Corrigendum: A modified formula using energy system contributions to calculate pure maximal rate of lactate accumulation during a maximal sprint cycling test
- 1Graduate School of Sports Medicine, CHA University, Pocheon-si, Gyeonggi-do, Republic of Korea
- 2Department of Medicine, General Graduate School, CHA University, Pocheon-si, Gyeonggi-do, Republic of Korea
- 3Olympic Base Center Rhineland, Cologne, Germany
- 4Department for Biosciences of Sports, Institute of Sports Science, University of Hildesheim, Hildesheim, Germany
- 5Institute of Cardiovascular Research and Sports Medicine, German Sport University Cologne, Cologne, Germany
Purpose: This study aimed at comparing previous calculating formulas of maximal lactate accumulation rate (νLa.max) and a modified formula of pure νLa.max (PνLa.max) during a 15-s all-out sprint cycling test (ASCT) to analyze their relationships.
Methods: Thirty male national-level track cyclists participated in this study (n = 30) and performed a 15-s ASCT. The anaerobic power output (Wpeak and Wmean), oxygen uptake, and blood lactate concentrations (La−) were measured. These parameters were used for different calculations of νLa.max and three energy contributions (phosphagen, WPCr; glycolytic, WGly; and oxidative, WOxi). The PνLa.max calculation considered delta La−, time until Wpeak (tPCr−peak), and the time contributed by the oxidative system (tOxi). Other νLa.max levels without tOxi were calculated using decreasing time by 3.5% from Wpeak (tPCr −3.5%) and tPCr−peak.
Results: The absolute and relative WPCr were higher than WGly and WOxi (p < 0.0001, respectively), and the absolute and relative WGly were significantly higher than WOxi (p < 0.0001, respectively); νLa.max (tPCr −3.5%) was significantly higher than PνLa.max and νLa.max (tPCr−peak), while νLa.max (tPCr−peak) was lower than PνLa.max (p < 0.0001, respectively). PνLa.max and νLa.max (tPCr−peak) were highly correlated (r = 0.99; R2 = 0.98). This correlation was higher than the relationship between PνLa.max and νLa.max (tPCr −3.5%) (r = 0.87; R2 = 0.77). νLa.max (tPCr−peak), PνLa.max, and νLa.max (tPCr −3.5%) were found to correlate with absolute Wmean and WGly.
Conclusion: PνLa.max as a modified calculation of νLa.max provides more detailed insights into the inter-individual differences in energy and glycolytic metabolism than νLa.max (tPCr−peak) and νLa.max (tPCr −3.5%). Because WOxi and WPCr can differ remarkably between athletes, implementing their values in PνLa.max can establish more optimized individual profiling for elite track cyclists.
Introduction
Three human energy systems (phosphagen, glycolytic, and oxidative systems) are simultaneously used during different exercises (Beneke et al., 2002; Yang et al., 2020; Yang et al., 2022a; Yang et al., 2022b). However, their relative energy contribution, particularly the energy systems that are predominantly utilized at a given moment, depends critically on the exercise intensity and duration (Beneke et al., 2002; Yang et al., 2022b). Therefore, precise determinations of energetic contributions during specific sports are crucial for a better understanding of physiological responses to enhance exercise prescriptions (Julio et al., 2017; Franchini, 2020; Yang et al., 2022b).
Among various Olympic disciplines, there are sections such as football, handball, basketball, rugby, and track cycling (Hettinga et al., 2007; Wackerhage et al., 2022), in which maximal anaerobic power and capacity are essential to achieve a highly competitive performance. For instance, the anaerobic capacity of track cyclists is exploited during a 1,500-m Keirin race over approximately 90–120 s (Foster et al., 1993; Hettinga et al., 2007). A crucial factor that determines the winner of such races is particularly the final all-out sprint to the finish line (Fujii et al., 2018). Although a high aerobic performance is absolutely necessary, these athletes also require high anaerobic power (Hettinga et al., 2007; Fujii et al., 2018). The anaerobic performance of athletes (e.g., 100-m sprint) is proportional to their individual anaerobic energy capacity (Yang et al., 2020; Park et al., 2021; Yang et al., 2022b). The phosphagen system (adenosine triphosphate and phosphocreatine, ATP-PCr) ensures the highest metabolic power or energy flux rate, which is reflected by the rate of energy transfer per unit of time. Although the energy flux rate is very high, the general capacity is low because of limited substrate stores that lasts for only a few muscle contractions (Gastin, 2001; Robergs et al., 2004). The glycolytic system can re-synthesize ATP through the non-aerobic degradation of carbohydrates and supports the metabolic energy requirements during intense exercise. This system, when compared to the phosphagen system, is characterized by an intermediate energy flux rate and metabolic power because of the higher number of reactions and greater overall capacity from a large amount of stored carbohydrates (Gastin, 2001; Robergs et al., 2004; Franchini, 2023). In this regard, the Mader’s (1984) model proposed considering individual maximal glycolytic rates (νLa.max) to be utilized while determining the maximal glycolysis and power after an all-out sports-specific sprint test (Hauser et al., 2014; Quittmann et al., 2020; Quittmann et al., 2021; Wackerhage et al., 2022). Accordingly, athletes in sprint sports can maximally produce over 1.0 mmol lactate per liter per second (i.e., ≥1.0 mmol·L−1·s−1) (Wackerhage et al., 2022).
The formula of νLa.max (Mader, 1984) is considered the delta lactate concentration (∆La−) between maximal blood La− accumulation after exercising and resting blood La− levels are divided by the difference between the total exercise time (texer) and phosphagen system–contributed time (tPCr). Here, tPCr is assumed as the period in which no La− formation (“fictitiously”) takes place, beginning from the start of the maximal power output until it is reduced by 3.5% (the point of −3.5% from the peak watt, tPCr −3.5%). Previous studies have used the tPCr −3.5% definition without providing an in-depth explanation on why the reduced 3.5% time point of the maximal power output was determined as tPCr (Hauser et al., 2014; Adam et al., 2015; Nitzsche et al., 2018; Quittmann et al., 2020; Quittmann et al., 2021). However, this assumption likely originates from the precision of power measurement of an early SRM cycle ergometer (Weber, 2003). Another tPCr definition was determined as the time until peak watt (tPCr−peak) during the νLa.max test (Manunzio et al., 2016).
Indeed, the determination of νLa.max via these calculations may further have imprecisions with the involvement of oxidative energy metabolism, also in short time frames of exercising, when it is not separately subtracted from the total energy demand. Interestingly, previous studies have reported that oxidative energy metabolism is not considered in the νLa.max formula, which contributes to a total energy expenditure of approximately 10% during the 100-m sprint (Heck et al., 2003; Quittmann et al., 2020; Park et al., 2021).
