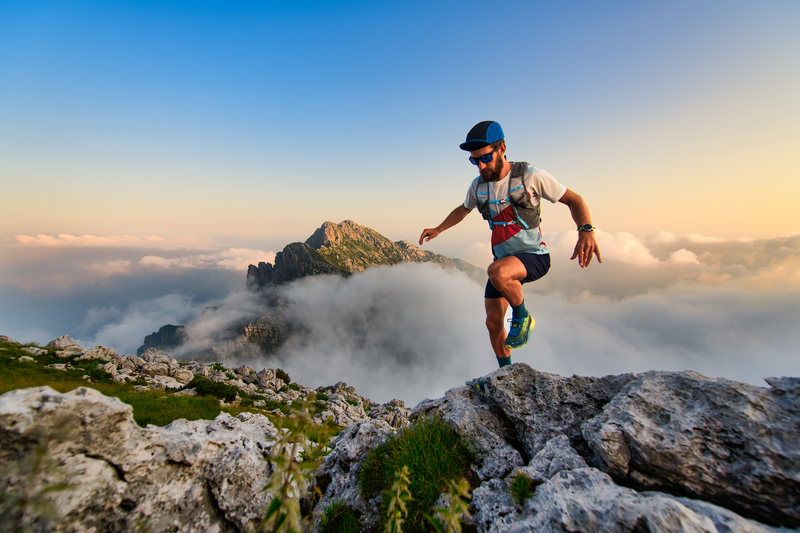
94% of researchers rate our articles as excellent or good
Learn more about the work of our research integrity team to safeguard the quality of each article we publish.
Find out more
ORIGINAL RESEARCH article
Front. Plant Sci. , 12 October 2011
Sec. Plant Physiology
Volume 2 - 2011 | https://doi.org/10.3389/fpls.2011.00054
This article is part of the Research Topic Computational approaches in aid of advancing understanding in Plant Physiology View all 12 articles
Holistic analysis of lipids is becoming increasingly popular in the life sciences. Recently, several interesting, mass spectrometry-based studies have been conducted, especially in plant biology. However, while great advancements have been made we are still far from detecting all the lipids species in an organism. In this study we developed an ultra performance liquid chromatography-based method using a high resolution, accurate mass, mass spectrometer for the comprehensive profiling of more than 260 polar and non-polar Arabidopsis thaliana leaf lipids. The method is fully compatible to the commonly used lipid extraction protocols and provides a viable alternative to the commonly used direct infusion-based shotgun lipidomics approaches. The whole process is described in detail and compared to alternative lipidomic approaches. Next to the developed method we also introduce an in-house developed database search software (GoBioSpace), which allows one to perform targeted or un-targeted lipidomic and metabolomic analysis on mass spectrometric data of every kind.
Holistic analysis of a cellular metabolome, the complement of all small molecules within a cell (Oliver et al., 1998), is still quite complicated due to the huge complexity and the large chemical heterogeneity of all the contained molecules. Besides the polar compounds, like sugars and amino- and organic-acids, there are also a large number of non-polar (water insoluble) compounds which need to be analyzed. The high complexity and chemical diversity, but also the huge difference in the molar abundance of these compounds explains why up to now no single analytical platform has been developed that is able to detect and quantify all of these compounds in a single analysis (Oldiges et al., 2007). As a consequence, different sample extraction and fractionation methods have been developed which allow a rough separation of the metabolites into less complex and more homogeneous fractions before their analysis (Vuckovic et al., 2010). One functionally and chemically distinct metabolic fraction that can be efficiently separated from crude extracts contains the water insoluble, generally hydrophobic lipids.
Lipids have essential functions for all living cells, not only because they are the building blocks of the membranes, which enclose the cell and the internal organelles (Van Meer et al., 2008), but also by functioning as energy storage or signaling molecules (Downes and Currie, 1998; Spiegel and Milstien, 2003; Wenk, 2005; Wymann and Schneiter, 2008). For this purpose it is not surprising that a complete new branch in the metabolomics area, namely the field of lipidomics, emerged, and has made great advancement within the last few years (Dennis, 2009; Blanksby and Mitchell, 2010; Wenk, 2010; Harkewicz and Dennis, 2011). Lipids, which are often defined by their inability to dissolve in water, do still cover a broad spectrum of diverse substances ranging from slightly polar [e.g., glycosylated sphingolipids (Merrill et al., 2009) to highly non-polar lipids like, e.g., triacylglycerol (Kuksis, 2007)]. Estimations on lipid numbers within eukaryotic cells range from a few 100 to several 1,000 lipid species (Dennis, 2009), indicating the expected high complexity. To structure this complexity and to generate a uniform nomenclature for the known lipids a general classification and nomenclature system was required. The publicly funded LIPID MAPS Consortium (Fahy et al., 2005, 2009) provided a new definition system, which is mostly based on the biosynthetic origin of the different lipids and not only on the solubility of the compound. Therefore the lipids are now defined as hydrophobic or amphiphatic small molecules, which originate from carbanion-based condensation of thioesters or by carbocation-based condensation of isoprene units (Fahy et al., 2005). This new definition is not only more precise then the old water insolubility-based definition, but it also allows to classify the commonly known lipids into homogenous functional subclasses: namely the fatty acids, glycerolipids, glycerophospholipids, sphingolipids, sterols, prenols, saccharolipids, and polyketides (Fahy et al., 2005).
The fact that no single analytical technology has allowed the identification and quantification of all metabolite species in a single experiment is also true for the analysis of all the different lipids from a cell (Wenk, 2010). Historically, lipids have been analyzed by diverse chromatography-based separation methods (Bausch, 1993). Commonly used technologies comprised methods like one or two dimensional thin layer chromatography in combination with different visualization strategies (Touchstone, 1995), but also high performance liquid chromatography (HPLC) methods in combination with various detection systems (Picchioni et al., 1996). Even though these methods have proven useful for many purposes, it seems that their limitations for large scale quantitative lipid analysis are more evident (Blanksby and Mitchell, 2010). As a consequence, mass spectrometry (MS)-based methods, with or without chromatographic separation techniques, have evolved to fill this technological gap (Welti et al., 2007b; Griffiths and Wang, 2009; Blanksby and Mitchell, 2010; Wenk, 2010; Harkewicz and Dennis, 2011).
There are many different MS instruments available which can be combined with an even larger number of separation systems (Griffiths and Wang, 2009; Wenk, 2010). Still, only two main strategies for the analysis of lipids have been used in most of the described reports: on one hand there is the most successfully used method, namely shotgun lipidomics, which relies on a separation free (direct infusion) analysis of a crude lipid extract on triple quadrupole (QqQ) or quadrupole time-of-flight (qTOF) mass spectrometers (Welti and Wang, 2004; Han and Gross, 2005; Ejsing et al., 2009; Yang et al., 2009), on the other hand there is chromatography-based separation prior to the mass spectrometric measurement for the lipid analysis, which has been used only in a small number of studies thus far (Markham and Jaworski, 2007; Rainville et al., 2007; Glauser et al., 2008b; Nakanishi et al., 2009; Nygren et al., 2011). Both methods have their advantages and disadvantages: for example, the shotgun approach is prone to strong ion suppression effects, which can in part be compensated for by large sample dilutions or by the use of internal reference compounds (Moore et al., 2007). While the chromatography-based methods are less sensitive to these suppression effects, due to the chromatographic separation (Muller et al., 2002; Annesley, 2003), these approaches were thus far unsuitable for absolute lipid quantification (Stahlman et al., 2009).
In the field of plant metabolomics both technologies have found their applications, while the polar glycerolipids have been widely analyzed by the shotgun lipidomic approach (Devaiah et al., 2007; Welti et al., 2007b; Zhang et al., 2009; Kilaru et al., 2010), sphingolipids have been most successfully analyzed by targeted LC–MS-based approaches (Markham et al., 2006; Markham and Jaworski, 2007; Chen et al., 2008). Still, since most of these studies made use of highly sensitive, but low resolution mass spectrometers, they were mostly performed in a targeted way, by simply profiling a limited number of known lipid species (Lu et al., 2008).
In this report we describe a versatile and reproducible ultra performance liquid chromatography (UPLC)-based separation system, coupled to a high resolution mass spectrometer operating in MS as well as all-ion fragmentation mode. The developed system allows for the accurate qualitative and semi-quantitative targeted analysis of several hundred different lipid species extracted from a single plant sample. Additionally, due to the combination of chromatography and high resolution MS and all-ion MS/MS, the method allows to revisit the data long after the actual measurement and therefore extract and possibly elucidate novel structures (Harkewicz and Dennis, 2011). For the actual data mining we introduce a novel database search (GoBioSpace), which allows one to perform either targeted or un-targeted database searches with the acquired lipid data.
