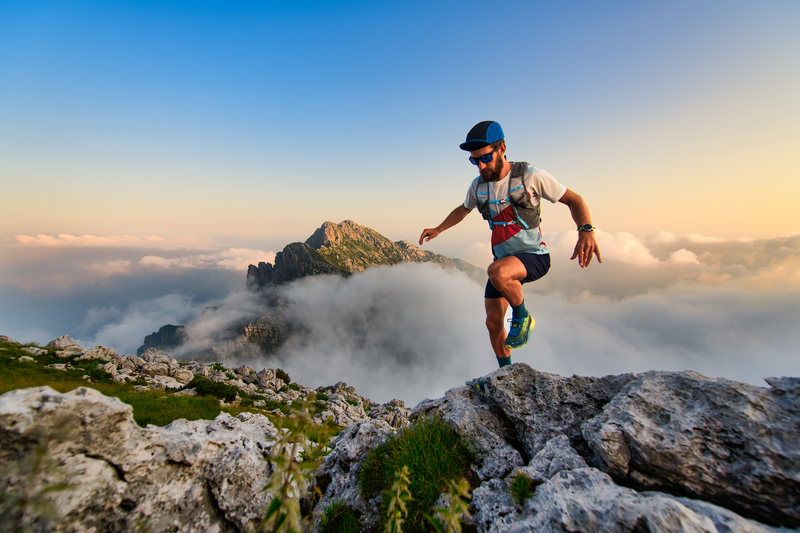
94% of researchers rate our articles as excellent or good
Learn more about the work of our research integrity team to safeguard the quality of each article we publish.
Find out more
ORIGINAL RESEARCH article
Front. Physiol. , 27 August 2024
Sec. Mitochondrial Research
Volume 15 - 2024 | https://doi.org/10.3389/fphys.2024.1395371
This article is part of the Research Topic Mechanisms and Novel Therapeutic Targets and Approaches for Mitochondrial Dysfunction in Neurological and Cardiovascular Diseases View all articles
Introduction: Cardiovascular disease (CVD) is responsible for over 30% of mortality worldwide. CVD arises from the complex influence of molecular, clinical, social, and environmental factors. Despite the growing number of autosomal genetic variants contributing to CVD, the cause of most CVDs is still unclear. Mitochondria are crucial in the pathophysiology, development and progression of CVDs; the impact of mitochondrial DNA (mtDNA) variants and mitochondrial haplogroups in the context of CVD has recently been highlighted.
Aims: We investigated the role of genetic variants in both mtDNA and nuclear-encoded mitochondrial genes (NEMG) in CVD, including coronary artery disease (CAD), hypertension, and serum lipids in the UK Biobank, with sub-group analysis for diabetes.
Methods: We investigated 371,542 variants in 2,527 NEMG, along with 192 variants in 32 mitochondrial genes in 381,994 participants of the UK Biobank, stratifying by presence of diabetes.
Results: Mitochondrial variants showed associations with CVD, hypertension, and serum lipids. Mitochondrial haplogroup J was associated with CAD and serum lipids, whereas mitochondrial haplogroups T and U were associated with CVD. Among NEMG, variants within Nitric Oxide Synthase 3 (NOS3) showed associations with CVD, CAD, hypertension, as well as diastolic and systolic blood pressure. We also identified Translocase Of Outer Mitochondrial Membrane 40 (TOMM40) variants associated with CAD; Solute carrier family 22 member 2 (SLC22A2) variants associated with CAD and CVD; and HLA-DQA1 variants associated with hypertension. Variants within these three genes were also associated with serum lipids.
Conclusion: Our study demonstrates the relevance of mitochondrial related variants in the context of CVD. We have linked mitochondrial haplogroup U to CVD, confirmed association of mitochondrial haplogroups J and T with CVD and proposed new markers of hypertension and serum lipids in the context of diabetes. We have also evidenced connections between the etiological pathways underlying CVDs, blood pressure and serum lipids, placing NOS3, SLC22A2, TOMM40 and HLA-DQA1 genes as common nexuses.
Cardiovascular disease (CVD) is an umbrella term that encompasses all diseases of the heart and blood vessels, including heart disease (involving the heart) and vascular disease (involving the blood vessels) (Lopez et al., 2023). Coronary artery disease (CAD), sometimes called coronary heart disease or ischemic heart disease, is the most common type of heart disease and is characterized by a narrowing or blockage of the coronary arteries (Coronary Artery Disease, 2023; Coronary Artery Disease (CAD): Symptoms & Treatment, n.d.). CVD is the major cause of deaths worldwide, accounting for more than 30% of mortality (WHO, 2023; Tsao et al., 2023). CAD is the leading cause of death, accounting for 16% of global mortality in 2019 (WHO, 2023). Diabetes is a major risk factor for development of CVD and for people with diabetes, CVD represents the leading cause of morbidity and mortality (WB and DL, 1979). Individuals with type 2 diabetes mellitus (T2DM) have a 2–4 times increased risk of CVD (Kishore et al., 2012; Visseren et al., 2021; Yang et al., 2021).
CVD is a complex multifactorial condition arising from the combined influence of environmental and hereditary factors. Well established modifiable risk factors for CVDs include hypertension, diabetes, hypercholesterolemia and smoking with these factors used in the estimation of the 10-year risk of incident cardiovascular events (Goff et al., 2014; Cadby et al., 2020). Serum lipids, namely, total cholesterol (Chol), low-density lipoprotein (LDL), high-density lipoprotein cholesterol (HDL) and triglycerides (TG) are directly implicated in the development of CVDs, and are used as risk factors to predict long-term CVD risk and adverse clinical outcomes (Goff et al., 2014; Cadby et al., 2020), and as therapeutic targets for CVDs (Ference et al., 2017; Echouffo-Tcheugui et al., 2020). The aetiology of CVD is clearly influenced by genetics, as evidenced in many studies (Marino and Digilio, 2000; Muntean et al., 2017; Silva et al., 2023; Safdar et al., 2024). CVD, and especially CAD, show polygenic architecture and a substantial heritability, estimated between 40% and 60% (Watkins and Farrall, 2006; Dai et al., 2016; Khera and Kathiresan, 2017; Inouye et al., 2018a; Drobni et al., 2022). Genome-wide association studies (GWAS) have identified associations between single nucleotide polymorphisms (SNPs) and CAD, myocardial infarction, and other CVDs (Companioni et al., 2011; Muntean et al., 2017; Silva et al., 2023). A recent systematic review confirmed at least 71 genetic variants as susceptibility loci for CAD (Silva et al., 2023). Beyond SNPs, there are other genetic causes of CVD, including chromosomal aberrations, copy number variations and epigenomics (Liu et al., 2023; Safdar et al., 2024). However, despite the growing number of hereditary factors contributing to CVD, the cause of the vast majority of CVDs remains unclear (Safdar et al., 2024). Mitochondria play a significant role in the pathophysiology, development and progression of CVDs, through key mechanisms such as excessive reactive oxygen species production, mitochondrial dysfunction, and genetic factors as mitochondrial DNA (mtDNA) damage or mutations (Venter et al., 2018; Siasos et al., 2018; Poznyak et al., 2020; Lin et al., 2021; Calabrese et al., 2022; Yang et al., 2022). In cardiac mitochondria, mtDNA is important in the mitochondrial life circle and the proper functioning of oxidative phosphorylation (OXPHOS). Irreversible mtDNA damage leads to mtDNA mutations, which in turn aggravate OXPHOS dysfunction and affect mitophagy, producing a leakage of both mtDNA and proteins outside the mitochondria, which triggers an innate immune response, causing cardiovascular damage (Liu et al., 2022).
Mitochondria, the organelles responsible for generating energy for cellular metabolism (Cooper, 2000; Lodish et al., 2012; Chaban et al., 2014) contain several copies of their own genome, a circular double-stranded DNA molecule of ≈16.6 kb which in humans includes a total of 37 genes, 13 coding for the subunits of respiratory complexes I, III, IV, and V (Meiklejohn et al., 2013), 22 code for transfer RNAs (tRNAs) for the 20 standard amino acids, an extra gene for leucine and serine (Taanman, 1999; Cooper, 2000; Gray et al., 2008), and two for ribosomal RNAs (rRNAs) (Chan, 2006). The replication origin(s) and promoters for mtDNA are contained in an additional displacement loop (D-loop). Additionally, the cell nucleus contains genes encoding proteins related to mitochondria functions which regulate mtDNA transcription, replication, cell apoptosis and mitophagy, nucleotide biosynthesis, metabolism, and iron and calcium homeostasis (Timmis et al., 2004; Dolezal et al., 2006). Common maternally inherited mtDNA variants have been associated with CVD risk factors such as hypertension, diabetes, and dyslipidaemia (Calabrese et al., 2022). Recently, the role of mitochondrial genetic variants in the lipidomic context of CAD has been highlighted in 1,409 Han Chinese CAD patients, showing associations of D-loop variants with TG, Chol, LDL and HDL (Wang et al., 2021). Specific mitochondrial haplogroups have shown to confer a significant risk for many CAD related traits, such as coronary atherosclerosis (Sawabe et al., 2011), ischemic stroke (Tsai et al., 2020), myocardial infarction (Nishigaki et al., 2007b), atherosclerotic cerebral infarction (Nishigaki et al., 2007a), essential hypertension (Tsai et al., 2020) and T2DM in Asians (Fuku et al., 2007), and CVD (Veronese et al., 2019), atherosclerosis (Zhelankin et al., 2015; Piotrowska-Nowak et al., 2018), CAD (Kofler et al., 2009; Palacín et al., 2011), ischemic stroke (Rosa et al., 2008), hypertrophic cardiomyopathy (Castro et al., 2006; Singh et al., 2021) and diabetic retinopathy (Kofler et al., 2009; Estopinal et al., 2014; Bregman et al., 2017) in Europeans. Not all the studies however indicate an influence of mitochondrial variants in CAD related traits. No role for mtDNA variation was identified for hypertension or hyperglycaemia in participants from the Sympathetic activity and Ambulatory Blood Pressure in Africans (SABPA) prospective cohort study (Venter et al., 2017). A large study in over 9,000 Europeans failed to find a role for mitochondrial haplogroups on morbidity or mortality secondary to CVD (Benn et al., 2008). Therefore, the identification of mitochondrial genetic patterns and different forms of CVD and related traits is important to gain deeper understanding of the biological links between CVDs, lipid metabolism and clinical outcomes.
In this study, we aimed to investigate the role of genetic variants in both mtDNA and nuclear-encoded mitochondrial genes (NEMG) in cardiovascular diseases (CVD, CAD and hypertension) and cardiovascular risk factors (serum lipids: Chol, HDL, LDL, and TG) in a large population cohort (UK Biobank), additionally exploring the impact of diabetes.
This investigation conformed to the principles outlined in the Declaration of Helsinki. Participants gave informed consent prior to their inclusion in the UK Biobank project.
This was a retrospective, cross-sectional study in participants of European ethnicity from the UK Biobank (Bycroft et al., 2018). To evaluate the effect of diabetes, the association of gene variants with the phenotypic outcomes were investigated with/without stratification by diabetes. Therefore, the total (overall) cohort was divided into two groups, according to the presence (diabetic cohort) or absence of diabetes (non-diabetic cohort) (Figure 1). Participants whose assessment of cardiovascular disease or diabetes was not possible were excluded from the analysis.
Figure 1. Design of the study. Abbreviations: chrMT: mitochondrial chromosome; HDL: high-density lipoprotein cholesterol; LDL: low-density lipoprotein cholesterol; NEMG: nuclear-encoded mitochondrial genes; PCA: principal component analysis.
The UK Biobank project is a large-scale biomedical database and research resource providing genetic, lifestyle and health information from half a million UK participants (Bycroft et al., 2018).
Outcome variables included CVD, CAD, hypertension, systolic and diastolic blood pressure (SBP, DBP) and serum lipids (cholesterol, HDL, LDL, and TG). Detailed definitions and disorders captured in every variable are provided within the “Supplementary Methods” section of the Supplementary Data Sheet.
The Applied Biosystems™ UK Biobank Axiom™ and UK BiLEVE Axiom™ Affymetrix Arrays were used for genotyping by the UK Biobank. Genotypes were imputed by the UK Biobank using a combination of the Haplotype Reference Consortium and merged UK10K and 1000 Genomes phase 3 reference panels (Bycroft et al., 2018). PLINK 1.90 beta and PLINK 2.00 alpha were used to perform quality control (QC) and association analysis (Chang et al., 2015; Chang and GRAIL, 2020). Before QC, the study was comprised of 488,377 participants, 711,188 variants in NEMG and 265 mitochondrial variants. Individuals with high missingness rate or call rate lower than 95% were removed. Related individuals (identity by kinship coefficient >0.0884) and principal component analysis (PCA) outliers, as calculated by the UK Biobank, were also removed (Bycroft et al., 2018). Variants with minor allele frequency (MAF) < 1%, minor allele count (MAC) < 20 or variant call rate <95% were removed from the analysis. Autosomal variants not fulfilling Hardy-Weinberg equilibrium (HWE) (p < 1E-20) or imputation score under 0.3 were also excluded. After QC, 381,994 participants (Overall Cohort), 371,542 variants in 2,527 NEMG, along with 192 variants in 32 mitochondrial genes for the combined arrays and 93 variants in 28 genes for the BiLEVE array remained. For variants present in both arrays, only results in the UK Biobank Axiom™ were considered (largest sample).