Also, calculations of the Mader’s model include the oxidative contribution timespan and not subtracting the energy contribution of oxidative metabolism. When considering an approximate contribution of 10% during short sprints, a “pure” νLa.max determination during the maximal anaerobic cycling test cannot be precisely calculated. To determine νLa.max, we minimize the oxidative contribution and lactate elimination during the aftermath of the test; its duration should be limited between 10 and 15 s (Heck et al., 2003; Quittmann et al., 2020). In this context, the PCr-La−-O2 method is yet another well-established methodology involving all three energy system contributions when calculating exercise-induced energy turnover (Beneke et al., 2004; de Moraes Bertuzzi et al., 2007; de Campos Mello et al., 2009; Hausen et al., 2017; Julio et al., 2017; Yang et al., 2018; Park et al., 2021; Yang et al., 2022b; Kaufmann et al., 2022). This method can easily be incorporated into the νLa.max formula as the time ratio of relative oxidative contribution (%) and then be used to calculate an optimized νLa.max (Beneke et al., 2004; de Moraes Bertuzzi et al., 2007; de Campos Mello et al., 2009; Campos et al., 2012; Hausen et al., 2017; Julio et al., 2017; Lopes-Silva et al., 2018; Yang et al., 2018; Franchini, 2020; Park et al., 2021; Yang et al., 2022b).
Therefore, this study compares three different νLa.max calculations based on the differences in determining the phosphagen-contributed time and incorporation of the oxidative energy system: first, νLa.max by using tPCr −3.5%; second, νLa.max by using tPCr−peak determinations; and third, by subtracting the contribution of the oxidative energy metabolism during a 15-s maximal sprint cycling test. The latter is substituted with a modified pure νLa.max (PνLa.max) formula using the analysis of the PCr-La−-O2 method. Finally, the relationships among the different νLa.max calculations, mean power output, and glycolytic energy contribution are analyzed.
Materials and methods
Participants
With an effect size of 0.32, an alpha error probability of 0.05, and statistical power of 0.95, the sample size was calculated using the G*Power software, version 3.1.9.4 (Heinrich Heine University, Düsseldorf, Germany). The effect size was considered based on previous studies (Hauser et al., 2014; Manunzio et al., 2016; Fujii et al., 2018; Quittmann et al., 2020; Quittmann et al., 2021). Considering a 10% dropout rate, 30 male national-level track cyclists were enrolled in this study. All participants were track cyclists (main discipline: Keirin) who train for 18–20 h per week. They have been enrolled in the Korean national championships over the last 8 years. The anthropometric parameters of these participants are age: 30 ± 6 years; height: 177.30 ± 6.21 cm; body mass: 85.11 ± 9.89; and body fat: 19.16% ± 4.78%. The participants had rested for 2 h after lunchtime and performed a 15-s all-out sprint cycling test (ASCT). They were instructed not to take any coffee, neuro stimulants, or medication immediately before the test. Alcohol and nicotine was not consumed at least 24 h before the experiment. The Institutional Review Board of CHA University approved this study (No. 1044308-202206-HR-032-02). Study protocols followed the ethical standards in the Declaration of Helsinki. All participants had signed an informed consent form.
Study procedure
All participants attended a single laboratory session. The test procedure was conducted and controlled in the same laboratory environment throughout testing (temperature: 23°C and relative humidity: 50%). The anthropometric parameters were measured with an eight-electrode segmental multi-frequency bioelectrical impedance analysis (20–100 kHz; InBody 270; InBody Co., Ltd., Seoul, Republic of Korea). Before the 15-s ASCT, the latest SRM ergometer (No. 2203, 04/2022, Schoberer Rad Messtechnik, GmbH, Jülich, Germany) was set up to fit each participant’s track bicycle position (i.e., crank length, saddle height, handle height, and saddle fore–aft position). An initial warm-up was performed for 10 min at 2 W·kg−1. They further rested passively in the sitting position on the ergometer was for 5 min (Figure 1). The 15-s ASCT was performed according to the manufacturer’s guidelines in an isokinetic mode set to a cadence of 120 rpm (Manunzio et al., 2016) in the ninth gear and with a 9.1 kg flywheel. The start and end of the 15-s ASCT were verbally announced by countdown. These settings enabled a reduction in the pedal force in a linear fashion during the initial acceleration phase and achieving a target pedaling rate of 120 rpm after ∼4 s (Manunzio et al., 2016; Dunst et al., 2023). The participants performed the test in the sitting position on the ergometer. They were verbally encouraged by the investigator to generate the maximum possible power output. Afterward, the absolute and relative peak and mean power outputs in watt and W·kg−1 were determined (Wpeak and Wmean, respectively) (Hauser et al., 2014; Manunzio et al., 2016; Quittmann et al., 2020; Quittmann et al., 2021). Oxygen uptake (resting oxygen uptake,
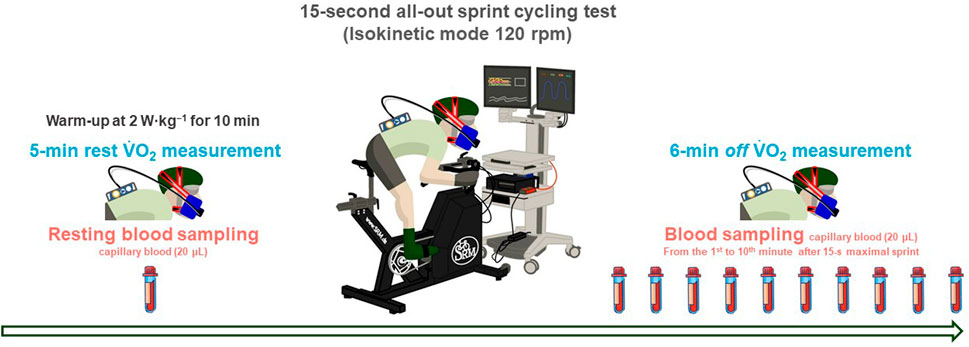
FIGURE 1. Study procedure. An initial warm-up was performed for 10 min at 2 W·kg−1. A further passive rest for 5 min followed. A 15-s all-out sprint cycling test was performed in the isokinetic mode set to a cadence of 120 rpm. Participants performed the test in the sitting position on the ergometer. Oxygen uptake at 5-min rest, and during as well as up to 6 min after 15-s all-out sprint cycling test was measured. Capillary blood was collected from the earlobe (20 μL) before and in minutes' interval (1st to 10th) after the 15-s all-out sprint cycling test.
