- 1Academy for Engineering and Technology, Fudan University, Shanghai, China
- 2Suzhou Institute of Biomedical Engineering and Technology, Chinese Academy of Sciences, Suzhou, China
- 3School of Biomedical Engineering (Suzhou), Division of Life Sciences and Medicine University of Science and Technology of China, Suzhou, China
- 4Department of Orthopedics, Huashan Hospital, Fudan University, Shanghai, China
- 5Cognitive Systems Lab, University of Bremen, Bremen, Germany
Extracting muscle synergies from surface electromyographic signals (sEMGs) during exercises has been widely applied to evaluate motor control strategies. This study explores the relationship between upper-limb muscle synergies and the performance of joystick manipulation tasks. Seventy-seven subjects, divided into three classes according to their maneuvering experience, were recruited to perform the left and right reciprocation of the joystick. Based on the motion encoder data, their manipulation performance was evaluated by the mean error, standard deviation, and extreme range of position of the joystick. Meanwhile, sEMG and acceleration signals from the upper limbs corresponding to the entire trial were collected. Muscle synergies were extracted from each subject’s sEMG data by non-negative matrix factorization (NMF), based on which the synergy coordination index (SCI), which indicates the size of the synergy space and the variability of the center of activity (CoA), evaluated the temporal activation variability. The synergy pattern space and CoA of all participants were calculated within each class to analyze the correlation between the variability of muscle synergies and the manipulation performance metrics. The correlation level of each class was further compared. The experimental results evidenced a positive correlation between manipulation performance and maneuvering experience. Similar muscle synergy patterns were reflected between the three classes and the structure of the muscle synergies showed stability. In the class of rich maneuvering experience, the correlation between manipulation performance metrics and muscle synergy is more significant than in the classes of trainees and newbies, suggesting that long-term training and practicing can improve manipulation performance, stability of synergy compositions, and temporal activation variability but not alter the structure of muscle synergies determined by a specific task. Our approaches and findings could be applied to 1) reduce manipulation errors, 2) assist maneuvering training and evaluation to enhance transportation safety, and 3) design technical support for sports.
1 Background
Fine manipulation refers to the ability to perform precise actions or delicate tasks using hands or tools, presented as coordination and precision of movements (Tang et al., 2017; Tang et al., 2021). In the training and selection of pilots, evaluating the control ability of the joystick is critical, as the joystick is one of the primary control devices in an aircraft and common interactive tools applied with muscle synergy. In (Bezerra et al., 2019), the mean and peak electromyographic activation values during joystick operation have been studied for favorable pilot load conditions. Previous research also revealed that muscle coordination changes during motor adaptation to the viscous force field generated by haptic interface (Fabio et al., 2016) or visuomotor rotation (Gentner et al., 2013).
Joystick manipulation is a multi-joint coordinated motion for which numerous control modes exist in the motion control of the musculoskeletal system. Movements are inherently variable and can be as consistent as possible by repetition of professional athletes. Various studies have evidenced that the central nervous system (CNS) can generate motor commands through a series of synergistic combinations of muscles (d’Avella et al., 2003; Bizzi et al., 2008; Bizzi and Cheung, 2013), known as muscle synergy. Muscle synergy has recently been considered a common method for studying complex motor control patterns, applied to research fields of nerve damage effects (Roh et al., 2013; Tang et al., 2017), human posture control (Torres-Oviedo and Ting, 2007; Robert and Latash, 2008; Asaka et al., 2011), and locomotion of robot-assisted technology (Pham et al., 2011; Salman et al., 2010; Wang et al., 2021a; Wang et al., 2021b; Scano et al., 2019). Muscle contractions during exercise can be divided into isometric and isotonic. In isometric tasks, the module structures of muscle synergies are robust to changes in speed, and the neural commands to muscle synergies change in response to speed changes (Kojima et al., 2017). The motor control strategy can be modified depending on the accuracy requirement of the isometric reach task (Ettema et al., 2005a; Sano et al., 2020). In joystick isometric dual degree-of-freedom torque tasks, the torque history affects coordination patterns, and the motor system controls different orthogonal combinations of torque oscillations and constant torques employing a single oscillating muscle synergy (Ettema et al., 2005b). In joystick isometric force feedback tasks (Camardella et al., 2021), divided muscle synergies into groups with specific motor strategies and the same global groups for all subjects, confirming that muscle sets can be reduced to achieve comparable hand force estimation performances. For isotonic contraction tasks, the literature shows that the CNS can perceive the relationship between stiffness level and the size of the endpoint deviation (Gribble et al., 2003; Osu et al., 2004) and alter the mechanical impedance of the limb through the simultaneous activation of antagonist muscle groups (Feldman and Levin, 1995; Burdet et al., 2001), revealing the critical role of co-contraction in arm movement performance.
In addition to co-contraction indices, inter- and intra-subject similarities are hotspots in motor coordination (Alnajjar and Shimoda, 2017; Barnamehei et al., 2018b; Esmaeili and Maleki, 2020). Studies have indicated that muscle synergies are a valuable tool for quantifying variability at the muscle level and reveal that intra-subject variability is lower than inter-subject variability in synergy modules and related temporal coefficients, and both intra-subject and inter-subject similarities are higher than random synergy matching, confirming shared underlying control structures (Zhao et al., 2021). During specific movements, two indices of muscle cooperative stability and cooperative space are introduced for the CNS, characterized by the best recruitment mechanism in the process of balance behavior proficiency (Alnajjar and Shimoda, 2017). Taking kicking as an example, elite players have a high similarity in the stability of muscle synergies (Barnamehei et al., 2018b). In a controlled experiment with overhead shots, individual muscle patterns ranged from moderate to highly similar between elite and non-elite groups (Barnamehei et al., 2018a). These results suggest that the CNS can create optimal sets of efficient behaviors by optimizing the size of the synergy space in the appropriate region by interacting with the environment (Alnajjar et al., 2013). However, the group comparison did not correlate muscle synergy results with the performance of each exercise.
Training is hypothesized to change manipulation performance and muscle synergies, and there is a specific correlation between them. Subjects with different maneuvering experiences are selected to perform the rocker manipulation task. This study explores an objective and effective method to explore the relationship between muscle synergy and manipulation performance, providing theoretical support for sports science and scientific training methods. To the best of our knowledge, this article is the first to study the relationship between muscle synergy and joystick manipulation position accuracy. This fine activity is practical and essential for sports and transportation, rather than the broader sense movements of the limb or joint, often studied in related fields.
2 Data preparation: subjects, hardware, and setup
2.1 Subjects, classes, and ethics
Seventy-seven healthy right-handed male subjects, detailed in Table 1, aged 17–55 (25.18 ± 13.73) years, volunteered to participate in this study and were divided into three classes according to their maneuvering experience.
• Class Experts consists of professional pilots with over 1,000 h of flight time;
• Class Trainees is formed by short-haul experienced pilots who have completed 7–8 h of flight time in the company of professionals;
• Class Newbies contains high school students without experience in flying missions or joystick operations.
All subjects were informed of the experiment procedure approved by the Ethics Review Committee of Fudan University (No. FE22064R).
2.2 Experimental apparatus
Figure 1A exhibits our self-developed experimental apparatus achieving rocker X/Y operation in two directions, for which the motor encoder recorded the position of the joystick in real-time. Users operate the experimental apparatus, interacting with an upper computer interface. Figures 1B,C exemplify one record of a trail.
