- Department of Biology, Brookhaven National Laboratory, Upton, NY, USA
Metabolic flux is a fundamental property of living organisms. In recent years, methods for measuring metabolic flux in plants on a network scale have evolved further. One major challenge in studying flux in plants is the complexity of the plant’s metabolism. In particular, in the presence of parallel pathways in multiple cellular compartments, the core of plant central metabolism constitutes a complex network. Hence, a common problem with the reliability of the contemporary results of 13C-Metabolic Flux Analysis in plants is the substantial reduction in complexity that must be included in the simulated networks; this omission partly is due to limitations in computational simulations. Here, I discuss recent emerging strategies that will better address these shortcomings.
Introduction
Isotopic tracers have different but important uses in metabolic research. Among the various approaches to stoichiometrical modeling of cell metabolism (Llaneras and Pico, 2008), 13C-Metabolic Flux Analysis (13C-MFA) is a method that combines a knowledge of cell metabolism with 13C-tracer experiments to analyze the in vivo flux distribution in the network of central cellular primary metabolism. It affords us a quantitative integrated view of core metabolism (Koschutzki et al., 2010) that unravels the in vivo function of biochemical pathways under different physiological conditions, or reveals the effect of manipulation by transgenic approaches. In plants, 13C-MFA mostly is applied to cultures of cells or tissue, growing heterotrophically or photoheterotrophically on 13C-labeled substrates. The increasing number of studies on different species over the last 10- to 15-years documents the development of researches with 13C-MFA in plants. Maize root tips, detached from germinating seeds, were first used as a model to study energy metabolism in non-photosynthetic tissues (Dieuaide-Noubhani et al., 1995; Alonso et al., 2005, 2007b,c). Other studies used the hairy root cultures of Catharanthus roseus, the Madagascar periwinkle (Sriram et al., 2007), and cell-suspension cultures of tomato or Arabidopsis thaliana (Rontein et al., 2002; Williams et al., 2008; Masakapalli et al., 2010). Various studies focused on the distribution of flux in central metabolism in the developing seeds and embryos of rapeseed and Arabidopsis (Schwender and Ohlrogge, 2002; Schwender et al., 2003, 2004a, 2006; Junker et al., 2007; Lonien and Schwender, 2009), soybean (Sriram et al., 2004; Iyer et al., 2008; Allen et al., 2009b), sunflower (Alonso et al., 2007a), and in developing maize endosperm or embryos (Ettenhuber et al., 2005; Spielbauer et al., 2006; Alonso et al., 2010, 2011). Several studies also began to assess the effect of physiological- or genotypical-perturbations of central metabolism (Alonso et al., 2007b; Junker et al., 2007; Iyer et al., 2008; Williams et al., 2008; Lonien and Schwender, 2009). Recent studies began to explore the synergy between plant 13C-MFA and the more predictive modeling approach of flux balance analysis (FBA; Williams et al., 2010; Hay and Schwender, 2011a,b).
A great deal of biological knowledge about an organism is needed to construct a model of its biochemical network. Even in the post-genomic age, the definition of metabolic networks is not straightforward (Sweetlove et al., 2008). Yet the results of the analytic process critically depend upon having a realistic network (van Winden et al., 2001a; Schwender et al., 2004b; Masakapalli et al., 2010). Due to the practices of computational analysis, the typical scale of a 13C-MFA network (Table 1) results from tailoring to a smaller size the detailed topology inferred from literature, e.g., by lumping the metabolite pools present in different subcellular compartments.
This paper offers some insights into the experimental- and computational-modeling practices of 13C-MFA to highlight the typical assumptions built into such models, and to discuss how their constructions and their general reliability can be improved. I discuss modeling related to applying 13CFLUX (Wiechert et al., 2001; www.13cflux.net), a software used by many groups in the field. The paper is not intended to be a comprehensive review of all recent work, but rather, to give my personal perspective based on the practice of experimental- and computational- modeling of plant central metabolism.