Mitochondrial haplogroups were estimated using HaploGrep2 (Weissensteiner et al., 2016), based on PhyloTree17 (van Oven, 2015). Only the major European haplogroups H, V, HV, J, T, U, K, Z, W, X, I, and N were considered, grouping the remaining options in the “Other” category.
A total of 2,527 unique autosomal genes coding for 22,713 transcripts were investigated. The selection process produced 2,448 unique genes returned from database searches with a further 180 genes identified from literature searches for genes influencing mitochondrial function (Skelly, 2020). Briefly, several online databases and literature resources were searched for NEMGs: Mitoproteome (Taylor et al., 2003; Cotter et al., 2004; Pagliarini et al., 2008; Calvo et al., 2016), MitoMiner (Smith and Robinson, 2016), MitoMap (Brandon et al., 2005), Ensembl (Zerbino et al., 2018) and UniProt (The UniProt Consortium, 2017). Genes extracted from individual sources were reviewed and duplicates were excluded. Gene names were then screened to ensure there was no duplication between the database searches and literature searches. Genes were annotated with their official HUGO Gene Nomenclature Committee (HGNC) gene symbol (Wain et al., 2002) using Ensembl BioMart release 67 (May 2012) based on the February 2009 Homo sapiens high coverage assembly GRCh37 from the Genome Reference Consortium (Zerbino et al., 2018). Any genes not found in the BioMart (Zerbino et al., 2018) search were manually annotated according to their official HGNC gene symbol (Wain et al., 2002). The list of genes was then checked again for duplicates based on HGNC symbols, known pseudonyms and gene positions. Only genes found in autosomes were included in the analysis. Any genes on sex chromosomes, non-human genes, or bacterial artificial chromosomes were excluded from the final list of genes encoding proteins required for mitochondrial function.
The online tool Functional Mapping and Annotation of Genome-wide Association Studies (FUMAGWAS) version 1.6.1 (Watanabe et al., 2017; 2019) was used to annotate, prioritise, visualise, and interpret the function of the genes statistically associated in the three cohorts. This tool automatically performs tissue specificity test and gene set/pathway enrichment analyses.
Descriptive and bivariate analyses were performed using R (R Core Team, 2024). Qualitative variables were expressed as percentage (%) of their total. Quantitative variables were expressed as the mean and the standard deviation.
Association analysis for individual variants was performed using PLINK 2.00 alpha using the “--glm” flag (Chang et al., 2015). For binary phenotypes (CVD, CAD and hypertension) --glm fits a logistic or Firth regression model (Chang et al., 2015). For quantitative phenotypes, --glm fits the linear model (Chang et al., 2015). Quantitative outcome variables were natural logarithmic transformed and analysed using the additional “--pheno-quantile-normalize” flag, to force quantitative phenotypes to a N (0, 1) distribution, preserving only the original rank orders (Chang et al., 2015). The --glm flag performs a multicollinearity check before each regression, which skips and reports “NA” results when it fails. Age, sex, genotyping batch and the 10 first PCAs values were included as covariates.
For the influence of traditional non-genomic risk factors for CVD on the outcomes (CAD, CVD and hypertension) multivariable logistic regression was performed in R (R Core Team, 2024).
To correct for multiple testing, a Bonferroni correction for the number of independent variants (estimated using a pruning procedure of our data; r2 <0.2, window size 50 bp, offset 5 bp) after QC was used (Wuttke et al., 2016). The pruning estimated 47 independent variants for the mitochondrial chromosome for the combined arrays of the UK Biobank (35 when only the BiLEVE array was considered), yielding a threshold of 1E-03, and 57,457 variants for NEMG, yielding a threshold of 9E-07.
Independent loci were identified using PLINK 1.90 beta clumping procedure (--clump-p1 5e-05 --clump-r2 0.1 --clump-kb 500) (Chang et al., 2015). A physical distance threshold for clumping of 1 kb was used for the mitochondrial chromosome. The independent loci were annotated using SNPnexus (Chelala et al., 2009; Dayem Ullah et al., 2012; 2013; Dayem Ullah et al., 2018; Oscanoa et al., 2020).
Association analysis for mitochondrial haplogroups was performed using logistic regression in R version 4.3.0 (21/04/2023) (R Core Team, 2024), including as covariates age, sex and genotyping. Each haplogroup was analysed separately using all the other haplogroups as reference, after constructing dummy variables taking the values of 0 and 1, with the R package “fastDummies” (Kaplan, 2020). Principal components were not used as covariates to account for ancestry because of their potential correlation with haplogroups. The Bonferroni correction was applied to account for multiple comparisons, adjusting the p-value threshold, dividing by the number of haplogroups in each dataset (0.05/number of haplogroups).
Power calculations were performed for the CVD phenotype in the overall cohort and two strata using the Genetic Association Study (GAS) Power Calculator, considering a genotype relative risk of 1.2 (Skol et al., 2006), disease allele frequency of 0.02 and a prevalence of 32.2% (Einarson et al., 2018). In the cohort with diabetes, the statistical power was 84.2% and 94% for significance levels of 9E-07 and 1E-04, respectively; 100% for the other cohorts.
The descriptive analysis of the population is detailed in Table 1. Individuals with diabetes were more likely to be taking medication to control blood pressure or cholesterol, with more than half having CVD. Traditional non-genomic risk factors for CVD were associated with CAD, CVD and hypertension in the three cohorts (Supplementary Table S3).
Table 1. Descriptive analysis of the participants included in the study, stratified by diabetes. For qualitative variables, frequencies are expressed as number and percentage in brackets. Quantitative variables are expressed as mean and standard deviation.
Mitochondrial variants showed associations with CVD, hypertension, Chol and HDL (Figure 2). Full summary statistics are available in (Supplementary Document 1). Seven variants in MT-ATP6, MT-CYB, MT-ND4, MT-ND5, MT-TR and MT-TT were associated with CVD in the overall cohort (MT-ND4-rs3088053 also in the non-diabetic cohort). The MT-ND2-rs3020602 variant was associated with hypertension in the diabetic cohort. Directions of effects were consistent among cohorts.
Figure 2. Mitochondrial variants associated with qualitative and quantitative phenotypes in any cohort (expressed as odds ratio and 95% confidence intervals for qualitative phenotypes and beta coefficients and 95% confidence intervals for quantitative phenotypes). Variants with p < 1E −03 are marked with an asterisk in the color of the corresponding cohort. The total number of participants per cohort was: 381,994 (overall cohort), 345,245 (non-diabetic cohort) and 36,749 (diabetic cohort). p-values correspond to the asymptotic p-value (or -log10(p)) for Z/chisq-stat (Qualitative variables, logistic regression) or for T/chisq-stat (Quantitative variables, linear regression). Abbreviations: chrMT: mitochondrial chromosome; Chol: total cholesterol; CVD: cardiovascular disease; DBP: diastolic blood pressure; DM: Diabetes Mellitus; HBP: hypertension; HDL: high-density lipoprotein cholesterol LDL: low-density lipoprotein cholesterol; SBP: systolic blood pressure.
The frequency of the mitochondrial haplogroups in the UK Biobank Cohort is shown in Supplementary Table S4. Mitochondrial haplogroup J showed associations with CAD, Chol and LDL, whereas mitochondrial haplogroups T and U were associated with CVD. There were no significant associations in the diabetic cohort (Figure 3). Directions of effects were consistent among cohorts. The association of mitochondrial haplogroup T and CVD was consistent, showing associations with four of its defining mutations (MT-ATP6-rs879233543, MT-TR-rs28358279, MT-CYB-rs193302983 and MT-TT-rs527236198; Figure 2).
Figure 3. Mitochondrial haplogroups associated with phenotypes in any cohort. Associations with p < 4E-03 are marked with an asterisk in the color of the corresponding cohort (expressed as odds ratio and 95% confidence intervals for qualitative phenotypes and beta coefficients and 95% confidence intervals for quantitative phenotypes). The total number of participants per cohort was: 381,994 (overall cohort), 345,245 (non-diabetic cohort) and 36,749 (diabetic cohort). p-values correspond to the asymptotic p-value (or -log10(p)) for Z/chisq-stat (Qualitative variables, logistic regression) or for T/chisq-stat (Quantitative variables, linear regression). Abbreviations: chrMT: mitochondrial chromosome; Chol: total cholesterol; DBP: diastolic blood pressure; DM: Diabetes Mellitus; HBP: hypertension; HDL: high-density lipoprotein cholesterol LDL: low-density lipoprotein cholesterol; SBP: systolic blood pressure.
Figures 4, 5 show the number of genes with associations to the different phenotypes, in any cohort. NOS3 was common to CVD, CAD, hypertension, SBP and DBP (Figure 4). In particular, the NOS3-rs3918226T variant was associated with an increased risk of CVD, CAD, hypertension, and values of SBP and DBP and decreased serum levels of Chol and LDL, whereas the NOS3-rs891511A variant was associated with decreased SBP and DBP in the overall and/or non-diabetic cohorts (Supplementary Document 2). The NOS3-rs2070744 and NOS3-rs1007311 variants were also associated with HDL in the overall/non-diabetic cohorts.
Figure 4. Venn diagrams showing the genes in the intersection among coronary artery disease, cardiovascular disease and hypertension (A) and also with diastolic and systolic blood pressure (B). Abbreviations: CAD: coronary artery disease; CVD: cardiovascular disease; DBP: diastolic blood pressure; HBP: hypertension; SBP: systolic blood pressure.
Figure 5. Venn diagrams showing the genes in the intersection among serum lipids and coronary artery disease (A), cardiovascular disease (B) and hypertension (C), respectively. Gene names are shown only for sets of five genes or less in the central (common to all phenotypes) and outer layers (uncommon genes). Abbreviations: CAD: coronary artery disease; Chol: total cholesterol; CVD: cardiovascular disease; DBP: diastolic blood pressure; HBP: hypertension; HDL: high-density lipoprotein cholesterol; LDL: low-density lipoprotein cholesterol; SBP: systolic blood pressure; TG: triglycerides.
TOMM40, one of the genes associated with CAD but not with CVD or the blood pressure related phenotypes (hypertension, SBP and DBP; Figure 4), was common for all the serum lipids, along with SLC22A2 (Figure 5A), which was common for CVD and all serum lipids (Figure 5B). Two variants in TOMM40 were associated with three phenotypes, rs34404554 (CAD, Chol and HDL) and rs61679753 (Chol, HDL and LDL) (Supplementary Document 2). Sixteen more variants were associated with Chol, HDL, LDL and TG (one or several) mainly in the overall and non-diabetic cohorts (Supplementary Document 2). As for SLC22A2, the rs10080815 variant was associated with CAD, CVD, Chol, LDL and TG; rs3127606 with CAD, Chol and LDL. Other 13 variants were associated with one or several phenotypes (CAD, CVD, Chol, HDL, LDL and TG), mainly in the overall and non-diabetic cohorts (Supplementary Document 2).
HLA-DQA1 was associated with hypertension and all the serum lipids (Figure 5C). In particular, the rs6938008 variant was associated with hypertension and HDL, whereas the rs3129770 variant was associated with Chol, LDL and TG (Supplementary Document 2). Two variants were associated only in the cohort with diabetes (rs1048372 with HDL and rs9272417 with TG; Supplementary Document 2).
Sixty-six variants in 35 NEMG were consistently significant in all three cohorts for Chol, HDL, LDL, TG and/or DBP (Supplementary Figures S1–S5). Supplementary Table S5 shows the number of gene variants and genes associated with each phenotype, along with the number of traits reported in GWAS Catalog for those genes, according to FUMAGWAS (Watanabe et al., 2017). Among them, six variants in TOMM40 were associated with LDL and Chol in all cohorts (rs71352238, rs2075650, rs1160983, rs11668327, rs111784051 and rs115881343). In addition, TOMM40-rs34404554 was associated with Chol and HDL in all cohorts (Supplementary Document 2). The GWAS Catalog reports TOMM40 associations mainly with multiple serum lipid traits, including Chol, HDL, LDL and TG, C-reactive protein and body-mass index (BMI) (Supplementary Table S6).
Nine variants in HLA-DQA1 were associated with LDL, TG and DBP in all cohorts (Supplementary Figures S3–S5). The HLA-DQA1-rs6938008 variant was also associated with hypertension in the overall cohort (Supplementary Document 2).
The rs7005363 variant in MSRA was associated with TG levels in all cohorts (along with other 12 in the overall/non-diabetic cohorts). Six other variants in this gene were also associated with CVD and hypertension in the overall/non-diabetic cohorts. In the enrichment analysis, MSRA appears along with TOMM40 as cellular components of the mitochondrion and associated with serum metabolite levels, according to GWAS Catalog (Supplementary Table S6).
