- 1 Department of Agronomy, University of Wisconsin Madison, Madison, WI, USA
- 2 Department of Genetics, University of Wisconsin Madison, Madison, WI, USA
- 3 Department of Biochemistry, University of Wisconsin Madison, Madison, WI, USA
Understanding the interactions of plants with beneficial and pathogenic microbes is a promising avenue to improve crop productivity and agriculture sustainability. Proteomic techniques provide a unique angle to describe these intricate interactions and test hypotheses. The various approaches for proteomic analysis generally include protein/peptide separation and identification, but can also provide quantification and the characterization of post-translational modifications. In this review, we discuss how these techniques have been applied to the study of plant–microbe interactions. We also present some areas where this field of study would benefit from the utilization of newly developed methods that overcome previous limitations. Finally, we reinforce the need for expanding, integrating, and curating protein databases, as well as the benefits of combining protein-level datasets with those from genetic analyses and other high-throughput large-scale approaches for a systems-level view of plant–microbe interactions.
Introduction
Plants continually interact with fungi, bacteria, and viruses. Whereas defense pathways are often mounted in response to pathogens, they are tightly controlled to allow beneficial associations with symbionts (Figure 1). Several symbioses also require specific signaling pathways to accommodate microbes intracellularly (endosymbioses) and trigger, in some cases, the development of root nodules (Riely et al., 2004, 2006). The aim of studying plant–microbe interactions is to better understand these responses, their regulation, and their relatedness. Insights gained from such research should aid in engineering plants with improved pathogen resistance and novel symbiotic interactions. Proteomics, the characterization of a set of proteins under specific conditions, is a valuable tool for advancing plant biology (Wilkins et al., 1996). However, plant tissues provide significant technical challenges for proteomics, as they generally have large dynamic ranges of protein abundances (McCabe et al., 2001; Bindschedler and Cramer, 2011). Plant–microbe proteomics poses additional challenges, such as the need to differentiate between plant and microbial proteins (Mathesius, 2009). Herein, we review the use of proteomics for plant protein analysis, emphasizing applications to plant–microbe interaction research.
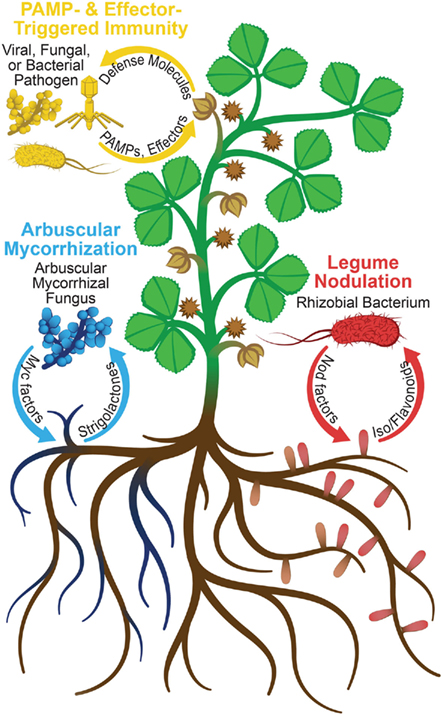
Figure 1. Signal exchanges between host plant and microbes. In legume nodulation and pathogenesis, respectively, Nod factors and PAMPs/effectors are secreted by the microbes in response to isoflavonoids and defense molecules secreted by the host plant. In arbuscular mycorrhization, the fungi secrete Myc factors, while the plant secretes strigolactones, causing hyphal branching of the fungus. Perception of microbial signals results in plant protein abundance and PTM changes, which can be detected using the proteomic techniques discussed herein.
Protein/Peptide Separation Techniques
Many protein and peptide separation methods have been developed, most being based on exploiting differences in size, charge, and/or hydrophobicity. A significant amount of information has been gained from proteomic studies using classical gel-based separation, as resolved proteins can often be identified and further characterized by mass spectrometry (MS). However, MS technologies are frequently more powerful for large-scale proteomics when combined with gel-free separation techniques (Roe and Griffin, 2006).
Gel-Based Separation
Two-dimensional gel electrophoresis (2-DE) involves resolving proteins by isoelectric point (pI) and molecular weight (Gorg et al., 2004; Kav et al., 2007). 2-DE has long been utilized for identifying plant protein abundance alterations in response to microbes and still remains a viable technique (Recorbet et al., 2010). Amiour et al. (2006) used 2-DE to analyze Glomus intraradices-induced proteome changes in wild-type Medicago truncatula plants in comparison with does not make infections 3 (dmi3) and supernumerary nodules (sunn) mutants. Proteins with roles in redox regulation and defense response exhibited differences in abundance upon infection in both mutants, with additional proteins changing specifically in the sunn mutant. Besides model plants like M. truncatula and Arabidopsis thaliana, proteomic studies using 2-DE have also been performed on major crops, including soybean, tobacco, and rice (Takahashi et al., 2003; Jones et al., 2004; Perez-Bueno et al., 2004; Subramanian et al., 2009).
