- 1Institute of Medical Research, Northwestern Polytechnical University, Xi’an, China
- 2Research & Development Institute of Northwestern Polytechnical University in Shenzhen, Shenzhen, China
- 3Northwestern Polytechnical University Hospital, Xi’an, China
Objective: A growing body of research shows the promise and efficacy of EMG-based robot interventions in improving the motor function in stroke survivors. However, it is still controversial whether the effect of EMG-based robot is more effective than conventional therapies. This study focused on the effects of EMG-based robot on upper limb motor control, spasticity and activity limitation in stroke survivors compared with conventional rehabilitation techniques.
Methods: We searched electronic databases for relevant randomized controlled trials. Outcomes included Fugl-Meyer assessment scale (FMA), Modified Ashworth Scale (MAS), and activity level.
Result: Thirteen studies with 330 subjects were included. The results showed that the outcomes post intervention was significantly improved in the EMG-based robot group. Results from subgroup analyses further revealed that the efficacy of the treatment was better in patients in the subacute stage, those who received a total treatment time of less than 1000 min, and those who received EMG-based robotic therapy combined with electrical stimulation (ES).
Conclusion: The effect of EMG-based robot is superior to conventional therapies in terms of improving upper extremity motor control, spasticity and activity limitation. Further research should explore optimal parameters of EMG-based robot therapy and its long-term effects on upper limb function in post-stroke patients.
Systematic Review Registration: https://www.crd.york.ac.uk/PROSPERO/; Identifier: 387070.
1 Introduction
Stroke is a prevalent neurological dysfunction syndrome characterized by high incidence, mortality and disability rate (Winstein et al., 2016). Upper limb disorders are present in 85% of stroke survivors, with motor dysfunction still affecting 55%–75% of patients 3–6 months after onset (Parker et al., 1986; Feys et al., 1998). The residual upper limb dysfunction hugely impacts the ability of post-stroke patients to live and work independently, leading to reduced quality of life and a burden on patients’ families and society (Micera et al., 2020). Therefore, there is an urgent need to promote upper extremity function in post-stroke patients. However, the need for effective rehabilitation techniques for upper limb in stroke survivors remains largely unmet. Thus, it is of great significance to develop effective and positive rehabilitation methods for the upper limb rehabilitation of stroke survivors.
Conventional rehabilitation techniques, such as constraint-induced movement therapy (CIMT), physical therapy (PT) and occupational therapy (OT) (Pollock et al., 2014; Corbetta et al., 2015), have been adopted to assist upper limb rehabilitation. These techniques require patients to perform full or partial-assisted movements under the supervision of therapists. Other rehabilitation methods such as electrical stimulation (ES) and robots can provide repetitive, high-intensity training and also have benefit to reduce the physical stress of rehabilitation staff (Doucet et al., 2012; Zhang et al., 2017; Wang et al., 2021). However, a lack of real-time feedback from patients and excessive electrical stimulation may impede the efficacy of repetitive, high-intensity training and, in certain instances, induce muscle fatigue, thereby hindering the facilitation of motor function recovery (Chae et al., 2002).
Electromyography (EMG) have been utilized to control electrical stimulations (Hu et al., 2010; Rong et al., 2015; Rong et al., 2017; Nam et al., 2022) and powered exoskeletal devices (Rosen et al., 2001; Cheng et al., 2003; Dipietro et al., 2005; Ferris et al., 2005; Song et al., 2008) and trigger robot-assisted training to provide movement assistance (Zhuang et al., 2021). The EMG-based robot is one of the novel techniques designed for maximizing the involvement of voluntary efforts during post-stroke training. Unlike traditional robot-assisted training, the EMG-based robot can detect residual EMG signals of the affected limb in real time and integrate the participants’ voluntary motor intention represented by the EMG signal from the residual muscles into training (Song et al., 2008; Rong et al., 2015; Chen et al., 2022). Once the EMG signals reach a specific threshold, the robot-assisted training will be activated, assisting the patients to complete a desired movement. The EMG-based robot could increase the interaction between participants and machines, potentially enhancing the effect of robot-assisted training, and reducing the pressure on medical staff. Studies have found that the EMG-based robot improved the Fugl-Meyer assessment (FMA) score and spasticity of the upper extremity in post-stroke patients when compared with conventional therapy, (Stein et al., 2007; Song et al., 2008; Hu et al., 2015; Nam et al., 2017). However, Chen et al., (Chen et al., 2022), and Page et al., (Stein et al., 2007; Page et al., 2013; Page et al., 2020), respectively found the efficacy of the EMG-based robot was not superior to task-oriented training and conventional hands-on manual therapy. Therefore, it remains unclear whether the effect of the EMG-based robot is superior to conventional therapies on upper limb function of stroke survivors.
To date, there is a lack of meta-analyses summarizing whether EMG-based robot training is superior to conventional treatment. Therefore, the objectives of this meta-analysis are twofold: 1) to determine the superiority of EMG-based robot therapy over conventional therapy, and 2) to analyze the effectiveness of different treatment options.
2 Methods
This review was on the basis of the Preferred Reporting Items for Systematic Reviews and Meta-Analyses (PRISMA) (Moher et al., 2009).
2.1 Search strategy
We searched studies published before 20 November2022 in these electronic databases: Embase, Scopus, PubMed (MEDLINE), Cochrane library and Web of science. The PICOS (participant, intervention, comparison, outcome and study design) framework was used to research. When determined the systematic keywords to retrieve, we only used P and I to avoid missing crucial articles. The mesh terms used in Embase were “Cerebrovascular Accident” (Participants), “Electromyography” AND “Robotics” (Intervention), and in other databases were related to “Stroke” (Participants), “Electromyography” AND “Robot” (Intervention). Detailed search strategy for those databases could be found in Supplementary Material.
2.2 Inclusion and exclusion criteria
The following was the inclusion criteria: 1) Participants: patients who have been suffering stroke; 2) Intervention: EMG-based robot therapies, including EMG-driven robot, smart rehabilitation systems with EMG, and electromechanical orthosis; 3) Outcomes: measures of upper extremity motor function, spasticity and activity limitation; 4) Study design: randomized controlled, cross-over clinical trials; 5) articles published in English.
The following was the exclusion criteria: 1) studies not focusing on post-stroke patients; 2) no EMG-based robot system described; 3) studies targeting the low limb functions of stroke; 4) case reports; 5) review articles; 6) studies without the full text. Two reviewers assessed each article for inclusion independently. When an identity of views was not reached between the two reviewers, the ratings were discussed among all co-authors until a consensus was reached.
2.3 Outcome measures
Based on the International Classification of Functioning Disability and Health model (ICF) (Reis et al., 2021), we classified outcomes into a) motor control of upper limb, b) muscle tension, and c) activity capacity. The above three aspects were respectively evaluated by Fugl-Meyer Assessment Scale (FMA), modified Ashworth Scale (MAS) and activity limitation (e.g., Box and Blocks Test [BBT], Nine Hole Peg Test, Jebsen-Taylor Hand Function Test, Action Research Arm Test [ARAT]) (Veerbeek et al., 2017; Reis et al., 2021).
2.4 Data extraction
Two authors analyzed the abstracts and contents of each article carefully and extracted the data systematically. If there was a disagreement, it would be resolved by consultation with a third author. We extracted data on the following two aspects: 1) the basic information of the study, including the type of study, demographic characteristics of the subjects, outcomes measures, and 2) information on the EMG-based robot systems, including robot types, parameters of intervention. We contacted authors for original data when partial data were not available.
2.5 Risk of bias assessments
We used the Cochrane risk of bias assessment tool (Higgins and Altman, 2008) to assess each study by two independent authors. This tool contains six items, selection bias, performance bias, detection bias, attrition bias, reporting bias, and other biases (Higgins et al., 2011). Disagreements were resolved by consultation with a third reviewer when necessary.
