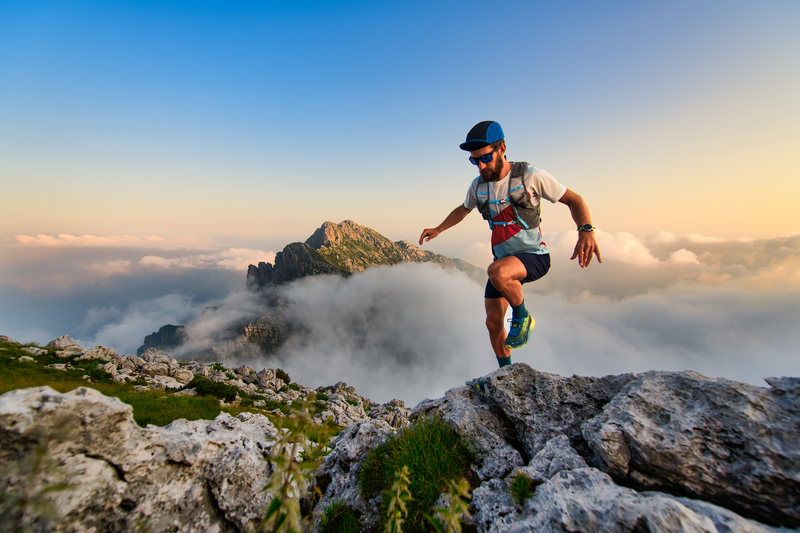
95% of researchers rate our articles as excellent or good
Learn more about the work of our research integrity team to safeguard the quality of each article we publish.
Find out more
ORIGINAL RESEARCH article
Front. Physiol. , 15 May 2023
Sec. Exercise Physiology
Volume 14 - 2023 | https://doi.org/10.3389/fphys.2023.1193501
Introduction: The fluctuation of external match load throughout a season is influenced by several contextual factors. While some, have been deeply analysed in men’s football literature, information is lacking on how other contextual elements, such as player’s age or experience, may affect the match-to-match variability of locomotor activities. In fact, aging has been described as a multifactorial process with the potential to affect human performance. The aim of this study is to assess if the variability of match locomotor performances fluctuates according to the players’ age.
Methods: 59 female players from four top-level clubs were divided into three age groups and monitored during two seasons using GPS APEX (STATSports, Northern Ireland), with a sampling frequency of 10Hz, in 150 official matches to determine the coefficient of variation (CV) of full-match and 1-min peak locomotor demands of total distance (TD), high-speed running distance, sprint distance (SpD), accelerations, and decelerations. To test whether there was a group effect of age on match-to-match variability we used a one-way ANOVA with CV% as the independent variable.
Results: CV values of full match variables ranged from 3.8% to 27.8%, with total distance (3.8%) in the peak age group and SpD (27.8%) in the pre-peak age group. Similarly, CV values of 1-min peaks ranged from 4.1% (post-peak group) in TD to 22.3% (peak group) in SpD.
Discussion: The main finding was that there were no significant differences between the different age groups in the metrics analysed although trends indicate less variability in the post-peak age group.
The daily monitoring of football players has become common practice in professional and semi-professional environments (Al Haddad et al., 2018). Researchers have used quantitative data captured to provide practitioners with novel insights into training (Akenhead et al., 2016) and match demands (Baptista et al., 2019). During the past decade, evidence-based insight concerning the players’ locomotor activity during competition has substantially increased, not only due to the rule change regarding the use of wearable technologies during official matches (FIFA, 2015), but also as a consequence of “new” approaches and perspectives (Kirkendall, 2020; Teixeira et al., 2021).