Principle of Steady-State 13C-MFA
Several recent detailed reviews summarize experimental procedures, the modeling process, as well as discuss important biological insights that have resulted from plant 13C-MFA studies, e.g., Libourel and Shachar-Hill (2008), Schwender (2008), Kruger and Ratcliffe (2009), Allen et al. (2009a), and Schwender (2009). Figure 1 illustrates a general experimental workflow. Zamboni et al. (2009) gave a very detailed and useful description of 13C-MFA, including a tutorial for 13CFLUX. In short, an organism is grown on a minimal culture medium with well-defined composition of organic- and inorganic-substrates. While a 13C-labeled carbon source (e.g., [1-13C]glucose) is being metabolized, 12C- and 13C-atoms are distributed throughout the organisms’ metabolic network. The fate of a 13C-labeled carbon position of the carbon source, or of pairs of adjacent 13C-atoms (13C–13C bond label) is traced through the network by detecting the labeling signatures of the intermediary metabolites by the techniques of mass spectrometry (MS; Dauner and Sauer, 2000; Schwender and Ohlrogge, 2002) or nuclear magnetic resonance (NMR; Dieuaide-Noubhani et al., 1995; Szyperski, 1995). For the widely used approach of metabolic- and isotopic-stationary 13C-MFA, the essential prerequisite is that the labeling state of each metabolite attains a steady-state before the cells are harvested, the metabolites extracted, and the labeling signatures analyzed (Wiechert, 2001). Thus, information is gained about intracellular fluxes for alternative pathways converging to the same metabolite (Szyperski, 1995; van Winden et al., 2001b), meaning that different labeling signatures are generated and mixed in one metabolite at the convergent node. For example, oxaloacetate (OxA) may be labeled differentially depending on whether it is formed by the carboxylation of phosphoenol pyruvate, or from α-ketoglutarate via the reactions of the tricarboxylic acid (TCA) cycle (Figure 2B). Whether we can evaluate the flux ratio at the OxA node rests upon the particular 13C-substrate label used in the culture. Other nodes of this kind are pyruvate, α-ketoglutarate, and 3-phosphoglyceric acid. Often the labeling pattern in these intermediates is not measured directly, but accessed indirectly through their anabolic products (Szyperski, 1995, 1998). Asp, for example, accumulates in protein and represents the labeling signature in OxA (Figure 2A). For studying plant flux, the analyses of protein-bound amino acids by NMR, or by gas chromatography/MS (GC/MS) methods, have emerged as standard practices (Allen and Ratcliffe, 2009).
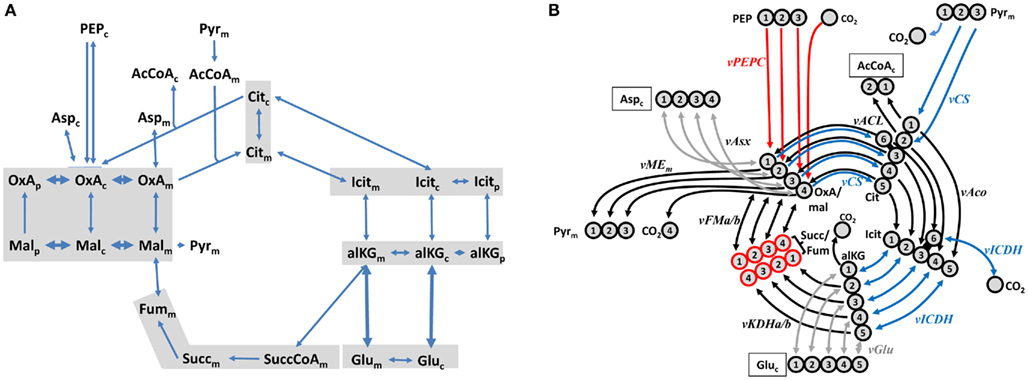
Figure 2. Difference in network topology between large-scale stoichiometric models and the models used in 13C-MFA. Two representations are shown of a sub-network representing the TCA cycle and some associated reactions in B. napus developing embryos. Indices c, p, and m indicate whether the localization of a metabolite is specified to be cytosol, plastid, or mitochondrium, respectively. Metabolites for which 13C-labeling signatures are measured are boxed. (A) Representation of the network in bna572 (Hay and Schwender, 2011a). Arrows depict individual reactions that are formulated in bna572 by a complete reaction stoichiometry. To make the topology understandable, in most cases only one substrate to product transition is shown for each reaction. Thick arrows indicate that two pools are inter-converted by multiple reactions. Sets of metabolite pools that are lumped in the 13C-MFA model are highlighted in gray. (B) Representation of the network for a related 13C-MFA model (Schwender et al., 2006) showing the carbon backbones of the metabolites. Biochemical reactions are carbon transitions as connecting arrows (double-headed arrows for reversible reactions). For succinate (Succ), the symmetric randomization of carbon atoms is indicated that is accomplished in the model by two reactions (e.g., KDHa: alKG(#ABCDE) > Succ(#ABCD) + CO2(#E); KDHb: alKG(#ABCDE) > Succ(#DCBA) + CO2(#E)) occurring at the same rate. Metabolites with measured 13C-label signatures are boxed. Metabolite abbreviations: AcCoA, acetyl coenzyme A; alKG, α-ketoglutarate; Cit, citrate; Fum, fumarate; Ici, isocitrate; Mal, malate; OxA, oxaloacetate; Pyr, pyruvate; Succ, succinate; SuccCoA, succinyl coenzymeA.
Defining the Model Boundaries
A 13C-MFA experiment allows to explore the distribution of in vivo flux under a particular physiological condition. For all organic substrates present in the medium, such as sucrose or glutamine, their uptake reactions must be defined. Furthermore, by quantitatively breaking down the cell components, we can identify the most abundant compounds to result from biosynthetic fluxes (Figure 1). This approach defines several biomass drain fluxes that are responsible for cell growth. Typically neglected are the growth demands for synthesizing a multitude of low-abundance free intermediary metabolites, as well as enzyme cofactors, pigments, and phytohormones. The inclusion of such minor compounds into the metabolic network would not significantly affect the flux distribution in central metabolism. Finally, measurements of growth kinetics can serve to scale the model fluxes relative to a specific growth rate.