The Arabidopsis thaliana Col-0 plants used for the metabolite extraction were grown in a light and temperature controlled phytotron under constant CO2 conditions using a BioBox growth chamber (GMS Gaswechsel-Messsysteme GmbH, Berlin, Germany). The plant material preparation and the experimental settings for the BioBox were as previously described (Huege et al., 2007). Plant growth in the BioBox was performed for 42 days. The aerial parts of the plants were separated from the roots by cutting, and immediately snap frozen in liquid nitrogen.
Lipids were extracted from three independent biological replicates of Arabidopsis thaliana leaves. In brief: 50 mg of frozen leaf tissue was homogenized in a 2 ml Eppendorf tube (Eppendorf, Hamburg, Germany) for two times 1 min at maximum speed within a Retsch mill (MM 301, Retsch, Düsseldorf, Germany). The lipids were extracted from each aliquot using 1 ml of a pre-cooled (−20°C) homogenous methanol:methyl-tert-butyl-ether (1:3) mixture, spiked with 0.1 μg/ml PE 34:0 (17:0, 17:0), and PC 34:0 (17:0, 17:0) as internal standards. For the extraction, the samples were incubated for 10 min in a shaker at 4°C (Thermostat Plus, Eppendorf), followed by another 10 min incubation in an ultrasonication bath at RT. After adding 500 μl of UPLC grade water:methanol (3:1), the homogenate was vortexed and centrifuged for 5 min at 4°C in a table top centrifuge (Eppendorf). The addition of water:methanol leads to a phase separation producing an upper organic phase, containing the lipids, and a lower phase containing the polar and semi-polar metabolites. The upper organic phase was removed, dried in a speed-vac concentrator, and stored at −80°C until used.
The dried lipid extracts were re-suspended in 500 μl buffer B (see below) and transferred to a glass vial. Two microliters of this sample were injected on a C8 reversed phase column (100 mm × 2.1 mm × 1.7 μm particles waters), using a Waters Acquity UPLC system. The two mobile phases were water (UPLC MS grade, BioSolve) with 1% 1 M NH4Ac, 0.1% acetic acid (Buffer A,), and acetonitrile:isopropanol (7:3, UPLC grade BioSolve) containing 1% 1 M NH4Ac, 0.1% acetic acid (Buffer B). The gradient separation, which was performed at a flow rate of 400 μl/min, was: 1 min 45% A, 3 min linear gradient from 45% A to 35% A, 8 min linear gradient from 25 to 11% A, 3 min linear gradient from 11% A to 1% A. After washing the column for 3 min with 1% A the buffer was set back to 45% A and the column was re-equilibrated for 4 min (22 min total run time).
The mass spectra were acquired using an Exactive mass spectrometer (Thermo-Fisher, Bremen, Germany). The spectra were recorded using altering full scan and all-ion fragmentation scan mode, covering a mass range from 100–1500 m/z. The resolution was set to 10,000 with 10 scans per second, restricting the Orbitrap loading time to a maximum of 100 ms with a target value of 1E6 ions. The capillary voltage was set to 3 kV with a sheath gas flow value of 60 and an auxiliary gas flow of 35. The capillary temperature was set to 150°C, while the drying gas in the heated electro spray source was set to 350°C. The skimmer voltage was held at 25 V while the tube lens was set to a value of 130 V. The spectra were recorded from min 1 to min 20 of the UPLC gradients.
Chromatograms from the UPLC–FT–MS runs were analyzed and processed either by using Xcalibur (Version 2.10, Thermo-Fisher, Bremen, Germany), ToxID (Version 2.1.1, Thermo-Fisher), or automatically with the Refiner MS® software (Version 6.0, GeneData, Basel, Switzerland). In the automated approach the molecular masses, retention time, and associated peak intensities for the three replicates of each sample were extracted from the raw files, which contained the full scan MS and the all-ion fragmentation MS data. The processing of the MS data included the separate processing of the full scan spectra and the all-ion fragmentation spectra. Chemical noise was automatically removed from the spectra before the chromatograms were aligned using a pair wise-based alignment tree algorithm (Refiner MS 6.0).
Further peak filtering on the manually extracted spectra or the aligned data matrices was performed in Excel or Access (Microsoft, Seattle, WA, USA).
Based on the fact that the masses measured in the mass spectrometer are almost directly connected to the elemental composition of a measured analyte, considering either an addition or loss of a sub structure – so called adducts (i.e., [M + H]+ protonation, [M − H]− de-protonation, M + NH4]+ Ammonium-, [M + Na]+ Sodium-, [M + Ca]+ Calcium-adduct), GoBioSpace (Golm Biochemical Space) was conceptualized as a repository of elemental compositions with source tagged annotations for properties such as InChI strings, CAS numbers, IUPAC names, synonyms, cross references or KEGG Pathway names, among others.
The source of an annotation – the so called depositor – facilitates as a filter for the biological relevance of elemental compositions. The meaningful interpretation of search results in a biological context is accomplished by a targeted search limiting the formula to biology related depositors such as KEGG and BioCyc, among others. In contrast, relaxed searches in regard to the formula’s depositor (i.e., including those elemental compositions only reported from vendors of potentially synthesized chemicals) result in search hits with lower biological interpretability.
To date, we collected more than 366 million meta information for 2.1 million unique elemental compositions from more than 150 public available databases (143 included in PubChem), such as the chemical focused databases PubChem Substance1 and ChemSpider2 or biological focused databases such as the Human Metabolome Database3 and Metabolome.JP4 into the GoBioSpace repository. Our approach also facilitates the search against potentially putative elemental compositions such as described for lipids in the chapter “Targeting Specific Lipids within the Total Ion Chromatogram: Pick What You Know.”
For high resolution mass search queries, the accurate isotopic masses for either ambient 12C or fully isotopic labeled 13C, 15N, and 34S formula were calculated according to Böhlke et al. (2005). An indexed view in the database allows the single step matching of measured masses to elemental compositions, tolerating a given mass error and considering user defined sets of expected analytical adducts and depositors to correct the measured masses. In addition, the client side search application supports the restriction of elemental composition hits based on atom number constraints.
To make the mass search functionality accessible to the community, we implemented a Web Service within the Golm Metabolome Database (GMD5; Kopka et al., 2005; Hummel et al., 2010) and integrated this web service into a graphical user interface which is also made available http://gmd.mpimp-golm.mpg.de/GoBioSpace.aspx. Here, elemental compositions and individual or batched (tabulator formatted text files) masses can easily be configured and searched against databases of interest. The matching results are returned as browse- and sort-able tables which can be exported for further analysis as tabular formatted text files. However, the web services can be integrated for non-commercial use into any data processing pipeline. All software is implemented using the Microsoft .NET 4.0 framework, the C# language, and Microsoft Visual Studio® 2010. The data back end is based on a Microsoft® SQL Server® 2005.
Arabidopsis thaliana lipids were extracted using a buffer system containing methyl-tert-butyl-ether instead of chloroform as the organic solvent (Matyash et al., 2008). This extraction protocol enabled us not only to extract the lipids with a higher efficiency, but also to extract lipids, polar and semi-polar metabolites, starch, and proteins from a single sample (Giavalisco et al., 2011). The extracted lipids were analyzed on a C8 reversed phase UPLC column, using 1.7 μm particles (Rainville et al., 2007), in a 22 min method. Both steps, the extraction as well as the chromatographic separation are simple and high-throughput compatible methods, and are applicable for several different plants but also other, non-photosynthetic organisms like, e.g., yeast, Drosophila, C. elegans, or mammalian tissue (data not shown).
All mass spectrometric measurements were performed on a standalone high resolution Orbitrap (Exactive) mass spectrometer (Lu et al., 2010), coupled to an ultra performance liquid chromatography system. This “smaller” version of an Orbitrap (lacking a the linear ion trap in front of the Orbitrap analyzer), which actually does not cost more than a QqQ mass spectrometer, still matches all the demands of an high resolution mass spectrometer [fast scanning (up to 10 Hz), high resolution (up to 100,000 R), and accurate mass (<2 ppm)]. The combination of these attributes therefore allows one not only to distinguish compounds with very similar masses, but also to directly annotate elemental compositions, without a need for a reference compound, based on the measured accurate masses (Giavalisco et al., 2008; Xu et al., 2010).
