- 1Henan University School of Stomatology, Kaifeng, China
- 2The Key Laboratory of Clinical Resources Translation, Henan University, Kaifeng, China
- 3The First Affiliated Hospital of Henan University, Kaifeng, China
Objectives: The aim of the present study was to develop a machine learning model to predict the risk of molar incisor hypomineralization (MIH) and to identify factors associated with MIH in an endemic fluorosis region in central China.
Methods: A cross-sectional study was conducted with 1,568 schoolchildren from selected regions. The clinical examination included an investigation of MIH based on the European Academy of Paediatric Dentistry (EAPD) criteria. In this study, supervised machine learning (e.g., logistic regression) and correlation analysis (e.g., Spearman correlation analysis) were used for classification and prediction.
Results: The overall prevalence of MIH was 13.7%. The nomograph showed that non-dental fluorosis (DF) had a considerable influence on the early occurrence of MIH and that this influence became weaker as DF severity increased. We examined the association between MIH and DF and found that DF had a protective correlation with MIH; the protective effect became stronger as DF severity increased. Furthermore, children with defective enamel were more likely to experience caries, and dental caries were positively correlated with MIH (OR = 1.843; 95% CI: 1.260–2.694). However, gender, oral hygiene, and exposure to poor-quality shallow underground water did not increase the likelihood of developing MIH.
Conclusions: DF should be considered a protective factor within the multifactorial etiology of MIH.
1 Introduction
The European Academy of Paediatric Dentistry (EAPD) defines molar incisor hypomineralization (MIH) as enamel mineralization defects in one to four permanent first molars, with or without the involvement of the permanent incisors (Weerheijm et al., 2003). MIH-affected teeth clinically display demarcated opacity on the occlusal or buccal surfaces of the crowns (da Costa-Silva et al., 2010; Jeremias et al., 2013). MIH may be confused with dental fluorosis (DF), which shows diffuse opacity when the same teeth are affected. To date, researchers have performed many studies on the prevalence of MIH, but the study of MIH in endemic fluorosis regions is very limited.
The correlation between MIH and DF remains unclear. Studies have indicated that the presence of naturally fluoridated waters does not increase the incidence of MIH (Balmer et al., 2012; Schmalfuss et al., 2016); however, the severity of MIH is likely to be associated with DF (Fernandes et al., 2021). Significantly, the prevalence rate of MIH was lower in fluoridated areas of Northern England than in non-fluoridated areas (Balmer et al., 2012). A similar situation existed in Brazil, where a significant negative association between MIH and DF at the tooth level has been observed (Duarte et al., 2021). Therefore, it is important to study the relationship between MIH and DF, as well as the severity of MIH and DF.
Recently, machine learning methods have been used to predict a variety of diseases. Machine learning methods may be used to overcome some limitations of current analytical approaches and to find associations by applying computer algorithms to large datasets with numerous, multidimensional variables, capturing high-dimensional relationships among clinical features to obtain data-driven outcomes (Schwalbe and Wahl, 2020). Thus, we sought to develop a machine learning-based risk stratification model to explore the risk of MIH in an endemic fluorosis region in central China.
The aim of this study was to determine the prevalence of MIH and to predict its occurrence by utilizing machine learning and to explore the association between MIH and dental fluorosis in children living in an endemic fluorosis region in central China.
2 Material and methods
2.1 Ethical considerations and sample
The present study was performed with the approval of the Medical Ethics Committee of The First Affiliated Hospital of Henan University (2019LCSY-002). Signed informed consent was obtained from the caregivers and children prior to their participation in the study.
A cross-sectional study was conducted from April to June 2021 with a representative sample of schoolchildren aged 8 and 10 years in Lankao County, which is located on the eastern boundary of Henan Province. This county has endemic fluorosis, with fluoride concentrations ranging from 1.22 to 3.90 mg/L (Wang et al., 2008; Wang and Cao, 2013), which exceed the standard for drinking water quality in China (1.0 mg/L; GB5749-2006).
The formula for calculating a minimum number of randomly selected children was as follows: sample size (n) = [Z2×P(1-P)]/d2, where Z is the statistical level of confidence for a 95% confidence interval (CI; Z = 1.96), P is the expected prevalence, and d is the precision (Naing et al., 2006). Recent studies have revealed that the global average prevalence of MIH is 12.9% (11.7%–14.3%) (Schwendicke et al., 2018). According to the formula, this study required 169 participants. Schools were selected randomly according to the number of schools in each town, and a stratified sample of pupils was selected from each school according to the total number of pupils in the school. The inclusion criteria were as follows: residents of both sexes, aged 8–10 years, born and raised locally, with all four permanent first molars and incisors fully erupted. The exclusion criteria were as follows: having no erupted permanent first molar and incisor, undergoing fixed orthodontic treatment with brackets or bands on permanent first molars, and defects less than 1 mm in diameter.