Encoding Biochemical Reactions
In 13C-MFA all reaction stoichiometries must be augmented by carbon transitions. Any particular biochemical reaction may be formulated as a set of carbon-atom transitions, defining how each one moves between the main substrates and products. For example, a textual notation following the style of Wiechert and de Graaf (1996) for the carboxylation of phosphoenol pyruvate (PEP) is

with A, B, and C respectively denoting the carbons one, two, and three of PEP being converted into carbons one, two, and three of OxA. The carbon chain #ABC joins with #a (CO2), becoming carbon four of OxA (Figure 2B). Co-substrates, such as ATP, phosphate, or H2O are not considered; hence, both PEP carboxylase (EC 4.1.1.31) and PEP carboxykinase (EC 4.1.1.32) would be encoded by the above equation. In addition to carbon transitions, we must decide if the reaction is a unidirectional- or bidirectional-one. Several plant models define the above reaction as unidirectional, assuming it to be PEP carboxylase, which reportedly is unidirectional. Such reactions with very large standard enthalpy can safely be assumed to be unidirectional in any organism under any condition. Yet, for reactions with smaller standard enthalpy a highly reliable definition of a reaction’s directionality would require knowledge of organism- or tissue-specific in vivo concentrations of all enzyme substrates (Heinrich and Schuster, 1996).
Essential Computational Aspects of 13C-MFA
Based on the definition of all reactions in the network outlined above, the modeling framework 13CFLUX (Wiechert et al., 2001) automatically generates the necessary equation systems to simulate the distribution of the 13C-label in the network. Labeling state variables can be encoded as the relative abundance of isotope isomers (isotopomers; Schmidt et al., 1997). Accordingly, in the above example, PEP would be represented by the fractional abundances of the eight isotopomer species #000, #100, #010, #001, #110, #011, #101, and #111 (with “1” denoting 13C, and “0” denoting 12C). Recently, the efficiency of computations increased, based on derived concepts like cumulated isotopomers (cumomers; Wiechert, 2001), bond isomers (bondomers; van Winden et al., 2002; Sriram et al., 2007), and elementary metabolic units (EMUs; Antoniewicz et al., 2007). The network examples in Table 1 range between about 2000- and 4000-simulated cumomer species, while the EMU approach reduces by several-fold the number of labeling state variables (Table 1); in future, this should support the simulation of much larger networks.
The goal of the computational analysis is to determine the unknown fluxes that best explain the experimental data. While various studies can differ considerably in their details, a general outline is given here. To determine the fluxes in the system, we use a search algorithm (iterative least-squares fitting procedure). Starting from an initial guess, a set of flux values is sought wherein the fluxes and labeling signatures predicted by the model are the closest to the experimentally determined flux and labeling measurements. Various studies repeated this optimization process between about 200- and 1000-times (Allen et al., 2009b; Lonien and Schwender, 2009; Masakapalli et al., 2010) to assure an adequate exploration of the possible existence of alternative solutions. In addition, the search algorithm might converge repeatedly to flux values that represent only a local optimum. The more often the search is done, the more confident can the modeler be that the global optimum solution is uncovered. For the network definition process discussed below, it is important to note that the required computation time per optimization run increases with the network’s size and complexity, as does the computational effort necessary to analyze a solution space of increasing complexity (alternative optima). For modeling four genotypes (model variants) of a larger network (Lonien and Schwender, 2009), access to cluster computing has proven indispensable.
Finally, an important part of computational analysis is to determine the statistical confidence in the obtained flux values (statistical analysis). Flux values that are not obtained consistently by multiple optimizations and have large statistical uncertainty are called “not resolved.” Additionally, to assess how strong is the foundation of the flux results in the experimental data, we can determine in a sensitivity analysis how the values for optimal flux depend on the label measurements and other model parameters. By further considering the choice of substrate labeling, experiments can be optimized to resolve particular fluxes of interest (experimental design; Libourel et al., 2007).
Network Definition and Validation in 13C-MFA
The core of a formulated network in 13C-MFA typically consists of the reactions in glycolysis, the pentose-phosphate pathway and Calvin cycle, the TCA cycle, and in anaplerosis. This formulation could be based on a consensus from the biochemical literature on the plant’s central metabolic pathways. For example, while the presence of mitochondrial- and plastidic-isoforms of pyruvate dehydrogenase in higher plants is well established (Tovar-Méndez et al., 2003), including a cytosolic isoform in a model would be unrealistic unless there was good evidence from the particular plant being studied. Beyond a consensus, experimental evidence in the literature or databases about a particular plant species and cell type typically also is considered.
A key to resolving fluxes of pathways organized in parallel in different compartments is to obtain compartment-specific labeling information. For example, Val, Leu, and Ile are formed from pyruvate in the plastid, i.e., their carbon chains store label information of plastidic pyruvate (Singh and Shaner, 1995). Furthermore, protein-bound Asp, Ala, and Glu, respectively, are assumed to represent cytosolic oxaloacetate, pyruvate, and α-ketoglutarate, provided that the following two assumptions are valid: (1) Asp, Ala, and Glu in the cytosol are isotopically equilibrated with their respective corresponding α-ketocarboxylic acids due to the high activity of the reversible aminotransferases, and, (2) Most of the analyzed protein is synthesized from cytosolic amino acids, i.e., in the analyzed biomass only very small fractions of proteins originated from plastidic or mitochondrial protein synthesis.