2.6 Statistical analysis
Review Manager 5.4 (The Nordic Cochrane Centre, The Cochrane Collaboration, Copenhagen, Denmark) was used to analysis. We compared variation in effect sizes on the outcome measures of upper extremity function between the experimental group (EG, using EMG-based robot) and control group (CG, using conventional therapies) before and after intervention.
Then, we used mean difference (MD) or standardized mean difference (SMD) and 95% confidence intervals (CIs) to calculate the pooled effects of outcome measures. In addition, we evaluated heterogeneity by examining forest plots, chi2 test and I2 statistic were used to assess the heterogeneity between RCTs. I2 values range from 0% to 100%, and are considered low at <25%, modest at 25%–50%, and high at >50%, and the t-statistic is being used for the degrees of freedom in the random effects analysis, when the number of studies is small (e.g., <10). Subgroup analyses, meta-regression and sensitivity analysis were computed to evaluate heterogeneity.
3 Results
3.1 Study screening
Figure 1 shows the details of the whole selection process. A total of 2,469 articles were retrieved after searching the databases. And 1750 articles were screened after removing 719 duplicates, of which 1,691 articles were excluded according to the PICOS principle in titles and abstracts. The rest of 59 studies were submit to full-text checking, of which 13 studies were included for meta-analysis.
3.2 Quality of the included studies
Risk of bias for included 13 studies was assessed by two reviewers independently. The results were shown in Figure 2, and sensitive analysis indicated that the results appeared to be stable.
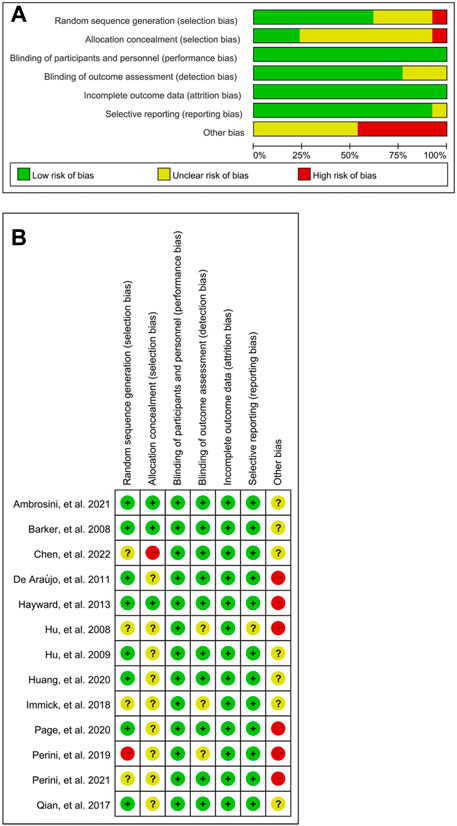
FIGURE 2. Risk of bias in the systematic review. Bias of the included articles is relatively low and stable. (A) Risk of bias for all included studies. (B) Risk of bias item for each included study.
3.3 Study characteristics
Table 1 shows the details of basic information of the included trials. In total, this meta-analysis included 330 subjects (EG, n = 175; CG, n = 155). One study was extracted two experimental groups (Page et al., 2020). For randomized cross-over trial (Chen et al., 2022), data before crossover were extracted. The study with fewest number of participants was carried out by Hayward (n = 8, EG:4, CG:4) (Hayward et al., 2013). The mean duration of post-stroke ranged from 0.87 months to 8.3 years. Most studies concentrated on stroke rehabilitation in chronic stage.
In terms of setting EMG-based robot intervention parameters, the session duration of EMG-based robot ranged from 30 min to 90 min, and the average was 47 min. Eight studies set a session less than 60 min. Three studies (Barker et al., 2008; Hayward et al., 2013; Chen et al., 2022) set a session duration of 60 min, and others were more than 60 min. The average frequency of intervention was 3.79 times/week, and most studies focused on a frequency of 3 times/week. The average intervention period was 6.04 weeks, and most studies continued 4 weeks.
According to the statistics, the effect of the intervention on participants was mainly confirmed by measuring subjects’ upper limb motor function, spasticity and activity limitation. The FMA, MAS and assessment for activity level, such as ARAT, BBT were the most commonly used scales.
3.4 Synthesis of results
3.4.1 Effect of EMG-based robot therapy compared with control group
In the subgroup analyses, the EMG-based robot, non EMG-based robot (i.e., conventional rehabilitation robot such as InMotion), and conventional (non-robotic) rehabilitation were compared for the improvement of motor control, spasticity and activity limitation post intervention. Nine studies measured the effect of EMG-based robot on FMA. The results showed that the score of FMA was significantly increased (SMD:0.62, 95% CI:0.29 to 0.95) (Figure 3A). Seven studies focused on MAS, and the results showed MAS changed significantly (MD: −0.42, 95% CI: −0.82 to −0.03) (Figure 3B). Eight studies aimed at activity limitation, the results showed the activity level also improved notably (SMD:0.43, 95% CI:0.05 to 0.82) (Figure 3C). The results of subgroup analyses showed that the effect of EMG-based robot in motor control was superior to both conventional therapies (SMD:0.46, 95% CI:0.03 to 0.89) and robotic therapies (SMD:0.94, 95% CI:0.43 to 1.45) (Figure 4A). In terms of spasticity, no obvious advantage was found for the EMG-based robot over conventional treatment (Figure 4B). The EMG-based robot was more effective than the robotic therapies in the activity limitation (SMD:0.90, 95% CI:0.02 to 1.79) (Figure 4C). However, there is high heterogeneity in the outcome of MAS and activity limitation. The results of meta-regression (Supplementary Figure A1) and sensitivity analysis (Supplementary Figure A2) showed that the sample size contributed to the outcome of MAS and the duration of disease contributed to activity limitation.
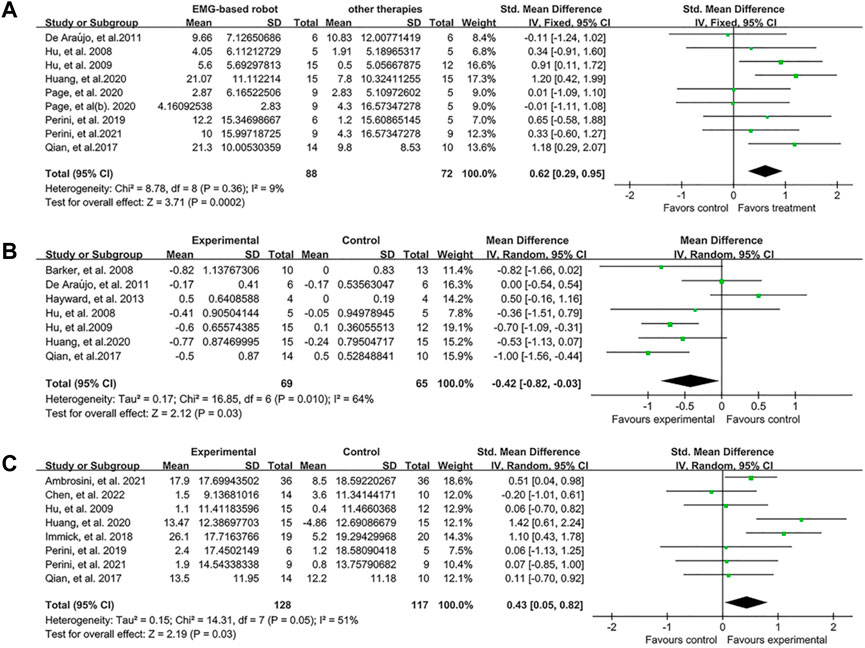
FIGURE 3. Forest plot analysis of the effect of EMG-based robot therapy vs. conventional therapies. (A) Forest plot analysis of the effect of EMG-based robot therapy vs. conventional therapies on FMA. (B) Forest plot analysis of the effect of EMG-based robot therapy vs. conventional therapies on MAS. (C) Forest plot analysis of the effect of EMG-based robot therapy vs. conventional therapies on activity limitation.