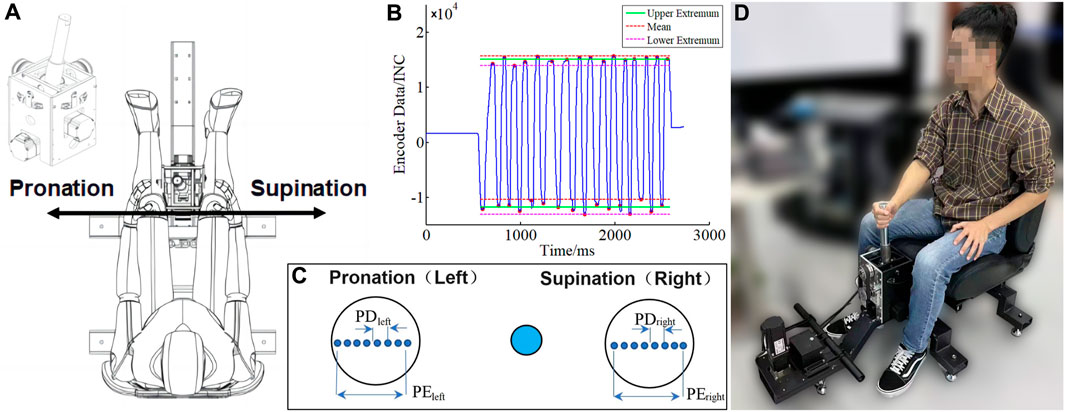
FIGURE 1. Self-developed experimental device and software interface. (A) Top view of the device for experiments; (B) An exemplar result record. PD: position deviation; PE: extreme range of the position. (C) A screenshot of the software interface; (D) Schematic experimental scene.
The angle of the joystick ranges from ±15°, normalizing the angle to the spacing of the two black circles on the screen. The precision of the joystick is determined by the clearance of the planetary reducer, which has a backlash of two arc minutes, that is, 0.03 mm on the screen. The sensitivity of the device is determined by the resolution of the motor encoder, 0.005 mm. The self-implemented prototype acquired position data with a position sampling frequency of 200 Hz.
The coordination of the shoulder, elbow, wrist, and fingers enables the manipulation of the joystick. The reciprocating motion in this study is designed under a constant load condition (see Figure 1B). The internal and external rotation of the shoulder and the pronation/supination of the forearm were performed during the experiment, while the elbow joint was retracted in the coronal plane and flexed/extended in the sagittal plane. The range of motion of the forearm was more evident than that of the elbow and shoulder joints.
Figure 1D illustrates the schematic experimental scene. All subjects carried out experiments in their own habitual postures that remained consistent and could have a slight forward lean. The base of the experimental apparatus was fixed, and the seat backrest was lowered backward to maintain enough space for the human body. Therefore, no contact between the electrodes and the backrest was observed or detected in the experiment.
2.3 Acquisition details
For convenience in the discussion, the entire experimental procedure was described in terms of supination and pronation. During the experiment, subjects were asked to sit upright on the chair. The joystick moved back and forth along the X-axis, as depicted in Figure 1A. The blue ball (10 mm) and the black circle (20 mm) were initialized in the center and on the left/right sides, respectively (see Figure 1C); the joystick moved right along the +X-axis, while the blue ball synchronized with the right circle and vice versa.
Subjects were asked to maintain a certain distance between their elbows and thighs, thus exerting force with the upper limbs. They were required to place the blue ball precisely in the circle center as quickly as possible while maintaining accuracy. The blue ball reciprocated between the black circles, providing instant feedback on user operations while prioritizing accuracy. All participants performed 16 repetitions in each test to ensure that at least 14 were completed. The manipulation procedures and the corresponding sEMG data were recorded simultaneously.
Operating the rocker arm requires rotation of the forearm and the shoulder. The internal rotation of the shoulder mainly involves the anterior deltoid (DA), the pectoralis major (PM), the latissimus dorsi, and the teres minor (TM), while the external rotation involves the infraspinatus (INF), the teres minor (TM), and the posterior deltoid (DP). The energy of inner rotation is much higher than that of outer rotation (Ijiri et al., 2020). The biceps brachii (short/long head) can be used as a supinator muscle, where the biceps play a role in the activity of medium or high-power supination. The triceps brachii (long/lateral head) can be activated by the same length and neutralize the tendency of the biceps to bend the elbow (Soubeyrand et al., 2017). The pronator muscle consists of the pronator muscle and the pronator teres muscle. The brachioradialis (BRAD) is used primarily for elbow flexion but can also be used for pronation.
As exhibited in Figure 2, a DELSYS Trigno system was used to collect sEMG and acceleration data from 10 muscles of the upper arm and shoulder, including BRAD, short head of the biceps (BICS), long head of the biceps (BICL), PM, DA, long head of the triceps (TRIL), lateral head of the triceps (TRILA), INF, TM, and DP. The placement of the sEMG electrodes follows the guidelines for noninvasive electromyographic assessment of muscles, as shown in Figure 2, at a sampling rate of 1,249 Hz, while the acceleration was sampled at 149 Hz. The acquired signals were processed by MATLAB (R2017b, MathWorks Natick, United States).
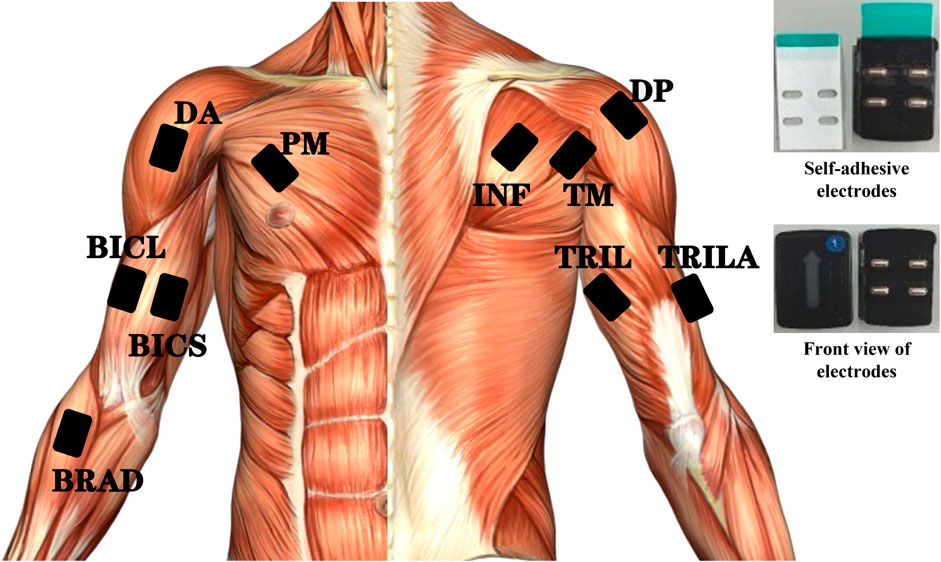
FIGURE 2. Placement and sensing muscles of sEMG sensors. Top right: self-adhesive electrodes. BRAD: brachioradialis; BICS: the short head of biceps; BICL: the long head of biceps; PM: pectoralis major; DA: anterior deltoid; TRIL: the long head of triceps; TRILA: the lateral head of triceps; INF: infraspinatus; TM: teres minor; DP: posterior deltoid.
3 Methodology
3.1 Processing of motion encoder data
The position data were derived from the motor encoder (see Figure 1C) and processed in the following steps to provide valid manipulation performance data.
• Normalization and median filtering: Normalized values within the (−1, 1) interval of the raw data were filtered in the median to eliminate interference noise from abnormal burrs. The filter used 30 points and a window length of 150 ms.
• Indexing: Each successive data portion that contains all values (above a threshold T or below −T) was indexed, resulting in no fewer than 14 valid manipulation data partitions, as instantiated by the red dots in Figure 1B. T defaulted to 0.8 and was manually adjusted in a few cases to obtain the correct number of manipulation repetitions.
• Averaging and deviation: The position deviation (PD) is the deviation between the mean value of all actual positions on all trails, as instantiated by the green baselines in Figure 1B and the theoretical value of the black circle, indicating the precision of the position. The repeatability of the position (PR) represents the standard deviation of all actual positions. The extreme range of the position (PE) means the range of operation on each side.