In 13C-MFA models, the intrinsic complexity of the metabolic network often is reduced extensively by lumping metabolic pools (van Winden et al., 2001b), as demonstrated for the highly connected sub-network of the TCA cycle in B. napus (Figure 2). Pools of OxA and malate (Mal), localized in the cytosol and mitochondria (Figure 2A), are lumped into one pool (Oxa/Mal, Figure 2B). This combination was justified mainly by observations made in labeling signatures in Asp, derived from storage protein (Schwender et al., 2006). Symmetries in the labeling pattern suggested that OxA, after its derivation from the carboxylation of PEP in the cytosol, undergoes a randomization, attributed to the symmetry in succinate (Succ) and fumarate (Fum; Figure 2B). Therefore, the equilibration of the carbon-labeling signatures of the C-4 carboxylic acids OxA, Mal, Succ, and Fum supposedly reflects the large fluxes that inter-convert those pools within cytosol and mitochondria, and across the mitochondrial membrane (Figure 2A). Therefore, for the 13C-MFA model, the complexity of the C-4 carboxylic acids inter-conversions was reduced by defining two lumped pools, i.e., OxA/Mal and Succ/Fum, and condensing the various reversible inter-conversions (Figure 2A) into one reversible reaction (vFM, Figure 2B). The consequence of this network reduction is that the net and exchange flux of vFM can be determined with good precision, although the parallel reactions in the cytosol and mitochondria cannot be resolved.
Typically, the modeling process also considers whether adding or removing particular reactions in an existing model might generate a model that better fits the labeling measurements (Schwender et al., 2006; Williams et al., 2008; Lonien and Schwender, 2009; Masakapalli et al., 2010). For example, the isocitrate dehydrogenase reaction is often considered unidirectional from citrate to α-ketoglutarate. Yet, in Brassica napus (rapeseed) and soy embryos, the labeling pattern in citrate is explained only if the model also allows for conversion of α-ketoglutarate back to citrate (Schwender et al., 2006; Allen et al., 2009b). This finding showed that, in contrast to the common assumption in the literature, the isocitrate dehydrogenase reaction (Figure 2B) must be reversible in vivo. Other observations on labeling signatures in B. napus justified the assumption that the conversions of PEP to OxA, or PEP to Pyr are in vivo irreversible (Schwender et al., 2006).
In conclusion, the topology of published 13C-MFA networks often reflects several assumptions and circumstantial experimental evidence used to justify using lumped networks. Often the underlying unreduced (large-scale) network, and the reduction process are not documented fully and transparently. Lumped metabolic models might depend in part on intuition, and only somewhat result from a transparent process to reduce network complexity. Yet, in 13C-MFA, the resulting values for flux and their interpretation critically depend upon the network’s topology (van Winden et al., 2001a). In addition, once flux results are obtained, projecting the lumped metabolic models on to large-scale models involves substantial ambiguity. This means that mapping fluxes to pathways from pathway databases is problematic.
Generally, more organized, transparent, and reproducible workflows might improve model reconstruction; this is a major topic in other fields of biological computational research (Dalman et al., 2010; Goecks et al., 2010; Mesirov, 2010). With this in mind, we can employ some recently published genome-scale plant metabolic models used for FBA (Table 1; Poolman et al., 2009; de Oliveira Dal’Molin et al., 2010a,b; Williams et al., 2010; Saha et al., 2011) as a reference for a more unbiased and more clearly defined network reconstruction in 13C-MFA. Yet, although the genome-scale networks claim to be unbiased representations of the whole genome (Covert et al., 2001), they suffer from incompleteness and from the limited accuracy of gene annotation; certainly, for eukaryotes (plants) they reflect the very limited availability and reliability of predictions of the subcellular localization of the gene products (Poolman et al., 2006; Sweetlove et al., 2008). A particular problem arising in deriving compartmentalized networks is that many of the intracellular transporters functionally required remain unidentified and uncharacterized. Also, there is the ambiguous affinity of many of the known transporters to different substrates of similar structure (Linka and Weber, 2010). Furthermore, if a whole plant-genome is the template for network reconstruction, the result must be a generalized network rather than a network specific for a certain cell type. In addition, despite the recent comprehensive atom mapping of an E. coli genome-scale model (Ravikirthi et al., 2011), the carbon transitions in such large plant networks cannot yet be straightforwardly derived from databases.