Each lipid extract was separated and measured twice, once in positive ionization (Figure 1A) and once in negative ionization mode (Figure 1B). The reason for this duplicated measurement can be easily seen by looking at the two chromatograms, as they appear quite different. The explanation for this difference comes from the chemical nature of the detected lipid species (Han and Gross, 2005; Devaiah et al., 2006). Even though all of these lipids are constructed from a small number of building blocks (a glycerol backbone linked to a number of fatty acids), their general mass spectrometric behavior is controlled by the chemical property of their class-specific head group (Yang et al., 2009). Accordingly, even though most of these lipids ionize in both ionization modes, they do have a clear bias for a specific adduct and, as a consequence, a specific polarity (Table 1).
Figure 1. Ultra performance liquid chromatography chromatograms and selected mass spectra from Arabidopsis thaliana leaf lipid extracts. (A) Total ion chromatogram (TIC, upper part) of mass spectra recorded in positive ion mode. The lower part shows the mass spectrum from the apex of the MGDG 34:6 peak with the retention time of 7.08 min and its associated ionization adducts. (B) As above, but here the TIC and the spectrum of the negative ion mode measurements are shown.
For example, monogalactosyldiacylglycerol (MGDG) 34:6 can be detected with three different adducts in the positive ionization mode ([M + H], [M + NH4], and [M + Na]) and another two adducts can be detected in the negative ionization mode ([M − H], [M + Acetate − H]). The appearance of these multiple adducts proves to be an extremely useful feature, even if it increases the spectral complexity, since it improves the analysis and the correct annotation of the measured lipid classes. As can be seen in Figure 1A, peak pairs with precise distances can be identified. A difference of m/z 21.98 (±5 ppm) indicates a [M + H] and a [M + Na] ion pair, while distances of m/z 17.02 (±5 ppm) indicate a [M + H] and a [M + NH4] ion pair (Figure 1A).
The correct adduct annotation is of particular importance, especially if looking at lipids where the different adducts might have very similar (or even identical) masses. One example for such a case is given in Figure 2 for a phosphatidylserine (PS) and phosphatidylglycerol (PG) lipid. As the protonated PS 34:2 is only 0.02 ppm different from the ammonium adduct of PG 34:4, which means that for the mass of 760.51385 ± 5 ppm we will get two lipid peaks from our positive mode spectrum. Looking at the adduct patterns of the spectra (including also the negative ion mode spectra), helps to solve the above mentioned annotation dilemma for these two compounds, since only the peak with a retention time of 7.17 min pairs to a sister peak with a distance of 17.02, which indicates that this peak is the ammonium adduct of PG 34:4, while the peak at RT 7.97 min can be annotated as the PS 34:2.
Figure 2. Positive and negative ion mode spectra and adduct annotations of PG 34:4 (red boxed) and PS 34:2 (blue boxed).
In almost all cases lipidomics studies performed in the plant field were conducted in a targeted way, meaning that a number (a few dozen to several 100) expected lipids species were profiled (Devaiah et al., 2006; Markham and Jaworski, 2007). To validate our system, we decided to profile the lipids from these previously conducted studies by selectively extracting the expected masses from our chromatograms. In total we prepared a target list containing 332 different lipid species types [168 sphingolipids (Markham and Jaworski, 2007), 147 phosphoglycero- or galacto-lipids (Devaiah et al., 2006), and 17 oxylipin species (Buseman et al., 2006)], which were detected in three independent studies, using three different extraction protocols, and three different types of mass spectrometers. As illustrated in Figure 3A we conducted the peak extraction by simply extracting each single mass associated to a specific lipid and relatively quantified the intensity of the different adducts from each chromatogram (Table S1 in Supplementary Material). In the same way it is also possible to extract several masses, belonging to different lipids, within a specific lipids class or from different classes, and quantitatively compare them to each other in parallel (e.g., whole PC 36: 1–6 and PC 34: 1–6 series is displayed in Figure 3B).
Figure 3. Extracted ion chromatograms of a single lipid species [PC 36:6 (A)] or a whole series of lipids [PCs (B)]. The spectra were recorded in and extracted from positive ion mode lipid chromatograms (Figure 1A).
By manually extracting the masses from the chromatograms we matched 187 of the 332 different lipids, including 127/147 of the previously described phospho-, lysophospho-, and galacto-lipids (Devaiah et al., 2006), all 17 of the 17 previously described oxylipins (Buseman et al., 2006), and 43 of the 168 possible sphingolipids (Markham and Jaworski, 2007). Compared to the excellent coverage of lipid species from the phosphoglycero and galacto lipids the result achieved for the sphingolipids were less comprehensive, only covering the most abundant lipid species from the Markham and Jaworski (2007) study. This indicated that we were not having a general loss of sphingolipids in our method, but rather a sensitivity problem, which can often be observed if ion trap-like mass spectrometers are compared to QqQ-type mass spectrometers (Mcluckey and Wells, 2001). Additionally, we noticed that the sample preparation method used in the sphingolipid study was highly sophisticated and specifically tailored to this lipid class, including a depletion step of the highly abundant phospholipids, which will lead to a higher detection sensitivity due to strongly decreased ion suppression effects (Markham and Jaworski, 2007).
Taken together we can conclude that we do see most of the expected lipid species in our samples and most of them with several different ion species (different adducts). The data of these initially extracted and validated lipid species is collected in Table S1 in Supplementary Material.
Confidence in the annotation of a measured compound can be increased with the number of parameters this compound shares with related compounds. Since lipids are constructed as modular molecules (Fahy et al., 2009; Yang et al., 2009), which usually vary only slightly between the different species within a lipid class (extension of the fatty acid chain length or the degree of saturation), they have a very systematic mass and retention time behavior (Hermansson et al., 2005). Therefore, both these parameters allows the validation of lipids within a specific class by simply plotting the m/z and RT values of the measured species of the most abundant adduct in a scatter plot. As can be seen for Figure 4 (scatter plot for the measured PCs from Table S1 in Supplementary Material), the lipids with longer fatty acid chains lead to a higher mass and increased retention time, while fatty acids with higher degrees of un-saturation result in lipids with lower masses and decreased retention times. As a consequence, a diagonal series appears within the plots. These contain lipid species with the same number of carbons atoms in the fatty acid chains but show decreasing number of double bonds from left to right (Figure 4). Wrongly annotated or unusually distributed lipids can be easily detected within these patterns since they appear as dots outside the systematic scatter pattern. A curious and unexplained example is given for the PCs with 40 carbons in the two fatty acid chains (Figure 4). Even though these lipids are systematically distributed by themselves, it is evident from the plot that they are not matching the distribution of the other, shorter fatty acid chain lipids in this lipid class. The PC 40:2 for example, which would be predicted to have a later elution time than the PC 38:2, does actually elute almost a minute earlier than the shorter chain classmate (Figure 4). This could indicate that the PC 40:X lipids have been either annotated wrongly or there is a systematic shift in these longer fatty acid chain lipids.
Figure 4. Scatter plot of all lipids annotated as phosphocholines (Table S2 in Supplementary Material). The plot contains the measured retention time in minutes on the x-axes and the recorded mass of the [M + H]+ adduct on the y-axes. Due to the modular building block structure of lipids within a homogenous class, systematic patterns of parallel lines should be observed. From bottom to top these lines should contain lipids with increasing fatty acid chain length, while the number of double bond should decrease from left to right. The star under PC 38:1 indicates that this lipid was not detected in the analyzed samples, but it would have been expected at this retention time. The PC 40:X series is highlighted since these compounds seem to elute too early and therefore do not match the expected elution pattern given by the whole class.
Next to the exclusion of possibly wrongly annotated lipids, the scatter plot representation allows one to also quickly detect missing lipid species within a systematic series. In this case one or several dots would be missing within the diagonal line. In Figure 4 we can see for example that we could not detect PC 38:1. Even rechecking the spectra at the expected retention time did not allow us to detect the expected peak.
Using high resolution accurate mass data is in many cases sufficient to predict an elemental composition of a measured peak (Giavalisco et al., 2009). Still the accuracy and probability for a correct annotation is increased if along with the accurate mass of the intact molecule (precursor) an additional mass of a compound-specific fragment can be detected. The measurement of the mass of the intact precursor and one or several fragments are the essential values for the peak identification in shotgun lipidomic analysis (Han and Gross, 2005). The occurrence of these specific fragment ions results from either a specific loss of a charged molecule (e.g., choline head group from PC lipids) or from the loss of an uncharged fragment (neutral loss). This technique can also be used on LC–MS-based systems in non-shotgun lipidomic studies, but only if fragmentation mass spectra are recorded.