Calculations of energetic contributions (PCr-La−-O2 method)
Phosphagen (WPCr), glycolytic (WGly), and oxidative (WOxi) contributions in kilojoules, and their percentages were calculated by measuring
WPCr was calculated using
The WGly calculation was performed by determining La− before and after the 15-s ASCT (Larest and Lamax), assuming that the production of 1 mmol·L−1 was equivalent to 3 mL O2·kg−1 of body mass (di Prampero and Ferretti, 1999). The difference in La− (∆La−) was determined by La− after the 15-s ASCT minus La− before the 15-s ASCT (Beneke et al., 2004; Campos et al., 2012; Yang et al., 2018; Yang et al., 2022b).
The WOxi contribution was calculated by subtracting
Different formulas of νLa.max calculations
νLa.max (tPCr −3.5%) was calculated as ∆La− between Larest and Lamax, which was divided by the difference between the total exercise time (tExer) and the −3.5% time point from the peak watt (tPCr −3.5%) (Mader, 1984; Weber, 2003) (Eq. 1):
Furthermore, only tPCr was differently determined according to previous studies (Serresse et al., 1988; Beneke et al., 2002; Manunzio et al., 2016) (Eq. 2):
The accuracy of the latest SRM cycle ergometer (power meter in the science system) is reported to be ± 0.5–1% according to the manufacturer and previous studies (Bouillod et al., 2017; Nimmerichter et al., 2017; Montalvo-Pérez et al., 2021). Therefore, a representation of tPCr−peak was determined as the time until Wpeak during the 15-s ASCT because energy was influenced by the ATP-PCr system until peak power during the initial seconds of a maximal short-term exercise (Serresse et al., 1988; Beneke et al., 2002).
A modified pure νLa.max was calculated as ∆La− divided by the difference between tExer and tPCr−peak plus relative WOxi in percentage of the PCr-La−-O2 method during the 15-s ASCT. This percentage was converted to time in seconds (tOxi) related to the total ASCT time (Eq. 3):
Statistical analyses
All collected data were analyzed using GraphPad Prism 9.4.1. (GraphPad Prism Software Inc., La Jolla, CA, United States). The data of collected parameters are presented as mean ± standard deviation (SD). The normal distribution of all data was performed using the Shapiro−Wilk test. The contributions of the three energy systems and different νLa.max values were compared using a repeated-measures analysis of variance (ANOVA) with the Bonferroni post hoc test. The Mauchly’s sphericity test was used to determine whether the assumption of sphericity was being violated by the data. The Greenhouse−Geisser correction was required when necessary. A comparison between tPCr −3.5% and tPCr−peak was conducted using a paired t-test. The significance level was set at p < 0.05. The effect sizes (partial eta squared [
Results
Energetic contributions during 15-s ASCT
The repeated-measures ANOVA indicated significant differences in energetic contributions in kilojoules and their percentages (p < 0.0001, effect size [
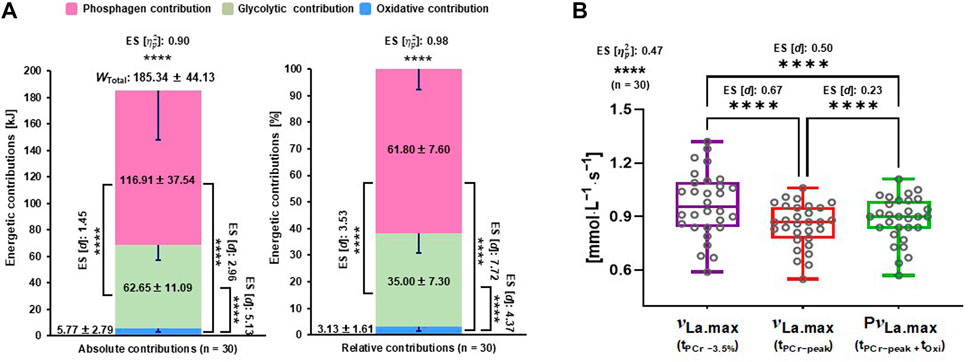
FIGURE 2. Energetic contributions and different calculations of νLa.max. (A) Comparisons of three energy contributions in kilojoules and percentage, and (B) comparisons of three different νLa.max calculations. ES, effect sizes; νLa.max, maximal rate of lactate accumulation; PνLa.max, pure maximal rate of lactate accumulation; tOxi, time of oxidative energy system contribution; tPCr−peak, phosphagen energy system–contributed time until the peak power output; tPCr −3.5%, phosphagen energy system contributed −3.5% time point from the peak power output; WTotal, total energy demand. Significant difference; ****p < .0001.
Physiological parameters and relationships between different calculations of νLa.max
The time duration (s) of the νLa.max-related tPCr −3.5% was significantly higher than tPCr−peak (p < 0.0001, [d]: 1.42; Table 1). The data of the other physiological parameters of anaerobic performance are presented as mean ± SD in Table 1.
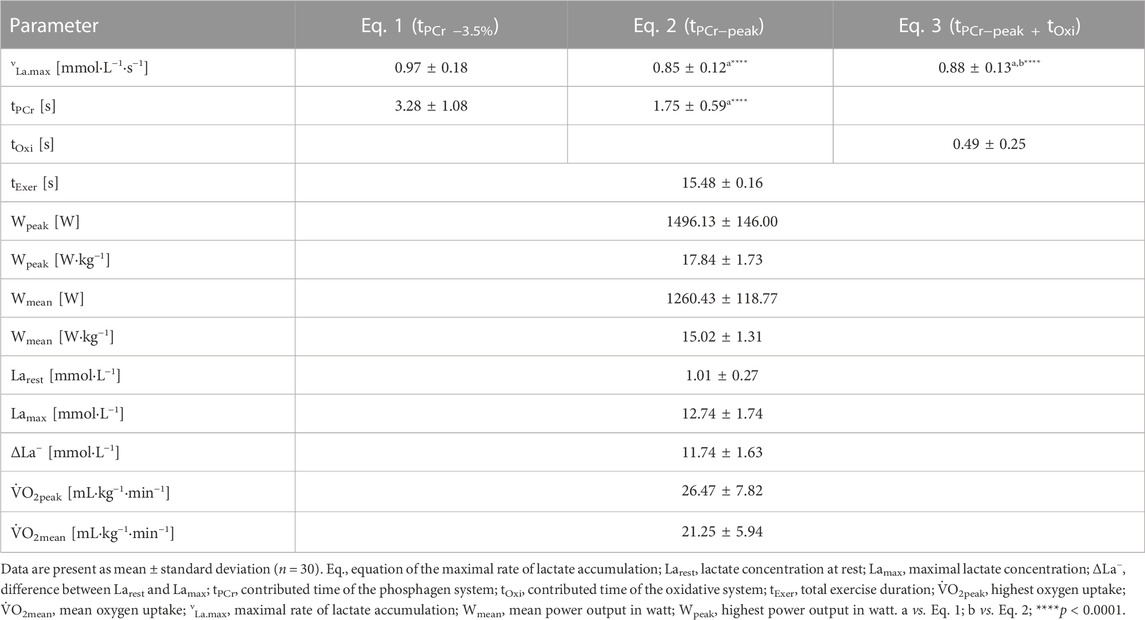
TABLE 1. Anaerobic performances and physiological parameters during a 15-s all-out sprint cycling test.
