%) and M. truncatula (~3%). Conducting GWAS on wild plant species can be a successful starting point to identify homologous genes in other species belonging or not to the same botanical family (Huard-chauveau et al., 2013). In addition, studying the spatial-temporal evolutionary dynamics of an adaptive resistance gene in an ecologically realistic context can help drawing new strategies for disease management in crops in an agro-ecological context (Bergelson et al., 2001; Barrett et al., 2009; Burdon et al., 2014; Karasov et al., 2014; Roux et al., 2014a).
Fourthly, a large variety of genetic lines, from natural accessions to elite breeding lines, were used for scoring disease resistance (Table 1). Noteworthy is the use of the combined advantages of both traditional QTL mapping and GWA mapping in a Nested Association Mapping (NAM) population in maize (for a total of ~4,000 RILs resulting from 25 crosses between diverse inbred lines and the reference line B73; Buckler et al., 2009) in four GWAS (Kump et al., 2011; Poland et al., 2011; Bian et al., 2014; Benson et al., 2015). Across the 35 GWAS, the number of genetic lines scored for disease resistance follows an L-shaped distribution with a median of ~196 lines (mean number ~817 lines, minimum number ~44 lines, maximum number ~7,431 lines; Table 1). Increasing genetic diversity remains the major goal in the design of a GWA mapping population and is mainly achieved by assembling lines collected over the entire geographic range of a plant species. Such panels may however increase the effect of population demographic history on the rate of false-positive phenotype-genotype associations (Zhao et al., 2007). Statistical methods controlling for population structure can drastically reduce the inflation of false-positive associations (Price et al., 2006, 2010; Kang et al., 2010; Zhang et al., 2010), but with the cost of increasing the rate of false-negative associations (i.e., when causative variants are lost after applying a correction for the effects of population structure; Brachi et al., 2010). In addition, because the same phenotype can be achieved by different combinations of genes, a higher genetic diversity increases the probability of genetic and/or allelic heterogeneity which may in turn limit the detection of polymorphic markers linked to phenotypic variation (Bergelson and Roux, 2010). Controlling the effects of genetic and allelic heterogeneity may be achieved by building panels of lines belonging to the same genetic cluster that is often geographically restricted. While of smaller size, those panels can lead to a higher power and resolution to fine-map loci associated with phenotypic variation. For example, more significant and neat association peaks for phenological variation in A. thaliana were found in set of 121 natural accessions collected in the region of Burgundy (France) than in a set a 167 worldwide natural accessions (Brachi et al., 2013). It remains to be tested whether a similar pattern is also observed for disease resistance.
Fifthly, the environmental conditions in which phenotyping of disease resistance was performed are well balanced between laboratory (greenhouse/growth chamber) controlled conditions (60%) and field conditions (40%) (Table 1), with only three GWAS performed both in controlled and/or field conditions (Chang et al., 2016; Desgroux et al., 2016; Rincker et al., 2016). Controlled and field conditions are complementary. In the field, plants are exposed to greater temporal abiotic fluctuations that may affect plant responses to pathogen invasions than are typically encountered in laboratory conditions. To limit the effects of those fluctuations, field experiments are repeated over several years. Despite recurrent observations of pathogen infections in natural populations, it is interesting to note that no GWAS was performed in the model plant species A. thaliana and M. truncatula in their local habitats. Adding ecology to the studies of “disease resistance—genotype” association can however help to better understand the evolutionary trajectories of a given adaptive resistance gene (Karasov et al., 2014; Roux and Bergelson, 2016). On the other hand, performing experiments in controlled conditions can help to test the effect of a specific abiotic stress on plant resistance to pathogen infection. This is especially relevant in the context of climate change where (i) the severity of epidemics is predicted to increase due to the shift and broadening of geographic distributions of pathogen species (Evans et al., 2008; Bebber et al., 2014), and (ii) a permanent increase in temperature was demonstrated to alleviate major known defense mechanisms in plants (Suzuki et al., 2014). In addressing this issue, Aoun and colleagues reported a GWAS performed on A. thaliana challenged with the bacterial pathogen Ralstonia solanacearum at 27 and 30°C (Aoun et al., in review). Based on traditional QTL mapping performed on one RIL family, the immune receptor pair of TIR-NBS-LRR proteins RESISTANT TO P. SYRINGAE 4 (RPS4)/RESISTANT TO R. SOLANACEARUM 1 (RRS1) has been map-based cloned and identified as the major genetic determinant conferring resistance to the R. solanacearum GMI1000 strain at 27°C (Deslandes et al., 2002). GWA mapping performed on 176 worldwide accessions of A. thaliana phenotyped at 27°C revealed a strong and unique association peak with the most associated SNP located within RPS4, suggesting that natural variation for resistance to the strain GMI1000 is also caused by RPS4/RRS1 at the species level (Aoun et al., in review). At 30°C, GWA mapping performed on the same set of accessions revealed multiple and smaller association peaks not located in the vicinity of RPS4/RRS1. Based on the phenotyping of T-DNA knockout mutants, the authors identified an enzyme encoding a strictosidine synthase (STRICTOSIDINE SYNTHASE-LIKE 4, SLL4) as underlying one of these small QTLs (Aoun et al., in review).
Sixthly, the first GWAS of disease resistance were performed in A. thaliana on natural variation of the hypersensitive response (HR), i.e., a highly effective local resistance response that is often associated with hypersensitive cell death (Table 1). This binary trait was useful to demonstrate the power of GWA mapping in plants because of previously known resistance genes underlying HR (Aranzana et al., 2005; Atwell et al., 2010). However, quantitative resistance (continuum of symptoms) is much more prevalent than qualitative resistance (presence/absence of symptoms) in crops and natural plant populations (Young, 1996; Poland et al., 2008; Roux et al., 2014a). Accordingly, recent GWAS performed in A. thaliana and M. truncatula and all GWAS performed in crops were based on a quantitative scoring of plant response to pathogen infections (but see Rincker et al., 2016; Table 1). The quantitative genetic architecture was highly diverse among pathosystems, ranging from the identification of few medium-effect QTLs to the identification of up to hundreds (and even thousands) of small-effect QTLs (Corwin et al., 2016). We should however be cautious on the complexity of the quantitative genetic architecture described in most GWAS because the number of QTLs identified might be highly dependent on the number of genetic lines used, the number of polymorphic markers genotyped and the accuracy in scoring the disease symptoms (Table 1). Because the development of NGS technologies will speed up the accumulation of genomic resources, the next frontier is high-throughput phenotyping of precise quantitative disease symptoms. This challenge can be achieved by the combination of the development of automated platforms (such as the Toulouse Plant Microbe Phenotyping Platform) updated by the International Plant Phenomics Network (IPPN) with the development of image-based quantification of disease symptoms (Laflamme et al., 2016).