Fluorescence difference gel electrophoresis (DIGE) was developed as a more quantitative form of 2-DE. Here, samples are differentially covalently labeled with fluorophores, allowing for distinction between proteins resolved on the same gel (Unlu et al., 1997; Hamdan and Righetti, 2002). This approach controls for gel-to-gel variation, but does not overcome the issues of spot overlap which confound quantification (Campostrini et al., 2005; Roe and Griffin, 2006). DIGE was, for instance, applied to compare antibody-mediated compatible plant–virus interactions using transgenic plant tissue, demonstrating the power of coupling proteomics with genetics for improved characterization of protein function. Here, proteomic comparison of challenged wild-type and transgenic cucumber mosaic virus-resistant tomato plants demonstrated that the coat protein-specific antibody present in the transgenic plants could hinder the spread of the virus to uninoculated tissues (Di Carli et al., 2010). Schenkluhn et al. (2010) used DIGE to investigate M. truncatula root proteomic responses to symbionts (G. intraradices and Sinorhizobium meliloti), both alone and combined with secondary pathogenic (Aphanomyces euteiches) infection. In mixed infections, no changes in pathogenesis-related (PR) proteins and decreased abundance of pathogen-induced proteins were observed, demonstrating that this symbiotic association provides some level of “bio-protection” to the host plant.
Gel-Free separation
While gel-based separation is still frequently utilized for certain proteomic applications, gel-free techniques for separating peptides after sequence-specific digestion have become standard for large-scale shotgun proteomics (Roe and Griffin, 2006). Most gel-free methods utilize two (2D LC) or more complementary dimensions of liquid chromatography, as extensive pre-fractionation of peptide mixtures significantly increases proteome coverage with MS-based peptide sequencing (Mallick and Kuster, 2010). Multidimensional protein identification technology (MudPIT), which combines strong cation exchange (SCX) with reversed phase (RP) chromatography, is perhaps the most well-known 2D LC technique, but many other complementary forms of peptide separation exist (Gilmore and Washburn, 2010). As opposed to MudPIT in its original implementation, however, SCX and other pre-fractionation techniques are now most commonly performed “offline” prior to LC-MS/MS (Gilmore and Washburn, 2010).
Several studies have demonstrated the power of gel-free proteomics for identifying protein changes in plant–microbe interaction systems. 2D LC-MS/MS was applied to identify 377 unique plant proteins in the nodules of M. truncatula plants inoculated with S. meliloti (Larrainzar et al., 2007). This general approach has also been applied to crops, including rice and pea (Saalbach et al., 2002; Ventelon-Debout et al., 2004; Zhou and Yang, 2006). 2-DE and 2D LC are often used together as complementary separation techniques. Caplan et al. (2009) combined 2D-DIGE with MudPIT to identify cytoplasmic and endoplasmic reticulum (ER)-associated molecular chaperones as differentially regulated in Nicotiana glutinosa upon infection with tobacco mosaic virus. Interestingly, these results were followed up with reverse genetics using virus-induced gene silencing (VIGS) to test the biological significance of altered levels of ER chaperones.
Protein Identification Using Mass Spectrometry
Mass spectrometry is the most common technique for unbiased protein identification and has been widely applied to plant–microbe proteomics (Mathesius et al., 2003). Protein/peptide ionization, ion separation, and detection are the three major steps for MS analysis (Mann et al., 2001). The major protein/peptide ionization techniques are matrix assisted laser desorption/ionization (MALDI) and electrospray ionization (ESI), while mass analyzers include time-of-flight (TOF), quadrupole, ion trap, orbitrap, and Fourier transform ion cyclotron resonance (FT-ICR; Mann et al., 2001; Chait, 2011). In tandem MS (MS/MS), precursor ions observed in an initial MS scan (MS1) are isolated and subjected to fragmentation, resulting in sequence-informative product ions that are detected in subsequent MS scans (MS2; Gygi and Aebersold, 2000). Fragmentation methods include collision-based (e.g., CAD, HCD) and electron-based (e.g., ECD, ETD) dissociation (Coon, 2009). Post-data acquisition, search algorithms (e.g., SEQUEST, Mascot, OMSSA) are used to compare observed ions against databases containing known protein sequences to identify peptides at some false discovery rate (FDR; 1% is standard; Elias and Gygi, 2007; Kav et al., 2007).
The advancement of MS technologies has enabled a recent surge in the field of plant–microbe proteomics. Just over half a decade ago, MALDI-TOF/TOF was used to compare the responses of wild-type M. truncatula with the ethylene-insensitive mutant sickle (skl; Prayitno et al., 2006). In this analysis, ethylene-inducible proteins were found to be altered between genotypes. While MALDI has unique applications such as tissue imaging, compatibility with online LC separation has made ESI the method of choice for integrating large-scale shotgun proteomics into a systems biology approach. Very recently, Salavati et al. (2011) combined nano-LC-MS/MS with transcriptomic analysis to investigate the initiation of symbiosis between soybean and Bradyrhizobium japonicum by comparing super-nodulating and non-nodulating varieties to wild-type plants. The results support the hypothesis that defense and signal transduction-related processes are negatively regulated in super-nodulating lines, correlating with a suppression of the auto-regulation of nodulation (AON) mechanism.