The repeated-measures ANOVA showed significant differences of the three νLa.max values (p < 0.0001, [
A very strong correlation was found between PνLa.max and νLa.max (tPCr−peak) (r = 0.99; R2 = 0.98; 95% confidence interval [CI]: 0.98–0.99; p < 0.0001) (Figure 3A). A further high positive correlation between PνLa.max and νLa.max (tPCr −3.5%) was observed (r = 0.87; R2 = 0.77; 95% CI: 0.75–0.94; p < 0.0001) (Figure 3B). The Bland–Altman analysis showed that the bias between PνLa.max and νLa.max (tPCr−peak) calculations was 0.031 ± 0.017. Another bias between νLa.max (tPCr −3.5%) and PνLa.max calculations was 0.087 ± 0.092. PνLa.max and νLa.max (tPCr−peak) calculations indicated a smaller 95% limit of agreement (−0.002 to 0.066) followed by νLa.max (tPCr −3.5%) and PνLa.max (−0.095 to 0.269) (Figures 3C, D).
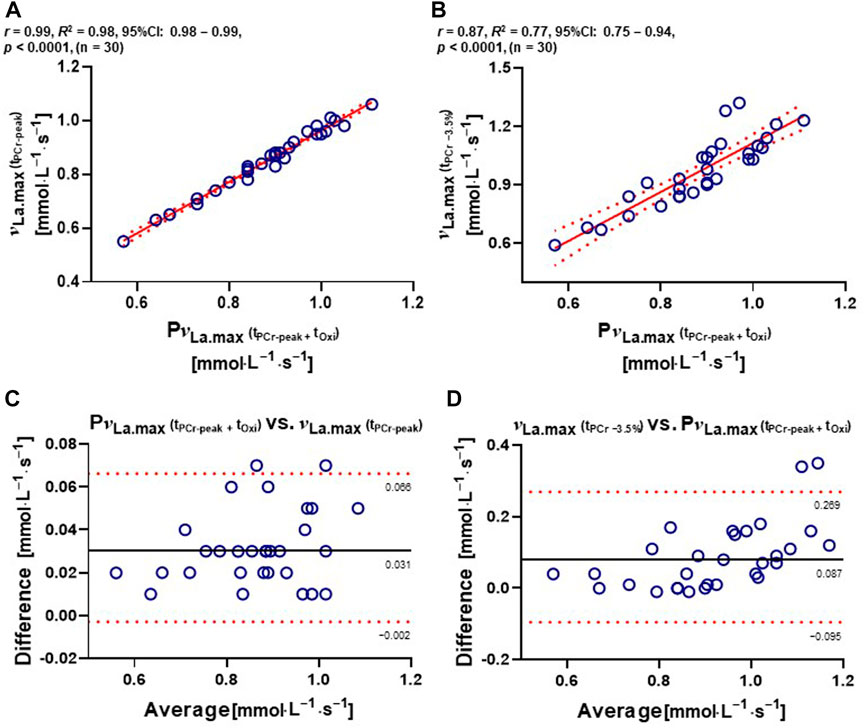
FIGURE 3. Relationships and Bland–Altman plots between different calculations of νLa.max. (A) Two-tailed Pearson’s correlation between PνLa.max and νLa.max (tPCr−peak). (B) Two-tailed Pearson’s correlation between PνLa.max and νLa.max (tPCr −3.5%). (C and D) Bland–Altman plots of differences between PνLa.max and νLa.max (tPCr−peak) and between νLa.max (tPCr −3.5%) and PνLa.max. The dashed line represents bias. The dotted line represents 95% limit of agreements. CI, confidence interval; ES, effect sizes; νLa.max, maximal rate of lactate accumulation; PνLa.max, pure maximal rate of lactate accumulation; tOxi, time of oxidative energy system contribution; tPCr−peak, phosphagen energy system–contributed time until the peak power output; tPCr −3.5%, phosphagen energy system contributed −3.5% time point from the peak power output. Significant difference; ****p < .0001.
Relationships between anaerobic performance and different νLa.max
Low positive correlations between νLa.max (tPCr−peak) and absolute Wmean, PνLa.max and absolute Wmean, and νLa.max (tPCr −3.5%) and absolute Wmean were observed (r = 0.48, R2 = 0.23, 95% CI: 0.14–0.72, and p = 0.0075; r = 0.45, R2 = 0.21, 95% CI: 0.11–0.70, and p = 0.0120; r = 0.43, R2 = 0.19, 95% CI: 0.08–0.69, and p = 0.0173, respectively) (Figures 4A−C). Furthermore, moderate to high positive correlations between νLa.max (tPCr−peak) and WGly, PνLa.max and WGly, and νLa.max (tPCr −3.5%) and WGly in kilojoules were found (r = 0.73, R2 = 0.53, 95% CI: 0.49–0.86, and p < 0.0001; r = 0.70, R2 = 0.49, 95% CI: 0.46–0.85, and p < 0.0001; r = 0.61, R2 = 0.37, 95% CI: 0.31–0.79, and p = 0.0004, respectively) (Figures 4D−F).
