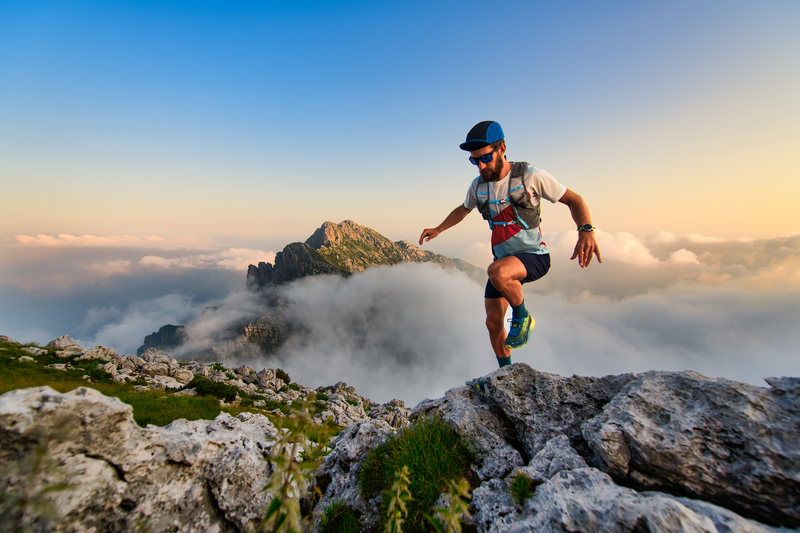
95% of researchers rate our articles as excellent or good
Learn more about the work of our research integrity team to safeguard the quality of each article we publish.
Find out more
OPINION article
Front. Physiol. , 26 July 2023
Sec. Computational Physiology and Medicine
Volume 14 - 2023 | https://doi.org/10.3389/fphys.2023.1223308
This article is part of the Research Topic Rising stars in Non-Neuronal Cells 2022 View all 5 articles
Brain development is a complex process that involves a cerebrovascular shifting landscape with marked expansion and refinement for metabolic homeostasis. This cerebrovascular system is a 3D network composed of arteries, capillaries, and veins (Coelho-Santos and Shih, 2020). Remarkable in several ways, the capillary network that forms the blood brain barrier (BBB), conferring brain protection from the periphery, it is also responsible for most of the nutrients and wastes exchange. Indeed, the wellbeing of the cerebrovascular network is essential to keep the metabolic homeostasis across the lifespan, by matching the brain’s high energy demand with the supply of energy substrates and oxygen from the blood, through a process called neurovascular coupling (Iadecola, 2017).
Cerebrovascular network architecture is not predetermined, but a result of biological responses (angiogenesis, remodelling, and pruning) of the vascular endothelial cells to the stimuli that they receive. During brain development, the growth of the initial vascular plexus, that resulted from vasculogenesis, occurs by angiogenesis (Crouch et al., 2022; Coelho-Santos et al., 2021), resulting in an increase of the local nutrient and oxygen supply at the demand of the active neural tissue. Neuronal and vascular systems grow in parallel and seem influence each other (Lacoste et al., 2014). This initial angiogenesis expansion of the vascular plexus is followed by a remodelling process driven by the blood flow and shear stress: the increase in shear stress promotes endothelial cell proliferation (Pries et al., 2015) and higher blood pressures promote vascular smooth muscle cell proliferation (Loerakker et al., 2018), leading to vessel widening. On the other hand, in already oxygenated regions or in vessels where the flow is very low, endothelial cells return to the parent’s vessels through pruning and vascular regression (Franco et al., 2015). Along this journey, mural cells (smooth muscle cells and pericytes) (Coelho-Santos et al., 2021; Crouch et al., 2022) and neurons (Lacoste et al., 2014) are already in intimacy contact within the endothelium participating in the expansion of the vascular system. However, other cells like astrocytes are recruited later to join the vasculature providing quiescence and maturation (Gilbert et al., 2019). Altogether, these cells constitute a functional neurogliovascular unit. Therefore, neurovascular coupling, the communication between neurons and the vascular system, becomes efficient after birth (Kozberg et al., 2016).