Finally, the major goal of performing GWA mapping in crops is to accelerate the identification of genetic markers that can be subsequently used for Marker-Assisted Selection (MAS) in breeding programs. In this context, GWAS are rarely followed up by studies aiming at functionally validating the causative genes. To date, after identification of genomic regions linked to disease resistance by GWA mapping, genes responsible for the QTL of interest were functionally validated in only six GWAS (Table 1). Unsurprisingly, most functional validations were performed in A. thaliana due to the impressive genetic tools available in this species, such as the availability of several public collections of T-DNA mutants, quantitative complementation (i.e., introducing alternative alleles in genetic lines lacking the candidate gene) or quantitative knockdown (i.e., gene silencing by amiRNA; Bergelson and Roux, 2010). Functional validation helped (i) to identify gene functions that have never been related to defense against aggressors, as illustrated by the atypical kinase RKS1 gene and a gene of unknown function, both conferring quantitative resistance to the bacterial pathogen Xanthomonas campestris (Huard-chauveau et al., 2013; Roux et al., 2014b; Debieu et al., 2016), and (ii) to establish the selective forces acting on the causative gene (Huard-chauveau et al., 2013; Karasov et al., 2014). In a further step, both analyzing the transcriptional and/or post-transcriptional regulation of the causative gene and searching for proteins directly interacting with the causative gene can lead to the identification of the downstream signaling pathways, thereby providing an additional list of candidate genes for breeding programs.
Why So Few GWAS of Pathogenicity in Bacteria, Fungi and Oomycetes?
Similarly to plants, we only considered in this study GWAS based on a substantial number of genetic markers covering the whole genome of the pathogen species. As described above, GWA mapping started to be extensively used to fine map resistance genes in plants. By contrast, since the publication of the first plant pathogenic bacterium genome (Xylella fastidiosa) in 2000 (Simpson et al., 2000), the first plant pathogenic fungal species Magnaporthe grisea in 2005 (Dean et al., 2005) and the first oomycete species Phytophthora ramorum in 2006 (Tyler et al., 2006), as well the establishment of the Fungal Genome Initiative (FGI) (Galagan et al., 2005), only five studies employed GWA mapping to identify candidate pathogenic genetic determinants in bacterial (Monteil et al., 2017) and fungal pathogens (Dalman et al., 2013; Gao et al., 2016; Talas et al., 2016, Wu et al., 2017) and to our knowledge, no GWAS was reported yet on a phytopathogenic oomycete. Several explanations can be advanced for this paucity of studies. Firstly, comparative genomics has been proved as a very efficient tool to identify important pathogenic genetic determinants and to elucidate the mechanisms that microbial phytopathogens employ during pathogenesis (Klosterman et al., 2016). As recently summarized by Sundin et al. (2016), comparative genomics performed on an ever-growing number of phytopathogenic sequenced genomes was extremely useful for the discovery of type III secretion system (T3SS) effectors and transcription activator-like effector nuclease (TAL-effector) of the three most important studied plant pathogenic bacteria P. syringae, X. campestris and R. solanacearum (Mansfield et al., 2012). Though powerful, comparative genomics has also several limitations. Comparative genomics is a powerful tool to compare closely related epidemic strains showing few genomic differences but it can be hardly applied to “reservoir populations” carrying a high degree of diversity among them. In addition, comparative genomics is mainly employed to detect phenotypic differences that are based on the presence/absence of genes, thereby strongly limiting the potential for identifying SNPs associated with natural variation of virulence/aggressiveness among microbial pathogenic populations.
Secondly, despite the presence of several molecular mechanisms that can generate genetic variation in microbes (i.e., frequent mutation events, homologous recombination, and horizontal gene transfer; Bartoli et al., 2016), fine mapping in GWAS can be limited by the long LD observed in many pathogen species (Read and Massey, 2014). In human pathogenic microbes, long LD is mainly observed in pathogen species with a clonal reproduction system and/or in sets of epidemics strains with limited genetic diversity (Chen and Shapiro, 2015; Read and Massey, 2014). While to our knowledge no LD extent has been estimated in phytopathogenic bacteria and oomycetes, a rapid LD decay was observed in two phytopathogenic fungi, i.e., Fusarium graminearum (mean LD ~1 kb) and Parastagonospora nodorum (mean LD ~5–10 kb; Gao et al., 2016; Talas et al., 2016), thereby allowing fine mapping of pathogenic determinants (see below).
Thirdly, as previously observed in plants, genomic diversity of pathogenic microbes can be strongly shaped by population stratification (Power et al., 2017). Such population stratification is particularly encountered in haploid and asexual bacteria and/or pathogenic microbes with limited dispersal (Chen and Shapiro, 2015). Because population structure can impede the identification of genomic regions associated with virulence/aggressiveness in pathogenic microbes, various methods have been recently developed to limit the rate of false positives (Sheppard et al., 2013; Earle et al., 2016). When applied in the field of human pathology, these methods allowed the identification of genes related to host specificity in the Campylobacter human pathogen (Sheppard et al., 2013) and genes associated with antibiotic resistance in both Mycobacterium tubercolosis and Streptococcus bacterial species (Read and Massey, 2014).
To limit the negative effects of long LD and population structure on the identification of pathogenic genetic determinants, we advise the use of a local/ regional set of microbial strains collected on wild plant species. Such a strategy should increase the level of genetic diversity that is available in the natural plant reservoirs, while limiting the problem of population stratification.
If the last 4 years are documented by a speed in the increasing of studies employing GWAS to detect genes important for pathogenicity in human pathogens (Power et al., 2017), GWA mapping is still poorly used in plant pathology to identify genes related to microbial pathogenicity phenotypes. To our knowledge, the study from Monteil et al. (2017) is the only one that attempted to apply GWA mapping to a phytopathogenic bacterium. By using a GWA mapping method previously developed for human bacterial pathogens and that takes into account both core and pan-genome while controlling for population structure (Sheppard et al., 2013; Pascoe et al., 2015; Yahara et al., 2017), the authors found that the T3SS effectors hopQ1 and hopD1 have probably shaped the adaptation of the ubiquitous plant pathogenic bacterium P. syringae to crops. In plant-fungus pathosystems, we identified four studies reporting the identification of genetic determinants associated with virulence/aggressiveness. Dalman et al. (2013) adopted a GWA mapping approach to identify the genetic components underlying virulence in the fungal necrotrophic pathogen Heterobasidion annosum sensu stricto that is responsible of severe damages in forest conifers. Based on 23 haploid whole-genome sequenced H. annosum isolates collected in different geographic European countries, the authors used 33,018 non-singleton SNPs to run GWA mapping on virulence scored on both Scots pine and Norway spruce in controlled conditions. Although the size of the mapping population was limited, 12 SNPs were found to be significantly associated with virulence on both host plants. In the study of Talas et al. (2016), 119 isolates of the fungal pathogen F. graminearum collected in Germany were phenotyped for aggressiveness on wheat under field conditions in two locations over 2 years (Talas et al., 2016). Based on ~29,000 SNPs and a short LD of <1 kb, the authors finely mapped 50 SNPs significantly associated with aggressiveness. Interestingly, highly significant interactions between the isolates and the field phenotyping conditions suggested an environment-dependent genetic architecture of F. graminearum. In the study of Gao et al. (2016), 191 isolates of the fungal necrotrophic wheat pathogen P. nodorum were phenotyped for virulence on two wheat lines and genotyped for ~3,000 SNPs distributed across the genome as well as genetic markers in candidate genes. The identification of the two previous cloned effector genes SnToxA and SnTox3 confirmed the power of GWA mapping to fine map virulence factors in P. nodorum. In a recent study, Wu et al. (2017) used a combined method between comparative genomics and GWA mapping by using 20 newly sequenced isolates of Puccina triticina from Australia. Based on 306,474 SNPs, the authors identified a polygenic architecture corresponding to 302 genes harboring at least one SNP associated with leaf rust virulence on wheat.