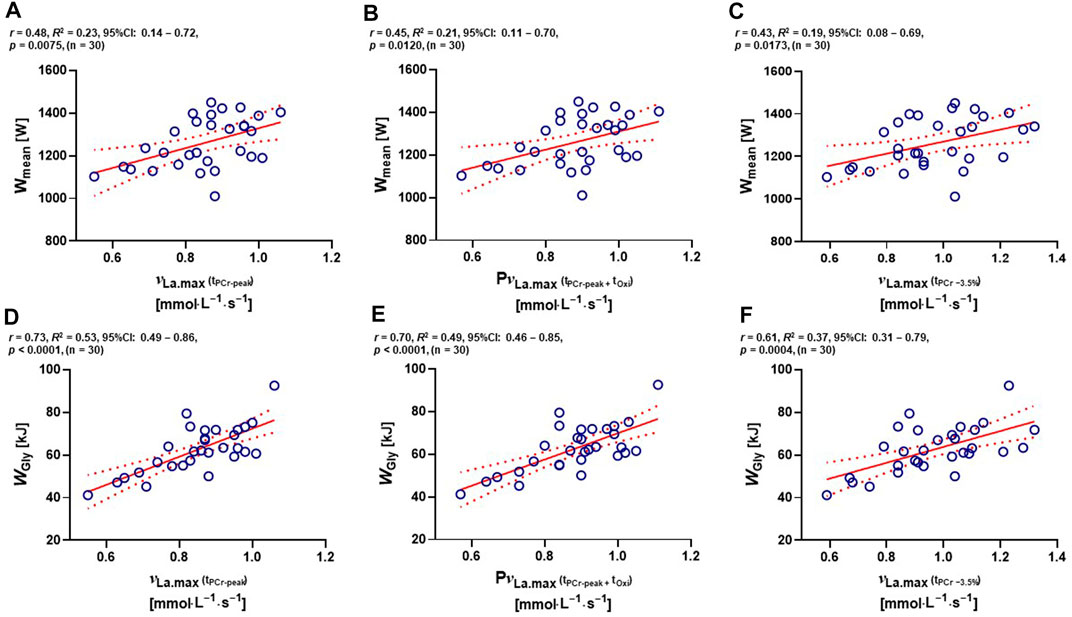
FIGURE 4. Two-tailed Pearson’s correlations (A) between νLa.max (tPCr−peak) and Wmean, (B) between PνLa.max and absolute Wmean, (C) between νLa.max (tPCr −3.5%) and absolute Wmean, (D) between νLa.max (tPCr−peak) and absolute WGly, (E) between PνLa.max and absolute WGly, and (F) between νLa.max (tPCr −3.5%) and absolute WGly. CI, confidence interval; νLa.max, maximal rate of lactate accumulation; PνLa.max, pure maximal rate of lactate accumulation; tOxi, time of oxidative energy system contribution; tPCr−peak, phosphagen energy system contribution time until peak power output; tPCr −3.5%, phosphagen energy system contribution at −3.5% time point from peak power output; WGly, absolute glycolytic energy contribution in kilohoules; Wmean, absolute mean power output in watt.
Discussion
In previous studies, calculations of νLa.max for the maximal glycolytic capacity after the 15-s ASCT were limited because tPCr and aerobic energy contribution were not considered. To the best of our knowledge, this study is the first to integrate the oxidative energy contribution and contributions of the three energy systems during the 15-s ASCT via a modified PνLa.max calculation. This modified PνLa.max was compared with previous νLa.max calculations for glycolytic performance and their correlations were determined.
The main findings of this study confirm that phosphagen and glycolytic systems are predominantly utilized, while the oxidative energy metabolism contributes to 3.13% ± 1.61% during the 15-s ASCT. The tPCr−peak and tOxi- subtracted PνLa.max values were lower than νLa.max (tPCr −3.5%) but higher than νLa.max (tPCr−peak) values. Moreover, very strong and high correlations between PνLa.max and νLa.max (tPCr−peak) and between PνLa.max and νLa.max (tPCr −3.5%) were found. Three different νLa.max values were positively associated with absolute Wmean and WGly values.
The phosphagen energy system has the highest absolute energetic contribution during the 15-s ASCT, followed by glycolytic and oxidative energy metabolism, thus being in agreement with the results of a previous study by Park et al. (2021). In this study, all three energy systems were analyzed using the same PCr-La−-O2 method during a 100-m sprint and the oxidative energy system contributed over 10% here to the total energy requirements. In comparison to this outcome, the oxidative contribution in the present study was lower (∼3%) during the 15-s ASCT (Figure 2A). These different results might be because 100-m sprint and sprint cycling are differently characterized by maximal anaerobic performances, which include different movements of the upper extremities. Previous studies have reported that smaller muscles such as those in the upper extremities do not significantly contribute to total lactate accumulation (Julio et al., 2017; Yang et al., 2022b). Therefore, glycolytic energy contribution during the 15-s ASCT likely had a higher percentage (35% ± 7%) than during a 100-m sprint (∼25 ± 7%) as obtained in a previous study outcome (Park et al., 2021), which in turn reduced the relative oxidative contribution.
Regarding the different calculations of νLa.max, the level of νLa.max (tPCr −3.5%) showed significantly higher values than νLa.max (tPCr−peak) and PνLa.max (Figure 2B) because the tPCr −3.5% value calculated was 3.2 ± 1.0 s during the 15-s ASCT (Table 1) such that the denominator (∆time) of the formula was smaller than the others. Due to the same reason, in including tPCr−peak + tOxi, PνLa.max showed higher values than νLa.max (tPCr−peak). According to our results, the calculation of νLa.max (tPCr −3.5%) was unsuitable to determine the glycolytic capacity or νLa.max because the determined tPCr time of −3.5% from Wpeak was based on an error of the early SRM cycle ergometer (Weber, 2003). On the other hand, previous researchers suggested no lactate production during tPCr which was incorrectly (fictitiously) assumed (Heck et al., 2003; Hauser et al., 2014; Adam et al., 2015; Nitzsche et al., 2018; Quittmann et al., 2020; Quittmann et al., 2021). In this regard, it is well known that all three energy systems start to work simultaneously and lactate production occurs independently of O2 availability such as under anoxic, hypoxic, and normoxic conditions (Gastin, 2001; Philp et al., 2005; Brooks, 2018; Yang et al., 2020; Brooks et al., 2022; Yang et al., 2022b). La− might be accumulated at a relatively low level during the initial seconds of the 15-s ASCT because ATP-PCr is a dominant energy contribution until the achievement of Wpeak (Serresse et al., 1988; Beneke et al., 2002; Park et al., 2021). Moreover, Heck et al. (2003) have suggested that aerobic power and contribution are of minor significance during maximal short-term exercise testing. However, this interpretation is based on the results of a computer simulation that originated from systems biology (Wackerhage et al., 2022). The calculated νLa.max values with and without tOxi show significant differences in our study's outcomes (Table 1; Figure 2B). Therefore, oxidative metabolism should be considered when aiming to analyze a pure maximal glycolytic rate. Considering the abovementioned factors, the accuracies of νLa.max (tPCr−peak) and PνLa.max calculations seem to be higher because the durations of tPCr−peak and tOxi are additionally involved in calculating formulas by subtracting them from the total exercise time. Accordingly, PνLa.max was very strongly correlated with νLa.max (tPCr−peak), and this correlation was higher than that of PνLa.max with νLa.max (tPCr −3.5%). The Bland–Altman plot between PνLa.max and νLa.max (tPCr−peak) also showed a smaller limit of agreement than the limit of agreement between νLa.max (tPCr −3.5%) and PνLa.max (Figures 3C, D). Furthermore, PνLa.max and νLa.max (tPCr−peak) were associated with absolute Wmean and WGly. Such associations were higher than those of νLa.max (tPCr −3.5%), with absolute Wmean and WGly (Figures 4A−F). These results indicate that PνLa.max and νLa.max (tPCr−peak) are better calculating methods for glycolytic capacity/power.