While current MS instrumentation allows for the collection of vast amounts of data, the importance of informatics in proteomics is especially evident for plant research, as the reliance on database searching becomes problematic when studying organisms without sequenced genomes. This hurdle can be overcome to a large extent by relying on homology, as has been demonstrated by identifying proteins from Araucaria angustifolia by searching against Oryza sativa, A. thaliana, and Solanum oleracea sequences using Mascot and MS BLAST (Junqueira et al., 2008).
Protein Quantification
Quantification can be applied to proteomics using either stable isotope labeling or label-free methods. In spectral counting, a popular label-free method, the number of peptide-associated MS/MS spectra are summed for each protein and statistical analyses are used to determine differential protein expression (Washburn et al., 2001). Spectral counting is effective, but is best-suited for detecting relatively large changes in moderately abundant proteins (Mallick and Kuster, 2010). Isotope-assisted quantification methods include in vitro chemical (e.g., ICAT, iTRAQ) or in vivo metabolic (e.g., SILAC, 15N-labeling) labeling of biological samples. In ICAT (isotope coded affinity tags), proteins are tagged with heavy and light isotopes using cysteine-modifying reagents, pooled, digested, and compared by MS (Gygi et al., 1999; Colas et al., 2010). In a more recently developed technique termed isobaric tagging, digested peptide samples are labeled on amines with commercially available iTRAQ (isobaric tags for relative and absolute quantification) or TMT (tandem mass tags) reagents (Thompson et al., 2003; Ross et al., 2004; Dunkley et al., 2006; Jones et al., 2006; Scherp et al., 2011). A major advantage of isobaric tagging is that this strategy can be applied to directly compare up to six (TMT) or eight (iTRAQ) separate samples in one experiment. With stable isotope labeling of amino acids in cell culture (SILAC), cells, or plants are grown on media supplemented with heavy isotope-containing amino acids, allowing for labeling of proteins as they are synthesized (Ong et al., 2002). Metabolic labeling of plants can also be performed using 15N, however this approach requires rigorous bioinformatics (McIlwain et al., 2008). When choosing a quantification method, other practical trade-offs to consider are that isotopic labeling experiments can be quite expensive, while label-free methods generally require significantly more instrument time.
The above techniques provide powerful tools for identifying proteome alterations of plants in response to microbes. Lee et al. (2009) quantified over 1,100 proteins from resistant and susceptible Phaseolus vulgaris plants post-infection using spectral counting, leading to the characterization of basal and R-gene-mediated plant defenses. Using iTRAQ-based quantitation, Kaffarnik et al. (2009) analyzed the secretome of A. thaliana in response to infection by Pseudomonas syringae. This work identified an alteration of extracellular proteins upon infection, suggesting a pathogen-mediated manipulation of host protein secretion. Keinath et al. (2010) employed 15N-labeling to reveal pathogen-associated molecular pattern (PAMP)-induced changes in detergent-resistant membranes of A. thaliana, identifying novel components of plant immunity. Future applications of isotope-assisted quantification to plant–microbe proteomics should reveal alterations in low-abundance membrane proteins and small changes probably missed with gel-based separation and label-free quantification.
Identification of PTMs
Post-translational modifications (PTMs) affect protein structure, activity, and stability, and play an important role in the regulation of a wide range of biological processes. While hundreds of PTMs have been described, only few have been analyzed using large-scale proteomic techniques, due to their reversible and labile nature, low stoichiometric abundances, and effects on protein digestion and ionization. These challenges can be addressed using selective enrichment and derivatization strategies. In this section, we focus primarily on phosphorylation since major advancements in high-throughput analyses of this PTM have allowed, over the last decade, significant insights into plant–microbe interactions.
Protein phosphorylation is a fundamental mechanism for signal transduction in which a phosphoryl group is transferred by a protein kinase to either a serine, threonine, tyrosine, or histidine residue (Benschop et al., 2007; Huber, 2007). While kinases phosphorylate proteins, phosphatases remove the phosphoryl group from them, allowing for reversible regulation. Upon perception of microbial signals, kinases, and phosphatases target specific proteins, often modifying complex signaling cascades that allow for rapid responses. A variety of proteomic techniques lend themselves to the identification of phosphorylated proteins and phosphorylation sites (Grimsrud et al., 2010a). 2-DE can be used to identify phosphorylated proteins, either by observing isoforms with lowered pI, by phospho-specific staining (e.g., ProQ® Diamond), 32P-labeling, or immunoblotting with phospho-specific antibodies (Bindschedler and Cramer, 2011; Bond et al., 2011). Due to the inherent difficulties with 2-DE, LC-MS/MS analysis following gel-free separation and phosphopeptide enrichment is often the method of choice for phosphoproteomics.