2.2 Training and calibration of examiners
The European Academy of Paediatric Dentistry (EAPD) (Weerheijm et al., 2003) criteria for MIH were used in this study. Calibration exercises were conducted among three MIH investigators using clinical photographs of 26 patients. The tooth defects of patients covered all the degrees of MIH and other enamel defects, such as dental fluorosis, hypoplasia, and amelogenesis. The validity of using clinical photographs to study enamel defects was previously confirmed by Sabieha and Rock (1998), Wong et al. (2006), and Yi et al. (2021). After 1 month of training, three examiners were able to correctly diagnose all cases independently. Cohen’s kappa coefficients for inter- and intra-rater reliability were 0.92 and 0.89 for dental fluorosis, 0.86 and 0.75 for dental caries, and 0.65 and 0.77 for MIH, respectively. Furthermore, during this month, three investigators examined 10 enamel defect patients who visited the Department of Stomatology, which guaranteed that the three investigators were familiar with the diagnosis and management of children with MIH.
2.3 Dental examination
Participants were advised to brush their teeth before the exam, and the teeth stayed slightly wet during the process of inspection. The items to be prepared included a simple dental chair with a dental light source (DYNAMIC, China), disposable oral treatment plates, disposable gloves, and cotton balls.
MIH: Clinical examinations were carried out by unified trained specialist dentists and comprised examination for developmental enamel defects and dental caries using the EAPD criteria (Weerheijm et al., 2003). To guarantee between-examiner reproducibility, the examinations were performed jointly by two dentists. A specially designed chart was used to record sex, the year of birth, the presence of MIH, the number of affected incisors and molars, and the maximum degree of severity. Severity was quantified according to clinical appearance (Ghanim et al., 2017) and was classed as 1) mild, including white and yellow demarcated opacities; or 2) severe, including posteruptive enamel breakdown (PEB), atypical restoration, atypical carious lesions, and missing due to MIH.
Dental fluorosis: The Thysltrup–Fejerskov (TF) criteria were used to determine the occurrence of dental fluorosis with an ordinal scale from 0 to 9 (Thylstrup and Fejerskov, 1978). Based on the loss of structure, teeth with a TF of 0 were classified as normal, those with a TF of 1-4 were classified as mild, and those with a TF greater than 5 were classified as severe.
Dental caries: Clinical dental caries (manifest caries) were recorded as decayed, missing, or filled teeth (DMF). Decay was defined as visible tooth substance loss without the characteristics of developmental defects, pits, or fissures.
Oral hygiene status: Oral hygiene was recorded using the simplified oral hygiene index (OHI-S) described by Greene and Vermillion (1964). The level of oral hygiene was evaluated according to the debris index and was classed as fair (0) or poor (1).
2.4 Databases and data preprocessing
To develop the machine learning models, we used a derivation cohort of children who met the inclusion criteria. The raw dataset contained the study subjects’ demographics and the results of the comprehensive oral examination. Initially, the dataset used for preprocessing and classification was collected. The main characteristics of this dataset included MIH, MIH severity, DF, DF severity, dental decay, oral hygiene, water quality, and sex.
2.5 Machine learning methods and statistical validation strategies
The derivation cohort was randomly split into two datasets: a training cohort (70%) used to train the machine learning model and tune the parameters, and an internal validation cohort (30%) used to test the developed model on unseen data and to fine-tune the hyperparameters. For training, the original data space was balanced by oversampling using SMOTE. It worked by adding small samples from the data space to diminish the biased behavior of imbalanced data, thus changing the size of the training data space. In this study, supervised machine learning (e.g., logistic regression) and correlation analysis (e.g., Spearman correlation analysis) were used for classification and prediction. Once the number of models was considered for this particular study, we used accuracy (ACC), specificity (SPEC), and the ROC curve and area under the curve (AUC) to validate the prediction performance for binary classes (Figure 1).