Understanding cerebrovascular network development has been challenging due to its complexity and poor accessibility during development. Much of the important knowledge we have nowadays about vascular network development has been gathered from studies done in zebrafish, heart or retina (Franco et al., 2015; Pries et al., 2015). Although some characteristics of the vascular systems may be common in different organs due to their similarities, we need to individualise this system to each organ, namely, the mammalian brain. Brain endothelium has particular features such as BBB and consequently its vascular system is unique. Our insight about the mammalian cerebrovascular network development is still evolving. Just recently, we have opened the “window” to explore the cortical cerebrovascular development in the neonatal brain (Harb et al., 2013; Letourneur et al., 2014; Coelho-Santos et al., 2021). The appearance of cutting-edge techniques such as multiphoton microscopy allowed the longitudinal study in greater detail of the capillary network construction (Coelho-Santos et al., 2021). Multiphoton microscopy is a preferred method to image in vivo dynamic cellular processes such as neurovascular coupling due its high resolution and low toxicity for dynamic live imaging (Shih et al., 2012). Right now, only cortical live imaging data is available for the neonatal brain but hopefully more brain regions will start to be explored such as visual cortex, deep cortical layers (grey-white matter interface), hippocampus or cerebellum and the new models can be adapted to different regions and their vascular heterogeneity. The multiphoton high-resolution data from adult mice have been coupled with mathematical modelling to investigate flow after vascular diameter increase (Berthiaume et al., 2022; Schmid et al., 2021). However, inferring neonatal cerebrovascular function from adult data should be avoided since the newborn brain is more than ‘a tiny adult brain’ with particular characteristics. We have learned from imaging studies that brain vasculature in infants is extremely dynamic, developing new branches and remodelling existing vessels to fulfil the function of irrigating the brain tissue. Nevertheless, some of the modelling framework used to analyse the adult brain can be translated to the infant brain. For instance, the network segmentation acquired through multiphoton microscopy to obtain the vascular morphology and organisational architecture, and then the implementation of mathematical models of flow and irrigation to follow longitudinally the gain of function in the infant brain. In particular, through the vascular segmentation of the infant brain could be obtain the vascular network architecture and structure (by identifying how the network organises its hierarchy, the connections between vessels and their length, branches points and diameters overtime), point out the inlets and outlets in the network, oxygenation levels, and the vascular distribution within other cells of the brain. Additionally, we can collect information about how other cells such as glial and mural cells modulate the capillary formation and maturation. These data can then be fed into mathematical models to evaluate the vascular network function in infant brains of different ages.
Strikingly, vascular development in tissues has been thoroughly modelled during the last 20 years. Though some angiogenesis mathematical models are focused on cellular traction forces, cell-cell communication and rearrangement during sprout extension (Santos-Oliveira et al., 2015; Gómez-Escudero et al., 2019), several others have simulated the growth of a complete bifurcating vasculature following local gradients of vascular growth factors produced by hypoxic tissue cells (Caforio et al., 2022; Sousa et al., 2021; Xu et al., 2016; Vavourakis et al., 2017). Moreover, models of vascular remodelling and pruning have been developed both at cell level (Loerakker et al., 2018), as well as at vessel and network levels (Pries et al., 2015). Therefore, in tandem with microscopy observation, mathematical modelling of blood flow, angiogenesis and vascular remodelling has the ability to explore the increase in function of the growing vascular network as it develops, leading to a better understanding of the mechanisms of vascular adaptation in vivo.
