- 1Department of Thoracic Surgery, The First Hospital of Jilin University, Changchun, China
- 2School of Public Health, Jilin University, Changchun, China
- 3Department of Critical Medicine, The First Affiliated Hospital of Jiamusi University, Jiamusi, China
Objective: This study aims to explore the clinical application of an AI-3D reconstruction system in measuring lung volume and analyze its practical value in donor-recipient size matching in lung transplantation.
Methods: The study retrospectively collected data from 75 subjects who underwent a plethysmography examination and lung CT at the First Hospital of Jilin University. General data and information related to lung function, and imaging results were collected. The correlation between actual total lung volume (aTLV), predicted total lung volume (pTLV), and artificial intelligence three-dimensional reconstruction CT lung volume (AI-3DCTVol) was analyzed for the overall, male, and female groups. The correlation coefficient and the absolute error percentage with pTLV and AI-3DCTVol were obtained.
Results: In the overall, male, and female groups, there were statistical differences (p <0.05) between the pTLV formula and AI-3D reconstruction compared to the plethysmography examination value. The ICC between pTLV and aTLV for all study participants was 0.788 (95% CI: 0.515–0.893), p <0.001. Additionally, the ICC value between AI-3D reconstruction and aTLV was 0.792 (95% CI: 0.681–0.866), p <0.001. For male study participants, the ICC between pTLV and aTLV was 0.330 (95% CI: 0.032–0.617), p = 0.006. Similarly, the ICC value between AI-3D reconstruction and aTLV was 0.413 (95% CI: 0.089–0.662), p = 0.007. In the case of female research subjects, the ICC between pTLV and aTLV was 0.279 (95% CI: 0.001–0.523), p = 0.012. Further, the ICC value between AI-3D reconstruction and aTLV was 0.615 (95% CI: 0.561–0.870), p <0.001.
Conclusion: The AI-3D reconstruction, as a convenient method, has significant potential for application in lung transplantation.
Introduction
The evaluation of lung volume has numerous clinical applications, including the assessment of lung function and treatment of chronic diseases, which are crucial for managing patient care effectively (Kojima et al., 2011). The lung volume calculation can help assess patients’ lung function, diagnose diseases and monitor the progression, evaluate surgical risks, and provide auxiliary references for the formulation of diagnosis and treatment plans (Milner, 2000). In recent years, surgical techniques of lung transplantation have made significant progress due to technical advancements in organ transplantation (Valapour et al., 2015; Weill, 2016; Chambers et al., 2018). Accurate lung volume assessment has been increasingly important in lung transplantations, leading to improved organ-matching efficiency and better recipient outcomes (Eberlein et al., 2012a; Eberlein et al., 2012b; Eberlein et al., 2015). Several primary methods such as lung function testing, radiological examination, electrical impedance tomography, and plethysmography are currently available to determine lung volumes (Wolf and Arnold, 2005). Developed by Dubois et al., in 1956, the plethysmography examination measures the residual volume (RV) and the total lung volume (TLV) in the chest cavity based on Boyle’s law (Dubois et al., 1956). The plethysmography examination of lung function testing is considered the most accurate method for lung volume assessment (Goldman and Becklake, 1959). Currently, the lung function evaluation for donor patients with brain death heavily relies on the predicted total lung volume (pTLV) formula, which is developed based on a small group sample from the non-Chinese population (Stocks and Quanjer, 1995; Clayton, 2007). Considering the vast population size of China, the need of a lung volume measurement explicitly designed for the Chinese population is highly demanded (Elmaleh-Sachs et al., 2022; McCormack et al., 2022).