Consequently, deriving reliable networks from plant-genome databases should require an enormous amount of manual curation. Alternatively, more useful may be the well- documented “bottom-up” reconstructions of large-scale plant models based on published biochemical- and tissue-specific-evidence (Table 1; Grafahrend-Belau et al., 2009; Hay and Schwender, 2011a,b). These models might be developed into large-scale 13C-MFA models. While current 13C-MFA models encompass between ∼50 and 100 reactions (Table 1), Suthers et al. (2007, 2010) modeled a large-scale E. coli network with 238 reactions. Recent advances in the mathematical formulation of isotope models, like the simulation of EMU support the representation of such networks with substantially less computation time than presently required (Antoniewicz et al., 2007). If large-scale plant 13C-MFA models are to be simulated, certain aspects must be dealt with as detailed for the E. coli large-scale 13C-MFA model (Suthers et al., 2007). No single optimal flux solution is obtained, and a complex analysis of the solution space is necessary, implying that, for many fluxes, a range of optimum values will be obtained rather than a discrete one. This problem can be attributed largely to parallel pathways that produce redundant labeling patterns and cannot be resolved. Some redundant solutions involving parallel pathways can contain substrate cycles that expectedly are of little biological relevance; thus Suthers et al. (2007) suggested a multi-step reduction in network size. They verified that each time metabolic pools are merged or a parallel pathway is removed, the model fit is not worsened, i.e., simplifying the model does not introduce bias. This kind of approach could replace the more intuitive “classical” model definition of lumped 13C-MFA networks.
A further improvement of the definition of large-scale metabolic networks could lie in using quantitative analysis of the transcriptome by deep-sequencing technologies (RNA-seq; Wang et al., 2009). This technology requires having a genome sequence but should assure a more precise definition about which gene products are present in a particular cell type under specific conditions. The definition of central core metabolism would be improved, in particular since the subcellular localization of core metabolism enzymes can differ between cell types or species. For example, phosphoglyceromutase is only present in plastids of certain cell types (Stitt and ap Rees, 1979). The subcellular localization of ADP-Glucose Pyrophosphorylase differs between gramineous and non-gramineous species (Beckles et al., 2001).
Conclusion
In plant-specific 13C-MFA studies published to date lumped network topologies are required. These networks represent a substantial simplification relative to the real complexity inherent to plant central metabolism. Often the validity of network simplifications has to be justified by vague assumptions or circumstantial experimental evidence. Constructing large-scale metabolic models can provide fully detailed networks, useful as a clearly defined reference point for deriving lumped 13C models. Moreover, without lumping, 13C-MFA with plant models of about 500 reactions in size should become computationally feasible, as indicated by recent microbial studies using large-scale 13C-MFA (Suthers et al., 2007, 2010).
The large-scale reference models also offer the potential to develop approaches that combine FBA with 13C-MFA (Blank et al., 2005). Some explorations of the synergies between the two approaches were reported (Williams et al., 2010; Chen et al., 2011; Hay and Schwender, 2011b). With FBA, different physiological conditions can be simulated in silico to analyze situations in which steady-state 13C-tracer experiments are impossible.
Another important goal in plant 13C-MFA is to improve the precision of the flux estimates. This can be achieved by simulation of different experiments with differently 13C-labeled tracers in one flux model (Schwender et al., 2006; Alonso et al., 2007b, 2011; Junker et al., 2007; Masakapalli et al., 2010).
Furthermore, analysis of how the distribution of cellular flux changes in response to targeted perturbations can help to unravel the kinetic- and regulatory-controls in metabolism (Lonien and Schwender, 2009). Such approaches should be particularly promising if for experimental systems that have been well established for 13C-MFA, metabolomic, transcriptomic, and proteomic data are recorded in parallel.
Conflict of Interest Statement
The author declares that the research was conducted in the absence of any commercial or financial relationships that could be construed as a potential conflict of interest.
Acknowledgments
Current funding from the U.S. Department of Energy (Division of Chemical Sciences, Geosciences, and Biosciences, Office of Basic Energy Sciences, Field Work Proposal BO-133) as well as by Bayer Bioscience is much appreciated. I like to thank Avril Woodhead (Brookhaven National Laboratory) for English language edits.
References
Allen, D. K., Libourel, I. G. L., and Shachar-Hill, Y. (2009a). Metabolic flux analysis in plants: coping with complexity. Plant Cell Environ. 32, 1241–1257.
Allen, D. K., Ohlrogge, J. B., and Shachar-Hill, Y. (2009b). The role of light in soybean seed filling metabolism. Plant J. 58, 220–234.
Allen, D. K., and Ratcliffe, R. G. (2009). “Quantification of isotope label,” in Plant Metabolic Networks, ed. J. Schwender (New York: Springer), 105–149.
Alonso, A. P., Dale, V. L., and Shachar-Hill, Y. (2010). Understanding fatty acid synthesis in developing maize embryos using metabolic flux analysis. Metab. Eng. 12, 488–497.
Alonso, A. P., Goffman, F. D., Ohlrogge, J. B., and Shachar-Hill, Y. (2007a). Carbon conversion efficiency and central metabolic fluxes in developing sunflower (Helianthus annuus L.) embryos. Plant J. 52, 296–308.
Alonso, A. P., Raymond, P., Hernould, M., Rondeau-Mouro, C., De Graaf, A., Chourey, P., Lahaye, M., Shachar-Hill, Y., Rolin, D., and Dieuaide-Noubhani, M. (2007b). A metabolic flux analysis to study the role of sucrose synthase in the regulation of the carbon partitioning in central metabolism in maize root tips. Metab. Eng. 9, 419–432.