Other genes previously reported as risk factors for lipid traits and found significant in the three cohorts were GCKR, SLC39A8, FADS2, PGS1, HNF4A and PLA2G6 (Supplementary Table S6).
Among the NEMG variants significantly associated in the overall and non-diabetic cohorts, some of them showed different direction of association in the diabetic cohort, although not significantly (Supplementary Figures S6–S12). As an exception to this, the HLA-DQA1-rs9272417 variant was significant only in the diabetic cohort (Figure 6; Supplementary Figure S10 and Supplementary Document 2).
Figure 6. Variants in nuclear-encoded mitochondrial genes associated with several phenotypes after stratification by diabetes (expressed as beta coefficients and 95% confidence intervals). Abbreviations: chrMT: mitochondrial chromosome; Chol: total cholesterol; DM: Diabetes Mellitus; HBP: hypertension; HDL: high-density lipoprotein cholesterol.
Eight variants in seven NEMG showed associations with HDL, TG, Chol and DBP only in participants with diabetes (Figure 6). In particular, two variants in HLA-DQA1 were associated with HDL (rs1048372) and TG (rs9272417).
Traditional non-genomic risk factors for CVD are associated with cardiac phenotypes in the UK Biobank cohort (Littlejohns et al., 2019; Razieh et al., 2022; Zhang et al., 2024). Although these clinical factors account for much of the CVD risk, it is valuable to explore the association between genomic factors, including mitochondrial DNA variation and variation in mitochondrial related genes, and cardiac phenotypes.
Our study shows a consistent association between mitochondrial haplogroup T and CVD, reflected through associations not only with the haplogroup itself, but also with four of its defining mutations. The importance of this mitochondrial haplogroup on CVD had been previously evidenced by more than 3.5 times increase in the risk of hypertrophic cardiomyopathy in males, the most common genetic disorder of the heart (Castro et al., 2006; Singh et al., 2021). The mtDNA haplogroup T was associated with higher risk of CAD (14.8% vs. 8.3%; p = 0.002) in the study of Middle European Caucasians, including 487 patients with angiographically documented CAD and 1,527 control subjects without clinical manifestations of atherosclerotic disease (Kofler et al., 2009). One of the defining variants of haplotype T (MT-TT-rs527236198A) has recently been associated with higher risk of CAD in Iranian patients, demonstrating a transethnic effect (Heidari et al., 2020). Furthermore, JT haplogroups (HR = 0.75; 95%CI: 0.54–0.96; p = 0.03), and particularly J (HR = 0.71; 95%CI: 0.46–0.95; p = 0.02) have been associated with a reduced risk of CVD after a median follow-up of 8 years in 3,288 Caucasian participants (Veronese et al., 2019). In our study, mitochondrial haplogroup J was associated with higher risk of CVD along with lower levels of Chol and LDL. Interestingly, MT-ND5-rs28359172, one of the defining mutations of mitochondrial haplogroup J, was also associated with Chol levels in 321,188 individuals. We have recently observed this variant associated with eGFR levels in 329,235 participants from the UK Biobank (Cañadas-Garre et al., 2024). Other variants in MT-ND5 (rs2853503), and MT-CYB-rs2853506, both associated with CVD in our analysis, were also associated with renal function in previous works in the UK Biobank (SCr, SCysC and eGFR) (Yonova-Doing et al., 2021; Cañadas-Garre et al., 2024) MT-ND5 encodes the NADH dehydrogenase 5 subunit gene. Mutations in MT-ND5 have been associated with tubulo-interstitial kidney disease, clinically characterised by proteinuria and hypertension (Bakis et al., 2020), which could partially explain its role in the overlap between CKD and CVD. Mitochondrial genes like MT-ATP6, MT-CYB, MT-ND4, MT-ND5, MT-TR and MT-TT, all associated with CVD in our study, have also been associated with CVD in other ethnic populations, e.g., CAD in Iranians (Heidari et al., 2020), and hypertension and ischaemic stroke in Chinese (Zhu et al., 2016; 2018; Guo et al., 2022). Mitochondrial genes, particularly those coding the oxidative phosphorylation (OXPHOS) enzyme complexes I-IV, play significant roles in CVD due to their involvement in mitochondrial function and energy production. Variants in these genes can disrupt normal electron transport chain activity, leading to decreased ATP levels and increased oxidative stress, causing mitochondrial dysfunction, which is increasingly recognized as a contributing factor to various forms of heart disease, including CAD and cardiomyopathies (Venter et al., 2018; Campbell et al., 2022). Many of the conditions causing CVD, such as atherosclerosis, hypertension, cardiomyopathy and T2DM, are associated with inflammation caused by oxidative stress (Venter et al., 2018).
The findings of our study place NOS3 as the gene most consistently associated with CVD, CAD and blood pressure related traits (hypertension, SBP and DBP). NOS3, encoding for nitric oxide synthase 3, may play a role in the development and progression of CAD (Nikpay et al., 2015; Van Der Harst and Verweij, 2018; Zhou et al., 2018; Hartiala et al., 2021; Aragam et al., 2022; Temprano-Sagrera et al., 2022; Rai et al., 2023), which appears to be mediated mainly through blood pressure regulation across ancestries, according to many GWAS (Liu et al., 2016; Hoffmann et al., 2017; Wain et al., 2017; Feitosa et al., 2018; Sung et al., 2018; Kichaev et al., 2019; Giri et al., 2019; Wojcik et al., 2019; Sakaue et al., 2021; Plotnikov et al., 2022; Schoeler et al., 2023). Our results confirm the role of NOS3-rs3918226T as a marker of susceptibility for CAD (Nikpay et al., 2015; Van Der Harst and Verweij, 2018; Zhou et al., 2018; Aragam et al., 2022; Temprano-Sagrera et al., 2022) and CVD (Kichaev et al., 2019), plus NOS3-rs891511A as a potential reducer of blood pressure (Liu et al., 2016; Hoffmann et al., 2017; Wain et al., 2017; Feitosa et al., 2018; Sung et al., 2018; Kichaev et al., 2019; Giri et al., 2019; Wojcik et al., 2019; Sakaue et al., 2021; Plotnikov et al., 2022; Schoeler et al., 2023). We have recently reported the association of these two variants in NOS3 (rs3918226 and rs891511) with kidney damage in the UK Biobank cohort (Cañadas-Garre et al., 2024). Other variants in NOS3 have shown a clear link with end-stage renal disease (ESRD) (Elsaid et al., 2021; Padhi et al., 2022), chronic kidney disease (CKD) (Gunawan et al., 2020), CKD progression (Medina et al., 2018) and diabetic kidney disease (DKD) (Chen et al., 2016; Roumeliotis et al., 2021). For patients with ESRD receiving haemodialysis, CVD is the major cause of morbidity and mortality (Fox et al., 2012; Mahmoodi et al., 2012) and CVD is present in over 50% of them (Cozzolino et al., 2018). Longitudinal cohorts such as the UK Biobank in time will allow further investigation of common genetic risk factors contributing to early detection, predisposition and multimorbidity for both CVD and ESRD.
In our study, up to 15 variants in SLC22A2 gene were associated with CAD and CVD and all serum lipids, but none of the blood pressure related phenotypes. SLC22A2 encodes the solute carrier family 22 member 2, a polyspecific organic cation transporter responsible from elimination of endogenous small organic cations, toxins and drugs (Gründemann and Schömig, 2000). These SLC22A2 gene variant associations confirm results from many previous GWAS identifying SLC22A2 not only as a susceptibility risk factor for CAD (Nikpay et al., 2015; Yeo et al., 2017; Lempiäinen et al., 2018; Van Der Harst and Verweij, 2018; Svishcheva et al., 2019; Shadrina et al., 2020; Aragam et al., 2022), but also as a marker of lipoprotein (a) levels (Mack et al., 2017; Liu et al., 2019; Sinnott-Armstrong et al., 2021), a well-known genetically determined risk factor of CAD (Berg et al., 1979; Tipping et al., 2009; Schatz et al., 2017; Foscolou et al., 2018). Regarding the association of SLC22A2 with serum lipid levels, as in our study, many others have found variants in the SLC22A2 gene influencing serum levels of atherogenic risk lipids, and potentially impacting lipid metabolism (Bar et al., 2020; Lotta et al., 2021; Sakaue et al., 2021; Sinnott-Armstrong et al., 2021; Koskeridis et al., 2022; Richardson et al., 2022; Davyson et al., 2023; Schoeler et al., 2023). Altogether, these findings indicate that SLC22A2 may play a role in regulating serum lipid levels, thereby potentially influencing the risk of atherosclerosis and CAD. SLC22A2 has been implicated in the regulation of plasma lactate levels, particularly in the context of CVD and T2DM, with TT-carriers of the SLCA22A2-rs316019 variant showing significantly higher fasting plasma lactate concentrations (Li et al., 2010). Increased lactatemia has shown to be a marker of poor prognosis in patients with acute heart failure (Ouyang et al., 2023). The influence of SLC22A2 variants on lactatemia could not be assessed, as our UK Biobank application did not include the participants metabolomics profiling, where lactate levels were measured. The relevance of SLC22A2 goes beyond CAD, since it is a shared susceptibility locus for T2DM, with common etiological pathways between them (Zhao et al., 2017; Xue et al., 2018; Ray and Chatterjee, 2020). Furthermore, we and others have previously demonstrated the importance of SLC22A2 in CKD, renal traits and function (Chambers et al., 2010; Köttgen et al., 2010; Pattaro et al., 2016; Cañadas-Garre et al., 2024), thus revealing one of the many potential biological connections between the etiologies of CVD and CKD. Of interest, CKD is one of the most important risk factors for the development of CVD, and most patients with CKD die from cardiovascular causes before they progress to kidney failure (Liu et al., 2014; Jankowski et al., 2021; Warrens et al., 2022; Zoccali et al., 2023). In fact, a recent study has highlighted the common genetic architectures overlapped between CAD and CKD using summary statistics publicly available from large scale GWAS, showing NOS3, SLC22A2 and TOMM40 among the genes with potential pleiotropy between these two conditions (Chen et al., 2020).
Among the NEMG investigated in our study, TOMM40 was associated with CAD and all the serum lipids, but not with CVD or blood pressure related traits. These results reinforce the robust association between the G-allele of TOMM40-rs2075650 and increased risk of CAD identified in GWAS (Middelberg et al., 2011; Deloukas et al., 2013; Christiansen et al., 2017b; Feng et al., 2017). TOMM40 codes for the channel-forming subunit of the translocase of the mitochondrial outer membrane (TOM) complex 40, essential for protein import into mitochondria (Humphries et al., 2005). The most investigated variant is rs2075650, located in an intronic region of the TOMM40 gene, just upstream of APOE, and APOC1, holding a relatively strong linkage disequilibrium that has suggested the potential causal variation to relay on the APOE gene (Deelen et al., 2011; Christiansen et al., 2017a; Palmer et al., 2021). Variants in TOMM40 have also been proposed as predictors of non-HDL-Chol in 2,800 African-Americans (Feng et al., 2017), LDL (Sandhu et al., 2008; Talmud et al., 2009; Middelberg et al., 2011; Radovica et al., 2014) and TG (Salakhov et al., 2014) in Europeans and dyslipidaemia in 1,962 Chinese Maonans (Miao et al., 2018). In our study, the rs2075650 variant was consistently associated with LDL and Chol serum levels in all cohorts, with the G allele reducing HDL levels and increasing the rest of the serum lipids and the risk of CAD, evidencing again the crucial role of this gene in the biological pathways involving serum lipids.
In addition to CAD and dyslipidemia, genetic variants in TOMM40 have been investigated in other contexts, having been associated with reduced BMI (Guo et al., 2013), lower levels of high-sensitivity C-reactive protein (hs-CRP) (Ellis et al., 2014; Christiansen et al., 2017a), healthy aging and longevity (Deelen et al., 2011; 2014; Chen et al., 2022; Torres et al., 2022) and increased risk of Alzheimer’s disease (Denny et al., 2013; Davies et al., 2015). A recent systematic review identified the rs2075650 and rs10524523 variants as the two most commonly associated with longevity. The outcomes associated with TOMM40 variants were changes in BMI, brain integrity, cognitive functions, altered inflammatory network, vulnerability to vascular risk factors (including hypertension, hyperlipidemia, and diabetes, among others), and longevity (Gui et al., 2021; Chen et al., 2022). Interestingly, TOMM40 polymorphisms strongly interact with vascular risk factors to influence cognitive performance, being markedly detrimental to cognition (Gui et al., 2021). Further analyses revealed TOMM40-rs2075650G allele also interacted with diabetes, dramatically reducing the Mini-Mental State Examination score, used to evaluate cognitive impairment (Gui et al., 2021). In line with this, other TOMM40 variant previously linked to Alzheimer’s disease (Nazarian et al., 2019) and cerebral amyloid deposition (Yan et al., 2021) is rs71352238, found consistently associated with LDL and Chol levels in all cohorts in our study, bringing more evidence to the link between TOMM40, serum lipids and development of Alzheimer’s disease.