The main advantage of high pressure sub 2 μm particle UPLC systems, compared to conventional, lower pressure, larger particle HPLC systems, is its fast, sensitive, and highly reproducible chromatography (Plumb et al., 2004). The faster chromatography and the smaller peak width, which is a consequence of the higher plate number achieved in the UPLC system, turns into a disadvantage when the number of scans/time of the mass spectrometer are too low to perform the survey full scans and data-dependent MS/MS measurements of the most abundant peaks (Schmitt-Kopplin et al., 2008). The FT–MS instrument used in this study, which has a scan speed of up to 10 Hz at a resolution of 10,000 can circumvent this problem partially, but still, even 10 scans/s are not enough time to perform classical data-dependent MS/MS analysis of several eluting masses while recording sufficient information for good peak integration, especially if the eluting peaks are only 3–6 s long (Figure 3A). The solution for this problem, which has originally been developed and implemented under the name MSe as a scan method for qTOF mass spectrometers (Bateman et al., 2007), and simply relies on the fragmentation of all precursor ions measured in the full scan instead of selecting individual masses. This approach has successfully been used in a proteomic study in the Exactive MS and was called all-ion fragmentation (Geiger et al., 2010). In Figure 5A an illustration of the measurement method used for our lipidomic analysis is given, showing that we constantly alter between low energy full scans and high energy all-ion fragmentation scans throughout the whole chromatographic separation. The advantage of this procedure is that two independent MS data-sets are generated, one contains the intact mass information for all the compounds eluting during the chromatographic separation, while the second contains the fragmentation data for the selfsame compounds. To integrate this data and to validate a predicted lipid it is only necessary to connect the elution profile of a full scan (low energy) mass to the similarly eluting masses from the all-ion MS/MS (high energy) spectra. In Figure 5B this procedure is illustrated for PC 36:6. As can be seen, three fragment masses (m/z 184.07381, m/z 500.31598, and m/z 518.32513) within the mass spectra between 7.2 and 7.8 min are exactly co-eluting to the phosphocholine lipid (m/z 778.53894) and should therefore be associated. Another two masses (m/z 728.52446 and m/z 573.48822), which are closely co-eluting, show clearly differential elution profiles and can therefore excluded to be associated to PC36:6, indicating that they should represent different lipids.
Figure 5. Ultra performance liquid chromatography–MS measurement strategy employed for the lipid analysis in this study: (A) illustration of high and low energy alteration for the acquisition of full scan and all-ion MS/MS spectra. (B) Extracted ion chromatograms of the indicated masses (derived either from the high or low energy mass spectra) from a representative positive ion modes UPLC chromatogram. Peaks with the same elution profile can be regarded as co-eluting masses, which are derived from the same precursor molecule. Differentially eluting peaks have to be regarded as different compounds, requiring different annotations.
The systematic analysis of these all-ion MS/MS spectra therefore allows us to uncover a number of lipid specific fragments, which can be used to validate a specific lipid species, e.g., the masses m/z 500.31598 and m/z 518.32513, which are specific fragments of PG 36:6 (Figure 5B). As well, we can also find class-specific fragments, like the m/z 184.07381, which is the positively charged choline fragment that can be detected for all phosphocholine lipids.
The strategy presented for the analysis of lipids thus far still requires a high manual input, especially for the validation of the lipid annotation. Of course this is only true if a novel sample (a new organism or a new tissue) is analyzed. Once a sample is annotated and no major changes in the extraction procedure or the chromatographic separation are introduced, the following lipid profiles can be simply matched to the results of the initially performed peak annotation.
The chromatographic and the spectral compatibility between different samples, namely the retention time and the spectral intensities, are achieved by using the two internal standards (PE 34:0 and PC 34:0), which we have spiked into the extraction buffer. Increasing the number of internal standards might be useful in the long run if the retention time system needs to be converted into a retention index system, which would possibly allow one to not only match lipids within a single experiment, but also between different experiments.
After having annotated the initial expected lipids from a novel matrix the data analysis can be automated by using one of the two different strategies depicted in Figure 6. The main distinction between the two approaches lies in the fact that one strategy directly targets only the peaks of interest by selectively extracting the masses of lipids of interest at specific retention times from the generated chromatograms (left part of Figure 6), while the second strategy relies on a slightly different approach. Here, all the peaks from the chromatograms are extracted and aligned into a data matrix before matching these peaks to the m/z and RT values of an annotated peak list (right part of Figure 6). The result in both cases should be almost identical. The major difference between the two approaches lies in the fact that in the first approach only annotated peaks can be used for the analysis, while the second approach allows for the further use of an un-annotated matrix, derived from the peak picking software, providing the basis for fully un-targeted lipidomics.
Figure 6. Automated, software-assisted, strategies for targeted but also un-targeted lipid profiling. On the left hand side a purely targeted strategy is depicted, where based on a target list several chromatograms are searched for the occurrence of specific m/z and retention times. If a peak is found, within certain tolerance boundaries, the intensity is loaded to a result table. The strategy on the right hand side indicates a diverse strategy. Here all peaks are extracted first and these are written into an un-annotated data matrix. This matrix can then be compared against a target list (same as for the first strategy) or used for statistical analysis of significantly differential peaks, which then have to be annotated.
For the purpose of targeted peak picking (left part of Figure 6), software is usually provided by the vendor of the mass spectrometer. This software can be used by uploading a target list containing the name, the m/z, and the RT of the peaks of interest. This target list is then used to query the chromatograms generated during the analysis. The output of such a search is a list where every peak of interest is associated to the compound name, the measured m/z and RT, and an intensity value, which is equivalent to the relative amount of the compound within the sample. For the analysis of Exactive or other Thermo-Fisher MS data two software packages are available: either a processing method [which has to be entered compound by compound within Xcalibur (Thermo-Fisher, Bremen, Germany)] can be generated, or if the ToxID software package (Thermo-Fisher) is used, a comma separated text file can be employed for the targeted analysis of the lipidomic data.
For the purpose of targeted, but also un-targeted data analysis (right part of Figure 6), peak picking and matrix alignment of all peaks is necessary first. Here several commercial, but also open source software packages are available (Katajamaa et al., 2006; Smith et al., 2006; Katajamaa and Oresic, 2007; Benton et al., 2008; Lommen, 2009; Pluskal et al., 2010). Once the initial, un-annotated matrix is generated from a suitable software package, this matrix can be further filtered and compared to the previously generated reference lists.
Usually a matrix from Arabidopsis leaf tissue contains 30,000 or more reproducible peaks which are above a minimal threshold of 10,000 counts (data not shown). The difference in dimensions between the target list and the global matrix already indicates that even though we are mining a significant portion of lipids from these samples (200–300 lipid species, Tables S1 and S2 in Supplementary Material), the majority of the detectable peaks remains un-annotated.
As shown in Figure 6 the un-targeted global matrix, which contains all the extractable peaks from the recorded mass spectra, can be compared against a reference list of annotated compounds. The size and the content of these lists can vary significantly: therefore one can use the reference list generated in this study (Table S1 in Supplementary Material) or other more comprehensive customer made lists. Furthermore public and commercial databases like, e.g., the Lipid Maps (Fahy et al., 2005, 2009), the KNApSAcK (Shinbo et al., 2006), KEGG (Kanehisa et al., 2008), PubChem (Wang et al., 2009), or ChemSpider (Williams, 2008) can be employed for even more comprehensive or specific database searches. The problem with these comparisons is that first of all not all these databases are easily accessible, but also even if they are, it still requires experience and personal effort with appropriate tools to compile these databases into a suitable resource. For this purpose we decided to develop a distributed client-server application utilizing a graphical user interface which supports the matching of measured masses to elemental compositions deposited in a relational database and make this tool publicly available.
We named this software GoBioSpace (for Golm Biochemical Space), which can be installed on Microsoft Windows XP Service Pack 3 and later desktop computers using the ClickOnce deployment6. The database server is accessed in-house directly using ADO.NET7, while internet users fall back to WSDL-based [W3C (2001) Web Services Description Language (WSDL)8] web services9.