The detailed calculation of blood flow from the network geometry is not trivial as it requires solving the 3D-Navier-Stokes equations inside the volume of the vessels with knowledge of the time dependent pressure profiles at the network inlets and outlets, as well as of the elasticity of the vessel and surrounding tissue (Quarteroni et al., 2016; Oliveira et al., 2020). However, simplifications of these complex calculations are often implemented either to address the propagation of pressure waves along the vessels, through so-called 1-D models (Quarteroni et al., 2016; Caforio et al., 2022), or to calculate the time average blood flow and tissue irrigation (Linninger et al., 2019; Secomb et al., 2013; Shih et al., 2015; Berthiaume et al., 2022). These latter simplified models, also called 0-D models of blood flow, normally consider laminar flow, but importantly may take into account the asymmetric division of hematocrit at the network bifurcations, and the blood viscosity dependence on vessel width (Fåhræus and Lindqvist, 1931; Schmid et al., 2019). In the smallest vessels the blood cannot be considered as a Newtonian fluid as the mechanics of erythrocyte deformation influence flow. Still, 0-D models can take into account both the non-newtonian behaviour of blood and the influence of vessel wall elasticity in flow, if required (Yáñez et al., 2019; Flores et al., 2013). The simplicity of these models has permitted the estimation of blood flow in very large networks as in whole murine brains (Linninger et al., 2019; Hartung et al., 2021). By computing the hematocrit in the network, these models can be coupled with descriptions of oxygen flux along the vessels (Secomb et al., 2013, 2004), so that the transport of oxygen within the tissue can be predicted. In this way, 0-D models have the capacity to identify the regions of low oxygen pressure in the developing cerebral tissue and may be coupled to angiogenesis modelling (Sousa et al., 2021) to predict blood vessel development in vivo. Consequently, the observation of vascular development through multiphoton microscopy coupled with irrigation models allows to understand how the growing vascular network operates towards improving the initial deficient irrigation.
At shorter timescales, mathematical modelling can readily analyse the increase in irrigation consequence of localised and rapid alterations in blood vessel diameter (Terman, 2023). For this aim, 0-D models are particularly useful as they predict flow and irrigation in large networks. It is thus possible to use these techniques to compare the irrigation alteration after the increase in diameter of a specific pial arteriole, for example, or of a large number of vessels in the same neighbourhood instead. Vessels’ diameter alterations may be both active (consequence of direct neurovascular coupling and dependent on the contractile mechanics of the mural cells, smooth muscle cells and pericytes, layers in the vessel) or passive (consequence of local alterations in blood pressure). To calculate passive diameter alterations mathematical models have to include the relation between vessel diameter and blood pressure. In the case of arterioles, this requires a detailed implementation of the myogenic effect in the code (Carlson et al., 2008; Flores et al., 2013). In addition, these models can be included into reinforcement learning algorithms that predict the minimal set of vessels needed to increase their diameter to irrigate a specific region after the neuronal activation. By comparing with in vivo vascular activity, it will be possible to learn if the adult brain follows an optimal strategy in setting the locations for neurovascular coupling. In fact, we are still learning and uncovering this concept that has divided opinions due some controversy about how the neurovascular coupling evolves and what are the cells and mechanisms involved. And through longitudinal microscopy imaging we may learn how the infant brain gains this capacity for blood flow regulation and what is the influence of glial or mural cells in the neurovascular coupling according to their appearance in development of the neurogliovascular unit.
Cerebrovascular growth and maturation results from a complex network of cellular and acellular interactions across different spatiotemporal scales. Nevertheless, the angio-adaptation models we described above have the limitation of not incorporating the multiscale nature of the cerebrovascular system, which is crucial. There is therefore room for improvement. Coupled with high resolution and longitudinal data collected from multiphoton microscopy, models of vascular remodelling, blood flow and irrigation with reinforcement learning will provide a playground to generate hypotheses and test mechanisms of cerebrovascular expansion and maturation. Following vascular development during the first days after birth will allow to parameterise the irrigation and blood flow models, by identifying the regions of low oxygen delivery where neo-vascularisation and remodelling occurs in a more realistic and biological way. These models can then be used to calculate irrigation after a myriad of combined vessel morphology alterations. Reinforcement learning will identify optimal strategies to increase irrigation locally, as well as the optimal mechanical properties of the vessels that will better adapt to changes in flow.
The challenge in the next few years will be to combine data from cellular interactions during development, since the blood flow as well as the oxygen content are changing at the same time the vascular connections are being made. The combination of these data into models could markedly improve the understanding how vascular dysfunction and malformation leads to neurodevelopmental and neurological diseases. And eventually improve the diagnosis, prognosis as well as the treatment, which is expected to revolutionise personalised medicine in the near future. We are in the right Era to build physiological angio-adaptation models using in vivo image data from neonatal construction of the cerebrovascular network.
