- 1Department of Emergency, Affiliated Dongyang Hospital of Wenzhou Medical University, Dongyang, Zhejiang Province, China
- 2Department of Biomedical Sciences Laboratory, Affiliated Dongyang Hospital of Wenzhou Medical University, Dongyang, China
Objective: The objective of this study is to identify patients with sepsis who are at a high risk of respiratory failure.
Methods: Data of 1,738 patients with sepsis admitted to Dongyang People’s Hospital from June 2013 to May 2023 were collected, including the age at admission, blood indicators, and physiological indicators. Independent risk factors for respiratory failure during hospitalization in the modeling population were analyzed to establish a nomogram. The area under the receiver operating characteristic curve (AUC) was used to evaluate the discriminative ability, the GiViTI calibration graph was used to evaluate the calibration, and the decline curve analysis (DCA) curve was used to evaluate and predict the clinical validity. The model was compared with the Sequential Organ Failure Assessment (SOFA) score, the National Early Warning Score (NEWS) system, and the ensemble model using the validation population.
Results: Ten independent risk factors for respiratory failure in patients with sepsis were included in the final logistic model. The AUC values of the prediction model in the modeling population and validation population were 0.792 and 0.807, respectively, both with good fit between the predicted possibility and the observed event. The DCA curves were far away from the two extreme curves, indicating good clinical benefits. Based on the AUC values in the validation population, this model showed higher discrimination power than the SOFA score (AUC: 0.682; p < 0.001) and NEWS (AUC: 0.520; p < 0.001), and it was comparable to the ensemble model (AUC: 0.758; p = 0.180).
Conclusion: Our model had good performance in predicting the risk of respiratory failure in patients with sepsis within 48 h following admission.
Introduction
Background
Sepsis is the result of an invasion of pathogens into the body, leading to immune disorders and organ dysfunction (Lelubre and Vincent, 2018; Rubio et al., 2019). Due to its high mortality and disability rates, sepsis has become a global concern (Singer et al., 2016; Rhodes et al., 2017). A meta-analysis of 27 studies from seven developed countries showed that the incidence of hospital-treated sepsis was 437/100,000, and the mortality rate was 17% (Fleischmann et al., 2016). In organ dysfunction caused by sepsis, the lung is a commonly affected organ, which can easily lead to respiratory failure (Erickson et al., 2009; Cochi et al., 2016; Singer et al., 2016; Fowler et al., 2019).
Respiratory failure is a decrease in PaO2 caused by various reasons. In the United States, the incidence rate of respiratory failure is 1,275/100,000, and the hospital mortality rate of patients with respiratory failure is 12% (Kempker et al., 2020). It is one of the common critical diseases, and the surviving patients may have physical, psychological, and cognitive problems (Brummel et al., 2015; Hashem et al., 2016). It is considered a burden on public health and the healthcare system (Bice et al., 2013). Studies have shown that the mortality rate of sepsis patients with respiratory failure is significantly increased (Angus et al., 2001; Bellani et al., 2016; Cochi et al., 2016; Fowler et al., 2019). Therefore, it is important to predict whether respiratory failure may occur during hospitalization in patients with sepsis so that patients with a high risk can be identified early and interventions can be undertaken to reduce the mortality rate.
Studies have shown that in patients with COVID-19, the SOFA scores (Vincent et al., 1996) and NEWS (Subbe et al., 2001) are useful for predicting respiratory failure (Lalueza et al., 2022). However, the scoring systems and models mentioned previously were not developed for predicting respiratory failure, and there are doubts about their efficacy in predicting the risk of respiratory failure in patients with sepsis. Some scholars have developed a model for predicting the risk of respiratory failure in patients with pancreatitis (Qiu et al., 2022). However, as the model is not aimed at patients with sepsis, there are doubts about its efficacy in predicting the risk of respiratory failure in patients with sepsis. Several models could predict acute respiratory distress syndrome (ARDS) in sepsis patients, but they are established based on the patients from the intensive care unit, indicating limited practice with those in the ordinary ward (Bai et al., 2022; Xu et al., 2023). In addition, respiratory failure is a progressed and deteriorated status of ARDS; thus, the prediction model might not be shared by them.
The aim of our study was to develop a useful predictive model for predicting the risk of respiratory failure within 48 h following admission in sepsis patients to help clinicians screen high-risk patients for timely intervention and communication.
Methods and materials
Research participants
In this retrospective study, a total of 1,738 sepsis patients admitted to Dongyang People’s Hospital from June 2013 to April 2023 were included. The inclusion criteria were meeting the diagnostic criteria of sepsis 3.0; infection caused the SOFA score to increase by 2 points on the original basis. The exclusion criteria were as follows: 1) patients younger than 18 years old, 2) patients with respiratory failure at admission (patients with SpO2 less than 90% at admission), 3) and patients who discontinued treatment. Respiratory failure within 48 h following admission was based on the lowest PaO2/FiO2 values of less than 300 mmHg.