A number of proteomic studies have identified protein phosphorylation events involved in plant–microbe interactions. Gerber et al. (2006, 2008) used ProQ® Diamond staining followed by MALDI-TOF–TOF MS/MS to identify differentially phosphorylated Nicotiana tabacum proteins upon elicitation with lipopolysaccharides from Burkholderia cepacia. Trapphoff et al. (2009) combined immunoblotting and MS to identify phosphorylated proteins in defense-related pathways in M. truncatula cell suspension cultures after inoculation with A. euteiches. A recent study used 2-DE to separate 33P-labeled samples of Lotus japonicus roots treated with symbiotic- (Nod factors) and pathogen- (flg22) elicited signaling molecules, revealing phosphorylation of both unique and shared proteins during symbiotic and defense responses (Serna-Sanz et al., 2011). In our own work, we have identified thousands of sites of in vivo protein phosphorylation in M. truncatula roots by combining gel-free separation and phosphopeptide enrichment with nano-LC-MS/MS using multiple peptide fragmentation methods (Grimsrud et al., 2010b). We have created an online resource (http://www.phospho.medicago.wisc.edu) for sharing this and future M. truncatula phosphoproteomic data.
While most PTM analyses to date have focused on phosphorylation, improvements in tools for studying a number of other important PTMs hold promise for future analysis of plant–microbe signal transduction, including protein prenylation, S-nitrosylation, and ubiquitylation (Rodriguez-Concepcion et al., 1999; Ciechanover, 2005; Angel Torres, 2010; Jaspers and Kangasjarvi, 2010). These three PTMs, specifically, have all been implicated in plant innate immunity and defense and may be critical for responses to microbes (Romero-Puertas et al., 2004; Zeng et al., 2006; Goritschnig et al., 2008).
Prenylated and S-nitrosylated proteins can be identified using a biotin-switch method in which residues are biotinylated and then purified and subsequently identified using MS (Jaffrey and Snyder, 2001; Kho et al., 2004). Cheng et al. (2011) studied the response of soybean treated with lactofen, a soybean disease resistance-inducing herbicide. The authors analyzed LC-MS data using a custom bioinformatics program followed by 1D and 2D NMR, leading to the identification of six prenylated proteins never detected before in soybean. While the study of the redox proteome has not been widely applied to plant–microbe proteomics, Wang et al. (2009) used biotin-switch technology followed by MALDI-TOF to identify S-nitrosylated proteins in A. thaliana upon infection with P. syringae pv. tomato DC3000 (avrB), implicating S-nitrosylation of AtSABP3 as affecting plant immunity. MS is also well-suited for identifying ubiquitylated proteins, as tryptic digestion generates missed cleavages at modified lysines, which can be used for differential identification between samples (Bond et al., 2011). In addition, digestion of the ubiquitin protein itself leaves behind a di-glycine remnant on the modified lysine of target proteins, which can be utilized for immuno-affinity enrichment as well as a diagnostic in MS/MS spectra (Xu et al., 2010). Ubiquitylation in plants, however, is much less understood than in animals and only four large-scale studies have been published to date (Maor et al., 2007; Manzano et al., 2008; Igawa et al., 2009; Saracco et al., 2009). Future large-scale analyses of these and other PTMs should provide important insights into signal transduction mechanisms in plant–microbe interaction systems.
Future Perspectives on Plant–Microbe Proteomics
Although major advancements in plant–microbe proteomics have been made using model plants, there has been a recent trend to develop similar approaches in crops. Further insights into how these plants respond to symbiotic and pathogenic microorganisms will lead to improved agricultural practices, including proteomics-based fungicides (Fernandez Acero et al., 2011).
The biggest hindrance to proteomic application is the scarcity of sequence information and well-annotated protein databases. While techniques such as de novo sequencing and “proteogenomics” compensate for this deficiency, a significant need exists to expand and curate plant protein databases (Castellana et al., 2008). Many existing databases, including plant proteome database, plprot, post-translational modification database, Medicago phosphoprotein database, rice proteome database, and LegProt, should be expanded and integrated in the future (Komatsu et al., 2004; Kleffmann et al., 2006; Sun et al., 2009; Grimsrud et al., 2010b; Gnad et al., 2011; Lei et al., 2011). Proteomic analyses of plant–microbe interactions have provided better understanding of plant defense- and symbiosis-induced responses. However, the lack of published work using quantitative and in vivo proteomic techniques is striking. There is also a need for performing more biological and not just technical replicates in experiments that do utilize quantification. Furthermore, while most proteomic studies provide protein identification and functional predictions, the majority of them do not test their hypotheses using genetics, even when the tools are available. Coupling proteomic analyses with genetics and other omics approaches, while not yet widely applied, would strengthen the biological significance of many studies (Kint et al., 2010). A more systematic integration of these complementary approaches will provide useful information that will allow for better prediction and manipulation of plant responses to symbiotic and pathogenic microbes.
Conflict of Interest Statement
The authors declare that the research was conducted in the absence of any commercial or financial relationships that could be construed as a potential conflict of interest.
Acknowledgments
We acknowledge NSF-IOS grants #0701846 and #1021196 for funding support. We thank A. J. Bureta for figure preparation.
References
Amiour, N., Recorbet, G., Robert, F., Gianinazzi, S., and Dumas-Gaudot, E. (2006). Mutations in DMI3 and SUNN modify the appressorium-responsive root proteome in arbuscular mycorrhiza. Mol. Plant Microbe Interact. 19, 988–997.