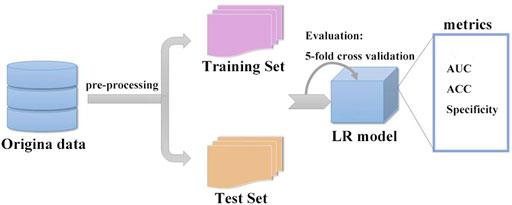
FIGURE 1. Structure of the supervised machine learning algorithm, which can describe this network working principle satisfactorily. The derivation cohort was randomly split into two datasets: a training set (70%) and an internal validation set (30%). After training and validation, we successfully constructed a logistic regression model (LR model).
2.6 Statistical analysis
The completed examination records were analyzed by the SPSS Statistics 22.0 program (IBM SPSS, Chicago, IL, United States of America), Python (version 3.9.7), and R (version 4.1.3). The presence of MIH was considered a dependent variable. Dental caries and dental fluorosis were considered independent variables. Confidence intervals of 95% were calculated for prevalence. Chi-squared tests and Fisher’s exact tests were used for comparisons and correlations. Logistic regression was conducted to analyze factors that could affect MIH. Significance was set at a p-value of < 0.05.
3 Results
3.1 Distribution characteristics of MIH in the population
A total of 1,586 children were invited to participate. Of these, 18 were not in accordance with the inclusion criteria. The clinical characteristics and demographics of the study population are shown in Table 1. The dataset consisted of 1,568 samples with two types: MIH (215, 13.7%) and non-MIH. This record included 755 male and 813 female. Patients with MIH consisted of a greater proportion of males—;109 (14.4%) were male and 106 (13.0%) were female, —but the difference was not statistically significant. For permanent teeth, caries activity in Lankao City was low, at 5.6% in only permanent teeth and 14.2% in permanent and deciduous teeth. As expected, the percentage of dental fluorosis was as high as 54.8% (Table 1). There was a higher percentage of children with poor oral hygiene in this region (91.5%).
A supervised machine learning algorithm (e.g., logistic regression) was used to check for the occurrence of MIH. A total of 1,568 samples were analyzed, and six variables were included. During data preprocessing, the diagnosis of non-MIH or MIH was encoded using a binary encoder as 0 and 1, respectively. The ROC curve analysis was significant for this model, displaying an area under the curve of 0.72 (Figure 2). This model showed an accuracy of 70% and a specificity of 72%.
3.2 Distribution of MIH severity
The typical clinical phenotype of MIH is shown in Figure 3, including mild and severe types. With respect to the distribution of MIH severity among affected index teeth, demarcated opacities comprised the predominant type of defects (Table 2).
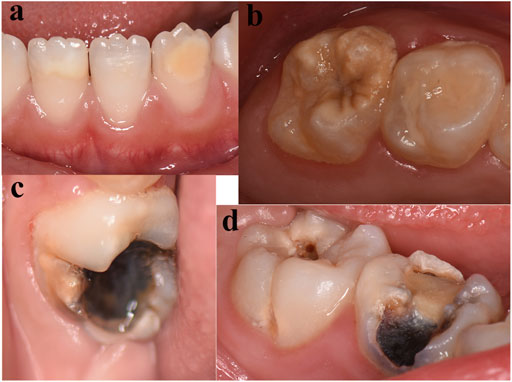
FIGURE 3. Phenotype of MIH. The majority of hypomineralized permanent teeth exhibited white (A) and yellow (B) demarcated lesions. (C) Atypical carious lesions associated with demarcated opacities in the first permanent molar. (D) Typical caries in the first permanent molars and atypical restorations in the primary molars.
3.3 Distribution of caries
The examined population presented a mixed dentition, and data from primary and permanent dentitions were presented separately (18,714 deciduous and 18,918 permanent teeth) (Table 3).
3.4 Nomogram development and validation
Based on a supervised machine learning algorithm, we constructed nomograms for predicting the occurrence of MIH, as shown in Figure 4. Non-DF had a great influence on the early occurrence of MIH. Significantly, the more severe the DF, the lower the occurrence.
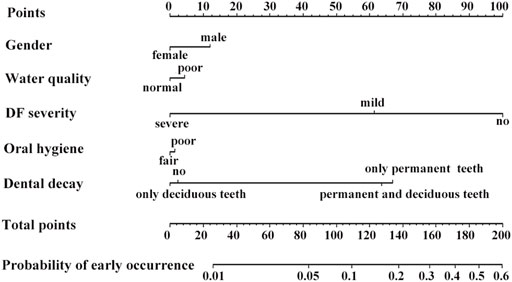
FIGURE 4. Nomograms to predict the probability of MIH occurrence. Non-DF had a significant influence on MIH occurrence, which decreased with increasing DF severity. Dental decay had a certain effect on it. However, exposure to low-quality shallow underground water did not increase the likelihood of developing MIH.