3.2 Preprocessing of sEMG data
Before extracting muscle synergies, the acquired sEMG signal was pre-processed.
• A mean shift procedure eliminated baseline shifts caused by trial or subject electrode displacements.
• A Butterworth filter of 20–250 Hz removed high-frequency noise, with which motion artifacts were also significantly eliminated.
• Fixed band-stop filters removed the frequencies of 50 Hz and 150 Hz.
• A full-wave rectification flipped the negative EMG signal above the baseline to ensure positive values.
• A Butterworth filter of 20 Hz filtered the rectified signal to provide a smooth envelope (Kieliba et al., 2018).
• Min-Max scaling normalized the signal.
The simultaneous use of sEMG and ACC signals is a paradigm often adopted for human activity studies (Liu and Schultz, 2022; Liu. et al., 2023). A single extraction have been performed over the whole trips (Oliveira et al., 2014). The acceleration signal (ACC) was interpolated due to different sampling rates and filtered from median to segment the results of muscle synergy activation. Pre-processed signals Vm×t (m is the channel of muscles) are decomposed:
where W is the basis matrix for muscle activation, n is the number of muscle synergies, and H holds the coefficients of the activation of the n muscle synergies. Wi (i = 1, 2, …, n) is a vector of the muscle synergy matrix, each element of which takes a value between 0 and 1. Wis, specifying the participation level of each muscle in each synergy and forming a muscle synergy, are activated by the activation coefficient matrix Hi, which represents the neural command of the CNS and determines the relative contribution of the muscle synergy matrix (Tang et al., 2017).
The reconstructed matrix
which is directly related to the value of n. Variability accounted for (VAF) is defined as
which could be used to decide the value of n. VAF quantifies the percentage of muscle cooperativity extracted relative to the original EMG signal. The literature shows that the signal is considered successfully reconstructed when the VAF is greater than 90% or until the addition of synergy does not further increase the VAF by an amount greater than 5% (Clark et al., 2010). The number of muscle synergies n ∈ [1, 10] is selected as the minimum number that could adequately reconstruct pre-processed signals in all trials (Tang et al., 2017).
3.3 Quantitative muscle synergy similarity
3.3.1 Muscle synergy spaces
SCI (synergy coordination index) is used to evaluate the size of the resulting synergy space, in other words, the coordination between the synergies utilized. In class c ∈ {Experts, Trainees, Newbies}, the subject k’s synergy matrix M(c, k) and H (c, k) are extracted from the sEMG signals of all the corresponding trials. Each segmented ACC signal for muscle synergy activation is regarded as a circle, interpolated to 100 data points based on time.
Only positive vector components have a cooperative space before the non-negative matrix decomposition for the estimation of W, Furthermore, the vectors Wi (i = 1, 2, …, n) are generally not orthogonal to each other. The size of the cooperative space depends on the relative angle of the vector Wi. To quantify the size of the collaborative space, we define SCI in terms of the inner product of Wi:
SCI values range from 0 to 1. An SCI of 1 means that all vectors Wi are identical, while 0 means that all vectors Wi are orthogonal. The larger the SCI, the smaller the synergy space. The median and standard deviation values of SCI describe the synergy space of the group.
3.3.2 Variability of muscle activation
In addition to computing the variability of W, an evaluation was also performed on the relationship between the variability of performance and the variability of synergy recruitment through the analysis of H, with the variability of the center of activity (CoA) in the form of standard deviation. The CoA of class c is defined as
where Kc denotes the number of subjects in class c (k = 1, 2, …, Kc) and
where the CoA during the mth part of Hi(c, k),
3.4 Statistical analysis
The descriptive statistics in this work involve mean values and standard deviations. Four one-way repeated analyses of variance (ANOVA) were performed on the synergy similarity and manipulation performance metrics (PR, PD, PE) among the three classes. In addition, the Pearson correlation coefficient r was analyzed through the manipulation performance (PRleft, PRright, PDleft, PDright, PEleft, and PEright), SCI,
4 Results
4.1 Statistical analysis of manipulation performance metrics
The performance metrics for the manipulation of the three classes, PR, PD, and PE, are analyzed by one-way ANOVA in Figure 3, where all data passed the homogeneity of variance test. Significant differences were discovered in the values of PRleft (F = 4.955; p = 0.010), PRright (F = 2.735; p = 0.071), and PEleft (F = 3.415; p = 0.038) between the three classes. Note that the asterisk indicates high significance (p < 0.050), as does the following. Comparing each pair of classes yields the following findings.
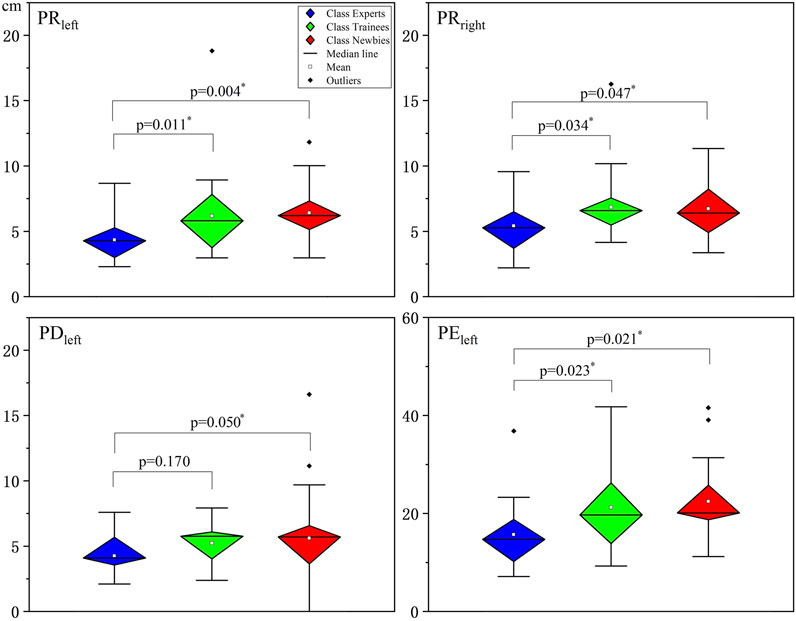
FIGURE 3. Significant results of the three classes’ manipulation performance metrics analyzed by one-way analyses of variance (ANOVA). The vertical axes indicate the error value. All data passed the homogeneity of variance test. The asterisks indicate high significance (p < 0.05). PR: repeatability of the position; PD: position deviation; PE: extreme range of the position. Note that PEleft differs from the other three subplots in the vertical axis range.
PRleft and PRright
Compared to Experts, Trainees (Δ = −1.80 ± 0.70; p = 0.011) and Newbies (Δ = −2.07 ± 0.70; p = 0.004) have larger PR-left values; However, Figure 3(PRleft) shows no significant difference between Trainees and Newbies. The case of PRright is similar: Trainees (Δ = −1.43 ± 0.66; p = 0.034) and Newbies (Δ = −1.32 ± 0.62; p = 0.047) are not significantly different, while both are larger than Experts, as diagnosed in Figure 3(PRright).
PDleft
Newbies’ PDleft (Δ = −1.35 ± 0.70; p = 0.050) is significantly larger than Experts’ PDleft. At the same time, Trainees’ PDleft did not show a significant difference (Δ = −0.96 ± 0.70; p = 0.177) compared to Newbies’ and Experts’ results, as reflected in Figure 3(PDleft).
PEleft
The relationship of the PEleft difference between Experts, Trainees (Δ = −6.95 ± 2.90; p = 0.021), and Newbies (Δ = −6.70 ± 2.90; p = 0.023) is the same as in the case of PRleft/right, which can be verified by Figure 3(PEleft).
4.2 Muscle synergy extraction
Figure 4 instantiates the non-negative matrix factorization (NMF) decomposition process of ten upper limb muscles. The basis matrix of muscle activation W and the activation coefficient matrix H are shown in Figure 4A.