RT and VC-S wrote equally the manuscript. All authors contributed to the article and approved the submitted version.
RT thanks the support of FEDER funds through the Operational Programme Competitiveness Factors—COMPETE and Fundação para a Ciência e a Tecnologia through the strategic projects UIDB/04564/2020 and UIDP/04564/2020. VC-S received the support of a Junior Leader fellowship from “La Caixa” Foundation (LCF/BQ/PI22/11910036).
The authors declare that the research was conducted in the absence of any commercial or financial relationships that could be construed as a potential conflict of interest.
All claims expressed in this article are solely those of the authors and do not necessarily represent those of their affiliated organizations, or those of the publisher, the editors and the reviewers. Any product that may be evaluated in this article, or claim that may be made by its manufacturer, is not guaranteed or endorsed by the publisher.
Berthiaume, A.-A., Schmid, F., Stamenkovic, S., Coelho-Santos, V., Cara, D. N., Weber, B., et al. (2022). Pericyte remodeling is deficient in the aged brain and contributes to impaired capillary flow and structure. Nat. Commun. 13 (1), 5912. doi:10.1038/s41467-022-33464-w
Caforio, F., Christoph, M. A., Alastruey, J., Gsell, M. A. F., and Plank, G. (2022). A coupling strategy for a first 3D-1D model of the cardiovascular system to study the effects of pulse wave propagation on cardiac function. Comput. Mech. 70 (4), 703–722. doi:10.1007/s00466-022-02206-6
Carlson, B. E., Arciero, J. C., and Secomb, T. W. (2008). Theoretical model of blood flow autoregulation: Roles of myogenic, shear-dependent, and metabolic responses. Am. J. Physiology. Heart Circulatory Physiology 295 (4), H1572–H1579. doi:10.1152/ajpheart.00262.2008
Coelho-Santos, V., Berthiaume, A.-A., Ornelas, S., Stuhlmann, H., and Shih, A. Y. (2021). Imaging the construction of capillary networks in the neonatal mouse brain. Proc. Natl. Acad. Sci. U. S. A. 118 (26), e2100866118. doi:10.1073/pnas.2100866118
Coelho-Santos, V., and Shih, A. Y. (2020). Postnatal development of cerebrovascular structure and the neurogliovascular unit. Wiley Interdiscip. Rev. Dev. Biol. 9 (2), e363. doi:10.1002/wdev.363
Crouch, E. E., Bhaduri, A., Andrews, M. G., Cebrian-Silla, A., Diafos, L. N., Ochoa Birrueta, J., et al. (2022). Ensembles of endothelial and mural cells promote angiogenesis in prenatal human brain. Cell 185 (20), 3753–3769.e18. doi:10.1016/j.cell.2022.09.004
Fåhræus, R., and Lindqvist, T. (1931). The viscosity of the blood in narrow capillary tubes. Am. J. Physiology-Legacy Content 96 (3), 562–568. doi:10.1152/ajplegacy.1931.96.3.562
Flores, J., Romero, A. M., Travasso, R. D. M., and Poiré, E. C. (2013). Flow and anastomosis in vascular networks. J. Theor. Biol. 317, 257–270. doi:10.1016/j.jtbi.2012.10.005
Franco, C. A., Jones, M. L., Miguel, O. B., Geudens, I., Mathivet, T., Rosa, A., et al. (2015). Dynamic endothelial cell rearrangements drive developmental vessel regression. PLoS Biol. 13 (4), e1002125. doi:10.1371/journal.pbio.1002125
Gilbert, A., Vidal, X. E., Estevez, R., Cohen-Salmon, M., and Boulay, A.-C. (2019). Postnatal development of the astrocyte perivascular MLC1/GlialCAM complex defines a temporal window for the gliovascular unit maturation. Brain Struct. Funct. 224 (3), 1267–1278. doi:10.1007/s00429-019-01832-w
Gómez-Escudero, J., Clemente, C., García-Weber, D., Acín-Pérez, R., Millán, J., Enríquez, J. A., et al. (2019). PKM2 regulates endothelial cell junction dynamics and angiogenesis via ATP production. Sci. Rep. 9 (1), 15022. doi:10.1038/s41598-019-50866-x