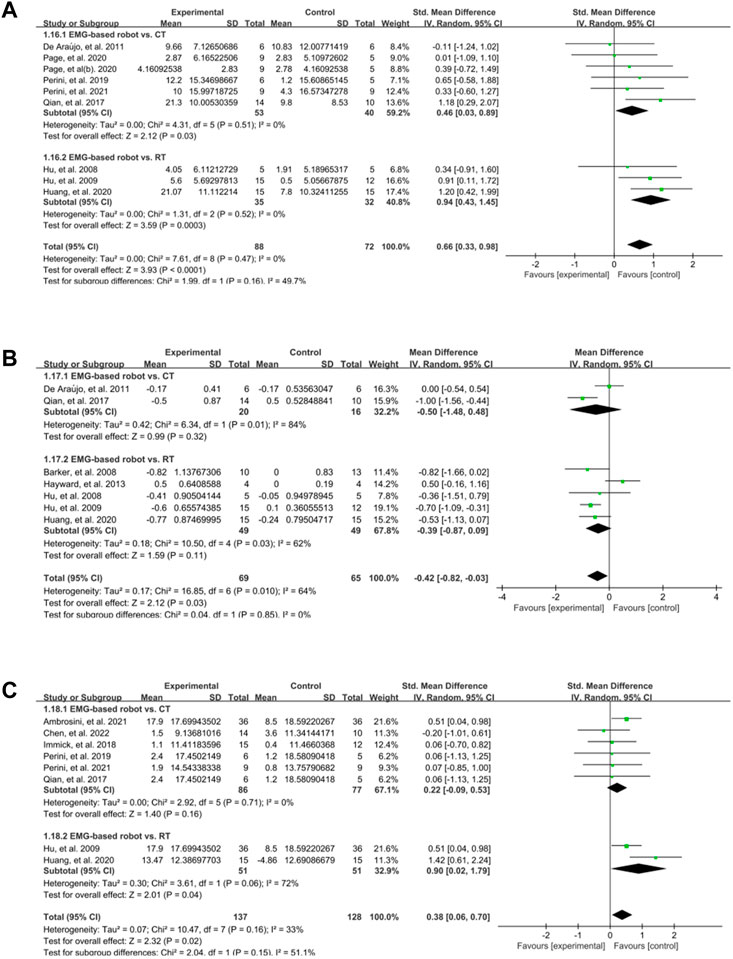
FIGURE 4. A subgroup analysis of the effect of EMG-based robot vs. different control groups on outcomes. (A) Forest plot analysis of the effects of different control groups on FMA. (B) Forest plot analysis of the effects of different control groups on MAS. (C) Forest plot analysis of the effects of different control groups on activity limitation.
3.4.2 The total time of training
As described in previous studies (Wang et al., 2021; Zhang et al., 2022), the amount of intervention was estimated by total time. We discovered that there was a significant difference in the upper limb motor function in both subgroups at the end of treatment between EMG-based robot therapy and conventional therapies, and the effect size was lager in subgroup with total training time ≤1000 min (SMD:0.67, 95% CI:0.25–1.09) than subset with total time >1000 min (SMD:0.58, 95% CI:0.14–1.03) (Figure 5A). In terms of spasticity, subgroup with total time ≤1000 min had a significant difference between EG and CG (MD: −0.77, 95% CI: −1.06 to −0.48), but no significant difference in the subset with total time >1000 min (MD: −0.02, 95% CI: −0.58 to 0.53) (Figure 5B). Activity limitation also changed significantly in subgroup with total time ≤1000 min (SMD:0.45, 95% CI:0.06–0.83), rather than subgroup with total time >1000 min (SMD:0.44, 95% CI: −0.58–1.45) (Figure 5C).
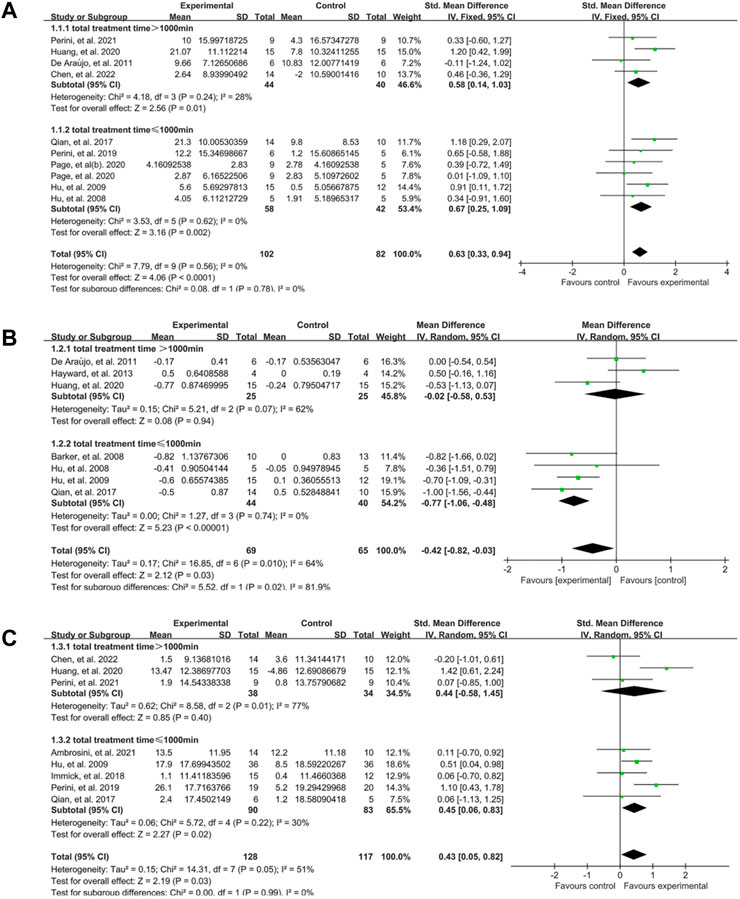
FIGURE 5. A subgroup analysis of the effect of EMG-based robot with different total training time vs. conventional therapies on outcomes. (A) Forest plot analysis of the effects of different total time of training on FMA. (B) Forest plot analysis of the effects of different total time of training on MAS. (C) Forest plot analysis of the effects of different total time of training on activity limitation.
3.4.3 The intervention mode
The intervention modes provided by EMG-based robot included EMG-driven robot with electrical stimulation (ES) and the type without ES. Subgroup analysis showed that the robot type with ES (SMD: 0.91, 95% CI:0.44–1.37) had larger effect size on the FMA than the type without ES (SMD: 0.42, 95% CI: 0.01–0.82) at the end of treatment (Figure 6A). The outcome of MAS at the end of treatment showed no significant difference between EG and CG in both subgroup (Figure 6B). In terms of the activity limitation, we found that the subgroup with ES (SMD:0.60, 95% CI:0.17–1.04) was better than the subgroup without ES (SMD: −0.06, 95% CI: −0.62–0.49) (Figure 6C).