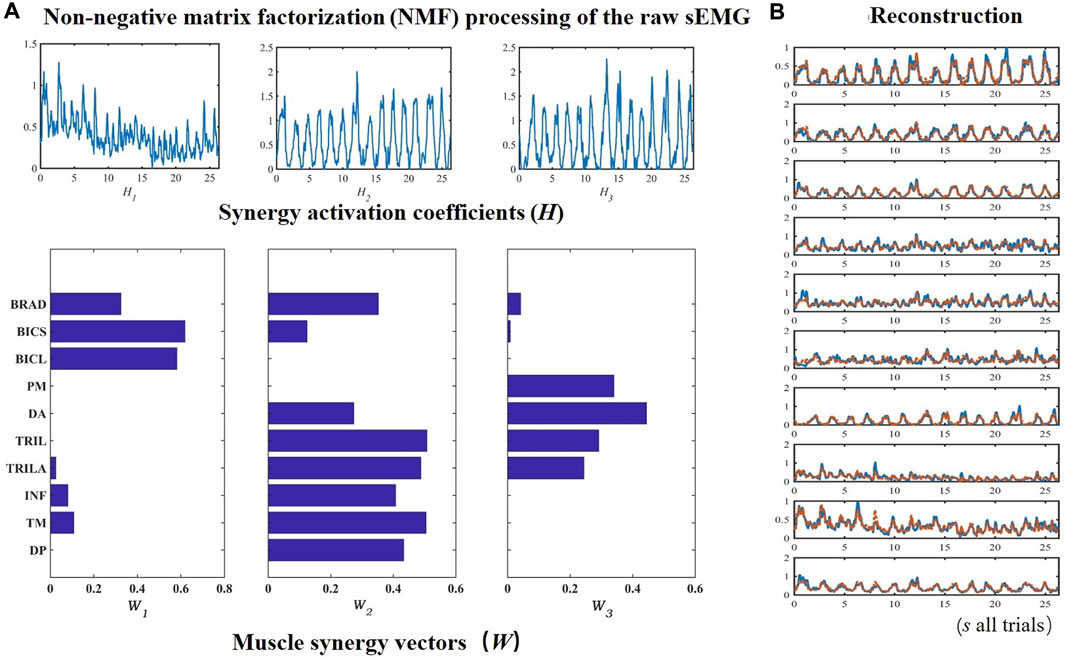
FIGURE 4. An example of extracting muscle synergies on ten upper limb muscles from Trainees. The sEMG data from ten upper limb muscles were processed by non-negative matrix factorization (A), based on which synergy activation coefficients and muscle synergy vectors were used to reconstruct the sEMG (B).
Figure 4B evinces that the synergy W1 between the muscle synergy vectors W mainly reflects the activation of BRAD, BICS, and BICL, whose synergies are greater than 0.3. W2 primarily comprises DP, TM, INF, TRIL, TRILA, BICL, and BRAD. The dominant composition elements of W3’s are DA, PM, TRILA, and TRIL. The sEMG could be reconstructed by H and W, as manifested with the raw sEMG of ten muscles of the upper limbs in Figure 4B. Figure 5 revealed that after the number of synergies reaches three, the mean VAF of each class exceeds 95%.
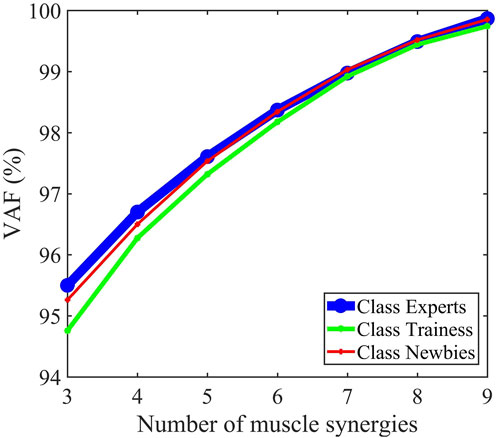
FIGURE 5. Mean percentage of variability accounted for (VAF) as a function describing the number of extracted synergies. Three synergies were identified for the three classes.
4.3 Intraclass muscle synergy extratcion
The colorized bars in Figure 6 indicate different subjects in classes Experts (19 subjects), Trainees (30 subjects), and Newbies (28 subjects), and the black bars represent the mean values among each class for each muscle. PM has a low proportion in both INF W1 and W2, while DP, TM, INF, and TRIL exhibit low values in W3, found in all three classes. Figure 7 embodies the Pearson correlation coefficients among the three classes for synergies W1, W2, and W3. Figures 6, 7 show that the three classes have the same muscle synergies patterns, occurring in the same order.
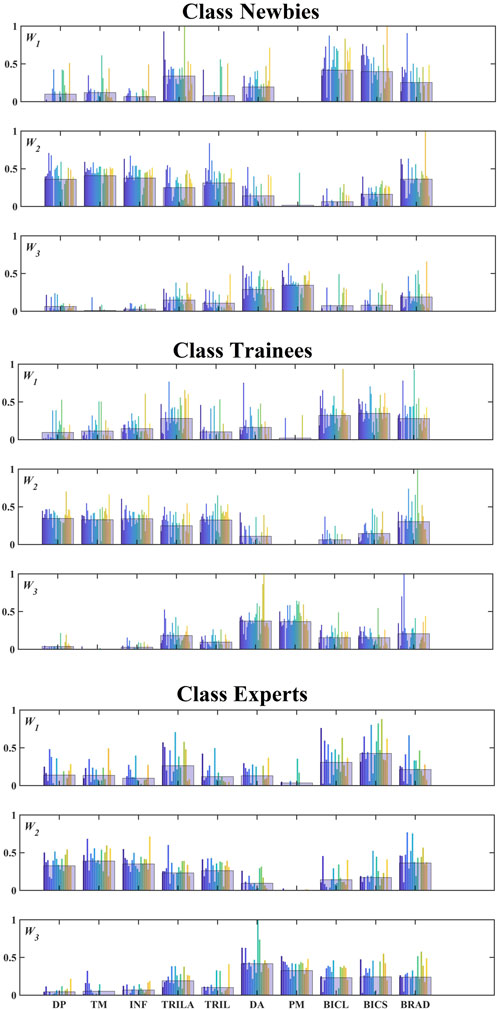
FIGURE 6. Composition of muscle synergies for the three classes. Three synergies were extracted from all subjects and matched among the classes. The colorized bars indicate different subjects. Note that the numbers of subjects in the three classes are 19 (Experts), 30 (Trainees), and 28 (Newbies). The black-bordered wide bar for each muscle indicates the mean value.
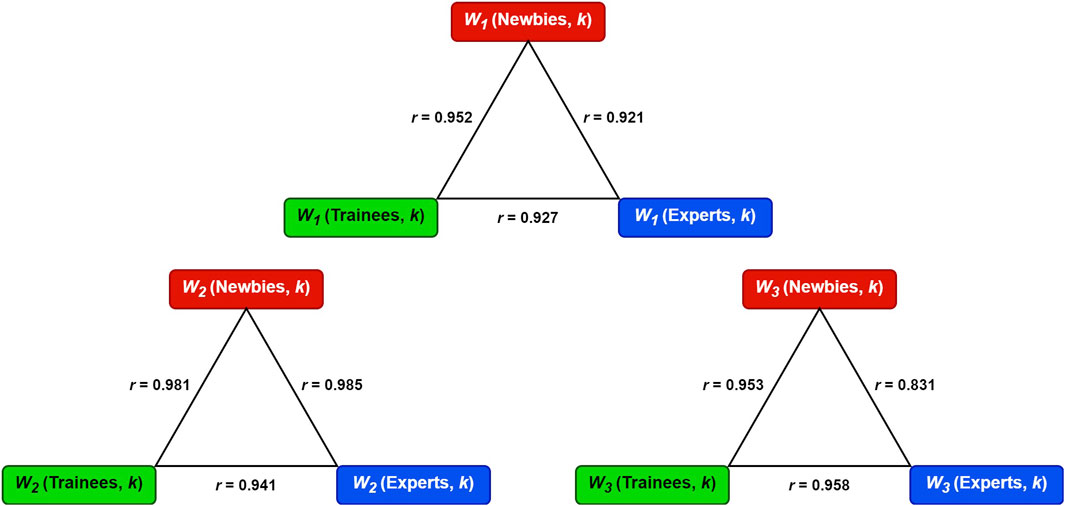
FIGURE 7. The Pearson correlation coefficients (r) among the three classes for synergies W1, W2, and W3.