Observation indicators
We collected some basic information of the patients, such as gender, age, and past medical history, of the following: hypertension, diabetes, cerebral infarction, chronic lung disease (including chronic obstructive pulmonary disease, interstitial pneumonia, and chronic pulmonary fibrosis), chronic heart disease (including cardiac insufficiency NYHA II grade and above and old myocardial infarction), chronic liver disease (cirrhosis), chronic kidney disease (chronic renal insufficiency and nephrotic syndrome), tumor, leukemia, and the involved infection sites, including the central nerve system, lung, bile duct, urinary duct, and peritonitis. We also collected the following first indicators at admission: hypersensitive C-reactive protein, procalcitonin, alanine transaminase, aspartate transaminase, triglyceride, total bilirubin, creatinine, blood urea nitrogen, lactic acid, pro-brain natriuretic peptide (Pro-BNP), cholinesterase, prothrombin time, D-dimer, activated partial thromboplastin time, potassium, sodium, magnesium, calcium, phosphorus, hemoglobin, white blood cell count, platelet count, albumin, globulin, mean arterial pressure, pulse oxygen saturation, body temperature, respiratory rate, heart rate, Glasgow Coma Score (GCS), SOFA score, and NEWS (i.e., a total of 46 indicators, two scoring systems).
Method description
First, the data were analyzed using R software (version 4.2.2). Continuous variables with a normal distribution were expressed as the mean plus or minus standard deviation and were tested using the t-test. Variables with a non-normal distribution were expressed as the median and interquartile range, and were tested using the Mann–Whitney U test. Categorical variables were expressed as a percentage and were tested using the chi-squared test.
The highest missing ratio among all variables was that of lactic acid (20.3%), and the missing ratios of the other variables were all less than 20%. We set up seeds and used the mice package to perform multiple imputations. In order to facilitate clinical interpretation and to ensure that there was a linear relationship between each variable and logitp when multivariate logistic regression analysis was conducted, some continuous variables were converted to categorical variables. The data were randomly divided into the modeling population and the validation population at the ratio of 7:3. Univariate analysis was performed on the modeling population; multicollinear analysis was performed on the significant indicators in the univariate analysis, which were evaluated using variance inflation coefficients (VIFs). VIFs of less than 10 indicated that there was no notable collinearity among the risk factors (Hsieh and Lavori, 2000). Whether there was a linear relationship between the variables and logitp was tested by the Box-Tidwell function, with a p-value < 0.05 indicating the absence of a linear relationship. After confirming that there was no multicollinearity among the variables and that there was a linear relationship with logitp, multivariate logistic regression analysis was performed, and then, stepwise regression analysis was performed to screen out the independent risk factors and establish a nomogram.
Evaluation of the established prediction model
The area under the receiver operation curve (AUC) was used to assess the discrimination ability of the predictive model. An AUC > 0.75 indicated that the model was good (Silva et al., 2015); a calibration graph was applied to evaluate the agreement between the predicted probability and the actual observed probability. When a p-value tested by Hosmer–Lemeshow was greater than 0.05, the model showed adequate fitness (Niu et al., 2017). Finally, the net clinical benefit of using the established model could be shown as the distance from the all curve to the none curve (the two extreme curves) in decision curve analysis. In another way, the more the distance of the model curve from the two extreme curves, the better the clinical significance and net benefit conferred to the clinic (Nattino et al., 2016); they were used to judge the discrimination, calibration, and clinical validity, respectively, of our model, and these were also verified in the validation population.
In addition, several other models were constructed using the NEWS and the SOFA score in the validation populations, with AUCs being compared using the DeLong test. When the significance in the DeLong test was less than 0.05, a significant difference was observed between the compared AUCs. An ensemble model based on the enrolled 46 variables was established using the ensemble methods, including support vector machines (SVMs), decision trees C5.0 (C5.0), extreme gradient boosting (XGBoost), and the decision by the following R packages: CARET, XGBoost, C50, e1071, and gbm (Jiang et al., 2022). The importance of involved variables in the ensemble method was evaluated using SHAP values.
Results
Comparison of the basic information between the modeling population and the validation population
A total of 1,915 patients with sepsis were included, including 154 patients with respiratory failure at admission, 13 patients who discontinued treatment, and 10 patients younger than 18 years (Figure 1). Among the study subjects, there were 345 patients with respiratory failure during hospitalization, accounting for 19.9% of the patients. The number of invasive ventilator users was 115, accounting for a percentage of 6.6%. There were 28% cases with lung infection, 10% with bile duct infection, 17% with urinary duct infection, and 45% with infection in other anatomic sites or unknown sites. A total of 1,217 patients were in the modeling population, and 521 patients were in the validation population. There was no significant difference in any variable between the modeling population and the validation population (p > 0.05; Table 1).
Variable screening and logistic model construction
Univariate analysis of the modeling population showed that procalcitonin, aspartate transaminase, triglyceride, blood urea nitrogen, lactic acid, Pro-BNP, cholinesterase, prothrombin time, D-dimer, phosphorus, albumin, globulin, pulse oxygen saturation, respiratory rate, GCS, lung infection, and peritonitis were associated with respiratory failure (p < 0.001; Table 2). The results showed that the included variables had no collinearity in predicting respiratory failure (VIFs < 10; Supplementary Table S1), and there was a linear relationship with logitp (p > 0.05; Supplementary Table S2), suggesting that they could be used to construct a logistic regression model. Multivariate logistic regression analysis and stepwise regression showed that the respiratory rate, lactic acid, Pro-BNP, D-dimer, albumin, globulin, pulse oxygen saturation, GCS, lung infection, and peritonitis were independent factors related to respiratory failure (Table 3).