One hot topic is the degrees of variability associated with the players’ physical performances across consecutive matches. Recent studies have revealed the importance of analysing the match-to-match variability of locomotor activities (expressed by the coefficient of variation (CV) of a particular measurement) by presenting its practical utility (Oliva-Lozano et al., 2021; Winther et al., 2022). The fluctuation of external match load throughout a season should be considered part of the nature of team sports (Baptista et al., 2022), and is influenced by many contextual factors. While some of these factors, such as playing position (Bush et al., 2015), seasonal period (Mohr et al., 2003), ball possession (da Mota et al., 2016), opposition (Rampinini et al., 2007), match location (Castellano et al., 2011), and playing style (Oliva-Lozano et al., 2021) have been deeply analysed in the literature in men’s football, information is lacking on how other contextual elements (e.g., player’s experience) may affect the match-to-match variability of locomotor activities especially in women´s football.
Players’ experience may be noted in several match-related behaviors, such as tactical decisions (Buchheit et al., 2010) or even pacing strategies (Lorenzo-Martínez et al., 2020). If team sports players pace their efforts, then it should be expected that more experienced players may have a better knowledge on how their body responds to the match demands and present more stable performances both within and between matches. However, previous findings in football populations showed divergent conclusions (Castagna et al., 2005; Lorenzo-Martínez et al., 2020), suggesting other factors associated with age (e.g., fitness level, the recovery process, etc.) may also affect match-to-match variability.
Despite not being the same as experience per se, aging has been described as a multifactorial process with the potential to affect human performance (Bruce, 1984; Castagna et al., 2005). This idea is corroborated in recent studies, where players over 30 years of age presented significantly lower total distance, high-speed running, and sprint distance covered than younger male players (Rey et al., 2019; Sal de Rellán-Guerra et al., 2019). Such a finding may be influenced by physical capacity. For example, there is a correlation between the Yo-YoIR1 test and high-intensity running (>18 km h−1) (Krustrup et al., 2005), and further, a u-shaped relationship exists between age and the Yo-YoIR1 test in female players (Datson et al., 2022). Furthermore, according to Hauer et al. (2021), older male players might be considered more experienced and tend to play a more tactically demanding game, thus preserving part of their physical capacities. The age range (∼18–38 years of age) of players in professional football teams is considerable (Dendir, 2016), which means differences in fitness level, tactical knowledge, and self-regulated performance are expected to appear within a team. To the best of our knowledge, the only study investigating the age- or experience-related variability of locomotor activities during match-play in professional football players is done by Lorenzo-Martínez et al. (2020). The authors studied Spanish male football teams and observed that younger players (<25.2 years of age) presented lower CVs for high-speed activities compared to older players (>33.1 years of age).
However, as previously reported for weightlifters (McGuigan and Kane, 2004) and cyclists (Paton and Hopkins, 2006), it seems that female athletes present higher CVs than men. Despite playing the same game, reference values for men’s football should not be used for decision-making in women’s football, thus requiring further investigation within this field with female players. Moreover, as several sources of bias are expected when a single team is analysed or when a small sample size is used, a multiple-team analysis is warranted (Gregson et al., 2010). Therefore, due to the lack of evidence in team sports in general and in women’s football in particular, the aim of this study is to assess if the variability of match locomotor performances (full match and peak demands) expresses different magnitudes according to the players’ age. We hypothesize that younger players present a minor/less pronounced variability than older players.