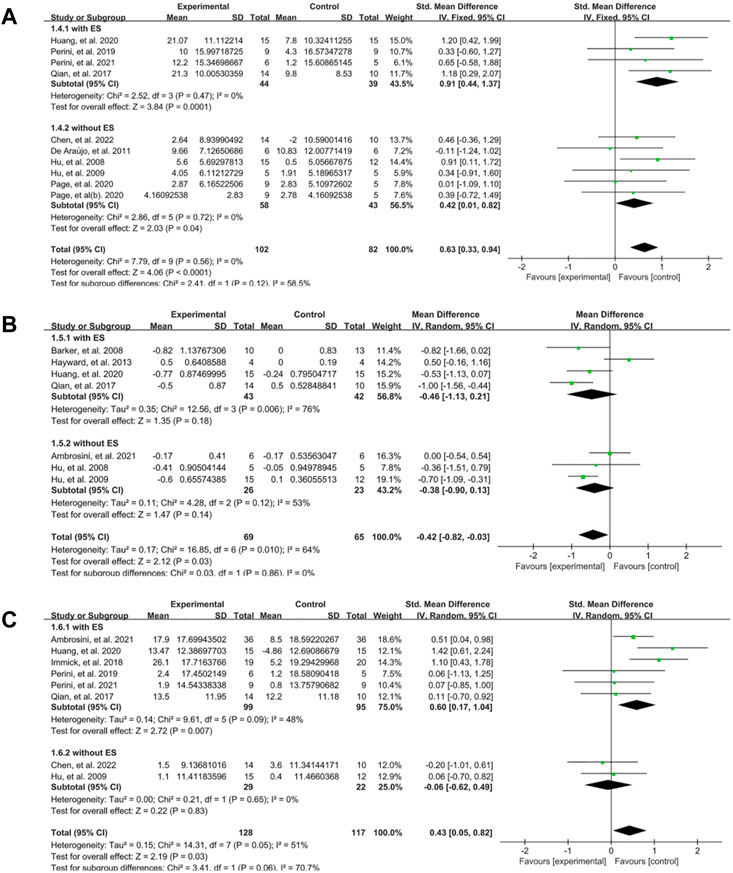
FIGURE 6. A subgroup analysis of the effect of different EMG-based robots vs. conventional therapies on outcomes. (A) Forest plot analysis of the effects of different intervention mode on FMA. (B) Forest plot analysis of the effects of different intervention mode on MAS. (C) Forest plot analysis of the effects of different intervention mode on activity limitation.
3.4.4 Stage of stroke
The stage of stroke was evaluated according to the duration after the onset time, and the participants were classified into subacute (≤6m) and chronic (>6m) group (Wang et al., 2021). For upper limb motor function, most studies focused on chronic stage and just one study designed by Qian, et al. (Qian et al., 2017) was in subacute stage. Analysis showed that FMA score was significantly changed in chronic group (SMD:0.55, 95% CI:0.23–0.88) and subacute group (SMD:1.18, 95% CI:0.29–2.07) (Figure 7A). For spasticity, there were no significant difference between two groups (Figure 7B). When comes to the activity limitation, the result showed the effect was better in subacute group (SMD:0.60, 95% CI:0.10–1.09) than chronic group (SMD:0.30, 95% CI: −0.31–0.91) (Figure 7C).
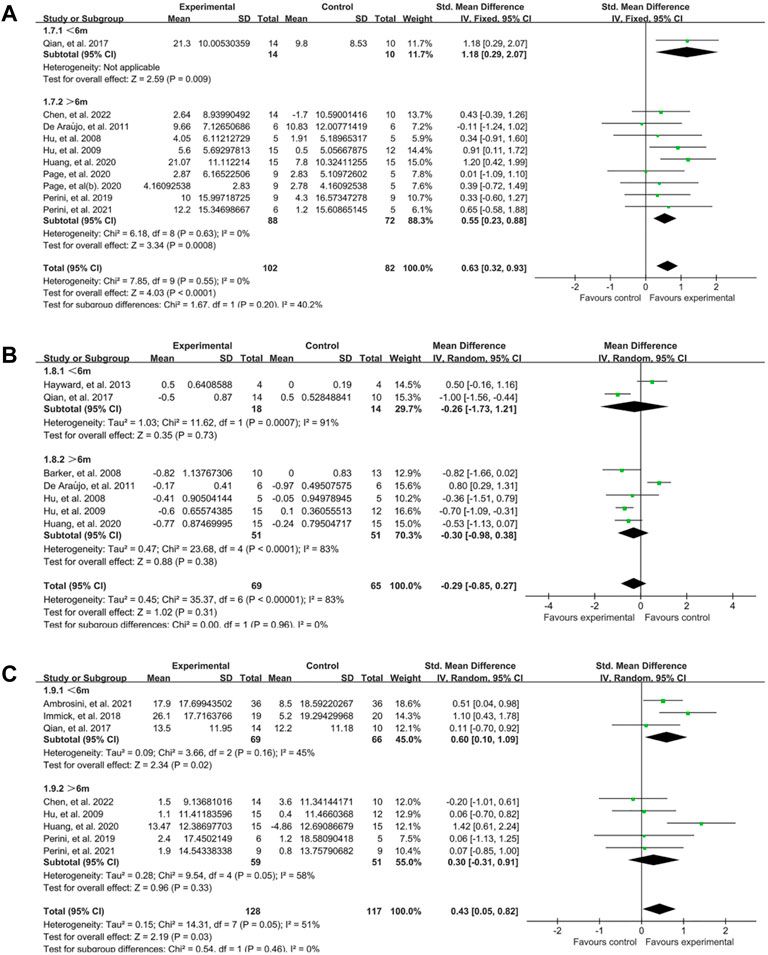
FIGURE 7. A subgroup analysis of the effect of EMG-based robot for different stage of stroke. (A) Forest plot analysis of the effects of different stage of stroke on FMA. (B) Forest plot analysis of the effects of stage of stroke on MAS. (C) Forest plot analysis of the effects of stage of stroke on activity limitation.
4 Discussion
In the present meta-analysis, a total of 12 RCTs and one cross-over clinical trial were analyzed, which included a total of 330 subjects (EG, n = 175; CG, n = 155). The results indicated that EMG-based robot was more effective than conventional therapies, including physical therapy, occupational therapy, passive training, and conventional robotic therapy. Results from subgroup analyses further revealed that the efficacy of the treatment was better in patients in the subacute stage, those who received a total treatment time of less than 1000 min, and those who received electromyography (EMG)-based robotic therapy combined with electrical stimulation (ES). These findings suggest that EMG-based robot therapy may be considered a promising rehabilitation method to improve upper limb dysfunction in post-stroke patients.