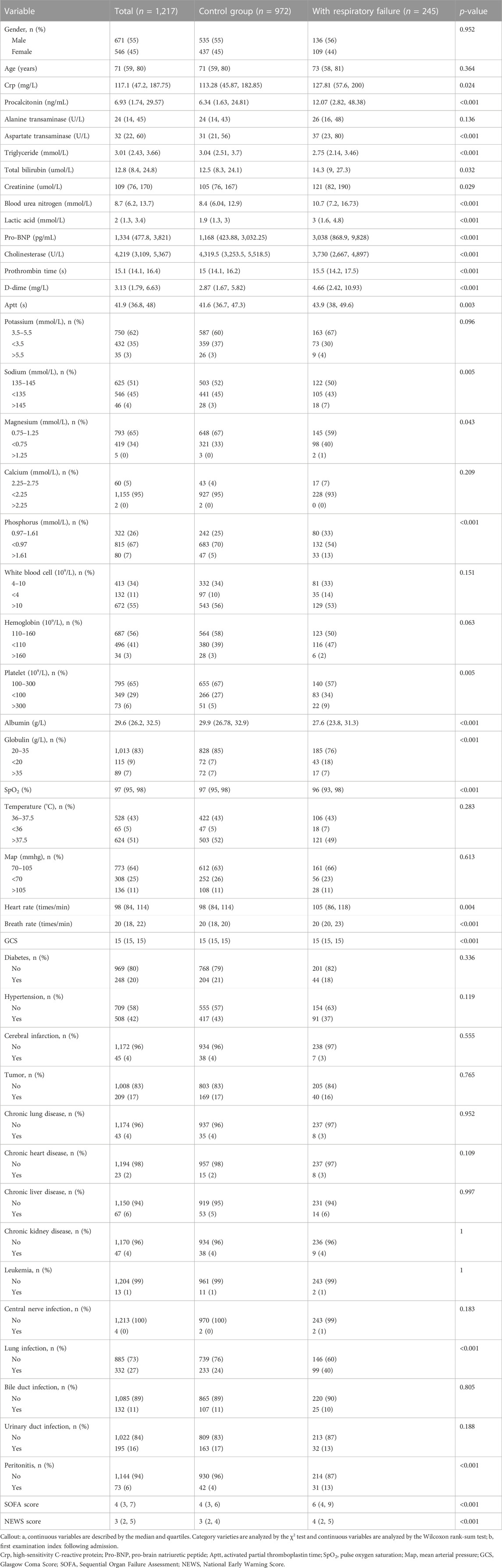
TABLE 2. Univariate analysis between respiratory failure and no respiratory failure in the modeling populationa.
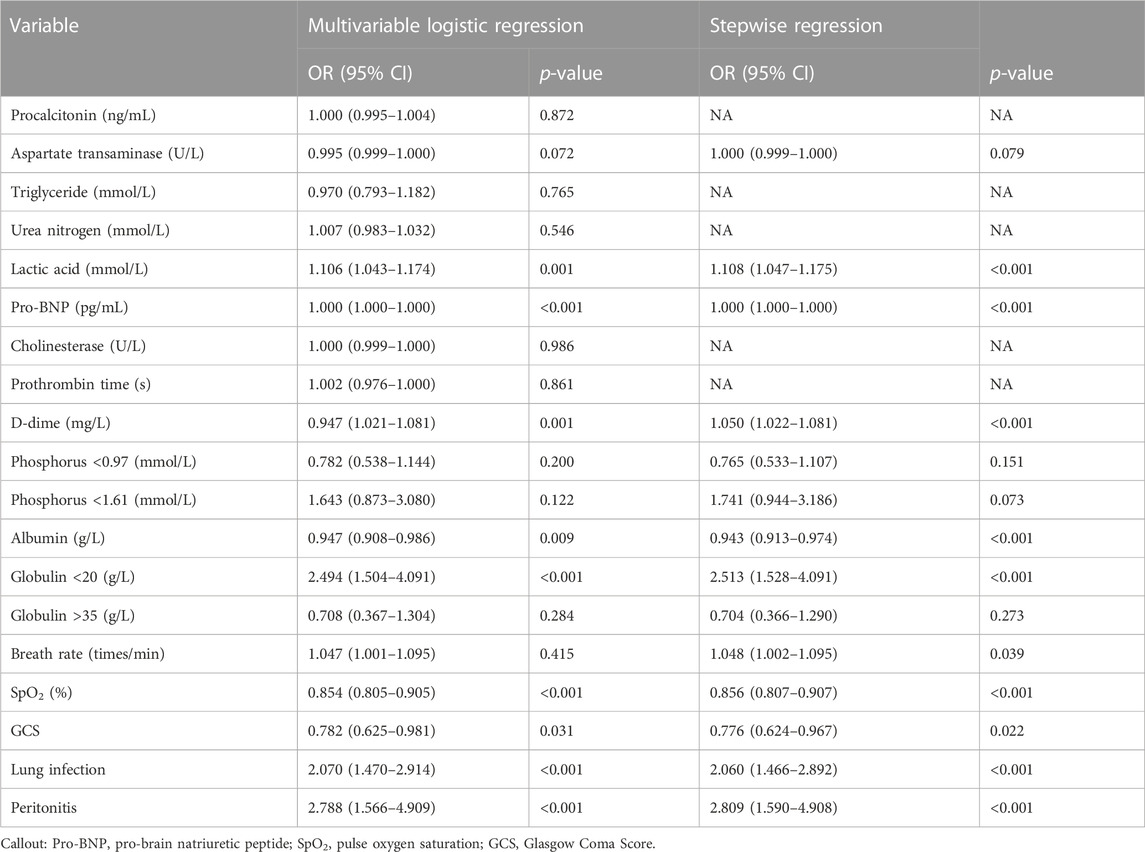
TABLE 3. Multivariable logistic regression analysis and stepwise regression analysis of involved variables in the modeling group.