The main functionality of GoBioSpace is to compare measured masses from mass spectrometric measurements, now including all kind of mass spectrometric data (high accurate mass but also lower mass accuracy), against a single or several databases (see Materials and Methods). As illustrated in Figure 7, the workflow for the data analysis is simple: a single mass or an elemental composition, but also a list of masses or formulas (tab-delimited text file) can be loaded into the software and searched against a single or several databases (at the moment more than 150 public databases are hosted, including the whole PubChem collection). Prior to the database search a number of parameters have to be specified, including the possible adducts of the measured mass (e.g., [M + H]+, [M + Na]+, [M + NH4]+, [M − 2H]2−, [M − Acetate + H]−), the mass accuracy of the entered data, and finally a selection of elements expected to be contained in the matching compounds. The database search by itself (the in-house version) is quite fast and can process easily 2,000 searches per second, meaning that even a large list containing 30,000 peaks is processed within 15 s. However, reasoned by the increased complexity of protocol layers utilizing xml (eXtensible Markup Language)10 and http (Hypertext Transport Protocol)11 for data encapsulation and transport over the internet, we expect the performance of the internet version to fall below this value, also depending on the final capacity of the web and database servers. The output format of the result list, which is again a tab-delimited text file, contains all the information contained in the input table (measured m/z, RT and intensity of the measured peaks) added by the possible elemental composition of the measured mass, the adduct used to match measured and calculated mass, the database this hit was derived from, one or several compound name(s) if specified within the selected databases, and the mass error between the measured mass and the matched hit.
Figure 7. Overview with screenshots of the GoBioSpace-assisted database search procedure. The workflow is separated in three steps: data input (single mass, mass list, or formula), specification of search criteria (databases, expected mass adducts, mass error tolerance, expected elements, and isotope label), and data output.
To re-validate our chromatographic data we searched the 30,000 peaks against an in-house assembled lipid database containing approximately 1,000 entries. This table contained the previously described lipids profiled in Arabidopsis thaliana lipids samples (Buseman et al., 2006; Devaiah et al., 2006; Markham et al., 2006; Markham and Jaworski, 2007; Glauser et al., 2008a,b), but also a large set of other lipid species including sterols (Benveniste, 2004; Hemmerlin et al., 2004), several di- and tri-acylglycerols, fatty acids, chlorophylls (Tanaka and Tanaka, 2006), and other plant pigments (Grotewold, 2006).
This database search resulted initially in a list of more than 4,000 hits for the positive mode spectra and 1,500 hits for the negative mode spectra. After correcting for the accurate adducts (Table 1) but also the expected retention times of the expected lipids within their lipid classes (Table S1 in Supplementary Material) we annotated, still very conservatively, 577 distinct peaks which were annotated to 265 unique elemental compositions (Tables S1 and S2 in Supplementary Material). Still, the number of hits within the already highly targeted database search seems to promise that this data-set contains many more compounds awaiting a proper annotation.
For overview purposes and to visualize the annotated data we mapped all the annotated lipids from Table S2 in Supplementary Material into a scatter plot (Figure A1 in Appendix) and the different lipid classes and their distribution within the positive mode UPLC chromatogram (Figure 8).
Figure 8. Positive ion mode chromatogram from Figure 1A delineating the retention time areas of the different detected lipids from Table S2 in Supplementary Material.
The most common approach for systematic lipid profiling is still the well-established shotgun lipidomic approach (Han et al., 2005; Welti et al., 2007b; Yang et al., 2009), which was conceptually developed more than 15 years ago (Han and Gross, 1994). Due to this fact, there are several publications available (including comprehensive plant studies), which either made directly use of the QqQ approach (Welti and Wang, 2004; Devaiah et al., 2006; Welti et al., 2007b) or modified it for the use on different mass spectrometers like qTOF (Ekroos et al., 2002; Ejsing et al., 2006; Esch et al., 2007) or the Orbitrap (Yang et al., 2007. As a consequence different commercial and open source software packages were developed to make use of this kind of data (Ejsing et al., 2006 #127; Graessler et al., 2009; Yang et al., 2009; Herzog et al., 2011).
The developments and the application of LC–MS lipidomics, especially in the plant field, seems to be less popular, even though a number of groups developed different open source software packages for these applications (Haimi et al., 2006, 2009; Taguchi and Ishikawa, 2010; Nygren et al., 2011). The lack of absolute quantification, or better the lack of control of ion suppression in LC–MS-based lipidomic studies and the increased analytical complexity seem to be the main reasons for this discrepancy (Stahlman et al., 2009).
Ion suppression in shotgun lipidomic studies cannot be eliminated, even if lipid class-specific internal standards are used. The function of these internal standards is basically to corrected for the differential suppression effects on each measured lipid molecule (Stahlman et al., 2009; Yang et al., 2009). Making use of mixtures of internal standards (in best case using one or two standard lipids per lipid class (Welti and Wang, 2004)) for LC–MS-based lipidomic studies could be possible if these mixtures are spiked in the eluting sample post-column, using a second pump and a t-connection. Such an on-line LC–MS approach using continuously infused internal standards at low concentrations, which has not been demonstrated yet, would definitely be an excellent compromise between complicated and time consuming off-line sample pre-fractionation (Stahlman et al., 2009), and the use of strongly ion-suppressed shotgun lipidomics. Our developed system could therefore provide an excellent test case for such an approach.
Alternatively, the use of fully labeled metabolomes or lipidomes (Ekroos et al., 2002; Hegeman et al., 2007; Giavalisco et al., 2008, 2009) could be an alternative way to quantify and annotate lipids in LC–MS-based studies. For this purpose analytical samples will be spiked with the same amount of the isotope-labeled matrix (Giavalisco et al., 2009). This approach, which has been tested by us (data not shown), is of course more complicated and expensive than the post-column spiking with a handful of reference compounds, but next to the relative quantification, it will also allow the reliable annotation of previously unknown compounds (Giavalisco et al., 2008, 2009).
One of the biggest differences between targeted and un-targeted lipid analysis lies in the fact that even though a number of 150 profiled and quantified lipids enables a meaningful analysis of an organism (Ejsing et al., 2009), there still remain many unidentified peaks to be annotated before we can really call it a lipidomic analysis. Looking at the data from our study already shows that of the 30,000 extractable peaks “only” 577 were annotated to a compound by using a targeted approach (Table S2 in Supplementary Material). Increasing the size of the employed databases would therefore directly provide a larger number of possible annotations, but this comes, in dependence of the database size used for the annotation, at the price of also annotating more false positives (Matsuda et al., 2009). Here the use of additional, orthogonal, physico-chemical properties can increase the validation of the recorded data. While the use of fragmentation data will greatly help to exclude false positives, also the use of the retention time information will improve the predictability of an annotation, which strongly argues in favor of LC–MS-based lipidomics (Figures 4 and 8).
Another advantage of LC–MS-based lipidomics in combination with global, un-targeted peak extraction lies in the statistically analyzed whole data-set consisting of 30,000 peaks prior to peak annotation. As a consequence, only the differential peaks would be regarded as potentially interesting and therefore subjected to more sophisticated peak annotation strategies. The annotation strategy could include isotope-labeling (see above) or analytical preparation techniques, including peak collection from the chromatographic run and subsequent analysis using higher order MS/MS, analysis on a high resolution mass spectrometer (Schwudke et al., 2007), or other orthogonal analytical techniques such as NMR.
High resolution full scan and all-ion fragmentation spectra containing thousands of peaks are not only a rich source of biological information for a “one-pass” analysis but could serve as a repository of information, which can be reused with new knowledge repeatedly.
We demonstrated in our study that the use of targeted data, derived from a limited number of plant lipidomic studies (Buseman et al., 2006; Devaiah et al., 2006; Esch et al., 2007; Markham and Jaworski, 2007; Welti et al., 2007a,b; Glauser et al., 2008a,b), allowed us to profile and annotate more than 260 lipid species. Increasing the list of targets by annotating novel lipid species, or simply checking literature for previously un-targeted lipids like N-acyl phosphatidylethanolamines (NAPE) and more complex sphingolipids (Welti and Wang, 2004), or tetra galactolipids (Moreau et al., 2008), will increase the length of the list of lipids which can be profiled. This includes the repercussive profiling of old data. Therefore, in the future more knowledge about thus far unidentified lipid moieties will allow us to annotate and profile more and more lipid species; we will not have to rerun all of our old experiments, since we can simply revisit our old high resolution chromatograms and reexamine them. This cannot be done using shotgun lipidomics with highly sensitive, but low resolution mass spectrometers.