For the past few years, artificial intelligence three-dimensional reconstruction (AI-3D) technology has been developed and applied to evaluate lung and digestive system diseases (Chan et al., 2015; Rahaman et al., 2020; Barmpoutis et al., 2022). The AI-3D reconstruction utilizes CT, MRI, and other scanning technologies to obtain continuous body structure data. Relevant software is used to recognize and process the image data prior to the construction of a virtual three-dimensional model of human organs. Compared to two-dimensional images, three-dimensional models are more intuitive, easier to observe, and provide better visualization of the characteristics and abnormalities of relevant tissues (Wang et al., 2011). Expanding from the outstanding potential of AI-3D reconstruction in our team’s previous research, this study utilizes AI-3D reconstruction technology to process CT image data of the subjects, and acquire lung volume data to ultimately conduct a comparative analysis with traditional calculation formulas and plethysmography examination (Cai et al., 2018; Ma et al., 2023).
Materials and method
Based on the inclusion and exclusion criteria, this study retrospectively collected data from 76 patients who had undergone plethysmography examination and lung CT at the Thoracic Surgery Department of the First Hospital of Jilin University from March 2021 to December 2022. Prior to the examination, every patient undergoes respiratory training to ensure their ability to cooperate with the plethysmography examination. The study also collected the patient’s sex, age, height, weight, and medical history. The Ethics Committee of the First Hospital of Jilin University approved this study (2021-069).
Inclusion criteria
(1) Subjects aged between 18 and 70 years old.
(2) Subjects who have not undergone chest surgery and who do not have severe spinal or thoracic deformities.
(3) Subjects who have normal heart function indicated by echocardiography.
(4) Subjects who can complete a plethysmography examination after respiratory training.
Exclusion criteria
(1) Subjects with a history of chronic lung disease that causes diffuse symptoms in the chest, such as extensive pneumonia and pleural effusion.
(2) Subjects with a history of lung, mediastinal mass, or heart disease or smoking history.
(3) Subjects whose lung CT examination was not performed within 72 h of the physical examination.
(4) Subjects with obstructive ventilation dysfunction, as indicated by an FEV1/FVC (%) of less than 70.
(5) Subjects with restrictive ventilation dysfunction, indicated by a measured/predicted VC or TLV (%) of less than 80.
Plethysmography examination
The examination process followed the plethysmography lung volume and airway resistance examination guidelines set by the Professional Group for Pulmonary Function of the Respiratory Branch of the Chinese Medical Association in 2015 (Association and CMARD, 2015). A master Screen Body plethysmograph (JAEGER Master Screen Body) was used. The testing was conducted 0.5–2 h after eating, and subjects were instructed not to have abdominal distension and to avoid medication such as corticosteroids and bronchodilators that could affect respiratory functions for at least 6 h before testing. The instrument was calibrated for atmospheric pressure and temperature prior to the first inspection on the same day to ensure the accuracy of the inspection. Subjects were also instructed not to wear tight clothing or belts that could restrict chest movement, and prior to testing, they were asked to clean their nasal and oral secretions. Subsequently, the technician explained the testing procedures and precautions, then the subjects entered the physical examination box and adjusted the seat height to a level that the subjects were able to use the mouthpiece without bending or extending their neck. After that, the box was closed, and the physical examination was conducted under the technician’s guidance. Data was collected for three to five sets of tests, with the difference between each set ranging from 0% to 10%, and groups with a coefficient of variation greater than 10% were eliminated manually.
Lung volume calculation formula
This study utilizes the prediction equations of the European Community for Steel and Coal (ECSC), (Quanjer, 1983), presented as follows:
Evaluation of lung volume using AI-3D reconstruction
To achieve optimal reconstruction results, the CT imaging data used in the evaluation process are carefully selected with specific scanning parameters, including a layer thickness of 1 mm, a minimum of 100 images, image continuity, and the inclusion of the complete lung area in the scanning area (512 × 512 pixels minimum) with no observation of breathing artifacts or foreign objects. The lung window and mediastinal data that meet the aforementioned conditions are compressed in the zip format and uploaded to the system of Infervisual AI-3D reconstruction V1, which autonomously desensitizes patient information and segments and reconstructs the lung lobes, trachea, pulmonary arteries, veins, and nodules (Figure 1). The entire model reconstruction is completed by AI independently, without any manual intervention (Xu et al., 2023).