Benschop, J. J., Mohammed, S., O’Flaherty, M., Heck, A. J., Slijper, M., and Menke, F. L. (2007). Quantitative phosphoproteomics of early elicitor signaling in Arabidopsis. Mol. Cell Proteomics 6, 1198–1214.
Bond, A. E., Row, P. E., and Dudley, E. (2011). Post-translation modification of proteins; methodologies and applications in plant sciences. Phytochemistry 72, 975–996.
Campostrini, N., Areces, L. B., Rappsilber, J., Pietrogrande, M. C., Dondi, F., Pastorino, F., Ponzoni, M., and Righetti, P. G. (2005). Spot overlapping in two-dimensional maps: a serious problem ignored for much too long. Proteomics 5, 2385–2395.
Caplan, J. L., Zhu, X., Mamillapalli, P., Marathe, R., Anandalakshmi, R., and Dinesh-Kumar, S. P. (2009). Induced ER chaperones regulate a receptor-like kinase to mediate antiviral innate immune response in plants. Cell Host Microbe 6, 457–469.
Castellana, N. E., Payne, S. H., Shen, Z., Stanke, M., Bafna, V., and Briggs, S. P. (2008). Discovery and revision of Arabidopsis genes by proteogenomics. Proc. Natl. Acad. Sci. U.S.A. 105, 21034–21038.
Cheng, J., Yuan, C., and Graham, T. L. (2011). Potential defense-related prenylated isoflavones in lactofen-induced soybean. Phytochemistry 72, 875–881.
Ciechanover, A. (2005). Proteolysis: from the lysosome to ubiquitin and the proteasome. Nat. Rev. Mol. Cell Biol. 6, 79–86.
Colas, I., Koroleva, O., and Shaw, P. J. (2010). Mass spectrometry in plant proteomic analysis. Plant Biosyst. 144, 703–714.
Coon, J. J. (2009). Collisions or electrons? Protein sequence analysis in the 21st century. Anal. Chem., 81, 3208–3215.
Di Carli, M., Villani, M. E., Bianco, L., Lombardi, R., Perrotta, G., Benvenuto, E., and Donini, M. (2010). Proteomic analysis of the plant-virus interaction in cucumber mosaic virus (CMV) resistant transgenic tomato. J. Proteome Res. 9, 5684–5697.
Dunkley, T. P., Hester, S., Shadforth, I. P., Runions, J., Weimar, T., Hanton, S. L., Griffin, J. L., Bessant, C., Brandizzi, F., Hawes, C., Watson, R. B., Dupree, P., and Lilley, K. S. (2006). Mapping the Arabidopsis organelle proteome. Proc. Natl. Acad. Sci. U.S.A. 103, 6518–6523.
Elias, J. E., and Gygi, S. P. (2007). Target-decoy search strategy for increased confidence in large-scale protein identifications by mass spectrometry. Nat. Methods 4, 207–214.
Fernandez Acero, F. J., Carbu, M., Rabie El-Akhal, M., Garrido, C., Gonzalez-Rodriguez, V. E., and Cantoral, J. M. (2011). Development of proteomics-based fungicides: new strategies for environmentally friendly control of fungal plant diseases. Int. J. Mol. Sci. 12, 795–816.
Gerber, I. B., Laukens, K., De Vijlder, T., Witters, E., and Dubery, I. A. (2008). Proteomic profiling of cellular targets of lipopolysaccharide-induced signalling in Nicotiana tabacum BY-2 cells. Biochim. Biophys. Acta 1784, 1750–1762.
Gerber, I. B., Laukens, K., Witters, E., and Dubery, I. A. (2006). Lipopolysaccharide-responsive phosphoproteins in Nicotiana tabacum cells. Plant Physiol. Biochem. 44, 369–379.
Gilmore, J. M., and Washburn, M. P. (2010). Advances in shotgun proteomics and the analysis of membrane proteomes. J. Proteomics 73, 2078–2091.
Gnad, F., Gunawardena, J., and Mann, M. (2011). PHOSIDA 2011: the posttranslational modification database. Nucleic Acids Res. 39, D253–D260.
Gorg, A., Weiss, W., and Dunn, M. J. (2004). Current two-dimensional electrophoresis technology for proteomics. Proteomics 4, 3665–3685.
Goritschnig, S., Weihmann, T., Zhang, Y., Fobert, P., McCourt, P., and Li, X. (2008). A novel role for protein farnesylation in plant innate immunity. Plant Physiol. 148, 348–357.
Grimsrud, P. A., Swaney, D. L., Wenger, C. D., Beauchene, N. A., and Coon, J. J. (2010a). Phosphoproteomics for the masses. ACS Chem. Biol. 5, 105–119.
Grimsrud, P. A., den Os, D., Wenger, C. D., Swaney, D. L., Schwartz, D., Sussman, M. R., Ane, J. M., and Coon, J. J. (2010b). Large-scale phosphoprotein analysis in Medicago truncatula roots provides insight into in vivo kinase activity in legumes. Plant Physiol. 152, 19–28.
Gygi, S. P., and Aebersold, R. (2000). Mass spectrometry and proteomics. Curr. Opin. Chem. Biol. 4, 489–494.