4.4 Muscle synergy space
In addition to the three extracted muscle synergies elucidated in Figure 6, Figure 8 presents the SCI values of the three classes, where SCI(Experts) is 0.419 ± 0.138, SCI(Trainees) is 0.290 ± 0.070, and SCI(Newbies) is 0.270 ± 0.087, respectively. The SCI value of experts was significantly higher (p = 0.000, p = 0.001) than the values of trainees and newbies, between which no significant difference (p = 0.608) can be concluded.
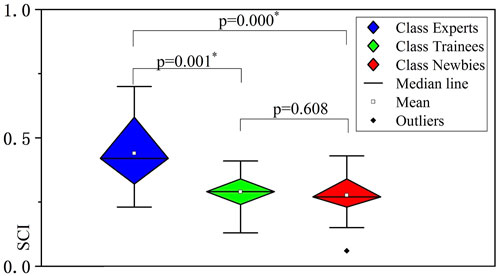
FIGURE 8. Significance results of the three classes’ spaces of muscle synergies analyzed by nonparametric test. The vertical axes indicate the synergy coordination index (SCI) values. The asterisks indicate high significance (p < 0.05).
4.5 The center of synergistic activities
Figure 9 exhibits the resulting CoA values and the interclass comparison.
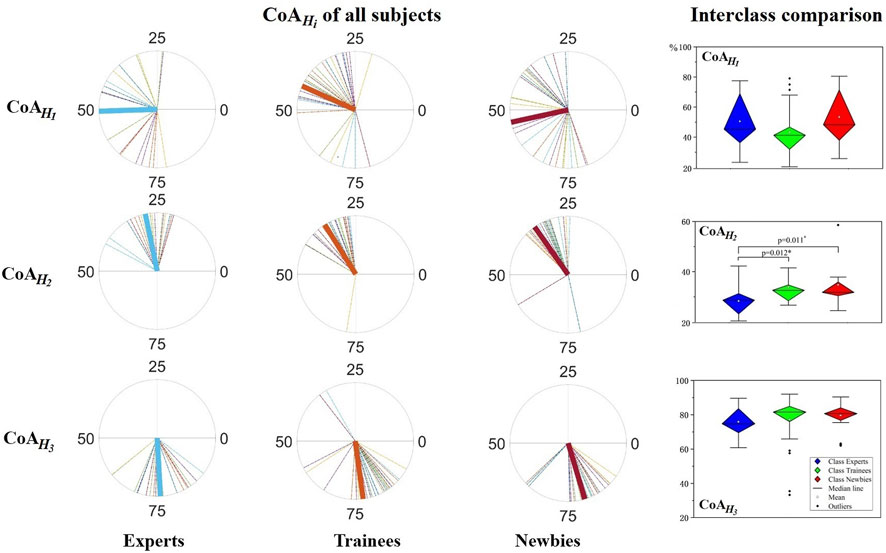
FIGURE 9. Center of activity (CoA) analysis for muscle synergy. Left:
Figure 10 provides a variability comparison between the three classes by STD values. Experts’ intraclass variability of
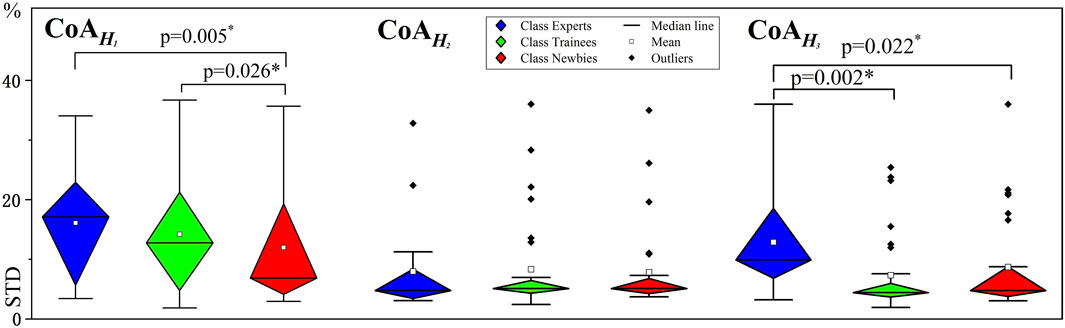
FIGURE 10. Variability analysis for muscle synergy. The vertical axes indicate standard deviation (STD) values. The asterisks indicate high significance (p < 0.05).
4.6 Correlation between manipulation performance and analytical metrics
Figure 11 details the Pearson correlation coefficients between manipulation performance metric and SCI/CoA/STD. In general, the results of Experts often show significant negative correlation values between the manipulation performance metrics PRleft/PRright/PEleft/PEright and SCI/CoA.
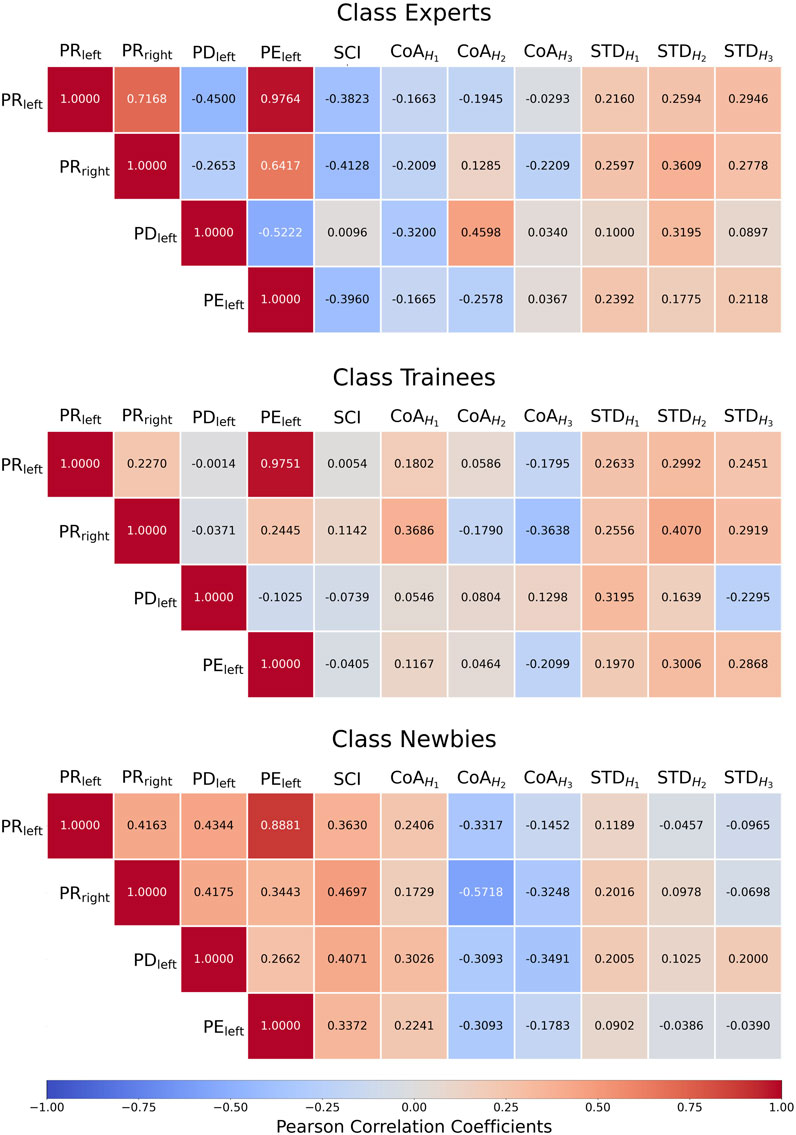
FIGURE 11. Three classes’ heatmaps of the Pearson correlation coefficients between manipulation performance metrics and synergy coordination index (SCI)/the center of activity (CoA)/CoA’s standard deviation (STD).