Error analysis
Plethysmography examination is conducted with the person in an upright position, whereas a CT examination is conducted with the person in a supine position. Due to the effect of gravity, the position of the diaphragm can change between the upright and supine positions could lead to variations in lung volume compliance. To control an average increase of 10% in actual total lung volume (aTLV) in the standing position compared to the supine position, we utilize the subsequent two equations for calibration (Yamada et al., 2020).
Statistical analysis
The data analysis was conducted using SPSS 26.0 and R software (4.2.2). Rates and component ratios were utilized to present counting data. To test the balance of general data, one-way ANOVA was applied for quantitative data. Mean ± standard deviation was used to express continuous variables that conform to a normal distribution. The independent sample t-test was used to evaluate continuous variables in two groups that conform to a normal distribution. Comparisons between multiple groups were conducted through an analysis of variance. In the case of continuous variables conforming to normal distribution within a group, a paired t-test was employed. Data that do not exhibit a normal distribution are organized using the median-quartile interval (P25-P75), and statistical analysis is conducted through non-parametric tests. The intra-group correlation coefficient is used to analyze the correlation between different measurement methods. A p-value less than 0.05 indicates statistical significance.
Result
According to the inclusion and exclusion criteria, we collected a sample of 125 subjects who underwent a plethysmography examination and lung CT scan at the Thoracic Surgery Department of the First Hospital of Jilin University between March 2021 and December 2022. After the selection process indicated in Figure 2, we included 75 eligible subjects, consisting of 31 males and 44 females. The basic information about the patients is presented in Table 1.
In the overall, male, and female groups, there were statistical differences (p <0.05) between the pTLV formula and AI-3D reconstruction compared to the plethysmography examination. The mean pTLV in all three groups was greater than aTLV, and the mean aTLV was greater than AI-3DCTVol, as shown in Table 2 and Table 3.
The intra-group correlation coefficient (ICC) between pTLV and aTLV for all study participants was 0.788 (95% CI: 0.515–0.893), p <0.001. Additionally, the ICC value between AI-3D reconstruction and aTLV was 0.792 (95% CI: 0.681–0.866), p <0.001. Notably, the value obtained by AI-3D reconstruction is more similar to the aTLV value. For male study participants, the ICC between pTLV and aTLV was 0.330 (95% CI: 0.032–0.617), p = 0.006, and the ICC value between AI-3D reconstruction and aTLV was 0.413 (95% CI: 0.089–0.662), p = 0.007, which more closely approximates the aTLV value compared to the pTLV method. In the case of female research subjects, the ICC between pTLV and aTLV was 0.279 (95% CI: 0.001–0.523), p = 0.012, and the ICC value between 3D reconstruction and aTLV was 0.615 (95% CI: 0.561–0.870), p <0.001. Similarly, the AI-3D reconstruction value aligns more with the aTLV value in this case. The specific correlation situation is illustrated in Table 4 and Figure 3.