Our study identified a consistent association for HLA-DQA1 with hypertension and serum lipids, with up to nine variants associated with LDL, TG and DBP in all cohorts. The HLA-DQA1 gene, also known as Major Histocompatibility Complex, Class II, DQ Alpha 1, is part of the human leukocyte antigen (HLA) complex, which plays a critical role in the immune system by presenting antigens to CD4-positive T-lymphocytes. Variants of the HLA-DQA1 gene have been associated with various autoimmune conditions, including T1DM (Scott et al., 2017; Onengut-Gumuscu et al., 2019; Liao et al., 2023), and have been proposed as markers of susceptibility for T2DM and diabetic nephropathy (Ma et al., 2013). The HLA-DQA1*0501 allele confers susceptibility to idiopathic dilated cardiomyopathy, while the DQA1 0201 allele provides protection (Limas et al., 1995; Liu et al., 2005). Unfortunately, the tag SNPs for these two markers were not among our postQC variants. In the context of hypertension, they have been proposed as a novel genetic risk and prognostic factor for pulmonary arterial hypertension in systemic lupus erythematosus patients (Qian et al., 2023), are associated with heart disease, stroke, diabetes, and hypertension among subjects with Graves’ disease (Liao et al., 2022) and may influence hypertension and renal outcomes in patients with membranous nephropathy (Fan et al., 2021). However, a direct association of HLA-DQA1 with serum lipids is not reported in the literature. Our study suggests a potential role for HLA-DQA1 in biological context for the development of hypertension in patients with altered serum lipid levels.
Among the associations only found in participants with diabetes, a TAP1 gene variant was associated with DBP. The transport associated with antigen processing 1 (TAP1) gene polymorphism at codon 637 is associated with hypertension with the GG genotype being linked to higher SBP and DBP (Shen et al., 2007). However, the exact biological role of TAP1 in the pathophysiology of hypertension is not yet fully understood.
We found the HEYL gene, encoding a member of the hairy and enhancer of split-related (HESR) family of basic helix-loop-helix (bHLH)-type transcription factors, associated with HDL only in the diabetic cohort. Although we have not found a specific relationship between the HEYL gene and HDL levels in the literature, HDL protects against inflammatory activation of the arterial system and may be involved in the regulation of Notch signaling, which in turn can impact the expression of HEYL and other related genes (Briot et al., 2016). In our participants with diabetes, the HLA-DQA1-rs1048372 variant showed a significant and different direction of effect over serum TG levels, compared with participants without diabetes. A recent GWAS in 56,664 individuals has identified other variant in HLA-DQA1 (rs17426593) associated with hypothyroidism (Kim and Park, 2023). In those individuals, the serum TG concentrations were also positively associated with hypothyroidism risk (Kim and Park, 2023). But given that no specific relationship between either HEYL and HDL levels or HLA-DQA1 and TG levels has been reported yet, further research may be needed to fully understand the connection between these genes and serum lipid levels.
A further variant with significant association with TG in participants with diabetes mapped to the tyrosine hydroxylase (TH) gene, coding an enzyme that catalyzes the first step in the synthesis of catecholamines, such as dopamine and noradrenaline. Reduced TH expression in brown adipose tissue can impact various physiological processes, including lipid metabolism. In TH heterozygous mice, the reduction of TH in brown adipose tissue affected the catecholaminergic response to cold exposure, leading to implications for cold adaptation (Vázquez et al., 2018). In rats, knockdown in the hypothalamus led to elevated plasma TG levels, inducing obesity and glucose intolerance (Zhang et al., 2023). Furthermore, there is evidence that circulating TG can influence dopamine-associated behaviours (Berland et al., 2020). These findings suggest a potential link between TH and triglycerides, indicating a potential role in lipid metabolism and related physiological functions.
For the rest of the genes with associations only in the cohort with diabetes (ZZEF1 and Chol, ATXN7, PC, HLA-DQA1 and HDL), this study is the first to identify an association and more research will be required to establish a comprehensive understanding of their impact.
We used a relatively large cohort, the UK Biobank, to investigate an extensive selection of variants in both mtDNA and NEMG in this cross-sectional study with sufficient power to detect associations in common variants, but our power was reduced for less common variants in sub-group analysis for diabetes (84.2% for NEMG, 94% for mitochondrial variants). Nonetheless, we have confirmed known and identified novel significant associations with CVDs. We have explored a variety of traits and approached cardiovascular conditions through three different phenotypes (CVD per se, CAD and hypertension) using standard definitions taken from the disease and medication information provided by the UK Biobank, combining data from different variables; these definitions, based on variables participant operations (Data Field #20004), non-cancer illness (Data Field #20002), and other medications (Data Field #20003) as ICD-10 codes were not available, may differ from those used by other authors. Although our study was limited to participants with European ancestry, it successfully identified mitochondrial gene variation associated with cardiovascular traits that have also been reported in other ethnic populations; however broader investigation in appropriately powered cohorts with all ethnicities would be necessary to confirm associations in diverse groups. Our study has also pinpointed mitochondrial and NEMGs variants capable to influence multiple phenotypes, as common nexus between CAD, CVD and hypertension, exhibiting pleiotropy or as a consequence of a shared genetic structure in these conditions. However, determining whether a phenotype is specifically associated with mitochondrial related variants, or influenced by other factors may be quite complex due to the inherent variability and pleiotropy of mtDNA variants.
Our study highlights the relevance of variants in both mitochondrial genes and NEMG in the context of CVDs, especially CAD and hypertension, and CVD modifiable risk factors such as serum lipids in people with and without diabetes. We have linked mitochondrial haplogroup U to CVD and consistently demonstrated an association between mitochondrial haplogroups J and T and CVD, confirming previous results. We have also proposed new markers of hypertension and serum lipids in the context of diabetes. The findings of our study also make evident connections between the etiological pathways underlying CVDs, blood pressure and serum lipids. Our results place NOS3 gene as the common nexus between CAD, CVD and hypertension, with serum lipids connected to CVD through SLC22A2, in combination with TOMM40 for CAD and to hypertension through HLA-DQA1.
These results may help future endeavors examining the common mechanisms underlying these traits to elucidate the biological pathways responsible for CVDs.
The original contributions presented in the study are included in the article/Supplementary Material, further inquiries can be directed to the corresponding author. The data analyzed in this study was obtained from the UK Biobank, through application number 14259.
The studies involving humans were approved by the UK Biobank Ethics Advisory Committee. The studies were conducted in accordance with the local legislation and institutional requirements. The participants provided their written informed consent to participate in this study.
MC-G: Conceptualization, Data curation, Formal Analysis, Investigation, Methodology, Project administration, Resources, Software, Supervision, Validation, Visualization, Writing–original draft, Writing–review and editing. JM: Data curation, Formal Analysis, Writing–original draft, Writing–review and editing, Investigation, Software, Visualization. BB-J: Data curation, Formal Analysis, Writing–original draft, Writing–review and editing, Investigation, Software, Visualization. CH: Formal Analysis, Writing–review and editing. RS: Data curation, Writing–review and editing. RC: Data curation, Writing–review and editing. EB: Resources, Validation, Writing–review and editing. RD: Validation, Writing–review and editing. CG: Funding acquisition, Resources, Validation, Writing–review and editing. APM: Conceptualization, Data curation, Funding acquisition, Methodology, Project administration, Resources, Supervision, Validation, Writing–original draft, Writing–review and editing. AJM: Conceptualization, Funding acquisition, Investigation, Methodology, Project administration, Resources, Supervision, Validation, Writing–original draft, Writing–review and editing.
The author(s) declare that financial support was received for the research, authorship, and/or publication of this article. This work was supported by Science Foundation Ireland and the Department for the Economy, Northern Ireland Investigator Program Partnership Award (15/IA/3152 to MC-G and CH); RS and RC were in receipt of PhD studentships from the NI Department for the Economy; BB-J and JM were supported by the Erasmus + EU programme for education, training, youth and sport for master students. This work was also supported by a US-Ireland Program Award from HSC Research and Development (R&D) division STL/5569/19, and the Medical Research Council, MC_PC_20026.
This research has been conducted using the UK Biobank Resource under Application Number 14259. Some elements of this research were included in PhD theses submitted by RC and RS at Queen´s University Belfast, UK, and Master’s theses by BB-J and JM at University of Granada, Spain.
The authors declare that the research was conducted in the absence of any commercial or financial relationships that could be construed as a potential conflict of interest.
The author(s) declared that they were an editorial board member of Frontiers, at the time of submission. This had no impact on the peer review process and the final decision.
All claims expressed in this article are solely those of the authors and do not necessarily represent those of their affiliated organizations, or those of the publisher, the editors and the reviewers. Any product that may be evaluated in this article, or claim that may be made by its manufacturer, is not guaranteed or endorsed by the publisher.
The Supplementary Material for this article can be found online at: https://www.frontiersin.org/articles/10.3389/fphys.2024.1395371/full#supplementary-material
ACEi, angiotensin-converting enzyme (ACE) inhibitors; ARBs, angiotensin II receptor blockers; CABG, coronary artery bypass graft; CAD, coronary artery disease; Chol, cholesterol; CKD, chronic kidney disease; CVD, cardiovascular disease; DBP, diastolic blood pressure; DKD, diabetic kidney disease; DM, diabetes mellitus; ESRD, end-stage renal disease; HDL, high-density lipoproteins; LDL, low-density lipoproteins; NEMG, nuclear-encoded mitochondrial genes; PTCA, percutaneous transluminal coronary angioplasty; RAASi, renin-angiotensin-aldosterone system inhibitors; SBP, systolic blood pressure; SNP, single nucleotide polymorphism; TG, triglycerides.
Aragam K. G., Jiang T., Goel A., Kanoni S., Wolford B. N., Atri D. S., et al. (2022). Discovery and systematic characterization of risk variants and genes for coronary artery disease in over a million participants. Nat. Genet. 54, 1803–1815. doi:10.1038/s41588-022-01233-6
Bakis H., Trimouille A., Vermorel A., Redonnet I., Goizet C., Boulestreau R., et al. (2020). Adult onset tubulo-interstitial nephropathy in MT-ND5-related phenotypes. Clin. Genet. 97, 628–633. doi:10.1111/CGE.13670
Bar N., Korem T., Weissbrod O., Zeevi D., Rothschild D., Leviatan S., et al. (2020). A reference map of potential determinants for the human serum metabolome. Nature 588, 135–140. doi:10.1038/S41586-020-2896-2
Benn M., Schwartz M., Nordestgaard B. G., Tybjaerg-Hansen A. (2008). Mitochondrial haplogroups: ischemic cardiovascular disease, other diseases, mortality, and longevity in the general population. Circulation 117, 2492–2501. doi:10.1161/CIRCULATIONAHA.107.756809
Berg K., Dahlen G., Borresen A. L. (1979). Lp(a) phenotypes, other lipoprotein parameters, and a family history of coronary heart disease in middle-aged males. Clin. Genet. 16, 347–352. doi:10.1111/J.1399-0004.1979.TB01014.X
Berland C., Montalban E., Perrin E., Di Miceli M., Nakamura Y., Martinat M., et al. (2020). Circulating triglycerides gate dopamine-associated behaviors through DRD2-expressing neurons. Cell Metab. 31, 773–790. doi:10.1016/J.CMET.2020.02.010
Brandon M. C., Lott M. T., Nguyen K. C., Spolim S., Navathe S. B., Baldi P., et al. (2005). MITOMAP: a human mitochondrial genome database—2004 update. Nucleic Acids Res. 33, D611–D613. doi:10.1093/nar/gki079
Bregman J. A., Herren D. J., Estopinal C. B., Chocron I. M., Harlow P. A., Warden C., et al. (2017). Mitochondrial haplogroups affect severity but not prevalence of diabetic retinopathy. Invest Ophthalmol. Vis. Sci. 58, 1346–1351. doi:10.1167/iovs.16-20616
Briot A., Bouloumié A., Iruela-Arispe M. L. (2016). Notch, lipids, and endothelial cells. Curr. Opin. Lipidol. 27, 513–520. doi:10.1097/MOL.0000000000000337
Bycroft C., Freeman C., Petkova D., Band G., Elliott L. T., Sharp K., et al. (2018). The UK Biobank resource with deep phenotyping and genomic data. Nature 562, 203–209. doi:10.1038/s41586-018-0579-z
Cadby G., Melton P. E., McCarthy N. S., Giles C., Mellett N. A., Huynh K., et al. (2020). Heritability of 596 lipid species and genetic correlation with cardiovascular traits in the Busselton Family Heart Study. J. Lipid Res. 61, 537–545. doi:10.1194/jlr.RA119000594
Calabrese C., Pyle A., Griffin H., Coxhead J., Hussain R., Braund P. S., et al. (2022). Heteroplasmic mitochondrial DNA variants in cardiovascular diseases. PLoS Genet. 18, e1010068. doi:10.1371/JOURNAL.PGEN.1010068
Calvo S. E., Clauser K. R., Mootha V. K. (2016). MitoCarta2.0: an updated inventory of mammalian mitochondrial proteins. Nucleic Acids Res. 44, D1251–D1257. doi:10.1093/nar/gkv1003
Campbell T., Slone J., Huang T. (2022). Mitochondrial genome variants as a cause of mitochondrial cardiomyopathy. Cells 11, 2835. doi:10.3390/CELLS11182835
Cañadas-Garre M., Baños-Jaime B., Maqueda J. J., Smyth L. J., Cappa R., Skelly R., et al. (2024). Genetic variants affecting mitochondrial function provide further insights for kidney disease. BMC Genomics 25, 576. doi:10.1186/S12864-024-10449-1
Cañadas-Garre M., Kunzmann A. T., Anderson K., Brennan E. P., Doyle R., Patterson C. C., et al. (2023). Albuminuria-related genetic biomarkers: replication and predictive evaluation in individuals with and without diabetes from the UK Biobank. Int. J. Mol. Sci. 24, 11209. doi:10.3390/ijms241311209
Castro M. G., Huerta C., Reguero J. R., Soto M. I., Domenech E., Alvarez V., et al. (2006). Mitochondrial DNA haplogroups in Spanish patients with hypertrophic cardiomyopathy. Int. J. Cardiol. 112, 202–206. doi:10.1016/j.ijcard.2005.09.008
CDC (2023). Coronary artery disease | cdc.gov. Available at: https://www.cdc.gov/heartdisease/coronary_ad.htm (Accessed December 28, 2023).