4.1 Effect of EMG-based robot
The findings presented in Figures 3, 4 demonstrate that EMG-based robot therapy can significantly enhance upper limb motor function, reduce spasticity, and improve activity level in post-stroke patients, as compared to the robotic therapies and conventional therapies. Following a stroke occurrence, the injured brain undergoes a reorganization process that involves recruiting pathways homologous to the damaged regions in function but distinct in anatomy, synaptogenesis, dendritic arborization, and reinforcing synaptic connections (Rossini et al., 2003). Such adaptive brain changes seem to be closely related to rehabilitation and motor training, contributing to the improvement of functional outcomes (Chen et al., 2018; Sampaio-Baptista et al., 2018; Sheng et al., 2022). High-intensity repetitive training is one of the principles of post-stroke rehabilitation (Langhorne et al., 2011). Although there are no clear guidelines for the optimal level of practice, it is widely accepted that more intensive training is beneficial. Robot-assisted therapy can provide patients with repetitive, high-intensity training and improve their motor function (Zhang et al., 2017; Kim et al., 2020; Wang et al., 2021; Yeung et al., 2021). The possible mechanism involves enhancing neural plasticity and neuronal activity to facilitate neuroplasticity change (Chen et al., 2019; Xing and Bai, 2020). According to the Hebbian learning rule, the connections between neurons are strengthened when neurons are simultaneously active (that is, long-term potentiation) (Orbach, 1998). In addition, signals from EMG-based robot can reflect the voluntary movement intention of patients in real time (Rong et al., 2015; Zhuang et al., 2021), which may increase active patient participation and promote interaction between humans and machines. Recording of muscle activity by EMG has proved to be helpful to explore the activity state of muscle tissue and the control mechanism of the nervous system under different task states after stroke (Ma et al., 2017; Chen et al., 2018), and then be useful for evaluating central and peripheral determinants of motor dysfunction which facilitates the understanding of mechanism behind rehabilitation intervention (Sheng et al., 2022; Li et al., 2023). Voluntary motor intention is crucial in the rehabilitation of motor function after stroke (Yang et al., 2022). Rehabilitation training that incorporates participants’ intention input is more effective which further facilitate active participation (Hu et al., 2009; Hu et al., 2021; Zhang et al., 2022) and interactive control (Cozens, 1999). The formation of a sensorimotor cycle by the voluntary intention output and the afferent sensor might facilitate motor relearning in post-stroke (Cauraugh et al., 2000). Furthermore, the effectiveness of repeated training increased substantially with the increase interaction between patients and machine (Hu et al., 2009; Hu et al., 2021). In general, the results of current study support the clinical application of EMG-based robot therapy in improving upper limb dysfunction in patients after stroke. However, more investigation is needed to reveal the relation between the changes of brain function and improvements of neuromuscular systems (i.e., by using cortical-muscular coherence technique) during the EMG-based robot training. This might be helpful for the understanding of the potential mechanism related to neuroplasticity in post-stroke patients.
4.2 The training intensity and mode of the EMG-based robotic intervention
The subgroup analysis suggested that the effect of the subgroup with total treatment time ≤ 1000 min was better than the subgroup with total time >1000 min (Figure 5), indicating the optimal treatment parameters to achieve the best effect of EMG-based robot therapy remains to be determined. Post-stroke fatigue is a common complication that negatively impacts patient’s rehabilitation outcomes (Duncan et al., 2012; Finsterer and Mahjoub, 2014; Maaijwee et al., 2015). One prevalent hypothesis is that physical deconditioning may contribute to fatigue following stroke (Duncan et al., 2012). One study focused on brain-computer interface (BCI) indicated that mental fatigue may also play a role in poorer BCI performance (Foong et al., 2020). Fatigue may affect patient motivation during training and interaction between human and machine, thereby hampering the efficiency of EMG-based robot. However, the relationship between post-stroke fatigue and motor training is rarely studied, and further research is needed to explore this aspect.
In the context of training mode, our results showed that the efficiency of the EMG-based robot with electrical stimulation (ES) was superior to the type without ES (Figure 6). Clinically, ES can be used to activate muscles, prevent muscle atrophy, and increase muscle strength (Hu et al., 2021; Li et al., 2022). In addition, sensory dysfunction is a common complication after stroke (Sullivan and Hedman, 2008; Tyson et al., 2008), which is related to the reduction of motor function recovery (Kusoffsky et al., 1982). ES may effectively improve sensory awareness of paralyzed muscles after stroke (Mäenpää et al., 2004), and elicit sensory feedback to the cortex during muscle contraction to facilitate motor relearning (Sujith, 2008). The sensory feedback is beneficial for motor function recovery post stroke (Sharififar et al., 2018). In the subgroup that received ES, the sensory feedback from the ES to the affected limb may be beneficial in motor function improvement. But excessive ES might impede the effect of motor training (Chae et al., 2002) and it is crucial to explore the optimal proportion of assistance from both ES and robot (Li et al., 2022). Hu et al., (Hu et al., 2011), reported that the performance of wrist tracking could be better with the 1:1 assistance from both ES and robot. But the small sample size and lack of long-term testing limited the findings of this study.
The subgroup analysis also showed that the EMG-based robot was more effective in subacute stage than chronic stage (Figure 7), which is consistent with previous studies (Mehrholz et al., 2018; Dehem et al., 2019; Dromerick et al., 2021). Early participation in rehabilitation is crucial for motor recovery as it can facilitate brain reorganization, optimize motor responsiveness and spontaneous neural plasticity, which may contribute to better rehabilitation outcomes in post-stroke patients, (Zeiler and Krakauer, 2013; Ng et al., 2015). Improved motor function in the subacute phase is more likely to generalize into activities of daily living (Flöel et al., 2014; Tomori et al., 2015).
4.3 Study limitations
While our subgroup analysis indicated that the effect of EMG-based robot was better in patients in the subacute stage, those who received a total treatment time of less than 1000 min, and those who received EMG-based robot combined with ES, it is important to acknowledge the limited sample size of the included studies and the potential impact on the validity of the results. Furthermore, the language restriction of our study to English-language articles may have introduced a selection bias which may also limit the generalizability of the results. The EMG-based robot requires active training and may not be suitable for patients with cognitive impairment. Future studies may explore the relationship between post-stroke fatigue and functional training to determine the best parameters of the EMG-based robot for the upper limb function, which could ultimately results in improved clinical outcomes for stroke survivors.
5 Conclusion
The present study provides evidence that EMG-based robot therapy is superior to conventional therapies in improving upper limb motor control, spasticity, and activity limitation in post-stroke patients. These findings suggest that EMG-based robot therapy could be a promising rehabilitation method for promoting the recovery of upper extremity function in this patient population. Further research should explore optimal parameters of EMG-based robot therapy and its long-term effects on upper limb function in post-stroke patients.
Data availability statement
The original contributions presented in the study are included in the article/supplementary materials, further inquiries can be directed to the corresponding authors.
Author contributions
LL, HH, and YH: conceptualization. YH, XW, and LL: methodology. YH and LL: writing–original draft preparation. YH, XW, WZ, HH, and LL: writing–review and editing. All authors contributed to the article and approved the submitted version.
Funding
This work was supported by the National Natural Science Foundation of China (No.32071316, 32211530049), the Key Research and Development Project of Shaanxi province (2022SF-117), the Natural Science Foundation of Shaanxi province (2022-JM482), Shenzhen Science and Technology Program (GJHZ20210705143401005) and the Education and Teaching Reform Funds for the Central Universities (No.22GZ230101).
Acknowledgments
We acknowledge the help of Dr. Le Ge during performance of this study.
Conflict of interest
The authors declare that the research was conducted in the absence of any commercial or financial relationships that could be construed as a potential conflict of interest.
Publisher’s note
All claims expressed in this article are solely those of the authors and do not necessarily represent those of their affiliated organizations, or those of the publisher, the editors and the reviewers. Any product that may be evaluated in this article, or claim that may be made by its manufacturer, is not guaranteed or endorsed by the publisher.