All the studies mentioned above were performed in controlled conditions or in common gardens with conditions that strongly differ from the habitats where the pathogenic microbes co-evolved with their natural hosts. Therefore, one of the next challenges is to perform phenotyping of the pathogenic trait of interest in more ecologically realistic conditions. In addition, because phytopathogenic microbes can be controlled by several members of the microbiota (Roux and Bergelson, 2016), it would be worthwhile to identify the genetic determinants in the pathogen species that are involved in the arms races with microbiota. Finally, as previously advised for plants, functional validation remains the gold standard to test whether the candidate genes identified by GWA mapping truly confer the aggressive/virulent phenotype to the microbial pathogen under study.
A Joint GWA Mapping Approach to Draw a Picture of the Network of Genetic Interactions between Plants and Pathogens
Plant GWAS have been demonstrated to be successful in identifying genomic regions associated with disease resistance (Table 1), whereas microbial GWAS reporting genomic regions associated with pathogenicity are still in the starting blocks. Despite this increasing number of GWAS, it is interesting to note that GWA mapping has never been performed on the two counterparts of the plant pathosystem, either separately or jointly. Characterizing the molecular landscape of plant-pathogen interactions can considerably increase our knowledge on the co-evolutionary processes driving adaptive dynamics of plant species in plant communities (Allen et al., 2004; Karasov et al., 2014; Roux and Bergelson, 2016), thereby increasing our understanding and predictions on ED (Lambrechts, 2010; Roux et al., 2014a).
To study co-evolutionary quantitative genetics, the first step will require (i) estimating the respective contributions of plant and pathogen genetic variation to disease variation and (ii) estimating the heritability of plant × pathogen interactions () that can be calculated by dividing the phenotypic variance associated with “plant genotype—by—pathogen genotype” interactions by the total phenotypic variance across genotypes of both biotic partners (Roux and Bergelson, 2016). Because extensive phenotypic data sets are required for estimating the joint genetic effect of the plant and the pathogen, studies reporting heritability estimates of plant × pathogen interactions remains scarce in the scientific literature. This observation reinforces the need for the development of automated high-throughput phenotyping platforms.
The next step requires the characterization of the genetic architecture of plant-pathogen interactions; that is the number of intergenomic epistatic QTLs, their physical locations in their respective genomes and their corresponding effect (Figure 1). Quantitative genetic methods have been developed to identify host-by-pathogen QTL interactions (Wang et al., 2006; Yang et al., 2008). These traditional QTL mapping based methods may however not be adapted to genome scans on both interacting species. New statistical methods must be developed with the challenge of performing joint GWA mapping by taking into account simultaneously the information provided by the genome sequences of the plant and the pathogen (Roux and Bergelson, 2016). An additional challenge relies on the correction for the effects of population structure of both interacting partners, by including separately the additive polygenic random effects of the plant species and the pathogen species.
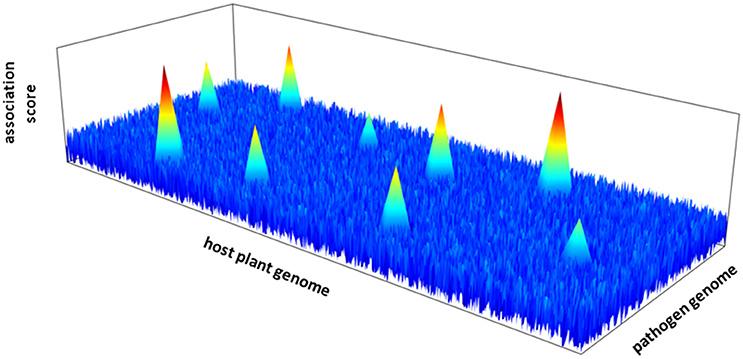
Figure 1. Hypothetical 3D-Manhattan plot of joint GWA mapping between the genome of a host plant and a pathogen species.
While technically challenging, identifying in both biotic partners the genes (and more precisely the causative variants) that confer the quantitative phenotypic variants likely retained by natural selection will help to understand and predict co-evolutionary dynamics between a host and its pathogen either in natural populations or in crop fields.
An Ecological Genomics Approach to Identify Natural Genetic Variants Driving the Interactions between Plants and Microbial Pathogens: A Free-Phenotyping Joint GWA Mapping Approach
Based on coevolution signatures in host and pathogen genomes, an innovative free-phenotyping strategy was recently developed for global genome-to-genome analysis and employed in the human-HIV pathosystem (Bartha et al., 2013). Using paired human and viral data from 1,071 individuals, HIV-1 sequence variants were used as “phenotypic” traits to finely map human genetic variants in interaction with viral genetic variants. The authors demonstrated that using HIV-1 sequence variants was much more powerful than viral load to finely map human SNPs in the major histocompatibility complex (MHC) region that was already reported as implicated in human-HIV coevolution. By adopting an ecological genomics approach, this strategy can also be applied in plant pathosystems. Here, we propose four steps to detect highly significant associations between plant DNA polymorphisms and pathogen sequence variation, without the need to obtain large phenotypic data sets (Figure 2). Firstly, plants are sampled across a given geographic area in conjunction with the corresponding microbiota. If the demographic history of the plant species has been reported, the sampling should be limited to genetically homogeneous subgroups, allowing a reduction of false positives due to population structure during the genome-to-genome statistical analysis. Secondly, our method requires the isolation of one representative strain (putative representative pathogen strain) on each plant individual. Traditional microbiological methods for strain isolation and identification can be time- and material-consuming. However, the systematic identification of a conspicuous number of microbial strains can be strongly facilitated by combining community-based culture collections (CBC), housekeeping gene amplicon pooling (16S or gyrB for bacteria, 18S for fungi, ITS for fungi and oomycetes) and NGS technologies (Armanhi et al., 2016). With ever-cheaper genome-sequencing SMRT methods, the third step consists in generating paired plant and pathogen genomic data. The fourth step consists in performing joint association analysis using both host and pathogen genomes, with the lofty goal of identifying genetic polymorphisms in strong LD across the two genomes. Similarly to joint GWA mapping approaches based on phenotypic data, new statistical methods must be developed for testing gene-gene interaction while accounting for population structure including interactions between the genetic backgrounds of the two organisms.