Establishment of a nomogram in the modeling population
A nomogram was established using 10 variables (Figure 2). Each variable of the patient could be matched with the point scoring line according to the specific values to obtain the respective scores. Finally, the scores of each variable were summed to obtain the total score, and then, the corresponding risk could be obtained with the risk scale at the bottom.
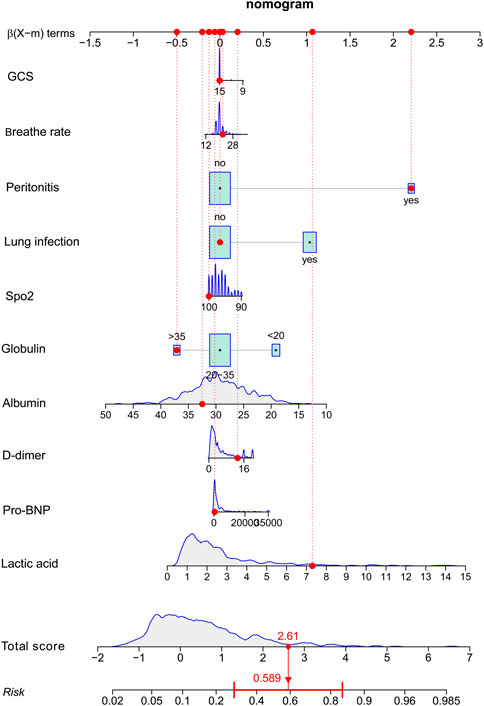
FIGURE 2. Risk-prediction nomogram for respiratory failure within 48 h following admission in patients with sepsis.
Model evaluation of the discrimination power, goodness of fit, and clinical benefit
The AUC value of the model in the modeling population was 0.792, and the 95% CI was 0.761–0.823, suggesting good discrimination (Figure 3A). The p-value of the calibration graph was 0.754, indicating a good fit (Figure 3B). In the DCA curve diagram, the curve of the model was far away from the two extreme curves, showing that the model had a good clinical benefit value (Figure 3C).
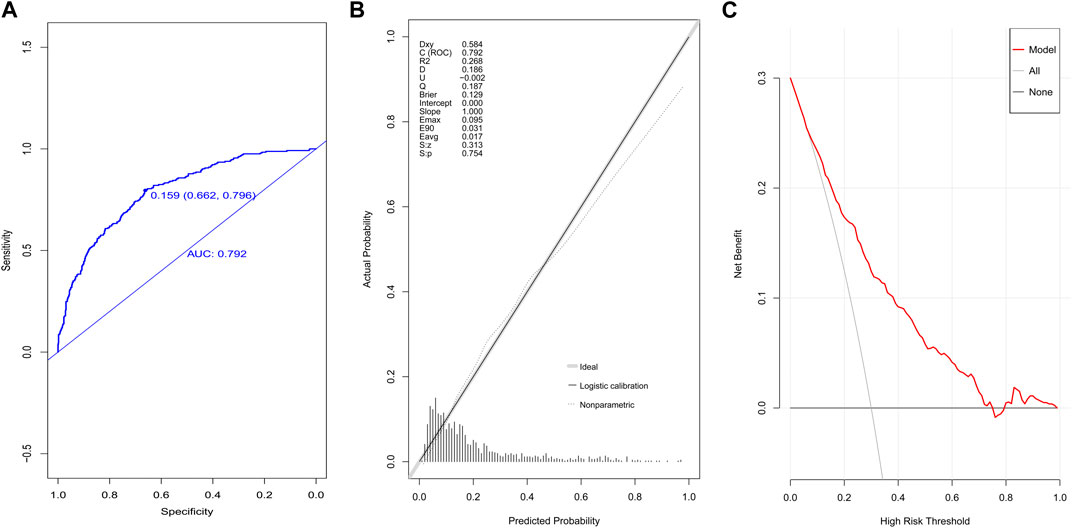
FIGURE 3. Evaluation of the prediction model in the modeling group. (A) ROC curves, (B) calibration chart, and (C) DCA curves.
The AUC value in the validation population was 0.807, the 95% CI was 0.759–0.856 (Figure 4A), and the p-value of the calibration graph was 0.796, suggesting a good fit (Figure 4B). In addition, the DCA curve was far away from the two extreme curves (Figure 4C).
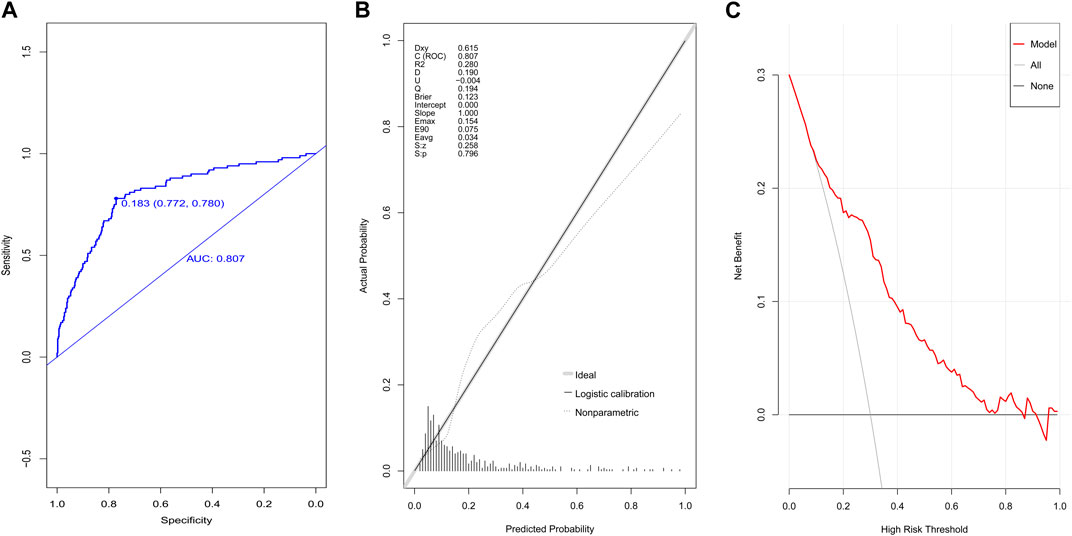
FIGURE 4. Evaluation of the prediction model in the validation group. (A) ROC curves, (B) calibration chart, and (C) DCA curves.