Supplementary material
The Supplementary Material for this article can be found online at: https://www.frontiersin.org/articles/10.3389/fphys.2023.1172958/full#supplementary-material
References
Ambrosini, E., Gasperini, G., Zajc, J., Immick, N., Augsten, A., Rossini, M., et al. (2021). A robotic system with EMG-triggered functional eletrical stimulation for restoring arm functions in stroke survivors. Neurorehabil. Neural Repair 35 (4), 334–345. doi:10.1177/1545968321997769
Barker, R. N., Brauer, S. G., and Carson, R. G. (2008). Training of reaching in stroke survivors with severe and chronic upper limb paresis using a novel nonrobotic device: A randomized clinical trial. Stroke 39 (6), 1800–1807. doi:10.1161/strokeaha.107.498485
Cauraugh, J., Light, K., Kim, S., Thigpen, M., and Behrman, A. (2000). Chronic motor dysfunction after stroke: Recovering wrist and finger extension by electromyography-triggered neuromuscular stimulation. Stroke 31 (6), 1360–1364. doi:10.1161/01.str.31.6.1360
Chae, J., Yang, G., Park, B. K., and Labatia, I. (2002). Muscle weakness and cocontraction in upper limb hemiparesis: Relationship to motor impairment and physical disability. Neurorehabil Neural Repair 16 (3), 241–248. doi:10.1177/154596830201600303
Chen, K., Zheng, Y., Wei, J. A., Ouyang, H., Huang, X., Zhang, F., et al. (2019). Exercise training improves motor skill learning via selective activation of mTOR. Sci. Adv. 5 (7), eaaw1888. doi:10.1126/sciadv.aaw1888
Chen, Y., Hu, H., Ma, C., Zhan, Y., Chen, N., Li, L., et al. (2018). Stroke-related changes in the complexity of muscle activation during obstacle-crossing using fuzzy approximate entropy analysis. Front. Neurol. 9, 131. doi:10.3389/fneur.2018.00131
Chen, Y. W., Chiang, W. C., Chang, C. L., Lo, S. M., and Wu, C. Y. (2022). Comparative effects of EMG-driven robot-assisted therapy versus task-oriented training on motor and daily function in patients with stroke: A randomized cross-over trial. J. Neuroeng Rehabil. 19 (1), 6. doi:10.1186/s12984-021-00961-w
Cheng, H. S., Ju, M. S., and Lin, C. C. (2003). Improving elbow torque output of stroke patients with assistive torque controlled by EMG signals. J. Biomech. Eng. 125 (6), 881–886. doi:10.1115/1.1634284
Corbetta, D., Sirtori, V., Castellini, G., Moja, L., and Gatti, R. (2015). Constraint-induced movement therapy for upper extremities in people with stroke. Cochrane Database Syst. Rev. 2015 (10), Cd004433. doi:10.1002/14651858.CD004433.pub3
Cozens, J. A. (1999). Robotic assistance of an active upper limb exercise in neurologically impaired patients. IEEE Trans. Rehabil. Eng. 7 (2), 254–256. doi:10.1109/86.769416
De Araújo, R. C., Junior, F. L., Rocha, D. N., Sono, T. S., and Pinotti, M. (2011). Effects of intensive arm training with an electromechanical orthosis in chronic stroke patients: A preliminary study. Arch. Phys. Med. Rehabil. 92 (11), 1746–1753. doi:10.1016/j.apmr.2011.05.021
Dehem, S., Gilliaux, M., Stoquart, G., Detrembleur, C., Jacquemin, G., Palumbo, S., et al. (2019). Effectiveness of upper-limb robotic-assisted therapy in the early rehabilitation phase after stroke: A single-blind, randomised, controlled trial. Ann. Phys. Rehabil. Med. 62 (5), 313–320. doi:10.1016/j.rehab.2019.04.002
Dipietro, L., Ferraro, M., Palazzolo, J. J., Krebs, H. I., Volpe, B. T., and Hogan, N. (2005). Customized interactive robotic treatment for stroke: EMG-triggered therapy. IEEE Trans. Neural Syst. Rehabil. Eng. 13 (3), 325–334. doi:10.1109/tnsre.2005.850423
Doucet, B. M., Lam, A., and Griffin, L. (2012). Neuromuscular electrical stimulation for skeletal muscle function. Yale J. Biol. Med. 85 (2), 201–215.
Dromerick, A. W., Geed, S., Barth, J., Brady, K., Giannetti, M. L., Mitchell, A., et al. (2021). Critical Period after stroke study (cpass): A phase II clinical trial testing an optimal time for motor recovery after stroke in humans. Proc. Natl. Acad. Sci. U. S. A. 118 (39), e2026676118. doi:10.1073/pnas.2026676118
Duncan, F., Kutlubaev, M. A., Dennis, M. S., Greig, C., and Mead, G. E. (2012). Fatigue after stroke: A systematic review of associations with impaired physical fitness. Int. J. Stroke 7 (2), 157–162. doi:10.1111/j.1747-4949.2011.00741.x
Ferris, D. P., Czerniecki, J. M., and Hannaford, B. (2005). An ankle-foot orthosis powered by artificial pneumatic muscles. J. Appl. Biomech. 21 (2), 189–197. doi:10.1123/jab.21.2.189
Feys, H. M., De Weerdt, W. J., Selz, B. E., Cox Steck, G. A., Spichiger, R., Vereeck, L. E., et al. (1998). Effect of a therapeutic intervention for the hemiplegic upper limb in the acute phase after stroke: A single-blind, randomized, controlled multicenter trial. Stroke 29 (4), 785–792. doi:10.1161/01.str.29.4.785
Finsterer, J., and Mahjoub, S. Z. (2014). Fatigue in healthy and diseased individuals. Am. J. Hosp. Palliat. Care 31 (5), 562–575. doi:10.1177/1049909113494748
Flöel, A., Werner, C., Grittner, U., Hesse, S., Jöbges, M., Knauss, J., et al. (2014). Physical fitness training in Subacute Stroke (PHYS-STROKE)-study protocol for a randomised controlled trial. Trials 15, 45. doi:10.1186/1745-6215-15-45
Foong, R., Ang, K. K., Quek, C., Guan, C., Phua, K. S., Kuah, C. W. K., et al. (2020). Assessment of the efficacy of EEG-based MI-BCI with visual feedback and EEG correlates of mental fatigue for upper-limb stroke rehabilitation. IEEE Trans. Biomed. Eng. 67 (3), 786–795. doi:10.1109/tbme.2019.2921198
Hayward, K. S., Barker, R. N., Brauer, S. G., Lloyd, D., Horsley, S. A., and Carson, R. G. (2013). SMART arm with outcome-triggered electrical stimulation: A pilot randomized clinical trial. Top. Stroke Rehabil. 20 (4), 289–298. doi:10.1310/tsr2004-289
Higgins, J. P. T., Altman, D. G., Gøtzsche, P. C., Jüni, P., Moher, D., Oxman, A. D., et al. (2011). The Cochrane Collaboration’s tool for assessing risk of bias in randomised trials. BMJ 343, d5928. doi:10.1136/bmj.d5928
Hu, C., Wang, T., Leung, K. W. C., Li, L., and Tong, K. Y. (2021). Muscle electrical impedance properties and activation alteration after functional electrical stimulation-assisted cycling training for chronic stroke survivors: A longitudinal pilot study. Front. Neurol. 12, 746263. doi:10.3389/fneur.2021.746263
Hu, X. L., Tong, K. Y., Li, R., Chen, M., Xue, J. J., Ho, S. K., et al. (2010). "Effectiveness of functional electrical stimulation (FES)-Robot assisted wrist training on persons after stroke", in: 32nd annual international conference of the IEEE engineering-in-medicine-and-biology-society (EMBC 10)), 5819–5822.