To determine the anaerobic performance, the 30-s Wingate anaerobic test is often used in related studies (Bar-Or, 1987; Gastin, 2001; Zupan et al., 2009; Jaafar et al., 2014). However, this time frame will likely increase the oxidative contribution to the total energy demand and may also lead to an inhibition of phosphofructokinase activity because of accumulated hydrogen ions from increased ATP hydrolysis and reduced pH-levels (Gastin, 2001; Heck et al., 2003; Robergs et al., 2004; Yang et al., 2022b). This increased oxidative contribution may then reduce lactate accumulation and increase lactate elimination, which results in a reduced likelihood of measuring the maximal lactate accumulation (Heck et al., 2003; Yang et al., 2020; Yang et al., 2022b; Wackerhage et al., 2022). Thus, the νLa.max determination combining the 15-s ASCT with the PνLa.max formula using the PCr-La−-O2 method is more suitable to limit the activation of oxidative metabolism as much as possible in decreasing intracellular lactate oxidation (Heck et al., 2003; Wackerhage et al., 2022).
In the current study, the levels of νLa.max (tPCr −3.5%) were higher than those of PνLa.max and νLa.max (tPCr−peak), while a very strong relationship between PνLa.max and νLa.max (tPCr−peak) was observed, one that was better than the relationship between PνLa.max and νLa.max (tPCr −3.5%). Therefore, the calculation of PνLa.max using the analysis of energetic contributions should be considered in scientific research to precisely analyze the maximal rate of lactate accumulation and anaerobic capacity/power. In practical diagnostics, the calculation of νLa.max (tPCr−peak) may be useful without a complicated analysis of energetic contributions or if it is not possible to be available for gas analyzer equipment eventually. Otherwise, the use of νLa.max (tPCr −3.5%) calculation is only suggested if the early version of the SRM cycle ergometer is available to perform the 15-s ASCT.
This study successfully identified the differences of three possible νLa.max calculations during the 15-s ASCT. However, the current study has some limitations. Because a spiroergometry system processing a dynamic micro-mixing chamber mode was not available during the test, the
Regarding the possible gender differences in energetic contributions, Yang et al. (2022a) recently calculated the energy system contributions during increasing exercise intensities. The results found different WPCr and WGly levels between males and females that were attributed to the differences in skeletal muscle mass and all fiber types. Therefore, there may be a need for further research into gender differences in the PνLa.max calculation. Furthermore, the distribution of muscle fiber types might be considered when interpreting high or low νLa.max values in individual athletes with higher muscle mass and more glycolytic type IIA and IIX fibers who show generally higher lactate production during aerobic and anaerobic exercises (Ivy et al., 1980; Wackerhage et al., 2022). Future studies must show to what extent νLa.max might shift during a training year in track athletes with a different νLa.max.
Moreover, there are currently no clear data available about which specific individual training sessions are helpful in improving νLa.max. A recent study by Schünemann et al. (2023) suggests that sprint training, resistance training, and hypoxic training may increase glycolytic capacity and anaerobic performance. However, direct interventional approaches related to improving maximal glycolytic metabolism as PνLa.max should be investigated further.
Conclusion
The findings of the current study indicate that a modified calculation of PνLa.max with the additional incorporation of further energetic contributions is precision enhancing and therefore valuable for determining the maximal glycolytic rate or νLa.max. Our modified calculation therefore allows for a more detailed input of individual characteristics of energy metabolism to becoming an increasingly popular method for determining differences in maximal rates of lactate accumulation. In light of inter-individual differences in maximal force generation, phosphagen levels, and oxidative capacity, our method allows for compensating this interaction during νLa.max measurements when compared to previous methods. According to our results, the calculated formula of PνLa.max should be used for scientific research in exercise sciences to determine the maximal anaerobic capacity/power more precisely and with the exclusion of the aerobic component. This is supported by a small limit of agreement (a small variation) and very high correlation with νLa.max (tPCr−peak). Furthermore, the calculation of νLa.max (tPCr−peak) might be recommended for a faster analysis of the practical approach in the field.
Data availability statement
The original contributions presented in the study are included in the article/Supplementary Material; further inquiries can be directed to the corresponding authors.
Ethics statement
The studies involving human participants were reviewed and approved by the Institutional Review Board of CHA University, along with this study (No. 1044308-202206-HR-032-02). The patients/participants provided their written informed consent to participate in this study.
Author contributions
W-HY, S-YP, TK, H-JJ, OH, and SG were involved in the conception and design of the research. W-HY, S-YP, TK, and H-JJ collected the data. W-HY and S-YP analyzed the data. W-HY wrote the first draft of the manuscript. W-HY, OH, and SG revised and edited the manuscript. All authors approved the final manuscript.
Acknowledgments
The authors would like to thank all participants for participating in this study.
Conflict of interest
The authors declare that the research was conducted in the absence of any commercial or financial relationships that could be construed as a potential conflict of interest.
Publisher’s note
All claims expressed in this article are solely those of the authors and do not necessarily represent those of their affiliated organizations, or those of the publisher, editors, and reviewers. Any product that may be evaluated in this article, or claim that may be made by its manufacturer, is not guaranteed or endorsed by the publisher.