4.6.1 Manipulation performance versus SCI
For Experts, PRleft, PRright, and PELeft in manipulation performance metrics show high negative correlations with SCI (−0.3823, −0.4128, and −0.3960), and PDleft has a positive, close to zero correlation with SCI (0.0096). On the contrary, newbies collectively have high positive correlations between PRleft/PRright/PDleft/PELeft and SCI (0.3630, 0.4697, 0.4071, and 0.3397). The absolute correlations of the trainees are low (−0.005, −0.1142, −0.0739, −0.0405). The experimental results are consistent with the important muscle learning methodology (Alnajjar et al., 2013). Newbies’ muscle status W) shows variability, which SCI reflects, whereas Experts tend to stabilize.
4.6.2 Manipulation performance versus CoA
Newbies show negative correlations between the
4.6.3 Manipulation performance versus STD (standard deviation of CoA)
The manipulation performance of Experts is positively correlated with the standard deviation of CoA in H1–H3 (r = 0.0897–0.3609). Trainees’ STD values are essentially similar to Experts’, except that the PDleft-
5 Discussion
Variability is a natural component of human movement. The notion of muscle synergy variability contains a lower-dimensional synergy space and time-dependent variables. Oriented towards better operation, variability affects the performance of motor tasks. As muscle synergy spaces are gradually optimized, movement performance will improve.
Based on the muscle synergy analysis of joystick manipulation in three classes of maneuvering experience, this study reveals the correlation between manipulation performance and muscle synergy variability, reflected by SCI and the standard deviation of CoA.
5.1 Structure of muscle synergies
The synergy structure corresponds to the synergy number, of which the patterns are related to the task. Consistency is demonstrated in different individuals. In this study, three muscle synergies were investigated, consistent with previous studies. Three to five muscles work together in the three-dimensional force generation of the upper extremity (Coscia et al., 2014). Four to five synergies can reconstruct the activation patterns of up to 19 muscles recorded during point-to-point reaching movements or forearm postures with different loads (d’Avella et al., 2003). In comparison, three synergies are sufficient to reconstruct multijoint movements performed in the horizontal plane (Roh et al., 2012). The consistency of the synergy number could be affected by individual differences such as muscle size, strength, subcutaneous fat thickness, and measurement electrode position, which is also in line with previous work. In addition to the physiological factors mentioned above, the synergy number may also be influenced by sEMG pre-processing. The variance accounted for (VAF) criterion is sensitive to the low-pass cut-off frequency, with higher cut-offs resulting in lower VAF (Kieliba et al., 2018).
The pattern of muscle synergies studied in this work is qualitatively similar to those underlying three-dimensional force generation (Roh et al., 2012). Their flexion pattern consisting of BRAD and BI with DP activity is similar to W1 identified in our experiments, while their flexion pattern comprised of TRILA, TRIL, and PM is similar to the W3 (see Figure 4). Figure 6 exhibits that neuromuscular strategies remain the same in the three classes, interpreting that joystick manipulation experience does not cause variations in the pattern of muscle synergies. Previous studies on lifting or shoulder movement had similar results: Muscle synergy is consistent between experts and normal people during lifting (Zhao et al., 2021) or shoulder movement (Turpin et al., 2020). In general, the structure of the muscle synergy was influenced by biomechanics and task constraints (Roh et al., 2012).
5.2 Muscle synergy variability
Muscle synergy spaces vary with training. Previous studies have suggested that muscle synergies may be formed by adaptive processes related to the individual’s experience. The activity of the primary motor cortex is adapted with training, associated with changes in either the amplitude of activation or the composition of the correlated synergy. As a result of maintaining balance, the body will achieve a stable pattern of muscle synergy and variable activation after training (Alnajjar et al., 2013). CNS searches for the most appropriate synergy space region, evoking various strategies by tuning W. In such a search stage, low stability of W is observed. The modulation of the muscle synergy composition will develop to a stable state during skill learning (Kargo and Nitz, 2003) (see Figure 8). Experts showed higher SCI, representing smaller muscle synergy spaces and more excellent stability. The relatively concentrated muscle synergy spaces observed among experts should be explained by their long-term maneuvering experience, which enables their CNS to create optimal sets of efficient behaviors by optimizing the size of the synergy space in the appropriate region (Alnajjar et al., 2013).
In addition to space properties, the variability of temporal activation is also affected by training. Previous studies have shown that CoA changes with walking speed (Kibushi et al., 2018b), load (Labini et al., 2011; Sylos-Labini et al., 2014), and cerebellar ataxia (Martino et al., 2014), among others. This study reveals a significant shift in
5.3 Significant negative correlation between manipulation performance metrics and muscle synergy variability
A smaller synergy space is often used for a better performance, which can answer why the coordinated movements respond to disturbance (Alnajjar and Shimoda, 2017). In this study, Figure 11 evinces that the stability of the muscle synergy space is positively correlated with the manipulating error in the novice stage (Newbies) and negatively correlated in the veteran stage (Experts). On Experts, the smaller the synergy space, the better the performance. In contrast, Newbies are in the search stage of W, so the larger the synergy space, the better the performance.
The CNS learning model suggests that the CNS attempts to reduce the degrees of freedom of the resulting motions by restricting the variability of the synergy coefficient matrix H) during the search stage of W and simplifies the handling of its high temporal variability (Alnajjar et al., 2013). Therefore, Newbies’ correlation between CoA and manipulation performance is more significant than that of the other two classes. In the Experts and Trainees classes, no significant correlation is detected between CoA and manipulation performance. Consistent with previous studies, manipulation performance can reflect proficiency degree: Once a certain proficiency level is reached, the body’s restrictions on muscle activation will be lifted, endowing a complete utilization of the body’s potency (i.e., W variability decreases, H variability increases) (Alnajjar et al., 2013).
Expert’s and Trainees’ variability (STD) of
6 Conclusion
This article analyses manipulation performance and muscle synergy in three classes of subjects with different maneuvering experiences. Different levels of experience and training lead to apparent differences in the space size of W and the variability of H. The precision of the manipulation is related to the variability of muscle synergy. Long-term training compresses muscle synergy space, which enhances muscle manipulation performance. Experts have significantly higher muscle synergy space stability and activation variability than the other two classes. Experts’ and Trainees’ correlation values between manipulation performance and the standard deviation of CoA are more significant than Newbies’.
This work has designed experiments, grouped 77 subjects, collected multimodal data, and conducted various experimental analyses, all of which have informed subsequent research. Our findings and validations are looked to for progressive practical applications that can provide important references for the development of software to aid pilot training, such as tips and training procedures to reduce manipulation errors as well as training result analysis and evaluation, which can improve efficiency, save costs, and potentially increase the safety of flight.
Data availability statement
The raw data supporting the conclusion of this article will be made available by the authors upon request, without undue reservation, except for commercial use.
Ethics statement
The studies involving humans were approved by Ethics Committee, Fudan University. The studies were conducted in accordance with the local legislation and institutional requirements. The participants provided their written informed consent to participate in this study.