Dental decay of permanent teeth was related to the occurrence of MIH. However, exposure to poor-quality shallow underground water did not increase the likelihood of developing MIH. Gender and oral hygiene had no influence on the occurrence.
3.5 Factors associated with MIH
A correlation heatmap of all parameters was generated using Spearman correlation coefficients. Figure 5 illustrates the correlation heatmaps of the core data features. MIH and MIH severity were negatively correlated with DF and DF severity. As in the logistic regression analysis, DF had a protective correlation with MIH, and the protective effect became stronger as DF severity increased. However, dental caries were positively correlated with MIH (OR = 1.843; 95% CI: 1.260–2.694) (Table 4), which was inconsistent with the heatmap. The heatmap showed a negligible correlation between MIH and dental caries.
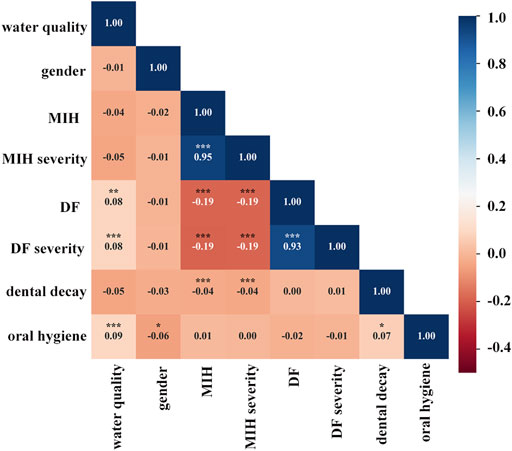
FIGURE 5. Correlation heatmap of clinical characteristics using Spearman correlations. Heatmap shows the positive (red) or negative (blue) correlations of all parameters, with color intensity reflecting the strength of the correlation (−0.4 to +1). MIH and MIH severity were negatively correlated with DF and DF severity and also had negligible associations with dental caries. *p < 0.05; **p < 0.01; ***p < 0.001.
Both the correlation heatmap and logistic regression analysis showed that the quality of underground water was not robustly correlated with MIH or MIH severity. Not surprisingly, the quality of underground water was correlated with DF and DF severity. A similar correlation was found between dental decay and oral hygiene.
To find the relationship between MIH and DF, we used a pair plot. In Python, the pair plot showed that MIH (Figure 6A) was strongly negatively correlated with DF and DF severity. The severity of MIH was reduced, followed by the incidence of DF and the strength of DF severity (Figure 6B).
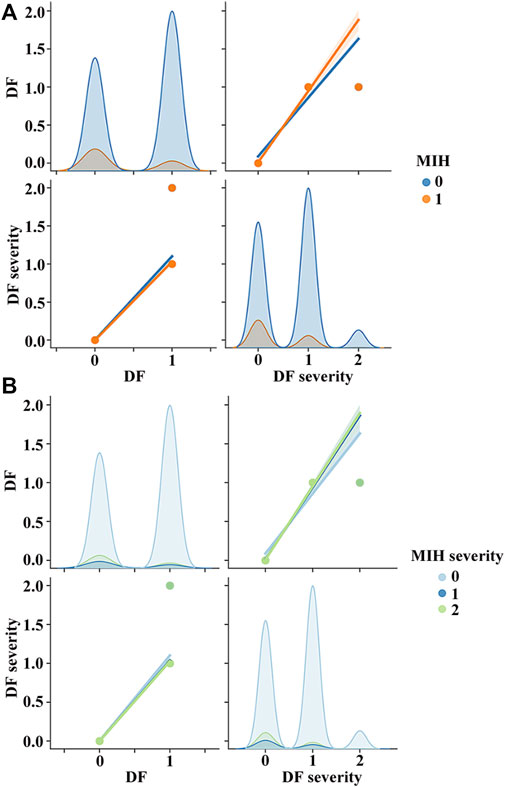
FIGURE 6. Pair plot was used to show relations among MIH, MIH severity, DF, and DF severity. During data preprocessing, the diagnoses of MIH or non-MIH, as well as DF or non-DF, were encoded using a binary encoder as 0 and 1. Thus, MIH or DF became 1, and non-MIH or non-DF became 0. Depending on the severity of MIH or DF, the level of MIH or DF was classed as normal (0), mild (1), or severe (2). (A) MIH was strongly negatively correlated with DF and DF severity. (B) Severity of MIH was reduced, followed by the incidence of DF and the strength of DF severity.