With ethical institutional approval from the Norwegian Centre for Research Data (reference number: 296155) and written informed consent from the participants, physical performances of 59 players from 150 official matches during two entire seasons (2020 and 2021) in four top level clubs were collected using GPS APEX (Statsports, Northern Ireland), with a sampling frequency of 10 Hz. The validity and accuracy levels (bias <5%) of this tracking system have been previously reported (Beato et al., 2018). Each player used the same GPS unit throughout the season to minimize inter-device error (Beato et al., 2018). Goalkeepers were excluded from analysis, and the playing positions (central defenders, full-backs, central midfielders, wide midfielders, and forwards) were classified according to previous research (Baptista et al., 2022). Match observations under 90 min and observations where players did not play in their main (modal) position were also excluded from the analysis. In addition, only players with three or more match observations were included in the sample. Next, we calculated the coefficient of variation (CV) and mean age for each player before dividing the players into three groups: pre-peak age (<23 years old), peak age (23–27 years old), and post-peak age (>27 years old) (Table 1). Given that peak age studies in women’s football are almost non-existent we based the peak age dichotomization on 1) the study by Barreira et al. (2021) reporting a peak age of approximately 25 years old, and 2) studies on male elite players showing peak age band between 25–27 years old (Dendir, 2016). We also felt extending this age band to include ages 23–25 years was appropriate due to the league being comparatively young in comparison to the men’s league. The final sample characteristics are shown in Table 1. The STROBE cohort reporting guidelines were used according to the recommendations for epidemiological observational studies (von Elm et al., 2014).
The physical parameters analysed included: total distance (TD), high-speed running distance (HSRD (>4.44 m s-1), sprint distance (SpD) (>5.55 m s-1), and the number of accelerations and decelerations (Acc/Dec). The speed thresholds were chosen according to previous research (Strauss et al., 2019; Baptista et al., 2022). Accelerations and decelerations were defined as a positive or negative change in speed of more than ±2.26 m s-2, with a minimal effort duration of 0.3 s, finishing when the rate of acceleration/deceleration reached 0 m s-2. All variables were used to analyse full match (absolute values) and peak locomotor demands (1-min rolling analysis period). The epoch length of 1 min to analyse the peak locomotor demands was chosen to find the highest relative intensities in accordance with previous research (Doncaster et al., 2020).
Doppler-derived speed data was exported from manufacturer software (StatSport Sonra 2.1.4), and we used Python 3.9.12 for processing (linearly interpolating any missing raw data) and to derive metrics. Raw acceleration was then calculated over 0.6 s. After deriving all the metrics, the data was transferred to R (R.4.1.3, R Core Team, 2022) for statistical analysis. To test whether there was a group effect of age on match-to-match variability we used a one-way ANOVA with CV% as the independent variable. If a significant effect was found (p ≤ 0.05), then pair-wise comparisons were done using Tukey’s Honestly Significant Difference.
Descriptive statistics of full-match and peak period demands of each playing position from the different age groups are presented in Table 2. Across the three playing positions (CD, WD and CM) represented in all age groups, the higher full match values observed in TD, HSRD, SpD, and Acc were from the players in the post-peak age group, with the lowest being observed in the pre-peak age group. When analysing the 1-min peak periods, the CD with the highest TD, HSRD, and SpD were from the peak age group, while for CM the highest values of these metrics were found in the post-peak age group.
TABLE 2. Means, standard deviation and coefficient of variation per playing position in the different age groups.
The variability of full match and 1-min peak locomotor demands per age group is presented in Table 3. All estimates of match-to-match variability from the three age groups analysed (pre-peak, peak, and post-peak) are expressed in percentage (CV) units. CV values of full match variables ranged from 3.8% to 27.8%, with the lowest CVs appearing in the TD of the peak age group (3.8%) and the highest in the SpD of the pre-peak age group (27.8%). Metrics of 1-min peaks presented similar values with the CV ranging from 4.1% (post-peak group) in TD to 22.3% (peak group) in SpD. For every age group analysed, the metrics with the lowest and the highest degrees of variability were TD and SpD, respectively. Post-peak age group presented the lowest full match CVs for HSRD (13.3%), SpD (22.1%), and Acc (12.6), while the pre-peak age group presented the highest full match CVs for TD (4.2%), SpD (27.8%), and Dec (13.8%). Conversely, the highest 1-min peak CV for TD (5.0%), HSRD (14.0%), SpD (22.3%), and Dec (13.6%) were observed in the peak age group. The only metric where the highest CV was observed in the post-peak age group was the 1-min peak Acc (14.4%), with all the others presenting their highest values both in the pre-peak or in the peak age groups. However, no significant differences were found in any metric analysed between the different age groups.