Alonso, A. P., Raymond, P., Rolin, D., and Dieuaide-Noubhani, M. (2007c). Substrate cycles in the central metabolism of maize root tips under hypoxia. Phytochemistry 68, 2222–2231.
Alonso, A. P., Val, D. L., and Shachar-Hill, Y. (2011). Central metabolic fluxes in the endosperm of developing maize seeds and their implications for metabolic engineering. Metab. Eng. 13, 96–107.
Alonso, A. P., Vigeolas, H., Raymond, P., Rolin, D., and Dieuaide-Noubhani, M. (2005). A new substrate cycle in plants. Evidence for a high glucose-phosphate-to-glucose turnover from in vivo steady-state and pulse-labeling experiments with [13C]glucose and [14C]glucose. Plant Physiol. 138, 2220–2232.
Antoniewicz, M. R., Kelleher, J. K., and Stephanopoulos, G. (2007). Elementary metabolite units (EMU): a novel framework for modeling isotopic distributions. Metab. Eng. 9, 68–86.
Beckles, D. M., Smith, A. M., and ap Rees, T. (2001). A cytosolic ADP-glucose pyrophosphorylase is a feature of graminaceous endosperms, but not of other starch-storing organs. Plant Physiol. 125, 818–827.
Blank, L. M., Kuepfer, L., and Sauer, U. (2005). Large-scale 13C-flux analysis reveals mechanistic principles of metabolic network robustness to null mutations in yeast. Genome Biol. 6, R49.
Chen, X., Alonso, A. P., Allen, D. K., Reed, J. L., and Shachar-Hill, Y. (2011). Synergy between 13C-metabolic flux analysis and flux balance analysis for understanding metabolic adaptation to anaerobiosis in E. coli. Metab. Eng. 13, 38–48.
Covert, M. W., Schilling, C. H., Famili, I., Edwards, J. S., Goryanin Ii Selkov, E., and Palsson, B. O. (2001). Metabolic modeling of microbial strains in silico. Trends Biochem. Sci. 26, 179–186.
Dalman, T., Droste, P., Weitzel, M., Wiechert, W., and Nöh, K. (2010). “Workflows for metabolic flux analysis: data integration and human interaction,” in Proceedings of the 4th International Conference on Leveraging Applications of Formal Methods, Verification, and Validation – Volume Part I (Heraklion: Springer-Verlag).
Dauner, M., and Sauer, U. (2000). GC-MS analysis of amino acids rapidly provides rich information for isotopomer balancing. Biotechnol. Prog. 16, 642–649.
de Oliveira Dal’Molin, C. G., Quek, L. E., Palfreyman, R. W., Brumbley, S. M., and Nielsen, L. K. (2010a). AraGEM, a genome-scale reconstruction of the primary metabolic network in Arabidopsis. Plant Physiol. 152, 579–589.
de Oliveira Dal’Molin, C. G., Quek, L. E., Palfreyman, R. W., Brumbley, S. M., and Nielsen, L. K. (2010b). C4GEM, a genome-scale metabolic model to study C4 plant metabolism. Plant Physiol. 154, 1871–1885.
Dieuaide-Noubhani, M., Raffard, G., Canioni, P., Pradet, A., and Raymond, P. (1995). Quantification of compartmented metabolic fluxes in maize root tips using isotope distribution from 13C- or 14C-labeled glucose. J. Biol. Chem. 270, 13147–13159.
Ettenhuber, C., Spielbauer, G., Margl, L., Hannah, L. C., Gierl, A., Bacher, A., Genschel, U., and Eisenreich, W. (2005). Changes in flux pattern of the central carbohydrate metabolism during kernel development in maize. Phytochemistry 66, 2632–2642.
Goecks, J., Nekrutenko, A., and Taylor, J. (2010). Galaxy: a comprehensive approach for supporting accessible, reproducible, and transparent computational research in the life sciences. Genome Biol. 11, R86.
Grafahrend-Belau, E., Schreiber, F., Koschützki, D., and Junker, B. H. (2009). Flux balance analysis of barley seeds: a computational approach to study systemic properties of central metabolism. Plant Physiol. 149, 585–598.
Hay, J. O., and Schwender, J. (2011a). Metabolic network reconstruction and flux variability analysis of storage synthesis in developing oilseed rape (Brassica napus L.) embryos. Plant J. 67, 526–541.
Hay, J. O., and Schwender, J. (2011b). Computational analysis of storage synthesis in developing Brassica napus L. (oilseed rape) embryos: flux variability analysis in relation to 13C metabolic flux analysis. Plant J. 67, 513–525.
Heinrich, R., and Schuster, S. (1996). The Regulation of Cellular Systems. New York: Chapman and Hall.
Iyer, V. V., Sriram, G., Fulton, D. B., Zhou, R., Westgate, M. E., and Shanks, J. V. (2008). Metabolic flux maps comparing the effect of temperature on protein and oil biosynthesis in developing soybean cotyledons. Plant Cell Environ. 31, 506–517.