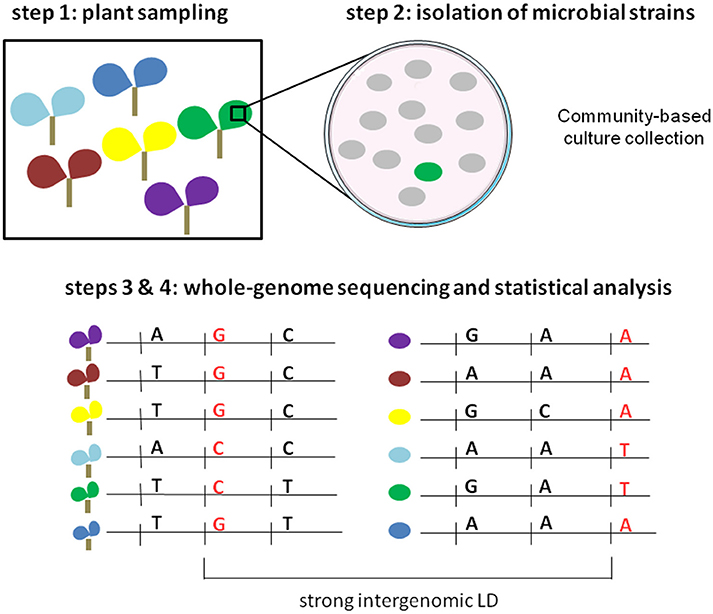
Figure 2. Illustration of the four steps of the free-phenotyping joint GWA mapping approach. Step 1: paired sampling of plants and microbiota in wild populations. Each color corresponds to a different plant population. Step 2: isolation of putative pathogenic strains. The green circle corresponds to the putative pathogenic strain whereas gray circles correspond to other members of the microbiota. Steps 3 and 4: whole-genome sequencing of both plants and microbial strains and genome-to-genome statistical analysis.
Based on co-evolutionary processes, combining paired plant, and pathogen genomic information represents therefore an exciting opportunity, especially in wild species, to describe the molecular landscape underlying plant–pathogen interactions.
Author Contributions
FR supervised the project. CB and FR wrote the manuscript.
Funding
This work was funded by the Région Midi-Pyrénées (CLIMARES project) and the LABEX TULIP (ANR-10-LABX-41, ANR-11-IDEX-0002-02).
Conflict of Interest Statement
The authors declare that the research was conducted in the absence of any commercial or financial relationships that could be construed as a potential conflict of interest.
Acknowledgments
We are grateful to colleagues from the Laboratory of Plant-Microbes Interactions for their helpful discussions.
References
Adeyanju, A., Little, C., Yu, J., and Tesso, T. (2015). Genome-wide association study on resistance to stalk rot diseases in grain sorghum. G3 16, 1165–1175. doi: 10.1534/g3.114.016394
Allen, R. L., Bittner-Eddy, P. D., Grenville-Briggs, L. J., Meitz, J. C., Rehmany, A. P., Rose, L. E., et al. (2004). Host-parasite coevolutionary conflict between Arabidopsis and downy mildew. Science 306, 1957–1960. doi: 10.1126/science.1104022
Aranzana, M. J., Kim, S., Zhao, K., Bakker, E., Horton, M., Jakob, K., et al. (2005). Genome-wide association mapping in Arabidopsis identifies previously known flowering time and pathogen resistance genes. PLoS Genet. 1:e60. doi: 10.1371/journal.pgen.0010060
Armanhi, J. S., de Souza, R. S., de Araújo, L. M., Okura, V. K., Mieczkowski, P., Imperial, J., et al. (2016). Multiplex amplicon sequencing for microbe identification in community-based culture collections. Sci. Rep. 6:29543. doi: 10.1038/srep29543
Atwell, S., Huang, Y. S., Vilhjalmsson, B. J., Willems, G., Horton, M., Li, Y., et al. (2010). Genome-wide association study of 107 phenotypes in Arabidopsis thaliana inbred lines. Nature 465, 627–631. doi: 10.1038/nature08800
Barret, M., Briand, M., Bonneau, S., Préveaux, A., Valière, S., Bouchez, O., et al. (2015). Emergence shapes the structure of the seed microbiota. Appl. Environ. Microbiol. 81, 1257–1266. doi: 10.1128/AEM.03722-14
Barrett, L. G., Kniskern, J. M., Bodenhausen, N., Zhang, W., and Bergelson, J. (2009). Continua of specificity and virulence in plant host—pathogen interactions: causes and consequences. New Phytol. 183, 513–529. doi: 10.1111/j.1469-8137.2009.02927.x
Bartha, I., Carlson, J. M., Brumme, C. J., McLaren, P. J., Brumme, Z. L., John, M., et al. (2013). A genome-to-genome analysis of associations between human genetic variation, HIV-1 sequence diversity, and viral control. Elife 2:e01123. doi: 10.7554/eLife.01123
Bartoli, C., Lamichhane, J.-R., Berge, O., Varvaro, L., and Morris, C. E. (2015). Mutability in Pseudomonas viridiflava as a programmed balance between antibiotic resistance and pathogenicity. Mol. Plant Pathol. 18, 860–869. doi: 10.1111/mpp.12243
Bartoli, C., Roux, F., and Lamichhane, J. R. (2016). Molecular mechanisms underlying the emergence of bacterial pathogens: an ecological perspective. Mol. Plant Pathol. 17, 303–310. doi: 10.1111/mpp.12284
Bastien, M., Sanah, H., and Belzile, F. (2014). Genome wide association mapping of Sclerotinia sclerotiorum resistance in soybean with a genotyping-by-sequencing approach. The Plant Gen. 7, 1–13. doi: 10.3835/plantgenome2013.10.0030
Bebber, D. P., Holmes, T., and Gurr, S. J. (2014). The global spread of crop pests and pathogens. Glob. Ecol. Biogeogr. 23, 1398–1407. doi: 10.1111/geb.12214
Benson, J. M., Poland, J. A., Benson, B. M., Stromberg, E. L., and Nelson, R. J. (2015). Resistance to lray leaf spot of maize: genetic architecture and mechanisms alucidated through nested association mapping and near-isogenic line analysis. PLoS Genet. 12, 1–23. doi: 10.1371/journal.pgen.1005045
Bergelson, J., Kreitman, M., Stahl, E. A., and Tian, D. (2001). Evolutionary dynamics of plant R-Genes. Plant Pathol. 292, 2281–2285. doi: 10.1126/science.1061337
Bergelson, J., and Roux, F. (2010). Towards identifying genes underlying ecologically relevant traits in Arabidopsis thaliana. Nat. Rev. Genet. 11, 867–879. doi: 10.1038/nrg2896
Bergot, M., Cloppet, E., Michel Déqué, V. P., Marçais, B., and Desperez-Loustau, M.-L. (2004). Simulation of potential range expansion of oak disease caused by Phytophthora cinnamomi under climate change. Glob. Chang. Biol. 10, 1539–1552. doi: 10.1111/j.1365-2486.2004.00824.x
Bian, Y., Yang, Q., Balint-kurti, P. J., Wisser, R. J., and Holland, J. B. (2014). Limits on the reproducibility of marker associations with southern leaf blight resistance in the maize nested association mapping population. BMC Genomics 15, 1–15. doi: 10.1186/1471-2164-15-1068
Bonhomme, M., André, O., Badis, Y., Ronfort, J., Burgarella, C., Chantret, N., et al. (2014). High-density genome-wide association mapping implicates an F-box encoding gene in Medicago truncatula resistance to Aphanomyces euteiches. New Phytol. 201, 1328–1342. doi: 10.1111/nph.12611
Brachi, B., Faure, N., Horton, M., Flahauw, E., Vazquez, A., Nordborg, M., et al. (2010). Linkage and association mapping of Arabidopsis thaliana flowering time in nature. PLoS Genet. 6, 1–17. doi: 10.1371/journal.pgen.1000940