Hu, X. L., Tong, K. Y., Li, R., Chen, M., Xue, J. J., Ho, S. K., et al. (2011). Post-stroke wrist rehabilitation assisted with an intention-driven functional electrical stimulation (FES)-robot system. IEEE Int. Conf. Rehabil. Robot. 2011, 5975424. doi:10.1109/icorr.2011.5975424
Hu, X. L., Tong, K. Y., Song, R., Zheng, X. J., and Leung, W. W. (2009). A comparison between electromyography-driven robot and passive motion device on wrist rehabilitation for chronic stroke. Neurorehabil Neural Repair 23 (8), 837–846. doi:10.1177/1545968309338191
Hu, X. L., Tong, R. K., Ho, N. S., Xue, J. J., Rong, W., and Li, L. S. (2015). Wrist rehabilitation assisted by an electromyography-driven neuromuscular electrical stimulation robot after stroke. Neurorehabil Neural Repair 29 (8), 767–776. doi:10.1177/1545968314565510
Huang, Y., Nam, C., Li, W., Rong, W., Xie, Y., Liu, Y., et al. (2020). A comparison of the rehabilitation effectiveness of neuromuscular electrical stimulation robotic hand training and pure robotic hand training after stroke: A randomized controlled trial. Biomed. Signal Process. Control 56. doi:10.1016/j.bspc.2019.101723
Immick, N., Ambrosini, E., Augsten, A., Rossini, M., Gasperini, G., Proserpio, D., et al. (2018). Hybrid robotic system for arm training after stroke: preliminary results of a randomized controlled trial. Converg. Clin. Eng. Res. Neurorehabilitation III.
Kim, W. S., Cho, S., Ku, J., Kim, Y., Lee, K., Hwang, H. J., et al. (2020). Clinical application of virtual reality for upper limb motor rehabilitation in stroke: Review of technologies and clinical evidence. J. Clin. Med. 9 (10), 3369. doi:10.3390/jcm9103369
Kusoffsky, A., Wadell, I., and Nilsson, B. Y. (1982). The relationship between sensory impairment and motor recovery in patients with hemiplegia. Scand. J. Rehabil. Med. 14 (1), 27–32.
Langhorne, P., Bernhardt, J., and Kwakkel, G. (2011). Stroke rehabilitation. Lancet 377 (9778), 1693–1702. doi:10.1016/s0140-6736(11)60325-5
Li, L., Hu, C., Leung, K. W. C., and Tong, K. Y. (2022). Immediate effects of functional electrical stimulation-assisted cycling on the paretic muscles of patients with hemiparesis after stroke: Evidence from electrical impedance myography. Front. Aging Neurosci. 14, 880221. doi:10.3389/fnagi.2022.880221
Li, L., Hu, H., Yao, B., Huang, C., Lu, Z., Klein, C. S., et al. (2023). Electromyography-force relation and muscle fiber conduction velocity affected by spinal cord injury. Bioengineering 10, 217. doi:10.3390/bioengineering10020217
Ma, C., Chen, N., Mao, Y., Huang, D., Song, R., and Li, L. (2017). Alterations of muscle activation pattern in stroke survivors during obstacle crossing. Front. Neurol. 8, 70. doi:10.3389/fneur.2017.00070
Maaijwee, N. A., Arntz, R. M., Rutten-Jacobs, L. C., Schaapsmeerders, P., Schoonderwaldt, H. C., van Dijk, E. J., et al. (2015). Post-stroke fatigue and its association with poor functional outcome after stroke in young adults. J. Neurol. Neurosurg. Psychiatry 86 (10), 1120–1126. doi:10.1136/jnnp-2014-308784
Mäenpää, H., Jaakkola, R., Sandström, M., and von Wendt, L. (2004). Effect of sensory-level electrical stimulation of the tibialis anterior muscle during physical therapy on active dorsiflexion of the ankle of children with cerebral palsy. Pediatr. Phys. Ther. 16 (1), 39–44. doi:10.1097/01.Pep.0000114629.20513.7a
Mehrholz, J., Pohl, M., Platz, T., Kugler, J., and Elsner, B. (2018). Electromechanical and robot-assisted arm training for improving activities of daily living, arm function, and arm muscle strength after stroke. Cochrane Database Syst. Rev. 9 (9), Cd006876. doi:10.1002/14651858.CD006876.pub5
Micera, S., Caleo, M., Chisari, C., Hummel, F. C., and Pedrocchi, A. (2020). Advanced neurotechnologies for the restoration of motor function. Neuron 105 (4), 604–620. doi:10.1016/j.neuron.2020.01.039
Moher, D., Liberati, A., Tetzlaff, J., and Altman, D. G.PRISMA Group (2009). Preferred reporting items for systematic reviews and meta-analyses: The PRISMA statement. Bmj 339, b2535. doi:10.1136/bmj.b2535
Nam, C., Rong, W., Li, W., Cheung, C., Ngai, W., Cheung, T., et al. (2022). An exoneuromusculoskeleton for self-help upper limb rehabilitation after stroke. Soft Robot. 9 (1), 14–35. doi:10.1089/soro.2020.0090
Nam, C., Rong, W., Li, W., Xie, Y., Hu, X., and Zheng, Y. (2017). The effects of upper-limb training assisted with an electromyography-driven neuromuscular electrical stimulation robotic hand on chronic stroke. Front. Neurol. 8, 679. doi:10.3389/fneur.2017.00679
Ng, K. L., Gibson, E. M., Hubbard, R., Yang, J., Caffo, B., O'Brien, R. J., et al. (2015). Fluoxetine maintains a state of heightened responsiveness to motor training early after stroke in a mouse model. Stroke 46 (10), 2951–2960. doi:10.1161/strokeaha.115.010471
Orbach, J. (1998). The neuropsychological theories of Lashley and Hebb: Contemporary perspectives fifty years after Hebb's the Organization of Behavior: Vanuxem Lectures and selected theoretical papers of Lashley. Lanham, MD, US: University Press of America.
Page, S., Griffin, C., and White, S. (2020). Efficacy of myoelectric bracing in moderately impaired stroke survivors: A randomized, controlled trial. J. rehabilitation Med. 52 (2), jrm00017. doi:10.2340/16501977-2644
Page, S. J., Hill, V., and White, S. (2013). Portable upper extremity robotics is as efficacious as upper extremity rehabilitative therapy: A randomized controlled pilot trial. Clin. Rehabil. 27 (6), 494–503. doi:10.1177/0269215512464795
Parker, V. M., Wade, D. T., and Langton Hewer, R. (1986). Loss of arm function after stroke: Measurement, frequency, and recovery. Int. Rehabil. Med. 8 (2), 69–73. doi:10.3109/03790798609166178
Perini, G., Lencioni, T., Bertoni, R., Marzegan, A., Thorsen, R., Bowman, T., et al. (2019). Rehabilitation of upper limb in chronic stroke patients: pilot study of functional and neuromotor outcome of a task oriented approach including MeCFES and robotic treatment. Gait & posture 74, 29–30. doi:10.1016/j.gaitpost.2019.07.486
Perini, G., Bertoni, R., Thorsen, R., Carpinella, I., Lencioni, T., Ferrarin, M., et al. (2021). Sequentially applied myoelectrically controlled FES in a task-oriented approach and robotic therapy for the recovery of upper limb in post-stroke patients: A randomized controlled pilot study. Technol. Health Care 29 (3), 419–429. doi:10.3233/thc-202371
Pollock, A., Farmer, S. E., Brady, M. C., Langhorne, P., Mead, G. E., Mehrholz, J., et al. (2014). Interventions for improving upper limb function after stroke. Cochrane Database Syst. Rev. 2014 (11), Cd010820. doi:10.1002/14651858.CD010820.pub2
Qian, Q., Hu, X., Lai, Q., Ng, S. C., Zheng, Y., and Poon, W. (2017). Early stroke rehabilitation of the upper limb assisted with an electromyography-driven neuromuscular electrical stimulation-robotic arm. Front. Neurol. 8, 447. doi:10.3389/fneur.2017.00447