Gygi, S. P., Rist, B., Gerber, S. A., Turecek, F., Gelb, M. H., and Aebersold, R. (1999). Quantitative analysis of complex protein mixtures using isotope-coded affinity tags. Nat. Biotechnol. 17, 994–999.
Hamdan, M., and Righetti, P. G. (2002). Modern strategies for protein quantification in proteome analysis: advantages and limitations. Mass Spectrom. Rev. 21, 287–302.
Huber, S. C. (2007). Exploring the role of protein phosphorylation in plants: from signalling to metabolism. Biochem. Soc. Trans. 35(Pt 1), 28–32.
Igawa, T., Fujiwara, M., Takahashi, H., Sawasaki, T., Endo, Y., Seki, M., Shinozaki, K., Fukao, Y., and Yanagawa, Y. (2009). Isolation and identification of ubiquitin-related proteins from Arabidopsis seedlings. J. Exp. Bot. 60, 3067–3073.
Jaffrey, S. R., and Snyder, S. H. (2001). The biotin switch method for the detection of S-nitrosylated proteins. Sci. STKE 2001, pl1.
Jaspers, P., and Kangasjarvi, J. (2010). Reactive oxygen species in abiotic stress signaling. Physiol. Plant. 138, 405–413.
Jones, A. M., Bennett, M. H., Mansfield, J. W., and Grant, M. (2006). Analysis of the defence phosphoproteome of Arabidopsis thaliana using differential mass tagging. Proteomics 6, 4155–4165.
Jones, A. M., Thomas, V., Truman, B., Lilley, K., Mansfield, J., and Grant, M. (2004). Specific changes in the Arabidopsis proteome in response to bacterial challenge: differentiating basal and R-gene mediated resistance. Phytochemistry 65, 1805–1816.
Junqueira, M., Spirin, V., Balbuena, T. S., Thomas, H., Adzhubei, I., Sunyaev, S., and Shevchenko, A. (2008). Protein identification pipeline for the homology-driven proteomics. J. Proteomics 71, 346–356.
Kaffarnik, F. A., Jones, A. M., Rathjen, J. P., and Peck, S. C. (2009). Effector proteins of the bacterial pathogen Pseudomonas syringae alter the extracellular proteome of the host plant, Arabidopsis thaliana. Mol. Cell Proteomics 8, 145–156.
Kav, N., Srivastava, S., Yajima, W., and Sharma, N. (2007). Application of proteomics to investigate plant-microbe interactions. Curr. Proteomics 4, 28–43.
Keinath, N. F., Kierszniowska, S., Lorek, J., Bourdais, G., Kessler, S. A., Shimosato-Asano, H., Grossniklaus, U., Schulze, W. X., Robatzek, S., and Panstruga, R. (2010). PAMP (pathogen-associated molecular pattern)-induced changes in plasma membrane compartmentalization reveal novel components of plant immunity. J. Biol. Chem. 285, 39140–39149.
Kho, Y., Kim, S. C., Jiang, C., Barma, D., Kwon, S. W., Cheng, J. K., Jaunbergs, J., Weinbaum, C., Tamanoi, F., Falck, J., and Zhao, Y. M. (2004). A tagging-via-substrate technology for detection and proteomics of farnesylated proteins. Proc. Natl. Acad. Sci. U.S.A. 101, 12479–12484.
Kint, G., Fierro, C., Marchal, K., Vanderleyden, J., and De Keersmaecker, S. C. J. (2010). Integration of ‘omics’ data: does it lead to new insights into host-microbe interactions? Future Microbiol. 5, 313–328.
Kleffmann, T., Hirsch-Hoffmann, M., Gruissem, W., and Baginsky, S. (2006). Plprot: a comprehensive proteome database for different plastid types. Plant Cell Physiol. 47, 432–436.
Komatsu, S., Kojima, K., Suzuki, K., Ozaki, K., and Higo, K. (2004). Rice proteome database based on two-dimensional polyacrylamide gel electrophoresis: its status in 2003. Nucleic Acids Res. 32, D388–D392.
Larrainzar, E., Wienkoop, S., Weckwerth, W., Ladrera, R., Arrese-Igor, C., and Gonzalez, E. M. (2007). Medicago truncatula root nodule proteome analysis reveals differential plant and bacteroid responses to drought stress. Plant Physiol. 144, 1495–1507.
Lee, J., Feng, J., Campbell, K. B., Scheffler, B. E., Garrett, W. M., Thibivilliers, S., Stacey, G., Naiman, D. Q., Tucker, M. L., Pastor-Corrales, M. A., and Cooper, B. (2009). Quantitative proteomic analysis of bean plants infected by a virulent and avirulent obligate rust fungus. Mol. Cell. Proteomics 8, 19–31.
Lei, Z., Dai, X., Watson, B. S., Zhao, P. X., and Sumner, L. W. (2011). A legume specific protein database (LegProt) improves the number of identified peptides, confidence scores and overall protein identification success rates for legume proteomics. Phytochemistry 72, 1020–1027.