Harb, R., Whiteus, C., Freitas, C., and Grutzendler, J. (2013). In vivo imaging of cerebral microvascular plasticity from birth to death. J. Cereb. Blood Flow Metabolism 33 (1), 146–156. doi:10.1038/jcbfm.2012.152
Hartung, G., Badr, S., Mihelic, S., Dunn, A., Cheng, X., Kura, S., et al. (2021). Mathematical synthesis of the cortical circulation for the whole mouse brain-Part II: Microcirculatory closure. Microcirculation 28 (5), e12687. doi:10.1111/micc.12687
Iadecola, C. (2017). The neurovascular unit coming of age: A journey through neurovascular coupling in health and disease. Neuron 96 (1), 17–42. doi:10.1016/j.neuron.2017.07.030
Kozberg, M. G., Ma, Y., Shaik, M. A., Kim, S. H., and Hillman, E. M. C. (2016). Rapid postnatal expansion of neural networks occurs in an environment of altered neurovascular and neurometabolic coupling. J. Neurosci. 36 (25), 6704–6717. doi:10.1523/JNEUROSCI.2363-15.2016
Lacoste, B., Comin, C. H., Ben-Zvi, A., Kaeser, P. S., Xu, X., Costa, L. F., et al. (2014). Sensory-related neural activity regulates the structure of vascular networks in the cerebral cortex. Neuron 83 (5), 1117–1130. doi:10.1016/j.neuron.2014.07.034
Letourneur, A., Chen, V., Waterman, G., and J Drew, P. (2014). A method for longitudinal, transcranial imaging of blood flow and remodeling of the cerebral vasculature in postnatal mice. Physiol. Rep. 2 (12), e12238. doi:10.14814/phy2.12238
Linninger, A., Grant, H., Badr, S., and Ryan, M. (2019). Mathematical synthesis of the cortical circulation for the whole mouse brain-Part I. Theory and image integration. Comput. Biol. Med. 110, 265–275. doi:10.1016/j.compbiomed.2019.05.004
Loerakker, S., Oscar, M. J. A. S., Huurne, F. M. T., Boareto, M., Bouten, C. V. C., and Sahlgren, C. M. (2018). Mechanosensitivity of jagged-notch signaling can induce a switch-type behavior in vascular homeostasis. Proc. Natl. Acad. Sci. U. S. A. 115 (16), E3682–E3691. doi:10.1073/pnas.1715277115
Oliveira, D. C., Laranjo, S., Tiago, J., Pinto, F. F., and Adélia, S. (2020). Numerical simulation of dilation patterns of the the ascending aorta in aortopathies. J. Mech. Med. Biol. 20 (01), 1950068. doi:10.1142/S0219519419500684
Pries, A. R., Badimon, L., Bugiardini, R., Camici, P. G., Dorobantu, M., J Duncker, D., et al. (2015). Coronary vascular regulation, remodelling, and collateralization: Mechanisms and clinical implications on behalf of the working group on coronary pathophysiology and microcirculation. Eur. Heart J. 36 (45), 3134–3146. doi:10.1093/eurheartj/ehv100
Quarteroni, A., Veneziani, A., and Vergara, C. (2016). Geometric multiscale modeling of the cardiovascular system, between theory and practice. Comput. Methods Appl. Mech. Eng. 302, 193–252. doi:10.1016/j.cma.2016.01.007
Santos-Oliveira, P., Correia, A., Rodrigues, T., Ribeiro-Rodrigues, T. M., Matafome, P., Seiça, R., et al. (2015). The force at the tip--modelling tension and proliferation in sprouting angiogenesis. PLoS Comput. Biol. 11 (8), e1004436. doi:10.1371/journal.pcbi.1004436
Schmid, F., Barrett, M. J. P., Obrist, D., Weber, B., and Jenny, P. (2019). Red blood cells stabilize flow in brain microvascular networks. PLoS Comput. Biol. 15 (8), e1007231. doi:10.1371/journal.pcbi.1007231
Schmid, F., Conti, G., Jenny, P., and Weber, B. (2021). The severity of microstrokes depends on local vascular topology and baseline perfusion. ELife 10, e60208. doi:10.7554/eLife.60208