Reis, S. B., Bernardo, W. M., Oshiro, C. A., Krebs, H. I., and Conforto, A. B. (2021). Effects of robotic therapy associated with noninvasive brain stimulation on upper-limb rehabilitation after stroke: Systematic review and meta-analysis of randomized clinical trials. Neurorehabil Neural Repair 35 (3), 256–266. doi:10.1177/1545968321989353
Rong, W., Li, W., Pang, M., Hu, J., Wei, X., Yang, B., et al. (2017). A Neuromuscular Electrical Stimulation (NMES) and robot hybrid system for multi-joint coordinated upper limb rehabilitation after stroke. J. Neuroeng Rehabil. 14 (1), 34. doi:10.1186/s12984-017-0245-y
Rong, W., Tong, K. Y., Hu, X. L., and Ho, S. K. (2015). Effects of electromyography-driven robot-aided hand training with neuromuscular electrical stimulation on hand control performance after chronic stroke. Disabil. Rehabil. Assist. Technol. 10 (2), 149–159. doi:10.3109/17483107.2013.873491
Rosen, J., Brand, M., Fuchs, M. B., and Arcan, M. (2001). A myosignal-based powered exoskeleton system. IEEE Transactions on Systems, Man, and Cybernetics—Part A: Systems and Humans 31 (3), 210–222. doi:10.1109/3468.925661
Rossini, P. M., Calautti, C., Pauri, F., and Baron, J. C. (2003). Post-stroke plastic reorganisation in the adult brain. Lancet Neurol. 2 (8), 493–502. doi:10.1016/s1474-4422(03)00485-x
Sampaio-Baptista, C., Sanders, Z. B., and Johansen-Berg, H. (2018). Structural plasticity in adulthood with motor learning and stroke rehabilitation. Annu. Rev. Neurosci. 41, 25–40. doi:10.1146/annurev-neuro-080317-062015
Sharififar, S., Shuster, J. J., and Bishop, M. D. (2018). Adding electrical stimulation during standard rehabilitation after stroke to improve motor function. A systematic review and meta-analysis. Ann. Phys. Rehabil. Med. 61 (5), 339–344. doi:10.1016/j.rehab.2018.06.005
Sheng, W., Li, S., Zhao, J., Wang, Y., Luo, Z., Lo, W., et al. (2022). Upper limbs muscle co-contraction changes correlated with the impairment of the corticospinal tract in stroke survivors: Preliminary evidence from electromyography and motor-evoked potential. Front. Neurosci. 16, 886909. doi:10.3389/fnins.2022.886909
Song, R., Tong, K.-y., Hu, X., and Li, L. (2008). Assistive control system using continuous myoelectric signal in robot-aided arm training for patients after stroke. Ieee Trans. Neural Syst. Rehabilitation Eng. 16 (4), 371–379. doi:10.1109/tnsre.2008.926707
Stein, J., Narendran, K., McBean, J., Krebs, K., and Hughes, R. (2007). Electromyography-controlled exoskeletal upper-limb-powered orthosis for exercise training after stroke. Am. J. Phys. Med. Rehabil. 86 (4), 255–261. doi:10.1097/PHM.0b013e3180383cc5
Sujith, O. K. (2008). Functional electrical stimulation in neurological disorders. Eur. J. Neurol. 15 (5), 437–444. doi:10.1111/j.1468-1331.2008.02127.x
Sullivan, J. E., and Hedman, L. D. (2008). Sensory dysfunction following stroke: Incidence, significance, examination, and intervention. Top. Stroke Rehabil. 15 (3), 200–217. doi:10.1310/tsr1503-200
Tomori, K., Nagayama, H., Ohno, K., Nagatani, R., Saito, Y., Takahashi, K., et al. (2015). Comparison of occupation-based and impairment-based occupational therapy for subacute stroke: A randomized controlled feasibility study. Clin. Rehabil. 29 (8), 752–762. doi:10.1177/0269215514555876
Tyson, S. F., Hanley, M., Chillala, J., Selley, A. B., and Tallis, R. C. (2008). Sensory loss in hospital-admitted people with stroke: Characteristics, associated factors, and relationship with function. Neurorehabil Neural Repair 22 (2), 166–172. doi:10.1177/1545968307305523
Veerbeek, J. M., Langbroek-Amersfoort, A. C., van Wegen, E. E., Meskers, C. G., and Kwakkel, G. (2017). Effects of robot-assisted therapy for the upper limb after stroke. Neurorehabil Neural Repair 31 (2), 107–121. doi:10.1177/1545968316666957
Wang, L., Zheng, Y., Dang, Y., Teng, M., Zhang, X., Cheng, Y., et al. (2021). Effects of robot-assisted training on balance function in patients with stroke: A systematic review and meta-analysis. J. Rehabil. Med. 53 (4), jrm00174. doi:10.2340/16501977-2815
Winstein, C. J., Stein, J., Arena, R., Bates, B., Cherney, L. R., Cramer, S. C., et al. (2016). Guidelines for adult stroke rehabilitation and recovery: A guideline for healthcare professionals from the American heart association/American stroke association. Stroke 47 (6), e98–e169. doi:10.1161/STR.0000000000000098
Xing, Y., and Bai, Y. (2020). A review of exercise-induced neuroplasticity in ischemic stroke: Pathology and mechanisms. Mol. Neurobiol. 57 (10), 4218–4231. doi:10.1007/s12035-020-02021-1
Yang, R., Shen, Z., Lyu, Y., Zhuang, Y., Li, L., and Song, R. (2022). Voluntary assist-as-needed controller for an ankle power-assist rehabilitation robot. IEEE Trans. BME, 1–9. doi:10.1109/TBME.2022.3228070
Yeung, L. F., Lau, C. C. Y., Lai, C. W. K., Soo, Y. O. Y., Chan, M. L., and Tong, R. K. Y. (2021). Effects of wearable ankle robotics for stair and over-ground training on sub-acute stroke: A randomized controlled trial. J. Neuroeng Rehabil. 18 (1), 19. doi:10.1186/s12984-021-00814-6
Zeiler, S. R., and Krakauer, J. W. (2013). The interaction between training and plasticity in the poststroke brain. Curr. Opin. Neurol. 26 (6), 609–616. doi:10.1097/wco.0000000000000025
Zhang, C., Li-Tsang, C. W., and Au, R. K. (2017). Robotic approaches for the rehabilitation of upper limb recovery after stroke: A systematic review and meta-analysis. Int. J. Rehabil. Res. 40 (1), 19–28. doi:10.1097/mrr.0000000000000204
Zhang, L., Jia, G., Ma, J., Wang, S., and Cheng, L. (2022). Short and long-term effects of robot-assisted therapy on upper limb motor function and activity of daily living in patients post-stroke: A meta-analysis of randomized controlled trials. J. Neuroeng Rehabil. 19 (1), 76. doi:10.1186/s12984-022-01058-8
Keywords: EMG-based robot, stroke, upper extremity, meta-analysis, review
Citation: Huo Y, Wang X, Zhao W, Hu H and Li L (2023) Effects of EMG-based robot for upper extremity rehabilitation on post-stroke patients: a systematic review and meta-analysis. Front. Physiol. 14:1172958. doi: 10.3389/fphys.2023.1172958
Received: 24 February 2023; Accepted: 20 April 2023;
Published: 03 May 2023.
Edited by:
Qipeng Song, Shandong Sport University, ChinaReviewed by:
Lehua Yu, Chongqing Medical University, ChinaNing Jiang, Sichuan University, China
Suncheol Kwon, Korea Institute of Industrial Technology, Republic of Korea
Copyright © 2023 Huo, Wang, Zhao, Hu and Li. This is an open-access article distributed under the terms of the Creative Commons Attribution License (CC BY). The use, distribution or reproduction in other forums is permitted, provided the original author(s) and the copyright owner(s) are credited and that the original publication in this journal is cited, in accordance with accepted academic practice. No use, distribution or reproduction is permitted which does not comply with these terms.
*Correspondence: Huijing Hu, huhuijing@nwpu.edu.cn; Le Li, lile5@nwpu.edu.cn