TABLE 3. Match-to-match variability of full match and 1-min peak demands expressed as coefficients of variation (%).
This study is the first attempting to analyse potential influence of age on the match-to-match variability of physical performances in elite women´s football. Although presenting trends indicating less variability in the post-peak age group, the main finding was that there were no significant differences between the different age groups in the metrics analysed. Causality of performance fluctuations related to age may have some intricate aspects that are worth reflecting over. One aspect is how player experience impacts his/her own energy expenditure over a game, e.g., pacing strategies based on experience spreading the expected load over 90 min (Paul et al., 2015). Another aspect may be related with the coaches’ strategies, where style of play and selection of players for more demanding positions might take the age factor into account. However, this hypothesis was not confirmed in the present study, with the results revealing no significant differences between the age groups in any of the analysed parameters. Our results suggest that, either age has a small impact in the variability of match physical performance, or that other contextual factors may have a higher influence, masking eventual differences. Opponent’s level, for instance, is one of these contextual factors that might have influenced the outcomes of the study (Rampinini et al., 2007), since we did not control if there were discrepancies in the level of the opponents across age groups. Furthermore, the non-significant differences observed between age groups can also be a consequence of the dynamic and stochastic nature of football matches, which makes it hard to predict or to establish a rationale behind the players’ physical performances (Baptista et al., 2022).
Surprisingly, when analysing the differences in match physical performance between the different age groups, we observed that the highest values in the various parameters were performed by the post-peak age group. This evidence contrasts with previous research on male players where more experienced players performed significantly lower TD, HSRD, and SpD than less experienced players (Rey et al., 2019; Sal de Rellán-Guerra et al., 2019). While in specific playing positions (i.e., CM) these results may be biased by the considerably lower number of subjects allocated in the post-peak age group (3) than in the pre-peak (13) and peak (9) age groups, in the other playing positions (CD and WD) such differences in the sample size were not presented. Another possible explanation, for the above-mentioned differences between studies, is that there might be a sex difference in the variability of match physical performance between the sexes. On the other hand, it seems to exist a higher heterogeneity in the 1-min peak variables, where no trend was observed across age group performances. Altogether, these results might reveal that, in women’s football, age is not a limiting factor for match performance and that older players can equally cope with the peak match demands as the younger players do.
In order to increase data heterogeneity and diminish the risk of bias, we followed the suggestion of previous research (Al Haddad et al., 2018; Oliva-Lozano et al., 2021) for the necessity to conduct multi-club studies, by including four different top-level teams. However, the peak age calculation and the age group distribution resulted in different number of players per playing position and an unequal number of playing positions per age group. This may be seen as a limitation of the study since previous studies have reported different CVs across positions (Gregson et al., 2010; Bush et al., 2015; Carling et al., 2016; Al Haddad et al., 2018). For instance, the pre-peak age group was represented only by players from three playing positions (CD, WD and CM), while WM and FW were only included in the peak and post-peak age group, respectively.
Furthermore, in the present study only univariate peak locomotor demands were considered in the analysis of the peak periods’ variability, which may have influenced the results obtained. Further research within this field should attempt to include multivariate peak periods in their analyses. Moreover, a direct comparison with male football should be interpreted with caution since peak age cut-offs of female and male football players are expected to be different. Finally, one of the challenges in research with female top-level football clubs is the size of the samples available. Therefore, a statistical type-2 error should not completely be ruled out.
Differences in the match-to-match variability of physical performances between age groups in women´s elite football are probably small. Although no significant differences were revealed in the present study, the influence of age in the players’ ability to maintain stable physical performance across matches seems to be logical and reasonable and should be deeper studied in further research, where the study limitations here presented may be considered in study designs. Despite an eventual influence on the players’ capability to pace their energy expenditure during and across matches, age seems to not be a limiting factor for peak match performance.