Secomb, T. W., Alberding, J. P., Hsu, R., Dewhirst, M. W., and Axel, R. P. (2013). Angiogenesis: An adaptive dynamic biological patterning problem. PLoS Comput. Biol. 9 (3), e1002983. doi:10.1371/journal.pcbi.1002983
Secomb, T. W., Hsu, R., Park, E. Y. H., and Dewhirst, M. W. (2004). Green’s function methods for analysis of oxygen delivery to tissue by microvascular networks. Ann. Biomed. Eng. 32 (11), 1519–1529. doi:10.1114/b:abme.0000049036.08817.44
Shih, A. Y., Driscoll, J. D., Drew, P. J., Nishimura, N., Schaffer, C. B., and Kleinfeld, D. (2012). Two-photon microscopy as a tool to study blood flow and neurovascular coupling in the rodent brain. J. Cereb. Blood Flow Metabolism 32 (7), 1277–1309. doi:10.1038/jcbfm.2011.196
Shih, A. Y., Rühlmann, C., Blinder, P., Anna, D., Drew, P. J., Friedman, B., et al. (2015). Robust and fragile aspects of cortical blood flow in relation to the underlying angioarchitecture. Microcirculation 22 (3), 204–218. doi:10.1111/micc.12195
Sousa, F., Costa-Pereira, A. I., Cruz, A., Ferreira, F. J., Gouveia, M., Bessa, J., et al. (2021). Intratumoral VEGF nanotrapper reduces gliobastoma vascularization and tumor cell mass. J. Control. Release Official J. Control. Release Soc. 339, 381–390. doi:10.1016/j.jconrel.2021.09.031
Terman, D. (2023). Modeling the response of homogeneous and heterogeneous cerebral capillary networks to local changes in vessel diameters. J. Theor. Biol. 568, 111509. doi:10.1016/j.jtbi.2023.111509
Vavourakis, V., Wijeratne, P. A., Shipley, R., Loizidou, M., Stylianopoulos, T., and Hawkes, D. J. (2017). A validated multiscale in-silico model for mechano-sensitive tumour angiogenesis and growth. PLoS Comput. Biol. 13 (1), e1005259. doi:10.1371/journal.pcbi.1005259
Xu, J., Vilanova, G., and Gomez, H. (2016). A mathematical model coupling tumor growth and angiogenesis. PloS One 11 (2), e0149422. doi:10.1371/journal.pone.0149422
Keywords: vascular biology, mathematics, modelling, neurodevelopment, neurovascular
Citation: Travasso RDM and Coelho-Santos V (2023) Image-based angio-adaptation modelling: a playground to study cerebrovascular development. Front. Physiol. 14:1223308. doi: 10.3389/fphys.2023.1223308
Received: 19 May 2023; Accepted: 12 July 2023;
Published: 26 July 2023.
Edited by:
Camila Cabral Portugal, Universidade do Porto, PortugalReviewed by:
Daniel Goldman, Western University, CanadaCopyright © 2023 Travasso and Coelho-Santos. This is an open-access article distributed under the terms of the Creative Commons Attribution License (CC BY). The use, distribution or reproduction in other forums is permitted, provided the original author(s) and the copyright owner(s) are credited and that the original publication in this journal is cited, in accordance with accepted academic practice. No use, distribution or reproduction is permitted which does not comply with these terms.
*Correspondence: Rui D. M. Travasso, cnVpdEB1Yy5wdA==; Vanessa Coelho-Santos, dmNzYW50b3NAdWMucHQ=
†These authors have contributed equally to this work
Disclaimer: All claims expressed in this article are solely those of the authors and do not necessarily represent those of their affiliated organizations, or those of the publisher, the editors and the reviewers. Any product that may be evaluated in this article or claim that may be made by its manufacturer is not guaranteed or endorsed by the publisher.
Research integrity at Frontiers
Learn more about the work of our research integrity team to safeguard the quality of each article we publish.