Brachi, B., Villoutreix, R., Faure, N., Hautekèete, N., Piquot, Y., Pauwels, M., et al. (2013). Investigation of the geographical scale of adaptive phenological variation and its underlying genetics in Arabidopsis thaliana. Mol. Ecol. 22, 4222–4240. doi: 10.1111/mec.12396
Bruyne, L. D. E., Poucke, C. V. A. N., Jose, D., Mavungu, D. I., Ain, N. U. R., Mohd, I., et al. (2016). Comparative chemical screening and genetic analysis reveal tentoxin as a new virulence factor in Cochliobolus miyabeanus, the causal agent of brown spot disease on rice. Mol. Plant Pathol. 6, 805–817. doi: 10.1111/mpp.12329
Buckler, E. S., Holland, J. B., Bradbury, P. J., Acharya, C. B., Brown, P. J., Browne, C., et al. (2009). The genetic architecture of maize flowering time. Science 325, 714–718. doi: 10.1126/science.1174276
Burdon, J. J., Barrett, L. G., Rebetzke, G., and Thrall, P. H. (2014). Guiding deployment of resistance in cereals using evolutionary principles. Evol. Appl. 7, 609–624. doi: 10.1111/eva.12175
Chang, H. X., Lipka, A. E., Domier, L. L., and Hartman, G. L. (2016). Characterization of disease resistance loci in the USDA soybean germplasm collection using genome-wide association studies. Phytopathology 106, 1139–1151. doi: 10.1094/PHYTO-01-16-0042-FI
Chen, P. E., and Shapiro, B. J. (2015). The advent of genome-wide association studies for bacteria. Curr. Opin. Microbiol. 25, 17–24. doi: 10.1016/j.mib.2015.03.002
Corwin, J. A., Copeland, D., Feusier, J., and Subedy, A. (2016). The quantitative basis of the Arabidopsis innate immune system to endemic pathogens depends on pathogen genetics. PLoS Genet. 12:e1005789. doi: 10.1371/journal.pgen.1005789
Dalman, K., Himmelstrand, K., Olson, Å., Lind, M., Brandström-Durling, M., and Stenlid, J. (2013). A genome-wide association study identifies genomic regions for virulence in the non-model organism Heterobasidion annosum s.s. PLoS ONE 8:e53525. doi: 10.1371/journal.pone.0053525
Dean, R. A., Talbot, N. J., Ebbole, D. J., Farman, M. L., Mitchell, T. K., Orbach, M. J., et al. (2005). The genome sequence of the rice blast fungus Magnaporthe grisea. Nature 434, 980–986. doi: 10.1038/nature03449
Debieu, M., Huard-chauveau, C., Genissel, A., Roux, F., and Roby, D. (2016). Quantitative disease resistance to the bacterial pathogen Xanthomonas campestris involves an Arabidopsis immune receptor pair and a gene of unknown function. Mol. Plant Pathol. 17, 510–511. doi: 10.1111/mpp.12298
Desgroux, A., L'Anthoëne, V., Roux-duparque, M., Rivière, J., Aubert, G., Tayeh, N., et al. (2016). Genome-wide association mapping of partial resistance to Aphanomyces euteiches in pea. BMC Genomics 17:124. doi: 10.1186/s12864-016-2429-4
Deslandes, L., Olivier, J., Theulieres, F., Hirsch, J., Feng, D. X., Bittner-Eddy, P., et al. (2002). Resistance to Ralstonia solanacearum in Arabidopsis thaliana is conferred by the recessive RRS1-R gene, a member of a novel family of resistance genes. Proc. Natl. Acad. Sci. U.S.A. 99, 2404–2409. doi: 10.1073/pnas.032485099
Earle, S. G., Wu, C. H., Charlesworth, J., Stoesser, N., Gordon, N. C., Walker, T. M., et al. (2016). Identifying lineage effects when controlling for population structure improves power in bacterial association studies. Nat. Microbiol. 1:16041. doi: 10.1038/nmicrobiol.2016.41
Elena, S. F., Bedhomme, S., Carrasco, P., Cuevas, J. M., de la Iglesia, F., Lafforgue, G., et al. (2011). The Evolutionary genetics of emerging plant RNA viruses. Mol. Plant Microbe Interact. 24, 287–293. doi: 10.1094/MPMI-09-10-0214
Engering, A., Hogerwerf, L., and Slingenbergh, J. (2013). Pathogen-host-environment interplay and disease emergence. Emerg. Microbes Infect. 2:e5. doi: 10.1038/emi.2013.5
Evans, N., Baierl, A., Semenov, M. A., Gladders, P., and Fitt, B. D. L. (2008). Range and severity of a plant disease increased by global warming. J. R. Soc. Interface 5, 525–531. doi: 10.1098/rsif.2007.1136
Fopa Fomeju, B., Falentin, C., Lassalle, G., Maîtres-à-danser, M. J., and Delourme, R. (2014). Homoeologous duplicated regions are involved in quantitative resistance of Brassica napus to stem canker. BMC Genomics 15:498. doi: 10.1186/1471-2164-15-498
Galagan, J. E., Henn, M. R., Ma, L. J., and Cuomo, C. A. (2005). Genomics of the fungal kingdom : insights into eukaryotic biology. Genome Res. 15, 1620–1631. doi: 10.1101/gr.3767105
Gao, Y., Liu, Z., Faris, J. D., Richards, J., Brueggeman, R. S., Li, X., et al. (2016). Validation of genome-wide association studies as a tool to identify virulence factors in Parastagonospora nodorum. Phytopathology 106, 1177–1185. doi: 10.1094/PHYTO-02-16-0113-FI
Garrett, K. A., Dendy, S. P., Frank, E. E., Rouse, M. N., and Travers, S. E. (2006). Climate change effects on plant disease : genomes to ecosystems. Annu. Rev. Phytopathol. 44, 489–509. doi: 10.1146/annurev.phyto.44.070505.143420
Goodwin, S., McPherson, J. D., and McCombie, W. R. (2016). Coming of age: ten years of next-generation sequencing technologies. Nat. Rev. Genet. 17, 333–351. doi: 10.1038/nrg.2016.49
Horton, M. W., Bodenhausen, N., Beilsmith, K., Meng, D., Muegge, B. D., Subramanian, S., et al. (2014). Genome-wide association study of Arabidopsis thaliana leaf microbial community. Nat. Commun. 5, 5320. doi: 10.1038/ncomms6320
Huard-chauveau, C., Perchepied, L., Debieu, M., Rivas, S., Kroj, T., Kars, I., et al. (2013). An atypical kinase under balancing selection confers broad-spectrum disease resistance in Arabidopsis. PLoS Genet. 9:e1003766. doi: 10.1371/journal.pgen.1003766
Iakovidis, M., Teixeira, P. J. P. L., Exposito-Alonso, M., Cowper, M. G., Law, T. F., et al. (2016). Effector-triggered immune response in Arabidopsis thaliana is a quantitative trait. Genetics 204, 337–353. doi: 10.1534/genetics.116.190678
Institute of Medicine (2003). Microbial Threats to Health: Emergence, Detection and Response. Washington, DC: The National Academies Press.