Mallick, P., and Kuster, B. (2010). Proteomics: a pragmatic perspective. Nat. Biotechnol. 28, 695–709.
Mann, M., Hendrickson, R. C., and Pandey, A. (2001). Analysis of proteins and proteomes by mass spectrometry. Annu. Rev. Biochem. 70, 437–473.
Manzano, C., Abraham, Z., Lopez-Torrejon, G., and Del Pozo, J. C. (2008). Identification of ubiquitinated proteins in Arabidopsis. Plant Mol. Biol. 68, 145–158.
Maor, R., Jones, A., Nuhse, T. S., Studholme, D. J., Peck, S. C., and Shirasu, K. (2007). Multidimensional protein identification technology (MudPIT) analysis of ubiquitinated proteins in plants. Mol. Cell. Proteomics 6, 601–610.
Mathesius, U. (2009). Comparative proteomic studies of root-microbe interactions. J. Proteomics 72, 353–366.
Mathesius, U., Imin, N., Natera, S. H., and Rolfe, B. G. (2003). Proteomics as a functional genomics tool. Methods Mol. Biol. 236, 395–414.
McCabe, M. S., Garratt, L. C., Schepers, F., Jordi, W. J., Stoopen, G. M., Davelaar, E., van Rhijn, J. H., Power, J. B., and Davey, M. R. (2001). Effects of P(SAG12)-IPT gene expression on development and senescence in transgenic lettuce. Plant Physiol. 127, 505–516.
McIlwain, S., Page, D., Huttlin, E. L., and Sussman, M. R. (2008). Matching isotopic distributions from metabolically labeled samples. Bioinformatics 24, i339–i347.
Ong, S. E., Blagoev, B., Kratchmarova, I., Kristensen, D. B., Steen, H., Pandey, A., and Mann, M. (2002). Stable isotope labeling by amino acids in cell culture, SILAC, as a simple and accurate approach to expression proteomics. Mol. Cell. Proteomics 1, 376–386.
Perez-Bueno, M. L., Rahoutei, J., Sajnani, C., Garcia-Luque, I., and Baron, M. (2004). Proteomic analysis of the oxygen-evolving complex of photosystem II under biotec stress: studies on Nicotiana benthamiana infected with tobamoviruses. Proteomics 4, 418–425.
Prayitno, J., Imin, N., Rolfe, B. G., and Mathesius, U. (2006). Identification of ethylene-mediated protein changes during nodulation in Medicago truncatula using proteome analysis. J. Proteome Res. 5, 3084–3095.
Recorbet, G., Valot, B., Robert, F., Gianinazzi-Pearson, V., and Dumas-Gaudot, E. (2010). Identification of in planta-expressed arbuscular mycorrhizal fungal proteins upon comparison of the root proteomes of Medicago truncatula colonised with two Glomus species. Fungal Genet. Biol. 47, 608–618.
Riely, B. K., Ané, J. M., Penmetsa, R. V., and Cook, D. R. (2004). Genetic and genomic analysis in model legumes bring nod-factor signaling to center stage. Curr. Opin. Plant Biol. 7, 408–413.
Riely, B. K., Mun, J. H., and Ané, J. M. (2006). Unravelling the molecular basis for symbiotic signal transduction in legumes. Mol. Plant Pathol. 7, 197–207.
Rodriguez-Concepcion, M., Yalovsky, S., Zik, M., Fromm, H., and Gruissem, W. (1999). The prenylation status of a novel plant calmodulin directs plasma membrane or nuclear localization of the protein. EMBO J. 18, 1996–2007.
Roe, M. R., and Griffin, T. J. (2006). Gel-free mass spectrometry-based high throughput proteomics: tools for studying biological response of proteins and proteomes. Proteomics 6, 4678–4687.
Romero-Puertas, M. C., Perazzolli, M., Zago, E. D., and Delledonne, M. (2004). Nitric oxide signalling functions in plant-pathogen interactions. Cell. Microbiol. 6, 795–803.
Ross, P. L., Huang, Y. N., Marchese, J. N., Williamson, B., Parker, K., Hattan, S., Khainovski, N., Pillai, S., Dey, S., Daniels, S., Purkayastha, S., Juhasz, P., Martin, S., Bartlet-Jones, M., He, F., Jacobson, A., and Pappin, D. J. (2004). Multiplexed protein quantitation in Saccharomyces cerevisiae using amine-reactive isobaric tagging reagents. Mol. Cell Proteomics 3, 1154–1169.
Saalbach, G., Erik, P., and Wienkoop, S. (2002). Characterisation by proteomics of peribacteroid space and peribacteroid membrane preparations from pea (Pisum sativum) symbiosomes. Proteomics 2, 325–337.
Salavati, A., Bushehri, A. A., Taleei, A., Hiraga, S., and Komatsu, S. (2011). A comparative proteomic analysis of the early response to compatible symbiotic bacteria in the roots of a supernodulating soybean variety. J. Proteomics 75, 819–832.
Saracco, S. A., Hansson, M., Scalf, M., Walker, J. M., Smith, L. M., and Vierstra, R. D. (2009). Tandem affinity purification and mass spectrometric analysis of ubiquitylated proteins in Arabidopsis. Plant J. 59, 344–358.