4 Discussion
In this study, we used data from 1,568 children to develop machine learning-based risk scores to predict the risk for MIH in an endemic fluorosis region in central China. Lankao is a typical quality-induced water shortage area, where there are various underground waters of poor quality, such as saltwater, brackish water, and high-hardness water. However, some regions have normal-quality groundwater that can be drunk directly. All participants were born and raised locally, and the natural groundwater source was the only drinking water before 2015. We have certitude that local shallow groundwater affected the permanent tooth mineralization of the recruited children.
MIH prevalence values reported by different studies are heterogeneous, varying from 2.4% to 44% in different areas (Dietrich et al., 2003; Calderara et al., 2005; Jasulaityte et al., 2007; Preusser et al., 2007; Ng et al., 2015) and from 2.8% to 25.5% in China (Cho et al., 2008; Sui et al., 2017; Li and Li, 2012; Zhang et al., 2020, Zhang et al.). The prevalence in this study was 13.7%, similar to the estimated world average of 12.9% (Schwendicke et al., 2018). Although there was a higher proportion of female patients with MIH, which is in agreement with a previous study (Mejare et al., 2005), the difference was not statistically significant. Spearman correlation analysis also indicated that sex does not seem to be a determining factor.
We qualified MIH as mild or severe to evaluate its severity, according to the criteria described by Mathu-Muju and Wright (2006). MIH is most often encountered in a mild form (Garcia-Margarit et al., 2014; Mittal and Sharma, 2015), which is consistent with the results of this study, in which 59.1% of the MIH cases were mild and 40.9% were severe at the tooth level (Table 2).
Recent developments in MIH research have focused on prevalence, and there are few prediction studies of early occurrence. In this study, a supervised machine learning model was constructed by incorporating a variety of factors that impact the occurrence of MIH in endemic fluorosis regions. The nomograms showed that sex had no influence on occurrence, which is consistent with previous studies (Yi et al., 2021; Sosa-Soto et al., 2022). Exposure to low-quality shallow underground water did not increase the likelihood of developing MIH. Significantly, non-DF had a substantial influence on the early occurrence of MIH, and the more severe the DF, the lower the occurrence.
A definitive conclusion has not been reached regarding the association between MIH prevalence and dental fluorosis. Thus, we explored the relationship between MIH and DF in children living in this endemic fluorosis region. As expected, the prevalence of fluorosis was as high as 54.8%. Spearman correlation analysis showed that MIH was negatively correlated with DF and DF severity; logistic regression analysis showed that DF had a protective correlation with MIH and that the effect of protection became more obvious with increasing severity. This finding accords with previous studies, showing that the prevalence of MIH was lower in the fluoridated area (10.8%) than in all non-fluoridated areas combined (17.35%) (Balmer et al., 2012). At the surface level, MIH frequency was lower in the presence of DF (Restrepo et al., 2022). Poisson regression analysis in a previous study showed that the p-value of the association between dental fluorosis and MIH was 0.084 and the OR ratio 0.63 (95% CI: 0.37–1.06) (Fernandes et al., 2021), which is similar to our findings. Enamel in patients diagnosed with MIH and fluorosis may not have completed maturation during amelogenesis (Malmberg et al., 2019; Fernandes et al., 2021). One possible explanation is that the affected teeth that initially erupt are hypomineralized; however, relatively long-term exposure to optimum levels of fluoride encourages remineralization. Finally, this continued remineralization could reduce or even change the defective clinical appearance.
According to the Fourth National Oral Health Survey in 2005, the caries prevalence in permanent teeth in China was 38.5% for the 12-year age group (Chen et al., 2018; Quan et al., 2018). The caries activity in Lankao City was low (14.2% of all teeth and 5.6% of only permanent teeth), which indicated that the high fluoride concentration of local water endowments truly prevented dental caries. In the nomograms, children with defective enamel were more prone to experiencing caries; logistic regression analysis showed that dental caries were positively correlated with MIH (OR = 1.843; 95% CI: 1.260–2.694). Most studies have shown a relationship between increased dental caries and children with MIH compared to those without MIH (Heitmüller et al., 2013; Petrou et al., 2015; Kosma et al., 2016). Furthermore, severe MIH cases had significantly higher caries prevalence than those with mild MIH (91.7% and 68.7%, respectively, p < 0.01; data not shown), suggesting that severe MIH increases the likelihood of caries in hypomineralized teeth. Teeth affected by MIH present a porous enamel surface due to poor mineral quality, which increases the likelihood of developing dental caries.