The authors wish to thank Prof. Dr. Lothar Willmitzer for discussions, support, and the opportunity to develop the project. Furthermore we would like to thank Dr. Leonard Krall for proof reading and commenting on the manuscript. Antony Williams is acknowledged for providing data from ChemSpider, while Drs. Dirk Walther and Joachim Kopka are acknowledged for hosting the GoBioSpace DB in the frame of the Golm Metabolome Database (GMD), which is currently funded by the German Research Foundation (Deutsche Forschungsgemeinschaft, DFG) under the LIS-program call Information Infrastructures for Research Projects, grant WA 1285/2-1 “Development of the Golm Metabolome Database as a central plant metabolomics information resource.” Last but not least Änne Eckardt and Gudrun Wolter are most kindly acknowledged for the outstanding technical support.
The authors declare that the research was conducted in the absence of any commercial or financial relationships that could be construed as a potential conflict of interest.
Bateman, K. P., Castro-Perez, J., Wrona, M., Shockcor, J. P., Yu, K., Oballa, R., and Nicoll-Griffith, D. A. (2007). MSE with mass defect filtering for in vitro and in vivo metabolite identification. Rapid Commun. Mass Spectrom. 21, 1485–1496.
Benton, H. P., Wong, D. M., Trauger, S. A., and Siuzdak, G. (2008). XCMS2: processing tandem mass spectrometry data for metabolite identification and structural characterization. Anal. Chem. 80, 6382–6389.
Benveniste, P. (2004). Biosynthesis and accumulation of sterols. Annu. Rev. Plant Biol. 55, 429–457.
Blanksby, S. J., and Mitchell, T. W. (2010). Advances in mass spectrometry for lipidomics. Annu. Rev. Anal. Chem. (Palo Alto Calif.) 3, 433–465.
Böhlke, J. K., De Laeter, J. R., De Bièvre, P., Hidaka, H., Peiser, H. S., Rosman, K. J. R., and Taylor, P. D. P. (2005). Isotopic compositions of the elements, 2001. J. Phys. Chem. Ref. Data 34, 57–67.
Buseman, C. M., Tamura, P., Sparks, A. A., Baughman, E. J., Maatta, S., Zhao, J., Roth, M. R., Esch, S. W., Shah, J., Williams, T. D., and Welti, R. (2006). Wounding stimulates the accumulation of glycerolipids containing oxo phytodienoic acid and dinor-oxophytodienoic acid in Arabidopsis leaves. Plant Physiol. 142, 28–39.
Chen, M., Markham, J. E., Dietrich, C. R., Jaworski, J. G., and Cahoon, E. B. (2008). Sphingolipid long-chain base hydroxylation is important for growth and regulation of sphingolipid content and composition in Arabidopsis. Plant Cell 20, 1862–1878.
Dennis, E. A. (2009). Lipidomics joins the omics evolution. Proc. Natl. Acad. Sci. U.S.A. 106, 2089–2090.
Devaiah, S. P., Pan, X., Hong, Y., Roth, M., Welti, R., and Wang, X. (2007). Enhancing seed quality and viability by suppressing phospholipase D in Arabidopsis. Plant J. 50, 950–957.
Devaiah, S. P., Roth, M. R., Baughman, E., Li, M., Tamura, P., Jeannotte, R., Welti, R., and Wang, X. (2006). Quantitative profiling of polar glycerolipid species from organs of wild-type Arabidopsis and a phospholipase Dalpha1 knockout mutant. Phytochemistry 67, 1907–1924.
Ejsing, C. S., Duchoslav, E., Sampaio, J., Simons, K., Bonner, R., Thiele, C., Ekroos, K., and Shevchenko, A. (2006). Automated identification and quantification of glycerophospholipid molecular species by multiple precursor ion scanning. Anal. Chem. 78, 6202–6214.
Ejsing, C. S., Sampaio, J. L., Surendranath, V., Duchoslav, E., Ekroos, K., Klemm, R. W., Simons, K., and Shevchenko, A. (2009). Global analysis of the yeast lipidome by quantitative shotgun mass spectrometry. Proc. Natl. Acad. Sci. U.S.A. 106, 2136–2141.
Ekroos, K., Chernushevich, I. V., Simons, K., and Shevchenko, A. (2002). Quantitative profiling of phospholipids by multiple precursor ion scanning on a hybrid quadrupole time-of-flight mass spectrometer. Anal. Chem. 74, 941–949.
Esch, S. W., Tamura, P., Sparks, A. A., Roth, M. R., Devaiah, S. P., Heinz, E., Wang, X., Williams, T. D., and Welti, R. (2007). Rapid characterization of the fatty acyl composition of complex lipids by collision-induced dissociation time-of-flight mass spectrometry. J. Lipid Res. 48, 235–241.
Fahy, E., Subramaniam, S., Brown, H. A., Glass, C. K., Merrill, A. H. Jr., Murphy, R. C., Raetz, C. R., Russell, D. W., Seyama, Y., Shaw, W., Shimizu, T., Spener, F., Van Meer, G., Vannieuwenhze, M. S., White, S. H., Witztum, J. L., and Dennis, E. A. (2005). A comprehensive classification system for lipids. J. Lipid Res. 46, 839–861.
Fahy, E., Subramaniam, S., Murphy, R. C., Nishijima, M., Raetz, C. R., Shimizu, T., Spener, F., Van Meer, G., Wakelam, M. J., and Dennis, E. A. (2009). Update of the LIPID MAPS comprehensive classification system for lipids. J. Lipid Res. 50, S9–S14.
Geiger, T., Cox, J., and Mann, M. (2010). Proteomics on an Orbitrap benchtop mass spectrometer using all-ion fragmentation. Mol. Cell Proteomics 9, 2252–2261.
Giavalisco, P., Hummel, J., Lisec, J., Inostroza, A. C., Catchpole, G., and Willmitzer, L. (2008). High-resolution direct infusion-based mass spectrometry in combination with whole 13C metabolome isotope labeling allows unambiguous assignment of chemical sum formulas. Anal. Chem. 80, 9417–9425.
Giavalisco, P., Kohl, K., Hummel, J., Seiwert, B., and Willmitzer, L. (2009). 13C isotope-labeled metabolomes allowing for improved compound annotation and relative quantification in liquid chromatography-mass spectrometry-based metabolomic research. Anal. Chem. 81, 6546–6551.
Giavalisco, P., Li, Y., Matthes, A., Eckhardt, A., Hubberten, H. M., Hesse, H., Segu, S., Hummel, J., Köhl, K., and Willmitzer, L. (2011). Elemental formula annotation of polar and lipophilic metabolites using (13) C, (15) N and (34) S isotope labelling, in combination with high-resolution mass spectrometry. Plant J. doi: 10.1111/j.1365-313X.2011.04682.x. [Epub ahead of print].
Glauser, G., Grata, E., Dubugnon, L., Rudaz, S., Farmer, E. E., and Wolfender, J. L. (2008a). Spatial and temporal dynamics of jasmonate synthesis and accumulation in Arabidopsis in response to wounding. J. Biol. Chem. 283, 16400–16407.
Glauser, G., Grata, E., Rudaz, S., and Wolfender, J. L. (2008b). High-resolution profiling of oxylipin-containing galactolipids in Arabidopsis extracts by ultra-performance liquid chromatography/time-of-flight mass spectrometry. Rapid Commun. Mass Spectrom. 22, 3154–3160.
Graessler, J., Schwudke, D., Schwarz, P. E., Herzog, R., Shevchenko, A., and Bornstein, S. R. (2009). Top-down lipidomics reveals ether lipid deficiency in blood plasma of hypertensive patients. PLoS ONE 4, e6261. doi: 10.1371/journal.pone.0006261
Griffiths, W. J., and Wang, Y. (2009). Mass spectrometry: from proteomics to metabolomics and lipidomics. Chem. Soc. Rev. 38, 1882–1896.
Grotewold, E. (2006). The genetics and biochemistry of floral pigments. Annu. Rev. Plant Biol. 57, 761–780.
Haimi, P., Chaithanya, K., Kainu, V., Hermansson, M., and Somerharju, P. (2009). Instrument-independent software tools for the analysis of MS-MS and LC-MS lipidomics data. Methods Mol. Biol. 580, 285–294.
Haimi, P., Uphoff, A., Hermansson, M., and Somerharju, P. (2006). Software tools for analysis of mass spectrometric lipidome data. Anal. Chem. 78, 8324–8331.