Ji, H., Peng, Y., Meckes, N., Ellen, S., Stewart, C. N. Jr., and Traw, M. B. (2014). ATP-dependent binding cassette transporter G family member 16 increases plant tolerance to abscisic acid and assists in basal resistance against Pseudomonas syringae DC30001[W][OPEN]. Plant Physiol. 166, 879–888. doi: 10.1104/pp.114.248153
Jia, G., Huang, X., Zhi, H., Zhao, Y., Zhao, Q., and Li, W. (2013). A haplotype map of genomic variations and genome-wide association studies of agronomic traits in foxtail millet (Setaria italica). Nat. Genet. 45, 957–961. doi: 10.1038/ng.2673
Jones, K. E., Patel, N. G., Levy, M. A., Storeygard, A., Balk, D., Gittleman, J. L., et al. (2008). Global trends in emerging infectious diseases. Nature 451, 990–993. doi: 10.1038/nature06536
Kamada, N., Chen, G. Y., Inohara, N., and Nunez, G. (2013). Control of pathogens and pathobionts by the gut microbiota. Nat. Immunol. 14, 685–690. doi: 10.1038/ni.2608
Kamoun, S., Furzer, O., Jones, J. D. G., Judelson, H. S., Shad Ali, G., Dalio, R. J. D., et al. (2015). The top 10 oomycete pathogen in molecular plant pathology. Mol. Plant Pathol. 16, 413–434. doi: 10.1111/mpp.12190
Kang, H. M., Sul, J. H., Service, S. K., Zaitlen, N. A., Kong, S. Y., Freimer, N. B., et al. (2010). Variance component model to account for sample structure in genome-wide association studies. Nat. Genet. 42, 348–354. doi: 10.1038/ng.548
Kang, H., Wang, Y., Peng, S., Zhang, Y., Xiao, Y., Wang, D., et al. (2016). Dissection of the genetic architecture of rice resistance to the blast fungus Magnaporthe oryzae. Mol. Plant Pathol. 17, 959–972. doi: 10.1111/mpp.12340
Karasov, T. L., Kniskern, J. M., Gao, L., Deyoung, B. J., Ding, J., Dubiella, U., et al. (2014). The long-term maintenance of a resistance polymorphism through diffuse interactions. Nature 512, 436–440. doi: 10.1038/nature13439
Kawakatsu, T., Huang, S. C., Jupe, F., Weigel, D., Nordborg, M., Ecker, J. R., et al. (2016). Epigenomic diversity in a global collection of Arabidopsis thaliana accessions resource epigenomic diversity in a global collection of Arabidopsis thaliana accessions. Cell 166, 492–505. doi: 10.1016/j.cell.2016.06.044
Kim, G. H., Kim, K. H., Son, K. I., Choi, E. D., Lee, Y. S., and Jung, J. S. (2016). Outbreak and spread of bacterial canker of kiwifruit caused by Pseudomonas syringae pv. actinidiae biovar 3 in Korea. Plant Pathol. J. 32, 545–551. doi: 10.5423/PPJ.OA.05.2016.0122
Klosterman, S. J., Rollins, J. R., Sudarshana, M. R., and Vinatzer, B. A. (2016). Disease management in the genomics era—summaries of focus issue papers. Phytopathology 106, 1068–1070. doi: 10.1094/PHYTO-07-16-0276-FI
Kniskern, J. M., Traw, M. B., and Bergelson, J. (2007). Salicylic acid and jasmonic acid signaling defense pathways reduce natural bacterial diversity on Arabidopsis thaliana. Mol. Plant Microbe Interact. 12, 1512–1522. doi: 10.1094/MPMI-20-12-1512
Korte, A., Vilhjalmsson, B. J., Segura, V., Platt, A., Long, Q., and Nordborg, M. (2012). A mixed-model approach for genome-wide association studies of correlated traits in structured populations. Nat. Genet. 44, 1066–1071. doi: 10.1038/ng.2376
Kover, P. X., Valdar, W., Trakalo, J., Scarcelli, N., Ehrenreich, I. M., Michael, D., et al. (2009). A Multiparent advanced generation inter-cross to fine- map quantitative traits in Arabidopsis thaliana. PLoS Genet. 5:e1000551. doi: 10.1371/journal.pgen.1000551
Kump, K. L., Bradbury, P. J., Wisser, R. J., Buckler, E. S., Belcher, A. R., Oropeza-rosas, M. A., et al. (2011). Genome-wide association study of quantitative resistance to southern leaf blight in the maize nested association mapping population. Nat. Genet. 43, 163–168. doi: 10.1038/ng.747
Laflamme, B., Middleton, M., Lo, T., Desveaux, D., and Guttman, D. S. (2016). Image-based quantification of plant immunity and disease. Mol. Plant Microbe Interact. 29, 919–924. doi: 10.1094/MPMI-07-16-0129-TA
Lambrechts, L. (2010). Dissecting the genetic architecture of host – pathogen specificity. PLoS Pathog. 6:e1001019. doi: 10.1371/journal.ppat.1001019
Lee, H., Gurtowski, J., Yoo, S., Nattestad, M., Marcus, S., Goodwin, S., et al. (2016). Third-generation sequencing and the future of genomics. BioRxiv doi: 10.1101/048603
Mansfield, J., Genin, S., Magori, S., Citovsky, V., Sriariyanum, M., Ronald, P., et al. (2012). Top 10 plant pathogenic bacteria in molecular plant pathology. Mol. Plant Pathol. 13, 614–629. doi: 10.1111/j.1364-3703.2012.00804.x
Mitchell-Olds, T., and Schmitt, J. (2006). Genetic mechanisms and evolutionary significance of natural variation in Arabidopsis. Nature 441, 947–952. doi: 10.1038/nature04878
Monteil, C. L., Yahara, K., Studholme, D. J., Mageiros, L., Méric, G., Swingle, B., et al. (2017). Population-genomic insights into emergence, crop adaptation and dissemination of Pseudomonas syringae pathogens. Microb. Genom. 2:e000089. doi: 10.1099/mgen.0.000089
Nemri, A., Atwell, S., Tarone, A. M., Huang, Y. S., Zhao, K., Studholme, D. J., et al. (2010). Genome-wide survey of Arabidopsis natural variation in downy mildew resistance using combined association and linkage mapping. Proc. Natl. Acad. Sci. U.S.A. 107, 10302–10307. doi: 10.1073/pnas.0913160107