Junker, B. H., Lonien, J., Heady, L. E., Rogers, A., and Schwender, J. (2007). Parallel determination of enzyme activities and in vivo fluxes in Brassica napus embryos grown on organic or inorganic nitrogen source. Phytochemistry 68, 2232–2242.
Koschutzki, D., Junker, B. H., Schwender, J., and Schreiber, F. (2010). Structural analysis of metabolic networks based on flux centrality. J. Theor. Biol. 265, 261–269.
Kruger, N. J., and Ratcliffe, R. G. (2009). Insights into plant metabolic networks from steady-state metabolic flux analysis. Biochimie 91, 697–702.
Libourel, I. G., Gehan, J. P., and Shachar-Hill, Y. (2007). Design of substrate label for steady state flux measurements in plant systems using the metabolic network of Brassica napus embryos. Phytochemistry 68, 2211–2221.
Libourel, I. G. L., and Shachar-Hill, Y. (2008). Metabolic flux analysis in plants: from intelligent design to rational engineering. Annu. Rev. Plant Biol. 59, 625–650.
Linka, N., and Weber, A. P. (2010). Intracellular metabolite transporters in plants. Mol. Plant 3, 21–53.
Llaneras, F., and Pico, J. (2008). Stoichiometric modelling of cell metabolism. J. Biosci. Bioeng. 105, 1–11.
Lonien, J., and Schwender, J. (2009). Analysis of metabolic flux phenotypes for two Arabidopsis mutants with severe impairment in seed storage lipid synthesis. Plant Physiol. 151, 1617–1634.
Masakapalli, S. K., Le Lay, P., Huddleston, J. E., Pollock, N. L., Kruger, N., and Ratcliffe, R. G. (2010). Subcellular flux analysis of central metabolism in a heterotrophic Arabidopsis cell suspension using steady-state stable isotope labeling. Plant Physiol. 152, 602–619.
Poolman, M. G., Bonde, B. K., Gevorgyan, A., Patel, H. H., and Fell, D. A. (2006). Challenges to be faced in the reconstruction of metabolic networks from public databases. Syst. Biol. (Stevenage) 153, 379–384.
Poolman, M. G., Miguet, L., Sweetlove, L. J., and Fell, D. A. (2009). A genome-scale metabolic model of Arabidopsis and some of its properties. Plant Physiol. 151, 1570–1581.
Ravikirthi, P., Suthers, P. F., and Maranas, C. D. (2011). Construction of an E. Coli genome-scale atom mapping model for MFA calculations. Biotechnol. Bioeng. 108, 1372–1382.
Rontein, D., Dieuaide-Noubhani, M., Dufourc, E. J., Raymond, P., and Rolin, D. (2002). The metabolic architecture of plant cells. Stability of central metabolism and flexibility of anabolic pathways during the growth cycle of tomato cells. J. Biol. Chem. 277, 43948–43960.
Saha, R., Suthers, P. F., and Maranas, C. D. (2011). Zea mays iRS1563: a comprehensive genome-scale metabolic reconstruction of maize metabolism. PLoS ONE 6, e21784. doi:10.1371/journal.pone.0021784
Schmidt, K., Carlsen, M., Nielsen, J., and Villadsen, J. (1997). Modeling isotopomer distributions in biochemical networks using isotopomer mapping matrices. Biotechnol. Bioeng. 55, 831–840.
Schwender, J. (2008). Metabolic flux analysis as a tool in metabolic engineering of plants. Curr. Opin. Biotechnol. 19, 131–137.
Schwender, J. (2009). “Isotopic steady-state flux analysis,” in Plant Metabolic Networks, ed. J. Schwender (New York: Springer), 245–284.
Schwender, J., Goffman, F., Ohlrogge, J. B., and Shachar-Hill, Y. (2004a). Rubisco without the Calvin cycle improves the carbon efficiency of developing green seeds. Nature 432, 779–782.
Schwender, J., Ohlrogge, J., and Shachar-Hill, Y. (2004b). Understanding flux in plant metabolic networks. Curr. Opin. Plant Biol. 7, 309–317.
Schwender, J., and Ohlrogge, J. B. (2002). Probing in vivo metabolism by stable isotope labeling of storage lipids and proteins in developing Brassica napus embryos. Plant Physiol. 130, 347–361.
Schwender, J., Ohlrogge, J. B., and Shachar-Hill, Y. (2003). A flux model of glycolysis and the oxidative pentose phosphate pathway in developing Brassica napus embryos. J. Biol. Chem. 278, 29442–29453.
Schwender, J., Shachar-Hill, Y., and Ohlrogge, J. B. (2006). Mitochondrial metabolism in developing embryos of Brassica napus. J. Biol. Chem. 281, 34040–34047.
Singh, B. K., and Shaner, D. L. (1995). Biosynthesis of branched chain amino acids: from test tube to field. Plant Cell 7, 935–944.
Spielbauer, G., Margl, L., Hannah, L. C., Romisch, W., Ettenhuber, C., Bacher, A., Gierl, A., Eisenreich, W., and Genschel, U. (2006). Robustness of central carbohydrate metabolism in developing maize kernels. Phytochemistry 67, 1460–1475.