Author contributions
LC: Conceptualization, Funding acquisition, Methodology, Software, Writing–original draft, Visualization. SY: Data curation, Writing–original draft, Writing–review and editing. CO: Data curation, Writing–review and editing. TZ: Investigation, Writing–review and editing. JZ: Formal Analysis, Writing–review and editing. LC: Formal Analysis, Writing–review and editing. XM: Formal Analysis, Supervision, Validation, Writing–review and editing. HL: Formal Analysis, Funding acquisition, Supervision, Writing–review and editing, Validation, Visualization, Writing–original draft.
Funding
The author(s) declare financial support was received for the research, authorship, and/or publication of this article. The APC was funded by the Open Access Initiative of the University of Bremen and the DFG via SuUB Bremen. The research was supported by Jiangsu Province Industrial Foresight and Key Core Technology Project, No. BE2022064-2: Research and Development of Key Technologies for Demonstration and Application of Multi-scenario Brain-Computer Interaction System for Smart Elderly Care.
Acknowledgments
We acknowledge the support of Jun Zhong and Xiankai Cheng for developing experimental equipment.
Conflict of interest
The authors declare that the research was conducted in the absence of any commercial or financial relationships that could be construed as a potential conflict of interest.
Publisher’s note
All claims expressed in this article are solely those of the authors and do not necessarily represent those of their affiliated organizations, or those of the publisher, the editors and the reviewers. Any product that may be evaluated in this article, or claim that may be made by its manufacturer, is not guaranteed or endorsed by the publisher.
References
Alnajjar F., Shimoda S. (2017). “Muscle synergies indices to quantify the skilled behavior in human,” in 3rd International Conference on NeuroRehabilitation (ICNR), 959–963. of Biosystems and Biorobotics. doi:10.1007/978-3-319-46669-9_15515
Alnajjar F., Wojtara T., Kimura H., Shimoda S. (2013). Muscle synergy space: learning model to create an optimal muscle synergy. Front. Comput. Neurosci. 7, 136. doi:10.3389/fncom.2013.00136
Asaka T., Yahata K., Mani H., Wang Y. (2011). Modulations of muscle modes in automatic postural responses induced by external surface translations. J. Mot. Behav. 43, 165–172. doi:10.1080/00222895.2011.552079
Barnamehei H., Ghomsheh F. T., Cherati A. S., Pouladian M. (2018a). Upper limb neuromuscular activities and synergies comparison between elite and nonelite athletics in badminton overhead forehand smash. Appl. Bionics Biomechanics 2018, 6067807. doi:10.1155/2018/6067807
Barnamehei H., Razaghi M., Panahi S., Modabberibejandi M., Lashgari M., Safaei M. A., et al. (2018b). “Identification and quantification of modular control during roundhouse kick executed by elite taekwondo players,” in 25th Iranian Conference on Biomedical Engineering/3rd International Iranian Conference on Biomedical Engineering (ICBME), 54–59.
Bezerra T. A. R., Shimano A., Santiago P. R. P. (2019). Revitalization of the force simulator and adequacy of joystick loads compatible with toucan t-27 and super toucan at-29 aircraft.
Bizzi E., Cheung V. C. K., d’Avella A., Saltiel P., Tresch M. (2008). Combining modules for movement. Brain Res. Rev. 57, 125–133. doi:10.1016/j.brainresrev.2007.08.004
Bizzi E., Cheung V. C. K. (2013). The neural origin of muscle synergies. Front. Comput. Neurosci. 7, 51. doi:10.3389/fncom.2013.00051
Burdet E., Osu R., Franklin D. W., Milner T. E., Kawato M. (2001). The central nervous system stabilizes unstable dynamics by learning optimal impedance. Nature 414, 446–449. doi:10.1038/35106566
Camardella C., Junata M., Tse K. C., Frisoli A., Tong R. K.-Y. (2021). How many muscles? Optimal muscles set search for optimizing myocontrol performance. Front. Comput. Neurosci. 15, 668579. doi:10.3389/fncom.2021.668579
Clark D. J., Ting L. H., Zajac F. E., Neptune R. R., Kautz S. A. (2010). Merging of healthy motor modules predicts reduced locomotor performance and muscle coordination complexity post-stroke. J. neurophysiology 103, 844–857. doi:10.1152/jn.00825.2009
Coscia M., Cheung V. C. K., Tropea P., Koenig A., Monaco V., Bennis C., et al. (2014). The effect of arm weight support on upper limb muscle synergies during reaching movements. J. NeuroEngineering Rehabilitation 11, 22. doi:10.1186/1743-0003-11-22
d’Avella A., Saltiel P., Bizzi E. (2003). Combinations of muscle synergies in the construction of a natural motor behavior. Nat. Neurosci. 6, 300–308. doi:10.1038/nn1010
Esmaeili J., Maleki A. (2020). Muscle coordination analysis by time-varying muscle synergy extraction during cycling across various mechanical conditions. Biocybern. Biomed. Eng. 40, 90–99. doi:10.1016/j.bbe.2019.10.005
Ettema G. J. C., Taylor E., North J. D., Kippers V. (2005a). Muscle synergies at the elbow in static and oscillating isometric torque tasks with dual degrees of freedom. Mot. Control 9, 59–74. doi:10.1123/mcj.9.1.59
Ettema G. J. C., Taylor E., North J. D., Kippers V. (2005b). Muscle synergies at the elbow in static and oscillating isometric torque tasks with dual degrees of freedom. Mot. control 9, 59–74. doi:10.1123/mcj.9.1.59
Fabio O., Christian F., Steve A., Giulio R. (2016). Changes in muscle coordination patterns induced by exposure to a viscous force field. J. NeuroEngineering Rehabilitation 13, 1–16. doi:10.1186/s12984-016-0164-3
Feldman A. G., Levin M. F. (1995). The origin and use of positional frames of reference in motor control. Behav. Brain Sci. 18, 723–744. doi:10.1017/s0140525x0004070x
Franklin D. W., Liaw G., Milner T. E., Osu R., Burdet E., Kawato M. (2007). Endpoint stiffness of the arm is directionally tuned to instability in the environment. J. Neurosci. 27, 7705–7716. doi:10.1523/jneurosci.0968-07.2007
Gentner R., Edmunds T., Pai D. K., d’Avella A. (2013). Robustness of muscle synergies during visuomotor adaptation. Front. Comput. Neurosci. 7, 120. doi:10.3389/fncom.2013.00120
Gribble P. L., Mullin L. I., Cothros N., Mattar A. (2003). Role of cocontraction in arm movement accuracy. J. Neurophysiology 89, 2396–2405. doi:10.1152/jn.01020.2002
Ijiri T., Urabe Y., Maeda N., Sasadai J., Suzuki T. (2020). Comparative study of the differences in shoulder muscle activation according to arm rotation angle. Hum. Mov. Sci. 69, 102567. doi:10.1016/j.humov.2019.102567
Kargo W. J., Nitz D. A. (2003). Early skill learning is expressed through selection and tuning of cortically represented muscle synergies. J. Neurosci. 23, 11255–11269. doi:10.1523/JNEUROSCI.23-35-11255.2003
Kibushi B., Hagio S., Moritani T., Kouzaki M. (2018a). Speed-dependent modulation of muscle activity based on muscle synergies during treadmill walking. Front. Hum. Neurosci. 12, 4. doi:10.3389/fnhum.2018.00004
Kibushi B., Hagio S., Moritani T., Kouzaki M. (2018b). Speed-dependent modulation of muscle activity based on muscle synergies during treadmill walking. Front. Hum. Neurosci. 12, 4. doi:10.3389/fnhum.2018.00004
Kieliba P., Tropea P., Pirondini E., Coscia M., Micera S., Artoni F. (2018). How are muscle synergies affected by electromyography pre-processing? Ieee Trans. Neural Syst. Rehabilitation Eng. 26, 882–893. doi:10.1109/tnsre.2018.2810859
Kojima S., Takeda M., Nambu I., Wada Y. (2017). “Relations between required accuracy and muscle synergy in isometric contraction tasks,” in IEEE International Conference on Systems, Man, and Cybernetics (SMC), 1191–1195.