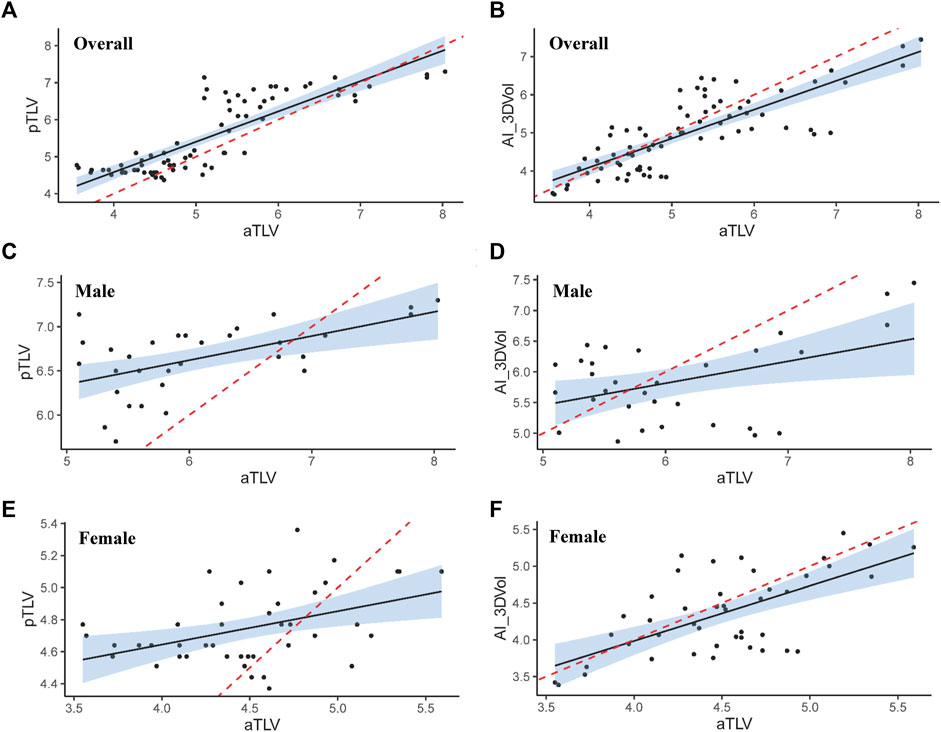
FIGURE 3. The overall correlation between aTLV and pTLV (A), aTLV and AI-3DCTVol (B); Correlation between male aTLV and pTLV (C), aTLV and AI-3DCTVol (D); Correlation between aTLV and pTLV (E), aTLV and AI-3DCTVol (F) (the red dashed line serves as the identification line).
Absolute error percentage analysis of aTLV and pTLV, aTLV and AI-3DCTVol
The percentage of absolute error of pTLV and AI-3DCTVol is calculated as follows:
The absolute error percentage of pTLV and aTLV is greater than that of AI-3DCTVol and aTLV, with significant differences observed in both the overall and male populations (p <0.05). However, no statistical difference was found in the female population (p = 0.464), as shown in Table 5.
Discussion
This study revealed that in all study subjects and subgroups of males and females, the pTLV value was greater than the aTLV value, while the AI-3DCTVol measurement value was less than the aTLV value. The pTLV may be influenced by factors such as age, race, sex, and disease history, resulting in significant numerical deviations (Bellemare et al., 2001; Harik-Khan et al., 2001). The inability to calculate the volume of the upper respiratory tract during the calculation process of AI-3DCTVol possibly led to the small measurement value obtained by AI-3DCTVol. Published sources have indicated that the irregular upper respiratory tract structure often makes it difficult for 3D reconstruction to accurately identify and measure this volume (Sun et al., 2006). The estimated volume of the upper respiratory tract is approximately 300 mL, which is a difference of about 100 mL compared to the difference of 170 mL observed between AI-3DCTVol and aTLV in the results of this study (Blair and Hickam, 1955). It is also possible that this difference is related to segmentation errors when identifying the lung contour boundary using the AI-3D model (Tanaka et al., 2022). Compared to plethysmography examination, it is more difficult to clearly determine the achievement of maximal inspiratory capacity during CT imaging, despite CT technicians instructing subjects to take a full inhalation. Therefore, the team will continue to reduce the occurrence of overfitting phenomena and enhance the modeling accuracy of the AI-3D system. Moreover, compared to the pTLV formula, the intra-group correlation analysis revealed a stronger correlation between the AI-3D measurement and the aTLV benchmark. To further validate these conclusions, an error analysis was conducted and showed that the AI Iterative reconstruction achieved an error control of approximately 5% compared to the approximately 10% error using pTLV, and further demonstrated the improved accuracy in lung volume calculations completed by the AI-3D reconstruction system.