Chaban Y., Boekema E. J., Dudkina N. V. (2014). Structures of mitochondrial oxidative phosphorylation supercomplexes and mechanisms for their stabilisation. Biochim. Biophys. Acta 1837, 418–426. doi:10.1016/j.bbabio.2013.10.004
Chambers J. C., Zhang W., Lord G. M., van der Harst P., Lawlor D. A., Sehmi J. S., et al. (2010). Genetic loci influencing kidney function and chronic kidney disease. Nat. Genet. 42, 373–375. doi:10.1038/ng.566
Chan D. C. (2006). Mitochondria: dynamic organelles in disease, aging, and development. Cell 125, 1241–1252. doi:10.1016/j.cell.2006.06.010
Chang C., Grail I. (2020). Human longevity, I., and department of biomedical data science, S PLINK 2.00 alpha. Available at: https://www.cog-genomics.org/plink/2.0/.
Chang C. C., Chow C. C., Tellier L. C. A. M., Vattikuti S., Purcell S. M., Lee J. J. (2015). Second-generation PLINK: rising to the challenge of larger and richer datasets. Gigascience 4, 7–16. doi:10.1186/s13742-015-0047-8
Chelala C., Khan A., Lemoine N. R. (2009). SNPnexus: a web database for functional annotation of newly discovered and public domain single nucleotide polymorphisms. Bioinformatics 25, 655–661. doi:10.1093/bioinformatics/btn653
Chen F., Li Y.-M., Yang L.-Q., Zhong C.-G., Zhuang Z.-X. (2016). Association of NOS2 and NOS3 gene polymorphisms with susceptibility to type 2 diabetes mellitus and diabetic nephropathy in the Chinese Han population. IUBMB Life 68, 516–525. doi:10.1002/iub.1513
Chen H., Wang T., Yang J., Huang S., Zeng P. (2020). Improved detection of potentially pleiotropic genes in coronary artery disease and chronic kidney disease using GWAS summary statistics. Front. Genet. 11, 592461. doi:10.3389/FGENE.2020.592461
Chen S., Sarasua S. M., Davis N. J., DeLuca J. M., Boccuto L., Thielke S. M., et al. (2022). TOMM40 genetic variants associated with healthy aging and longevity: a systematic review. BMC Geriatr. 22, 667. doi:10.1186/s12877-022-03337-4
Christiansen M. K., Larsen S. B., Nyegaard M., Neergaard-Petersen S., Ajjan R., Würtz M., et al. (2017a). Coronary artery disease-associated genetic variants and biomarkers of inflammation. PLoS One 12, e0180365. doi:10.1371/journal.pone.0180365
Christiansen M. K., Nyegaard M., Larsen S. B., Grove E. L., Würtz M., Neergaard-Petersen S., et al. (2017b). A genetic risk score predicts cardiovascular events in patients with stable coronary artery disease. Int. J. Cardiol. 241, 411–416. doi:10.1016/j.ijcard.2017.04.045
Companioni O., Rodríguez Esparragón F., Fernández-Aceituno A. M., Rodríguez Pérez J. C. (2011). Genetic variants, cardiovascular risk and genome-wide association studies. Rev. Esp. Cardiol. 64, 509–514. doi:10.1016/j.recesp.2011.01.010
Coronary Artery Disease (CAD) (2023). Symptoms and treatment. Available at: https://my.clevelandclinic.org/health/diseases/16898-coronary-artery-disease (Accessed December 28, 2023).
Cotter D., Guda P., Fahy E., Subramaniam S. (2004). MitoProteome: mitochondrial protein sequence database and annotation system. Nucleic Acids Res. 32, D463–D467. doi:10.1093/nar/gkh048
Cozzolino M., Mangano M., Stucchi A., Ciceri P., Conte F., Galassi A. (2018). Cardiovascular disease in dialysis patients. Nephrol. Dial. Transplant. 33, iii28–iii34. doi:10.1093/NDT/GFY174
Dai X., Wiernek S., Evans J. P., Runge M. S. (2016). Genetics of coronary artery disease and myocardial infarction. World J. Cardiol. 8, 1–23. doi:10.4330/WJC.V8.I1.1
Davies G., Armstrong N., Bis J. C., Bressler J., Chouraki V., Giddaluru S., et al. (2015). Genetic contributions to variation in general cognitive function: a meta-analysis of genome-wide association studies in the CHARGE consortium (N = 53949). Mol. Psychiatry 20, 183–192. doi:10.1038/mp.2014.188
Davyson E., Shen X., Gadd D. A., Bernabeu E., Hillary R. F., McCartney D. L., et al. (2023). Metabolomic investigation of major depressive disorder identifies a potentially causal association with polyunsaturated fatty acids. Biol. Psychiatry 94, 630–639. doi:10.1016/J.BIOPSYCH.2023.01.027
Dayem Ullah A. Z., Lemoine N. R., Chelala C. (2012). SNPnexus: a web server for functional annotation of novel and publicly known genetic variants (2012 update). Nucleic Acids Res. 40, W65–W70. doi:10.1093/nar/gks364
Dayem Ullah A. Z., Lemoine N. R., Chelala C. (2013). A practical guide for the functional annotation of genetic variations using SNPnexus. Brief. Bioinform 14, 437–447. doi:10.1093/bib/bbt004
Dayem Ullah A. Z., Oscanoa J., Wang J., Nagano A., Lemoine N. R., Chelala C. (2018). SNPnexus: assessing the functional relevance of genetic variation to facilitate the promise of precision medicine. Nucleic Acids Res. 46, W109-W113–W113. doi:10.1093/nar/gky399
Deelen J., Beekman M., Uh H., Helmer Q., Kuningas M., Christiansen L., et al. (2011). Genome-wide association study identifies a single major locus contributing to survival into old age; the APOE locus revisited. Aging Cell 10, 686–698. doi:10.1111/j.1474-9726.2011.00705.x
Deelen J., Beekman M., Uh H.-W., Broer L., Ayers K. L., Tan Q., et al. (2014). Genome-wide association meta-analysis of human longevity identifies a novel locus conferring survival beyond 90 years of age. Hum. Mol. Genet. 23, 4420–4432. doi:10.1093/hmg/ddu139
Deloukas P., Kanoni S., Willenborg C., Farrall M., Assimes T. L., Thompson J. R., et al. (2013). Large-scale association analysis identifies new risk loci for coronary artery disease. Nat. Genet. 45, 25–33. doi:10.1038/ng.2480
Denny J. C., Bastarache L., Ritchie M. D., Carroll R. J., Zink R., Mosley J. D., et al. (2013). Systematic comparison of phenome-wide association study of electronic medical record data and genome-wide association study data. Nat. Biotechnol. 31, 1102–1110. doi:10.1038/nbt.2749
Dolezal P., Likic V., Tachezy J., Lithgow T. (2006). Evolution of the molecular machines for protein import into mitochondria. Science 313, 314–318. doi:10.1126/science.1127895
Drobni Z. D., Kolossvary M., Karady J., Jermendy A. L., Tarnoki A. D., Tarnoki D. L., et al. (2022). Heritability of coronary artery disease: insights from a classical twin study. Circ. Cardiovasc Imaging 15, e013348. doi:10.1161/CIRCIMAGING.121.013348
Echouffo-Tcheugui J. B., Jain M., Cheng S. (2020). Breaking through the surface: more to learn about lipids and cardiovascular disease. J. Clin. Investigation 130, 1084–1086. doi:10.1172/JCI134696
Einarson T. R., Acs A., Ludwig C., Panton U. H. (2018). Prevalence of cardiovascular disease in type 2 diabetes: a systematic literature review of scientific evidence from across the world in 2007–2017. Cardiovasc. Diabetol. 17 (1 17), 83–19. doi:10.1186/S12933-018-0728-6
Ellis J., Lange E. M., Li J., Dupuis J., Baumert J., Walston J. D., et al. (2014). Large multiethnic Candidate Gene Study for C-reactive protein levels: identification of a novel association at CD36 in African Americans. Hum. Genet. 133, 985–995. doi:10.1007/s00439-014-1439-z
Elsaid A., Samir eid O., Said S. B., Zahran R. F. (2021). Association of NOS3 (rs 2070744) and SOD2Val16Ala (rs4880) gene polymorphisms with increased risk of ESRD among Egyptian patients. J. Genet. Eng. and Biotechnol. 19, 158. doi:10.1186/S43141-021-00260-W
Estopinal C. B., Chocron I. M., Parks M. B., Wade E. A., Roberson R. M., Burgess L. G., et al. (2014). Mitochondrial haplogroups are associated with severity of diabetic retinopathy. Invest Ophthalmol. Vis. Sci. 55, 5589–5595. doi:10.1167/iovs.14-15149
Fan S., Wang Q., Wang A. Y., Zhang P., Zhong X., Chen S., et al. (2021). The association between variants in PLA2R and HLA-DQA1 and renal outcomes in patients with primary membranous nephropathy in Western China. BMC Med. Genomics 14, 123. doi:10.1186/s12920-021-00969-0
Feitosa M. F., Kraja A. T., Chasman D. I., Sung Y. J., Winkler T. W., Ntalla I., et al. (2018). Novel genetic associations for blood pressure identified via gene-alcohol interaction in up to 570K individuals across multiple ancestries. PLoS One 13, e0198166. doi:10.1371/JOURNAL.PONE.0198166
Feng Q., Wei W.-Q., Levinson R. T., Mosley J. D., Stein C. M. (2017). Replication and fine-mapping of genetic predictors of lipid traits in African-Americans. J. Hum. Genet. 62, 895–901. doi:10.1038/jhg.2017.55
Ference B. A., Ginsberg H. N., Graham I., Ray K. K., Packard C. J., Bruckert E., et al. (2017). Low-density lipoproteins cause atherosclerotic cardiovascular disease. 1. Evidence from genetic, epidemiologic, and clinical studies. A consensus statement from the European Atherosclerosis Society Consensus Panel. Eur. Heart J. 38, 2459–2472. doi:10.1093/eurheartj/ehx144
Foscolou A., Georgousopoulou E., Magriplis E., Naumovski N., Rallidis L., Matalas A. L., et al. (2018). The mediating role of Mediterranean diet on the association between Lp(a) levels and cardiovascular disease risk: a 10-year follow-up of the ATTICA study. Clin. Biochem. 60, 33–37. doi:10.1016/J.CLINBIOCHEM.2018.07.011
Fox C. S., Matsushita K., Woodward M., Bilo H. J. G., Chalmers J., Lambers Heerspink H. J., et al. (2012). Associations of kidney disease measures with mortality and end-stage renal disease in individuals with and without diabetes: a meta-analysis. Lancet 380, 1662–1673. doi:10.1016/S0140-6736(12)61350-6
Fuku N., Park K. S., Yamada Y., Nishigaki Y., Cho Y. M., Matsuo H., et al. (2007). Mitochondrial haplogroup N9a confers resistance against type 2 diabetes in Asians. Am. J. Hum. Genet. 80, 407–415. doi:10.1086/512202
Giri A., Hellwege J. N., Keaton J. M., Park J., Qiu C., Warren H. R., et al. (2019). Trans-ethnic association study of blood pressure determinants in over 750,000 individuals. Nat. Genet. 51, 51–62. doi:10.1038/S41588-018-0303-9
Goff D. C., Lloyd-Jones D. M., Bennett G., Coady S., D’Agostino R. B., Gibbons R., et al. (2014). 2013 ACC/AHA guideline on the assessment of cardiovascular risk: a report of the American college of cardiology/American heart association task force on practice guidelines. Circulation 129, S49–S73. doi:10.1161/01.cir.0000437741.48606.98
Gray M. W., Gray M. W., Burger G., Lang B. F. (2008). Mitochondrial evolution. Science 1476, 1476–1481. doi:10.1126/science.283.5407.1476
Gründemann D., Schömig E. (2000). Gene structures of the human non-neuronal monoamine transporters EMT and OCT2. Hum. Genet. 106, 627–635. doi:10.1007/S004390000309
Gui W., Qiu C., Shao Q., Li J. (2021). Associations of vascular risk factors, APOE and TOMM40 polymorphisms with cognitive function in dementia-free Chinese older adults: a community-based study. Front. Psychiatry 12, 617773. doi:10.3389/fpsyt.2021.617773
Gunawan A., Fajar J. K., Tamara F., Mahendra A. I., Ilmawan M., Purnamasari Y., et al. (2020). Nitride oxide synthase 3 and klotho gene polymorphisms in the pathogenesis of chronic kidney disease and age-related cognitive impairment: a systematic review and meta-analysis. F1000Res 9, 252. doi:10.12688/F1000RESEARCH.22989.2
Guo H., Guo L., Yuan Y., Liang X. Y., Bi R. (2022). Co-Occurrence of m.15992A>G and m.15077G>A is associated with a high penetrance of maternally inherited hypertension in a Chinese pedigree. Am. J. Hypertens. 35, 96–102. doi:10.1093/AJH/HPAB123
Guo Y., Lanktree M. B., Taylor K. C., Hakonarson H., Lange L. A., Keating B. J., et al. (2013). Gene-centric meta-analyses of 108 912 individuals confirm known body mass index loci and reveal three novel signals. Hum. Mol. Genet. 22, 184–201. doi:10.1093/hmg/dds396
Hartiala J. A., Han Y., Jia Q., Hilser J. R., Huang P., Gukasyan J., et al. (2021). Genome-wide analysis identifies novel susceptibility loci for myocardial infarction. Eur. Heart J. 42, 919–933. doi:10.1093/EURHEARTJ/EHAA1040
Heidari M. M., Mirfakhradini F. S., Tayefi F., Ghorbani S., Khatami M., Hadadzadeh M. (2020). Novel point mutations in mitochondrial MT-CO2 gene may Be risk factors for coronary artery disease. Appl. Biochem. Biotechnol. 191, 1326–1339. doi:10.1007/S12010-020-03275-0
Hoffmann T. J., Ehret G. B., Nandakumar P., Ranatunga D., Schaefer C., Kwok P. Y., et al. (2017). Genome-wide association analyses using electronic health records identify new loci influencing blood pressure variation. Nat. Genet. 49, 54–64. doi:10.1038/ng.3715
Humphries A. D., Streimann I. C., Stojanovski D., Johnston A. J., Yano M., Hoogenraad N. J., et al. (2005). Dissection of the mitochondrial import and assembly pathway for human Tom40. J. Biol. Chem. 280, 11535–11543. doi:10.1074/jbc.M413816200
Inouye M., Abraham G., Nelson C. P., Wood A. M., Sweeting M. J., Dudbridge F., et al. (2018a). Genomic risk prediction of coronary artery disease in 480,000 adults: implications for primary prevention. J. Am. Coll. Cardiol. 72, 1883–1893. doi:10.1016/J.JACC.2018.07.079
Inouye M., Abraham G., Nelson C. P., Wood A. M., Sweeting M. J., Dudbridge F., et al. (2018b). Genomic risk prediction of coronary artery disease in nearly 500,000 adults: implications for early screening and primary prevention. bioRxiv, 250712. doi:10.1101/250712
Jankowski J., Floege J., Fliser D., Böhm M., Marx N. (2021). Cardiovascular disease in chronic kidney disease: pathophysiological insights and therapeutic options. Circulation 143, 1157–1172. doi:10.1161/CIRCULATIONAHA.120.050686
Kaplan J. (2020). fastDummies: fast creation of dummy (binary) columns and rows from categorical variables. Available at: https://cran.r-project.org/package=fastDummies.