References
Adam J., Öhmichen M., Öhmichen E., Rother J., Müller U. M., Hauser T., et al. (2015). Reliability of the calculated maximal lactate steady state in amateur cyclists. Biol. Sport 32 (2), 97–102. doi:10.5604/20831862.1134311
Bar-Or O. (1987). The Wingate anaerobic test. An update on methodology, reliability and validity. Sports Med. 4 (6), 381–394. doi:10.2165/00007256-198704060-00001
Beneke R., Beyer T., Jachner C., Erasmus J., Hütler M. (2004). Energetics of karate kumite. Eur. J. Appl. Physiol. 92 (4-5), 518–523. doi:10.1007/s00421-004-1073-x
Beneke R., Pollmann C., Bleif I., Leithäuser R. M., Hütler M. (2002). How anaerobic is the wingate anaerobic test for humans? Eur. J. Appl. Physiol. 87 (4-5), 388–392. doi:10.1007/s00421-002-0622-4
Bland J. M., Altman D. G. (1995). Comparing methods of measurement: Why plotting difference against standard method is misleading. Lancet 346 (8982), 1085–1087. doi:10.1016/s0140-6736(95)91748-9
Bouillod A., Pinot J., Soto-Romero G., Bertucci W., Grappe F. (2017). Validity, sensitivity, reproducibility, and robustness of the PowerTap, stages, and garmin vector power meters in comparison with the SRM device. Int. J. Sports Physiol. Perform. 12 (8), 1023–1030. doi:10.1123/ijspp.2016-0436
Brooks G. A. (2018). The science and translation of lactate shuttle theory. Cell Metab. 27 (4), 757–785. doi:10.1016/j.cmet.2018.03.008
Brooks G. A., Curl C. C., Leija R. G., Osmond A. D., Duong J. J., Arevalo J. A. (2022). Tracing the lactate shuttle to the mitochondrial reticulum. Exp. Mol. Med. 54 (9), 1332–1347. doi:10.1038/s12276-022-00802-3
Campos F. A. D., Bertuzzi R., Dourado A. C., Santos V. G. F., Franchini E. (2012). Energy demands in taekwondo athletes during combat simulation. Eur. J. Appl. Physiol. 112 (4), 1221–1228. doi:10.1007/s00421-011-2071-4
de Campos Mello F., de Moraes Bertuzzi R. C., Grangeiro P. M., Franchini E. (2009). Energy systems contributions in 2,000 m race simulation: A comparison among rowing ergometers and water. Eur. J. Appl. Physiol. 107 (5), 615–619. doi:10.1007/s00421-009-1172-9
de Moraes Bertuzzi R. C., Franchini E., Kokubun E., Kiss M. A. P. D. M. (2007). Energy system contributions in indoor rock climbing. Eur. J. Appl. Physiol. 101 (3), 293–300. doi:10.1007/s00421-007-0501-0
di Prampero P. E., Ferretti G. (1999). The energetics of anaerobic muscle metabolism: A reappraisal of older and recent concepts. Respir. Physiol. 118 (2), 103–115. doi:10.1016/s0034-5687(99)00083-3
Dunst A. K., Hesse C., Ueberschär O., Holmberg H. C. (2023). A novel approach to the determination of time- and fatigue-dependent efficiency during maximal cycling sprints. Sports (Basel) 11 (2), 29. doi:10.3390/sports11020029
Foster C., Snyder A. C., Thompson N. N., Green M. A., Foley M., Schrager M. (1993). Effect of pacing strategy on cycle time trial performance. Med. Sci. Sports Exerc 25 (3), 383–388. doi:10.1249/00005768-199303000-00014
Franchini E. (2023). Energy system contributions during olympic combat sports: A narrative Review. Metabolites 13 (2), 297. doi:10.3390/metabo13020297
Franchini E. (2020). High-intensity interval training prescription for combat-sport athletes. Int. J. Sports Physiol. Perform. 15 (6), 767–776. doi:10.1123/ijspp.2020-0289
Fritz C. O., Morris P. E., Richler J. J. (2012). Effect size estimates: Current use, calculations, and interpretation. J. Exp. Psychol. Gen. 141 (1), 2–18. doi:10.1037/a0024338
Fujii N., Nishida Y., Ogawa T., Tanigawa S., Nishiyasu T. (2018). Effects of work-matched moderate- and high-intensity warm-up on power output during 2-min supramaximal cycling. Biol. Sport 35 (3), 223–228. doi:10.5114/biolsport.2018.74633
Gastin P. B. (2001). Energy system interaction and relative contribution during maximal exercise. Sports Med. 31 (10), 725–741. doi:10.2165/00007256-200131100-00003
Hausen M., Soares P. P., Araújo M. P., Porto F., Franchini E., Bridge C. A., et al. (2017). Physiological responses and external validity of a new setting for taekwondo combat simulation. PloS One 12 (2), e0171553. doi:10.1371/journal.pone.0171553
Hauser T., Adam J., Schulz H. (2014). Comparison of calculated and experimental power in maximal lactate-steady state during cycling. Theor. Biol. Med. Model 11, 25–12. doi:10.1186/1742-4682-11-25
Heck H., Schulz H., Bartmus U. (2003). Diagnostics of anaerobic power and capacity. Eur. J. Sport Sci. 3 (3), 1–23. doi:10.1080/17461390300073302
Hettinga F. J., De Koning J. J., Meijer E., Teunissen L., Foster C. (2007). Biodynamics. Effect of pacing strategy on energy expenditure during a 1500-m cycling time trial. Med. Sci. Sports Exerc 39 (12), 2212–2218. doi:10.1249/mss.0b013e318156e8d4
Ivy J. L., Withers R. T., Van Handel P. J., Elger D. H., Costill D. L. (1980). Muscle respiratory capacity and fiber type as determinants of the lactate threshold. J. Appl. Physiol. Respir. Environ. Exerc Physiol. 48 (3), 523–527. doi:10.1152/jappl.1980.48.3.523
Jaafar H., Rouis M., Coudrat L., Attiogbé E., Vandewalle H., Driss T. (2014). Effects of load on wingate test performances and reliability. J. Strength Cond. Res. 28 (12), 3462–3468. doi:10.1519/jsc.0000000000000575
Julio U. F., Panissa V. L. G., Esteves J. V., Cury R. L., Agostinho M. F., Franchini E. (2017). Energy-system contributions to simulated judo matches. Int. J. Sports Physiol. Perform. 12 (5), 676–683. doi:10.1123/ijspp.2015-0750
Kaufmann S., Latzel R., Beneke R., Hoos O. (2022). Reliability of the 3-component model of aerobic, anaerobic lactic, and anaerobic alactic energy distribution (PCr-la-O2) for energetic profiling of continuous and intermittent exercise. Int. J. Sports Physiol. Perform. 17, 1642–1648. doi:10.1123/ijspp.2022-0115
Lopes-Silva J. P., Da Silva Santos J. F., Artioli G. G., Loturco I., Abbiss C., Franchini E. (2018). Sodium bicarbonate ingestion increases glycolytic contribution and improves performance during simulated taekwondo combat. Eur. J. Sport Sci. 18, 431–440. doi:10.1080/17461391.2018.1424942
Mader A. (1984). Eine Theorie zur Berechnung der Dynamik und des steady state von Phosphorylierungszustand und Stoffwechselaktivität der Muskelzelle als Folge des Energiebedarfs. Cologne: German Sport University Cologne.