In fact, with the advancement of CT technology, there have been several studies that have applied CT scans to measure lung volumes (Konheim et al., 2016; Yamada et al., 2022). However, the AI-3D method exhibits favorable advantages. AI-based lung segmentation utilizes deep learning technologies to identify lung tissue using a large volume of training data. The AI segmentation logic for lungs extends beyond CT values obtained in the traditional method with more sensitivity, accuracy, and consistency. Traditional CT-based volume calculation is based on algorithms similar to integration, whereby the lung segmentation area of each CT layer is multiplied by the number of layers and layer thickness, potentially leading to significant errors. In contrast, the AI-3D method takes the two-dimensional segmentation and generates a three-dimensional lung model, which provides a higher level of simulation and more vivid lung representation, consequently presenting a more precise lung volume. Such advantages in accurately measuring lung volume possess potential in the matching process of lung volume for lung transplantation donors and recipients.
Lung transplantation is the most effective treatment for end-stage lung disease, aiming to improve patients’ quality of life and extend their survival (Barnard et al., 2013). Matching the donor and recipient size in lung transplantation is essential for the preoperative evaluation of the procedure, as the donor lung must fit within the recipient’s chest cavity. Previous research has shown that improper size matching can lead to surgical complications, primary graft dysfunction (PGD), higher mortality rates, and chronic lung allograft dysfunction (CLAD) (Ganapathi et al., 2017; Li et al., 2021; Montoya et al., 2021). A fast and accurate size matching between the donor’s lung and the recipient’s thoracic cavity can drastically improve the efficiency of the organ transplantation process and prevent the wastage of medical resources.
This study selected healthy individuals as research subjects to verify the accuracy of the AI-3D method to examine the potential application in lung transplantation. The AI-3D technology reconstructed a detailed view of the lungs, which is critical in diagnosing and treating various pulmonary conditions, reducing the need for additional testing or invasive procedures, and ultimately leading to improved treatment planning and patient outcomes. The AI-based reconstruction nature allows patients with severe lung diseases and brain death to accurately calculate their lung volume, which makes the AI-3D method suitable for situations like lung transplantation.
Although this study has possessed certain advantages, it also has several limitations. This study is a single-center study, which lacks data support from multiple clinical institutes. Additionally, the sample size is relatively small and requires an increased sample size for further research validation. Therefore, conducting research using a larger sample size and focusing on the practical application of lung transplantation patients is necessary.
Conclusion
The AI-3D reconstruction as a convenient method, has significant potential for application in lung transplantation.
Data availability statement
The original contributions presented in the study are included in the article/Supplementary Material, further inquiries can be directed to the corresponding authors.
Ethics statement
The studies involving humans were approved by the First Hospital of Jilin University. The studies were conducted in accordance with the local legislation and institutional requirements. The participants provided their written informed consent to participate in this study.
Author contributions
WR and SY contributed to the concept of the study, performed the experiment and data analysis, and wrote the manuscript. LT, CY, LY, and YY contributed significantly to the analysis and research preparation; MX and QD helped perform the data analysis and offered constructive advice. TZ and ZY helped with the manuscript revision. All authors contributed to the article and approved the submitted version.
Funding
Application of 3D Simulation Technology in Diagnosis and Treatment of Thoracic Diseases 20130604050TC. Science and Technology Department of Jilin Province. Research on Artificial Intelligence (AI) for Predicting Benign and Malignant Pulmonary Nodules Based on Machine Deep Learning. 20210204123YY, Science and Technology Department of Jilin Province. National Nature Science Foundation of China 82002429.
Acknowledgments
We would like to express our gratitude to InferVision and engineer Wu Fule for their invaluable technical support in conducting this study, and Mingzhou (Noa) Han for their contribution to the revision process.