The raw data supporting the conclusions of this article will be made available by the authors, without undue reservation.
The studies involving human participants were reviewed and approved by Norwegian Centre for Research Data. The patients/participants provided their written informed consent to participate in this study.
Conceptualization, IB, AW, DJ and SAP; Data curation, AW; Formal analysis, AW; Investigation, IB and AW; Methodology, IB, AW, SP, DJ, and SAP; Project administration, SAP; Supervision, DJ, and SAP; Writing–original draft, IB; Writing–review and editing, AW, SP, DJ, and SAP. All authors have read and agreed to the published version of the manuscript. All authors listed have made a substantial, direct, and intellectual contribution to the work and approved it for publication. All authors contribution to the article and approved the submitted version.
This research was funded by Tromsø Research Foundation with the following grant number: 19_FFC_SAP.
The authors would like to thank Astrid Grøttå Ree, Helene Davidsen Moa, Thomas Lyngbø, Mads Pettersen, Elias Koppen, and Atle Ingebrigtsen for their help in the data collection.
The authors declare that the research was conducted in the absence of any commercial or financial relationships that could be construed as a potential conflict of interest.
All claims expressed in this article are solely those of the authors and do not necessarily represent those of their affiliated organizations, or those of the publisher, the editors and the reviewers. Any product that may be evaluated in this article, or claim that may be made by its manufacturer, is not guaranteed or endorsed by the publisher.
Akenhead, R., Harley, J. A., and Tweddle, S. P. (2016). Examining the external training load of an English premier league football team with special reference to acceleration. J. Strength Cond. Res. 30 (9), 2424–2432. doi:10.1519/jsc.0000000000001343
Al Haddad, H., Méndez-Villanueva, A., Torreño, N., Munguía-Izquierdo, D., and Suárez-Arrones, L. (2018). Variability of GPS-derived running performance during official matches in elite professional soccer players. J. Sports Med. Phys. Fit. 58 (10), 1439–1445. doi:10.23736/s0022-4707.17.07500-4
Baptista, I., Johansen, D., Figueiredo, P., Rebelo, A., and Pettersen, S. A. (2019). A comparison of match-physical demands between different tactical systems: 1-4-5-1 vs 1-3-5-2. PLOS ONE 14 (4), e0214952. doi:10.1371/journal.pone.0214952
Baptista, I., Winther, A. K., Johansen, D., Randers, M. B., Pedersen, S., and Pettersen, S. A. (2022). The variability of physical match demands in elite women's football. Sci. Med. Footb. 6, 559–565. doi:10.1080/24733938.2022.2027999
Barreira, J., Bueno, B., and Chiminazzo, J. G. C. (2021). Relative age effect and age of peak performance: An analysis of women's football players in the olympic games (1996-2016). Motriz: Revista de Educação Física.