Nordborg, M., and Weigel, D. (2010). Next-generation genetics in plants. Nature 456, 10–13. doi: 10.1038/nature07629
Davila Olivas, N. H., Kruijer, W., Gort, G., Wijnen, C. L., van Loon, J. J. A., and Dicke, M. (2016). Genome-wide association analysis reveals distinct genetic architectures for single and combined stress responses in Arabidopsis thaliana. New Phytopathol. 213, 838–851. doi: 10.1111/nph.14165
Olukolu, B. A., Tracy, W. F., Wisser, R., De Vries, B., and Balint-Kurti, P. J. (2016). A genome-wide association study for partial resistance to maize common rust. Phytopathology 106, 745–751. doi: 10.1094/PHYTO-11-15-0305-R
Olukolu, B. A., Wang, G. F., Vontimitta, V., Venkata, B. P., Marla, S., Ji, J., et al. (2014). A Genome-Wide association study of the maize hypersensitive defense response identifies genes that cluster in related pathways. PLoS Genet. 10:e1004562. doi: 10.1371/journal.pgen.1004562
Parker, J. E., Warrilow, A. G., Price, C. L., Mullinis, J. G. L., Kelly, D. E., and Kelly, S. L. (2014). Resistance to antifungals that target CYP51. J. Chem. Biol. 7, 143–161. doi: 10.1007/s12154-014-0121-1
Pascoe, B., Méric, G., Murray, S., Yahara, K., Mageiros, L., Bowen, R., et al. (2015). Enhanced biofiml formation and multi-host transmission evolve from divergent genetic backgrounds in Campylobacter jejuni. Environ. Microbiol. 17, 4779–4789. doi: 10.1111/1462-2920.13051
Poland, J. A., Balint-kurti, P. J., Wisser, R. J., Pratt, R. C., and Nelson, R. J. (2008). Shades of gray : the world of quantitative disease resistance. Trends Plant Sci. 14, 21–29. doi: 10.1016/j.tplants.2008.10.006
Poland, J. A., Bradbury, P. J., Buckler, E. S., and Nelson, R. J. (2011). Genome-wide nested association mapping of quantitative resistance to northern leaf blight in maize. Proc. Natl. Acad. Sci. U.S.A. 108, 6893–6898. doi: 10.1073/pnas.1010894108
Power, R. A., Parkhill, J., and Oliveira, T. (2017). Microbial genome-wide association studies: lessons from human GWAS. Nat. Rev. Gen. 18, 41–50. doi: 10.1038/nrg.2016.132
Price, A. L., Patterson, N. J., Plenge, R. M., Weinblatt, M. E., Shadick, N. A., and Reich, D. (2006). Principal components analysis corrects for stratification in genome-wide association studies. Nat. Genet. 38, 904–909. doi: 10.1038/ng1847
Price, A. L., Zaitlen, N. A., Reich, D., and Patterson, N. (2010). New approaches to population stratification in genome-wide association studies. Nat. Rev. Genet. 11, 459–463. doi: 10.1038/nrg2813
Raffaele, S., Farrer, R. A., Cano, L. M., Studholme, D. J., MacLean, D., Thines, M., et al. (2010). Genome evolution following host jumps in the irish potato famine pathogen lineage. Science 330, 1540–1543. doi: 10.1126/science.1193070
Raman, H., Raman, R., Coombes, N., Song, J., Diffey, S., Kilian, A., et al. (2016). Genome-wide association study identifies new loci for resistance to Leptosphaeria maculans in canola. Front. Plant Sci. 7:1513. doi: 10.3389/fpls.2016.01513
Read, T. D., and Massey, R. C. (2014). Characterizing the genetic basis of bacterial phenotypes using genome-wide association studies : a new direction for bacteriology. Genome Med. 6, 1–11. doi: 10.1186/s13073-014-0109-z
Rincker, K., Lipka, A. E., and Diers, B. W. (2016). Genome-wide association study of brown stem rot resistance in soybean across multiple populations. Plant Genome 9, 1–11. doi: 10.3835/plantgenome2015.08.0064
Roux, F., and Bergelson, J. (2016). The Genetics underlying natural variation in the biotic interactions of Arabidopsis thaliana: the challenges of linking evolutionary genetics and community ecology. Curr. Top. Dev. Biol. 119, 111–156. doi: 10.1016/bs.ctdb.2016.03.001
Roux, F., Colomé-Tatché, M., Edelist, C., Wardenaar, R., Guerche, P., Hospital, F., et al. (2011). Genome-wide epigenetic perturbation jump-starts patterns of heritable variation found in nature. Genetics 188, 1015–1017. doi: 10.1534/genetics.111.128744
Roux, F., Voisin, D., Badet, T., Balagué, C., Barlet, X., Huard-chauveau, C., et al. (2014a). Resistance to phytopathogens e tutti quanti : placing plant quantitative disease resistance on the map. Mol. Plant Pathol. 15, 427–432. doi: 10.1111/mpp.12138
Roux, F., Noël, L., Rivas, S., and Roby, D. (2014b). ZRK atypical kinases: emerging signaling components of plant immunity. New Phytol. 203, 713–716. doi: 10.1111/nph.12841
Sheppard, S. F., Didelot, X., Meric, G., Torralbo, A., Jolley, K. A., Kelly, D. J., et al. (2013). Genome-wide association study identifies vitamin B5 biosynthesis as a host specificity factor in Campylobacter. Proc. Natl. Acad. Sci. U.S.A. 29, 11923–11927. doi: 10.1073/pnas.1305559110
Simpson, A. J., Reinach, F. C., Arruda, P., Abreu, F. A., Acencio, M., Alvarenga, R., et al. (2000). The genome sequence of the plant pathogen Xylella fastidiosa. Nature 406, 151–157. doi: 10.1038/35018003
Smolinski, M. S., Hamburg, M. A., and Ledeberg, J. (2003). Microbial Threats to Health: Emergence, Detection, and Response. Washington, DC: The National Academies Press.