Khera A. V., Kathiresan S. (2017). Genetics of coronary artery disease: discovery, biology and clinical translation. Nat. Rev. Genet. 18, 331–344. doi:10.1038/nrg.2016.160
Kichaev G., Bhatia G., Loh P. R., Gazal S., Burch K., Freund M. K., et al. (2019). Leveraging polygenic functional enrichment to improve GWAS power. Am. J. Hum. Genet. 104, 65–75. doi:10.1016/J.AJHG.2018.11.008
Kim D. S., Park S. (2023). Interactions between polygenetic variants and lifestyle factors in hypothyroidism: a hospital-based cohort study. Nutrients 15, 3850. doi:10.3390/nu15173850
Kishore P., Kim S. H., Crandall J. P. (2012). Glycemic control and cardiovascular disease: what’s a doctor to do? Curr. Diab Rep. 12, 255–264. doi:10.1007/S11892-012-0268-5
Kofler B., Mueller E. E., Eder W., Stanger O., Maier R., Weger M., et al. (2009). Mitochondrial DNA haplogroup T is associated with coronary artery disease and diabetic retinopathy: a case control study. BMC Med. Genet. 10, 35. doi:10.1186/1471-2350-10-35
Koskeridis F., Evangelou E., Said S., Boyle J. J., Elliott P., Dehghan A., et al. (2022). Pleiotropic genetic architecture and novel loci for C-reactive protein levels. Nat. Commun. 13, 6939. doi:10.1038/S41467-022-34688-6
Köttgen A., Pattaro C., Böger C. A., Fuchsberger C., Olden M., Glazer N. L., et al. (2010). New loci associated with kidney function and chronic kidney disease. Nat. Genet. 42, 376–384. doi:10.1038/ng.568
Lempiäinen H., Brænne I., Michoel T., Tragante V., Vilne B., Webb T. R., et al. (2018). Network analysis of coronary artery disease risk genes elucidates disease mechanisms and druggable targets. Sci. Rep. 8, 3434. doi:10.1038/S41598-018-20721-6
Li Q., Liu F., Zheng T. S., Tang J. L., Lu H. J., Jia W. P. (2010). SLC22A2 gene 808 G/T variant is related to plasma lactate concentration in Chinese type 2 diabetics treated with metformin. Acta Pharmacol. Sin. 31, 184–190. doi:10.1038/APS.2009.189
Liao W.-L., Huang Y.-N., Chang Y.-W., Liu T.-Y., Lu H.-F., Tiao Z.-Y., et al. (2023). Combining polygenic risk scores and human leukocyte antigen variants for personalized risk assessment of type 1 diabetes in the Taiwanese population. Diabetes Obes. Metab. 25, 2928–2936. doi:10.1111/dom.15187
Liao W. L., Liu T. Y., Cheng C. F., Chou Y. P., Wang T. Y., Chang Y. W., et al. (2022). Analysis of HLA variants and Graves’ disease and its comorbidities using a high resolution imputation system to examine electronic medical health records. Front. Endocrinol. (Lausanne) 13, 842673. doi:10.3389/FENDO.2022.842673
Limas C. J., Limas C., Goldenberg I. F., Blair R. (1995). Possible involvement of the HLA-DQB1 gene in susceptibility and resistance to human dilated cardiomyopathy. Am. Heart. J. 129, 1141–1144. doi:10.1016/0002-8703(95)90395-X
Lin K.-L., Chen S.-D., Lin K.-J., Liou C.-W., Chuang Y.-C., Wang P.-W., et al. (2021). Quality matters? The involvement of mitochondrial quality control in cardiovascular disease. Front. Cell Dev. Biol. 9, 636295. doi:10.3389/fcell.2021.636295
Littlejohns T. J., Sudlow C., Allen N. E., Collins R. (2019). UK Biobank: opportunities for cardiovascular research. Eur. Heart J. 40, 1158–1166. doi:10.1093/EURHEARTJ/EHX254
Liu C., Kraja A. T., Smith J. A., Brody J. A., Franceschini N., Bis J. C., et al. (2016). Meta-analysis identifies common and rare variants influencing blood pressure and overlapping with metabolic trait loci. Nat. Genet. 48, 1162–1170. doi:10.1038/NG.3660
Liu H., Liu X., Zhou J., Li T. (2022). Mitochondrial DNA is a vital driving force in ischemia-reperfusion injury in cardiovascular diseases. Oxid. Med. Cell Longev. 2022, 6235747. doi:10.1155/2022/6235747
Liu M., Li X.-C., Lu L., Cao Y., Sun R.-R., Chen S., et al. (2014). Cardiovascular disease and its relationship with chronic kidney disease. Eur. Rev. Med. Pharmacol. Sci. 18, 2918–2926.
Liu W., Li W. M., Sun N. L. (2005). HLA-DQA1, -DQB1 polymorphism and genetic susceptibility to idiopathic dilated cardiomyopathy in Hans of northern China. Ann. Hum. Genet. 69, 382–388. doi:10.1111/J.1529-8817.2005.00166.X
Liu X., Sun X., Zhang Y., Jiang W., Lai M., Wiggins K. L., et al. (2023). Association between whole blood–derived mitochondrial DNA copy number, low-density lipoprotein cholesterol, and cardiovascular disease risk. J. Am. Heart Assoc. 12, e029090. doi:10.1161/JAHA.122.029090
Liu Y., Ma H., Zhu Q., Zhang B., Yan H., Li H., et al. (2019). A genome-wide association study on lipoprotein (a) levels and coronary artery disease severity in a Chinese population. J. Lipid Res. 60, 1440–1448. doi:10.1194/JLR.P091009
Lodish H., Berk A., Zipursky S., Lawrence Matsudaira P., Baltimore D., Darnell J. (2012). Electron transport and oxidative phosphorylation. Mol. Cell Biol. 474. doi:10.1016/S1470-8175(01)00023-6
Lotta L. A., Pietzner M., Stewart I. D., Wittemans L. B. L., Li C., Bonelli R., et al. (2021). A cross-platform approach identifies genetic regulators of human metabolism and health. Nat. Genet. 53, 54–64. doi:10.1038/S41588-020-00751-5
Ma Z.-J., Sun P., Guo G., Zhang R., Chen L.-M. (2013). Association of the HLA-DQA1 and HLA-DQB1 alleles in type 2 diabetes mellitus and diabetic nephropathy in the han ethnicity of China. J. Diabetes Res. 2013, 452537. doi:10.1155/2013/452537
Mack S., Coassin S., Rueedi R., Yousri N. A., Seppälä I., Gieger C., et al. (2017). A genome-wide association meta-analysis on lipoprotein (a) concentrations adjusted for apolipoprotein (a) isoforms. J. Lipid Res. 58, 1834–1844. doi:10.1194/jlr.M076232
Mahmoodi B. K., Matsushita K., Woodward M., Blankestijn P. J., Cirillo M., Ohkubo T., et al. (2012). Associations of kidney disease measures with mortality and end-stage renal disease in individuals with and without hypertension: a meta-analysis. Lancet 380, 1649–1661. doi:10.1016/S0140-6736(12)61272-0
Marino B., Digilio M. C. (2000). Congenital heart disease and genetic syndromes: specific correlation between cardiac phenotype and genotype. Cardiovasc Pathol. 9, 303–315. doi:10.1016/s1054-8807(00)00050-8
Medina A. M., Zubero E. E., Jiménez M. A. A., Barragan S. A. A., García C. A. L., Ramos J. J. G., et al. (2018). NOS3 polymorphisms and chronic kidney disease. J. Bras. Nefrol. 40, 273–277. doi:10.1590/2175-8239-JBN-3824
Meiklejohn C. D., Holmbeck M. A., Siddiq M. A., Abt D. N., Rand D. M., Montooth K. L. (2013). An incompatibility between a mitochondrial tRNA and its nuclear-encoded tRNA synthetase compromises development and fitness in Drosophila. PLoS Genet. 9, e1003238. doi:10.1371/journal.pgen.1003238
Miao L., Yin R.-X., Pan S.-L., Yang S., Yang D.-Z., Lin W.-X. (2018). BCL3-PVRL2-TOMM40 SNPs, gene-gene and gene-environment interactions on dyslipidemia. Sci. Rep. 8, 6189. doi:10.1038/s41598-018-24432-w
Middelberg R. P., Ferreira M. A., Henders A. K., Heath A. C., Madden P. A., Montgomery G. W., et al. (2011). Genetic variants in LPL, OASL and TOMM40/APOE-C1-C2-C4 genes are associated with multiple cardiovascular-related traits. BMC Med. Genet. 12, 123. doi:10.1186/1471-2350-12-123
Muntean I., Togănel R., Benedek T. (2017). Genetics of congenital heart disease: past and present. Biochem. Genet. 55, 105–123. doi:10.1007/s10528-016-9780-7
National Institute for Health and Care Excellence [NICE] (2017). Hypertension in adults: diagnosis and management. Evidence review for targets NICE guideline NG136.
National Institute for Health and Care Excellenve (NICE) (2019). Hypertension in adults: diagnosis and management.