Unexpectedly, the prevalence of poor oral hygiene reached 91.5% in this region (Table 1), which increased the incidence of caries and worsened the severity of lesions. The nomogram showed that oral hygiene status did not affect the occurrence of MIH, and Spearman correlation analysis also found no relationship between oral hygiene status and MIH or MIH severity.
Altogether, the present study constructed a supervised machine learning algorithm to predict the occurrence of MIH in an endemic fluorosis region in central China, and the nomograph showed that MIH occurrence decreased with increasing DF severity. Then, we examined the association between MIH and DF and found a negative relationship, suggesting that DF should be considered a protective factor within the multifactorial etiology of MIH Zang et al., 2019.
Data availability statement
The original contributions presented in the study are included in the article/supplementary material; further inquiries can be directed to the corresponding author.
Ethics statement
The studies involving human participants were reviewed and approved by the Medical Ethics Committee of The First Affiliated Hospital of Henan University (2019LCSY-002). Written informed consent to participate in this study was provided by each participant’s legal guardian or next of kin. Written informed consent was obtained from the individual(s) and minor(s) and their legal guardians or next of kin for the publication of any potentially identifiable images or data included in this article.
Author contributions
YZ, YuW, and JJ designed the research and wrote the manuscript. YZ, YuW, ZZ, and YqW conducted the epidemiological studies. All authors reviewed the results and approved the final version of the manuscript.
Funding
This study was supported by the scientific research project of the Public Health Department of Henan (SBGJ202102197) and the scientific research project of the Education Department of Henan (21A320005).
Acknowledgments
The authors thank Professor Yanfang Ren (Eastman Institute for Oral Health, University of Rochester Medical Center) for guidance in this epidemiological study.
Conflict of interest
The authors declare that the research was conducted in the absence of any commercial or financial relationships that could be construed as a potential conflict of interest.
Publisher’s note
All claims expressed in this article are solely those of the authors and do not necessarily represent those of their affiliated organizations, or those of the publisher, the editors, and the reviewers. Any product that may be evaluated in this article, or claim that may be made by its manufacturer, is not guaranteed or endorsed by the publisher.
References
Balmer, R., Toumba, J., Godson, J., and Duggal, M. (2012). The prevalence of molar incisor hypomineralisation in Northern England and its relationship to socioeconomic status and water fluoridation. Int. J. Paediatr. Dent. 22, 250–257.
Calderara, P. C., Gerthoux, P. M., Mocarelli, P., Lukinmaa, P. L., Tramacere, P. L., and Alaluusua, S. (2005). The prevalence of Molar Incisor Hypomineralisation (MIH) in a group of Italian school children. Eur. J. Paediatr. Dent. 6, 79–83.
Chen, X., Ye, W., Zhan, J. Y., Wang, X., Tai, B. J., Hu, Y., et al. (2018). Periodontal status of Chinese adolescents: Findings from the 4th national oral health survey. Chin. J. Dent. Res. 21, 195–203.
Cho, S. Y., Ki, Y., and Chu, V. (2008). Molar incisor hypomineralization in Hong Kong Chinese children. Int. J. Paediatr. Dent. 18, 348–352.
Da Costa-Silva, C. M., Jeremias, F., De Souza, J. F., Cordeiro Rde, C., Santos-Pinto, L., and Zuanon, A. C. (2010). Molar incisor hypomineralization: Prevalence, severity and clinical consequences in Brazilian children. Int. J. Paediatr. Dent. 20, 426–434.
Dietrich, G., Sperling, S., and Hetzer, G. (2003). Molar incisor hypomineralisation in a group of children and adolescents living in Dresden (Germany). Eur. J. Paediatr. Dent. 4, 133–137.
Duarte, M. B. S., Carvalho, V. R., Hilgert, L. A., Ribeiro, A. P. D., Leal, S. C., and Takeshita, E. M. (2021). Is there an association between dental caries, fluorosis, and molar-incisor hypomineralization? J. Appl. Oral Sci. 29, e20200890.
Fernandes, I. C., Forte, F. D. S., and Sampaio, F. C. (2021). Molar-incisor hypomineralization (MIH), dental fluorosis, and caries in rural areas with different fluoride levels in the drinking water. Int. J. Paediatr. Dent. 31, 475–482.