Han, X., and Gross, R. W. (1994). Electrospray ionization mass spectroscopic analysis of human erythrocyte plasma membrane phospholipids. Proc. Natl. Acad. Sci. U.S.A. 91, 10635–10639.
Han, X., and Gross, R. W. (2005). Shotgun lipidomics: electrospray ionization mass spectrometric analysis and quantitation of cellular lipidomes directly from crude extracts of biological samples. Mass Spectrom. Rev. 24, 367–412.
Han, X., Yang, K., Cheng, H., Fikes, K. N., and Gross, R. W. (2005). Shotgun lipidomics of phosphoethanolamine-containing lipids in biological samples after one-step in situ derivatization. J. Lipid Res. 46, 1548–1560.
Harkewicz, R., and Dennis, E. A. (2011). Applications of mass spectrometry to lipids and membranes. Annu Rev Biochem. 80, 301–325.
Hegeman, A. D., Schulte, C. F., Cui, Q., Lewis, I. A., Huttlin, E. L., Eghbalnia, H., Harms, A. C., Ulrich, E. L., Markley, J. L., and Sussman, M. R. (2007). Stable isotope assisted assignment of elemental compositions for metabolomics. Anal. Chem. 79, 6912–6921.
Hemmerlin, A., Gerber, E., Feldtrauer, J. F., Wentzinger, L., Hartmann, M. A., Tritsch, D., Hoeffler, J. F., Rohmer, M., and Bach, T. J. (2004). A review of tobacco BY-2 cells as an excellent system to study the synthesis and function of sterols and other isoprenoids. Lipids 39, 723–735.
Hermansson, M., Uphoff, A., Kakela, R., and Somerharju, P. (2005). Automated quantitative analysis of complex lipidomes by liquid chromatography/mass spectrometry. Anal. Chem. 77, 2166–2175.
Herzog, R., Schwudke, D., Schuhmann, K., Sampaio, J. L., Bornstein, S. R., Schroeder, M., and Shevchenko, A. (2011). A novel informatics concept for high-throughput shotgun lipidomics based on the molecular fragmentation query language. Genome Biol. 12, R8.
Huege, J., Sulpice, R., Gibon, Y., Lisec, J., Koehl, K., and Kopka, J. (2007). GC-EI-TOF-MS analysis of in vivo carbon-partitioning into soluble metabolite pools of higher plants by monitoring isotope dilution after (13)CO(2) labelling. Phytochemistry 68, 2258–2272.
Hummel, J., Strehmel, N., Selbig, J., Walther, D., and Kopka, J. (2010). Decision tree supported substructure prediction of metabolites from GC-MS profiles. Metabolomics 6, 322–333.
Kanehisa, M., Araki, M., Goto, S., Hattori, M., Hirakawa, M., Itoh, M., Katayama, T., Kawashima, S., Okuda, S., Tokimatsu, T., and Yamanishi, Y. (2008). KEGG for linking genomes to life and the environment. Nucleic Acids Res. 36, D480–D484.
Katajamaa, M., Miettinen, J., and Oresic, M. (2006). MZmine: toolbox for processing and visualization of mass spectrometry based molecular profile data. Bioinformatics 22, 634–636.
Katajamaa, M., and Oresic, M. (2007). Data processing for mass spectrometry-based metabolomics. J. Chromatogr. A 1158, 318–328.
Kilaru, A., Isaac, G., Tamura, P., Baxter, D., Duncan, S. R., Venables, B. J., Welti, R., Koulen, P., and Chapman, K. D. (2010). Lipid profiling reveals tissue-specific differences for ethanolamide lipids in mice lacking fatty acid amide hydrolase. Lipids 45, 863–875.
Kopka, J., Schauer, N., Krueger, S., Birkemeyer, C., Usadel, B., Bergmüsller, E., Dörmann, P., Weckwerth, W., Gibon, Y., Stitt, M., Willmitzer, L., Fernie, A. R., and Steinhauser, D. (2005).R01EQENTQi5EQg==: the Golm Metabolome Database. Bioinformatics 21, 1635–1638.
Kuksis, A. (2007). Lipidomics in triacylglycerol and cholesteryl ester oxidation. Front. Biosci. 12, 3203–3246.
Lommen, A. (2009). MetAlign: interface-driven, versatile metabolomics tool for hyphenated full-scan mass spectrometry data preprocessing. Anal. Chem. 81, 3079–3086.
Lu, W., Bennett, B. D., and Rabinowitz, J. D. (2008). Analytical strategies for LC-MS-based targeted metabolomics. J. Chromatogr. B Analyt. Technol. Biomed. Life Sci. 871, 236–242.
Lu, W., Clasquin, M. F., Melamud, E., Amador-Noguez, D., Caudy, A. A., and Rabinowitz, J. D. (2010). Metabolomic analysis via reversed-phase ion-pairing liquid chromatography coupled to a stand alone orbitrap mass spectrometer. Anal. Chem. 82, 3212–3221.
Markham, J. E., and Jaworski, J. G. (2007). Rapid measurement of sphingolipids from Arabidopsis thaliana by reversed-phase high-performance liquid chromatography coupled to electrospray ionization tandem mass spectrometry. Rapid Commun. Mass Spectrom. 21, 1304–1314.
Markham, J. E., Li, J., Cahoon, E. B., and Jaworski, J. G. (2006). Separation and identification of major plant sphingolipid classes from leaves. J. Biol. Chem. 281, 22684–22694.
Matsuda, F., Shinbo, Y., Oikawa, A., Hirai, M. Y., Fiehn, O., Kanaya, S., and Saito, K. (2009). Assessment of metabolome annotation quality: a method for evaluating the false discovery rate of elemental composition searches. PLoS ONE 4, e7490. doi: 10.1371/journal.pone.0007490
Matyash, V., Liebisch, G., Kurzchalia, T. V., Shevchenko, A., and Schwudke, D. (2008). Lipid extraction by methyl-tert-butyl ether for high-throughput lipidomics. J. Lipid Res. 49, 1137–1146.
Mcluckey, S. A., and Wells, J. M. (2001). Mass analysis at the advent of the 21st century. Chem. Rev. 101, 571–606.
Merrill, A. H. Jr., Stokes, T. H., Momin, A., Park, H., Portz, B. J., Kelly, S., Wang, E., Sullards, M. C., and Wang, M. D. (2009). Sphingolipidomics: a valuable tool for understanding the roles of sphingolipids in biology and disease. J. Lipid Res. 50, S97–S102.
Moore, J. D., Caufield, W. V., and Shaw, W. A. (2007). Quantitation and standardization of lipid internal standards for mass spectroscopy. Methods Enzymol. 432, 351–367.
Moreau, R. A., Doehlert, D. C., Welti, R., Isaac, G., Roth, M., Tamura, P., and Nunez, A. (2008). The identification of mono-, di-, tri-, and tetragalactosyl-diacylglycerols and their natural estolides in oat kernels. Lipids 43, 533–548.
Muller, C., Schafer, P., Stortzel, M., Vogt, S., and Weinmann, W. (2002). Ion suppression effects in liquid chromatography-electrospray-ionisation transport-region collision induced dissociation mass spectrometry with different serum extraction methods for systematic toxicological analysis with mass spectra libraries. J. Chromatogr. B Analyt. Technol. Biomed. Life Sci. 773, 47–52.
Nakanishi, H., Ogiso, H., and Taguchi, R. (2009). Qualitative and quantitative analyses of phospholipids by LC-MS for lipidomics. Methods Mol. Biol. 579, 287–313.
Nygren, H., Seppanen-Laakso, T., Castillo, S., Hyotylainen, T., and Oresic, M. (2011). Liquid chromatography-mass spectrometry (LC-MS)-based lipidomics for studies of body fluids and tissues. Methods Mol. Biol. 708, 247–257.
Oldiges, M., Lutz, S., Pflug, S., Schroer, K., Stein, N., and Wiendahl, C. (2007). Metabolomics: current state and evolving methodologies and tools. Appl. Microbiol. Biotechnol. 76, 495–511.
Oliver, S. G., Winson, M. K., Kell, D. B., and Baganz, F. (1998). Systematic functional analysis of the yeast genome. Trends Biotechnol. 16, 373–378.
Picchioni, G. A., Watada, A. E., and Whitaker, B. D. (1996). Quantitative high-performance liquid chromatography analysis of plant phospholipids and glycolipids using light-scattering detection. Lipids 31, 217–221.