Comparison with the models established by the SOFA score, NEWS, and ensemble method
In the validation population, the AUC value of the model established by the NEWS was 0.520 (95% CI: 0.457–0.583). The AUC value of the model established by the SOFA score was 0.682 (95% CI: 0.621–0.742; Figure 5A). Our model had better discrimination than the NEWS model (p < 0.001) and the SOFA score model (p < 0.001). The ensemble model in our study had an AUC value of 0.758 in the validation population, with a 95% CI of 0.704–0.813 (Figure 5B), which was comparable to the established logistic prediction model (p = 0.180). The calibration curve indicated that the ensemble model was poorly calibrated (Supplementary Figure S1). Pro-BNP, lactic acid, SpO2, albumin, and creatinine were the top five variables among the 29 important variables in the ensemble model, as indicated by SHAP values (Supplementary Figure S2).
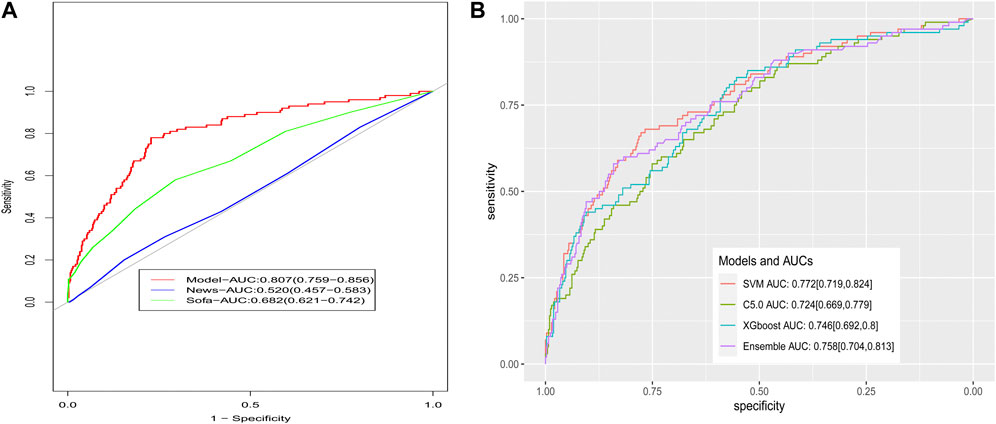
FIGURE 5. Comparison of ROCs for models. (A) Comparison to the models based on the SOFA and NEWS scoring system and (B) comparison to the models based on the ensemble method.
Discussion
In this retrospective study, 10 variables were identified as independent risk factors for respiratory failure after hospital admission in septic patients, namely, respiratory rate, lactic acid, Pro-BNP, D-dimer, albumin, globulin, pulse oxygen saturation, GCS, lung infection, and peritonitis. After evaluating the discrimination, calibration, and clinical validity, our model has a good potential clinical value and interpretability. Because the prediction model incorporates 10 indicators that can be obtained as soon as patients are admitted to the hospital, it could be a helpful tool in screening for sepsis patients with a high risk of respiratory failure within 48 h following admission.
In our model, D-dimer acted as a biomarker of abnormal hemostasis and an indicator of intravascular thrombus formation (Johnson et al., 2019). The elevated D-dimer level indicates the presence of thrombus in the microvessels or large blood vessels of the lung, which can lead to imbalance of the ventilatory blood flow. Recent studies have pointed out that in patients with COVID-19, high levels of D-dimer are significantly related to respiratory failure (Takeshita et al., 2021). Some studies have pointed out that the albumin level at admission can predict the risk of respiratory failure (Thongprayoon et al., 2020), which may be related to the fact that low albumin indicates malnutrition, which leads to the atrophy of the diaphragm and respiratory muscles and affects the respiratory function. Low albumin also leads to a decrease in the plasma osmotic pressure, which is more likely to cause pulmonary edema (Blunt et al., 1998). Globulin is composed of immunoglobulin and complements, playing a key role in the antivirus and anti-bacterium response. In this study, a lower globulin level was associated with increased respiratory failure risk, which could be indirectly explained by the fact that globulin is associated with sepsis severity and lung injury would more likely occur in patients with severe sepsis (Nenke et al., 2015; Horvath-Szalai et al., 2018). Patients with a low GCS have reduced airway protection ability, which can easily lead to sputum blockage and aspiration. In some studies, GCSs have also been found to be associated with respiratory failure (Lu et al., 2022). Inflammation in the lungs would cause dysfunction in mucosal immunity, macrophages, and monocytes, which might consequently increase the susceptivity to secondary infections and respiratory distress (Bouras et al., 2018; Roquilly et al., 2020). SpO2 is a sensitive indicator representing whether the patient is hypoxic, and the increase in the respiratory rate is often due to the compensation caused by hypoxia. In patients with sepsis, lung damage is prone to occur (Tasaka et al., 2008), and the indicators can be reflected in the decrease of SpO2 and the increase of the respiratory frequency at admission; thus, these patients may be more prone to respiratory failure. Lactic acid is an indicator of the oxygen metabolism and is used to gauge the prognosis in sepsis and other critical diseases (Liu et al., 2015; Houwink et al., 2016; Luo and He, 2023), and it could serve as a predictive factor for respiratory failure in this study and ARDS in previous data (Mikkelsen et al., 2013). Pro-BNP, as an indicator of cardiac function, could also be an indicator for other disease deterioration, and its elevated levels would be closely related to respiratory failure (Takasu et al., 2013; Cheng et al., 2015). A high respiratory rate at admission indicated the presence of hypoxia and the need for compensation, which would cause fatigue in the respiratory muscle. Moreover, the presence of peritonitis was the risk factor for respiratory failure, which might be caused by surgical operation for the diagnosis and therapy of peritonitis.