Garcia-Margarit, M., Catalá-Pizarro, M., Montiel-Company, J. M., and Almerich-Silla, J. M. (2014). Epidemiologic study of molar-incisor hypomineralization in 8-year-old Spanish children. Int. J. Paediatr. Dent. 24, 14–22.
Ghanim, A., Silva, M. J., Elfrink, M. E. C., Lygidakis, N. A., Marino, R. J., Weerheijm, K. L., et al. (2017). Molar incisor hypomineralisation (MIH) training manual for clinical field surveys and practice. Eur. Arch. Paediatr. Dent. 18, 225–242.
Greene, J. C., and Vermillion, J. R. (1964). The simplified oral hygiene index. J. Am. Dent. Assoc. 68, 7–13.
HeitmüLLER, D., Thiering, E., Hoffmann, U., Heinrich, J., Manton, D., KüHNISCH, J., et al. (2013). Is there a positive relationship between molar incisor hypomineralisations and the presence of dental caries? Int. J. Paediatr. Dent. 23, 116–124.
Jasulaityte, L., Veerkamp, J. S., and Weerheijm, K. L. (2007). Molar incisor hypomineralization: Review and prevalence data from the study of primary school children in kaunas/Lithuania. Eur. Arch. Paediatr. Dent. 8, 87–94.
Jeremias, F., Koruyucu, M., Kuchler, E. C., Bayram, M., Tuna, E. B., Deeley, K., et al. (2013). Genes expressed in dental enamel development are associated with molar-incisor hypomineralization. Arch. Oral Biol. 58, 1434–1442.
Kosma, I., Kevrekidou, A., Boka, V., Arapostathis, K., and Kotsanos, N. (2016). Molar incisor hypomineralisation (MIH): Correlation with dental caries and dental fear. Eur. Arch. Paediatr. Dent. 17, 123–129.
Li, L., and Li, J. (2012). Investigation of molar-incisor hypomineralization among children from 6 to 11 years in Lucheng district, Wenzhou city. Shanghai Kou Qiang Yi Xue 21, 576–579.
Malmberg, P., NoréN, J. G., and Bernin, D. (2019). Molecular insights into hypomineralized enamel. Eur. J. Oral Sci. 127, 340–346.
Mathu-Muju, K., and Wright, J. T. (2006). Diagnosis and treatment of molar incisor hypomineralization. Compend Contin. Educ. Dent. 27, 604–610.
Mejare, I., Bergman, E., and Grindefjord, M. (2005). Hypomineralized molars and incisors of unknown origin: Treatment outcome at age 18 years. Int. J. Paediatr. Dent. 15, 20–28.
Mittal, N., and Sharma, B. B. (2015). Hypomineralised second primary molars: Prevalence, defect characteristics and possible association with molar incisor hypomineralisation in Indian children. Eur. Arch. Paediatr. Dent. 16, 441–447.
Naing, L., Winn, T., and Rusli, B. N. (2006). Practical issues in calculating the sample size for prevalence studies. Archives Orofac. Sci. 1.
Ng, J. J., Eu, O. C., Nair, R., and Hong, C. H. (2015). Prevalence of molar incisor hypomineralization (MIH) in Singaporean children. Int. J. Paediatr. Dent. 25, 73–78.
Petrou, M. A., Giraki, M., Bissar, A. R., Wempe, C., SchäFER, M., Schiffner, U., et al. (2015). Severity of MIH findings at tooth surface level among German school children. Eur. Arch. Paediatr. Dent. 16, 271–276.
Preusser, S. E., Ferring, V., Wleklinski, C., and Wetzel, W. E. (2007). Prevalence and severity of molar incisor hypomineralization in a region of Germany -- a brief communication. J. Public Health Dent. 67, 148–150.
Quan, J. K., Wang, X. Z., Sun, X. Y., Yuan, C., Liu, X. N., Wang, X., et al. (2018). Permanent teeth caries status of 12- to 15-year-olds in China: Findings from the 4th national oral health survey. Chin. J. Dent. Res. 21, 181–193.
Restrepo, M., Rojas-GualdróN, D. F., De Farias, A. L., Girotto-Bussaneli, D., and Santos-Pinto, L. (2022). Association between frequency and severity of dental fluorosis and molar incisor hypomineralization. J. Clin. Pediatr. Dent. 46, 30–34.