Spanu, P. D., Abbott, J. C., Amselem, J., Burgis, T. A., Soanes, D. M., Stüber, K., et al. (2010). Genome expansion and gene loss in powdery mildew fungi reveal tradeoffs in extreme parasitism. Science 330, 1543–1546. doi: 10.1126/science.1194573
Sundin, G. W., Wang, N., Charkowski, A. O., Castiblanco, L. F., Jia, H., and Zhao, Y. (2016). Perspectives on the transition from bacterial phytopathogen genomics studies to applications enhancing disease management : from promise to practice. Phytopathology 106, 1071–1082. doi: 10.1094/PHYTO-03-16-0117-FI
Suzuki, N., Rivero, R. M., Shulaev, V., Blumwald, E., and Mittler, R. (2014). Abiotic and biotic stress combinations. New Phytol. 203, 32–43. doi: 10.1111/nph.12797
Talas, F., Kalih, R., Miedaner, T., and McDonald, B. A. (2016). Genome-wide association study identifies novel candidate genes for aggressiveness, deoxynivalenol production, and azole sensitivity in natural field populations of Fusarium graminearum. Mol. Plant-Microbe Interact. 29, 417–430. doi: 10.1094/MPMI-09-15-0218-R
Thoen, M. P., Olivas, N. H., Kloth, K. J., Coolen, S., Huang, P., Aarts, M. G., et al. (2016). Genetic architecture of plant stress resistance : multi-trait genome-wide association mapping. New Phytol. 213, 1346–1362. doi: 10.1111/nph.14220
Turuspekov, Y., Ormanbekova, D., Rsaliev, A., and Abugalieva, S. (2016). Genome-wide association study on stem rust resistance in Kazakh spring barley lines. BMC Plant Biol. 16:6. doi: 10.1186/s12870-015-0686-z
Tyler, B. M., Tripathy, S., Zhang, X., Dehal, P., Jiang, R. H., Aerts, A., et al. (2006). Phytophthora genome sequences uncover evolutionary origins and mechanisms of pathogenesis. Science 313, 1261–1266. doi: 10.1126/science.1128796
Tylianakis, J. M., Didham, K., Bascompte, J., and Wardle, D. A. (2008). Global change and species interactions in terrestrial ecosystems. Ecol. Lett. 11, 1351–1363. doi: 10.1111/j.1461-0248.2008.01250.x
Van Inghelandt, D., Melchinger, A. E., and Martinant, J.-P, Stich, B. (2012). Genome-wide association mapping of flowering time and northern corn leaf blight (Setosphaeria turcica) resistance in a vast commercial maize germplasm set. BMC Plant Biol. 12:56. doi: 10.1186/1471-2229-12-56
Wang, Z., Hou, W., and Wu, R. (2006). A statistical model to analyse quantitative trait locus interactions for HIV dynamics from the virus and human genomes. Stat. Med. 25, 495–511. doi: 10.1002/sim.2219
Wang, M., Yan, J., Zhao, J., Song, W., Zhang, X., Xiao, Y., et al. (2012). Genome-wide association study (GWAS) of resistance to head smut in maize. Plant Sci. 196, 125–131. doi: 10.1016/j.plantsci.2012.08.004
Wen, Z., Tan, R., Yuan, J., Bales, C., Du, W., Zhang, S., et al. (2014). Genome-wide association mapping of quantitative resistance to sudden death syndrome in soybean. BMC Genomics 15:809. doi: 10.1186/1471-2164-15-809
Wisser, R. J., Kolkman, J. M., Patzoldt, M. E., Holland, J. B., Yu, J., and Krakowsky, M. (2011). Multivariate analysis of maize disease resistances suggests a pleiotropic genetic basis and implicates a GST gene. Proc. Natl. Acad. Sci. U.S.A. 108, 7339–7344. doi: 10.1073/pnas.1011739108
Wu, J. Q., Sakthikumar, S., Dong, C., Zhang, P., Cuomon, C. A., and Park, R. F. (2017). Comparative genomics integrated with association analysis identifies candidate effector genes corresponding to Lr20 in phenotype-paires Puccina triticina isolates from Australia. Front. Plant Sci. 8:148. doi: 10.3389/fpls.2017.00148
Yahara, K., Méric, G., Taylor, A. J., de Vries, S. P. W., Murray, S., Pascoe, B., et al. (2017). Genome-wide association of functional traits linked with Campylobacter jejuni survival from farm to folk. Environ. Microbiol. 19, 361–380. doi: 10.1111/1462-2920.13628
Yang, J., Wu, W., and Zhu, J. (2008). Mapping interspecific genetic architecture in a host–parasite interaction system. Genetics 178, 1737–1743. doi: 10.1534/genetics.107.081430
Young, N. D. (1996). QTL mapping and quantitative disease resistance. Annu. Rev. Phytopathol. 34, 479–501. doi: 10.1146/annurev.phyto.34.1.479
Yu, L.-X., Zheng, P., Zhang, T., Rodrguez, J., and Main, D. (2016). Genotyping-by-sequencing-based genome-wide association studies on Verticillium wilt resistance in autotetraploid alfalfa (Medicago sativa L.). Mol. Plant Pathol. 18, 187–194. doi: 10.1111/mpp.12389
Zhang, Z., Ersoz, E., Lai, C. Q., Todhunter, R. J., Tiwari, H. K., Gore, M. A., et al. (2010). Mixed linear model approach adapted for genome-wide association studies. Nat. Genet. 42, 355–360. doi: 10.1038/ng.546
Zhang, J., Singh, A., Mueller, D. S., and Singh, A. K. (2015). Genome-wide association and epistasis studies unravel the genetic architecture of sudden death syndrome resistance in soybean. Plant J. 84, 1124–1136. doi: 10.1111/tpj.13069
Zhao, K., Aranzana, M. J., Kim, S., Lister, C., Shindo, C., Tang, C., et al. (2007). An Arabidopsis example of association mapping in structured samples. PLoS Genet. 3:e4. doi: 10.1371/journal.pgen.0030004
Zhao, K., Tung, C.-W., Eizenga, G. C., Wright, M. H., Ali, M. L., and Price, A. H. (2011). Genome-wide association mapping reveals a rich genetic architecture of complex traits in Oryza sativa. Nat. Comm. 2:467. doi: 10.1038/ncomms1467
Keywords: genome-wide association mapping, disease resistance, pathogenicity, crops, microbiota, pathobiota, genome-to-genome analysis, co-evolution
Citation: Bartoli C and Roux F (2017) Genome-Wide Association Studies In Plant Pathosystems: Toward an Ecological Genomics Approach. Front. Plant Sci. 8:763. doi: 10.3389/fpls.2017.00763
Received: 22 February 2017; Accepted: 24 April 2017;
Published: 23 May 2017.
Edited by:
Mahmut Tör, University of Worcester, United KingdomReviewed by:
Weixing Shan, Northwest A&F University, ChinaGuus Bakkeren, Agriculture & Agri-Food Canada, Canada
Copyright © 2017 Bartoli and Roux. This is an open-access article distributed under the terms of the Creative Commons Attribution License (CC BY). The use, distribution or reproduction in other forums is permitted, provided the original author(s) or licensor are credited and that the original publication in this journal is cited, in accordance with accepted academic practice. No use, distribution or reproduction is permitted which does not comply with these terms.
*Correspondence: Fabrice Roux, ZmFicmljZS5yb3V4QGlucmEuZnI=