Schenkluhn, L., Hohnjec, N., Niehaus, K., Schmitz, U., and Colditz, F. (2010). Differential gel electrophoresis (DIGE) to quantitatively monitor early symbiosis- and pathogenesis-induced changes of the Medicago truncatula root proteome. J. Proteomics 73, 753–768.
Scherp, P., Ku, G., Coleman, L., and Kheterpal, I. (2011). Gel-based and gel-free proteomic technologies. Methods Mol. Biol. 702, 163–190.
Serna-Sanz, A., Parniske, M., and Peck, S. C. (2011). Phosphoproteome analysis of Lotus japonicus roots reveals shared and distinct components of symbiosis and defense. Mol. Plant Microbe Interact. 24, 932–937.
Subramanian, S., Cho, U., Keyes, C., and Yu, O. (2009). Distinct changes in soybean xylem sap proteome in response to pathogenic and symbiotic microbe interactions. BMC Plant Biol. 9, 119.
Sun, Q., Zybailov, B., Majeran, W., Friso, G., Olinares, P. D., and van Wijk, K. J. (2009). PPDB, the plant proteomics database at Cornell. Nucleic Acids Res. 37, D969–D974.
Takahashi, A., Kawasaki, T., Wong, H. L., Suharsono, U., Hirano, H., and Shimamoto, K. (2003). Hyperphosphorylation of a mitochondrial protein, prohibitin, is induced by calyculin A in a rice lesion-mimic mutant cdr1. Plant Physiol. 132, 1861–1869.
Thompson, A., Schafer, J., Kuhn, K., Kienle, S., Schwarz, J., Schmidt, G., Neumann, T., Johnstone, R., Mohammed, A. K., and Hamon, C. (2003). Tandem mass tags: a novel quantification strategy for comparative analysis of complex protein mixtures by MS/MS. Anal. Chem. 75, 1895–1904.
Trapphoff, T., Beutner, C., Niehaus, K., and Colditz, F. (2009). Induction of distinct defense-associated protein patterns in Aphanomyces euteiches (oomycota)-elicited and -inoculated Medicago truncatula cell-suspension cultures: a proteome and phosphoproteome approach. Mol. Plant Microbe Interact. 22, 421–436.
Unlu, M., Morgan, M. E., and Minden, J. S. (1997). Difference gel electrophoresis: a single gel method for detecting changes in protein extracts. Electrophoresis 18, 2071–2077.
Ventelon-Debout, M., Delalande, F., Brizard, J. P., Diemer, H., Van Dorsselaer, A., and Brugidou, C. (2004). Proteome analysis of cultivar-specific deregulations of Oryza sativa indica and O. sativa japonica cellular suspensions undergoing rice yellow mottle virus infection. Proteomics 4, 216–225.
Wang, Y., Feechan, A., Yun, B., Shafiei, R., Hofmann, A., Taylor, P., Xue, P., Yang, F., Xie, Z., Pallas, J. A., Chu, C., and Loake, G. J. (2009). S-nitrosylation of AtSABP3 antagonizes the expression of plant immunity. J. Biol. Chem. 284, 2131–2137.
Washburn, M. P., Wolters, D., and Yates, J. R. III. (2001). Large-scale analysis of the yeast proteome by multidimensional protein identification technology. Nat. Biotechnol. 19, 242–247.
Wilkins, M. R., Sanchez, J. C., Gooley, A. A., Appel, R. D., Humphery-Smith, I., Hochstrasser, D. F., and Williams, K. L. (1996). Progress with proteome projects: why all proteins expressed by a genome should be identified and how to do it. Biotechnol. Genet. Eng. Rev. 13, 19–50.
Xu, G., Paige, J. S., and Jaffrey, S. R. (2010). Global analysis of lysine ubiquitination by ubiquitin remnant immunoaffinity profiling. Nat. Biotechnol. 28, 868–873.
Zeng, L. R., Vega-Sanchez, M. E., Zhu, T., and Wang, G. L. (2006). Ubiquitination-mediated protein degradation and modification: an emerging theme in plant-microbe interactions. Cell Res. 16, 413–426.
Keywords: proteomics, plants, symbiosis, defense, signaling, microbes
Citation: Jayaraman D, Forshey KL, Grimsrud PA and Ané J-M (2012) Leveraging proteomics to understand plant–microbe interactions. Front. Plant Sci. 3:44. doi: 10.3389/fpls.2012.00044
Received: 20 January 2012; Paper pending published: 06 February 2012;
Accepted: 21 February 2012; Published online: 08 March 2012.
Edited by:
Jay Thelen, University of Missouri, USACopyright: © 2012 Jayaraman, Forshey, Grimsrud and Ané. This is an open-access article distributed under the terms of the Creative Commons Attribution Non Commercial License, which permits non-commercial use, distribution, and reproduction in other forums, provided the original authors and source are credited.
*Correspondence: Jean-Michel Ané, Department of Agronomy, University of Wisconsin Madison, 1575 Linden Drive, Madison, WI 53706, USA. e-mail:amFuZUB3aXNjLmVkdQ==