The NEWS is helpful in identifying patients with early critical illnesses (Subbe et al., 2001), and the SOFA score is clinically used to predict the severity of sepsis patients (Vincent et al., 1996). Although these two scoring systems were not developed for the prediction of respiratory failure, previous studies have shown that in some patients, the aforementioned two scoring systems are helpful in predicting the risk of respiratory failure (Lalueza et al., 2022). According to the results of the current study, the AUC value of our model was significantly higher than those of the models using the aforementioned two scoring systems, suggesting higher discrimination of our model. The increased prediction power of our model could be explained by the inclusion of the variables related to the worsening of the gas exchange function (such as respiratory rate and lactic acid); however, some of them are expensive and not routinely tested in clinical practice. Our findings raise the significance of novel variables in predicting the respiratory failure risk.
We also built an ensemble model, which was comparable to our logistic model on discrimination, but lower fitness was found for the ensemble model. Moreover, the decision support provided by models based on machine learning algorithms is often difficult to be explained clinically (Kelly et al., 2019) due to its complexity. Thus, we believe that our logistic model is more functional in prediction in terms of clinical interpretation than machine learning models, such as the ensemble model.
Our study has the following limitations: It is a single-center study without validation with external data, so it is unclear if it is also applicable in other regions. There were 10 variables enrolled in the final model; some of them might be not available for a source-limited region. Our study could serve as a reference, especially our flowchart on variable selection and model evaluation, for other research workers who would like to predict the possibility of respiratory failure.
Conclusion
Based on clinical indicators, we constructed a model for predicting the risk of respiratory failure within 48 h following admission in sepsis patients. This established model is effective, and it can help clinical staff in the decision-making process involved in the management of patients with sepsis.
Data availability statement
The raw data supporting the conclusion of this article will be made available by the authors, without undue reservation.
Ethics statement
This study was approved by the Ethics Committee of Dongyang People’s Hospital (approval number 2023-YX-154). Written informed consent was provided by the participants, or by their legal guardian/next of kin.
Author contributions
BW: writing–original draft. JC: data curation, investigation, and writing–review and editing. MW: writing–review and editing.
Funding
The author(s) declare that no financial support was received for the research, authorship, and/or publication of this article.
Acknowledgments
The authors are grateful for technical support provided by the LE9 Health platform and for the efforts and contributions of each author in this study.
Conflict of interest
The authors declare that the research was conducted in the absence of any commercial or financial relationships that could be construed as a potential conflict of interest.
Publisher’s note
All claims expressed in this article are solely those of the authors and do not necessarily represent those of their affiliated organizations, or those of the publisher, the editors, and the reviewers. Any product that may be evaluated in this article, or claim that may be made by its manufacturer, is not guaranteed or endorsed by the publisher.
Supplementary material
The Supplementary Material for this article can be found online at: https://www.frontiersin.org/articles/10.3389/fphys.2023.1288226/full#supplementary-material
References
Angus D. C., Linde-Zwirble W. T., Lidicker J., Clermont G., Carcillo J., Pinsky M. R. (2001). Epidemiology of severe sepsis in the United States: analysis of incidence, outcome, and associated costs of care. Crit. Care Med. 29, 1303–1310. doi:10.1097/00003246-200107000-00002
Bai Y., Xia J., Huang X., Chen S., Zhan Q. (2022). Using machine learning for the early prediction of sepsis-associated ARDS in the ICU and identification of clinical phenotypes with differential responses to treatment. Front. Physiol. 13, 1050849. doi:10.3389/fphys.2022.1050849
Bellani G., Laffey J. G., Pham T., Fan E., Brochard L., Esteban A., et al. (2016). Epidemiology, patterns of care, and mortality for patients with acute respiratory distress syndrome in intensive care units in 50 countries. JAMA 315, 788–800. doi:10.1001/jama.2016.0291
Bice T., Cox C. E., Carson S. S. (2013). Cost and health care utilization in ARDS--different from other critical illness? Semin. Respir. Crit. Care Med. 34, 529–536. doi:10.1055/s-0033-1351125