Sabieha, A. M., and Rock, W. P. (1998). A comparison of clinical and photographic scoring using the TF and modified DDE indices. Community Dent. Health 15, 82–87.
Schmalfuss, A., Stenhagen, K. R., Tveit, A. B., Crossner, C. G., and Espelid, I. (2016). Canines are affected in 16-year-olds with molar-incisor hypomineralisation (MIH): An epidemiological study based on the tromsø study: "Fit futures. Eur. Arch. Paediatr. Dent. 17, 107–113.
Schwalbe, N., and Wahl, B. (2020). Artificial intelligence and the future of global health. Lancet 395, 1579–1586.
Schwendicke, F., Elhennawy, K., Reda, S., Bekes, K., Manton, D. J., and Krois, J. (2018). Global burden of molar incisor hypomineralization. J. Dent. 68, 10–18.
Sosa-Soto, J., PadróN-Covarrubias, A. I., MáRQUEZ-Preciado, R., Ruiz-RodríGUEZ, S., Pozos-GuilléN, A., Pedroza-Uribe, I. M., et al. (2022). Molar incisor hypomineralization (MIH): Prevalence and degree of severity in a Mexican pediatric population living in an endemic fluorosis area. J. Public Health Dent. 82, 3–10.
Sui, W., Wu, H. Y., Yuan, J., L., Yao, H., and Hong, Y., L. (2017). Epidemiological study of molar incisor hypomineralization in primary school students in Soozhow China. Chin. J. Conservative Dent. 27, 103–107.
Thylstrup, A., and Fejerskov, O. (1978). Clinical appearance of dental fluorosis in permanent teeth in relation to histologic changes. Community Dent. Oral Epidemiol. 6, 315–328.
Wang, C., and Cao, P. (2013). Distribution characteristics and causes of main components exceeding standard of primary inferior groundwater in Lankao County. Resour. Guide·Earth Sci. Technol. 46-47, 50.
Wang, J., S., Feng, L., Huang, J., and Yang, L., and (2008). Groundwater quality analysis and protection countermeasures in Lankao county of henan province. Ground Water 30, 105–107.
Weerheijm, K. L., Duggal, M., MejàRE, I., Papagiannoulis, L., Koch, G., Martens, L. C., et al. (2003). Judgement criteria for molar incisor hypomineralisation (MIH) in epidemiologic studies: A summary of the European meeting on MIH held in athens, 2003. Eur. J. Paediatr. Dent. 4, 110–113.
Wong, H. M., Mcgrath, C., Lo, E. C., and King, N. M. (2006). Association between developmental defects of enamel and different concentrations of fluoride in the public water supply. Caries Res. 40, 481–486.
Yi, X., Chen, W., Liu, M., Zhang, H., Hou, W., and Wang, Y. (2021). Prevalence of MIH in children aged 12 to 15 years in Beijing, China. Clin. Oral Investig. 25, 355–361.
Zang, P.,N., Gao, Y., Y., Tian, J., and Huang, R., Z. (2019). Epidemiological sampling survey of MIH in children in Shanxi Province. 19th Natl. Annu. Meet. Oral Prev. Med. Prof. Comm. Chin. Stomatological Assoc.
Keywords: molar incisor hypomineralization (MIH), prevalence, dental fluorosis (DF), dental caries, machine learning (ML)
Citation: Zhang Y, Wang Y, Zhang Z, Wang Y and Jia J (2023) Study on machine learning of molar incisor hypomineralization in an endemic fluorosis region in central China. Front. Physiol. 14:1088703. doi: 10.3389/fphys.2023.1088703
Received: 03 November 2022; Accepted: 22 February 2023;
Published: 15 March 2023.
Edited by:
Rodrigo S. Lacruz, New York University, United StatesReviewed by:
Thomas G. H. Diekwisch, University of Rochester Medical Center, United StatesCarmen Llena Puy, University of Valencia, Spain
Copyright © 2023 Zhang, Wang, Zhang, Wang and Jia. This is an open-access article distributed under the terms of the Creative Commons Attribution License (CC BY). The use, distribution or reproduction in other forums is permitted, provided the original author(s) and the copyright owner(s) are credited and that the original publication in this journal is cited, in accordance with accepted academic practice. No use, distribution or reproduction is permitted which does not comply with these terms.
*Correspondence: Jie Jia, aGRqaWFqaWVAZm94bWFpbC5jb20=
†These authors have contributed equally to this work and share first authorship