Nazarian A., Yashin A. I., Kulminski A. M. (2019). Genome-wide analysis of genetic predisposition to Alzheimer’s disease and related sex disparities. Alzheimers Res. Ther. 11, 5. doi:10.1186/s13195-018-0458-8
Nikpay M., Goel A., Won H. H., Hall L. M., Willenborg C., Kanoni S., et al. (2015). A comprehensive 1,000 Genomes-based genome-wide association meta-analysis of coronary artery disease. Nat. Genet. 47, 1121–1130. doi:10.1038/NG.3396
Nishigaki Y., Yamada Y., Fuku N., Matsuo H., Segawa T., Watanabe S., et al. (2007a). Mitochondrial haplogroup A is a genetic risk factor for atherothrombotic cerebral infarction in Japanese females. Mitochondrion 7, 72–79. doi:10.1016/j.mito.2006.11.002
Nishigaki Y., Yamada Y., Fuku N., Matsuo H., Segawa T., Watanabe S., et al. (2007b). Mitochondrial haplogroup N9b is protective against myocardial infarction in Japanese males. Hum. Genet. 120, 827–836. doi:10.1007/s00439-006-0269-z
Onengut-Gumuscu S., Chen W.-M., Robertson C. C., Bonnie J. K., Farber E., Zhu Z., et al. (2019). Type 1 diabetes risk in african-ancestry participants and utility of an ancestry-specific genetic risk score. Diabetes Care 42, 406–415. doi:10.2337/dc18-1727
Oscanoa J., Sivapalan L., Gadaleta E., Dayem Ullah A. Z., Lemoine N. R., Chelala C. (2020). SNPnexus: a web server for functional annotation of human genome sequence variation (2020 update). Nucleic Acids Res. 48, W185-W192–W192. doi:10.1093/NAR/GKAA420
Ouyang J., Wang H., Huang J. (2023). The role of lactate in cardiovascular diseases. Cell Commun. Signal. 21 (1), 317–414. doi:10.1186/S12964-023-01350-7
Padhi U. N., Mulkalwar M., Saikrishna L., Verma H. K., Bhaskar L. (2022). NOS3 gene intron 4 a/b polymorphism is associated with ESRD in autosomal dominant polycystic kidney disease patients. J. Bras. Nefrol. 44, 224–231. doi:10.1590/2175-8239-JBN-2021-0089
Pagliarini D. J., Calvo S. E., Chang B., Sheth S. A., Vafai S. B., Ong S.-E., et al. (2008). A mitochondrial protein compendium elucidates complex I disease biology. Cell 134, 112–123. doi:10.1016/j.cell.2008.06.016
Palacín M., Alvarez V., Martín M., Díaz M., Corao A. I., Alonso B., et al. (2011). Mitochondrial DNA and TFAM gene variation in early-onset myocardial infarction: evidence for an association to haplogroup H. Mitochondrion 11, 176–181. doi:10.1016/j.mito.2010.09.004
Palmer N. D., Kahali B., Kuppa A., Chen Y., Du X., Feitosa M. F., et al. (2021). Allele-specific variation at APOE increases nonalcoholic fatty liver disease and obesity but decreases risk of Alzheimer’s disease and myocardial infarction. Hum. Mol. Genet. 30, 1443–1456. doi:10.1093/hmg/ddab096
Pattaro C., Teumer A., Gorski M., Chu A. Y., Li M., Mijatovic V., et al. (2016). Genetic associations at 53 loci highlight cell types and biological pathways relevant for kidney function. Nat. Commun. 7, 10023. doi:10.1038/ncomms10023
Piotrowska-Nowak A., Elson J. L., Sobczyk-Kopciol A., Piwonska A., Puch-Walczak A., Drygas W., et al. (2018). New mtDNA association model, MutPred variant load, suggests individuals with multiple mildly deleterious mtDNA variants are more likely to suffer from atherosclerosis. Front. Genet. 9, 702. doi:10.3389/fgene.2018.00702
Plotnikov D., Huang Y., Khawaja A. P., Foster P. J., Zhu Z., Guggenheim J. A., et al. (2022). High blood pressure and intraocular pressure: a mendelian randomization study. Invest Ophthalmol. Vis. Sci. 63, 29. doi:10.1167/IOVS.63.6.29
Poznyak A. V., Ivanova E. A., Sobenin I. A., Yet S. F., Orekhov A. N. (2020). The role of mitochondria in cardiovascular diseases. Biol. (Basel) 9 (6), 137. doi:10.3390/BIOLOGY9060137
Qian J., Chen Y., Yang X., Wang Q., Zhao J., Deng X., et al. (2023). Association study identified HLA-DQA1 as a novel genetic risk of systemic lupus erythematosus-associated pulmonary arterial hypertension. Arthritis Rheumatol. 75, 2207–2215. doi:10.1002/art.42641
Radovica I., Fridmanis D., Silamikelis I., Nikitina-Zake L., Klovins J. (2014). Association between CETP, MLXIPL, and TOMM40 polymorphisms and serum lipid levels in a Latvian population. Meta Gene 2, 565–578. doi:10.1016/j.mgene.2014.07.006
Rai H., Fitzgerald S., Coughlan J. J., Spence M., Colleran R., Joner M., et al. (2023). Glu298Asp variant of the endothelial nitric oxide synthase gene and acute coronary syndrome or premature coronary artery disease: a systematic review and meta-analysis. Nitric Oxide 138–139, 85–95. doi:10.1016/J.NIOX.2023.07.001
Ray D., Chatterjee N. (2020). A powerful method for pleiotropic analysis under composite null hypothesis identifies novel shared loci between Type 2 Diabetes and Prostate Cancer. PLoS Genet. 16, e1009218. doi:10.1371/JOURNAL.PGEN.1009218
R Core Team (2024). R: A Language and Environment for Statistical Computing. Vienna: R Foundation for Statistical Computing. Available at: https://www.r-project.org/.
Razieh C., Zaccardi F., Miksza J., Davies M. J., Hansell A. L., Khunti K., et al. (2022). Differences in the risk of cardiovascular disease across ethnic groups: UK Biobank observational study. Nutr. Metab. Cardiovasc. Dis. 32, 2594–2602. doi:10.1016/J.NUMECD.2022.08.002
Richardson T. G., Leyden G. M., Wang Q., Bell J. A., Elsworth B., Smith G. D., et al. (2022). Characterising metabolomic signatures of lipid-modifying therapies through drug target mendelian randomisation. PLoS Biol. 20, e3001547. doi:10.1371/JOURNAL.PBIO.3001547
Rosa A., Fonseca B. V., Krug T., Manso H., Gouveia L., Albergaria I., et al. (2008). Mitochondrial haplogroup H1 is protective for ischemic stroke in Portuguese patients. BMC Med. Genet. 9, 57. doi:10.1186/1471-2350-9-57
Roumeliotis A., Roumeliotis S., Tsetsos F., Georgitsi M., Georgianos P. I., Stamou A., et al. (2021). Oxidative stress genes in diabetes mellitus type 2: association with diabetic kidney disease. Oxid. Med. Cell Longev. 2021, 2531062. doi:10.1155/2021/2531062
Safdar M., Ullah M., Wahab A., Hamayun S., Ur Rehman M., Khan M. A., et al. (2024). Genomic insights into heart health: exploring the genetic basis of cardiovascular disease. Curr. Probl. Cardiol. 49, 102182. doi:10.1016/j.cpcardiol.2023.102182
Sakaue S., Kanai M., Tanigawa Y., Karjalainen J., Kurki M., Koshiba S., et al. (2021). A cross-population atlas of genetic associations for 220 human phenotypes. Nat. Genet. 53, 1415–1424. doi:10.1038/S41588-021-00931-X
Salakhov R. R., Goncharova I. A., Makeeva O. A., Golubenko M. V., Kulish E. V., Kashtalap V. V., et al. (2014). TOMM40 gene polymorphisms association with lipid profile. Russ. J. Genet. 50, 198–204. doi:10.1134/S1022795413120090
Sandhu M. S., Waterworth D. M., Debenham S. L., Wheeler E., Papadakis K., Zhao J. H., et al. (2008). LDL-cholesterol concentrations: a genome-wide association study. Lancet 371, 483–491. doi:10.1016/S0140-6736(08)60208-1
Sawabe M., Tanaka M., Chida K., Arai T., Nishigaki Y., Fuku N., et al. (2011). Mitochondrial haplogroups A and M7a confer a genetic risk for coronary atherosclerosis in the Japanese elderly: an autopsy study of 1,536 patients. J. Atheroscler. Thromb. 18, 166–175. doi:10.5551/jat.6742
Schatz U., Tselmin S., Müller G., Julius U., Hohenstein B., Fischer S., et al. (2017). Most significant reduction of cardiovascular events in patients undergoing lipoproteinapheresis due to raised Lp(a) levels - a multicenter observational study. Atheroscler. Suppl. 30, 246–252. doi:10.1016/J.ATHEROSCLEROSISSUP.2017.05.047
Schoeler T., Speed D., Porcu E., Pirastu N., Pingault J. B., Kutalik Z. (2023). Participation bias in the UK Biobank distorts genetic associations and downstream analyses. Nat. Hum. Behav. 7, 1216–1227. doi:10.1038/S41562-023-01579-9
Scott R. A., Scott L. J., Mägi R., Marullo L., Gaulton K. J., Kaakinen M., et al. (2017). An expanded genome-wide association study of type 2 diabetes in Europeans. Diabetes 66, 2888–2902. doi:10.2337/db16-1253
Shadrina A. S., Shashkova T. I., Torgasheva A. A., Sharapov S. Z., Klarić L., Pakhomov E. D., et al. (2020). Prioritization of causal genes for coronary artery disease based on cumulative evidence from experimental and in silico studies. Sci. Rep. 10, 10486. doi:10.1038/S41598-020-67001-W
Shen C., Guo Z., Wu M., Hu X., Yang G., Yu R., et al. (2007). Association study between hypertension and A/G polymorphism at codon 637 of the transporter associated with antigen processing 1 gene. Hypertens. Res. 30, 683–690. doi:10.1291/hypres.30.683
Siasos G., Tsigkou V., Kosmopoulos M., Theodosiadis D., Simantiris S., Tagkou N. M., et al. (2018). Mitochondria and cardiovascular diseases-from pathophysiology to treatment. Ann. Transl. Med. 6, 256. doi:10.21037/atm.2018.06.21
Silva S., Nitsch D., Fatumo S. (2023). Genome-wide association studies on coronary artery disease: a systematic review and implications for populations of different ancestries. PLoS One 18, e0294341. doi:10.1371/JOURNAL.PONE.0294341
Singh L. N., Ennis B., Loneragan B., Tsao N. L., Lopez Sanchez M. I. G., Li J., et al. (2021). MitoScape: a big-data, machine-learning platform for obtaining mitochondrial DNA from next-generation sequencing data. PLoS Comput. Biol. 17, e1009594. doi:10.1371/journal.pcbi.1009594
Sinnott-Armstrong N., Tanigawa Y., Amar D., Mars N., Benner C., Aguirre M., et al. (2021). Genetics of 35 blood and urine biomarkers in the UK Biobank. Nat. Genet. 53, 185–194. doi:10.1038/s41588-020-00757-z
Skelly R. (2020). Next generation sequencing and genome-wide association studies to identify mitochondrial genomic features associated with diabetic kidney disease.