Blunt M. C., Nicholson J. P., Park G. R. (1998). Serum albumin and colloid osmotic pressure in survivors and nonsurvivors of prolonged critical illness. Anaesthesia 53, 755–761. doi:10.1046/j.1365-2044.1998.00488.x
Bouras M., Asehnoune K., Roquilly A. (2018). Contribution of dendritic cell responses to sepsis-induced immunosuppression and to susceptibility to secondary pneumonia. Front. Immunol. 9, 2590. doi:10.3389/fimmu.2018.02590
Brummel N. E., Balas M. C., Morandi A., Ferrante L. E., Gill T. M., Ely E. W. (2015). Understanding and reducing disability in older adults following critical illness. Crit. Care Med. 43, 1265–1275. doi:10.1097/CCM.0000000000000924
Cheng H., Fan W. Z., Wang S. C., Liu Z. H., Zang H. L., Wang L. Z., et al. (2015). N-terminal pro-brain natriuretic peptide and cardiac troponin I for the prognostic utility in elderly patients with severe sepsis or septic shock in intensive care unit: a retrospective study. J. Crit. Care 30, e9–e14. doi:10.1016/j.jcrc.2014.12.008
Cochi S. E., Kempker J. A., Annangi S., Kramer M. R., Martin G. S. (2016). Mortality trends of acute respiratory distress syndrome in the United States from 1999 to 2013. Ann. Am. Thorac. Soc. 13, 1742–1751. doi:10.1513/AnnalsATS.201512-841OC
Erickson S. E., Martin G. S., Davis J. L., Matthay M. A., Eisner M. D., Network N. N. A. (2009). Recent trends in acute lung injury mortality: 1996-2005. Crit. Care Med. 37, 1574–1579. doi:10.1097/CCM.0b013e31819fefdf
Fleischmann C., Scherag A., Adhikari N. K., Hartog C. S., Tsaganos T., Schlattmann P., et al. (2016). Assessment of global incidence and mortality of hospital-treated sepsis. Current estimates and limitations. Am. J. Respir. Crit. Care Med. 193, 259–272. doi:10.1164/rccm.201504-0781OC
Fowler A. A., Truwit J. D., Hite R. D., Morris P. E., DeWilde C., Priday A., et al. (2019). Effect of vitamin C infusion on organ failure and biomarkers of inflammation and vascular injury in patients with sepsis and severe acute respiratory failure: the CITRIS-ALI randomized clinical trial. JAMA 322, 1261–1270. doi:10.1001/jama.2019.11825
Hashem M. D., Nallagangula A., Nalamalapu S., Nunna K., Nausran U., Robinson K. A., et al. (2016). Patient outcomes after critical illness: a systematic review of qualitative studies following hospital discharge. Crit. Care 20, 345. doi:10.1186/s13054-016-1516-x
Horvath-Szalai Z., Kustan P., Szirmay B., Lakatos A., Christensen P. H., Huber T., et al. (2018). Predictive value of serum gelsolin and Gc globulin in sepsis - a pilot study. Clin. Chem. Lab. Med. 56, 1373–1382. doi:10.1515/cclm-2017-0782
Houwink A. P., Rijkenberg S., Bosman R. J., van der Voort P. H. (2016). The association between lactate, mean arterial pressure, central venous oxygen saturation and peripheral temperature and mortality in severe sepsis: a retrospective cohort analysis. Crit. Care 20, 56. doi:10.1186/s13054-016-1243-3
Hsieh F. Y., Lavori P. W. (2000). Sample-size calculations for the Cox proportional hazards regression model with nonbinary covariates. Control Clin. Trials 21, 552–560. doi:10.1016/s0197-2456(00)00104-5
Jiang X., Hu Y., Guo S., Du C., Cheng X. (2022). Prediction of persistent acute kidney injury in postoperative intensive care unit patients using integrated machine learning: a retrospective cohort study. Sci. Rep. 12, 17134. doi:10.1038/s41598-022-21428-5
Johnson E. D., Schell J. C., Rodgers G. M. (2019). The D-dimer assay. Am. J. Hematol. 94, 833–839. doi:10.1002/ajh.25482
Kelly C. J., Karthikesalingam A., Suleyman M., Corrado G., King D. (2019). Key challenges for delivering clinical impact with artificial intelligence. BMC Med. 17, 195. doi:10.1186/s12916-019-1426-2
Kempker J. A., Abril M. K., Chen Y., Kramer M. R., Waller L. A., Martin G. S. (2020). The epidemiology of respiratory failure in the United States 2002-2017: a serial cross-sectional study. Crit. Care Explor 2, e0128. doi:10.1097/CCE.0000000000000128
Lalueza A., Lora-Tamayo J., de la Calle C., Sayas-Catalan J., Arrieta E., Maestro G., et al. (2022). The early use of sepsis scores to predict respiratory failure and mortality in non-ICU patients with COVID-19. Rev. Clin. Esp. Barc. 222, 293–298. doi:10.1016/j.rce.2020.10.004
Lelubre C., Vincent J. L. (2018). Mechanisms and treatment of organ failure in sepsis. Nat. Rev. Nephrol. 14, 417–427. doi:10.1038/s41581-018-0005-7
Liu W., Peng L., Hua S. (2015). Clinical significance of dynamic monitoring of blood lactic acid, oxygenation index and C-reactive protein levels in patients with severe pneumonia. Exp. Ther. Med. 10, 1824–1828. doi:10.3892/etm.2015.2770
Lu H., Zhao Y., Xu L., Lin H. (2022). Risk factors for acute respiratory failure in individuals with trauma: a meta-analysis. Asian J. Surg. 45, 886–887. doi:10.1016/j.asjsur.2021.11.057
Luo M., He Q. (2023). Development of a prognostic nomogram for sepsis associated-acute respiratory failure patients on 30-day mortality in intensive care units: a retrospective cohort study. BMC Pulm. Med. 23, 43. doi:10.1186/s12890-022-02302-6