Manunzio C., Mester J., Kaiser W., Wahl P. (2016). Training intensity distribution and changes in performance and Physiology of a 2nd place finisher team of the race across America over a 6 Month preparation period. Front. Physiol. 7, 642. doi:10.3389/fphys.2016.00642
Montalvo-Pérez A., Alejo L. B., Valenzuela P. L., Castellanos M., Gil-Cabrera J., Talavera E., et al. (2021). Validity of the favero assioma duo power pedal system for measuring power output and cadence. Sensors 21 (7), 2277. doi:10.3390/s21072277
Nimmerichter A., Schnitzer L., Prinz B., Simon D., Wirth K. (2017). Validity and reliability of the garmin vector power meter in laboratory and field cycling. Int. J. Sports Med. 38 (6), 439–446. doi:10.1055/s-0043-101909
Nitzsche N., Baumgärtel L., Maiwald C., Schulz H. (2018). Reproducibility of blood lactate concentration rate under isokinetic force loads. Sports 6 (4), 150. doi:10.3390/sports6040150
Park S. B., Park D. S., Kim M., Lee E., Lee D., Jung J., et al. (2021). High-intensity warm-up increases anaerobic energy contribution during 100-m sprint. Biology 10 (3), 198. doi:10.3390/biology10030198
Philp A., Macdonald A. L., Watt P. W. (2005). Lactate–a signal coordinating cell and systemic function. J. Exp. Biol. 208 (24), 4561–4575. doi:10.1242/jeb.01961
Quittmann O. J., Appelhans D., Abel T., Strüder H. K. (2020). Evaluation of a sport-specific field test to determine maximal lactate accumulation rate and sprint performance parameters in running. J. Sci. Med. Sport 23 (1), 27–34. doi:10.1016/j.jsams.2019.08.013
Quittmann O. J., Schwarz Y. M., Mester J., Foitschik T., Abel T., Strüder H. K. (2021). Maximal lactate accumulation rate in all-out exercise differs between cycling and running. Int. J. Sports Med. 42 (4), 314–322. doi:10.1055/a-1273-7589
Robergs R. A., Ghiasvand F., Parker D. (2004). Biochemistry of exercise-induced metabolic acidosis. Am. J. Physiol. Regul. Integr. Comp. Physiol. 287 (3), R502–R516. doi:10.1152/ajpregu.00114.2004
Schünemann F., Park S.-Y., Wawer C., Theis C., Yang W.-H., Gehlert S. (2023). Diagnostics of νLa. Max and glycolytic energy contribution indicate individual characteristics of anaerobic glycolytic energy metabolism contributing to rowing performance. Metabolites 13 (3), 317. doi:10.3390/metabo13030317
Serresse O., Lortie G., Bouchard C., Boulay M. R. (1988). Estimation of the contribution of the various energy systems during maximal work of short duration. Int. J. Sports Med. 9 (6), 456–460. doi:10.1055/s-2007-1025051
Wackerhage H., Gehlert S., Schulz H., Weber S., Ring-Dimitriou S., Heine O. (2022). Lactate thresholds and the simulation of human energy metabolism: Contributions by the cologne sports medicine group in the 1970s and 1980s. Front. Physiol. 13, 899670. doi:10.3389/fphys.2022.899670
Weber S. (2003). Berechnung leistungsbestimmender Parameter der metabolischen Aktivität auf zellulärer Ebene mittels fahrradergometrischer Untersuchungen. Dipl.-Thesis. Cologne: German Sport University Cologne.
Yang W.-H., Heine O., Grau M. (2018). Rapid weight reduction does not impair athletic performance of Taekwondo athletes–A pilot study. PloS One 13 (4), e0196568. doi:10.1371/journal.pone.0196568
Yang W.-H., Park H., Grau M., Heine O. (2020). Decreased blood glucose and lactate: Is a useful indicator of recovery ability in athletes? Int. J. Environ. Res. Public Health 17 (15), 5470. doi:10.3390/ijerph17155470
Yang W.-H., Park J.-H., Park S.-Y., Park Y. (2022a). Energetic contributions including gender differences and metabolic flexibility in the general population and athletes. Metabolites 12 (10), 965–1014. doi:10.3390/metabo12100965
Yang W.-H., Park J.-H., Shin Y.-C., Kim J. (2022b). Physiological profiling and energy system contributions during simulated epée matches in elite fencers. Int. J. Sports Physiol. Perform. 17 (6), 943–950. doi:10.1123/ijspp.2021-0497
Keywords: anaerobic performance, diagnostics, glycolytic metabolism, lactate, anaerobic power output
Citation: Yang W-H, Park S-Y, Kim T, Jeon H-J, Heine O and Gehlert S (2023) A modified formula using energy system contributions to calculate pure maximal rate of lactate accumulation during a maximal sprint cycling test. Front. Physiol. 14:1147321. doi: 10.3389/fphys.2023.1147321
Received: 18 January 2023; Accepted: 27 March 2023;
Published: 13 April 2023.
Edited by:
Silvia Pogliaghi, University of Verona, ItalyReviewed by:
Leonardo Henrique Dalcheco Messias, Sao Francisco University, BrazilKazushige Goto, Ritsumeikan University, Japan
Copyright © 2023 Yang, Park, Kim, Jeon, Heine and Gehlert. This is an open-access article distributed under the terms of the Creative Commons Attribution License (CC BY). The use, distribution or reproduction in other forums is permitted, provided the original author(s) and the copyright owner(s) are credited and that the original publication in this journal is cited, in accordance with accepted academic practice. No use, distribution or reproduction is permitted which does not comply with these terms.
*Correspondence: Woo-Hwi Yang, eXdoMTIzNUBjaGEuYWMua3I=; Sebastian Gehlert, Z2VobGVydEB1bmktaGlsZGVzaGVpbS5kZQ==
†ORCID ID: Woo-Hwi Yang, orcid.org/0000-0001-6618-1834; So-Young Park, orcid.org/0000-0002-1871-8366; Sebastian Gehlert, orcid.org/0000-0001-6940-3084