Kristiansen M., Madeleine P., Hansen E. A., Samani A. (2015). Inter-subject variability of muscle synergies during bench press in power lifters and untrained individuals. Scand. J. Med. Sci. Sports 25, 89–97. doi:10.1111/sms.12167
Labini F. S., Ivanenko Y. P., Cappellini G., Gravano S., Lacquaniti F. (2011). Smooth changes in the emg patterns during gait transitions under body weight unloading. J. neurophysiology 106, 1525–1536. doi:10.1152/jn.00160.2011
Liu H., Schultz T. (2022). “How long are various types of daily activities? Statistical analysis of a multimodal wearable sensor-based human activity dataset,” in Proceedings of the 15th International Joint Conference on Biomedical Engineering Systems and Technologies (BIOSTEC 2022) - HEALTHINF (INSTICC (SciTePress), 680–688. doi:10.5220/0010896400003123
Liu H., Xue T., Schultz T. (2023). “On a real real-time wearable human activity recognition system,” in Proceedings of the 16th International Joint Conference on Biomedical Engineering Systems and Technologies - WHC (INSTICC (SciTePress), 711–720. doi:10.5220/0011927700003414
Martino G., Ivanenko Y. P., Serrao M., Ranavolo A., d’Avella A., Draicchio F., et al. (2014). Locomotor patterns in cerebellar ataxia. J. neurophysiology 112, 2810–2821. doi:10.1152/jn.00275.2014
McKay J. L., Ting L. H. (2008). Functional muscle synergies constrain force production during postural tasks. J. Biomechanics 41, 299–306. doi:10.1016/j.jbiomech.2007.09.012
Oliveira A. S., Gizzi L., Farina D., Kersting U. G. (2014). Motor modules of human locomotion: influence of emg averaging, concatenation, and number of step cycles. Front. Hum. Neurosci. 8, 335. doi:10.3389/fnhum.2014.00335
Osu R., Kamimura N., Iwasaki H., Nakano E., Harris C. M., Wada Y., et al. (2004). Optimal impedance control for task achievement in the presence of signal-dependent noise. J. Neurophysiology 92, 1199–1215. doi:10.1152/jn.00519.2003
Pham H. T. T., Kimura M., Hirai H., Miyazaki F. (2011). “Extraction and implementation of muscle synergies in hand-force control,” in IEEE International Conference on Robotics and Automation (ICRA), 2658–2663.
Robert T., Latash M. L. (2008). Time evolution of the organization of multi-muscle postural responses to sudden changes in the external force applied at the trunk level. Neurosci. Lett. 438, 238–241. doi:10.1016/j.neulet.2008.04.052
Roh J., Rymer W. Z., Beer R. F. (2012). Robustness of muscle synergies underlying three-dimensional force generation at the hand in healthy humans. J. Neurophysiology 107, 2123–2142. doi:10.1152/jn.00173.2011
Roh J., Rymer W. Z., Perreault E. J., Yoo S. B., Beer R. F. (2013). Alterations in upper limb muscle synergy structure in chronic stroke survivors. J. Neurophysiology 109, 768–781. doi:10.1152/jn.00670.2012
Salman B., Vahdat S., Lambercy O., Dovat L., Burdet E., Milner T., et al. (2010). “Changes in muscle activation patterns following robot-assisted training of hand function after stroke,” in IEEE/RSJ International Conference on Intelligent Robots and Systems. IEEE International Conference on Intelligent Robots and Systems, 5145–5150. doi:10.1109/iros.2010.5650175
Sano T., Takeda M., Nambu I., Wada Y. (2020). “Relations between speed-accuracy trade-off and muscle synergy in isometric contraction tasks,” in 42nd Annual International Conference of the IEEE-Engineering-in-Medicine-and-Biology-Society (EMBC). IEEE Engineering in Medicine and Biology Society Conference Proceedings, 4803–4806.
Scano A., Dardari L., Molteni F., Giberti H., Tosatti L. M., d’Avella A. (2019). A comprehensive spatial mapping of muscle synergies in highly variable upper-limb movements of healthy subjects. Front. Physiology 10, 1231. doi:10.3389/fphys.2019.01231
Soubeyrand M., Assabah B., Begin M., Laemmel E., Dos Santos A., Creze M. (2017). Pronation and supination of the hand: anatomy and biomechanics. Hand Surg. Rehabilitation 36, 2–11. doi:10.1016/j.hansur.2016.09.012
Sylos-Labini F., La Scaleia V., d’Avella A., Pisotta I., Tamburella F., Scivoletto G., et al. (2014). Emg patterns during assisted walking in the exoskeleton. Front. Hum. Neurosci. 8, 423. doi:10.3389/fnhum.2014.00423
Tang L., Chen X., Cao S., Wu D., Zhao G., Zhang X. (2017). Assessment of upper limb motor dysfunction for children with cerebral palsy based on muscle synergy analysis. Front. Hum. Neurosci. 11, 130. doi:10.3389/fnhum.2017.00130
Tang W., Xiao Y., Xu F., Wang C. (2021). Design and application of fine operation ability test. Manned Spacefl. 27, 227–233. doi:10.16329/j.cnki.zrht.2021.02.014
Ting L. H., Macpherson J. M. (2005). A limited set of muscle synergies for force control during a postural task. J. Neurophysiology 93, 609–613. doi:10.1152/jn.00681.2004
Torres-Oviedo G., Ting L. H. (2007). Muscle synergies characterizing human postural responses. J. Neurophysiology 98, 2144–2156. doi:10.1152/jn.01360.2006
Turpin N. A., Martinez R., Begon M. (2020). Shoulder muscle activation strategies differ when lifting or lowering a load. Eur. J. Appl. Physiology 120, 2417–2429. doi:10.1007/s00421-020-04464-9
Wang C., Zhang S., Hu J., Huang Z., Shi C. (2021a). Upper-limb muscle synergy features in human-robot interaction with circle-drawing movements. Appl. Bionics Biomechanics 2021, 8850785. doi:10.1155/2021/8850785
Wang T., Okada S., Guo A., Makikawa M., Shiozawa N. (2021b). “Effect of assist robot on muscle synergy during sit-to-stand movement,” in 2nd IEEE International Conference on Intelligence and Safety for Robotics (IEEE-ISR) (Ieee), 367–368. doi:10.1109/isr50024.2021.9419525
Keywords: muscle synergy extraction, muscle synergy similarity, joystick manipulation, maneuvering, electromyography, EMG
Citation: Cai L, Yan S, Ouyang C, Zhang T, Zhu J, Chen L, Ma X and Liu H (2023) Muscle synergies in joystick manipulation. Front. Physiol. 14:1282295. doi: 10.3389/fphys.2023.1282295
Received: 23 August 2023; Accepted: 27 September 2023;
Published: 13 October 2023.
Edited by:
Kelly Johnson, Coastal Carolina University, United StatesReviewed by:
Eduardo Mendonca Scheeren, Pontifícia Universidade Católica do Paraná, BrazilDamian Kelty-Stephen, Grinnell College, United States
Copyright © 2023 Cai, Yan, Ouyang, Zhang, Zhu, Chen, Ma and Liu. This is an open-access article distributed under the terms of the Creative Commons Attribution License (CC BY). The use, distribution or reproduction in other forums is permitted, provided the original author(s) and the copyright owner(s) are credited and that the original publication in this journal is cited, in accordance with accepted academic practice. No use, distribution or reproduction is permitted which does not comply with these terms.
*Correspondence: Xin Ma, xinma_faet@fudan.edu.cn; Hui Liu, hui.liu@uni-bremen.de