Mikkelsen M. E., Shah C. V., Meyer N. J., Gaieski D. F., Lyon S., Miltiades A. N., et al. (2013). The epidemiology of acute respiratory distress syndrome in patients presenting to the emergency department with severe sepsis. Shock 40, 375–381. doi:10.1097/SHK.0b013e3182a64682
Nattino G., Finazzi S., Bertolini G. (2016). A new test and graphical tool to assess the goodness of fit of logistic regression models. Stat. Med. 35, 709–720. doi:10.1002/sim.6744
Nenke M. A., Rankin W., Chapman M. J., Stevens N. E., Diener K. R., Hayball J. D., et al. (2015). Depletion of high-affinity corticosteroid-binding globulin corresponds to illness severity in sepsis and septic shock; clinical implications. Clin. Endocrinol. (Oxf). 82, 801–807. doi:10.1111/cen.12680
Niu X. K., He W. F., Zhang Y., Das S. K., Li J., Xiong Y., et al. (2017). Developing a new PI-RADS v2-based nomogram for forecasting high-grade prostate cancer. Clin. Radiol. 72, 458–464. doi:10.1016/j.crad.2016.12.005
Qiu X., Huang F., Li Z., Wei X., Wu J., Huang J., et al. (2022). Develop a novel nomogram to predict respiratory failure in acute pancreatitis at early stage. Clin. Lab. 68, 68. doi:10.7754/Clin.Lab.2021.210826
Rhodes A., Evans L. E., Alhazzani W., Levy M. M., Antonelli M., Ferrer R., et al. (2017). Surviving sepsis campaign: international guidelines for management of sepsis and septic shock: 2016. Intensive Care Med. 43, 304–377. doi:10.1007/s00134-017-4683-6
Roquilly A., Jacqueline C., Davieau M., Molle A., Sadek A., Fourgeux C., et al. (2020). Alveolar macrophages are epigenetically altered after inflammation, leading to long-term lung immunoparalysis. Nat. Immunol. 21, 636–648. doi:10.1038/s41590-020-0673-x
Rubio I., Osuchowski M. F., Shankar-Hari M., Skirecki T., Winkler M. S., Lachmann G., et al. (2019). Current gaps in sepsis immunology: new opportunities for translational research. Lancet Infect. Dis. 19, e422–e436. doi:10.1016/S1473-3099(19)30567-5
Silva T. B., Oliveira C. Z., Faria E. F., Mauad E. C., Syrjanen K. J., Carvalho A. L. (2015). Development and validation of a nomogram to estimate the risk of prostate cancer in Brazil. Anticancer Res. 35, 2881–2886.
Singer M., Deutschman C. S., Seymour C. W., Shankar-Hari M., Annane D., Bauer M., et al. (2016). The third international consensus definitions for sepsis and septic shock (Sepsis-3). JAMA 315, 801–810. doi:10.1001/jama.2016.0287
Subbe C. P., Kruger M., Rutherford P., Gemmel L. (2001). Validation of a modified early warning score in medical admissions. QJM 94, 521–526. doi:10.1093/qjmed/94.10.521
Takasu O., Gaut J. P., Watanabe E., To K., Fagley R. E., Sato B., et al. (2013). Mechanisms of cardiac and renal dysfunction in patients dying of sepsis. Am. J. Respir. Crit. Care Med. 187, 509–517. doi:10.1164/rccm.201211-1983OC
Takeshita Y., Terada J., Hirasawa Y., Kinoshita T., Tajima H., Koshikawa K., et al. (2021). Elevated tat in COVID-19 patients with normal D-dimer as a predictor of severe respiratory failure: a retrospective analysis of 797 patients. J. Clin. Med. 11, 134. doi:10.3390/jcm11010134
Tasaka S., Amaya F., Hashimoto S., Ishizaka A. (2008). Roles of oxidants and redox signaling in the pathogenesis of acute respiratory distress syndrome. Antioxid. Redox Signal 10, 739–753. doi:10.1089/ars.2007.1940
Thongprayoon C., Cheungpasitporn W., Chewcharat A., Mao M. A., Thirunavukkarasu S., Kashani K. B. (2020). Risk of acute respiratory failure among hospitalized patients with various admission serum albumin levels: a cohort study. Med. Baltim. 99, e19352. doi:10.1097/MD.0000000000019352
Vincent J. L., Moreno R., Takala J., Willatts S., De Mendonca A., Bruining H., et al. (1996). The SOFA (Sepsis-related organ failure assessment) score to describe organ dysfunction/failure. On behalf of the working group on sepsis-related problems of the European society of intensive care medicine. Intensive Care Med. 22, 707–710. doi:10.1007/BF01709751
Keywords: sepsis, respiratory failure, predictive models, machine learning, risk factors
Citation: Wang B, Chen J and Wang M (2023) Establishment and validation of a predictive model for respiratory failure within 48 h following admission in patients with sepsis: a retrospective cohort study. Front. Physiol. 14:1288226. doi: 10.3389/fphys.2023.1288226
Received: 04 September 2023; Accepted: 30 October 2023;
Published: 09 November 2023.
Edited by:
Alysson Roncally Silva Carvalho, University of Porto, PortugalReviewed by:
Mariana Avila, Rio de Janeiro State University, BrazilLuciana Moisés Camilo, Universidade do Estado do Rio de Janeiro, Brazil
Copyright © 2023 Wang, Chen and Wang. This is an open-access article distributed under the terms of the Creative Commons Attribution License (CC BY). The use, distribution or reproduction in other forums is permitted, provided the original author(s) and the copyright owner(s) are credited and that the original publication in this journal is cited, in accordance with accepted academic practice. No use, distribution or reproduction is permitted which does not comply with these terms.
*Correspondence: Maofeng Wang, wzmcwmf@163.com