Plumb, R., Castro-Perez, J., Granger, J., Beattie, I., Joncour, K., and Wright, A. (2004). Ultra-performance liquid chromatography coupled to quadrupole-orthogonal time-of-flight mass spectrometry. Rapid Commun. Mass Spectrom. 18, 2331–2337.
Pluskal, T., Castillo, S., Villar-Briones, A., and Oresic, M. (2010). MZmine 2: modular framework for processing, visualizing, and analyzing mass spectrometry-based molecular profile data. BMC Bioinformatics 11, 395. doi: 10.1186/1471-2105-11-395
Rainville, P. D., Stumpf, C. L., Shockcor, J. P., Plumb, R. S., and Nicholson, J. K. (2007). Novel application of reversed-phase UPLC-oaTOF-MS for lipid analysis in complex biological mixtures: a new tool for lipidomics. J. Proteome Res. 6, 552–558.
Schmitt-Kopplin, P., Englmann, M., Rossello-Mora, R., Schiewek, R., Brockmann, K. J., Benter, T., and Schmitz, O. J. (2008). Combining chip-ESI with APLI (cESILI) as a multimode source for analysis of complex mixtures with ultrahigh-resolution mass spectrometry. Anal. Bioanal. Chem. 391, 2803–2809.
Schwudke, D., Hannich, J. T., Surendranath, V., Grimard, V., Moehring, T., Burton, L., Kurzchalia, T., and Shevchenko, A. (2007). Top-down lipidomic screens by multivariate analysis of high-resolution survey mass spectra. Anal. Chem. 79, 4083–4093.
Shinbo, Y., Nakamura, Y., Altaf-Ul-Amin, M., Asah, H., Kurokawa, K., Arita, M., Saito, K., Ohta, D., Shibata, D., and Kanaya, S. (2006). KNApSAcK: a comprehensive species-metabolite relationship database. Biotechnol. Agr. Forest. 57, 165–181.
Smith, C. A., Want, E. J., O’maille, G., Abagyan, R., and Siuzdak, G. (2006). XCMS: processing mass spectrometry data for metabolite profiling using nonlinear peak alignment, matching, and identification. Anal. Chem. 78, 779–787.
Spiegel, S., and Milstien, S. (2003). Sphingosine-1-phosphate: an enigmatic signalling lipid. Nat. Rev. Mol. Cell Biol. 4, 397–407.
Stahlman, M., Ejsing, C. S., Tarasov, K., Perman, J., Boren, J., and Ekroos, K. (2009). High-throughput shotgun lipidomics by quadrupole time-of-flight mass spectrometry. J. Chromatogr. B Analyt. Technol. Biomed. Life Sci. 877, 2664–2672.
Taguchi, R., and Ishikawa, M. (2010). Precise and global identification of phospholipid molecular species by an Orbitrap mass spectrometer and automated search engine lipid search. J. Chromatogr. A 1217, 4229–4239.
Touchstone, J. C. (1995). Thin-layer chromatographic procedures for lipid separation. J. Chromatogr. B Biomed. Appl. 671, 169–195.
Van Meer, G., Voelker, D. R., and Feigenson, G. W. (2008). Membrane lipids: where they are and how they behave. Nat. Rev. Mol. Cell Biol. 9, 112–124.
Vuckovic, D., Zhang, X., Cudjoe, E., and Pawliszyn, J. (2010). Solid-phase microextraction in bioanalysis: new devices and directions. J. Chromatogr. A 1217, 4041–4060.
Wang, Y., Xiao, J., Suzek, T. O., Zhang, J., Wang, J., and Bryant, S. H. (2009). PubChem: a public information system for analyzing bioactivities of small molecules. Nucleic Acids Res. 37, W623–W633.
Welti, R., Mui, E., Sparks, A., Wernimont, S., Isaac, G., Kirisits, M., Roth, M., Roberts, C. W., Botte, C., Marechal, E., and Mcleod, R. (2007a). Lipidomic analysis of Toxoplasma gondii reveals unusual polar lipids. Biochemistry 46, 13882–13890.
Welti, R., Shah, J., Li, W., Li, M., Chen, J., Burke, J. J., Fauconnier, M. L., Chapman, K., Chye, M. L., and Wang, X. (2007b). Plant lipidomics: discerning biological function by profiling plant complex lipids using mass spectrometry. Front. Biosci. 12, 2494–2506.
Welti, R., and Wang, X. (2004). Lipid species profiling: a high-throughput approach to identify lipid compositional changes and determine the function of genes involved in lipid metabolism and signaling. Curr. Opin. Plant Biol. 7, 337–344.
Williams, A. J. (2008). Public chemical compound databases. Curr. Opin. Drug Discov. Devel. 11, 393–404.
Wymann, M. P., and Schneiter, R. (2008). Lipid signalling in disease. Nat. Rev. Mol. Cell Biol. 9, 162–176.
Xu, Y., Heilier, J. F., Madalinski, G., Genin, E., Ezan, E., Tabet, J. C., and Junot, C. (2010). Evaluation of accurate mass and relative isotopic abundance measurements in the LTQ-orbitrap mass spectrometer for further metabolomics database building. Anal. Chem. 82, 5490–5501.
Yang, K., Cheng, H., Gross, R. W., and Han, X. (2009). Automated lipid identification and quantification by multidimensional mass spectrometry-based shotgun lipidomics. Anal. Chem. 81, 4356–4368.
Yang, K., Zhao, Z., Gross, R. W., and Han, X. (2007). Shotgun lipidomics identifies a paired rule for the presence of isomeric ether phospholipid molecular species. PLoS ONE 2, e1368. doi: 10.1371/journal.pone.0001368
Zhang, Y., Zhu, H., Zhang, Q., Li, M., Yan, M., Wang, R., Wang, L., Welti, R., Zhang, W., and Wang, X. (2009). Phospholipase dalpha1 and phosphatidic acid regulate NADPH oxidase activity and production of reactive oxygen species in ABA-mediated stomatal closure in Arabidopsis. Plant Cell 21, 2357–2377.
Figure A1. Overview scatter plot of all lipids from Table S2 in Supplementary Material. The plot contains the measured retention time in minutes on the x-axes and the recorded mass of the [M + H]+ adduct on the y-axes. Due to the modular building block structure of lipids within a homogenous class, systematic patterns of parallel lines should be observed. From bottom to top these lines should contain lipids with increasing fatty acid chain length, while the number of double bond should decrease from left to right. Abbreviations are as follows: PC, phosphatidylcholine; PE, phosphatidylethanolamine; PG, phosphatidylglycerol; PI, phosphatidylinositol; PS, phosphatidylserine; MGDG, monogalactosyldiacylglycerol; DGDG, digalactosyldiacylglycerol; SQDG, Sulfoquinovosyldiacylglycerol; Cer, ceramide; GlcCer, glucosylceramides; GIPC, glycosylinositolphosphoceramides; TAG, triacylglycerols; DAG, diacylglycerols; FA, fatty acids.
Keywords: lipidomics, ultra performance liquid chromatography, high resolution mass spectrometry, accurate mass, database, all-ion fragmentation, Arabidopsis thaliana, metabolomics
Citation: Hummel J, Segu S, Li Y, Irgang S, Jueppner J and Giavalisco P (2011) Ultra performance liquid chromatography and high resolution mass spectrometry for the analysis of plant lipids. Front. Plant Sci. 2:54. doi: 10.3389/fpls.2011.00054
Received: 08 May 2011;
Accepted: 05 September 2011;
Published online: 12 October 2011.
Edited by:
Alisdair Fernie, Max Planck Institute for Plant Physiology, GermanyReviewed by:
Kazuki Saito, Chiba University, JapanCopyright: © 2011 Hummel, Segu, Li, Irgang, Jueppner and Giavalisco. This is an open-access article subject to a non-exclusive license between the authors and Frontiers Media SA, which permits use, distribution and reproduction in other forums, provided the original authors and source are credited and other Frontiers conditions are complied with.
*Correspondence: Patrick Giavalisco, Max Planck Institute of Molecular Plant Physiology, Am Mühlenberg 1, Golm, 14476 Potsdam, Germany. e-mail:Z2lhdmFsaXNjb0BtcGltcC1nb2xtLm1wZy5kZQ==
Disclaimer: All claims expressed in this article are solely those of the authors and do not necessarily represent those of their affiliated organizations, or those of the publisher, the editors and the reviewers. Any product that may be evaluated in this article or claim that may be made by its manufacturer is not guaranteed or endorsed by the publisher.
Research integrity at Frontiers
Learn more about the work of our research integrity team to safeguard the quality of each article we publish.