Conflict of interest
The authors declare that the research was conducted in the absence of any commercial or financial relationships that could be construed as a potential conflict of interest.
Publisher’s note
All claims expressed in this article are solely those of the authors and do not necessarily represent those of their affiliated organizations, or those of the publisher, the editors and the reviewers. Any product that may be evaluated in this article, or claim that may be made by its manufacturer, is not guaranteed or endorsed by the publisher.
References
Association, CMARD (2015). Guidelines for pulmonary function testing - volume tracing method for lung volume and airway resistance testing. Chin. J. Tuberc. Respir. Dis. 38 (5), 342–347.
Barmpoutis P., Waddingham W., Yuan J., Ross C., Kayhanian H., Stathaki T., et al. (2022). A digital pathology workflow for the segmentation and classification of gastric glands: study of gastric atrophy and intestinal metaplasia cases. PLoS One 17, e0275232. doi:10.1371/journal.pone.0275232
Barnard J. B., Davies O., Curry P., Catarino P., Dunning J., Jenkins D., et al. (2013). Size matching in lung transplantation: an evidence-based review. J. Heart Lung Transpl. 32, 849–860. doi:10.1016/j.healun.2013.07.002
Bellemare J. F., Cordeau M. P., Leblanc P., Bellemare F. (2001). Thoracic dimensions at maximum lung inflation in normal subjects and in patients with obstructive and restrictive lung diseases. Chest 119, 376–386. doi:10.1378/chest.119.2.376
Blair E., Hickam J. B. (1955). The effect of change in body position on lung volume and intrapulmonary gas mixing in normal subjects. J. Clin. Invest. 34, 383–389. doi:10.1172/JCI103086
Cai H., Wang R., Li Y., Yang X., Cui Y. (2018). Role of 3d reconstruction in the evaluation of patients with lower segment oesophageal cancer. J. Thorac. Dis. 10, 3940–3947. doi:10.21037/jtd.2018.06.119
Chambers D. C., Cherikh W. S., Goldfarb S. B., Hayes D., Kucheryavaya A. Y., Toll A. E., et al. (2018). The international thoracic organ transplant registry of the international society for heart and lung transplantation: thirty-fifth adult lung and heart-lung transplant report-2018; focus theme: multiorgan transplantation. J. Heart Lung Transpl. 37, 1169–1183. doi:10.1016/j.healun.2018.07.020
Chan E. G., Landreneau J. R., Schuchert M. J., Odell D. D., Gu S., Pu J., et al. (2015). Preoperative (3-dimensional) computed tomography lung reconstruction before anatomic segmentectomy or lobectomy for stage i non-small cell lung cancer. J. Thorac. Cardiovasc Surg. 150, 523–528. doi:10.1016/j.jtcvs.2015.06.051
Clayton N. (2007). Lung function made easy: assessing lung size. Chron. Respir. Dis. 4, 151–157. doi:10.1177/1479972307081647
Dubois A. B., Botelho S. Y., Bedell G. N., Marshall R., Comroe J. H. (1956). A rapid plethysmographic method for measuring thoracic gas volume: a comparison with a nitrogen washout method for measuring functional residual capacity in normal subjects. J. Clin. Invest. 35, 322–326. doi:10.1172/JCI103281
Eberlein M., Permutt S., Chahla M. F., Bolukbas S., Nathan S. D., Shlobin O. A., et al. (2012a). Lung size mismatch in bilateral lung transplantation is associated with allograft function and bronchiolitis obliterans syndrome. Chest 141, 451–460. doi:10.1378/chest.11-0767