Sriram, G., Fulton, D. B., Iyer, V. V., Peterson, J. M., Zhou, R., Westgate, M. E., Spalding, M. H., and Shanks, J. V. (2004). Quantification of compartmented metabolic fluxes in developing soybean embryos by employing biosynthetically directed fractional 13C labeling, two-dimensional [13C, 1H] nuclear magnetic resonance, and comprehensive isotopomer balancing. Plant Physiol. 136, 3043–3057.
Sriram, G., Fulton, D. B., and Shanks, J. V. (2007). Flux quantification in central carbon metabolism of Catharanthus roseus hairy roots by 13C labeling and comprehensive bondomer balancing. Phytochemistry 68, 2243–2257.
Stitt, M., and ap Rees, T. (1979). Capacities of pea chloroplasts to catalyze the oxidative pentose phosphate pathway and glycolysis. Phytochemistry 18, 1905–1911.
Suthers, P. F., Burgard, A. P., Dasika, M. S., Nowroozi, F., Van Dien, S., Keasling, J. D., and Maranas, C. D. (2007). Metabolic flux elucidation for large-scale models using 13C labeled isotopes. Metab. Eng. 9, 387–405.
Suthers, P. F., Chang, Y. J., and Maranas, C. D. (2010). Improved computational performance of MFA using elementary metabolite units and flux coupling. Metab. Eng. 12, 123–128.
Sweetlove, L. J., Fell, D., and Fernie, A. R. (2008). Getting to grips with the plant metabolic network. Biochem. J. 409, 27–41.
Szyperski, T. (1995). Biosynthetically directed fractional 13C-labeling of proteinogenic amino acids. An efficient analytical tool to investigate intermediary metabolism. Eur. J. Biochem. 232, 433–448.
Szyperski, T. (1998). 13C-NMR, MS and metabolic flux balancing in biotechnology research. Q. Rev. Biophys. 31, 41–106.
Tovar-Méndez, A., Miernyk, J. A., and Randall, D. D. (2003). Regulation of pyruvate dehydrogenase complex activity in plant cells. Eur. J. Biochem. 270, 1043–1049.
van Winden, W., Verheijen, P., and Heijnen, S. (2001a). Possible pitfalls of flux calculations based on 13C-labeling. Metab. Eng. 3, 151–162.
van Winden, W. A., Heijnen, J. J., Verheijen, P. J., and Grievink, J. (2001b). A priori analysis of metabolic flux identifiability from (13)C-labeling data. Biotechnol. Bioeng. 74, 505–516.
van Winden, W. A., Heijnen, J. J., and Verheijen, P. J. (2002). Cumulative bondomers: a new concept in flux analysis from 2D [13C,1H] COSY NMR data. Biotechnol. Bioeng. 80, 731–745.
Wang, Z., Gerstein, M., and Snyder, M. (2009). RNA-Seq: a revolutionary tool for transcriptomics. Nat. Rev. Genet. 10, 57–63.
Wiechert, W., and de Graaf, A. A. (1996). In vivo stationary flux analysis by 13C labeling experiments. Adv. Biochem. Eng. Biotechnol. 54, 109–154.
Wiechert, W., Möllney, M., Petersen, S., and De Graaf, A. A. (2001). A universal framework for 13C metabolic flux analysis. Metab. Eng. 3, 265–283.
Williams, T. C., Miguet, L., Masakapalli, S. K., Kruger, N. J., Sweetlove, L. J., and Ratcliffe, R. G. (2008). Metabolic network fluxes in heterotrophic Arabidopsis cells: stability of the flux distribution under different oxygenation conditions. Plant Physiol. 148, 704–718.
Williams, T. C., Poolman, M. G., Howden, A. J., Schwarzlander, M., Fell, D. A., Ratcliffe, R. G., and Sweetlove, L. J. (2010). A genome-scale metabolic model accurately predicts fluxes in central carbon metabolism under stress conditions. Plant Physiol. 154, 311–323.
Keywords: 13C-metabolic flux analysis, primary metabolism, flux balance analysis, carbon partitioning, constraint-based model
Citation: Schwender J (2011) Experimental flux measurements on a network scale. Front. Plant Sci. 2:63. doi: 10.3389/fpls.2011.00063
Received: 16 May 2011; Accepted: 14 September 2011;
Published online: 10 October 2011.
Edited by:
Alisdair Fernie, Max Planck Institute for Plant Physiology, GermanyReviewed by:
Alisdair Fernie, Max Planck Institute for Plant Physiology, GermanyLee Sweetlove, University of Oxford, UK
Ganesh Sriram, University of Maryland, USA
Copyright: © 2011 Schwender. This is an open-access article subject to a non-exclusive license between the authors and Frontiers Media SA, which permits use, distribution and reproduction in other forums, provided the original authors and source are credited and other Frontiers conditions are complied with.
*Correspondence: Jörg Schwender, Department of Biology, Brookhaven National Laboratory, Building 463, Upton, NY 11973, USA. e-mail:c2Nod2VuZEBibmwuZ292