Beato, M., Coratella, G., Stiff, A., and Iacono, A. D. (2018). The validity and between-unit variability of GNSS units (STATSports apex 10 and 18 Hz) for measuring distance and peak speed in team sports. Front. Physiol. 9, 1288. doi:10.3389/fphys.2018.01288
Bruce, R. A. (1984). Exercise, functional aerobic capacity, and aging--another viewpoint. Med. Sci. Sports Exerc 16 (1), 8–13. doi:10.1249/00005768-198401000-00004
Buchheit, M., Mendez-Villanueva, A., Simpson, B. M., and Bourdon, P. C. (2010). Match running performance and fitness in youth soccer. Int. J. Sports Med. 31 (11), 818–825. doi:10.1055/s-0030-1262838
Bush, M. D., Archer, D. T., Hogg, R., and Bradley, P. S. (2015). Factors influencing physical and technical variability in the English Premier League. Int. J. Sports Physiol. Perform. 10 (7), 865–872. doi:10.1123/ijspp.2014-0484
Carling, C., Bradley, P., McCall, A., and Dupont, G. (2016). Match-to-match variability in high-speed running activity in a professional soccer team. J. Sports Sci. 34 (24), 2215–2223. doi:10.1080/02640414.2016.1176228
Castagna, C., Abt, G., D'Ottavio, S., and Weston, M. (2005). Age-related effects on fitness performance in elite-level soccer referees. J. Strength Cond. Res. 19 (4), 785–790. doi:10.1519/r-14984.1
Castellano, J., Blanco-Villasenor, A., and Alvarez, D. (2011). Contextual variables and time-motion analysis in soccer. Int. J. Sports Med. 32 (6), 415–421. doi:10.1055/s-0031-1271771
da Mota, G. R., Thiengo, C. R., Gimenes, S. V., and Bradley, P. S. (2016). The effects of ball possession status on physical and technical indicators during the 2014 FIFA World Cup Finals. J. Sports Sci. 34 (6), 493–500. doi:10.1080/02640414.2015.1114660
Datson, N., Weston, M., Drust, B., Atkinson, G., Lolli, L., and Gregson, W. (2022). Reference values for performance test outcomes relevant to English female soccer players. Sci. Med. Footb. 6, 589–596. doi:10.1080/24733938.2022.2037156
Dendir, S. (2016). When do soccer players peak? A note. J. Sports Anal. 2, 89–105. doi:10.3233/JSA-160021
Doncaster, G., Page, R., White, P., Svenson, R., and Twist, C. (2020). Analysis of physical demands during youth soccer match-play: Considerations of sampling method and epoch length. Res. Q. Exerc Sport 91 (2), 326–334. doi:10.1080/02701367.2019.1669766
FIFA (2015). FIFA and IFAB to develop global standard for electronic performance and tracking systems. Retrieved from: https://www.fifa.com/who-we-are/news/fifa-and-ifab-to-develop-global-standard-for-electronic-performance-an-2709918.
Gregson, W., Drust, B., Atkinson, G., and Salvo, V. D. (2010). Match-to-match variability of high-speed activities in premier league soccer. Int. J. Sports Med. 31 (4), 237–242. doi:10.1055/s-0030-1247546
Hauer, R., Störchle, P., Karsten, B., Tschan, H., and Baca, A. (2021). Internal, external and repeated-sprint demands in small-sided games: A comparison between bouts and age groups in elite youth soccer players. PLoS One 16 (4), e0249906. doi:10.1371/journal.pone.0249906
Kirkendall, D. T. (2020). Evolution of soccer as a research topic. Prog. Cardiovasc Dis. 63 (6), 723–729. doi:10.1016/j.pcad.2020.06.011
Krustrup, P., Mohr, M., Ellingsgaard, H., and Bangsbo, J. (2005). Physical demands during an elite female soccer game: Importance of training status. Med. Sci. Sports Exerc 37 (7), 1242–1248. doi:10.1249/01.mss.0000170062.73981.94
Lorenzo-Martínez, M., Rey, E., and Padrón-Cabo, A. (2020). The effect of age on between-match physical performance variability in professional soccer players. Res. Sports Med. 28 (3), 351–359. doi:10.1080/15438627.2019.1680985
McGuigan, M. R., and Kane, M. K. (2004). Reliability of performance of elite Olympic weightlifters. J. Strength Cond. Res. 18 (3), 650–653. doi:10.1519/12312.1
Mohr, M., Krustrup, P., and Bangsbo, J. (2003). Match performance of high-standard soccer players with special reference to development of fatigue. J. Sports Sci. 21 (7), 519–528. doi:10.1080/0264041031000071182