Eberlein M., Reed R. M., Bolukbas S., Diamond J. M., Wille K. M., Orens J. B., et al. (2015). Lung size mismatch and primary graft dysfunction after bilateral lung transplantation. J. Heart Lung Transpl. 34, 233–240. doi:10.1016/j.healun.2014.09.030
Eberlein M., Reed R. M., Permutt S., Chahla M. F., Bolukbas S., Nathan S. D., et al. (2012b). Parameters of donor-recipient size mismatch and survival after bilateral lung transplantation. J. Heart Lung Transpl. 31, 1207–1213. doi:10.1016/j.healun.2011.07.015
Elmaleh-Sachs A., Balte P., Oelsner E. C., Allen N. B., Baugh A., Bertoni A. G., et al. (2022). Race/ethnicity, spirometry reference equations, and prediction of incident clinical events: the multi-ethnic study of atherosclerosis (mesa) lung study. Am. J. Respir. Crit. Care Med. 205, 700–710. doi:10.1164/rccm.202107-1612OC
Ganapathi A. M., Mulvihill M. S., Englum B. R., Speicher P. J., Gulack B. C., Osho A. A., et al. (2017). Transplant size mismatch in restrictive lung disease. Transpl. Int. 30, 378–387. doi:10.1111/tri.12913
Goldman H. I., Becklake M. R. (1959). Respiratory function tests; normal values at median altitudes and the prediction of normal results. Am. Rev. Tuberc. 79, 457–467. doi:10.1164/artpd.1959.79.4.457
Harik-Khan R. I., Fleg J. L., Muller D. C., Wise R. A. (2001). The effect of anthropometric and socioeconomic factors on the racial difference in lung function. Am. J. Respir. Crit. Care Med. 164, 1647–1654. doi:10.1164/ajrccm.164.9.2106075
Kojima K., Kato K., Oto T., Mitsuhashi T., Shinya T., Sei T., et al. (2011). Preoperative graft volume assessment with 3d-ct volumetry in living-donor lobar lung transplantations. Acta Med. Okayama 65, 265–268. doi:10.18926/AMO/46852
Konheim J. A., Kon Z. N., Pasrija C., Luo Q., Sanchez P. G., Garcia J. P., et al. (2016). Predictive equations for lung volumes from computed tomography for size matching in pulmonary transplantation. J. Thorac. Cardiovasc Surg. 151, 1163–1169. doi:10.1016/j.jtcvs.2015.10.051
Li D., Weinkauf J., Hirji A., Nagendran J., Kapasi A., Lien D., et al. (2021). Chest x-ray sizing for lung transplants reflects pulmonary diagnosis and body composition and is associated with primary graft dysfunction risk. Transplantation 105, 382–389. doi:10.1097/TP.0000000000003238
Ma X., Lu T., Qin D., Cai H., Tang Z., Yang Y., et al. (2023). Analysis of pulmonary artery variation based on 3d reconstruction of ct angiography. Front. physiology 14, 1156513. doi:10.3389/fphys.2023.1156513
McCormack M. C., Balasubramanian A., Matsui E. C., Peng R. D., Wise R. A., Keet C. A. (2022). Race, lung function, and long-term mortality in the national health and nutrition examination survey iii. Am. J. Respir. Crit. Care Med. 205, 723–724. doi:10.1164/rccm.202104-0822LE
Milner A. D. (2000). Lung volume measurements in childhood. Paediatr. Respir. Rev. 1 (2), 135–140. doi:10.1053/prrv.2000.0039
Montoya P., Bello I., Ascanio F., Romero L., Pérez J., Rosado J., et al. (2021). Graft reduction surgery is associated with poorer outcome after lung transplantation: a single-centre propensity score-matched analysis. Eur. J. Cardiothorac. Surg. 60, 1308–1315. doi:10.1093/ejcts/ezab234
Quanjer P. H. (1983). Standardised lung function testing. Summary equations of reference values. Bull. Eur. Physiopathol. Respir. 19, 45–51.