Skol A. D., Scott L. J., Abecasis G. R., Boehnke M. (2006). Joint analysis is more efficient than replication-based analysis for two-stage genome-wide association studies. Nat. Genet. 38, 209–213. doi:10.1038/ng1706
Smith A. C., Robinson A. J. (2016). MitoMiner v3.1, an update on the mitochondrial proteomics database. Nucleic Acids Res. 44, D1258–D1261. doi:10.1093/nar/gkv1001
Sung Y. J., Winkler T. W., de las Fuentes L., Bentley A. R., Brown M. R., Kraja A. T., et al. (2018). A large-scale multi-ancestry genome-wide study accounting for smoking behavior identifies multiple significant loci for blood pressure. Am. J. Hum. Genet. 102, 375–400. doi:10.1016/J.AJHG.2018.01.015
Svishcheva G. R., Belonogova N. M., Zorkoltseva I. V., Kirichenko A. V., Axenovich T. I. (2019). Gene-based association tests using GWAS summary statistics. Bioinformatics 35, 3701–3708. doi:10.1093/BIOINFORMATICS/BTZ172
Taanman J.-W. (1999). The mitochondrial genome: structure, transcription, translation and replication. Biochimica Biophysica Acta (BBA) - Bioenergetics 1410, 103–123. doi:10.1016/S0005-2728(98)00161-3
Talmud P. J., Drenos F., Shah S., Shah T., Palmen J., Verzilli C., et al. (2009). Gene-centric association signals for lipids and apolipoproteins identified via the HumanCVD BeadChip. Am. J. Hum. Genet. 85, 628–642. doi:10.1016/j.ajhg.2009.10.014
Taylor S. W., Fahy E., Zhang B., Glenn G. M., Warnock D. E., Wiley S., et al. (2003). Characterization of the human heart mitochondrial proteome. Nat. Biotechnol. 21, 281–286. doi:10.1038/nbt793
Temprano-Sagrera G., Sitlani C. M., Bone W. P., Martin-Bornez M., Voight B. F., Morrison A. C., et al. (2022). Multi-phenotype analyses of hemostatic traits with cardiovascular events reveal novel genetic associations. J. Thromb. Haemost. 20, 1331–1349. doi:10.1111/JTH.15698
The UniProt Consortium (2017). UniProt: the universal protein knowledgebase. Nucleic Acids Res. 45, D158–D169. doi:10.1093/nar/gkw1099
Timmis J. N., Ayliffe M. A., Huang C. Y., Martin W. (2004). Endosymbiotic gene transfer: organelle genomes forge eukaryotic chromosomes. Nat. Rev. Genet. 5, 123–135. doi:10.1038/nrg1271
Tipping R. W., Ford C. E., Simpson L. M., Walldius G., Jungner I., Folsom A. R., et al. (2009). Lipoprotein(a) concentration and the risk of coronary heart disease, stroke, and nonvascular mortality. JAMA J. Am. Med. Assoc. 302, 412–423. doi:10.1001/JAMA.2009.1063
Torres G. G., Dose J., Hasenbein T. P., Nygaard M., Krause-Kyora B., Mengel-From J., et al. (2022). Long-lived individuals show a lower burden of variants predisposing to age-related diseases and a higher polygenic longevity score. Int. J. Mol. Sci. 23, 10949. doi:10.3390/ijms231810949
Tsai M.-H., Kuo C.-W., Lin T.-K., Ho C.-J., Wang P.-W., Chuang J.-H., et al. (2020). Ischemic stroke risk associated with mitochondrial haplogroup F in the asian population. Cells 9, 1885. doi:10.3390/cells9081885
Tsao C. W., Aday A. W., Almarzooq Z. I., Anderson C. A. M., Arora P., Avery C. L., et al. (2023). Heart disease and stroke statistics-2023 update: a report from the American heart association. Circulation 147, e93–e621. doi:10.1161/CIR.0000000000001123
Van Der Harst P., Verweij N. (2018). Identification of 64 novel genetic loci provides an expanded view on the genetic architecture of coronary artery disease. Circ. Res. 122, 433–443. doi:10.1161/CIRCRESAHA.117.312086
van Oven M. (2015). PhyloTree Build 17: growing the human mitochondrial DNA tree. Forensic Sci. Int. Genet. Suppl. Ser. 5, e392–e394. doi:10.1016/j.fsigss.2015.09.155
Vázquez P., Hernández-Sánchez C., Escalona-Garrido C., Pereira L., Contreras C., López M., et al. (2018). Increased FGF21 in brown adipose tissue of tyrosine hydroxylase heterozygous mice: implications for cold adaptation. J. Lipid Res. 59, 2308–2320. doi:10.1194/jlr.M085209
Venter M., Malan L., van Dyk E., Elson J. L., van der Westhuizen F. H. (2017). Using MutPred derived mtDNA load scores to evaluate mtDNA variation in hypertension and diabetes in a two-population cohort: the SABPA study. J. Genet. Genomics 44, 139–149. doi:10.1016/j.jgg.2016.12.003
Venter M., Van Der Westhuizen F. H., Elson J. L. (2018). The aetiology of cardiovascular disease: a role formitochondrial DNA? Cardiovasc J. Afr. 29, 122–132. doi:10.5830/CVJA-2017-037
Veronese N., Stubbs B., Koyanagi A., Vaona A., Demurtas J., Schofield P., et al. (2019). Mitochondrial genetic haplogroups and cardiovascular diseases: data from the Osteoarthritis Initiative. PLoS One 14, e0213656. doi:10.1371/journal.pone.0213656
Visseren F. L. J., Mach F., Smulders Y. M., Carballo D., Koskinas K. C., Bäck M., et al. (2021). 2021 ESC Guidelines on cardiovascular disease prevention in clinical practice. Eur. Heart J. 42, 3227–3337. doi:10.1093/eurheartj/ehab484
Wain H. M., Bruford E. A., Lovering R. C., Lush M. J., Wright M. W., Povey S. (2002). Guidelines for human gene nomenclature. Genomics 79, 464–470. doi:10.1006/geno.2002.6748
Wain L. V., Vaez A., Jansen R., Joehanes R., Van Der Most P. J., Erzurumluoglu A. M., et al. (2017). Novel blood pressure locus and gene discovery using genome-wide association study and expression data sets from blood and the kidney. Hypertension 70, e4–e19. doi:10.1161/HYPERTENSIONAHA.117.09438
Wang Z., Chen H., Qin M., Liu C., Ma Q., Chen X., et al. (2021). Associations of mitochondrial variants with lipidomic traits in a Chinese cohort with coronary artery disease. Front. Genet. 12, 630359. doi:10.3389/fgene.2021.630359
Warrens H., Banerjee D., Herzog C. A. (2022). Cardiovascular complications of chronic kidney disease: an introduction. Eur. Cardiol. Rev. 17, e13. doi:10.15420/ECR.2021.54
Watanabe K., Taskesen E., van Bochoven A., Posthuma D. (2017). Functional mapping and annotation of genetic associations with FUMA. Nat. Commun. 8, 1826. doi:10.1038/s41467-017-01261-5
Watanabe K., Umićević Mirkov M., de Leeuw C. A., van den Heuvel M. P., Posthuma D. (2019). Genetic mapping of cell type specificity for complex traits. Nat. Commun. 10, 3222. doi:10.1038/s41467-019-11181-1
Watkins H., Farrall M. (2006). Genetic susceptibility to coronary artery disease: from promise to progress. Nat. Rev. Genet. 7, 163–173. doi:10.1038/nrg1805
Wb K., Dl M. (1979). Diabetes and cardiovascular disease. The Framingham study. JAMA 241, 2035–2038. doi:10.1001/JAMA.241.19.2035
Weissensteiner H., Pacher D., Kloss-Brandstätter A., Forer L., Specht G., Bandelt H.-J., et al. (2016). HaploGrep 2: mitochondrial haplogroup classification in the era of high-throughput sequencing. Nucleic Acids Res. 44, W58–W63. doi:10.1093/nar/gkw233
Whelton P. K., Carey R. M., Aronow W. S., Casey D. E., Collins K. J., Dennison Himmelfarb C., et al. (2018). 2017 ACC/AHA/AAPA/ABC/ACPM/AGS/APhA/ASH/ASPC/NMA/PCNA guideline for the prevention, detection, evaluation, and management of high blood pressure in adults: a report of the American college of cardiology/American heart association task force on clinical practice guidelines. J. Am. Coll. Cardiol. 71, e127–e248. doi:10.1016/j.jacc.2017.11.006
WHO (2023). The top 10 causes of death. Available at: https://www.who.int/news-room/fact-sheets/detail/the-top-10-causes-of-death (Accessed December 28, 2023).
Wojcik G. L., Graff M., Nishimura K. K., Tao R., Haessler J., Gignoux C. R., et al. (2019). Genetic analyses of diverse populations improves discovery for complex traits. Nature 570, 514–518. doi:10.1038/S41586-019-1310-4
Wuttke M., Wong C. S., Wuhl E., Epting D., Luo L., Hoppmann A., et al. (2016). Genetic loci associated with renal function measures and chronic kidney disease in children: the Pediatric Investigation for Genetic Factors Linked with Renal Progression Consortium. Nephrol. Dial. Transpl. 31, 262–269. doi:10.1093/ndt/gfv342
Xue A., Wu Y., Zhu Z., Zhang F., Kemper K. E., Zheng Z., et al. (2018). Genome-wide association analyses identify 143 risk variants and putative regulatory mechanisms for type 2 diabetes. Nat. Commun. 9, 2941. doi:10.1038/S41467-018-04951-W
Yan Q., Nho K., Del-Aguila J. L., Wang X., Risacher S. L., Fan K.-H., et al. (2021). Genome-wide association study of brain amyloid deposition as measured by Pittsburgh Compound-B (PiB)-PET imaging. Mol. Psychiatry 26, 309–321. doi:10.1038/s41380-018-0246-7
Yang J., Guo Q., Feng X., Liu Y., Zhou Y. (2022). Mitochondrial dysfunction in cardiovascular diseases: potential targets for treatment. Front. Cell Dev. Biol. 10, 841523. doi:10.3389/fcell.2022.841523
Yang R., Xu H., Pedersen N. L., Li X., Yu J., Bao C., et al. (2021). A healthy lifestyle mitigates the risk of heart disease related to type 2 diabetes: a prospective nested case–control study in a nationwide Swedish twin cohort. Diabetologia 64, 530–539. doi:10.1007/s00125-020-05324-z
Yeo A., Li L., Warren L., Aponte J., Fraser D., King K., et al. (2017). Pharmacogenetic meta-analysis of baseline risk factors, pharmacodynamic, efficacy and tolerability endpoints from two large global cardiovascular outcomes trials for darapladib. PLoS One 12, e0182115. doi:10.1371/JOURNAL.PONE.0182115
Yonova-Doing E., Calabrese C., Gomez-Duran A., Schon K., Wei W., Karthikeyan S., et al. (2021). An atlas of mitochondrial DNA genotype–phenotype associations in the UK Biobank. Nat. Genet. 53 (7), 982–993. doi:10.1038/S41588-021-00868-1
Zerbino D. R., Achuthan P., Akanni W., Amode M. R., Barrell D., Bhai J., et al. (2018). Ensembl 2018. Nucleic Acids Res. 46, D754-D761–D761. doi:10.1093/nar/gkx1098
Zhang S., Jiang Z., Zhang H., Liu Y., Qi J., Yan Y., et al. (2024). Association of cigarette smoking, smoking cessation with the risk of cardiometabolic multimorbidity in the UK Biobank. BMC Public Health 24, 1910. doi:10.1186/S12889-024-19457-Y
Zhang Y., Tsai T.-H., Ezrokhi M., Stoelzel C., Cincotta A. H. (2023). Tyrosine hydroxylase knockdown at the hypothalamic supramammillary nucleus area induces obesity and glucose intolerance. Neuroendocrinology 114, 483–510. doi:10.1159/000535944
Zhao W., Rasheed A., Tikkanen E., Lee J. J., Butterworth A. S., Howson J. M. M., et al. (2017). Identification of new susceptibility loci for type 2 diabetes and shared etiological pathways with coronary heart disease. Nat. Genet. 49, 1450–1457. doi:10.1038/NG.3943
Zhelankin A. V., Sazonova M. A., Khasanova Z. B., Sinyov V. V., Mitrofanov K. Y., Sobenin I. A., et al. (2015). Analysis of mitochondrial haplogroups in persons with subclinical atherosclerosis based on high-throughput mtDNA sequencing. Patol. Fiziol. Eksp. Ter. 59, 12–16.
Zhou W., Nielsen J. B., Fritsche L. G., Dey R., Gabrielsen M. E., Wolford B. N., et al. (2018). Efficiently controlling for case-control imbalance and sample relatedness in large-scale genetic association studies. Nat. Genet. 50 (9 50), 1335–1341. doi:10.1038/s41588-018-0184-y
Zhu Y., Gu X., Xu C. (2016). A mitochondrial DNA A8701G mutation associated with maternally inherited hypertension and dilated cardiomyopathy in a Chinese pedigree of a consanguineous marriage. Chin. Med. J. Engl. 129, 259–266. doi:10.4103/0366-6999.174491
Zhu Y., Gu X., Xu C. (2018). Mitochondrial DNA 7908–8816 region mutations in maternally inherited essential hypertensive subjects in China. BMC Med. Genomics 11, 89. doi:10.1186/S12920-018-0408-0
Keywords: cardiovascular disease, coronary artery disease, mitochondrial DNA, blood pressure, hypertension, diabetes, UK Biobank, mitochondrial haplogroups
Citation: Cañadas-Garre M, Maqueda JJ, Baños-Jaime B, Hill C, Skelly R, Cappa R, Brennan E, Doyle R, Godson C, Maxwell AP and McKnight AJ (2024) Mitochondrial related variants associated with cardiovascular traits. Front. Physiol. 15:1395371. doi: 10.3389/fphys.2024.1395371
Received: 03 March 2024; Accepted: 05 August 2024;
Published: 27 August 2024.
Edited by:
Harpreet Singh, The Ohio State University, United StatesReviewed by:
Devasena Ponnalagu, University of Washington, United StatesCopyright © 2024 Cañadas-Garre, Maqueda, Baños-Jaime, Hill, Skelly, Cappa, Brennan, Doyle, Godson, Maxwell and McKnight. This is an open-access article distributed under the terms of the Creative Commons Attribution License (CC BY). The use, distribution or reproduction in other forums is permitted, provided the original author(s) and the copyright owner(s) are credited and that the original publication in this journal is cited, in accordance with accepted academic practice. No use, distribution or reproduction is permitted which does not comply with these terms.
*Correspondence: Marisa Cañadas-Garre, bWFyaXNhY2dhcnJlQGdtYWlsLmNvbQ==
†These authors have contributed equally to this work
Disclaimer: All claims expressed in this article are solely those of the authors and do not necessarily represent those of their affiliated organizations, or those of the publisher, the editors and the reviewers. Any product that may be evaluated in this article or claim that may be made by its manufacturer is not guaranteed or endorsed by the publisher.
Research integrity at Frontiers
Learn more about the work of our research integrity team to safeguard the quality of each article we publish.