Oliva-Lozano, J. M., Muyor, J. M., Fortes, V., and McLaren, S. J. (2021). Decomposing the variability of match physical performance in professional soccer: Implications for monitoring individuals. Eur. J. Sport Sci. 21 (11), 1588–1596. doi:10.1080/17461391.2020.1842513
Paton, C. D., and Hopkins, W. G. (2006). Ergometer error and biological variation in power output in a performance test with three cycle ergometers. Int. J. Sports Med. 27 (6), 444–447. doi:10.1055/s-2005-865781
Paul, D. J., Bradley, P. S., and Nassis, G. P. (2015). Factors affecting match running performance of elite soccer players: Shedding some light on the complexity. Int. J. Sports Physiol. Perform. 10 (4), 516–519. doi:10.1123/ijspp.2015-0029
R Core Team (2022). R: A Language and Environment for Statistical ComputingVienna, Austria: R Foundation for Statistical Computing. Available at: https://www.R-project.org/
Rampinini, E., Coutts, A. J., Castagna, C., Sassi, R., and Impellizzeri, F. M. (2007). Variation in top level soccer match performance. Int. J. Sports Med. 28 (12), 1018–1024. doi:10.1055/s-2007-965158
Rey, E., Costa, P. B., Corredoira, F. J., and Sal de Rellán Guerra, A. (2019). Effects of age on physical match performance in professional soccer players. J. Strength Cond. Res. 35 (10), 2790–2795. doi:10.1519/jsc.0000000000003244
Sal de Rellán-Guerra, A., Rey, E., Kalén, A., and Lago-Peñas, C. (2019). Age-related physical and technical match performance changes in elite soccer players. Scand. J. Med. Sci. Sports 29 (9), 1421–1427. doi:10.1111/sms.13463
Strauss, A., Sparks, M., and Pienaar, C. (2019). The use of GPS analysis to quantify the internal and external match demands of semi-elite level female soccer players during a tournament. J. Sports Sci. Med. 18 (1), 73–81.
Teixeira, J. E., Forte, P., Ferraz, R., Leal, M., Ribeiro, J., Silva, A. J., et al. (2021). Monitoring accumulated training and match load in football: A systematic review. Int. J. Environ. Res. Public Health 18 (8), 3906. doi:10.3390/ijerph18083906
von Elm, E., Altman, D. G., Egger, M., Pocock, S. J., Gøtzsche, P. C., Vandenbroucke, J. P., et al. (2014). The strengthening the reporting of observational studies in epidemiology (STROBE) statement: Guidelines for reporting observational studies. Int. J. Surg. 12 (12), 1495–1499. doi:10.1016/j.ijsu.2014.07.013
Keywords: high-intensity actions, locomotor demands, playing position, peak periods, player experience
Citation: Baptista I, Winther AK, Pedersen S, Johansen D and Pettersen SA (2023) The influence of age on the match-to-match variability of physical performance in women’s elite football. Front. Physiol. 14:1193501. doi: 10.3389/fphys.2023.1193501
Received: 24 March 2023; Accepted: 05 May 2023;
Published: 15 May 2023.
Edited by:
Emiliano Cè, University of Milan, ItalyReviewed by:
Luís Branquinho, Higher Institute of Educational Sciences of the Douro, PortugalCopyright © 2023 Baptista, Winther, Pedersen, Johansen and Pettersen. This is an open-access article distributed under the terms of the Creative Commons Attribution License (CC BY). The use, distribution or reproduction in other forums is permitted, provided the original author(s) and the copyright owner(s) are credited and that the original publication in this journal is cited, in accordance with accepted academic practice. No use, distribution or reproduction is permitted which does not comply with these terms.
*Correspondence: Ivan Baptista, aXZhbnRtQGZhZGUudXAucHQ=
Disclaimer: All claims expressed in this article are solely those of the authors and do not necessarily represent those of their affiliated organizations, or those of the publisher, the editors and the reviewers. Any product that may be evaluated in this article or claim that may be made by its manufacturer is not guaranteed or endorsed by the publisher.
Research integrity at Frontiers
Learn more about the work of our research integrity team to safeguard the quality of each article we publish.