Rahaman M. M., Li C., Yao Y., Kulwa F., Rahman M. A., Wang Q., et al. (2020). Identification of covid-19 samples from chest x-ray images using deep learning: a comparison of transfer learning approaches. J. X-ray Sci. Technol. 28, 821–839. doi:10.3233/XST-200715
Stocks J., Quanjer P. H. (1995). Reference values for residual volume, functional residual capacity and total lung capacity. Ats workshop on lung volume measurements Official statement of the european respiratory society. Eur. Respir. J. 8, 492–506. doi:10.1183/09031936.95.08030492
Sun X., Zhang H., Duan H. (2006). 3d computerized segmentation of lung volume with computed tomography. Acad. Radiol. 13, 670–677. doi:10.1016/j.acra.2006.02.039
Tanaka Y., Suzuki Y., Hasegawa H., Yokomura K., Fukada A., Inoue Y., et al. (2022). Standardised 3d-ct lung volumes for patients with idiopathic pulmonary fibrosis. Respir. Res. 23, 142. doi:10.1186/s12931-022-02062-1
Valapour M., Skeans M. A., Heubner B. M., Smith J. M., Hertz M. I., Edwards L. B., et al. (2015). Optn/srtr 2013 annual data report: lung. Am. J. Transpl. 15 (2), 1–28. doi:10.1111/ajt.13200
Wang S., Ruan Z., Zhang J., Jin W. (2011). The value of pulmonary contusion volume measurement with three-dimensional computed tomography in predicting acute respiratory distress syndrome development. Ann. Thorac. Surg. 92, 1977–1983. doi:10.1016/j.athoracsur.2011.05.020
Weill D. (2016). Access to lung transplantation. The long and short of it. Am. J. Respir. Crit. Care Med. 193, 605–606. doi:10.1164/rccm.201511-2257ED
Wolf G. K., Arnold J. H. (2005). Noninvasive assessment of lung volume: respiratory inductance plethysmography and electrical impedance tomography. Crit. care Med. 33, S163–S169. doi:10.1097/01.ccm.0000155917.39056.97
Xu H., Zhao H., Jin J., Geng J., Sun C., Wang D., et al. (2023). An atlas of anatomical variants of subsegmental pulmonary arteries and recognition error analysis. Front. Oncol. 13, 1127138. doi:10.3389/fonc.2023.1127138
Yamada Y., Chubachi S., Yamada M., Yokoyama Y., Tanabe A., Matsuoka S., et al. (2022). Comparison of lung, lobe, and airway volumes between supine and upright computed tomography and their correlation with pulmonary function test in patients with chronic obstructive pulmonary disease. Respiration 101, 1110–1120. doi:10.1159/000527067
Keywords: lung volume calculation, AI-3D reconstruction, ICC, donor-recipient lung volume matching, lung transplantation
Citation: Rui W, Yuhang S, Yang L, Yue Y, Ze T, Yujie Z, Xiaochao M, Da Q, Youbin C and Tianyu L (2023) A new method for evaluating lung volume: AI-3D reconstruction. Front. Physiol. 14:1217411. doi: 10.3389/fphys.2023.1217411
Received: 05 May 2023; Accepted: 07 August 2023;
Published: 14 September 2023.
Edited by:
An-Kwok Ian Wong, Duke University, United StatesReviewed by:
Peter Lee, Brown University, United StatesChen Li, Northeastern University, China
Qing Geng, Renmin Hospital of Wuhan University, China
Yuh-Chin Huang, Duke University, United States
Copyright © 2023 Rui, Yuhang, Yang, Yue, Ze, Yujie, Xiaochao, Da, Youbin and Tianyu. This is an open-access article distributed under the terms of the Creative Commons Attribution License (CC BY). The use, distribution or reproduction in other forums is permitted, provided the original author(s) and the copyright owner(s) are credited and that the original publication in this journal is cited, in accordance with accepted academic practice. No use, distribution or reproduction is permitted which does not comply with these terms.
*Correspondence: Cui Youbin, cuiyb@jlu.edu.cn; Lu Tianyu, luty17@mails.jlu.edu.cn